- 1Department of Infectious Diseases, Shulan (Hangzhou) Hospital Affiliated to Zhejiang Shuren University Shulan International Medical College, Hangzhou, China
- 2College of Medicine, Zhejiang University, Hangzhou, China
- 3College of Basic Medical Sciences, Zhejiang Chinese Medical University, Hangzhou, China
- 4Shulan International Medical College, Zhejiang Shuren University, Hangzhou, China
- 5Research and Development Division, Oriomics Biotech Inc, Hangzhou, China
- 6Infection and Immunity Institute and Translational Medical Center of Huaihe Hospital, Henan University, Kaifeng, China
- 7State Key Laboratory for Diagnosis and Treatment of Infectious Diseases, First Affiliated Hospital, School of Medicine, Zhejiang University, Hangzhou, China
- 8National Clinical Research Center for Infectious Diseases, First Affiliated Hospital, School of Medicine, Zhejiang University, Hangzhou, China
- 9Collaborative Innovation Centre for Diagnosis and Treatment of Infectious Diseases, First Affiliated Hospital, College of Medicine, Zhejiang University, Hangzhou, China
- 10Department of Microbiology & Infectious Disease Center, School of Basic Medical Sciences, Peking University Health Science Center, Beijing, China
- 11Hepatology Institute, Peking University People’s Hospital, Beijing, China
Background: GALAD model is a statistical model used to estimate the possibility of hepatocellular carcinoma (HCC) in patients with chronic liver disease. Many studies with other ethnic populations have shown that it has high sensitivity and specificity. However, whether this model can be used for Chinese patients remains to be determined. Our study was conducted to verify the performance of GALAD model in a Chinese cohort and construct a new model that is more appropriately for Chinese populations.
Methods: There are total 512 patients enrolled in the study, which can be divided into training set and validation set. 80 patients with primary liver cancer, 139 patients with chronic liver disease and 87 healthy people were included in the training set. Through the ROC(receiver operating characteristic) curve analysis, the recognition performance of GALAD model for liver cancer was evaluated, and the GAADPB model was established by logistic regression, including gender, age, AFP, DCP, total protein, and total bilirubin. The validation set (75 HCC patients and 130 CLD patients) was used to evaluate the performance of the GAADPB model.
Result: The GALAD and GAADPB achieved excellent performance (area under the receiver operating characteristic curve [AUC], 0.925, 0.945), and were better than GAAP, Doylestown, BALAD-2, aMAP, AFP, AFP-L3%, DCP and combined detection of AFP, AFP-L3 and DCP (AUCs: 0.894, 0.870, 0.648, 0.545, 0.879, 0.782, 0.820 and 0.911) for detecting HCC from CLD in the training set. As for early stage of HCC (BCLC 0/A), GAADPB had the best sensitivity compared to GALAD, ADP and DCP (56.3%, 53.1%, 40.6%, 50.0%). GAADPB had better performance than GALAD in the test set, AUC (0.896 vs 0.888).
Conclusions: The new GAADPB model was powerful and stable, with better performance than the GALAD and other models, and it also was promising in the area of HCC prognosis prediction. Further study on the real-world HCC patients in China are needed.
Introduction
With approximately 906,000 new cases and 830,000 deaths globally in 2020, hepatocellular carcinoma (HCC) was known as the sixth most diagnosed cancer and the third leading cause of cancer-related mortality (1).The etiology of HCC is geographically diversified. Worldwide, most cases are related to HBV infection, while HCV infection is the most common case of HCC in some Western countries as well as Japan (2). In China, HBV infection is the leading cause of HCC (3). Surgical resection, ablation, or liver transplantation can be used to cure early-stage HCC. However, those patients who are diagnosed at an advanced stage have limited access to treatments and a poor prognosis (3). A lot of patients in China are already at an advanced stage at the time of diagnosis. Given that situation, early surveillance of HCC among high-risk population is of great importance.
For better surveillance of high-risk groups, abdominal ultrasound is recommended every six months, with or without alpha-fetoprotein (AFP) serum test. However, the test results are operator-dependent. The sensitivity could vary from 47% to 84% (4). Further, obesity might affect the performance of ultrasound (5). As a surveillance test, ultrasound still has some limitation, so AFP is often used in combination with ultrasound. While detection rate can be increased by combining these two methods, there is also an increased suspicion of false-positive and cost (6). Besides, patients with chronic viral hepatitis can also have an elevated alpha-fetoprotein (5). To monitor HCC more accurately at an early stage,other serum-based biomarkers, such as Lens culinaris agglutinin-reactive fraction of AFP (AFP-L3), and Des-gamma-carboxy prothrombin (DCP), have been put into use (7). However, none of the single serum marker for early surveillance of HCC has been proved to meet the clinical demands (8, 9).
The GALAD score, as well as Doylestown, BALAD and aMAP, which is derived from gender, age, AFP, AFP-L3, DCP, bilirubin, platelets and albumen, has showed high sensitivity for HCC detection in reported studies (9, 10). However, the studied samples of these reports had characteristics different from those of Chinese HCC patients, where hepatitis B virus (HBV) infection is the most common etiology of HCC in China whereas HCV infection was the main reason in other ethnic populations.
This study aimed to assess the performance of the GALAD model for HCC diagnosis and explore a better model for Chinese patients.
Materials and methods
Study populations
This study enrolled 307 participants in training set, consisting of 80 HCC patients, 140 non-cancer controls with CLD [100 patients with liver cirrhosis (LC) and 40 patients with chronic hepatitis B (CHB)] and 87 healthy controls (HC). In testing set, a total of 205 participants was enrolled, including 75 HCC patients and 130 CLD controls (106 LC/24 CHB). All participants were recruited between 2019 and 2021 from Shulan hospital in Hangzhou. For all patients, the diagnosis was established by the histologic examination of tumor tissue or characteristic medical imaging including computed tomography (CT), magnetic resonance imaging (MRI), according to clinical practice guidelines. We collected information on 29 clinical characteristics and other variables for all participants, consisting of age, gender, etiology of liver disease, laboratory results, and baseline tumor characteristics at the time of diagnosis (Tables 1, S1). Written informed consent was obtained from every participant and the study protocol was approved by the Ethics Committee of Shulan hospital.
Inclusion criteria
HCC patients were diagnosed by referring “Guidelines for the diagnosis and treatment of primary liver cancer”: 1) Histopathological diagnosis; or 2) Medical imaging diagnosis, includes of computed tomography (CT), magnetic resonance imaging (MRI), Contrast enhanced-ultrasonography(CEUS) or Gd-EOB-DTPA-MRI(EOB-MRI): A) if liver had a nodule equal or less than 2 cm, and more than 2 medicals imaging results revealing typical imaging lesions of HCC; B) if liver had a nodule greater than 2 cm, and more than 1 medical imaging results revealing typical imaging lesions of HCC; or C) if liver had not nodule, but AFP values was positive and more than 1 medical imaging results revealing typical imaging lesions of HCC. CHB patients were diagnosed by referring “The guideline for the prevention and treatment of CHB infection from the Chinese Society of Hepatology”: HBV infection more than 6 months, alanine aminotransferase (ALT) is persistently or repeatedly elevated, or hepatitis lesions are identified by liver biopsy. LC patients were diagnosed by referring “Chinese guidelines on the management of liver cirrhosis”: 1) Histopathological diagnosis; or 2) Medical imaging diagnosis, Ultrasound(US), CT or MRI imaging results revealing splenomegaly without liver space-occupying lesions. Healthy participants were a group of people with normal physical examination in Shulan Hospital: 1) No family history of cancer, no history of liver disease diagnosis and treatment; (2) no HBV or HCV infection; 3) liver function, renal function, and routine blood tests were normal; 4) Ultrasound results were normal in the liver or gallbladder system; and (5) liver fiber scan results were normal.
Exclusion criteria
(1) Subjects with HCC with other tumors; (2) Subjects who cannot be sampled, have insufficient sample size or have unqualified samples; (3) Subjects with liver metastases or HCC treatment (such as: surgery, ablation, radiotherapy or chemotherapy).
AFP, AFP-L3%, and DCP assay
About 10 mL peripheral blood was collected from each participant. For HCC and CLD patients, blood sample was drawn prior to the treatment. Serum AFP, AFP-L3%, and DCP were assayed by Hotgen Biotech Co., Ltd (Beijing, China) by using chemiluminescence microparticle immunoassay. The quantitative limit of AFP, AFP-L3, AFP-L3%, and DCP was 0.6-20000ng/mL, 0.6-20000ng/mL, 5–50% and 0.6-20000ng/mL, respectively. If these biomarker values exceeded extreme ones, we used extreme values to represent. On the other hand, if both AFP and AFP-L3 exceeded extreme values, we then used 10% (AFP-L3/AFP) to represent AFP-L3% positive.
Performance evaluation of five models for the discrimination of HCC from CLD
We compared five previous developed models for assessing their discrimination ability of HCC from CLD in our sample. GALAD score was calculated based on five variables (age, gender, AFP, AFP-L3 and DCP) (11). GAAP score was calculated on the basis of four variables (age, gender, AFP and DCP) (12). Doylestown score was calculated based on five variables (age, gender, AFP, ALP and ALT) (13). BALAD-2 score was calculated based on five variables (bilirubin, total albumin, AFP, AFP-L3 and DCP) (14). aMAP score was calculated based on five variables (age, gender, total bilirubin, albumin and PLT) (15). Table S2 shows the detailed equations of these models. Cutoff values of these models were based on published thresholds (GALAD: -0.63, GAAP: -0.65, Doylestown: 0.5, BALAD-2: 0.66, and aMAP: 60). Performance of these models was performed by calculating the area under each receiver operating characteristic (ROC) curve.
Model development
Logistic regression for multivariate analysis was used to assess the association of the 20 variables, including demographics, HCC biomarkers, liver function, blood routine test, and blood coagulation parameters; see Tables 1, S1) with HCC based on the use of a forward-backward stepwise approach. Participants with missing data were excluded from the statistical analysis. Logarithmic transformation of AFP, AFP-L3 and DCP values was applied for logistic regression analysis due to extreme skewness (11). Age has been considered as a good variable for HCC risk assessment (11, 14, 15). Therefore, we built a new HCC risk assessment model, called GAADPB based on variables (gender, AFP, DCP, TP and TB) selected from multivariate analyses and age. The formula was shown as follows:
GAADPB score = 0.176 + 0.162*gender +0.002*age +0.178*log10AFP+0.164*log10DCP-0.007*TP-0.002*TB
The probability of having HCC was calculated using the following formula: P(HCC) = exp[score]/(1 + exp[score]).
Statistical analysis methods
SPSS (version 22.0) software (IBM/SPSS Inc., Chicago, IL) or R language (version 3.4.4) were used to perform the statistical analysis and draw figures. Continuous variables were presented as medians (interquartile range) and categorical variables were presented as frequencies (percentage). Characteristics differences were tested using Wilcoxon test for continuous variables and Chi-square tests for categorical variables. SPSS software was used to analyze ROC curve. The results of sensitivity, specificity, and AUC were used to reveal models/biomarkers performance. Comparisons of ROC curves were performed using the “roc.test” function in pROC package in R language (parameter paired = “TRUE” and method = “delong”). All statistical tests were two-sided. P value ≤0.05 was considered to be statistically significant.
Results
Demographic data and clinical characteristics of enrolled participants in training set
A total of 307 participants were enrolled in the training set, including 80 HCC patients, 140 CLD (100 HC/40 CHB) and 87 HC participants. The demographic data, clinical characteristics and laboratory results of the study participants were described in Tables 1, S1. HCC patients were significantly older than those with chronic liver disease (median 54.5 years vs 52 years; P=0.021), and the proportion of males in HCC patients were significantly higher compared to that in CLD population (92.5% vs 75.7%, P=0.002). In addition,41.3% HCC patients were at a very early/early stage (BCLC 0/A).
Screening candidate biomarkers for model construction
To find potential biomarkers that can be used to distinguish HCC patients from non-HCC controls among Chinese population, two approaches were employed. Firstly, we applied logistic regression analysis to screen independent factors associated with HCC. After excluding one CHB patient due to incomplete data, we finally included 80 HCC, 100 LC and 39 CHB samples for logistic regression analysis, which contained 20 variables from demographic characteristics, routine blood test, liver function test, and blood clotting test (Tables 1, S1). The results demonstrated that gender, AFP, DCP, TP and TB were independent factors for HCC (Table 2).
We also compared the levels of 18 blood indicators between HCC patients and patients at high-risk for HCC (CLD group; Tables 1, S1) to screen significantly difference indicators. According to our samples, the mean values of AFP, DCP, AFP-L3, WBC, PLT, ALT, AST, ALP, GGT and ALB had significant difference between HCC and CLD groups (P<0.05). We further compared these variables between different disease subgroups (Figures 1, S1), and found that the levels of AFP, DCP, AFP-L3, AST and GGT in HCC group were significantly higher than that in LC and CHB subgroups. However, TP, RBC, HGB, ALP, DBIL, ALB, A/G, PT and INR had a similar level between HCC and LC group, and TB, WBC and ALT could not discriminate HCC from CHB group. In addition, the level of PLT in HCC group were significantly higher than that in LC group, but significantly lower than that in CHB and HC groups. These results suggested that the AFP, DCP, AFP-L3, AST and GGT biomarkers might be able to potentially discriminate HCC patients from non-HCC patients.
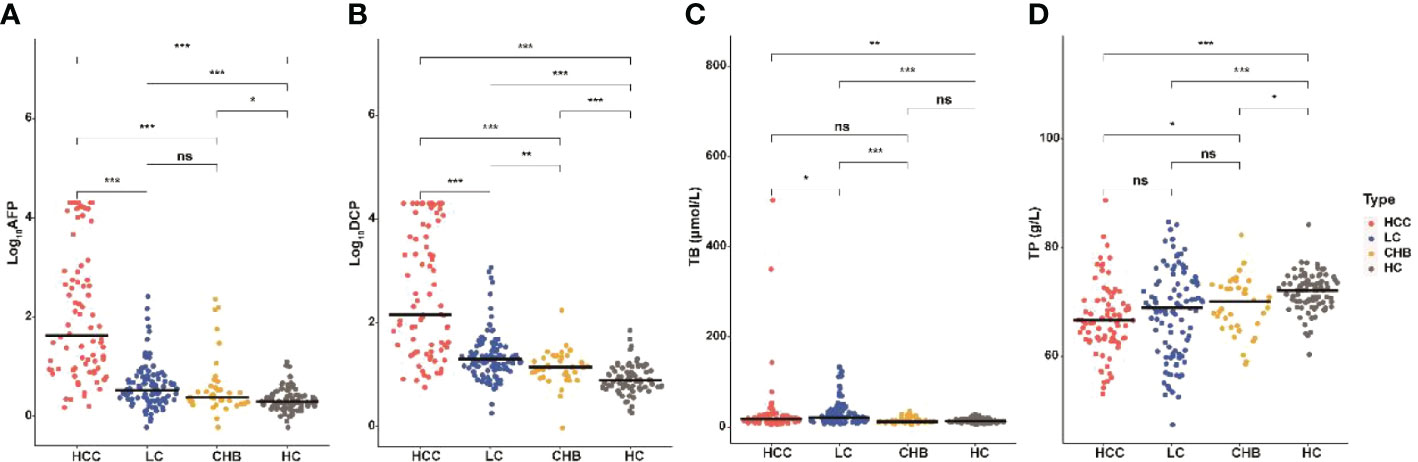
Figure 1 Serum AFP, DCP, TB and TP in HCC and non-HCC groups. Comparison of AFP (A), DCP (B), TB (C), and TP (D) among HCC, LC, CHB, and HC groups. The horizontal bar represents median values. Characteristics differences were tested using Wilcoxon test. ns P>0.05, *P <0.05, **P <0.01, ***P <0.001. HCC, hepatocellular carcinoma; LC, liver cirrhosis; CHB, chronic hepatitis B; HC, healthy controls; TP, total protein; TB, total bilirubin.
Development of a new HCC risk assessment model
In order to build a more suitable HCC diagnostic model, we referred to the ideas developed by GALAD (16). HCC and high-risk groups for HCC were used in the construction of a diagnostic model for HCC. We included above identified independent factors, gender combined with blood indicators showing significant difference between HCC and CLD groups, and all candidate biomarkers obtained from two approaches in a multivariate model to construct models 1, 2 and 3, respectively. The performance of each model is showed in Table 3. The AUC of models 1, 2 and 3 was 0.941, 0.927 and 0.94, respectively. These results suggested that, although the values of AFP-L3, AST and GGT alone were significantly different between HCC and non-HCC groups, these variables were not effective in improving the performance of HCC diagnostic models. Furthermore, we found that the age variable was filtered out by logistic regression analysis. However, numerous studies (15–17)had shown that age variable can be enrolled into models related to HCC, and “Guidelines for the diagnosis and treatment of primary liver cancer” also suggested that men more than 40 years old is also part of the high-risk group for HCC. Therefore, we enrolled the age variable into model 1 and developed a new model for HCC diagnosis, GAADPB. The performance of GAADPB (AUC=0.941, Table 3) was similar to model 1 and slightly higher than GALAD (P= 0.0545).
Subgroup analysis of model performance
We analyzed the performance of GAADPB for differentiating HCC from different disease subgroups (training set) and health controls in our sample, and the results are shown in Figure 2 and Table 4. The AUC of GAADPB for differentiating HCC from LC was 0.939, which was significantly higher than that of GALAD (AUCLC=0.913; P=0.01), AFP (AUCLC=0.874; P=0.001) and DCP (AUCLC=0.801; P <0.001). In addition, the AUC of GAADPB for differentiating HCC from CHB and HC subgroups was 0.946 and 0.991, respectively, which was similar to the performance of GALAD (AUCCHB=0.954, P=0.453; AUCHC=0.991, P= 0.857) and significantly higher than that of AFP (AUCCHB= 0.879, P=0.003; AUCHC=0.953, P=0.005) and DCP (AUCCHB=0.871, P=0.009; AUCHC=0.94, P=0.001). Collectively, the performance of GAADPB for different subgroups was significantly better than that of a single protein biomarker, and it was more suitable than GALAD for distinguishing HCC from LC patients and similar to GALAD for distinguishing HCC from CHB and HC.
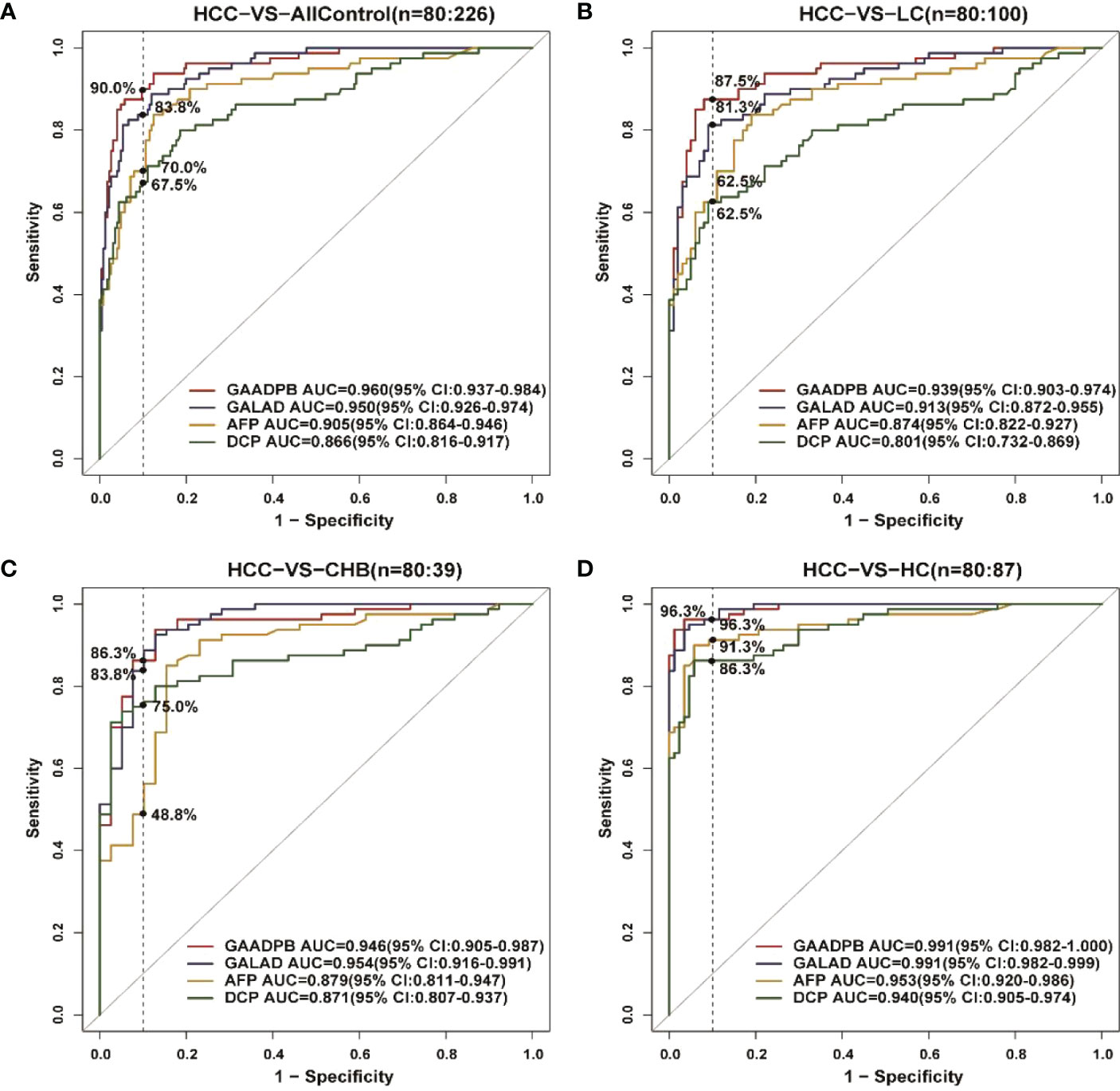
Figure 2 ROC curves comparing performance among GAADPB, GALAD and individual protein biomarkers for discriminating HCC from different disease subgroups and healthy controls. (A), HCC from all control (B), HCC from LC (C), HCC form CHB (D), HCC form HC. The vertical line represents specificity at 90%. HCC, hepatocellular carcinoma; LC, liver cirrhosis; CHB, chronic hepatitis B; HC, healthy controls.
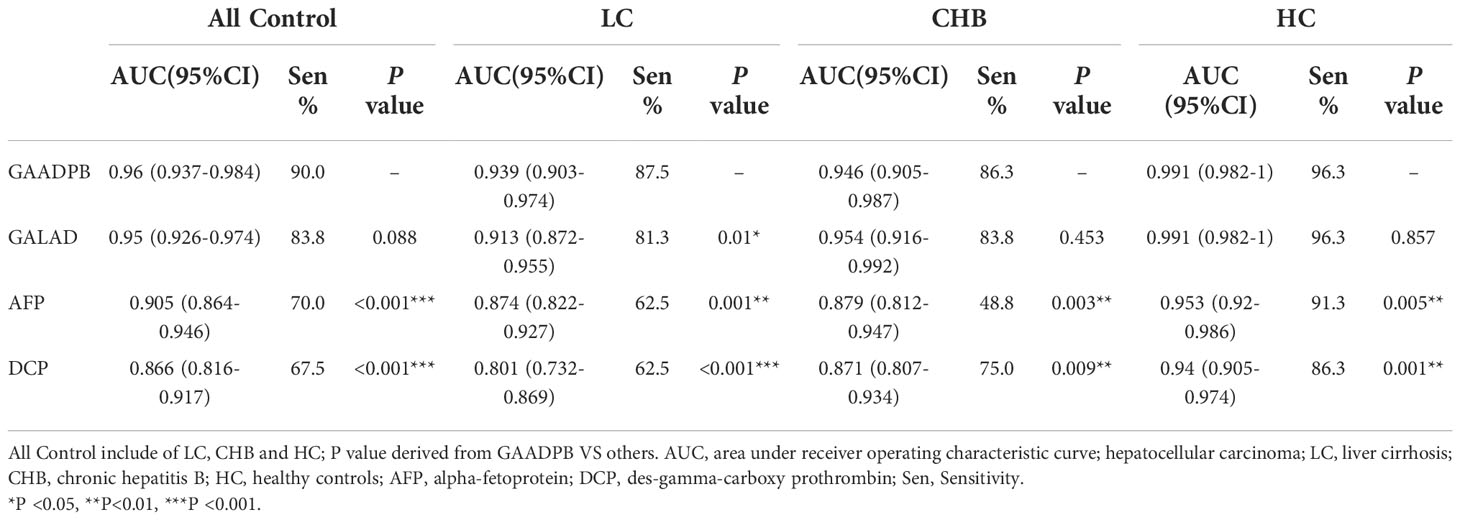
Table 4 Comparison between GAADPB, GALAD and the individual biomarkers within disease subgroups (Specificity=90%).
In addition, we analyzed the performance of GAADPB in different cancer subgroups in training set. GAADPB performed significantly better for distinguishing HCC than individual protein biomarkers in many HCC subgroups and was almost identical to GALAD (Figure 3 and Table S3). As a diagnostic model, it is necessary to maximize the detection rate of the model under the premise of avoiding excessive medical treatment. Therefore, we compared the sensitivity of GAADPB and GALAD at a specificity of 90%. The results showed that GAADPB appeared more sensitive than GALAD for detecting different HCC subgroups with very early/early stage (BCLC 0/A), small size (diameter < 3 cm), single lesion, absent PPVT, absent metastases, AFP-negative (20 ng/ml) and DCP-negative (<40 ng/ml), especially in (very) early-stage (BCLC 0/A), small size (diameter< 3 cm) and AFP-negative HCC, which improved its sensitivity by 12.1%, 12.5%, and 12.5% than GALAD, respectively. These results suggested that GAADPB performed even better in detecting HCC compared to GALAD in a more subtle situation.
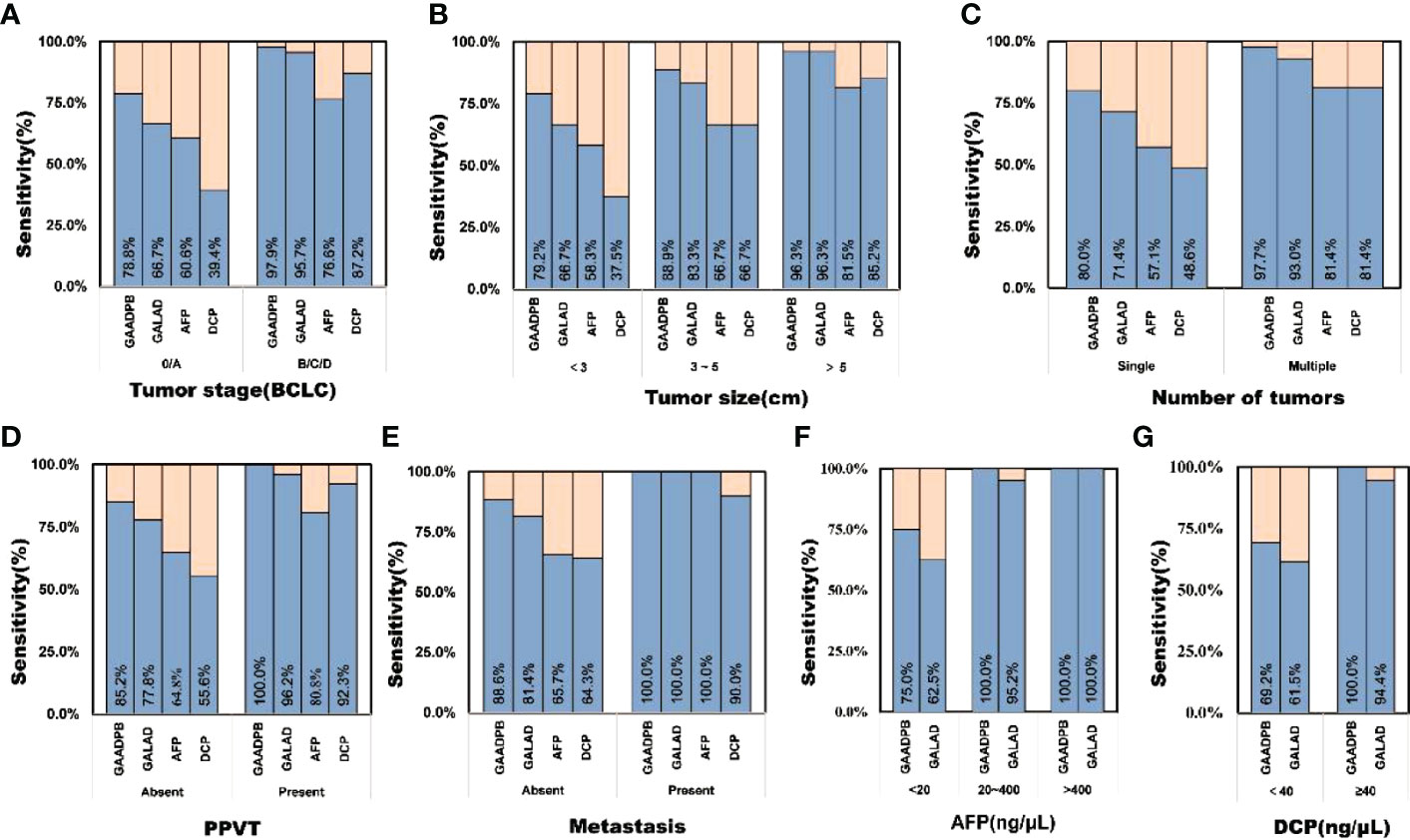
Figure 3 Sensitivity comparison of GAADPB and GALAD at 90% specificity in different cancer subgroups. (A), Tumor stage (BCLC) (B), Tumor size (C), Number of tumors (D), PPVT (E), Metastasis (F), AFP (G), DCP. HCC, hepatocellular carcinoma; LC, liver cirrhosis; CHB, chronic hepatitis B; HC, healthy controls, PPVT, portal vein tumor thrombus.
Performances of GAADPB in testing set
To evaluate the stability of GAADPB in distinguishing HCC from high-risk population. We included an independent testing set for model validation. The information for demographic data and clinical characteristics of the participants in the testing set are presented in Table 5. In the testing set, the AUC of GAADPB was 0.896 for distinguishing HCC from CLD patients, and the sensitivity was 76.7% at a specificity of 90% (Figure 4). The preformation for different disease subgroups is showed in Figure 4. The AUC of GAADPB was 0.889 and 0.928 for distinguishing HCC from LC and CHB, respectively. When the specificity was 90%, the sensitivity was 74.7% in both LC and CHB subgroups. Furthermore, we assessed GAADPB performance for distinguishing HCC from different cancer subgroups, the results revealed that at a 90% specificity, GAADPB still had the highest sensitivity compared to the individual biomarkers for detecting HCC subgroups with very early/early stage (BCLC 0/A), small size (diameter < 3 cm), single lesion, absent PPVT, absent metastases, AFP-negative (20 ng/ml) and DCP-negative (<40 ng/ml)] (Figure 5 and Table S4). These results indicated that GAADPB model was a stable and robust diagnostic tool for differentiating HCC from high-risk population.
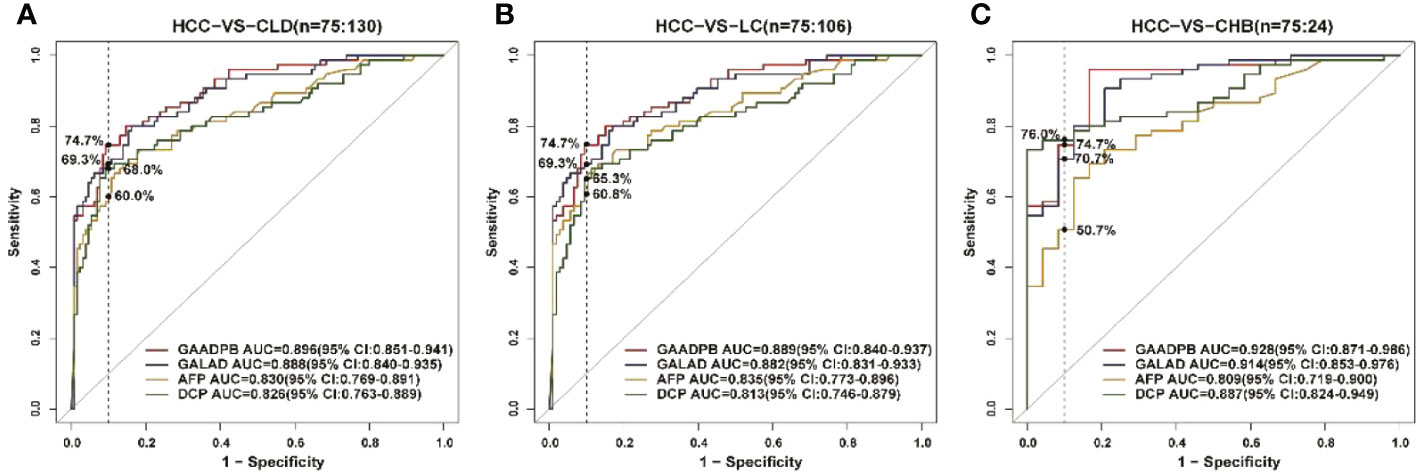
Figure 4 ROC curve analysis of GAADPB in different disease subgroups of the test set. (A), HCC from CLD (B), HCC from LC (C), HCC form CHB. The vertical line represented specificity was at 90%. HCC, hepatocellular carcinoma; LC, liver cirrhosis; CHB, chronic hepatitis B; HC, healthy controls.
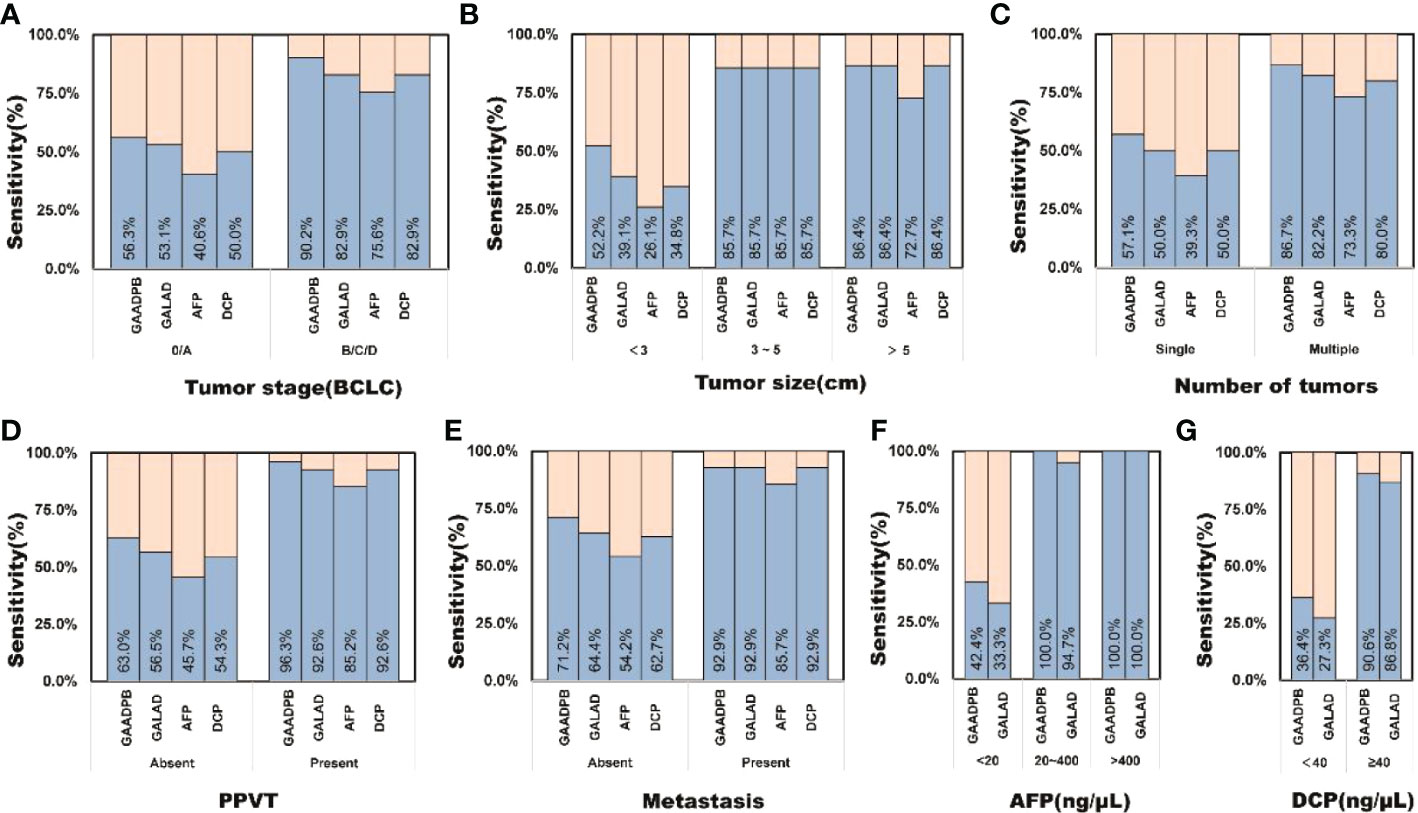
Figure 5 The sensitivity of GAADPB in different cancer subgroups of test set. (A), Tumor stage (BCLC) (B), Tumor size (C), Number of tumors (D), PPVT (E), Metastasis (F), AFP (G), DCP. HCC, hepatocellular carcinoma; LC, liver cirrhosis; CHB, chronic hepatitis B; HC, healthy controls, PPVT, portal vein tumor thrombus.
Discussion
About 350 million people are infected with HBV globally, and the lifetime risk of developing HCC among HBV carriers ranges from 10% to 25% (18). Most HCC cases in China are related to HBV infection, which is the same in our study population (19). Currently, the methods based on ultrasound and AFP are not sensitive enough to detect early HCC, so a more effective, objective and accurate Chinese population monitoring method is in need.
Several HCC risk predictions scoring systems had been developed for estimating the risk of HCC development from CHB, diagnose the ability of HCC from CLD, stage HCC and so on (11–13). Since they were all HCC-related models, we wanted to determine whether all these models could be used for the diagnosis of HCC. Therefore, we evaluated and compared the performance of these five models as well as three protein biomarkers for the diagnosis of HCC in our training sample. Our results showed that GALAD had the highest accuracy for HCC detection, with an area under the receiver operating characteristic curve (AUC) of 0.925 (Table 6). In addition, combined detection of AFP, AFP-L3 and DCP (AUC=0.91) and GAAP (AUC=0.89) could also better distinguish the HCC patients from the non-HCC population. However, BALAD-2 and aMAP could not predict HCC with a relative high performance, even with significantly lower AUC than individual protein markers (AUCAFP=0.876, PBALAD-2 VS AFP <0.001, PaMAP VS AFP <0.001), which may be related to the scenario of model development (15, 17).
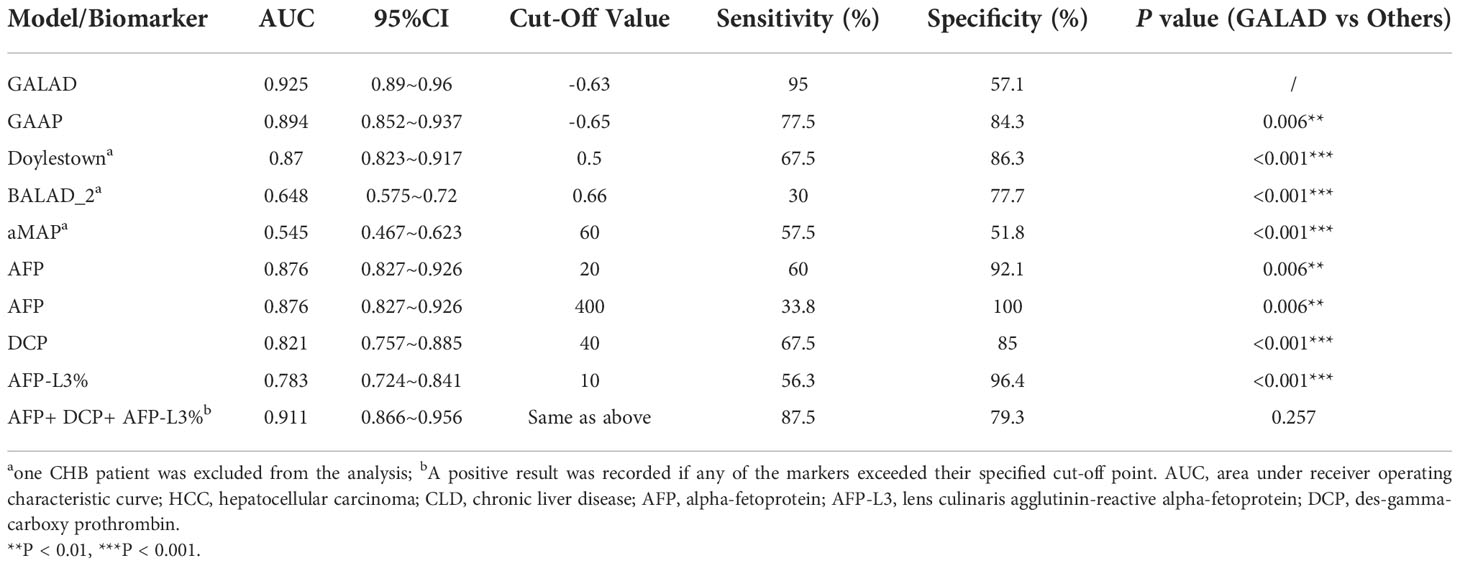
Table 6 ROC curve analysis of HCC prediction models and serum biomarkers for discriminating HCC (n=80) and CLD groups (n=140).
In order to build a more suitable HCC diagnostic model, we referred to the ideas developed by GALAD and constructed a new model for HCC diagnosis, the GAADPB model (11). Differing from GALAD, in our multivariate logistic regression analysis, total protein (TP) and total bilirubin (TB) are independent factors associated with the developing of HCC, they reflect the synthetic function and the underlying liver function and they are also included in the BALAD-2 and aMAP (11, 12). We excluded AFP-L3, because the contribution of AFP-L3 was small in our study, and the GAAP study had the same results (12). The age variable was filtered out by logistic regression analysis, but according to previous reported studies, the age variable is associated with the incidence of HCC and it is included in many models (11, 13, 15). Therefore, we included the age variable in our model. The performance of GAADPB (AUC=0.941) was better than GALAD (AUC=0.925), GAAP (AUC=0.894) and other models according to our training set. And in the validation set, the performance of GAADPB (AUC=0.896) was also better than the GALAD model (AUC=0.888) and single serum marker. We also assessed GAADPB performance for distinguishing HCC from different cancer subgroups, the results revealed that at 90% specificity, GAADPB still had the highest sensitivity compared to the individual biomarkers and GALAD to detect HCC subgroups. These results suggested that GAADPB model is a stable and robust diagnostic tool to distinguish HCC from high-risk groups.
Despite the poor performance of some models in our training set, these models have shown excellent results in predicting prognosis of HCC. A study based on 3700 HCC patients from Japan and the UK showed that patients with different BALAD scores might have a different prognosis. Patients with lower BALAD scores tended to show worse prognostic features (20). Similarly, a multicenter study by Berhane et al. demonstrated that the BALAD-2 model could, to some extent, predict the survival months in HCC patients (8). Besides, the aMAP score had been shown to be strongly related to HCC development in patients with chronic hepatitis, and late recurrence after radiofrequency ablation of HBV-related HCC patients (21). In our current study, the GAADPB model had a higher AUC in the diagnosis of liver cancer compared to BALAD-2 and aMAP, and GAADPB introduced indicators of liver injury such as TB compared to GALAD, which implied the performance of GAADPB in the areas of liver cancer progression, recurrence and prognosis could be promising in the future.
Early diagnosis of HCC for detecting HCC has been a research hotspot recently. Lots of new technologies such as multitarget HCC blood test (mt-HBT) and liquid biopsies were used to improve early cancer detection, but at the same time they come at a higher cost (22). Our results suggested that GAADPB model had higher sensitivity compared to the individual biomarkers (Figure 3), when we were detecting HCC subgroups with very early/early stage (BCLC 0/A), small size (diameter < 3 cm), single lesion, absent PPVT, absent metastases, AFP-negative (20 ng/ml) and DCP-negative (<40 ng/ml). And the performance was confirmed to be stable in the testing set. Therefore, when it comes to early stage HCC that are difficult to diagnose by imaging and traditional biomarkers, GAADPB might be an economic method of auxiliary diagnosis.
This study had some limitations. First of all, in our training set, age did not show a significant correlation with HCC occurrence, but previous reports had demonstrated a correlation between age and HCC occurrence, which may be related to the relatively insufficient sample size compared to the number of independent variables in the multivariate analyses (11, 13, 15). We plan to increase the sample size and make further validation and correction of the GAADPB model to improve the reliability of our findings in future. Besides, this is a retrospective design-based study with data from a single medical center. And the diagnostic performance of DCP was higher than that of Doylestown in the validation set (AUC of DCP: 0.884, 95% CI: 0.841-0.927; Doylestown: 0.845, 95% CI: 0.794-0.896; P <.05). Our further study will focus on validating the GAADPB model based on the real-world HBV-caused HCC in Southeast China.
Conclusions
In sum, the performance of GALAD in discriminating HCC in CLD people of China was excellent. Our GAADPB model, which also enrolled TB and TP, had better performance than GALAD, especially in detecting early stage HCC. GAADPB was also promising in predicting the prognosis of HCC patients. Further study is needed to proving the function of our GAADPB model in Chinese patients.
Data availability statement
The original contributions presented in the study are included in the article/Supplementary Material. Further inquiries can be directed to the corresponding author.
Ethics statement
The studies involving human participants were reviewed and approved by Shulan Hangzhou Hospital. Written informed consent for participation was not required for this study in accordance with the national legislation and the institutional requirements.
Author contributions
All authors listed have made a substantial, direct, and intellectual contribution to the work, and approved it for publication.
Funding
This study received funding from Research Project of Jinan Microecological Biomedicine Shandong Laboratory, the National Key R&D Program of China (2021YFA1301104), the opening foundation of the State Key Laboratory for Diagnosis and Treatment of Infectious Diseases, The First Affiliated Hospital, Zhejiang University School of Medicine (SKLID2022KF03), and the Medical and Health Technology Program of Zhejiang Province (2020RC032). The funder was not involved in the study design, collection, analysis, interpretation of data, the writing of this article or the decision to submit it for publication.
Conflict of interest
Authors JW, JZ and XK were employed by company Oriomics Biotech Inc.
The remaining authors declare that the research was conducted in the absence of any commercial or financial relationships that could be construed as a potential conflict of interest.
The handling editor JC declared a shared parent affiliation with the authors LL, HS, and YC at the time of the review.
Publisher’s note
All claims expressed in this article are solely those of the authors and do not necessarily represent those of their affiliated organizations, or those of the publisher, the editors and the reviewers. Any product that may be evaluated in this article, or claim that may be made by its manufacturer, is not guaranteed or endorsed by the publisher.
Supplementary material
The Supplementary Material for this article can be found online at: https://www.frontiersin.org/articles/10.3389/fonc.2022.1037742/full#supplementary-material
References
1. Sung H, Ferlay J, Siegel RL, Laversanne M, Soerjomataram I, Jemal A, et al. Global cancer statistics 2020: GLOBOCAN estimates of incidence and mortality worldwide for 36 cancers in 185 countries. CA: A Cancer J Clin (2021) 71(3):209–49. doi: 10.3322/caac.21660
2. Villanueva A. Hepatocellular carcinoma. N Engl J Med (2019) 380(15):1450–62. doi: 10.1056/NEJMra1713263
3. Xi L, Zhu J, Zhang H, Muktiali M, Xu C, Wu A. Epidemiological trends in gastrointestinal cancers in China: An ecological study. Dig Dis Sci (2019) 64(2):532–43. doi: 10.1007/s10620-018-5335-6
4. Tzartzeva K, Obi J, Rich NE, Parikh ND, Marrero JA, Yopp A, et al. Surveillance imaging and alpha fetoprotein for early detection of hepatocellular carcinoma in patients with cirrhosis: A meta-analysis. Gastroenterology (2018) 154(6):1706–1718.e1. doi: 10.1053/j.gastro.2018.01.064
5. Yang JD, Addissie BD, Mara KC, Harmsen WS, Dai J, Zhang N, et al. GALAD score for hepatocellular carcinoma detection in comparison with liver ultrasound and proposal of GALADUS score. Cancer Epidemiol Biomarkers Prev (2019) 28(3):531–8. doi: 10.1158/1055-9965.EPI-18-0281
6. Forner A, Reig M, Bruix J. Hepatocellular carcinoma. Lancet (2018) 391(10127):1301–14. doi: 10.1016/S0140-6736(18)30010-2
7. Kokudo N, Takemura N, Hasegawa K, Takayama T, Kubo S, Shimada M, et al. Clinical practice guidelines for hepatocellular carcinoma: The Japan society of hepatology 2017 (4th JSH-HCC guidelines) 2019 update. Hepatol Res (2019) 49(10):1109–13. doi: 10.1111/hepr.13411
8. Berhane S, Toyoda H, Tada T, Kumada T, Kagebayashi C, Satomura S, et al. Role of the GALAD and BALAD-2 serologic models in diagnosis of hepatocellular carcinoma and prediction of survival in patients. Clin Gastroenterol Hepatol (2016) 14(6):875–886.e6. doi: 10.1016/j.cgh.2015.12.042
9. Best J, Bechmann LP, Sowa JP, Sydor S, Dechene A, Pflanz K, et al. GALAD score detects early hepatocellular carcinoma in an international cohort of patients with nonalcoholic steatohepatitis. Clin Gastroenterol Hepatol (2020) 18(3):728–735.e4. doi: 10.1016/j.cgh.2019.11.012
10. Singal AG, Tayob N, Mehta A, Marrero JA, El-Serag H, Jin Q, et al. GALAD demonstrates high sensitivity for HCC surveillance in a cohort of patients with cirrhosis. Hepatology (2022) 75(3):541–9. doi: 10.1002/hep.32185
11. Johnson PJ, Pirrie SJ, Cox TF, Berhane S, Teng M, Palmer D, et al. The detection of hepatocellular carcinoma using a prospectively developed and validated model based on serological biomarkers. Cancer Epidemiol Biomarkers Prev (2014) 23(1):144–53. doi: 10.1158/1055-9965.EPI-13-0870
12. Liu M, Wu R, Liu X, Xu H, Chi X, Wang X, et al. Validation of the GALAD model and establishment of GAAP model for diagnosis of hepatocellular carcinoma in Chinese patients. J Hepatocell Carcinoma (2020) 7:219–32. doi: 10.2147/JHC.S271790
13. Wang M, Devarajan K, Singal AG, Marrero JA, Dai J, Feng Z, et al. The doylestown algorithm: A test to improve the performance of AFP in the detection of hepatocellular carcinoma. Cancer Prev Res (Phila) (2016) 9(2):172–9. doi: 10.1158/1940-6207.CAPR-15-0186
14. Toyoda H, Kumada T, Osaki Y, Oka H, Urano F, Kudo M, et al. Staging hepatocellular carcinoma by a novel scoring system (BALAD score) based on serum markers. Clin Gastroenterol Hepatol (2006) 4(12):1528–36. doi: 10.1016/j.cgh.2006.09.021
15. Fan R, Papatheodoridis G, Sun J, Innes H, Toyoda H, Xie Q, et al. aMAP risk score predicts hepatocellular carcinoma development in patients with chronic hepatitis. J Hepatol (2020) 73(6):1368–78. doi: 10.1016/j.jhep.2020.07.025
16. Chalasani NP, Ramasubramanian TS, Bhattacharya A, Olson MC, Edwards VD, Roberts LR, et al. A novel blood-based panel of methylated DNA and protein markers for detection of early-stage hepatocellular carcinoma. Clin Gastroenterol Hepatol (2021) 19(12):2597–2605.e4. doi: 10.1016/j.cgh.2020.08.065
17. Wongjarupong N, Negron-Ocasio GM, Chaiteerakij R, Addissie BD, Mohamed EA, Mara KC, et al. Model combining pre-transplant tumor biomarkers and tumor size shows more utility in predicting hepatocellular carcinoma recurrence and survival than the BALAD models. World J Gastroenterol (2018) 24(12):1321–31. doi: 10.3748/wjg.v24.i12.1321
18. McGlynn KA, Petrick JL, London WT. Global epidemiology of hepatocellular carcinoma: An emphasis on demographic and regional variability. Clin Liver Dis (2015) 19(2):223–38. doi: 10.1016/j.cld.2015.01.001
19. Caines A, Selim R, Salgia R. The changing global epidemiology of hepatocellular carcinoma. Clin Liver Dis (2020) 24(4):535–47. doi: 10.1016/j.cld.2020.06.001
20. Fox R, Berhane S, Teng M, Cox T, Tada T, Toyoda H, et al. Biomarker-based prognosis in hepatocellular carcinoma: Validation and extension of the BALAD model. Br J Cancer (2014) 110(8):2090–8. doi: 10.1038/bjc.2014.130
21. Xin Y, Zhang X, Yang Y, Chen Y, Wang Y, Zhou X, et al. Prediction of late recurrence after radiofrequency ablation of HBV-related hepatocellular carcinoma with the age-male-albumin-bilirubin-platelets (aMAP) risk score: a multicenter study. J Gastrointest Oncol (2021) 12(6):2930–42. doi: 10.21037/jgo-21-506
Keywords: hepatocellular carcinoma, alpha-fetoprotein, DCP, GALAD, GAADPB
Citation: Chen P, Song H, Xu W, Guo J, Wang J, Zhou J, Kang X, Jin C, Cai Y, Feng Z, Gao H, Lu F and Li L (2022) Validation of the GALAD model and establishment of a new model for HCC detection in Chinese patients. Front. Oncol. 12:1037742. doi: 10.3389/fonc.2022.1037742
Received: 06 September 2022; Accepted: 24 November 2022;
Published: 23 December 2022.
Edited by:
Jiang Chen, Zhejiang University, ChinaReviewed by:
Fei Peng, Baylor College of Medicine, United StatesYanhong Zhang, Brigham and Women’s Hospital and Harvard Medical School, United States
Kangsheng Tu, The First Affiliated Hospital of Xi’an Jiaotong University, China
Copyright © 2022 Chen, Song, Xu, Guo, Wang, Zhou, Kang, Jin, Cai, Feng, Gao, Lu and Li. This is an open-access article distributed under the terms of the Creative Commons Attribution License (CC BY). The use, distribution or reproduction in other forums is permitted, provided the original author(s) and the copyright owner(s) are credited and that the original publication in this journal is cited, in accordance with accepted academic practice. No use, distribution or reproduction is permitted which does not comply with these terms.
*Correspondence: Lanjuan Li, bGpsaUB6anUuZWR1LmNu; Fengmin Lu, bHUuZmVuZ21pbkBoc2MucGt1LmVkdS5jbg==
†These authors have contributed equally to this work