- 1Department of Hepatobiliary and Pancreatic Surgery, The Second Affiliated Hospital, Zhejiang University School of Medicine, Hangzhou, China
- 2Department of Pathology, The Second Affiliated Hospital, Zhejiang University School of Medicine, Hangzhou, China
- 3Department of Radiology, The First Affiliated Hospital, Zhejiang University School of Medicine, Hangzhou, China
Background: Microvascular invasion (MVI) has been shown to be closely associated with postoperative recurrence and metastasis in patients with intrahepatic cholangiocarcinoma (ICC). We aimed to develop a radiomics prediction model based on contrast-enhanced CT (CECT) to distinguish MVI in patients with mass-forming ICC.
Methods: 157 patients were included and randomly divided into training (n=110) and test (n=47) datasets. Radiomic signatures were built based on the recursive feature elimination support vector machine (Rfe-SVM) algorithm. Significant clinical-radiologic factors were screened, and a clinical model was built by multivariate logistic regression. A nomogram was developed by integrating radiomics signature and the significant clinical risk factors.
Results: The portal phase image radiomics signature with 6 features was constructed and provided an area under the receiver operating characteristic curve (AUC) of 0.804 in the training and 0.769 in the test datasets. Three significant predictors, including satellite nodules (odds ratio [OR]=13.73), arterial hypo-enhancement (OR=4.31), and tumor contour (OR=4.99), were identified by multivariate analysis. The clinical model using these predictors exhibited an AUC of 0.822 in the training and 0.756 in the test datasets. The nomogram combining significant clinical factors and radiomics signature achieved satisfactory prediction efficacy, showing an AUC of 0.886 in the training and 0.80 in the test datasets.
Conclusions: Both CECT radiomics analysis and radiologic factors have the potential for MVI prediction in mass-forming ICC patients. The nomogram can further improve the prediction efficacy.
Introduction
Intrahepatic cholangiocarcinoma (ICC) arises from the epithelial cells of the intrahepatic bile ducts and is the second leading primary liver malignancy (1). ICC can be classified into intraductal, periductal infiltrative, and mass-forming types with macroscopic growth classification. The mass-forming ICC is the most predominant type, accounting for 80% to 90% of all ICC cases (2, 3). According to statistics, the incidence and mortality of ICC are continuously increasing worldwide over the years (4, 5). Surgery is the mainstay of therapy for localized, resectable ICC; Nevertheless, the prognosis remains unsatisfactory, with 5-year overall survival ranging from 15% to 23% (6, 7). High rates of recurrence and metastasis following resection are the leading causes of poor prognosis. In fact, recurrence is estimated to occur in 45% to 70% of patients with ICC (7–10).
Studies have revealed that microvascular invasion (MVI) is a considerable poor-prognostic factor in ICC. MVI has been shown to be closely associated with postoperative recurrence and metastasis (11–13). Tsukamoto et al. (14) reported that the absence of MVI and lymph node metastasis were the only two independent factors for recurrence-free survival over 5 years after liver resection for ICC. Hu et al. (15) showed that ICC patients with MVI exhibited enhanced aggressive behavior with a higher incidence of adjacent tissue infiltration, organ invasion, and satellite lesions; In addition, patients with MVI had a significantly worse disease-free survival (DFS) than patients without MVI. Moreover, Ercolani et al. (16) demonstrated that patients without MVI significantly experienced favorable median overall survival (OS) time than those patients with MVI in all types of cholangiocarcinoma. In contrast to macrovascular invasion, which can be evaluated by radiologic images, MVI can only be detected by postoperative pathological examination, limiting its value in the clinical setting. Recently, some studies have been performed to identify preoperative predictive markers for ICC patients with MVI. Laboratory parameters, including routine blood tests, liver function, and cancer biomarkers such as ALT, AFP, CA-199, have been screened and defined as predictive indicators (17, 18). However, despite the relative ease of obtaining these data, some parameters are controversial and have to be systematically evaluated in clinical practice. Radiological characteristics, such as tumor morphology, arterial phase enhancement pattern, tumor diameter, and apparent diffusion coefficient (ADC) values, were associated with MVI in ICC patients (18, 19). However, imaging features were assessed subjectively and may lead to interobserver variability.
Radiomics, an omics-based approach allowing for the extraction of quantitative features from raw medical images, has been used to perform objective and quantitative analysis of tumor heterogeneity and cancer phenotype (20). Radiomics has been widely utilized in predicting MVI for patients with hepatocellular carcinoma (HCC), and its feasibility and potential benefits have been proved (21–23). However, few studies have evaluated the prediction value of radiomics for ICC. Zhou et al. (24) exacted features from MRI images and a fusion radiomics signature comprising seven features was established for MVI prediction in ICC patients with an area under the receiver operating characteristic curve (AUC) of 0.85. Contrast-enhanced CT (CECT) is the most common imaging modality for diagnosis and assessment of ICC; however, there are currently no studies that evaluated the radiomics analysis of CECT for MVI prediction in patients with ICC.
Therefore, we aimed to verify whether radiomics analysis based on CECT could be useful to predict MVI in mass-forming ICC. Additionally, clinical-radiologic predictors were also evaluated and compared with radiomics analysis.
Materials and Methods
Patient Characteristics
182 patients with pathologic diagnosis of ICC after hepatectomy were retrospectively identified in our hospital from March 2013 to May 2021. Inclusion criteria were as follows: (1) Malignancies were classified as mass-forming ICC; (2) CECT scans performed within two weeks before surgery; (3) MVI status was described on pathology reports. Exclusion criteria were: (1) Pathology-confirmed malignancies were mixed, periductal infiltrative or intraductal growing types of ICC; (2) prior intervention or partial hepatectomy; (3) Lack of contrast-enhanced CT scans or insufficient image quality; (4) Grossly tumor thrombus in the portal vein or bile duct tumor thrombosis. The detailed selection process is described in Figure 1. Of the 182 screened patients,157 patients were finally enrolled and randomly assigned to a training dataset (n=110) and a test dataset (n=47), with a split ratio of 7:3. The Ethics committee of our hospital approved the present retrospective study.
Clinical Characteristics and Radiological Evaluation
Demographic covariates were collected and compared between the MVI group and non-MVI group both in training and test datasets, including age, sex, hepatitis B immunology, platelet count (PLT), serum albumin (ALB), serum direct bilirubin (DB), serum total bilirubin (TB), γ-glutamyl transpeptidase (GGT), alkaline phosphatase (ALP), serum alanine aminotransferase (ALT), aspartate aminotransferase (AST), international normalized ratio (INR), prothrombin time (PT), carcinoembryonic antigen (CEA), Carbohydrate antigen199 (CA-199). Radiological features, including the number of segments involved, satellite nodules, lymph node status, intrahepatic duct dilatation, tumor contour, arterial rim enhancement, arterial hypo-enhancement, intratumor vascularity, hepatic capsular retraction, were blindly evaluated and recorded by two readers. The detail of radiological evaluation and our protocol for CECT scan acquisition is described in the Supplementary Presentation.
Histology
All resected specimens were examined and cross-checked by at least two senior pathologists. Seven tissues were harvested and examined for MVI diagnosis from the resected specimen, including the central and four sides of tumor tissues and two adjacent non-carcinoma tissues. MVI was defined as the invasion of tumor emboli into a vascular space that only can be detected on microscopy (25).
Radiomic Feature Extraction
The radiomics workflow is depicted in Figure 2. Region of interests (ROIs) of the whole tumor was contoured on arterial and portal venous phase of CT images using ITK-SNAP software (The specific ROIs segmentation was shown in the Supplementary Presentation). The Pyradiomics toolkit was used to extract features from each three-dimensional ROI (26). For each phase, 1130 radiomics features were extracted, including 18 first-order features, 14 shape features, 75 textural features, 279 Laplacian of Gaussian features (sigma=3.0,4.0,5.0), and 744 wavelet features. In total, 2260 features derived from arterial and portal phases were obtained for each patient. The parameter setting for radiomics features extraction and the detailed radiomics features is depicted in the Supplementary Presentation and Supplementary Table.
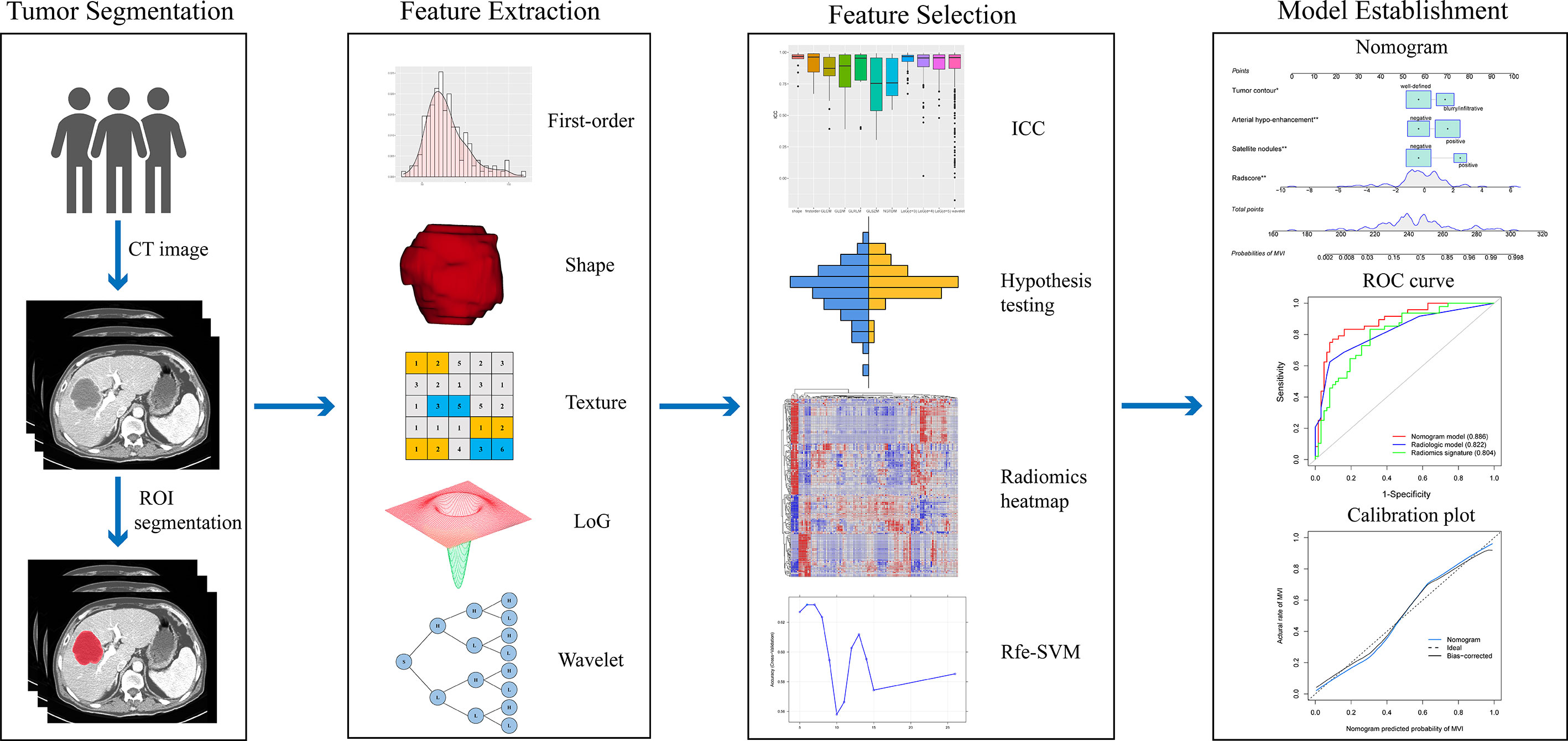
Figure 2 Workflow for the radiomics process. After CT images were acquired, segmentation of the tumor was performed. The extracted radiomics features include first-order, shape, Laplacian of Gaussian, texture, and wavelet features. A four-step approach was performed for feature dimension reduction. Intra- and inter-class correlation coefficient (ICC) was used to evaluate the reproductivity of features. Values lower than 0.8 were eliminated. Student’s t-test or Mann–Whitney-U test was performed to find the differential radiomics features. A heatmap shows the Pearson correlation coefficients matrix among radiomics features. Rfe-SVM was applied to develop the radiomics signature. A nomogram was constructed by integrating independent radiological predictors and portal phase image radiomics signature. The nomogram and radiologic model’s discriminative ability were compared with the ROC curve analysis and quantified by the AUC. The calibration curve demonstrated good agreement between the actual and nomogram predicted probabilities.
Radiomics Signature Construction
Before feature dimension reduction, values of radiomics features were transformed to a normal distribution by the z-score method. Then, feature dimension reduction and selection were performed in a four-step approach. Firstly, the intra- and inter-class correlation coefficient was used to evaluate the repeatability of radiomics features between the intra- and inter-observer. Features with intraclass and interclass correlation coefficients lower than 0.80 were eliminated. The specific process was described in the Supplementary Presentation. Secondly, Mann–Whitney-U test or Student’s t-test was applied to find the differential radiomics features with effects of p < 0.05 were considered statistically significant. Thirdly, Pearson correlation coefficient > 0.75 was used as a cutoff to eliminate the highly correlated features. Lastly, a recursive feature elimination support vector machine (Rfe-SVM) method was performed to construct the radiomics signature. The formula of the radiomics signature was displayed in the Supplementary Presentation.
According to the process set up above, three radiomics signatures were developed based on features exacted from the arterial phase, portal phase, and pooling features of the two phases. Receiver operating characteristic (ROC) analysis was performed to assess the three signatures’ predictive capability.
Development of the Clinical Model and the Nomogram
For constructing the clinical model, univariate analysis was applied to identify independent risk factors (including clinical characteristics and CECT radiological features) between the MVI and the non-MVI groups. The multivariate logistic model was built with significant factors (p<0.05) from the univariate analysis as inputs. Odds ratios (OR) and their 95% confidence intervals (CI) were used to express the estimate relative risk. The clinical-radiomics nomogram was built using the optimal radiomics signature and independent clinical-radiologic risk factors in the clinical model. Variance inflation factor (VIF) coefficients were examined to check the collinearity. Comparison of the ROC curves among nomogram model, radiomics signature, and clinical model was performed by using DeLong’s test. A calibration curve was plotted to evaluate the calibration of the nomogram.
Statistical Analysis
The Chi-square test or Fisher exact test was used for categorical variables comparison. For continuous variables, Student’s t-test or Mann-Whitney U test was used. We considered p < 0.05 (two-tailed) as statistically significant. Statistical analysis was conducted with SPSS (v. 26.0), R software (v. 3.6.1), and Python (v. 3.9.2).
Results
Patient Characteristics
The baseline clinical-radiological characteristics are demonstrated in Table 1. The incidence of MVI between the training and test datasets shows no statistical difference (48/110 vs. 20/47, p=0.900). Patients with MVI were associated with larger tumor size, more liver segments invasion, and blurry or infiltrative tumor contour, which was confirmed in the training and test datasets. Significant differences were found in terms of age, PLT, satellite nodules, lymph node metastasis and arterial hypo-enhancement in the training dataset but not validated in the test dataset. Other baseline variables did not differ between the training and test datasets.
Radiomics Signature Construction
A total of 1130 radiomics features were identified from each imaging phase. For features identified from the arterial phase image, 319 features were excluded with intraclass and interclass correlation coefficients lower than 0.8 (Figure S1). Then, 811 features were subjected to statistical hypothesis testing with Student’s t-test or Mann–Whitney-U test; 276 features were found significantly different between the MVI positive and non-MVI groups. Pearson correlation analysis found 254 features were highly correlated (correlation coefficient > 0.75) and were eliminated. Only 22 features were kept and subjected to Ref-SVM. Finally, 5 features were selected, and the arterial phase image radiomics signature was built.
The portal phase image radiomics signature with 6 features and fusion radiomics signature with 12 features were built with a similar process. The specific flow and the selected features of three radiomic signatures were shown in the Figure S2 and Table 2. The arterial phase image radiomics signature, portal phase image radiomics signature, and fusion radiomics signature showed good discriminative abilities for MVI prediction, with AUCs of 0.776 (95% CI 0.688–0.863), 0.804 (95% CI 0.723–0.885), and 0.779 (95% CI 0.692–0.865) in the training dataset, and AUCs of 0.726 (95% CI 0.581–0.871), 0.769 (95% CI 0.630–0.908), and 0.763 (95% CI 0.627–0.898), in the test dataset (Figure 3). The portal phase image radiomics signature achieved slightly better predictive performance than the other two radiomics signatures, but no statistical differences were found.
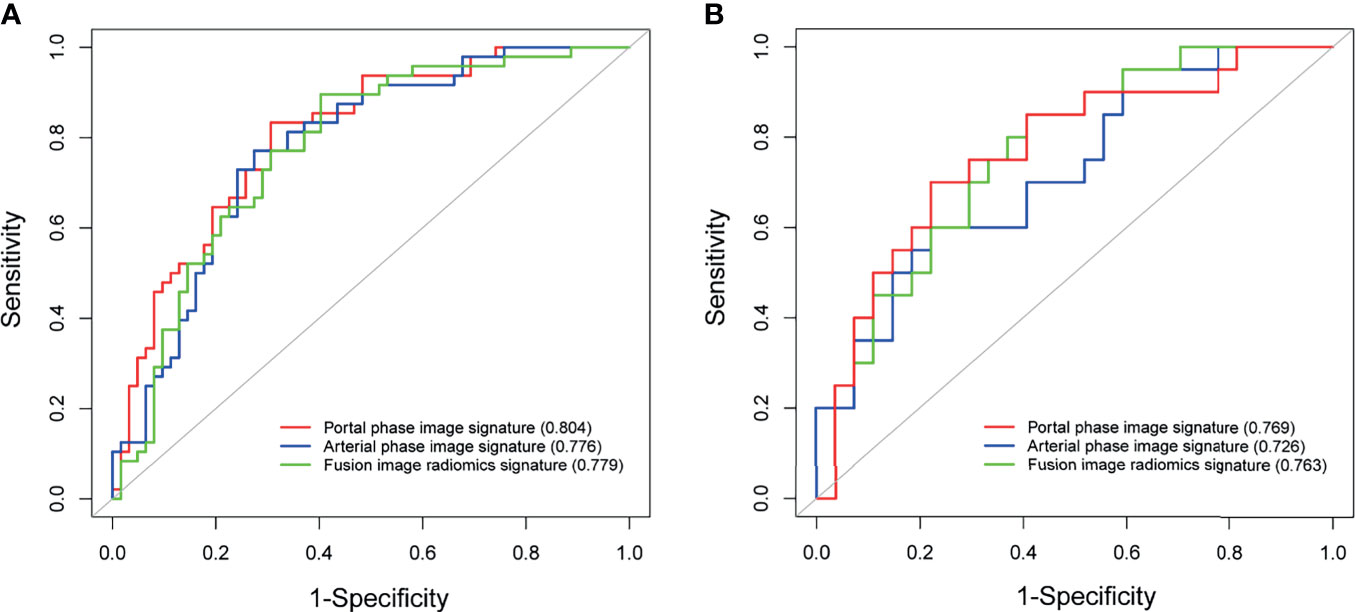
Figure 3 Predictive performance of radiomic signatures for microvascular invasion. ROC curves of radiomic signatures in the training dataset (A). ROC curves of radiomic signatures in the test dataset (B).
Compared to non-MVI group, the MVI positive group had a significantly higher portal phase image Rad-score [median (IQR)] in the training dataset [0.549 (-0.302∼1.244) vs −0.916 (-1.829∼-0.050), P < 0.001]. Consistent results were obtained in the test dataset [1.151 (-0.075∼1.855) vs −0.505 (-1.032∼0.511), P=0.003] (Figure S3).
Development of the Radiologic Model and Nomogram
Among all baseline variables, three significant predictors including satellite nodules [OR=13.73 (3.14–59.93), P<0.001], arterial hypo-enhancement [OR=4.31 (1.55–11.94), P=0.005], and tumor contour [OR=4.99 (1.76–14.18), P=0.003] were identified in the training dataset by univariate analysis and further confirmed in multivariate analysis (Table 3). The radiologic model developed with the three independent risk factors exhibited AUC of 0.822 (95% CI: 0.741–0.903) and 0.756 (95% CI: 0.616–0.895) in the training and test datasets.
We generated the nomogram using the independent predictors in the radiologic model and portal phase image radiomics signature with logistic regression (Figure 4). The VIFs for satellite nodules, arterial hypo-enhancement, tumor contour, and portal phase image radiomics signature were less than 10 (satellite nodules: 1.11; arterial hypo-enhancement: 1.14; tumor contour: 1.02; radiomics signature: 1.03), suggesting no collinearity between these variables. The nomogram demonstrated satisfactory prediction efficacy, with an AUC of 0.886 (95% CI: 0.823–0.949) and 0.80 (95% CI: 0.675–0.925) in the training and test datasets. The specific performances of nomogram are shown in Table 4. In the training dataset, the nomogram achieved higher AUC than the radiologic model (P =0.011) and portal phase image signature (P = 0.019) (Figure 5A). However, there were no statistical differences in the test dataset (nomogram vs radiologic model, portal phase image radiomics signature; P = 0.322, P = 0.642, respectively) (Figure 5B).
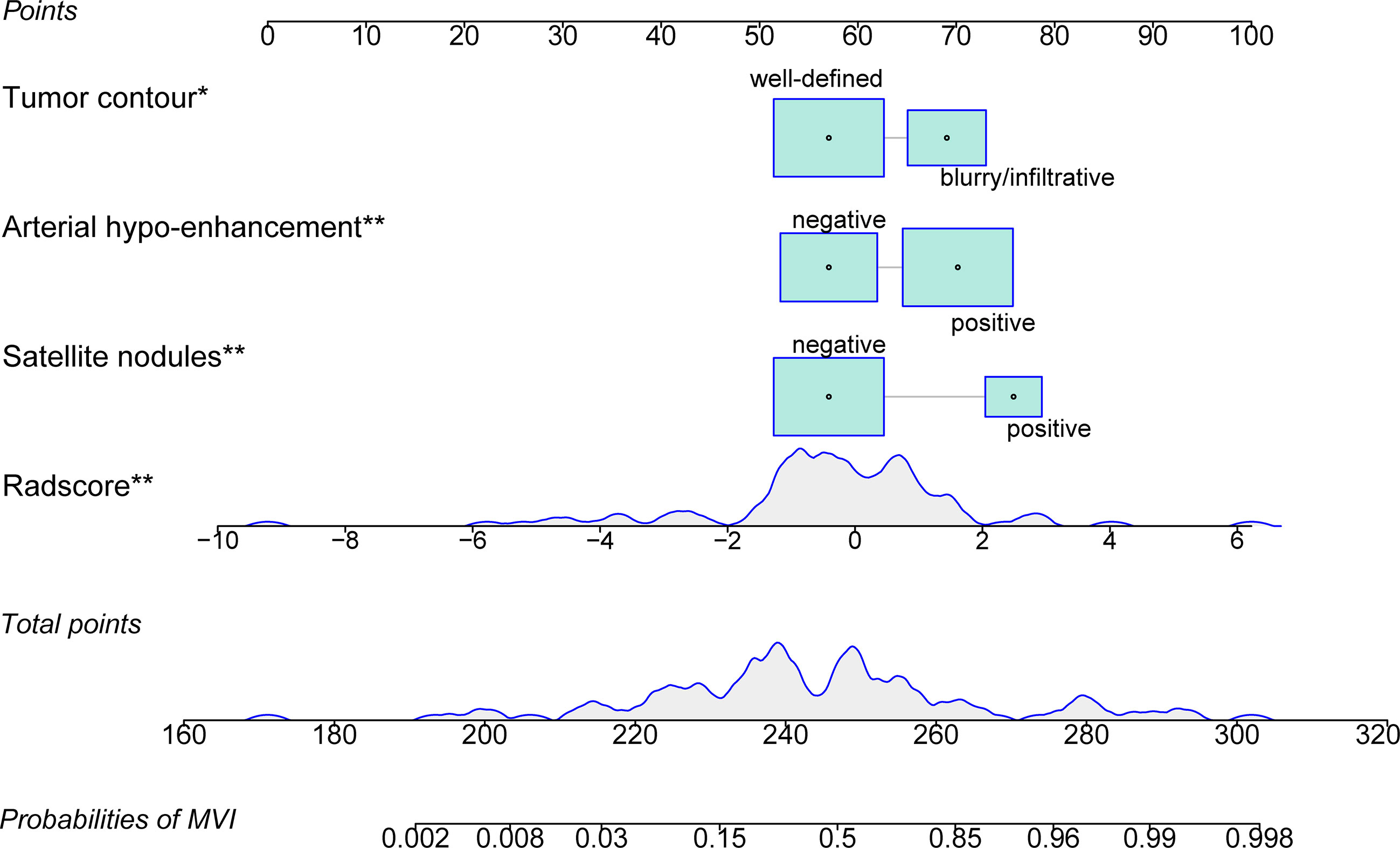
Figure 4 The radiomics nomogram was developed by incorporating the portal phase image radiomics signature, satellite nodules, arterial hypo-enhancement, and tumor contour. *p < 0.05; **p < 0.01.
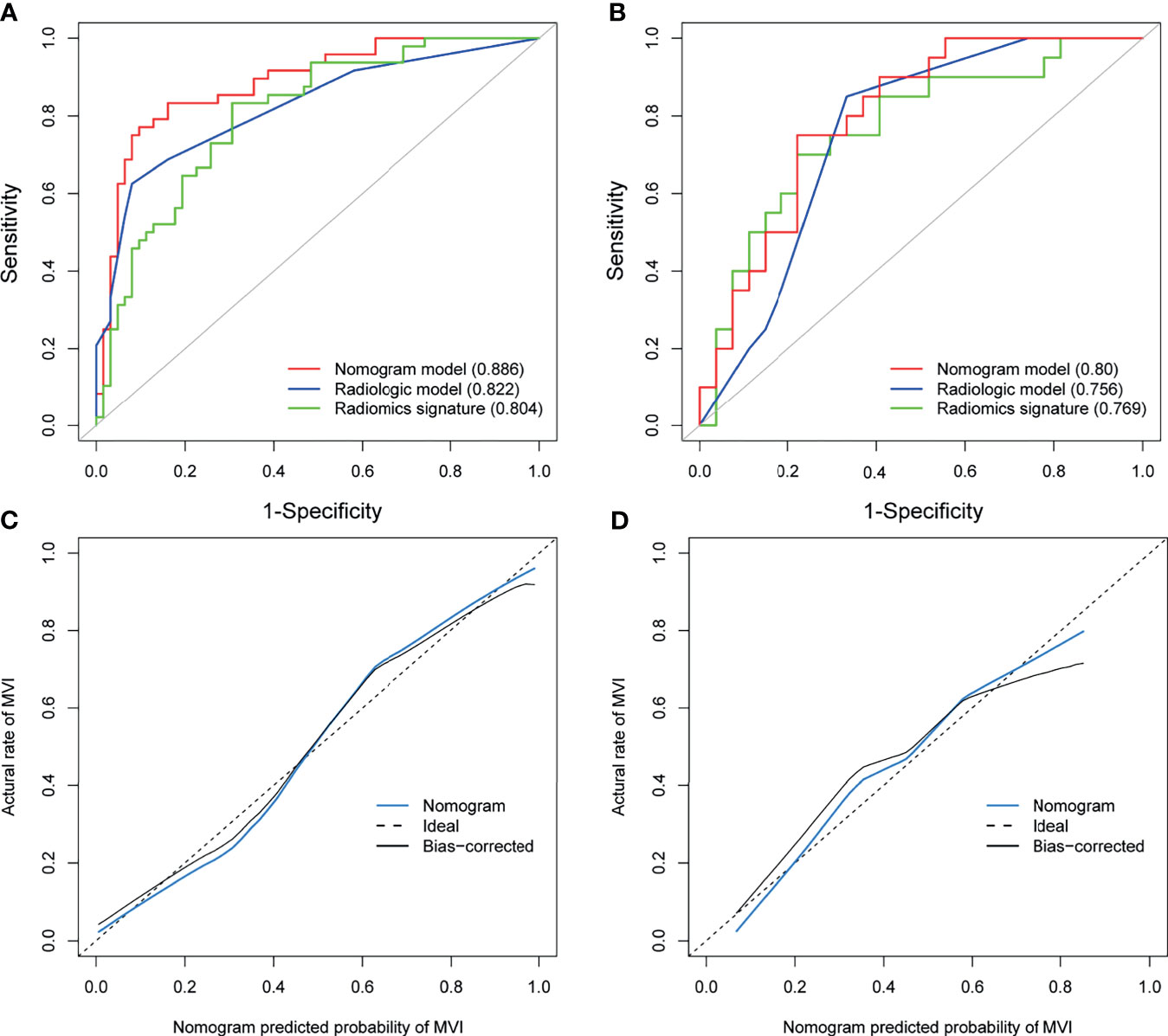
Figure 5 Assessing the discriminative performance of the nomogram and comparison with other predictive models. The nomogram showed a significantly higher discriminative power than the radiomics signature and the radiologic model for the prediction of microvascular invasion in the training dataset (A), but did not differ in the test dataset (B). The calibration plots demonstrate that the nomogram-predicted probabilities were consistent with actual MVI incidence in the training (C) and test (D) datasets.
The calibration plots (Figures 5C, D) were consistent between the nomogram prediction and the actual observed probability. Decision curve analysis (Figure S4) revealed that the nomogram achieved highest net benefit compared with the radiologic model and radiomics signature. The Hosmer-Lemeshow test showed no significant both in the training (p=0.206) and test datasets (p=0.529), indicating the nomogram was well fitted. A representative case showing the discriminative ability of the nomogram is depicted in Figure S5.
Discussion
With increasing recognition of MVI and its prognostic value after hepatectomy, preoperative MVI prediction has become a research hotspot in recent years. In fact, MVI status has been considered as an important event for preoperative surgical decision-making in China. Some studies recommend that anatomical liver resection or wide resection margin is a priority for HCC patients with MVI (27–30). In ICC patients, many studies suggested that a wide surgical margin may lead to favorable survival benefits (7, 31–33). Hu et al. (32) reported that very early recurrence (defined as recurrence within 6 months) after ICC hepatectomy mostly occurred in the surgical margin site. We think that MVI plays an important role in early recurrence after ICC hepatectomy, because MVI generally occurs on the tumor edges. Furthermore, Shao et al. (34) demonstrated that the distance of MVI from the tumor was associated with survival and recurrence in ICC patients, and it seemed that there was an incremental worsening DFS and OS as distance increased.
In this study, the radiomics approach and conventional clinical-radiologic method were used and compared to predict the presence of MVI in ICC patients. The two achieved comparable performance in the prediction of MVI. Furthermore, the nomogram combined radiomics signature and significant radiological factors achieved satisfactory discriminative ability, shown by an AUC of 0.886 in the training dataset and 0.80 in the test dataset. The radiomics features selected in this study were mainly wavelet transformation features consistent with other studies for MVI prediction (21, 22, 24). Among the six features in portal phase image signature, skewness measures the asymmetry of the gray level values distribution. Three GLCM features reflect the linear relation of gray level values or the local image homogeneity. GLSZM size zone nonuniformity reflects the image variability of size zone volumes. NGTDM strength is a measure of the primitives in an image. The wavelet transformation of raw images can further reflect more accurate spatial changes across multiple dimensions (26). Features included in this study varied from Zhou’s result (24), which may be attributed to the different image modalities analyzed.
From the comparison results of baseline characteristics, we found that the number of liver segments invaded, tumor size, tumor contour, age, PLT, satellite nodules, lymph node metastasis, arterial hypo-enhancement showed a significant difference between the MVI group and non-MVI group in the training dataset. However, only tumor contour, satellite nodules, and arterial hypo-enhancement were selected as significant risk factors by multiple regression. Tumor morphology is a key feature for MVI prediction both in HCC and ICC. It seems that MVI-negative patients are inclined to have a well-defined, spherical, oval tumor contour, whereas those with MVI tend to show irregular, blurry or infiltrative contours (18, 19, 35, 36). Our results are in agreement with these reports. In terms of satellite nodules, micro-metastases invade into the portal venous system and subsequently spread to the tumor-bearing portal territories and eventually develop into microsatellite nodules (37, 38). Such a mechanism has long been accepted as the main cause of intrahepatic metastasis and postoperative recurrence (39, 40). Satellite nodules are common in ICC patients, and the incidence is reported as high as 30% (41–43). The enhancement pattern of the mass-forming ICC in the hepatic arterial phase of CECT could be classified into three types: the hypo-enhancement, the rim-enhancement, and the hyper-enhancement (44). Several studies suggest that the different enhancement patterns of mass-forming ICC show varied prognosis, and the hypo-enhancement is often associated with worse survival (44–46). Yugawa et al. (47) reported that among the three enhancement patterns, the lowest tumor microvessel density was found in the hypo-enhancement ICC and was often accompanied by larger tumor size, more frequent microvascular invasion, and a higher rate of intrahepatic and lymph node metastasis.
Our study has some limitations. Due to the highly aggressive behavior of ICC, only a low number of patients admitted to our hospital have the chance for surgical resection, thus leading to limited sample size in our study. Secondly, retrospective nature of the study may introduce inevitable selection bias. Thirdly, this is a single-center study with internal validation was performed and in the absence of external validation. Therefore, further refinements with prospective multicenter studies are needed to check out our results.
Conclusion
The findings in this study verified that both radiomics analysis and radiologic factors have the potential for MVI prediction in mass-forming ICC patients. The advantage of radiomics is that it can detect microscopic structures and quantitatively measure the microscopic changes in tissue caused by disease. The radiologic method was convenient and fast and also demonstrated good diagnostic efficacy. The combined nomogram, which integrated radiologic factors and radiomics signature, further improved the predictive performance for MVI diagnosis.
Data Availability Statement
The original contributions presented in the study are included in the article/Supplementary Material. Further inquiries can be directed to the corresponding author.
Ethics Statement
The studies involving human participants were reviewed and approved by The Ethics committee of the Second Affiliated Hospital of Zhejiang University School of Medicine. The patients/participants provided their written informed consent to participate in this study.
Author Contributions
FX and SY were responsible for the conception of the work. SW reviewed pathological pictures. FX, XLia and XLiu obtained the data. FX and LY segmented the images. FX and XLia analyzed the data. FX wrote the manuscript. SY critically revised the manuscript. All authors are accountable for the contents of this work. All authors contributed to the article and approved the submitted version.
Funding
This study was supported by grants from the National Natural Science Foundation of China (No.81572975) and Key research and development project of science and technology department of Zhejiang (No.2015C03053).
Conflict of Interest
The authors declare that the research was conducted in the absence of any commercial or financial relationships that could be construed as a potential conflict of interest.
Publisher’s Note
All claims expressed in this article are solely those of the authors and do not necessarily represent those of their affiliated organizations, or those of the publisher, the editors and the reviewers. Any product that may be evaluated in this article, or claim that may be made by its manufacturer, is not guaranteed or endorsed by the publisher.
Supplementary Material
The Supplementary Material for this article can be found online at: https://www.frontiersin.org/articles/10.3389/fonc.2021.774117/full#supplementary-material
References
1. Bridgewater J, Galle PR, Khan SA, Llovet JM, Park JW, Patel T, et al. Guidelines for the Diagnosis and Management of Intrahepatic Cholangiocarcinoma. J Hepatol (2014) 60(6):1268–89. doi: 10.1016/j.jhep.2014.01.021
2. Chung T, Rhee H, Nahm JH, Jeon Y, Yoo JE, Kim YJ, et al. Clinicopathological Characteristics of Intrahepatic Cholangiocarcinoma According to Gross Morphologic Type: Cholangiolocellular Differentiation Traits and Inflammation- and Proliferation-Phenotypes. HPB (Oxford) (2020) 22(6):864–73. doi: 10.1016/j.hpb.2019.10.009
3. Meng ZW, Pan W, Hong HJ, Chen JZ, Chen YL. Macroscopic Types of Intrahepatic Cholangiocarcinoma and the Eighth Edition of AJCC/UICC TNM Staging System. Oncotarget (2017) 8(60):101165–74. doi: 10.18632/oncotarget.20932
4. Beal EW, Tumin D, Moris D, Zhang XF, Chakedis J, Dilhoff M, et al. Cohort Contributions to Trends in the Incidence and Mortality of Intrahepatic Cholangiocarcinoma. Hepatobiliary Surg Nutr (2018) 7(4):270–6. doi: 10.21037/hbsn.2018.03.16
5. Khan SA, Genus T, Morement H, Murphy A, Rous B, Tataru D. Global Trends in Mortality From Intrahepatic and Extrahepatic Cholangiocarcinoma. J Hepatol (2019) 71(6):1261–2. doi: 10.1016/j.jhep.2019.07.024
6. Qu WF, Zhou PY, Liu WR, Tian MX, Jin L, Jiang XF, et al. Age-Adjusted Charlson Comorbidity Index Predicts Survival in Intrahepatic Cholangiocarcinoma Patients After Curative Resection. Ann Transl Med (2020) 8(7):487. doi: 10.21037/atm.2020.03.23
7. Spolverato G, Yakoob MY, Kim Y, Alexandrescu S, Marques HP, Lamelas J, et al. The Impact of Surgical Margin Status on Long-Term Outcome After Resection for Intrahepatic Cholangiocarcinoma. Ann Surg Oncol (2015) 22(12):4020–8. doi: 10.1245/s10434-015-4472-9
8. Nickkholgh A, Ghamarnejad O, Khajeh E, Tinoush P, Bruckner T, Kulu Y, et al. Outcome After Liver Resection for Primary and Recurrent Intrahepatic Cholangiocarcinoma. BJS Open (2019) 3(6):793–801. doi: 10.1002/bjs5.50217
9. Langella S, Russolillo N, Ossola P, Luzzi AP, Casella M, Lo Tesoriere R, et al. Recurrence After Curative Resection for Intrahepatic Cholangiocarcinoma: How to Predict the Chance of Repeat Hepatectomy? J Clin Med (2021) 10(13):2820. doi: 10.3390/jcm10132820
10. Spolverato G, Kim Y, Alexandrescu S, Marques HP, Lamelas J, Aldrighetti L, et al. Management and Outcomes of Patients With Recurrent Intrahepatic Cholangiocarcinoma Following Previous Curative-Intent Surgical Resection. Ann Surg Oncol (2016) 23(1):235–43. doi: 10.1245/s10434-015-4642-9
11. Tsilimigras DI, Sahara K, Wu L, Moris D, Bagante F, Guglielmi A, et al. Very Early Recurrence After Liver Resection for Intrahepatic Cholangiocarcinoma: Considering Alternative Treatment Approaches. JAMA Surg (2020) 155(9):823–31. doi: 10.1001/jamasurg.2020.1973
12. Ali SM, Clark CJ, Mounajjed T, Wu TT, Harmsen WS, Reid-Lombardo KM, et al. Model to Predict Survival After Surgical Resection of Intrahepatic Cholangiocarcinoma: The Mayo Clinic Experience. HPB (Oxford) (2015) 17(3):244–50. doi: 10.1111/hpb.12333
13. Wang T, Zhang J, Wang W, Yang X, Kong J, Shen S, et al. Development and Validation of Nomograms for Predicting Cancer-Specific Survival in Elderly Patients With Intrahepatic Cholangiocarcinoma After Liver Resection: A Competing Risk Analysis. Cancer Manag Res (2020) 12:11015–29. doi: 10.2147/cmar.S272797
14. Tsukamoto M, Yamashita YI, Imai K, Umezaki N, Yamao T, Okabe H, et al. Predictors of Cure of Intrahepatic Cholangiocarcinoma After Hepatic Resection. Anticancer Res (2017) 37(12):6971–5. doi: 10.21873/anticanres.12164
15. Hu LS, Weiss M, Popescu I, Marques HP, Aldrighetti L, Maithel SK, et al. Impact of Microvascular Invasion on Clinical Outcomes After Curative-Intent Resection for Intrahepatic Cholangiocarcinoma. J Surg Oncol (2019) 119(1):21–9. doi: 10.1002/jso.25305
16. Ercolani G, Dazzi A, Giovinazzo F, Ruzzenente A, Bassi C, Guglielmi A, et al. Intrahepatic, Peri-Hilar and Distal Cholangiocarcinoma: Three Different Locations of the Same Tumor or Three Different Tumors? Eur J Surg Oncol (2015) 41(9):1162–9. doi: 10.1016/j.ejso.2015.05.013
17. Tang Z, Liu WR, Zhou PY, Ding ZB, Jiang XF, Wang H, et al. Prognostic Value and Predication Model of Microvascular Invasion in Patients With Intrahepatic Cholangiocarcinoma. J Cancer (2019) 10(22):5575–84. doi: 10.7150/jca.32199
18. Ma X, Liu L, Fang J, Rao S, Lv L, Zeng M, et al. MRI Features Predict Microvascular Invasion in Intrahepatic Cholangiocarcinoma. Cancer Imaging (2020) 20(1):40. doi: 10.1186/s40644-020-00318-x
19. Zhou Y, Wang X, Xu C, Zhou G, Liu X, Gao S, et al. Mass-Forming Intrahepatic Cholangiocarcinoma: Can Diffusion-Weighted Imaging Predict Microvascular Invasion? J Magn Reson Imaging (2019) 50(1):315–24. doi: 10.1002/jmri.26566
20. Gillies RJ, Kinahan PE, Hricak H. Radiomics: Images Are More Than Pictures, They Are Data. Radiology (2016) 278(2):563–77. doi: 10.1148/radiol.2015151169
21. Xu X, Zhang HL, Liu QP, Sun SW, Zhang J, Zhu FP, et al. Radiomic Analysis of Contrast-Enhanced CT Predicts Microvascular Invasion and Outcome in Hepatocellular Carcinoma. J Hepatol (2019) 70(6):1133–44. doi: 10.1016/j.jhep.2019.02.023
22. Zhang X, Ruan S, Xiao W, Shao J, Tian W, Liu W, et al. Contrast-Enhanced CT Radiomics for Preoperative Evaluation of Microvascular Invasion in Hepatocellular Carcinoma: A Two-Center Study. Clin Transl Med (2020) 10(2):e111. doi: 10.1002/ctm2.111
23. Yang L, Gu D, Wei J, Yang C, Rao S, Wang W, et al. A Radiomics Nomogram for Preoperative Prediction of Microvascular Invasion in Hepatocellular Carcinoma. Liver Cancer (2019) 8(5):373–86. doi: 10.1159/000494099
24. Zhou Y, Zhou G, Zhang J, Xu C, Wang X, Xu P. Radiomics Signature on Dynamic Contrast-Enhanced MR Images: A Potential Imaging Biomarker for Prediction of Microvascular Invasion in Mass-Forming Intrahepatic Cholangiocarcinoma. Eur Radiol (2021) 31(9):6846–55. doi: 10.1007/s00330-021-07793-1
25. Cong WM, Bu H, Chen J, Dong H, Zhu YY, Feng LH, et al. Practice Guidelines for the Pathological Diagnosis of Primary Liver Cancer: 2015 Update. World J Gastroenterol (2016) 22(42):9279–87. doi: 10.3748/wjg.v22.i42.9279
26. van Griethuysen JJM, Fedorov A, Parmar C, Hosny A, Aucoin N, Narayan V, et al. Computational Radiomics System to Decode the Radiographic Phenotype. Cancer Res (2017) 77(21):e104–7. doi: 10.1158/0008-5472.Can-17-0339
27. Shimada S, Kamiyama T, Yokoo H, Orimo T, Wakayama K, Einama T, et al. Clinicopathological Characteristics of Hepatocellular Carcinoma With Microscopic Portal Venous Invasion and the Role of Anatomical Liver Resection in These Cases. World J Surg (2017) 41(8):2087–94. doi: 10.1007/s00268-017-3964-0
28. Zhong XP, Zhang YF, Mei J, Li SH, Kan A, Lu LH, et al. Anatomical Versus Non-Anatomical Resection for Hepatocellular Carcinoma With Microscope Vascular Invasion: A Propensity Score Matching Analysis. J Cancer (2019) 10(17):3950–7. doi: 10.7150/jca.32592
29. Hirokawa F, Hayashi M, Miyamoto Y, Asakuma M, Shimizu T, Komeda K, et al. Outcomes and Predictors of Microvascular Invasion of Solitary Hepatocellular Carcinoma. Hepatol Res (2014) 44(8):846–53. doi: 10.1111/hepr.12196
30. Yang P, Si A, Yang J, Cheng Z, Wang K, Li J, et al. A Wide-Margin Liver Resection Improves Long-Term Outcomes for Patients With HBV-Related Hepatocellular Carcinoma With Microvascular Invasion. Surgery (2019) 165(4):721–30. doi: 10.1016/j.surg.2018.09.016
31. Watanabe Y, Matsuyama Y, Izumi N, Kubo S, Kokudo N, Sakamoto M, et al. Effect of Surgical Margin Width After R0 Resection for Intrahepatic Cholangiocarcinoma: A Nationwide Survey of the Liver Cancer Study Group of Japan. Surgery (2020) 167(5):793–802. doi: 10.1016/j.surg.2019.12.009
32. Hu LS, Zhang XF, Weiss M, Popescu I, Marques HP, Aldrighetti L, et al. Recurrence Patterns and Timing Courses Following Curative-Intent Resection for Intrahepatic Cholangiocarcinoma. Ann Surg Oncol (2019) 26(8):2549–57. doi: 10.1245/s10434-019-07353-4
33. Liu H, Lin L, Lin Z, Chen Y, Huang Q, Ding L, et al. Impact of Surgical Margin Width on Long-Term Outcomes for Intrahepatic Cholangiocarcinoma: A Multicenter Study. BMC Cancer (2021) 21(1):840. doi: 10.1186/s12885-021-08560-7
34. Shao C, Chen J, Chen J, Shi J, Huang L, Qiu Y. Histological Classification of Microvascular Invasion to Predict Prognosis in Intrahepatic Cholangiocarcinoma. Int J Clin Exp Pathol (2017) 10(7):7674–81.
35. Renzulli M, Brocchi S, Cucchetti A, Mazzotti F, Mosconi C, Sportoletti C, et al. Can Current Preoperative Imaging Be Used to Detect Microvascular Invasion of Hepatocellular Carcinoma? Radiology (2016) 279(2):432–42. doi: 10.1148/radiol.2015150998
36. Meng XP, Wang YC, Zhou JY, Yu Q, Lu CQ, Xia C, et al. Comparison of MRI and CT for the Prediction of Microvascular Invasion in Solitary Hepatocellular Carcinoma Based on a Non-Radiomics and Radiomics Method: Which Imaging Modality Is Better? J Magn Reson Imaging (2021) 54(2):526–36. doi: 10.1002/jmri.27575
37. Makuuchi M, Hasegawa H, Yamazaki S. Ultrasonically Guided Subsegmentectomy. Surg Gynecol Obstet (1985) 161(4):346–50.
38. Shindoh J, Makuuchi M, Matsuyama Y, Mise Y, Arita J, Sakamoto Y, et al. Complete Removal of the Tumor-Bearing Portal Territory Decreases Local Tumor Recurrence and Improves Disease-Specific Survival of Patients With Hepatocellular Carcinoma. J Hepatol (2016) 64(3):594–600. doi: 10.1016/j.jhep.2015.10.015
39. Zhou XP, Quan ZW, Cong WM, Yang N, Zhang HB, Zhang SH, et al. Micrometastasis in Surrounding Liver and the Minimal Length of Resection Margin of Primary Liver Cancer. World J Gastroenterol (2007) 13(33):4498–503. doi: 10.3748/wjg.v13.i33.4498
40. Shindoh J, Hasegawa K, Inoue Y, Ishizawa T, Nagata R, Aoki T, et al. Risk Factors of Post-Operative Recurrence and Adequate Surgical Approach to Improve Long-Term Outcomes of Hepatocellular Carcinoma. HPB (Oxford) (2013) 15(1):31–9. doi: 10.1111/j.1477-2574.2012.00552.x
41. Schiffman SC, Nowacki MR, Spencer L, McMasters KM, Scoggins CR, Martin RC. Molecular Factors Associated With Recurrence and Survival Following Hepatectomy in Patients With Intrahepatic Cholangiocarcinoma: A Guide to Adjuvant Clinical Trials. J Surg Oncol (2014) 109(2):98–103. doi: 10.1002/jso.23459
42. Tarchi P, Tabrizian P, Prigoff J, Schwartz M. Outcomes of Resection for Solitary ≤5 Cm Intrahepatic Cholangiocarcinoma. Surgery (2018) 163(4):698–702. doi: 10.1016/j.surg.2017.09.058
43. Zhao YJ, Chen WX, Wu DS, Zhang WY, Zheng LR. Differentiation of Mass-Forming Intrahepatic Cholangiocarcinoma From Poorly Differentiated Hepatocellular Carcinoma: Based on the Multivariate Analysis of Contrast-Enhanced Computed Tomography Findings. Abdom Radiol (NY) (2016) 41(5):978–89. doi: 10.1007/s00261-015-0629-z
44. Fujita N, Asayama Y, Nishie A, Ishigami K, Ushijima Y, Takayama Y, et al. Mass-Forming Intrahepatic Cholangiocarcinoma: Enhancement Patterns in the Arterial Phase of Dynamic Hepatic CT - Correlation With Clinicopathological Findings. Eur Radiol (2017) 27(2):498–506. doi: 10.1007/s00330-016-4386-3
45. Teraoku H, Morine Y, Uyama N, Ikemoto T, Iwahashi S, Saito Y, et al. Role of Central Hypo-Enhancement in the Hepatic Arterial Phase of Dynamic Computed Tomography in Patients With Mass-Forming Intrahepatic Cholangiocarcinoma. World J Surg (2020) 44(7):2350–8. doi: 10.1007/s00268-020-05456-8
46. Kim SA, Lee JM, Lee KB, Kim SH, Yoon SH, Han JK, et al. Intrahepatic Mass-Forming Cholangiocarcinomas: Enhancement Patterns at Multiphasic CT, With Special Emphasis on Arterial Enhancement Pattern–Correlation With Clinicopathologic Findings. Radiology (2011) 260(1):148–57. doi: 10.1148/radiol.11101777
Keywords: intrahepatic cholangiocarcinoma, microvascular invasion, radiomics, computed tomography, nomogram
Citation: Xiang F, Wei S, Liu X, Liang X, Yang L and Yan S (2021) Radiomics Analysis of Contrast-Enhanced CT for the Preoperative Prediction of Microvascular Invasion in Mass-Forming Intrahepatic Cholangiocarcinoma. Front. Oncol. 11:774117. doi: 10.3389/fonc.2021.774117
Received: 11 September 2021; Accepted: 02 November 2021;
Published: 19 November 2021.
Edited by:
Guang Yang, Imperial College London, United KingdomReviewed by:
Jue Jiang, Memorial Sloan Ketter Cancer Center, United StatesRoberto Cannella, University of Palermo, Italy
Changqiang Wu, North Sichuan Medical College, China
Copyright © 2021 Xiang, Wei, Liu, Liang, Yang and Yan. This is an open-access article distributed under the terms of the Creative Commons Attribution License (CC BY). The use, distribution or reproduction in other forums is permitted, provided the original author(s) and the copyright owner(s) are credited and that the original publication in this journal is cited, in accordance with accepted academic practice. No use, distribution or reproduction is permitted which does not comply with these terms.
*Correspondence: Sheng Yan, shengyan@zju.edu.cn
†These authors have contributed equally to this work and share first authorship