- 1Department of Biomedical Engineering, Case Western Reserve University, Cleveland, OH, United States
- 2GE Global Research, Niskayuna, NY, United States
- 3Department of Urology, University Hospitals Cleveland Medical Center, Cleveland, OH, United States
- 4Department of Population and Quantitative Health Sciences, Case Western Reserve University, Cleveland, OH, United States
- 5Department of Radiology, University Hospitals Cleveland Medical Center, Cleveland, OH, United States
- 6Department of Abdominal Imaging and Nuclear Radiology, Imaging Institute, Cleveland Clinic, Cleveland, OH, United States
Objective: To derive and evaluate the association of prostate shape distension descriptors from T2-weighted MRI (T2WI) with prostate cancer (PCa) biochemical recurrence (BCR) post-radical prostatectomy (RP) independently and in conjunction with texture radiomics of PCa.
Methods: This retrospective study comprised 133 PCa patients from two institutions who underwent 3T-MRI prior to RP and were followed up with PSA measurements for ≥3 years. A 3D shape atlas-based approach was adopted to derive prostate shape distension descriptors from T2WI, and these descriptors were used to train a random forest classifier (CS) to predict BCR. Texture radiomics was derived within PCa regions of interest from T2WI and ADC maps, and another machine learning classifier (CR) was trained for BCR. An integrated classifier CS+R was then trained using predictions from CS and CR. These models were trained on D1 (N = 71, 27 BCR+) and evaluated on independent hold-out set D2 (N = 62, 12 BCR+). CS+R was compared against pre-RP, post-RP clinical variables, and extant nomograms for BCR-free survival (bFS) at 3 years.
Results: CS+R resulted in a higher AUC (0.75) compared to CR (0.70, p = 0.04) and CS (0.69, p = 0.01) on D2 in predicting BCR. On univariable analysis, CS+R achieved a higher hazard ratio (2.89, 95% CI 0.35–12.81, p < 0.01) compared to other pre-RP clinical variables for bFS. CS+R, pathologic Gleason grade, extraprostatic extension, and positive surgical margins were associated with bFS (p < 0.05). CS+R resulted in a higher C-index (0.76 ± 0.06) compared to CAPRA (0.69 ± 0.09, p < 0.01) and Decipher risk (0.59 ± 0.06, p < 0.01); however, it was comparable to post-RP CAPRA-S (0.75 ± 0.02, p = 0.07).
Conclusions: Radiomic shape descriptors quantifying prostate surface distension complement texture radiomics of prostate cancer on MRI and result in an improved association with biochemical recurrence post-radical prostatectomy.
1 Introduction
An estimated 30%–35% of prostate cancer (PCa) patients experience biochemical recurrence (BCR) within 10 years post-radical prostatectomy (RP) (1). The occurrence of BCR is often found to be associated with metastasis (2) and PCa-specific mortality (3). Several predictors of BCR have been presented including pre-treatment CAPRA (4), post-surgical CAPRA-S (5), and Decipher risk (6). However, these models use invasive or post-treatment factors, are site dependent, and do not exclusively capture tumor heterogeneity and morphology. Non-invasive pre-treatment image-based prediction of BCR-free survival (bFS) may allow for treatment intensification or closer surveillance (7).
Multiparametric magnetic resonance imaging (mpMRI) is now increasingly used for PCa detection, staging, and prediction of the risk of BCR (8–17). Radiomic texture features provide an alternative representation for characterizing tumor heterogeneity and have been shown to improve PCa risk characterization (18–21) and also prognosticate BCR (22–24). However, they are susceptible to scanner variations, acquisition protocols, image artifacts, and non-standardized image intensities (25, 26).
There is evidence to suggest that cancerous lesions tend to induce mechanical stress in the surrounding tissue (27, 28). In the prostate, stresses induced by the tumors impact neighboring benign tissue (29) and cause deformation which may in turn impact the shape of the prostate capsule. Previous studies (30, 31) explored the idea of quantifying shape distension of the prostate between more and less aggressive diseases. Rusu et al. (30) have shown that prostate shape on T2-weighted MRI (T2WI) was significantly different between patients with and without cancerous lesions. Ghose et al. (31, 32) have shown that statistically significant differences in the shape of the prostate were observed between BCR+ and BCR− patients on T2WI. This now leads us to the hypothesis that radiomic descriptors that quantify differential distension of prostate shape may be associated with BCR outcome post-RP.
In this work, we present a new approach to quantify prostate distension in terms of radiomic shape descriptors from pre-treatment T2WI using a 3D shape atlas-based method. We seek to evaluate the association of these shape descriptors with BCR post-RP at 3 years. Since radiomic texture features of PCa from pre-treatment T2WI and apparent diffusion coefficient (ADC) maps have already been shown to be associated with BCR (17, 22–24), we evaluate the combination of prostate shape and tumor texture radiomics for their association with bFS. Additionally, we also sought to evaluate whether radiomic shape descriptors, which are less dependent on MRI intensities, are possibly more robust and resilient to scanner- and site-specific variations that could more substantially impact texture-based descriptors (25, 26). This was done by evaluating our approach using data from multiple cohorts acquired from two different sites. We also compared our integrated shape and texture radiomics approach against routine clinical variables and extant nomograms for predicting bFS.
2 Materials and Methods
2.1 Patient Selection and Data Characteristics
This retrospective study included patients from two different institutions (I1 and I2), compliant with HIPAA regulations and approved by the local IRB, with a waiver of informed consent. A total of 263 patients from three cohorts (C1–C3) were identified with biopsy-confirmed PCa who underwent 3T mpMRI prior to RP. Patient records between 2009 and 2017 were reviewed and included in the study if they 1) underwent RP without additional therapies, 2) followed up for at least 3 years and had documented BCR (BCR+) defined as two consecutive readings of prostate-specific antigen (PSA) ≥0.2 ng/ml post-RP [18] or no BCR (BCR−), and 3) had axial T2WI and ADC maps without acquisition artifacts. N = 133 studies identified through this process were partitioned into a training set D1 and independent hold-out validation set D2 as illustrated in Figure 1. After RP, all patients underwent periodic follow-up according to established clinical protocol (3–6 months in the first year and 6–12 months in the following years). BCR− patients were censored at their last follow-up date for survival analysis.
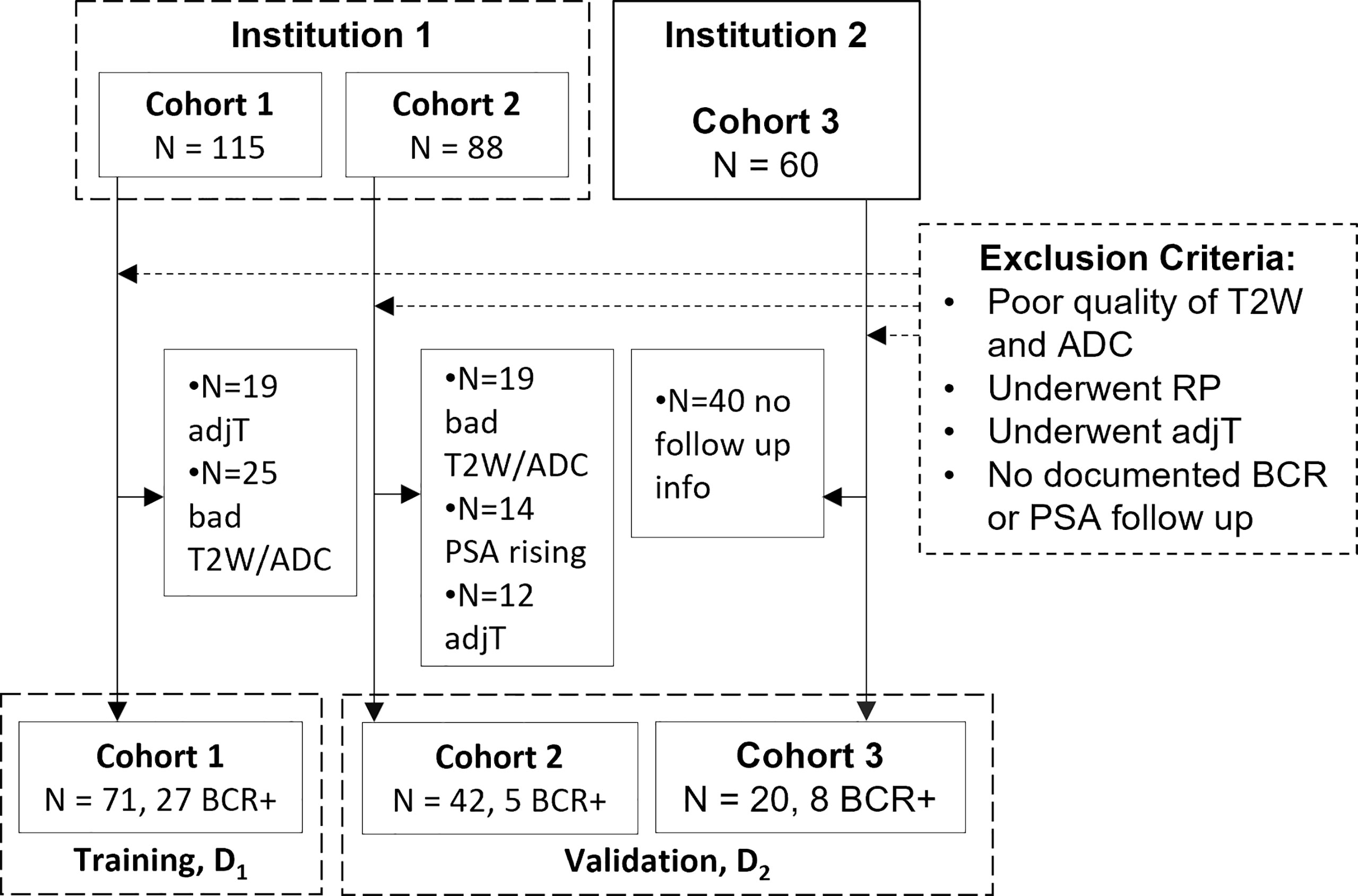
Figure 1 Flowchart of patient selection from two different institutions. adjT, adjuvant or neoadjuvant therapy; RP, radical prostatectomy; BCR, biochemical recurrence; PSA, prostate-specific antigen.
Biparametric MRI protocol was used in this study that included T2WI (in-plane: 0.3 mm, slice thickness: 3 mm, TR/TE: 3,802–5,151/105–115 ms) and DWI (1.4 mm, 3 mm, 3,751–4,880/50–74 ms, b-values: 0–2,000 s/mm2) along with ADC maps acquired via an endo-rectal coil (Achieva, Philips, Best, Netherlands) in C1, T2WI (0.5 mm, 3 mm, 3,730/121 ms) and DWI (1.6 mm, 3 mm, 4,700/86 ms, 0–1,500 s/mm2) acquired via a pelvic-phased array coil (PPAC) (Skyra, Siemens, Erlangen, Germany) in C2, and T2WI (0.6 mm, 3 mm, 7,200/96 ms) and DWI (1.2 mm, 3 mm, 7,900/88 ms, 0–1,400 s/mm2) via PPAC (Skyra, Siemens, Erlangen, Germany). Additional sequences, such as T1-weighted, dynamic contrast-enhanced (DCE), were acquired but not analyzed in the current study. Detailed dataset characteristics are provided in Table 1 and imaging parameters in Table 2.
2.2 Image Pre-Processing and Tumor Segmentation
A board-certified radiologist (10 years of experience in prostate imaging) reviewed the MRI scans in D1. They used the 3D Slicer software (33) to delineate prostate capsule and dominant PCa lesions on T2WI using histopathology template reports from RP as reference. Prostate and lesion delineation in D2 was performed by two board-certified radiologists (8 and 10 years of experience in prostate imaging) in a similar manner and assigned PI-RADS-v2.1 scores (34).
2.3 Methodology
The pipeline for radiomic prostate shape and lesion texture, along with their combination to evaluate the association with BCR, is illustrated in Figure 2. The term surface of interest (SOI) is used in the context of shape descriptors to describe a region on the 3D surface, while the region of interest (ROI) is used in the context of PCa lesions on 2D MRI slices.
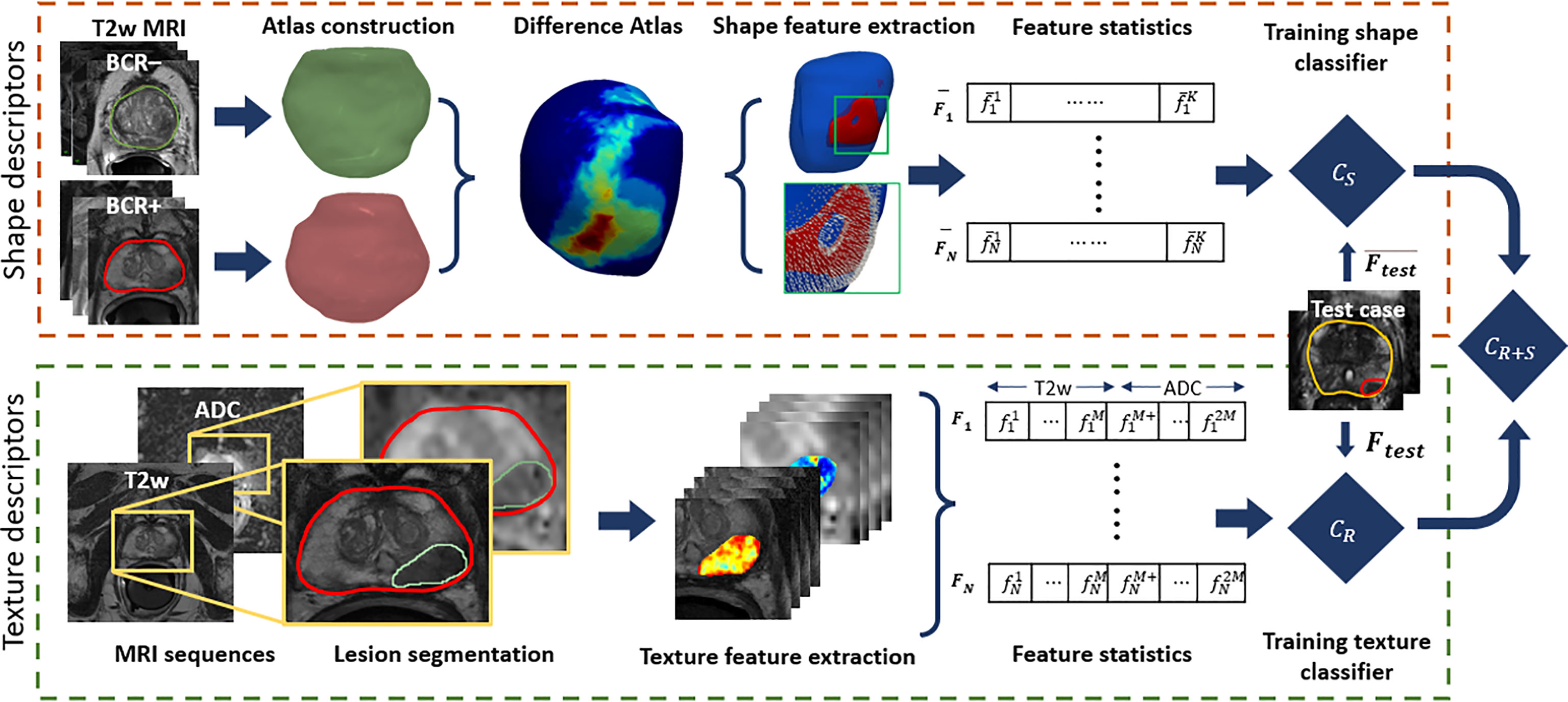
Figure 2 Illustration of the radiomic shape and lesion texture descriptors pipeline used in this study. The shape descriptors are computed from a surface of interest determined from a 3D differential shape atlas between BCR+ and BCR− cohorts on T2WI. These are used to train a model for predicting BCR-free survival and integrated with another model trained using lesion texture descriptors derived from T2WI and ADC maps.
2.3.1 Computation of a Consensus Surface of Interest
Prostate segmentations on T2WI within D1 were co-registered via rigid registration followed by a deformable registration to create a 3D shape atlas for the BCR+ and BCR− cohorts. A spatially contextual SOI of the prostate capsule was uniquely identified from statistically significant shape differences between BCR+ and BCR− atlases as described earlier by Ghose et al. (31) (details in the Supplementary Material). To minimize the effect of the choice of template in establishing the SOI, the entire process was repeated N times, each time with a different set of BCR+ and BCR− atlases generating an SOIi (where I = 1, 2, 3… N). Here, N was chosen as 27, equal to the number of patients in BCR+ class of D1. The individual SOIi was again co-registered to a common frame of reference using a rigid transformation. Next, a consensus SOI (SOIC) was computed as a mean of all the SOIi and the mean volume was binarized at a threshold of 0.5.
2.3.2 Prostate Shape Distension Features
Differential distension of the prostate capsule for each patient is quantified using the magnitudes of Gaussian curvature (κ) of the surface and orientation of the surface normal (θ, ϕ) at vertices of the prostate mesh. The Gaussian curvature measures the intrinsic degree of curvedness of a surface, and positive values indicate a greater differential expansion at the center, while negative values indicate expansion at the edges (35). Surface normal quantifies the local orientation of the surface. These features (κ, θ, and ϕ) are meaningful when extracted within the SOIC that comprises those vertices where statistically significant shape differences between BCR+ and BCR− prostates were observed. Further, the SOIC was cropped to include the mid-gland region alone after mapping it to individual prostate meshes to minimize the effect of inter-reader variations in prostate segmentation at the apex and base. The SOIC overlaid onto a BCR+ and a BCR− prostate is illustrated in Figure 3. Surface normal orientation (θ, ϕ) provides direction of the prostate distension and surface mean curvature (κ) quantifies local shape deformation, and these were extracted for every vertex on the mapped SOIC. For each patient, at each vertex, a vector of κ, θ, and ϕ was derived and a set of four statistical measures, namely, mean, standard deviation, skewness, and kurtosis, were computed resulting in 4 × 3 = 12 radiomic shape descriptors per patient.
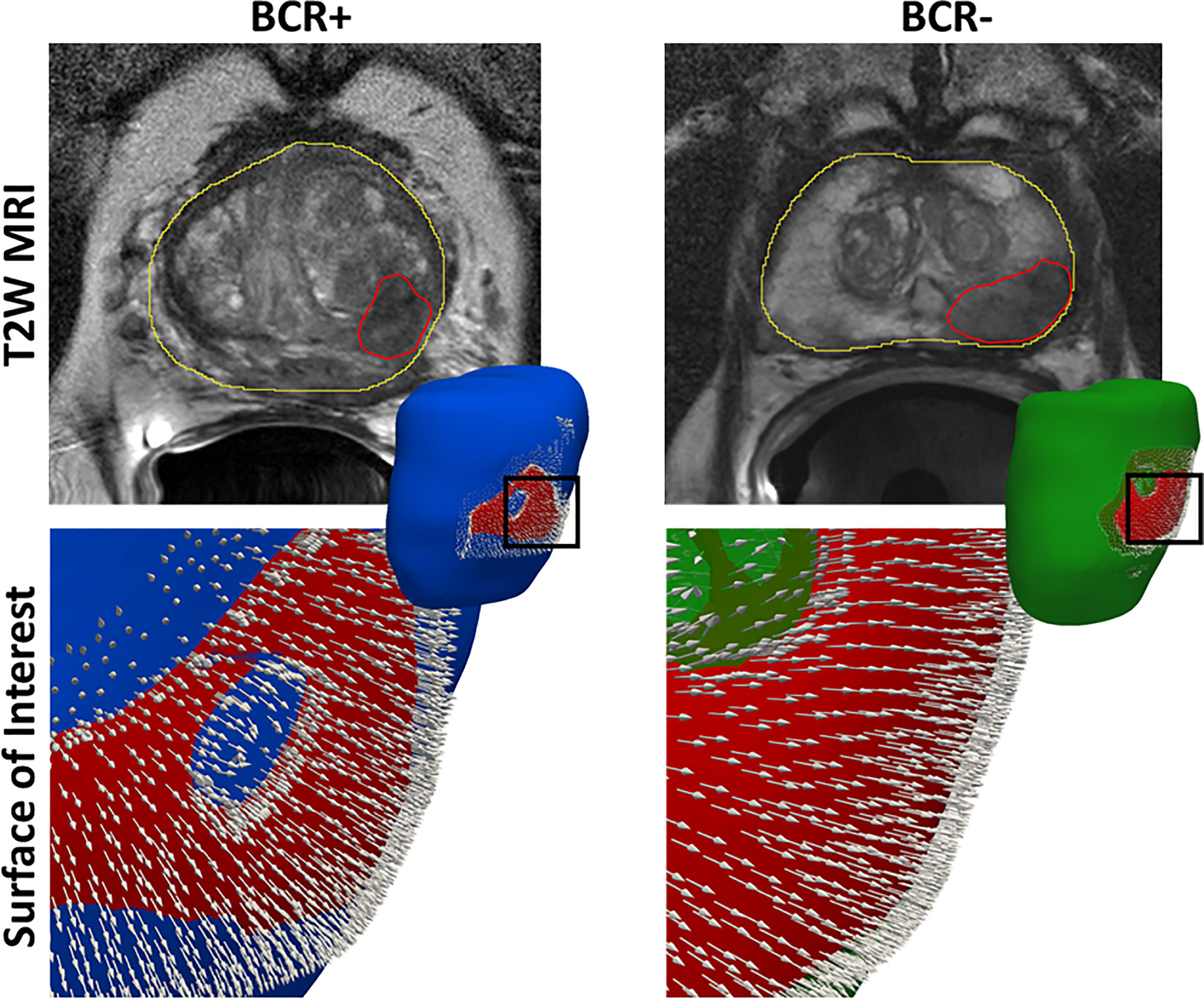
Figure 3 The surface of interest (SOIC) in red overlaid onto the prostate mesh of a BCR+ patient and a BCR− patient. We observe stronger surface distension in terms of more dense and divergent normal features in the BCR+ patient compared to the BCR− patient.
2.3.3 Radiomic Texture Features of Prostate Cancer
A set of 75 radiomic features were extracted on a per-voxel basis from each of the standardized T2WI and ADC maps within the radiologist-delineated PCa ROIs. These include first- and second-order statistics, Gabor (36), Laws (37), Haralick (38), and CoLlAGe (39) features. These features characterize the underlying tissue heterogeneity and have previously been shown to be prognostic of BCR (17, 22–24). Four statistics, namely, mean, standard deviation, skewness, and kurtosis, were calculated for voxel-wise radiomic feature within each PCa ROI resulting in 75 × 2 × 4 = 600 radiomic texture descriptors per patient. Strongly correlated features and unstable features were eliminated, and mRMR feature selection (40) was employed to identify a subset of features associated with BCR (details in the Supplementary Material).
2.4 Statistical Analysis and Comparison
A random forest machine learning classifier (CS) with 50 trees and 5 as the maximum tree depth was trained using prostate distension features from D1 to predict the binary outcome of BCR. The random forest classifier was chosen to ensure that parametric assumptions are not made for the distribution of shape distension features. A texture radiomics classifier (CR) was trained in a similar manner as CS.
A linear regression model (CS+R) was trained on predictions from CS and CR using patients from D1. The trained models (CS, CR, CS+R) were evaluated using patients from the independent validation test set D2. Kruskal–Wallis test was employed to assess differences in radiomic shape and texture features between the cohorts without a parametric assumption. Youden’s index was used to determine the optimal operating point within receiver-operating characteristics (ROC) analysis to convert the estimated posterior class probabilities to dichotomous labels (BCR+ and BCR−). DeLong’s test was used to compare the AUCs between the classification models.
Univariable and multivariable analyses of CS+R were conducted along with pre-RP clinical parameters including age, PI-RADS-v2, PSA, and biopsy Gleason grade (bGG) on D1. Post-RP pathological Gleason grade (pGG), extraprostatic extension (EPE), seminal vesical invasion (SVI), and positive surgical margins (PSM) were compared on patients from D2. A penalized Cox proportional hazards (CPH) regression model was used for this purpose given the class imbalance in D2. The model CS+R was also compared with other nomograms including CAPRA and CAPRA-S and the genomic assay Decipher on subsets of patients for whom sufficient clinical variables were available to estimate BCR-free survival on D2. Wald test was used to determine statistical significance with p-values under 0.05, and the concordance index (C-index) and hazard ratios (HRs) were computed. Kaplan–Meier survival curves were estimated to determine the differences in BCR-free survival based on predictions of CS+R and comparative nomograms. The log-rank test was used to determine statistical significance (p < 0.05).
3 Results
3.1 Evaluation of Shape and Texture Radiomics for Their Association With BCR
The top radiomic shape and tumor texture descriptors based on their random forest Gini feature importance in the training set D1 are listed in Table 3. Shape descriptors were consistent between the sites (p > 0.05), whereas all radiomic texture features except Haralick had significant variations (p < 0.05). Shape descriptors from the entire prostate mesh resulted in a lower AUC of 0.58 ± 0.08, p < 0.01, compared to using those from the SOIC. Also, shape features from the SOIC within the mid-gland region improved AUCs compared to those from the entire SOIC (AUC = 0.75 vs. 0.78, p = 0.04), and the mid-gland SOIC was used in all subsequent experiments. CS trained using individual SOIs resulted in AUC = 0.64 ± 0.08 on D1 in distinguishing BCR+ and BCR−, while the consensus SOIC resulted in improved performance (AUC = 0.78, p < 0.01) (Figure 4). On the hold-out validation set D2, the AUC using models trained from individual SOIs was 0.67 ± 0.12, while that from the SOIC was 0.69 (p = 0.02). Inter-reader variations in shape descriptors were evaluated on D2 and no significant differences were observed (p > 0.05) (Supplementary Figure 2).
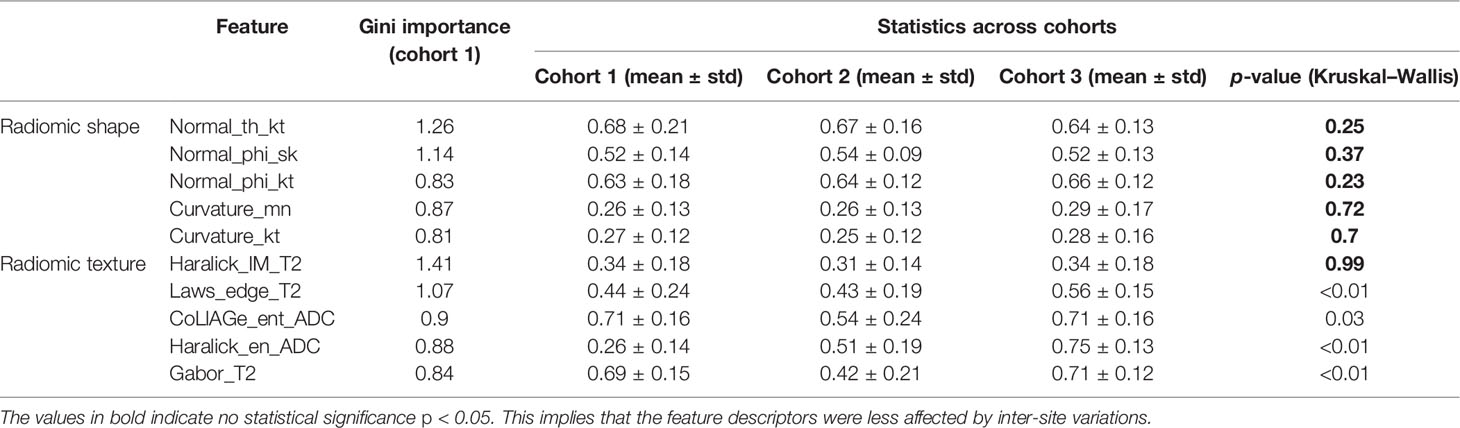
Table 3 Variations in the top 5-ranked radiomic shape and texture descriptors according to Gini importance between the cohorts.
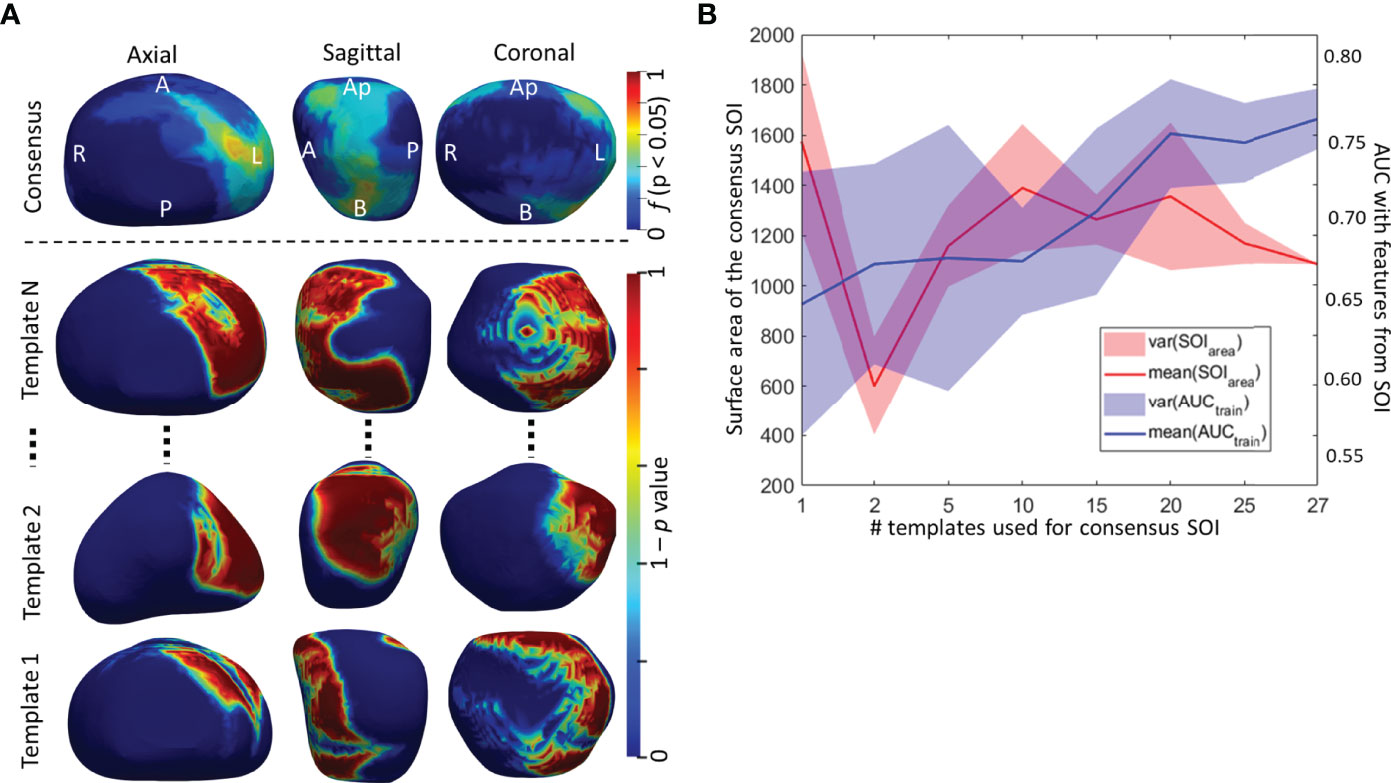
Figure 4 (A) Surface of interest (SOI) determined from individual templates and a consensus SOIC derived by averaging individual SOIs. (B) The surface area and AUC from the predictive model trained radiomic shape descriptors as a function of the number of SOIs used in building the consensus SOIC.
The BCR prediction model (CR) using radiomic texture descriptors of the PCa lesion resulted in a cross-validation AUC = 0.76 ± 0.09 on D1 and AUC = 0.70 on D2. The integrated model (CR+S) resulted in a significantly higher (p < 0.05) AUC both on D1 (0.85 ± 0.08) and D2 (0.75) compared to both CS and CR, respectively. The AUC, sensitivity, and specificity of the models CS, CR, and CR+S for predicting BCR status are summarized in Table 4.

Table 4 Classification performance of prostate distension, lesion texture, and integrated classifiers for predicting biochemical recurrence-free survival.
3.2 Comparison With Clinical Parameters and Extant Nomograms for Predicting bFS at 3 Years
On univariable analysis for predicting bFS, CS+R predictions resulted in the highest HR of 2.91 (95% CI 1.45–11.51, p = 0.02) on D1 compared to other pre-treatment clinical variables including age, PSA, bGG, and PI-RADS-v2. On multivariable analysis, CS+R and bGG showed independent predictive value (p < 0.05) for bFS (Table 5). Post-RP Gleason grade (pGG), EPE, and PSM had higher HRs of 2.63 (95% CI 1.16–5.93, p = 0.01), 2.51 (95% CI 1.06–11.26, p = 0.04), and 2.86 (95% CI 1.32–30.26, p = 0.03) on D2. The C-index followed the same trend with CS+R achieving the highest (0.76, p = 0.03) among pre-treatment clinical variables; however, it was lower than pGG (0.82, p = 0.01) (Table 5).
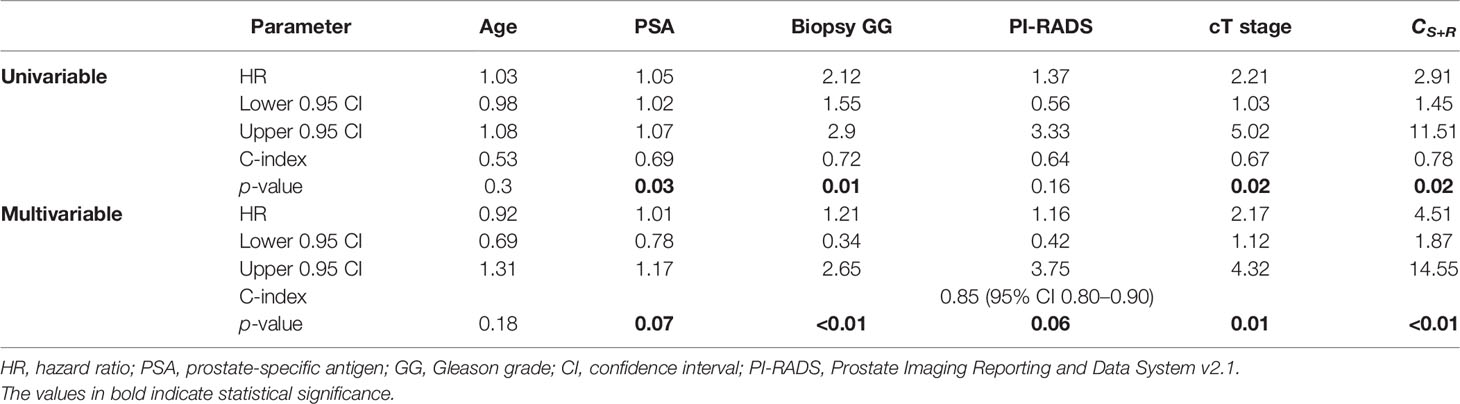
Table 5 Univariable and multivariable analyses for predicting BCR-free survival with pre-surgical variables (N = 71, NBCR+ = 27, NBCR− = 44).
On univariable comparison with extant assays for predicting bFS, CS+R resulted in higher HR (2.11, 95% CI 0.35–11.33, p = 0.03) compared to pre-treatment CAPRA (1.8, 95% CI 1.1–3.56, p = 0.02) and post-RP Decipher risk (1.41, 95% CI 0.61–2.45, p = 0.18); however, post-RP CAPRA-S resulted in comparable HR (2.12, 95% CI 1.2–5.72, p = 0.03). Integrating preoperative clinical variables with CS+R resulted in improved (p < 0.05) C-index (0.82, 95% CI 0.70–0.90) compared to CAPRA-S (0.75, 95% CI 0.69–0.78).
The C-indices also follow the same trend with CS+R and CAPRA-S showing comparable results, and CAPRA and Decipher risk have lower values (Table 6). CS+R and CAPRA-S predictions resulted in significant separation in bFS (p < 0.05), while CAPRA and Decipher risk showed no significant separation on D2 (Figure 5).
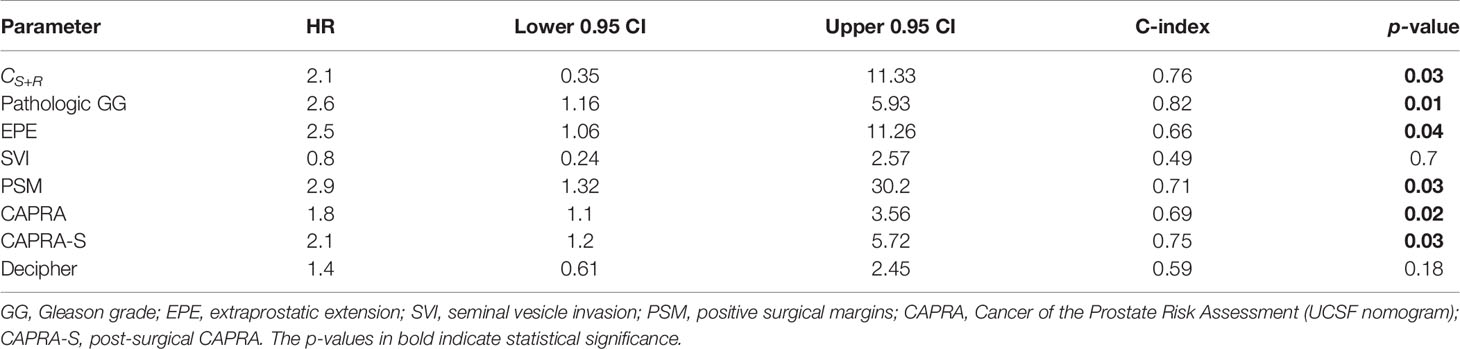
Table 6 Comparison of the integrated radiomic model CS+R with post-surgical variables, nomograms CAPRA and CAPRA-S, and Decipher risk scores on the validation set (N = 62, NBCR+ = 13, NBCR− = 49).
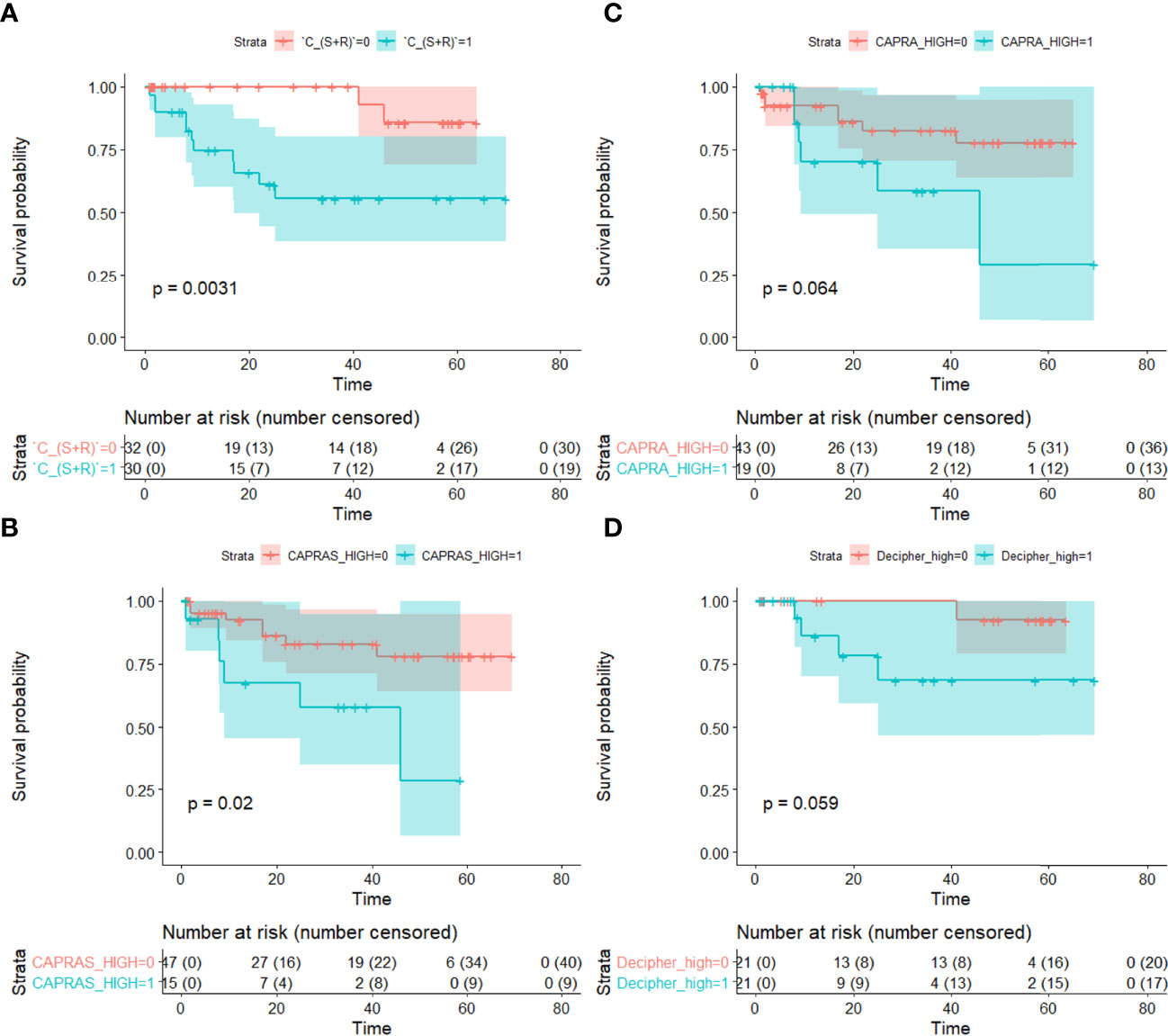
Figure 5 Kaplan–Meier survival curves showing differences in biochemical recurrence-free survival between BCR+ and BCR− patients based on predictions from (A) the integrated texture and shape classifier (CS+R), (B) CAPRA-S, (C) CAPRA and (D) Decipher risk. Statistically significant separation in BCR-free survival was observed with CS+R and CAPRA-S (p < 0.05). Statistical significance (p < 0.05) was established using the log-rank test.
4 Discussion
In this study, we presented prostate shape distension descriptors derived from a 3D shape atlas of the prostate on T2WI and explored their association with PCa BCR post-RP. We observed that the shape descriptors were prognostic of BCR and, in conjunction with radiomic texture features of PCa on T2WI and ADC maps, resulted in an improved BCR prediction model. Since shape descriptors are based on prostate segmentations, they are relatively robust to scanner variations and acquisition parameters compared to texture features. We also validated our approach on hold-out datasets that were acquired from multiple institutions validating robustness and generalizability.
Previously presented radiomic methods (17, 23, 41, 42) for predicting BCR post-RP exclusively focused on MRI texture. Our study is different from these approaches wherein we explored the shape distension of the prostate capsule within a surface of interest as a biomarker for predicting BCR. Patients experiencing BCR tend to have a relatively more aggressive phenotype of PCa (43), and hence, it appears to deform the prostate surface more substantially compared to more indolent cancers that do not result in BCR. Differential distension of the prostate capsule was observed within an SOI located toward the left posterior region, similar to the results reported by Rusu et al. (30) and Ghose et al. (31). We observed that BCR+ patients had more variations in the surface normal orientation arising out of a higher degree of prostate distension compared to BCR− patients (Figure 3). Prostate capsular bulge on MRI was found to be a predictor of pathologic EPE after RP by Martini et al. (44). Pathologic EPE has been shown to be a predictor of BCR in several studies (45, 46) and was observed in our study as well. Radiomic texture features including Haralick, Laws, and CoLlAGe features from T2WI and ADC sequences were the top-ranking features that were used in building CR. These features were also observed to be associated with BCR in previous works by Gnep et al. (22), Shiradkar et al. (23), and Li et al. (17).
We observed that the shape descriptors were largely consistent between the sites (p > 0.05). In terms of radiomic texture descriptors, other than the Haralick feature from PCa on T2W, all the texture features had significant variations between the cohorts (p < 0.05) (Figure 6; Table 3). Several previous studies (26, 47, 48) exploring intersite variations in radiomic texture features have reported texture features to be sensitive to site and scanner variations. Chirra et al. (26) have shown in the context of distinguishing PCa from normal regions that Haralick features remained relatively stable while Law’s features showed significant variations which is consistent with our observations. Since radiomic shape descriptors used in our study are based on the shape of the prostate, they remain largely insensitive to site- and scanner-specific variations. This was also observed in a previous study by Merisaari et al. (49) where morphology- and shape-based radiomics were stable in terms of test–retest repeatability compared to texture features.
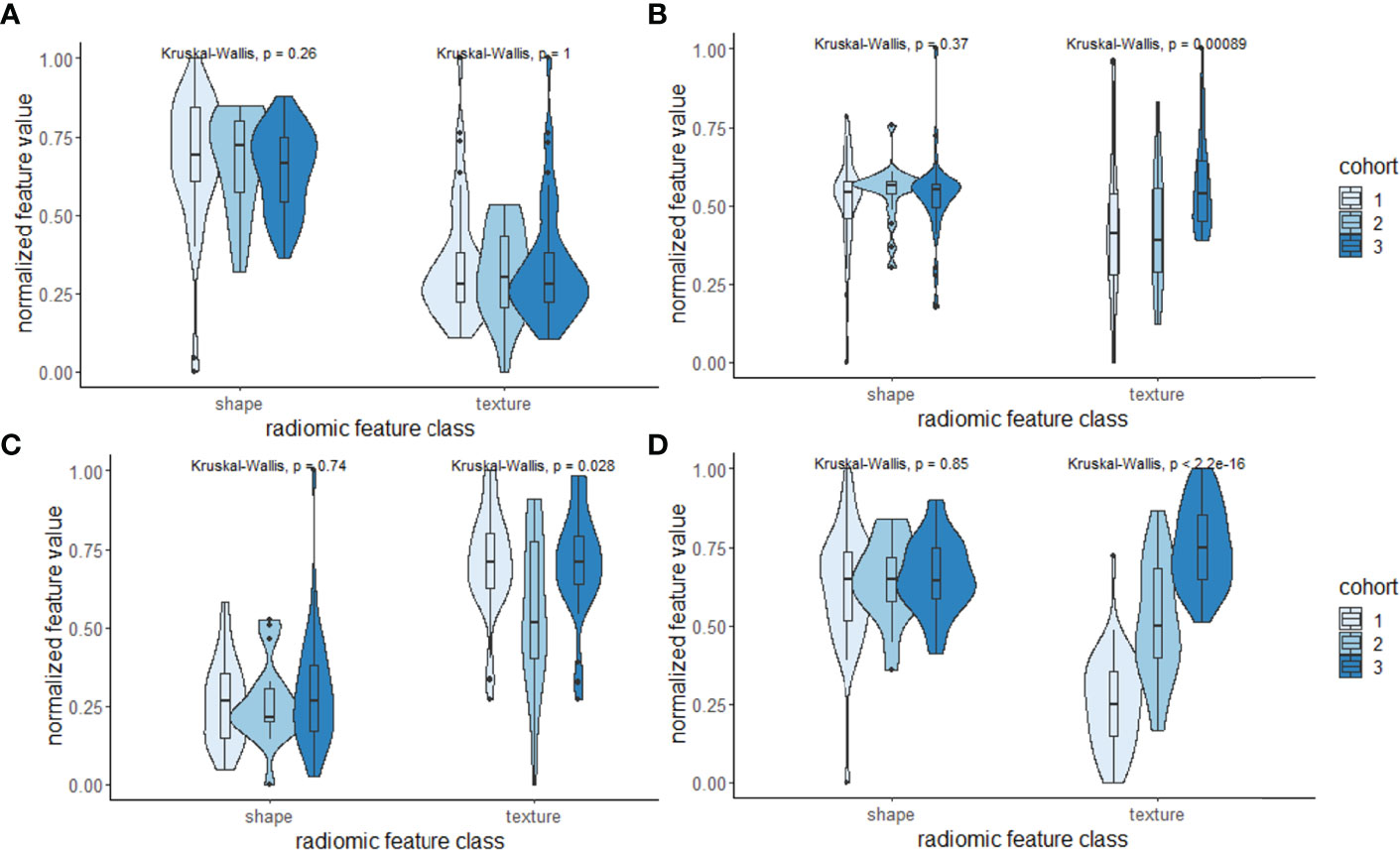
Figure 6 (A–D) Violin and box plots of the top-ranked radiomic shape and texture descriptors according to the Gini importance score within the three cohorts used in this study. Shape descriptors tend to be largely consistent and less sensitive to variations across sites compared to texture descriptors.
We observed that when compared to pre-treatment clinical variables including age, PSA, biopsy Gleason grade group (bGG), clinical T stage, or PI-RADS, CS+R predictions resulted in the highest HR and C-index in predicting bFS. We observed that the integrated classifier CS+R was independently prognostic of bFS compared to the other pre-treatment clinical variables and resulted in a high C-index in combination with those clinical variables. Post-RP Gleason grade group and EPE were however predictive of bFS on both univariable and multivariable analyses, also reported in previous studies (50, 51). The performance of the BCR predictive nomograms CAPRA and CAPRA-S in our study was in line with the results reported in previous large-scale validation studies (4). CAPRA score is derived from pre-treatment clinical variables and our radiomic shape and texture classifier CS+R was superior to CAPRA in predicting bFS. CAPRA-S score which includes the post-RP Gleason score resulted in a comparable performance to CS+R which includes pre-treatment parameters alone. On a subset of studies with the availability of Decipher in our study, we found no significant association between Decipher risk score and BCR. This was also observed in a previous study where Decipher down-classified a significant number of patients who experienced BCR (52).
We acknowledge that the study did have its limitations. Firstly, we did not explicitly control for the location of prostate cancer lesions and benign lesions and the presence of extraprostatic extension to explore their effect on prostate distension. Future studies need to be performed, involving controlling for the location of lesions in peripheral transition zones and for the size of lesions. Next, we explored inter-reader variations in prostate segmentation using only two readers and limited the SOI to the mid-gland region. Nevertheless, we observed that the shape features were robust to inter-reader and site-specific variations. We will continue to work on building a statistical model based on multireader segmentations to identify regions, where a high degree of confidence in prostate segmentation can be achieved, more precisely for subsequent shape feature analysis. We also had a smaller sample size in training and validating our approach, and our validation set had a significant imbalance between the BCR+ and BCR− classes. However, our results still generalized well over the hold-out validation set that was acquired from two different institutions. There were differences in the resolution of T2WI and ADC maps and differences in b-values of DWI sequences for generating ADC between the cohorts which were not explicitly accounted for in our study. We will explicitly account for the sensitivity of shape and texture radiomics to variations in resolution in our future study. We also aim to control for positive surgical margins and explore their effect on shape radiomic features in our future work.
In conclusion, radiomic shape descriptors of the prostate capsule derived from T2WI were found to be associated with BCR in our study. In combination with radiomic texture features of prostate cancer lesion from T2WI and ADC sequences, radiomic shape distension features resulted in a better predictor of BCR. Following large-scale validation studies, this approach could potentially be applied to pre-treatment prostate MRI scans at the clinic to provide clinicians with a decision support tool for assessing the risk of BCR, in turn allowing them to make better decisions for treatment management.
Data Availability Statement
The data analyzed in this study are subject to the following licenses/restrictions: The dataset is under the IRB regulations between Case Western Reserve University, Cleveland Clinic, and the University Hospitals Cleveland Medical Center. Requests to access these datasets should be directed to rxs558@case.edu.
Author Contributions
RS contributed to the experimental design, data acquisition, analysis, software, writing, and funding. SG provided software and contributed to the analysis. AmM helped with the data acquisition. LL provided software. IH contributed to the analysis and experiments. PF contributed to the statistical analysis. ST and AP contributed to the imaging interpretation, experimental design, and imaging analysis. LP contributed to the data acquisition, funding, and clinical interpretation. AnM contributed to the conception of the idea, experimental design, supervision, writing, and funding. All authors contributed to the article and approved the submitted version.
Funding
The research reported in this publication was supported by the National Cancer Institute under award numbers 1U24CA199374-01, R01CA249992-01A1, R01CA202752-01A1, R01CA208236-01A1, R01CA216579-01A1, R01CA220581-01A1, R01CA257612-01A1, 1U01CA239055-01, 1U01CA248226-01, and 1U54CA254566-01; the National Heart, Lung and Blood Institute (1R01HL15127701A1); the National Institute of Biomedical Imaging and Bioengineering (1R43EB028736-01); the National Center for Research Resources under award number 1 C06 RR12463-01; VA Merit Review Award IBX004121A from the United States Department of Veterans Affairs Biomedical Laboratory Research and Development Service; the Office of the Assistant Secretary of Defense for Health Affairs, through the Breast Cancer Research Program (W81XWH-19-1-0668); the Prostate Cancer Research Program (W81XWH-15-1-0558, W81XWH-20-1-0851); the Lung Cancer Research Program (W81XWH-18-1-0440, W81XWH-20-1-0595); the Peer-Reviewed Cancer Research Program (W81XWH-18-1-0404); the Kidney Precision Medicine Project (KPMP) Glue Grant; the Ohio Third Frontier Technology Validation Fund; the Clinical and Translational Science Collaborative of Cleveland (UL1TR0002548) from the National Center for Advancing Translational Sciences (NCATS) component of the National Institutes of Health and NIH Roadmap for Medical Research; The Wallace H. Coulter Foundation Program in the Department of Biomedical Engineering at Case Western Reserve University; the DoD Prostate Cancer Research Program Idea Development Award W81XWH-18-1-0524; and the Clinical and Translational Science Collaborative (CTSC) Cleveland Annual Pilot Award 2020 UL1TR002548. This study also had sponsored research agreements from Bristol Myers-Squibb, Boehringer-Ingelheim, and AstraZeneca.
Author Disclaimer
The content is solely the responsibility of the authors and does not necessarily represent the official views of the National Institutes of Health, the U.S. Department of Veterans Affairs, the Department of Defense, or the United States Government.
Conflict of Interest
AnM is an equity holder in Elucid Bioimaging and Inspirata Inc. He is also a scientific advisory consultant for Inspirata Inc. In addition, he has served as a scientific advisory board member for Inspirata Inc., AstraZeneca, Bristol Meyers-Squibb, and Merck. He also has sponsored research agreements with Philips and Inspirata Inc. His technology has been licensed to Elucid Bioimaging and Inspirata Inc. He is also involved in an NIH U24 grant with PathCore Inc. and three different R01 grants with Inspirata Inc.
The remaining authors declare that the research was conducted in the absence of any commercial or financial relationships that could be construed as a potential conflict of interest.
Publisher’s Note
All claims expressed in this article are solely those of the authors and do not necessarily represent those of their affiliated organizations, or those of the publisher, the editors and the reviewers. Any product that may be evaluated in this article, or claim that may be made by its manufacturer, is not guaranteed or endorsed by the publisher.
Supplementary Material
The Supplementary Material for this article can be found online at: https://www.frontiersin.org/articles/10.3389/fonc.2022.841801/full#supplementary-material
References
1. Boorjian SA, Thompson RH, Tollefson MK, Rangel LJ, Bergstralh EJ, Blute ML, et al. Long-Term Risk of Clinical Progression After Biochemical Recurrence Following Radical Prostatectomy: The Impact of Time From Surgery to Recurrence. Eur Urol (2011) 59(6):893–9. doi: 10.1016/j.eururo.2011.02.026
2. Antonarakis ES, Feng Z, Trock BJ, Humphreys EB, Carducci MA, Partin AW, et al. The Natural History of Metastatic Progression in Men With Prostate-Specific Antigen Recurrence After Radical Prostatectomy: Long-Term Follow-Up. BJU Int (2012) 109(1):32–9. doi: 10.1111/j.1464-410X.2011.10422.x
3. Freedland SJ, Humphreys EB, Mangold LA, Eisenberger M, Dorey FJ, Walsh PC, et al. Risk of Prostate Cancer-Specific Mortality Following Biochemical Recurrence After Radical Prostatectomy. JAMA (2005) 294(4):433–9. doi: 10.1001/jama.294.4.433
4. Brajtbord JS, Leapman MS, Cooperberg MR. The CAPRA Score at 10 Years: Contemporary Perspectives and Analysis of Supporting Studies. Eur Urol (2017) 71(5):705–9. doi: 10.1016/j.eururo.2016.08.065
5. Cooperberg MR, Hilton JF, Carroll PR. The CAPRA-S Score: A Straightforward Tool for Improved Prediction of Outcomes After Radical Prostatectomy. Cancer (2011) 117(22):5039–46. doi: 10.1002/cncr.26169
6. Kim HL, Li P, Huang H-C, Deheshi S, Marti T, Knudsen B, et al. Validation of the Decipher Test for Predicting Adverse Pathology in Candidates for Prostate Cancer Active Surveillance. Prostate Cancer Prostatic Dis (2019) 22(3):399–405. doi: 10.1038/s41391-018-0101-6
7. McKay RR, Berchuck J, Kwak L, Xie W, Silver R, Bubley GJ, et al. Outcomes of Post-Neoadjuvant Intense Hormone Therapy and Surgery for High Risk Localized Prostate Cancer: Results of a Pooled Analysis of Contemporary Clinical Trials. J Urol (2021) 205(6):1689–97. doi: 10.1097/JU.0000000000001632
8. Hegde JV, Mulkern RV, Panych LP, Fennessy FM, Fedorov A, Maier SE, et al. Multiparametric MRI of Prostate Cancer: An Update on State-of-the-Art Techniques and Their Performance in Detecting and Localizing Prostate Cancer. J Magn Reson Imaging (2013) 37(5):1035–54. doi: 10.1002/jmri.23860
9. Rouvière O, Vitry T, Lyonnet D. Imaging of Prostate Cancer Local Recurrences: Why and How? Eur Radiol (2010) 20(5):1254–66. doi: 10.1007/s00330-009-1647-4
10. Vargas HA, Wassberg C, Akin O, Hricak H. Mr Imaging of Treated Prostate Cancer. Radiology (2012) 262(1):26–42. doi: 10.1148/radiol.11101996
11. Panebianco V, Barchetti F, Grompone MD, Colarieti A, Salvo V, Cardone G, et al. Magnetic Resonance Imaging for Localization of Prostate Cancer in the Setting of Biochemical Recurrence. Urol Oncol (2016) 34(7):303–10. doi: 10.1016/j.urolonc.2016.01.004
12. Ho R, Siddiqui MM, George AK, Frye T, Kilchevsky A, Fascelli M, et al. Preoperative Multiparametric Magnetic Resonance Imaging Predicts Biochemical Recurrence in Prostate Cancer After Radical Prostatectomy. PloS One (2016) 11(6):e0157313. doi: 10.1371/journal.pone.0157313
13. McKenna DA, Coakley FV, Westphalen AC, Zhao S, Lu Y, Webb EM, et al. Prostate Cancer: Role of Pretreatment MR in Predicting Outcome After External-Beam Radiation Therapy—Initial Experience. Radiology (2008) 247(1):141–6. doi: 10.1148/radiol.2471061982
14. Riaz N, Afaq A, Akin O, Pei X, Kollmeier MA, Cox B, et al. Pretreatment Endorectal Coil Magnetic Resonance Imaging Findings Predict Biochemical Tumor Control in Prostate Cancer Patients Treated With Combination Brachytherapy and External-Beam Radiotherapy. Int J Radiat Oncol Biol Phys (2012) 84(3):707–11. doi: 10.1016/j.ijrobp.2012.01.009
15. Jambor I, Kähkönen E, Taimen P, Merisaari H, Saunavaara J, Alanen K, et al. Prebiopsy Multiparametric 3T Prostate MRI in Patients With Elevated PSA, Normal Digital Rectal Examination, and No Previous Biopsy. J Magn Reson Imaging (2015) 41(5):1394–404. doi: 10.1002/jmri.24682
16. Jambor I, Boström PJ, Taimen P, Syvänen K, Kähkönen E, Kallajoki M, et al. Novel Biparametric MRI and Targeted Biopsy Improves Risk Stratification in Men With a Clinical Suspicion of Prostate Cancer (IMPROD Trial). J Magn Reson Imaging (2017) 46(4):1089–95. doi: 10.1002/jmri.25641
17. Li L, Shiradkar R, Leo P, Algohary A, Fu P, Tirumani SH, et al. A Novel Imaging Based Nomogram for Predicting Post-Surgical Biochemical Recurrence and Adverse Pathology of Prostate Cancer From Pre-Operative Bi-Parametric MRI. EBioMedicine (2021) 63:103163. doi: 10.1016/j.ebiom.2020.103163
18. Litjens GJS, Barentsz JO, Karssemeijer N, Huisman HJ. Clinical Evaluation of a Computer-Aided Diagnosis System for Determining Cancer Aggressiveness in Prostate MRI. Eur Radiol (2015) 25(11):3187–99. doi: 10.1007/s00330-015-3743-y
19. Gillies RJ, Kinahan PE, Hricak H. Radiomics: Images are More Than Pictures, They Are Data. Radiology (2016) 278(2):563–77. doi: 10.1148/radiol.2015151169
20. Algohary A, Viswanath S, Shiradkar R, Ghose S, Pahwa S, Moses D, et al. Radiomic Features on MRI Enable Risk Categorization of Prostate Cancer Patients on Active Surveillance: Preliminary Findings. J Magn Reson Imaging (2018) 48(6):1626–36. doi: 10.1002/jmri.25983
21. Viswanath SE, Palumbo D, Chappelow J, Patel P, Bloch BN, Rofsky NM, et al. Empirical Evaluation of Bias Field Correction Algorithms for Computer-Aided Detection of Prostate Cancer on T2w MRI. In: Spie Medical Imaging. SPIE. Houston, TX, USA: Proceeings of the SPIE Conference on Medical Imaging (2011). p. 79630V. Available at: http://link.aip.org/link/?PSI/7963/79630V/1.
22. Gnep K, Fargeas A, Gutiérrez-Carvajal RE, Commandeur F, Mathieu R, Ospina JD, et al. Haralick Textural Features on T2 -Weighted MRI are Associated With Biochemical Recurrence Following Radiotherapy for Peripheral Zone Prostate Cancer. J Magn Reson Imaging (2016). doi: 10.1002/jmri.25335
23. Shiradkar R, Ghose S, Jambor I, Taimen P, Ettala O, Purysko AS, et al. Radiomic Features From Pretreatment Biparametric MRI Predict Prostate Cancer Biochemical Recurrence: Preliminary Findings. J Magn Reson Imaging (2018). doi: 10.1002/jmri.26584
24. Ginsburg SB, Rusu M, Kurhanewicz J, Madabhushi A. Computer Extracted Texture Features on T2w MRI to Predict Biochemical Recurrence Following Radiation Therapy for Prostate Cancer. San Diego, CA, USA: Proceedings of the SPIE Conference on Medical Imaging (2014). p. 903509. doi: 10.1117/12.2043937.
25. Palumbo D, Yee B, O’Dea P, Leedy S, Viswanath S, Madabhushi A. Interplay Between Bias Field Correction, Intensity Standardization, and Noise Filtering for T2-Weighted MRI. In: Conference Proceedings: Annual International Conference of the IEEE Engineering in Medicine and Biology Society IEEE Engineering in Medicine and Biology Society Annual Conference. Boston MA, USA: Annu Int Conf IEEE Eng Med Biol Soc (2011). pp. 5080–3.
26. Chirra P, Leo P, Yim M, Bloch BN, Rastinehad AR, Purysko A, et al. Multisite Evaluation of Radiomic Feature Reproducibility and Discriminability for Identifying Peripheral Zone Prostate Tumors on MRI. J Med Imaging (Bellingham) (2019) 6(2):024502. doi: 10.1117/1.JMI.6.2.024502
27. Gordon V. Measuring the Mechanical Stress Induced by an Expanding Multicellular Tumor System: A Case Study. Exp Cell Res (2003) 289(1):58–66. doi: 10.1016/S0014-4827(03)00256-8
28. Jain RK, Martin JD, Stylianopoulos T. The Role of Mechanical Forces in Tumor Growth and Therapy. Annu Rev BioMed Eng (2014) 16:321–46. doi: 10.1146/annurev-bioeng-071813-105259
29. Lorenzo G, Hughes TJR, Dominguez-Frojan P, Reali A, Gomez H. Computer Simulations Suggest That Prostate Enlargement Due to Benign Prostatic Hyperplasia Mechanically Impedes Prostate Cancer Growth. Proc Natl Acad Sci (2019) 116(4):1152–61. doi: 10.1073/pnas.1815735116
30. Rusu M, Purysko AS, Verma S, Kiechle J, Gollamudi J, Ghose S, et al. Computational Imaging Reveals Shape Differences Between Normal and Malignant Prostates on MRI. Sci Rep (2017) 7:41261. doi: 10.1038/srep41261
31. Ghose S, Shiradkar R, Rusu M, Mitra J, Thawani R, Feldman M, et al. Prostate Shapes on Pre-Treatment MRI Between Prostate Cancer Patients Who do and do Not Undergo Biochemical Recurrence are Different: Preliminary Findings. Sci Rep (2017) 7(1):15829. doi: 10.1038/s41598-017-13443-8
32. Ghose S, Shiradkar R, Rusu M, Mitra J, Thawani R, Feldman M, et al. Field Effect Induced Organ Distension (Forge) Features Predicting Biochemical Recurrence From Pre-treatment Prostate MRI. In: Descoteaux M, Maier-Hein L, Franz A, Jannin P, Collins DL, Duchesne S, editors. Medical Image Computing and Computer-Assisted Intervention – Miccai 2017. Cham: Springer International Publishing (2017). p. 442–9. doi: 10.1007/978-3-319-66185-8_50
33. Fedorov A, Beichel R, Kalpathy-Cramer J, Finet J, Fillion-Robin J-C, Pujol S, et al. 3D Slicer as an Image Computing Platform for the Quantitative Imaging Network. Magn Reson Imaging (2012) 30(9):1323–41. doi: 10.1016/j.mri.2012.05.001
34. Turkbey B, Choyke PL. Pirads 2.0: What is New? Diagn Interv Radiol (2015) 21(5):382–4. doi: 10.5152/dir.2015.15099
35. Deppe M, Marinell J, Krämer J, Duning T, Ruck T, Simon OJ, et al. Increased Cortical Curvature Reflects White Matter Atrophy in Individual Patients With Early Multiple Sclerosis. NeuroImage Clin (2014) 6:475–87. doi: 10.1016/j.nicl.2014.02.012
36. Jain AK, Farrokhnia F. Unsupervised Texture Segmentation Using Gabor Filters. Pattern Recognit (1991) 24(12):1167–86. doi: 10.1016/0031-3203(91)90143-S
38. Haralick RM. Statistical and Structural Approaches to Texture. Proc IEEE (1979) 67(5):786–804. doi: 10.1109/PROC.1979.11328
39. Prasanna P, Tiwari P, Madabhushi A. Co-Occurrence of Local Anisotropic Gradient Orientations (Collage): A New Radiomics Descriptor. Sci Rep (2016) 6:37241. doi: 10.1038/srep37241
40. Unler A, Murat A, Chinnam RB. Mr2PSO: A Maximum Relevance Minimum Redundancy Feature Selection Method Based on Swarm Intelligence for Support Vector Machine Classification. Inf Sci (2011) 181(20):4625–41. doi: 10.1016/j.ins.2010.05.037
41. Park SY, Oh YT, Jung DC, Cho NH, Choi YD, Rha KH, et al. Prediction of Biochemical Recurrence After Radical Prostatectomy With PI-RADS Version 2 in Prostate Cancers: Initial Results. Eur Radiol (2016) 26(8):2502–9. doi: 10.1007/s00330-015-4077-5
42. Wong NC, Lam C, Patterson L, Shayegan B. Use of Machine Learning to Predict Early Biochemical Recurrence After Robot-Assisted Prostatectomy. BJU Int (2019) 123(1):51–7. doi: 10.1111/bju.14477
43. Gandhi J, Afridi A, Vatsia S, Joshi G, Joshi G, Kaplan SA, et al. The Molecular Biology of Prostate Cancer: Current Understanding and Clinical Implications. Prostate Cancer Prostatic Dis (2018) 21(1):22–36. doi: 10.1038/s41391-017-0023-8
44. Martini A, Cumarasamy S, Gupta A, Falagario UG, Shah QN, Beksac AT, et al. Clinical Implications of Prostatic Capsular Abutment or Bulging on Multiparametric Magnetic Resonance Imaging. Minerva Urol Nefrol (2019) 71(5):502–7. doi: 10.23736/S0393-2249.19.03370-8
45. Ball MW, Partin AW, Epstein JI. Extent of Extraprostatic Extension Independently Influences Biochemical Recurrence-Free Survival: Evidence for Further pT3 Subclassification. Urology (2015) 85(1):161–4. doi: 10.1016/j.urology.2014.08.025
46. Takamatsu K, Matsumoto K, Shojo K, Tanaka N, Takeda T, Morita S, et al. The Prognostic Value of Zonal Origin and Extraprostatic Extension of Prostate Cancer for Biochemical Recurrence After Radical Prostatectomy. Urol Oncol (2019) 37(9):575.e19–575.e25. doi: 10.1016/j.urolonc.2019.03.012
47. Hagiwara A, Hori M, Cohen-Adad J, Nakazawa M, Suzuki Y, Kasahara A, et al. Linearity, Bias, Intrascanner Repeatability, and Interscanner Reproducibility of Quantitative Multidynamic Multiecho Sequence for Rapid Simultaneous Relaxometry at 3 T: A Validation Study With a Standardized Phantom and Healthy Controls. Invest Radiol (2019) 54(1):39–47. doi: 10.1097/RLI.0000000000000510
48. Hiremath A, Shiradkar R, Merisaari H, Prasanna P, Ettala O, Taimen P, et al. Test-Retest Repeatability of a Deep Learning Architecture in Detecting and Segmenting Clinically Significant Prostate Cancer on Apparent Diffusion Coefficient (ADC) Maps. Eur Radiol (2020). doi: 10.1007/s00330-020-07065-4
49. Merisaari H, Taimen P, Shiradkar R, Ettala O, Pesola M, Saunavaara J, et al. Repeatability of radiomics and machine learning for DWI: Short-term repeatability study of 112 patients with prostate cancer. Magn Reson Med (2019). doi: 10.1002/mrm.28058
50. Jambor I, Falagario U, Ratnani P, Perez IM, Demir K, Merisaari H, et al. Prediction of Biochemical Recurrence in Prostate Cancer Patients Who Underwent Prostatectomy Using Routine Clinical Prostate Multiparametric MRI and Decipher Genomic Score. J Magn Reson Imaging (2020) 51(4):1075–85. doi: 10.1002/jmri.26928
51. Roux V, Eyraud R, Brureau L, Gourtaud G, Senechal C, Fofana M, et al. [Impact of Gleason Score on Biochemical Recurrence Free Survival After Radical Prostatectomy With Positive Surgical Margins]. Prog Urol (2017) 27(8–9):467–73. doi: 10.1016/j.purol.2017.05.003
Keywords: magnetic resonance imaging, prostate cancer, retrospective studies, prostatectomy, artificial intelligence, machine learning
Citation: Shiradkar R, Ghose S, Mahran A, Li L, Hubbard I, Fu P, Tirumani SH, Ponsky L, Purysko A and Madabhushi A (2022) Prostate Surface Distension and Tumor Texture Descriptors From Pre-Treatment MRI Are Associated With Biochemical Recurrence Following Radical Prostatectomy: Preliminary Findings. Front. Oncol. 12:841801. doi: 10.3389/fonc.2022.841801
Received: 22 December 2021; Accepted: 13 April 2022;
Published: 20 May 2022.
Edited by:
Guang Yang, Imperial College London, United KingdomReviewed by:
Ugo Giovanni Falagario, University of Foggia, ItalyStefano Puliatti, University of Modena and Reggio Emilia, Italy
Copyright © 2022 Shiradkar, Ghose, Mahran, Li, Hubbard, Fu, Tirumani, Ponsky, Purysko and Madabhushi. This is an open-access article distributed under the terms of the Creative Commons Attribution License (CC BY). The use, distribution or reproduction in other forums is permitted, provided the original author(s) and the copyright owner(s) are credited and that the original publication in this journal is cited, in accordance with accepted academic practice. No use, distribution or reproduction is permitted which does not comply with these terms.
*Correspondence: Rakesh Shiradkar, rxs558@case.edu