- 1The First Laboratory of Cancer Institute, The First Hospital of China Medical University, Shenyang, China
- 2Department of Medical Oncology, Cancer Hospital of China Medical University, Liaoning Cancer Hospital & Institute, Shenyang, China
- 3Department of Oncology, Shanghai East Hospital, Tongji University School of Medicine, Shanghai, China
- 4Department of Gynecology, First Hospital of China Medical University, Shenyang, China
- 5The First Department of Oncology, Shenyang Fifth People’s Hospital, Shenyang, China
Epithelial ovarian cancer (EOC) has a poor prognosis and high mortality rate; patients are easy to relapse with standard therapies. So, there is an urgent need to develop novel drugs. In this study, differentially expressed genes (DEGs) of EOC were identified in The Cancer Genome Atlas (TCGA) and Gene Expression Omnibus (GEO) databases. Enrichment and protein–protein interaction (PPI) analyses were performed. The drug candidate which has the possibility to treat EOC was predicted by Connectivity Map (CMAP) databases. Moreover, molecular docking was selected to calculate the binding affinity between drug candidate and hub genes. The cytotoxicity of drug candidates was assessed by MTT and colony formation analysis, the proteins coded by hub genes were detected by Western blots, and apoptosis analysis was evaluated by flow cytometry. Finally, 296 overlapping DEGs (|log 2 fold change|>1; q-value <0.05), which were principally involved in the cell cycle (p < 0.05), and cyclin-dependent kinase 1 (CDK1) were screened as the significant hub gene from the PPI network. Furthermore, the 21 drugs were extracted from CMAPs; among them, piperlongumine (PL) showed a lower CMAP score (-0.80, -62.92) and was regarded as the drug candidate. Furthermore, molecular docking results between PL and CDK1 with a docking score of –8.121 kcal/mol were close to the known CDK1 inhibitor (–8.24 kcal/mol). Additionally, in vitro experiments showed that PL inhibited proliferation and induced apoptosis via targeting CDK1 in EOC SKOV3 cells. Our results reveal that PL may be a novel drug candidate for EOC by inhibiting cell cycle.
Introduction
Epithelial ovarian cancer (EOC) is an aggressive malignancy and is most frequently diagnosed at an advanced disease stage (1). Currently, the most common treatment is surgery combined with platinum-based combination chemotherapy (2). However, 60% of patients relapsed after first-line therapy (3), and 50% showed resistance to chemotherapy. It is generally believed that EOC chemotherapy resistance is involved in the DNA damage response (DDR) process of the cell cycle (4, 5), in particular single-strand DNA break repair by poly ADP-ribose polymerase (PARP) and double-stranded repair through homologous recombination repair (HRR) of the BRCA1/2 genes. Therefore, it is the key to discovering drugs that affect the mechanism of drug resistance.
Currently, for the treatment of ovarian cancer, agents that target certain stages of the cell cycle, such as cyclin-dependent kinase inhibitors (CDKIs), have shown good efficacy in clinical trials. For example, ribociclib (6), a CDK4/6 inhibitor, which acts on the G1 phase of the cell cycle has been approved by the US Food and Drug Administration (FDA) for the treatment of breast cancer; it has also been used to treat ovarian cancer in phase II clinical trials (NCT02657928). Furthermore, AZD5438 (7, 8), a CDK1/2 inhibitor, enhances radiosensitivity of non-small cell lung cancer by impairing HRR of double-stranded breaks (DSBs) and has already been tested in the preclinical stage. Thus, it has been suggested that targeting the cell cycle is a novel and effective method to treat tumors. However, only a few CDKIs have so far been developed to treat ovarian cancer; thus, more chemotherapeutic agents, which are more efficacious and safe, need to be developed for treating ovarian cancer.
High costs necessitating strong financial support, long timelines, and requirement of substantial resources make development of a novel drug a difficult venture. Drug repurposing is an unconventional approach to identify novel indications of an approved or experimental drug (9). There are several successful examples. For instance, thalidomide was originally developed as an antiemetic in pregnancy but has currently garnered a huge market for the management of multiple myelomas (10). Metformin, widely used for first-line therapy of type 2 diabetes, has been found to possess an additional anticancer property (11). Therefore, repurposing of known drugs is a feasible drug development strategy.
In the present study, by focusing directly on EOC datasets, we aimed to develop a new drug to treat EOC by integrated bioinformatics and in vitro experiments. It was principally based on the Connectivity Map (CMAP) databases, which connect genes, drugs, and diseases by numerous cell line experiments. Then, molecular docking was used to matching potential drugs and screened proteins. Furthermore, in vitro experiments were used to validate our prediction. To a certain extent, our study may provide a basis for the treatment of EOC.
Materials and Methods
Data Source and DEG Acquisition
Epithelial ovarian cancer-related mRNA data of cancer tissues and normal tissues were integrated from RNA-seq data and microarray expression datasets. First, the RNA-seq data were separately collected from The Cancer Genome Atlas (TCGA) database (http://cancergenome.nih.gov/) and the Genotype-Tissue Expression (GTEx) project (https://gtexportal.org/home/); the edition of the TCGA dataset on EOC was updated on July 20, 2019. The microarray expression datasets were obtained from the GEO database (https://www.ncbi.nlm.nih.gov/geo/), and the two gene expression profiles (GSE14407 and GSE54388) were both selected with the GPL590 platform. GSE14407 and GSE54388 were updated on March 25, 2019. Then, differential analyses of the two ways datasets were used by the R package Limma (https://bioconductor.org/packages/release/bioc/html/limma.html) (12) to determine differentially expressed genes (DEGs) with the criteria of |log2(FC)| > 1 and adjusted p-value < 0.05. Furthermore, the differential analysis of RNA-seq data were log2(TPM+1) transformed and analyzed by the Gene Expression Profiling Interactive Analysis (GEPIA) (http://gepia.cancer-pku.cn/) (13).
Functional Enrichment Analyses
The potential mechanisms of the genes selected were studied, which were imported from the online bioinformatics database Metascape (http://metascape.org/) (14), including gene ontology (GO) Biological Processes and Kyoto Encyclopedia of Genes and Genomes (KEGG) pathway enrichment analyses as well as Protein Protein Interaction (PPI) establishment. In this study, significant terms met the criteria of a p value < 0.05 and the number of enriched genes ≥ 3.
PPI Network Module Analyses and Identification of Hub Genes
To visualize and analyze the PPI network, we used Cytoscape (version 3.7.0) software (http://www.cytoscape.org/) (15). First, molecular modules were analyzed by Molecular Complex Detection (MCODE) (16) plugin of Cytoscape. The parameter settings were set to default. The criteria were set as follows: MCODE scores > 3 and number of nodes > 4. Next, hub genes were screened from the PPI network using CytoHubba (17) plugin of Cytoscape with the recommended maximal clique centrality (MCC) ranking methods. The top 10 genes were noted as hub genes.
EOC-Associated Drug Prediction and Gene Set Enrichment Analysis (GSEA)
The connectivity map, which aims to connect the genes, drugs, and disease states by querying the gene lists of upregulated and downregulated genes, was employed. A so-called connectivity score was estimated to assess the priority of the prediction; a positive score denotes a stimulant effect of a drug on the query signatures, whereas a negative score implicates a repressed effect of a drug on the query signatures. This was based on different data and algorithms. CMAP (18), the first-generation connectivity map platform, using a microarray platform (Affymatrix HT_HG_U133A with 22 283 probe sets), screened 1,309 FDA drugs treated in five cell lines, and the connectivity score was from –1 through 1. Then, LINCS (19), the next-generation connectivity map, including 476,251 genome-wide expression signature expression profiles gathered 27,927 perturbagens stimulated by 72 cell lines from 1.3M L1000 profiles. The connectivity score was from –100 through 100. In addition, to investigate the pathways affected by small molecule drugs, the raw data were selected from the CMAP database and analyzed using the function of GSEA from clusterProfiler package (20, 21) and the criteria of a p-value < 0.05, FDR <0.25.
Molecular Docking Between Drug Candidate and Hub Gene of EOC
The crystal structures of proteins coded by the hub gene were retrieved from the RCSB Protein Data Bank (PDB) (www.rcsb.org/pdb/home/home.do). Moreover, the three-dimensional structure of drugs was searched from PubChem (https://www.ncbi.nlm.nih.gov/pccompound). The molecular docking process involved preparing the proteins and ligands, setting up a grid, and docking the compounds; these were accomplished using the Schrodinger Glide docking protocol (Schrödinger, LLC, NY, USA) (22). The best pose was picked out by the docking score and the rationality of molecular conformation.
In Vitro Cell Lines and Chemicals
Human ovarian cancer cell lines SK-OV-3, CA-OV-3, and HO-8910 were obtained from the Cell Bank of Type Culture Collection of the Chinese Academy (Shanghai, China). SK-OV-3 was cultured in McCoy’s 5A Complete Medium (Thermo Fisher, Belgium). CA-OV-3 was cultured in DMEM medium, and HO-8910 was cultured in RPMI-1640 medium. All the cell lines were cultured in medium supplemented with 10% fetal bovine serum (Greiner Bio-One, Belgium) and antibiotics (penicillin/streptomycin, 100 U/ml, Beyotime, Beijing, China) at 37°C in 5% CO2.
PL was purchased from NeOnc Technologies, Inc. (Los Angeles, CA, USA) and diluted with DMSO to make stock solutions of 10 mM. In all cases of cell treatment, the final DMSO concentration in the culture medium never exceeded 0.5%. Stock solutions of all drugs were stored at −20°C.
Cell Viability Assay
The EOC cell lines were plated to 5 × 103 cells/well in 96-well plates for 24 h, then treated with the indicated concentrations of PL. Next, 50 μl of the MTT reagent (5 mg/ml) was added for 3 h, and then 150 μl of DMSO was admixed to dissolve the formazan crystals. Absorbance was measured at 570 nm using a spectrophotometer (Bio-Rad, Temse, Belgium). Cell viability was determined by dividing the absorbance values of treated cells with that of untreated cells.
Colony Formation Assay
Depending on the cell line, 200 cells were implanted in each well of a six-well plate and exposed to the indicated concentrations of PL for 24 h. Following this, drugs were withdrawn and cells were grown in normal culture for 14 days. Next, the cells were fixed with acetic acid-methanol (1:4) and stained with diluted crystal violet (1:30). Colonies that consisted of more than 50 cells were counted and calculated. The colony formation efficiency was calculated with the following formula: Survival Fraction = Colonies/Cell numbers × 100%. Three independent experiments were carried out.
Detection of Apoptotic Cells
Apoptosis was evaluated by using Annexin V-FITC Apoptosis Detection Kit (BD Biosciences Pharmingen, San Diego, CA, USA) according to the manufacturer’s instructions. EOC cell lines (2.5–4.5 × 105 cells/well) were seeded in a six-well plate and grown to 70% confluence. After being treated with various concentrations of PL for 24 h and 48 h, the cells were trypsinized, collected, and washed twice with phosphate-buffered saline (PBS) and stained with FITC-Annexin V and propidium iodide (PI) for 15 min in the dark. The stained cell populations were determined using a FACSCalibur flow cytometer (Becton Dickinson, Bedford, MA, USA), and the data were analyzed using FlowJo Software 7.6 (TreeStar, Inc., San Car-los, CA, USA). Three independent experiments were carried out.
Western Blots
Cells were extracted, and protein was quantified as described previously (23). The cells were washed twice with PBS, lysed in lysis buffer (1% Triton X-100, 50 mM Tris–HCl pH 7.4, 150 mM NaCl, 10 mM EDTA, 100 mM NaF, 1 mM Na3VO4, 1 mM PMSF, 2 µg/ml aprotinin), and quantified using a BCA protein quantification kit (cat. no. ab102536; Abcam). The cell lysates were separated by 8% or 15% SDS-PAGE, and the samples were transferred onto a nitrocellulose membrane (Immobilon-P, Millipore; Merck KGaA). After blocking with 5% evaporated skimmed milk in Tris-buffered saline Tween-20 (TBST) buffer (10 mM Tris–HCl pH 7.4, 150 mM NaCl, 0.1% Tween-20) at room temperature for 1 h, primary antibodies were probed and incubated overnight at 4°C. Following three washes with TBST buffer, the membrane was incubated with secondary goat anti-rabbit and goat anti-mouse antibodies for 30 min at room temperature. Finally, the protein bands were detected with enhanced chemiluminescence reagent (SuperSignal™ Western Pico Chemiluminescent Substrate; Pierce; Thermo Fisher Scientific, Inc.) and scanned using the Electrophoresis Gel Imaging Analysis System (DNR Bio Imaging Systems, Neve Yamin, Israel).
Statistical Analysis
Statistical significance was evaluated with data from at least three independent experiments. GraphPad Prism 7.00 (GraphPad Software, San Diego, CA, USA) was used for data analysis. Statistical analysis was carried out using the Student’s t-test for two groups, as well as one-way ANOVA for more than two groups. Data were presented as the mean ± SD. For all statistical tests, significance was established at p < 0.05. The number of asterisks in the figures indicates the level of statistical significance: *p < 0.05, **p < 0.01, ***p < 0.001, ****p < 0.0001.
Results
Identification of Overlapping DEGs for EOC
In this study, DEGs and their significant biological characteristics were identified based on various GEO mRNA microarray datasets (GSE14407 and GSE54388) and the TCGA mRNA-seq dataset by integrated bioinformatics analysis of EOC (Figure 1). There were a total of 560 samples, including 454 EOC tissues and 106 normal tissues (GSE54388: 16T/6N; GSE14407: 12T/12N; RNA-seq: 426T(TCGA)/88GTEx, respectively). After gene expression assays and data processing and normalizing, we screened DEGs among each mRNA data set using the Limma with the criteria of |log2(FC)| > 1 and an adjusted p-value < 0.05. Overall, a total of 1,188 DEGs were screened from the GSE54388 data set, including 518 upregulated and 670 downregulated genes (Figure 1A). There were 711 DEGs, including 255 upregulated and 456 downregulated genes in GSE14407 (Figure 1B). Additionally, 7,615 DEGs were selected from the TCGA data set, including 2,606 upregulated and 5,009 downregulated genes (Figure 1C). To confirm the reliability of DEGs in EOC, we obtained overlapping DEGs of the three datasets, including 115 common upregulated genes and 181 common downregulated genes (Figures 1D, E and Supplementary Table 1).
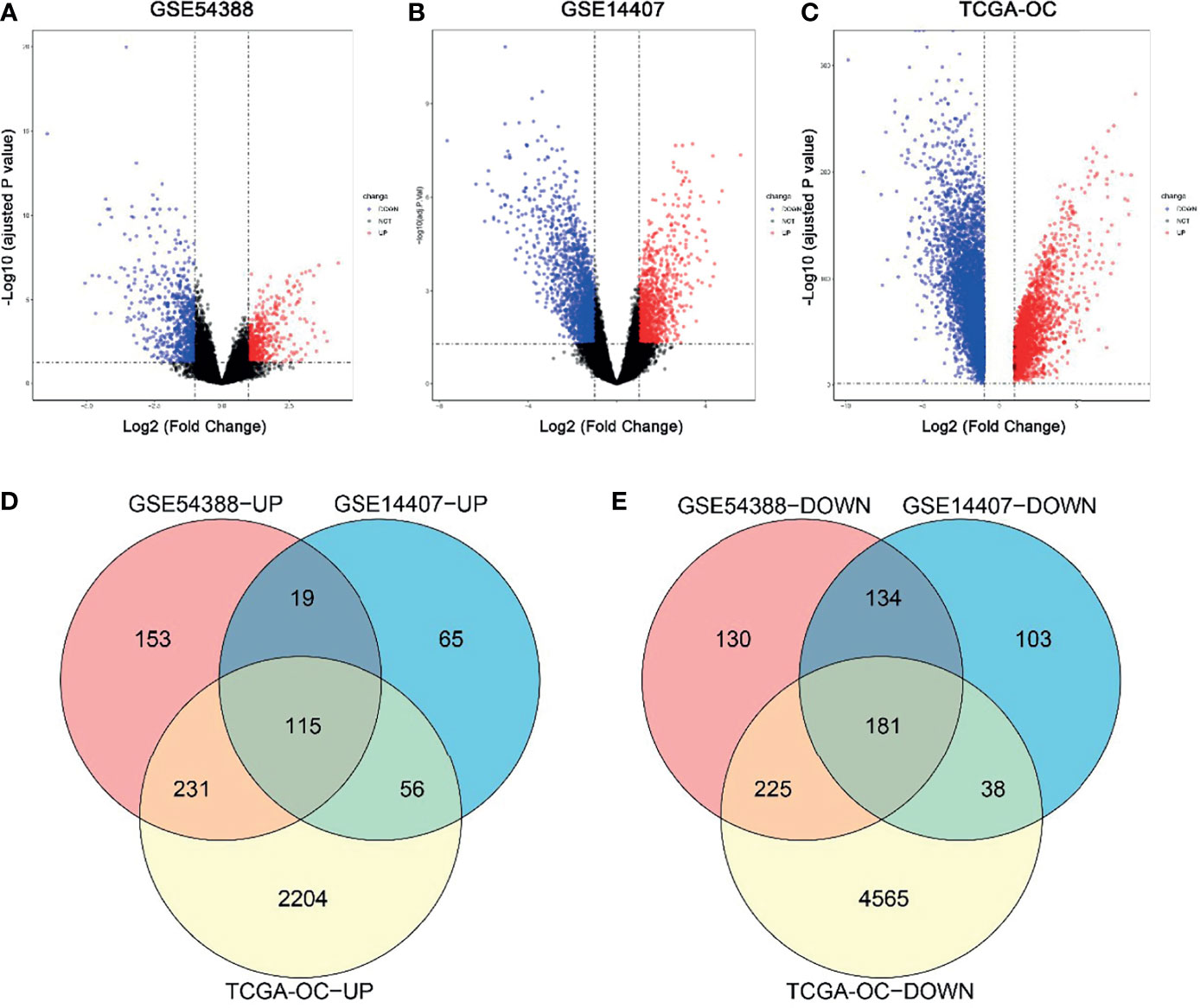
Figure 1 Identification of common differentially expressed genes (DEGs) in three independent datasets. (A–C) Volcano plot for the DEGs in GSE54388 (A), GSE14407 (B), and TCGA-OC (C) datasets when comparing epithelial ovarian cancer (EOC) to normal ovarian subjects. The x-axis represents log2 (fold change), and y-axis represents significant difference expressed as −log10 (adjusted p-value). DEGs were determined using the limma package. The gene with the adjusted p-value < 0.05,log2FC| ≥ 2 was considered significant. (D, E) A total of 115 common upregulated genes (D) and 181 common downregulated genes (E) were shared between these three independent datasets.
Functional Enrichment Analyses
We selected overlapping DEGs to investigate the enrichment of EOC in GO and KEGG pathways in Metascape. First, the KEGG pathways for DEGs were mainly cell cycle, oocyte meiosis, and p53 signaling pathway, which were found to be related to the development of multiple tumors and were involved in EOC tumorigenesis and pathogenesis (Figure 2A). For GO_BP enrichment analysis, they were enriched in cell cycle and apoptosis, such as cell division, mitotic nuclear division, and mitotic sister chromatid segregation (Figure 2B). For GO_MF analysis, they were enriched in DNA replication origine binding and microtubule binding (Figure 2C). For GO_CC analysis, they were enriched in spindle, chromosomal region, microtubule, and so on. These results indicated that DEGs might be related to the cell proliferation process (Figure 2D). In addition, the KEGG pathways of downregulated DEGs were enriched in tyrosine metabolism, drug metabolism-cytochrome P450, and retinol metabolism (Figure 2A).
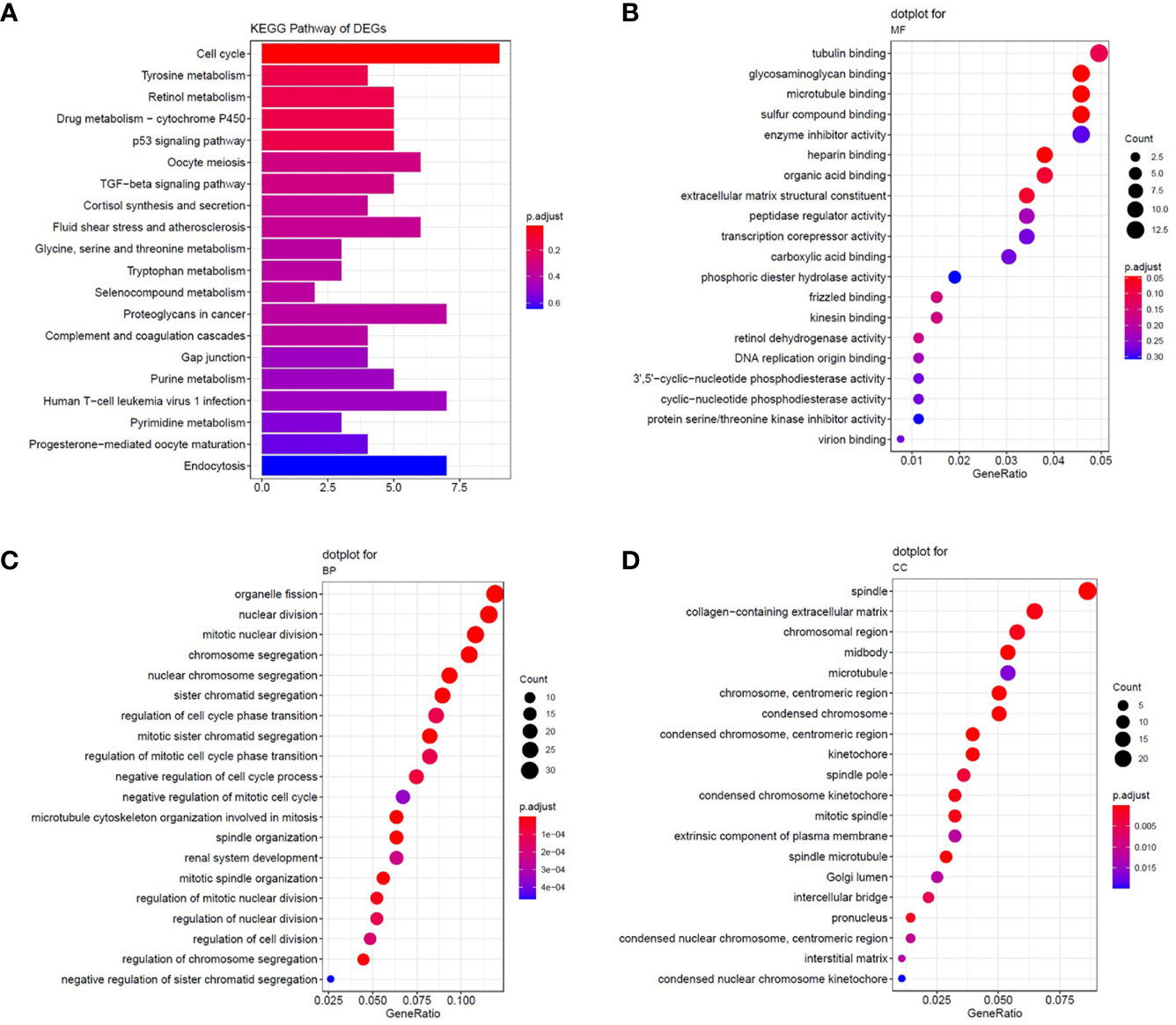
Figure 2 The enrichment analysis of common DEGs. (A) Significantly enriched Kyoto Encyclopedia of Genes and Genomes (KEGG) pathways. (B–D) The results of GO enrichment for DEGs. Enrichment analysis of the common DEGs was assessed by the Metascape database separately. P-value < 0.05 was considered statistically significant.
PPI Network Module Analyses and Identification of Hub Genes
Furthermore, we constructed the PPI network presented in Figures 3A, B, and the whole network was clustered in three modules by MCODE plugin of Cytoscape. Module 1 included all common upregulated genes and were enriched in cell division, chromosome segregation, and mitotic sister chromatid segregation, which were G2/M related. Module 2 consisted of common upregulated genes and one common downregulated gene, enriched in the regulation of mitotic cell cycle, cell cycle process, and mitotic cell cycle phase transition. Module 3 included all common downregulated genes but had no exact analysis results.
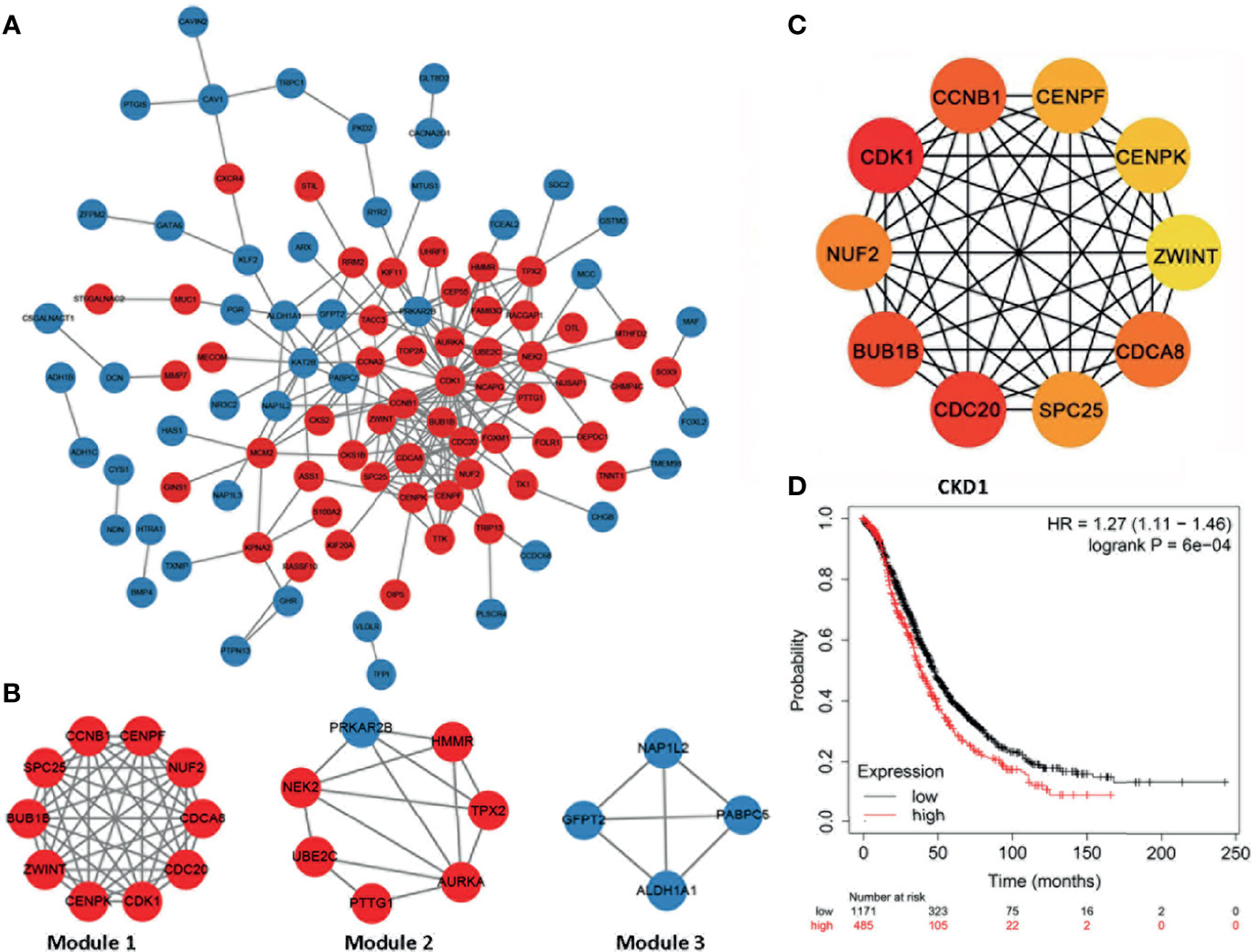
Figure 3 The protein–protein interaction network and hub genes. (A, B) EOC-related network; red indicates common upregulated genes and blue represents and common downregulated genes. The internal interactions between common DEGs were mined by the Metascape database, and the network was visualized using Cytoscape software. (C) Top 10 EOC-related hub genes. The network was analyzed by the cytoHubba plugin of Cytoscape software with the method of MCC. All the hub genes were upregulated in EOC tissues. (D) High CDK1 expression was correlated with poor prognosis of ovarian cancer patients (hazard ratio = 1.27, 95% CI: 1.11–1.46, p < 0.05).
Next, hub genes were selected among the overlapping DEGs by the CytoHubba plugin of the Cytoscape. The top 10 genes were screened as hub genes, including CDK1, CDC20, BUB1B, CCNB1, CDCA8, NUF2, SPC25, CENPF, CENPK, and ZWINT in descending order (Figure 3C). CDK1 received the maximum score among them, and it was selected as the significant hub gene. CDK1 expressed a significantly higher level in ovarian cancer tissues, compared with normal tissues (Supplementary Table 1). Further, a higher expression level of CDK1 was correlated with poor prognosis of ovarian cancer patients (Figure 3D).
EOC-Associated Drugs and GSEA
The overlapping DEGs generated for EOC were used to query CMAP and LINCS, respectively. By integrating the drugs from the two databases with score < 0, and p value < 0.05, we found that the 21 drugs were segregated into two clusters (Figure 4A); we selected the five drugs (piperlongumine, doxorubicin, vorinostat, methotrexate, and scriptaidin) in cluster 2, which had lower scores in both databases regarded as the potential drugs. Among them, four drugs (doxorubicin, vorinostat, methotrexate, and scriptaidin) have been used to treat EOC in clinical practice or clinical trials; PL received the lowest connectivity score, and there is litter evidence that it can treat EOC (23). Moreover, for piperlongumine, a total of 28 pathways were enriched (Figure 4B), including DNA replication, nucleotide excision repair, mismatch repair, and homologous recombination, which were closely related to the mechanism of EOC proliferation and drug resistance. Hence, we regarded PL as the candidate drug.
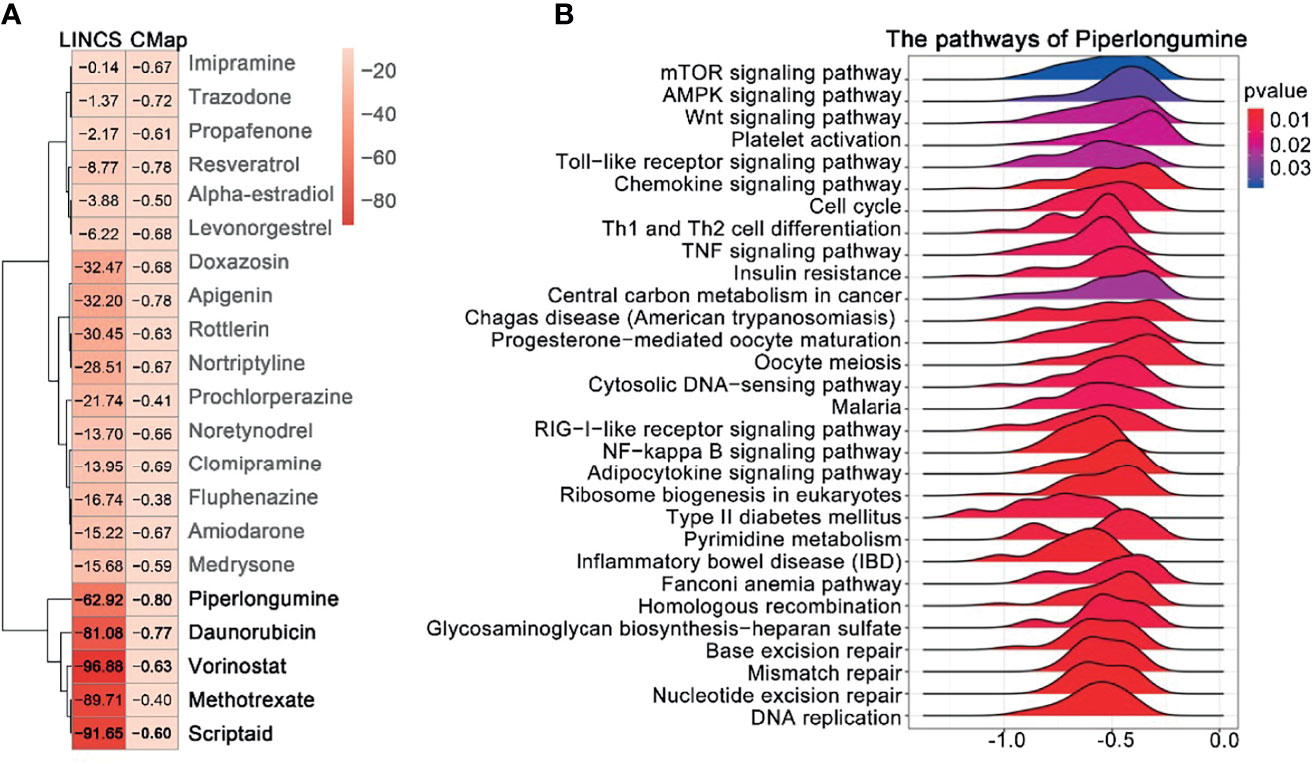
Figure 4 Prediction of drug candidates and drug GSEA analysis. (A) Similarity score table for the drugs having at least one significant association with connectivity map databases. Each row corresponds to a drug, and columns correspond to two-generation connectivity map databases. The score labels with numbers indicate the significance of the results. The row labels written in bold indicate the drugs we selected for further analysis. (B) GSEA analysis of the piperlongumine was assessed by the clusterProfiler package; p-value < 0.05 was considered statistically significant.
Interactions Between Drug Candidate and Hub Gene
To further predict whether PL could be a direct CDK1 inhibitor, we performed molecular docking using the Schrodinger Glide docking protocol. Surprisingly, we found that PL showed a good binding affinity for CDK1 protein with the docking glide score of –8.121 kcal/mol, which is close to that of the known CDK1 inhibitor, AZD5438 (–8.24 kcal/mol). Most of the drugs appeared to have an equivalent glide score range from –8.121 through –2.662 kcal/mol. As displayed in Figure 5, the top scoring ligands, such as PL, were observed to interact with three residues Leu-83, GLN-132, and GLN-49 through hydrogen bonding with their side chains. Taken together, our data indicated that PL can bind to a similar pocket on CDK1.
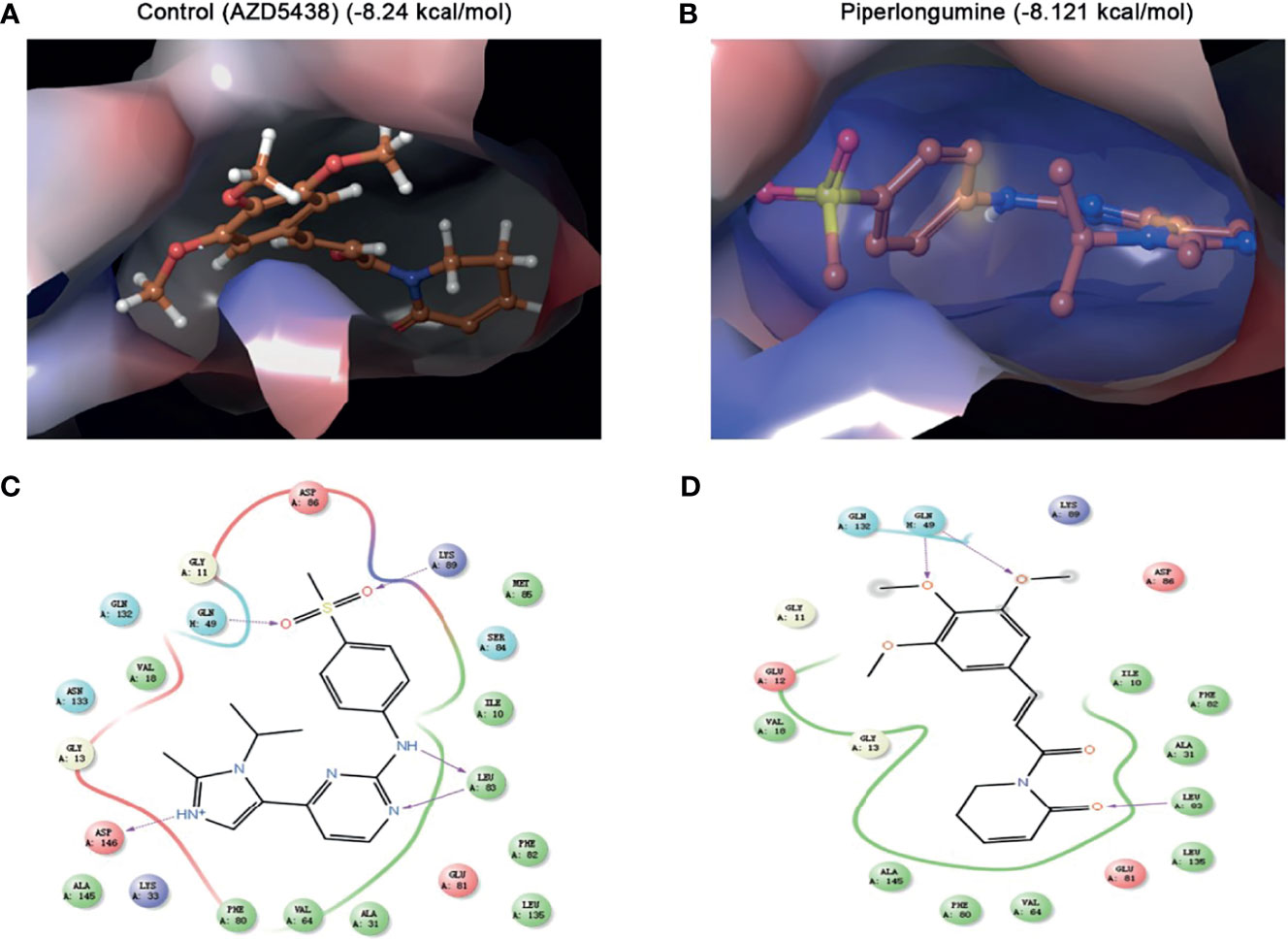
Figure 5 Molecular docking simulation for piperlongumine and CDK1. (A, B) Docked structure and interactions of drugs [(A) AZD5438 (control), (B) piperlongumine] binding to CDK1. (C, D) 2D interaction diagrams of the residues of CDK1 involved in the binding of drugs [(C) AZD5438 (control), (D) piperlongumine]. The Schrodinger Glide docking protocol was used for this analysis.
In Vitro Studies
SKOV3, CA-OV-3, and HO-8910 cell cultures were exposed to different concentrations of PL for 24 h or 48 h, and cell viability was determined by MTT assay. As shown in Figures 6A–C, PL decreased cell viability in a concentration- and time-dependent manner. The IC50 value of SK-OV-3 was 49.32 and 16.28 µM in 24 and 48 h, respectively. For CA-OV-3, the IC50 in 24 and 48 h was 18.76 and 11.58 µM. For HO-8910, the IC50 in 24 and 48 h was 12.70 and 6.80 µM, respectively. Subsequently, a colony formation assay was also carried out; PL exposure caused a dose-dependent reduction in the number and size of colonies formed, compared with the control (Figures 6D, E). These data supported the inhibitory role of PL in ovarian cancer cell growth and colony formation. Additionally, PL induced lower levels of CDK1 and CCNB1 in a concentration-dependent manner, which is necessary for G2/M phase transitions of the cell cycle (Figure 6F). These results suggested that PL could inhibit EOC cell proliferation and affect the expression of CDK1.
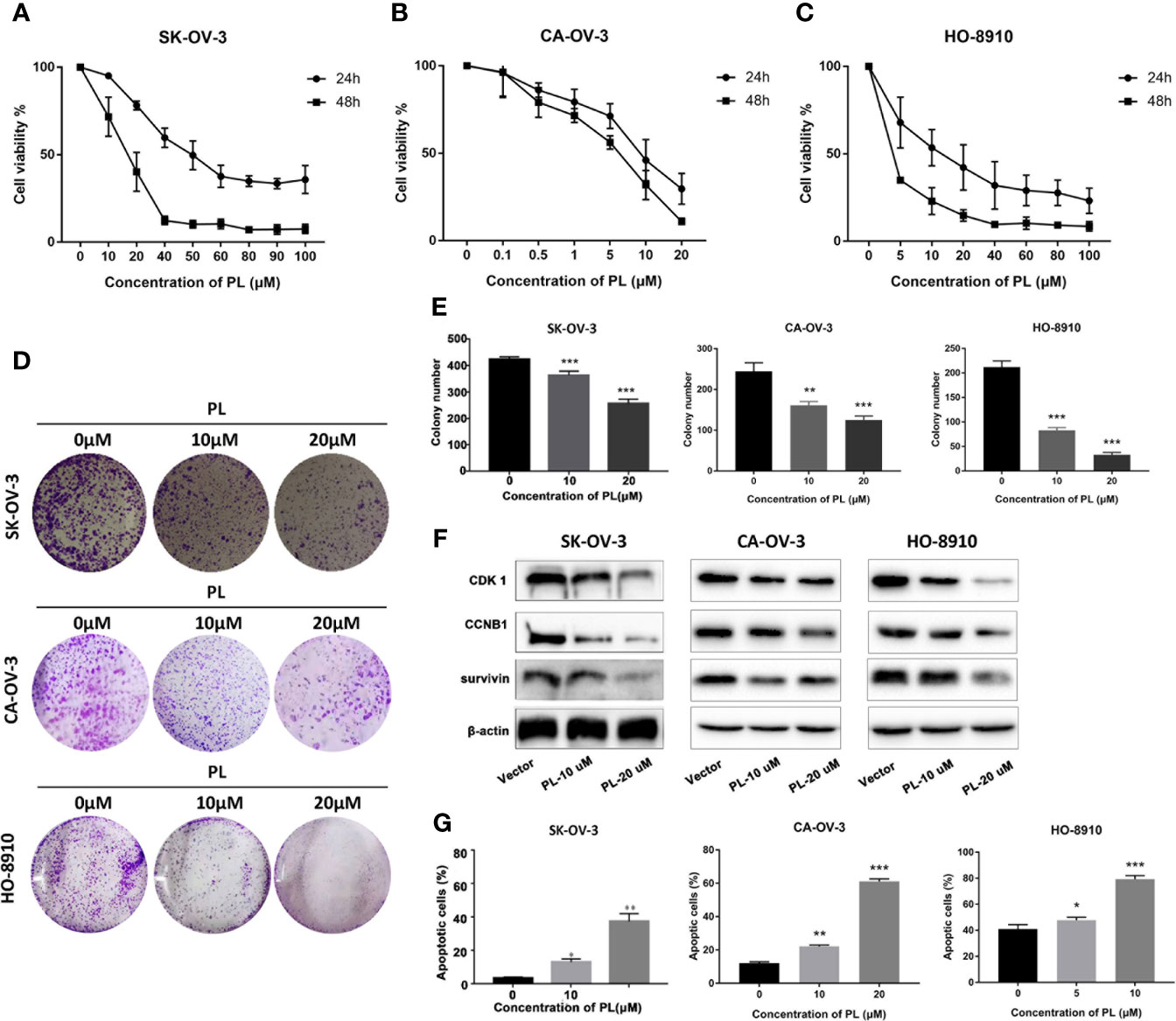
Figure 6 PL inhibited growth and induced apoptosis via targeting CDK1 in EOC cells. (A–C) Cell viability was assessed by MTT assay at 24 h and 48 h after treatment, and IC50 was computed accordingly. (D, E) Cell proliferation capacity was evaluated by colony formation assay, where cells after treatment were plated with a density of 200 cells/well and grown for 7 days followed by staining. (F) Expression levels of CDK1, CCNB1, and survivin proteins after treatment of PL at 10 or 20 µM for 24 h in EOC cells by Western blot analysis. β-Actin was used as an equal loading control for normalization. (G) Apoptotic cells were evaluated by Annexin-V and PI staining and analyzed by flow cytometry. All data are shown as mean ± standard deviation (n = 3). One-way ANOVA with the multiple-comparison test was used to calculate statistics: *p < 0.05; **p < 0.01; ***p < 0.001.
Furthermore, to determine whether apoptosis was involved in PL-induced cytotoxicity, SKOV3 cells exposed to PL were stained with Annexin-V/FITC followed by flow cytometric analysis (Figure 6G). We observed an increase in apoptosis to 37.6% and 53.4% at 20 µM after 24 and 48 h, respectively.
Discussion
In the current study, using gene expression data, a cluster of drugs that could potentially treat EOC was identified. Firstly, by merging TCGA mRNA-seq datasets and GEO mRNA microarray datasets, we generated overlapping DEGs as EOC signatures. Then, by integrating CMAP and LINCS databases, we identified potential drugs with lower negative connectivity scores that could evidently reverse EOC signatures. Based on the literature, four of these drugs were previously used clinically to treat EOC either as first-line treatment or as agents in clinical trials. This implies that we successfully predicted a group of known EOC drugs, without any hint of advanced drug information, suggesting that the remaining drug (piperlongumine) that we identified also has a high likelihood of treating EOC. Piperlongumine has been reported to inhibit several cancers, and only one study focused on ovarian cancer (24). In our study, the potential application of PL in EOC was further explored with a molecular docking test and in vitro experiments.
By merging EOC datasets from TCGA and GEO, 247 genes in total were considered as overlapping DEGs in EOC, including 103 common upregulated genes and 144 common downregulated genes. Then, common upregulated genes were mainly enriched in G2/M transition of the mitotic cell cycle, and the p53 signaling pathway, which are deemed to be a crucial pathway in the development and metastasis of EOC (25). Also, CDK1 was selected as the significant hub gene from the PPI network. It is well known that the abrogation of CDK1-CCNB1 activity blocks mitotic entry and arrests cells at the G2 phase (4).
We computed both CMAP and LINCS datasets to identify novel EOC drugs. Although CMAP has achieved remarkable success (26), some of its limitations cannot be ignored. For example, only five human cancer cell lines were used, and not all small molecules were tested on all these. The other drawbacks included limitations of dosages and time points and that several small molecules were tested using a 10-mM concentration with a 6-h perturbation time point. What is encouraging, LINCS covers 72 human cell lines and various cellular perturbations, including 15,000 small molecule compounds and 5,000 genes (gene silencing and overexpression). So we leveraged the LINCS dataset to increase the reliability of CMAP (27). In this study, the drugs with negative connectivity score were expected to be inversely correlated with EOC. Therefore, we focused on the five drugs with lower connectivity score from both CMAP and LINCS datasets. Among them, doxorubicin has been used clinically to treat ovarian cancer primarily by the mechanism of topoisomerase inhibition (28, 29). Vorinostat and scriptaid, two HDAC inhibitors, have been tested in ovarian cancer-associated clinical trials (30, 31). Methotrexate has been used as maintenance therapy in patients with advanced ovarian carcinoma (32). However, there is little evidence for PL treating ovarian cancer. Moreover, GSEA analysis showed that PL correlated with DNA replication, nucleotide excision repair, mismatch repair, and homologous recombination, which are important mechanisms for EOC drug resistance.
The molecular docking test, based on structure to design and understand the specific molecular mechanism, plays an important role in discovering drugs. There are a lot of successful examples in academia and industry. For instance, Mohamed et al. found that hesperidin formed a stable complex with a Polo-like kinase-1 active site by the approach of docking (33), Yu et al. used molecular docking to validate that Prestwick-685 and menadione may be the new esophageal carcinoma drugs (34). In our study, we selected the Schrodinger Glide docking protocol (35) to precisely simulate the interaction patterns and illustrate how PL acts on CDK1 proteins in the human body. Surprisingly, the results from docking tests demonstrated that PL could recognize and interact with CDK1 protein with a docking score of –8.121 kcal/mol, which was close to that of the known CDK1 inhibitor, AZD5438 (–8.24 kcal/mol). Therefore, it is suggested that PL has a considerable prospective role in the treatment of EOC by suppressing CDK1 proteins.
Piperlongumine, a biologically active alkaloid isolated from the roots of long pepper, is widely used as a traditional medicine in Ayurvedic medicine (36). It has been reported that PL selectively induces tumor cells death and delays tumor growth in hematologic tumors (37, 38) and diverse solid tumors (39–41). Furthermore, recent studies indicated that PL synergizes with cisplatin or paclitaxel to inhibit the growth of both chemoresistant and chemosensitive ovarian cancer cells (24). Currently, the cytotoxic effect of PL depends on the increase in reactive oxygen species (24, 42) and induction of apoptosis and autophagy, restoration of mutant p53, and cell cycle arrest (43–45). However, the arrest of G2/M triggered by PL was only detected as a phenotype. Hence, it needs a precise study to uncover how PL influences the cell cycle.
Due to the central role of CDK1 in the regulation of the G2/M phase, targeting CDK1 has emerged as a highly promising therapeutic strategy. Currently, several CDKIs have been investigated in clinical trials for treatment of various types of malignancies. For example, AZD5438, a CDK1/2 inhibitor, preferentially targets proliferating cells and typical chemosensitivity or radiosensitivity modulators (7, 46). However, the lack of inhibitor specificity currently limits clinical development. In our study, we predicted the possibility of PL being a novel selective CDK1 inhibitor. Mechanistically, in our study, PL inhibited cell viability in a dose- and time-dependent manner and induced apoptosis in ovarian cancer cells. In addition, PL led to decreased levels of the proteins CDK1 and cyclin B. Therefore, our study defines the details of PL which may target CDK1 to inhibit EOC.
In summary, we screened the DEGs from both two sources (TCGA and GEO), repurposed drugs by the two-generation CMAP database (CMAP and LINCS). Then, molecular docking and in vitro experiments were performed to explore and validate the drug–target interactions. However, there are also certain limitations; we only selected one ovarian cancer cell line and had no in vivo test to validate the exact function of PL to ovarian cancer. Therefore, to some extent, we utilized both in silico and in vitro experiments to predict that PL could be a novel drug to treat EOC. Furthermore, the results need more further research, as well as in vivo experiments.
Data Availability Statement
The original contributions presented in the study are included in the article/Supplementary Material. Further inquiries can be directed to the corresponding authors.
Author Contributions
YiZ and YeZ designed research; JB and EL performed bioinformatics research; DZ, CY, and ZJ performed cell research; JL, ZW, and MX sorted data; DZ, and LJ wrote the paper and revised the manuscript. All authors contributed to the article and approved the submitted version.
Funding
This work was supported by the National Natural Science Foundation of China (Nos. 82073244, 81270036, 30901736), the Plan to Focus on Research and Development from the Science and Technology Project of Liaoning Province (No. 2017225029), and Shenyang Youth Science and Technology Innovation Talent Project (RC200267).
Conflict of Interest
The authors declare that the research was conducted in the absence of any commercial or financial relationships that could be construed as a potential conflict of interest.
Publisher’s Note
All claims expressed in this article are solely those of the authors and do not necessarily represent those of their affiliated organizations, or those of the publisher, the editors and the reviewers. Any product that may be evaluated in this article, or claim that may be made by its manufacturer, is not guaranteed or endorsed by the publisher.
Supplementary Material
The Supplementary Material for this article can be found online at: https://www.frontiersin.org/articles/10.3389/fonc.2021.745590/full#supplementary-material
Abbreviations
CDK1, cyclin-dependent kinase 1; CMAP, Connectivity Map; DEGs, differentially expressed genes; EOC, epithelial ovarian cancer; GEO, Gene Expression Omnibus; GEPIA: the Gene Expression Profiling Interactive Analysis; GO, gene ontology; GTEx, the Genotype-Tissue Expression; KEGG, Kyoto Encyclopedia of Genes and Genomes; PPI, protein–protein interaction; PL, piperlongumine; TCGA, The Cancer Genome Atlas.
References
1. Miller KD, Goding Sauer A, Ortiz AP, Fedewa SA, Pinheiro PS, Tortolero-Luna G, et al. Cancer Statistics for Hispanics/Latinos, 2018. CA: Cancer J Clin (2018) 68(6):425–45. doi: 10.3322/caac.21494
2. du Bois A, Quinn M, Thigpen T, Vermorken J, Avall-Lundqvist E, Bookman M, et al. 2004 Consensus Statements on the Management of Ovarian Cancer: Final Document of the 3rd International Gynecologic Cancer Intergroup Ovarian Cancer Consensus Conference (GCIG OCCC 2004). Ann Oncol (2005) 16 Suppl 8:viii7–viii12. doi: 10.1093/annonc/mdi961
3. du Bois A, Reuss A, Pujade-Lauraine E, Harter P, Ray-Coquard I, Pfisterer J. Role of Surgical Outcome as Prognostic Factor in Advanced Epithelial Ovarian Cancer: A Combined Exploratory Analysis of 3 Prospectively Randomized Phase 3 Multicenter Trials: By the Arbeitsgemeinschaft Gynaekologische Onkologie Studiengruppe Ovarialkarzinom (AGO-OVAR) and the Groupe D’investigateurs Nationaux Pour Les Etudes Des Cancers De L’ovaire (GINECO). Cancer (2009) 115(6):1234–44. doi: 10.1002/cncr.24149
4. Hosoya N, Miyagawa K. Targeting DNA Damage Response in Cancer Therapy. Cancer Sci (2014) 105(4):370–88. doi: 10.1111/cas.12366
5. Coleman RL, Oza AM, Lorusso D, Aghajanian C, Oaknin A, Dean A, et al. Rucaparib Maintenance Treatment for Recurrent Ovarian Carcinoma After Response to Platinum Therapy (ARIEL3): A Randomised, Double-Blind, Placebo-Controlled, Phase 3 Trial. Lancet (2017) 390(10106):1949–61. doi: 10.1016/S0140-6736(17)32440-6
6. Curigliano G, Gomez Pardo P, Meric-Bernstam F, Conte P, Lolkema MP, Beck JT, et al. Ribociclib Plus Letrozole in Early Breast Cancer: A Presurgical, Window-of-Opportunity Study. Breast (2016) 28:191–8. doi: 10.1016/j.breast.2016.06.008
7. Raghavan P, Tumati V, Yu L, Chan N, Tomimatsu N, Burma S, et al. AZD5438, an Inhibitor of Cdk1, 2, and 9, Enhances the Radiosensitivity of Non-Small Cell Lung Carcinoma Cells. Int J Radiat Oncol Biol Phys (2012) 84(4):e507–14. doi: 10.1016/j.ijrobp.2012.05.035
8. Byth KF, Thomas A, Hughes G, Forder C, McGregor A, Geh C, et al. AZD5438, a Potent Oral Inhibitor of Cyclin-Dependent Kinases 1, 2, and 9, Leads to Pharmacodynamic Changes and Potent Antitumor Effects in Human Tumor Xenografts. Mol Cancer Ther (2009) 8(7):1856–66. doi: 10.1158/1535-7163.MCT-08-0836
9. Gupta SC, Sung B, Prasad S, Webb LJ, Aggarwal BB. Cancer Drug Discovery by Repurposing: Teaching New Tricks to Old Dogs. Trends Pharmacol Sci (2013) 34(9):508–17. doi: 10.1016/j.tips.2013.06.005
10. Moreau P, Attal M, Hulin C, Arnulf B, Belhadj K, Benboubker L, et al. Bortezomib, Thalidomide, and Dexamethasone With or Without Daratumumab Before and After Autologous Stem-Cell Transplantation for Newly Diagnosed Multiple Myeloma (CASSIOPEIA): A Randomised, Open-Label, Phase 3 Study. Lancet (2019) 394(10192):29–38. doi: 10.1016/S0140-6736(19)31240-1
11. Lee MS, Hsu CC, Wahlqvist ML, Tsai HN, Chang YH, Huang YC. Type 2 Diabetes Increases and Metformin Reduces Total, Colorectal, Liver and Pancreatic Cancer Incidences in Taiwanese: A Representative Population Prospective Cohort Study of 800,000 Individuals. BMC Cancer (2011) 11:20. doi: 10.1186/1471-2407-11-20
12. Smyth G. Limma: Linear Models for Microarray Data. In: Gentleman R, Carey V, Dudoit S, Irizarry R, Huber W, editors. Bioinformatics and Computational Biology Solutions Using R and Bioconductor. New York: Springer (2005). p. 397–420.
13. Tang Z, Li C, Kang B, Gao G, Li C, Zhang Z. GEPIA: A Web Server for Cancer and Normal Gene Expression Profiling and Interactive Analyses. Nucleic Acids Res (2017) 45(W1):W98–W102. doi: 10.1093/nar/gkx247
14. Tripathi S, Pohl MO, Zhou Y, Rodriguez-Frandsen A, Wang G, Stein DA, et al. Meta- and Orthogonal Integration of Influenza “OMICs” Data Defines a Role for UBR4 in Virus Budding. Cell Host Microbe (2015) 18(6):723–35. doi: 10.1016/j.chom.2015.11.002
15. Shannon P, Markiel A, Ozier O, Baliga NS, Wang JT, Ramage D, et al. Cytoscape: A Software Environment for Integrated Models of Biomolecular Interaction Networks. Genome Res (2003) 13(11):2498–504. doi: 10.1101/gr.1239303
16. Sun G, Li Y, Peng Y, Lu D, Zhang F, Cui X, et al. Identification of Differentially Expressed Genes and Biological Characteristics of Colorectal Cancer by Integrated Bioinformatics Analysis. J Cell Physiol (2019). doi: 10.1002/jcp.28163
17. Chin CH, Chen SH, Wu HH, Ho CW, Ko MT, Lin CY. cytoHubba: Identifying Hub Objects and Sub-Networks From Complex Interactome. BMC Syst Biol (2014) 8 Suppl 4:S11. doi: 10.1186/1752-0509-8-S4-S11
18. Lamb J, Crawford ED, Peck D, Modell JW, Blat IC, Wrobel MJ, et al. The Connectivity Map: Using Gene-Expression Signatures to Connect Small Molecules, Genes, and Disease. Science (2006) 313(5795):1929–35. doi: 10.1126/science.1132939
19. Subramanian A, Narayan R, Corsello SM, Peck DD, Natoli TE, Lu X, et al. A Next Generation Connectivity Map: L1000 Platform and the First 1,000,000 Profiles. Cell (2017) 171(6):1437–52.e17. doi: 10.1016/j.cell.2017.10.049
20. Wu T, Hu E, Xu S, Chen M, Guo P, Dai Z, et al. clusterProfiler 4.0: A Universal Enrichment Tool for Interpreting Omics Data. Innovation (2021) 2(3):100141. doi: 10.1016/j.xinn.2021.100141
21. Yu G, Wang L, Han Y, He Q. clusterProfiler: An R Package for Comparing Biological Themes Among Gene Clusters. OMICS: A J Integr Biol (2012) 16(5):284–7. doi: 10.1089/omi.2011.0118
22. Halgren TA, Murphy RB, Friesner RA, Beard HS, Frye LL, Pollard WT, et al. Glide: A New Approach for Rapid, Accurate Docking and Scoring. 2. Enrichment Factors in Database Screening. J Med Chem (2004) 47(7):1750–9. doi: 10.18632/oncotarget.3253
23. Zhang Y, Qu X, Teng Y, Li Z, Xu L, Liu J, et al. Cbl-B Inhibits P-Gp Transporter Function by Preventing Its Translocation Into Caveolae in Multiple Drug-Resistant Gastric and Breast Cancers. Oncotarget (2015) 6(9):6737–48. doi: 10.18632/oncotarget.3253
24. Gong LH, Chen XX, Wang H, Jiang QW, Pan SS, Qiu JG, et al. Piperlongumine Induces Apoptosis and Synergizes With Cisplatin or Paclitaxel in Human Ovarian Cancer Cells. Oxid Med Cell Longev (2014) 2014:906804. doi: 10.1155/2014/906804
25. Lin ZP, Zhu YL, Ratner ES. Targeting Cyclin-Dependent Kinases for Treatment of Gynecologic Cancers. Front Oncol (2018) 8:303. doi: 10.3389/fonc.2018.00303
26. Kunkel SD, Suneja M, Ebert SM, Bongers KS, Fox DK, Malmberg SE, et al. mRNA Expression Signatures of Human Skeletal Muscle Atrophy Identify a Natural Compound That Increases Muscle Mass. Cell Metab (2011) 13(6):627–38. doi: 10.1016/j.cmet.2011.03.020
27. Zador Z, King AT, Geifman N. New Drug Candidates for Treatment of Atypical Meningiomas: An Integrated Approach Using Gene Expression Signatures for Drug Repurposing. PloS One (2018) 13(3):e0194701. doi: 10.1371/journal.pone.0194701
28. Gabizon AA, Patil Y, La-Beck NM. New Insights and Evolving Role of Pegylated Liposomal Doxorubicin in Cancer Therapy. Drug Resist Update (2016) 29:90–106. doi: 10.1016/j.drup.2016.10.003
29. Coleman RL, Monk BJ, Sood AK, Herzog TJ. Latest Research and Treatment of Advanced-Stage Epithelial Ovarian Cancer. Nat Rev Clin Oncol (2013) 10(4):211–24. doi: 10.1038/nrclinonc.2013.5
30. Matulonis U, Berlin S, Lee H, Whalen C, Obermayer E, Penson R, et al. Phase I Study of Combination of Vorinostat, Carboplatin, and Gemcitabine in Women With Recurrent, Platinum-Sensitive Epithelial Ovarian, Fallopian Tube, or Peritoneal Cancer. Cancer Chemother Pharmacol (2015) 76(2):417–23. doi: 10.1007/s00280-015-2813-9
31. Takai N, Ueda T, Nishida M, Nasu K, Narahara H. A Novel Histone Deacetylase Inhibitor, Scriptaid, Induces Growth Inhibition, Cell Cycle Arrest and Apoptosis in Human Endometrial Cancer and Ovarian Cancer Cells. Int J Mol Med (2006) 17(2):323–9. doi: 10.3892/ijmm.17.2.323
32. El-Husseiny K, Motawei H, Ali MS. Continuous Low-Dose Oral Cyclophosphamide and Methotrexate as Maintenance Therapy in Patients With Advanced Ovarian Carcinoma After Complete Clinical Response to Platinum and Paclitaxel Chemotherapy. Int J Gynecol Cancer (2016) 26(3):437–42. doi: 10.1097/IGC.0000000000000647
33. AlAjmi MF, Rehman MT, Hussain A, Rather GM. Pharmacoinformatics Approach for the Identification of Polo-Like Kinase-1 Inhibitors From Natural Sources as Anti-Cancer Agents. Int J Biol Macromol (2018) 116:173–81. doi: 10.1016/j.ijbiomac.2018.05.023
34. Chen YT, Xie JY, Sun Q, Mo WJ. Novel Drug Candidates for Treating Esophageal Carcinoma: A Study on Differentially Expressed Genes, Using Connectivity Mapping and Molecular Docking. Int J Oncol (2019) 54(1):152–66. doi: 10.3892/ijo.2018.4618
35. Sastry GM, Adzhigirey M, Day T, Annabhimoju R, Sherman W. Protein and Ligand Preparation: Parameters, Protocols, and Influence on Virtual Screening Enrichments. J Comput Aided Mol Des (2013) 27(3):221–34. doi: 10.1007/s10822-013-9644-8
36. Bezerra DP, Pessoa C, de Moraes MO, Saker-Neto N, Silveira ER, Costa-Lotufo LV. Overview of the Therapeutic Potential of Piplartine (Piperlongumine). Eur J Pharm Sci (2013) 48(3):453–63. doi: 10.1016/j.ejps.2012.12.003
37. Han SS, Son DJ, Yun H, Kamberos NL, Janz S. Piperlongumine Inhibits Proliferation and Survival of Burkitt Lymphoma in vitro. Leuk Res (2013) 37(2):146–54. doi: 10.1016/j.leukres.2012.11.009
38. Han SS, Han S, Kamberos NL. Piperlongumine Inhibits the Proliferation and Survival of B-Cell Acute Lymphoblastic Leukemia Cell Lines Irrespective of Glucocorticoid Resistance. Biochem Biophys Res Commun (2014) 452(3):669–75. doi: 10.1016/j.bbrc.2014.08.131
39. Wang F, Mao Y, You Q, Hua D, Cai D. Piperlongumine Induces Apoptosis and Autophagy in Human Lung Cancer Cells Through Inhibition of PI3K/Akt/mTOR Pathway. Int J Immunopathol Pharmacol (2015) 28(3):362–73. doi: 10.1177/0394632015598849
40. Bharadwaj U, Eckols TK, Kolosov M, Kasembeli MM, Adam A, Torres D, et al. Drug-Repositioning Screening Identified Piperlongumine as a Direct STAT3 Inhibitor With Potent Activity Against Breast Cancer. Oncogene (2015) 34(11):1341–53. doi: 10.1038/onc.2014.72
41. Wang Y, Wu X, Zhou Y, Jiang H, Pan S, Sun B. Piperlongumine Suppresses Growth and Sensitizes Pancreatic Tumors to Gemcitabine in a Xenograft Mouse Model by Modulating the NF-Kappa B Pathway. Cancer Prev Res (Phila) (2016) 9(3):234–44. doi: 10.1158/1940-6207.CAPR-15-0306
42. Han LC, Stanley PA, Wood PJ, Sharma P, Kuruppu AI, Bradshaw TD, et al. Horner-Wadsworth-Emmons Approach to Piperlongumine Analogues With Potent Anti-Cancer Activity. Org Biomol Chem (2016) 14(31):7585–93. doi: 10.1039/C6OB01160H
43. Basak D, Punganuru SR, Srivenugopal KS. Piperlongumine Exerts Cytotoxic Effects Against Cancer Cells With Mutant P53 Proteins at Least in Part by Restoring the Biological Functions of the Tumor Suppressor. Int J Oncol (2016) 48(4):1426–36. doi: 10.3892/ijo.2016.3372
44. Wang Y, Wang JW, Xiao X, Shan Y, Xue B, Jiang G, et al. Piperlongumine Induces Autophagy by Targeting P38 Signaling. Cell Death Dis (2013) 4:e824. doi: 10.1038/cddis.2013.358
45. Bezerra DP, Moura DJ, Rosa RM, de Vasconcellos MC, e Silva AC, de Moraes MO, et al. Evaluation of the Genotoxicity of Piplartine, an Alkamide of Piper Tuberculatum, in Yeast and Mammalian V79 Cells. Mutat Res (2008) 652(2):164–74. doi: 10.1016/j.mrgentox.2008.02.001
46. Sorf A, Novotna E, Hofman J, Morell A, Staud F, Wsol V, et al. Cyclin-Dependent Kinase Inhibitors AZD5438 and R547 Show Potential for Enhancing Efficacy of Daunorubicin-Based Anticancer Therapy: Interaction With Carbonyl-Reducing Enzymes and ABC Transporters. Biochem Pharmacol (2019) 163:290–8. doi: 10.1016/j.bcp.2019.02.035
Keywords: epithelial ovarian cancer, CDK1, piperlongumine, molecular docking, cell cycle
Citation: Zou D, Bai J, Lu E, Yang C, Liu J, Wen Z, Liu X, Jin Z, Xu M, Jiang L, Zhang Y and Zhang Y (2021) Identification of Novel Drug Candidate for Epithelial Ovarian Cancer via In Silico Investigation and In Vitro Validation. Front. Oncol. 11:745590. doi: 10.3389/fonc.2021.745590
Received: 22 July 2021; Accepted: 28 September 2021;
Published: 21 October 2021.
Edited by:
Chi Zhang, Indiana University Bloomington, United StatesReviewed by:
Zongyue Zeng, First Affiliated Hospital of Chongqing Medical University, ChinaEnze Liu, Indiana University, United States
Copyright © 2021 Zou, Bai, Lu, Yang, Liu, Wen, Liu, Jin, Xu, Jiang, Zhang and Zhang. This is an open-access article distributed under the terms of the Creative Commons Attribution License (CC BY). The use, distribution or reproduction in other forums is permitted, provided the original author(s) and the copyright owner(s) are credited and that the original publication in this journal is cited, in accordance with accepted academic practice. No use, distribution or reproduction is permitted which does not comply with these terms.
*Correspondence: Yi Zhang, c3l6aUAxNjMuY29t; Ye Zhang, emhhbmd5ZWNtdUAxNjMuY29t
†These authors have contributed equally to this work and share first authorship