- 1Department of Radiology, Second Affiliated Hospital of Chongqing Medical University, Chongqing, China
- 2Department of Radiology, Third Affiliated Hospital of Chongqing Medical University, Chongqing, China
- 3Department of Radiology, The Second People’s Hospital of Jiulongpo District, Chongqing, China
- 4Department of Ultrasound, Chongqing Maternal and Child Health Hospital, Chongqing, China
- 5Medical Data Science Academy, Chongqing Medical University, Chongqing, China
- 6Department of Data Analysis, GE Healthcare, Shanghai, China
- 7Department of Radiology, The First Affiliated Hospital of Army Medical University, Chongqing, China
Purpose: To investigate whether the combination of radiomics derived from brain high-resolution T1-weighted imaging and automatic machine learning could diagnose subcortical ischemic vascular cognitive impairment with no dementia (SIVCIND) accurately.
Methods: A total of 116 right-handed participants involving 40 SIVCIND patients and 76 gender-, age-, and educational experience-matched normal controls (NM) were recruited. A total of 7,106 quantitative features from the bilateral thalamus, hippocampus, globus pallidus, amygdala, nucleus accumbens, putamen, caudate nucleus, and 148 areas of the cerebral cortex were automatically calculated from each subject. Six methods including least absolute shrinkage and selection operator (LASSO) were utilized to lessen the redundancy of features. Three supervised machine learning approaches of logistic regression (LR), random forest (RF), and support vector machine (SVM) employing 5-fold cross-validation were used to train and establish diagnosis models, and 10 times 10-fold cross-validation was used to evaluate the generalization performance of each model. Correlation analysis was performed between the optimal features and the neuropsychological scores of the SIVCIND patients.
Results: Thirteen features from the right amygdala, right hippocampus, left caudate nucleus, left putamen, left thalamus, and bilateral nucleus accumbens were included in the optimal subset. Among all the three models, the RF produced the highest diagnostic performance with an area under the receiver operator characteristic curve (AUC) of 0.990 and an accuracy of 0.948. According to the correlation analysis, the radiomics features of the right amygdala, left caudate nucleus, left putamen, and left thalamus were found to be significantly correlated with the neuropsychological scores of the SIVCIND patients.
Conclusions: The combination of radiomics derived from brain high-resolution T1-weighted imaging and machine learning could diagnose SIVCIND accurately and automatically. The optimal radiomics features are mostly located in the right amygdala, left caudate nucleus, left putamen, and left thalamus, which might be new biomarkers of SIVCIND.
Introduction
Dementia is a syndrome that involves severe loss of cognitive abilities as a result of disease or injury. It is a serious threat to the elderly and a heavy burden for society. Globally, the prevalence of dementia in the elderly over 65 is estimated to be as high as 7%; besides, it can reach 8%–10% in developed countries because of longer life spans (1). It often leads to the decline of intelligence, memory, orientation, computing, and comprehension, which can be accompanied by decreased language ability and emotional and personality changes (2). Vascular dementia (VaD) is the second most common type of dementia. Subcortical ischemic vascular cognitive impairment with no dementia (SIVCIND) is considered to be a prodromal stage of subcortical ischemic VaD (SIVD), which is an important subtype of VaD (3). Early diagnosis of SIVCIND has important clinical value because timely treatment can greatly prevent disease development and improve the prognosis (4).
Until now, the clinical diagnosis of SIVCIND is mainly based on neuropsychological scale testing, electrophysiological examination, and the evidence of subcortical cerebrovascular disease from clinical data or medical imaging. However, a formal neuropsychological evaluation is often time-consuming and lacks objectivity (5), while electrophysiological examination usually lacks specificity (6), which limits their clinical use greatly. In recent years, neuroimaging utilizing structural or functional methods has been regarded as a promising tool. Compared to the normal control group, the subcortical VaD group exhibited cortical atrophies in the frontal, occipital, and temporal areas and low integrity in the genu and splenium parts of the corpus callosum (7). Li et al. (8) also found that SIVCIND patients showed significant cerebral gray matter volume reduction in the insula, superior temporal gyrus, hippocampus, and parahippocampal gyrus, which have a closed correlation with language dysfunction, memory loss, and attention deficits. However, most of these studies only analyzed the changes in the macro structure of the brain, ignoring the micro changes in the subtle structure invisible to the naked eye (7–9).
Radiomics can extract high-dimensional image features from medical imaging (CT, MRI, positron emission tomography (PET), etc.) and analyze these features using high-throughput quantitative methods (10). Machine learning uses statistical techniques to grant computer systems the capability to “learn” from data to promote performance on an exact task without being explicitly programmed (11). The combination of radiomics and machine learning could help doctors diagnose diseases, evaluate prognosis, and even explore the correlation between images and genes. Recently, using this method, Betrouni et al. (12) proved that the texture features of the hippocampus and entorhinal cortex can help diagnose early cognitive impairment after stroke. The radiomics features of skewness and entropy in the hippocampus, thalamus, and amygdala were also found to be significantly different between the Parkinson’s group and healthy controls (13). Tozer et al. (14) reported that the texture parameters of white matter lesions could help diagnose cognitive impairment in cerebral small vessel disease and correlated with the global function and executive function significantly. However, until now, there is no study of the automatic diagnosis of SIVCIND by using radiomics and machine learning. In this study, we tried to use high-resolution T1-weighted imaging (T1WI) images to analyze the whole cerebral cortex and subcortical nuclei changes by radiomics to find characteristic biological features of SIVCIND and combine machine learning to establish diagnostic models to promote its early diagnosis.
Materials and Methods
Patient Cohort
This retrospective study was approved by the medical ethics committee of our hospital. All participants provided informed consent. A total of 116 right-handed participants involving 40 SIVCIND patients and 76 normal controls were recruited. Clinical performance was evaluated with the following neuropsychological tests: Mini-Mental State Examination (MMSE), Clinical Dementia Rating (CDR), Global Deterioration Scale (GDS), Activities of Daily Living Scale (ADL), Montreal Cognitive Assessment (MoCA), and Hachinski ischemic score (HIS) (15–17). According to the criterion proposed by Galluzzi et al. (18), only the SIVCIND patients who had a subcortical white matter hyperintensity (WMH) on T2-weighted imaging and had at least two lacunar infarcts were enrolled in this study. The SIVCIND patients were diagnosed in light of the following criteria (19): 1) subjective cognitive impairment reported by participants or their caregivers 2) insufficient cognitive deficits to reach the fifth revision of Diagnostic and Statistical Manual of Mental Disorders (DSM-5) for dementia; and 3) neuropsychological examination containing HIS judgment (HIS ≥ 7). Exclusion criteria included organic lesions of the brain (i.e., traumatic brain injury, acute phase of encephalorrhagia, epilepsy, encephalitis, encephaloma, and Parkinson’s disease), somatic disease (i.e., severe organ dysfunction syndrome, malnutrition, infection, anemia, and hypothyroidism), and mental illness that may influence cognitive abilities such as schizophrenia. Seventy-six gender-, age-, and educational experience-matched healthy volunteers were recruited as the normal controls, and all of them had no nervous system illness. None had current or a history of psychiatric diseases or risk factors of blood vessels that could lead to cognitive impairment. None of them had brain neoplasms, brain trauma, systemic illness, or MRI contraindications.
MRI Acquisition
All of the MR images were obtained on a 3.0-T scanner that was equipped with eight-channel phased-array head coils (Magnetom Trio; Siemens Medical Systems, Erlangen, Germany). The head motion minimization was controlled by foam padding. The high-resolution T1WI images were obtained by magnetization-prepared rapid gradient-echo (MP-RAGE) sequence (repetition time/echo time/inversion time (TR/TE/TI) = 1,900/2.52/900 ms, thickness = 1.0 mm, no gap, 176 slices, matrix = 256 × 256, voxel size = 1 mm × 1 mm × 1 mm, flip angle = 9°). The conventional MRI sequences were as follows: T1WI images (TR/TE = 200/2.78 ms, thickness = 4.0 mm, 25 slices, matrix = 384 × 384, voxel size = 0.7 mm × 0.6 mm × 5 mm, flip angle = 70°) and fluid-attenuated inversion recovery images (TR/TE/TI = 9,000/93/2,500 ms, thickness = 4.0 mm, 25 slices, matrix = 256 × 256, voxel size = 0.9 mm × 0.9 mm × 4 mm, flip angle = 130°).
Data Processing and Radiomics Feature Extraction
The subcortical brain region segmentation was performed by the Brainnetome fMRI Toolkit1 and Statistical parametric mapping 12 software (SPM12, Wellcome Department of Cognitive Neurology, UCL, London, UK). First, the high-resolution T1WI images of each subject were converted to Neuroimaging Informatics Technology Initiative (NIFTI) format. Then, all data were normalized to the Montreal Neurological Institute (MNI) standard T1 template (standard space: 181 × 217 × 181, resolution: 1 mm × 1 mm × 1 mm). Meanwhile, the Brainnetome Atlas were resliced to the standard MNI space with a resolution of 1 mm × 1 mm × 1 mm. Fourteen gray matter nuclei were further extracted as masks including the bilateral thalamus, hippocampus, globus pallidus, amygdala, nucleus accumbens, putamen, and caudate nucleus. Finally, the volumes of interest (VOIs) were gathered by point multiplication of the normalized T1 images and these masks for each subject. Four hundred twenty-three radiomics features were quantitatively excavated from each gray matter nucleus by the In-house MATLAB script (20, 21). A total of 5,922 (423 × 14) radiomics features were acquired. These features could be grouped into three categories. Category 1: first-order statistics features, which quantitatively described the distribution of voxel intensity within the MR images. Category 2: textural features, which were calculated from the gray-level run-length matrix (GLRLM) and the gray-level co-occurrence matrix (GLCM) representing the heterogeneity of regions. Category 3: higher-order statistics features, which were derived from wavelet transformation of the first-order statistics features and texture features in eight directions (HLL, LLL, HLH, HHL, LLH, LHH, LHL, and HHH).
The feature extraction of the brain cortex was performed by FreeSurfer (v6.0.0) software2. First, the high-resolution T1WI images of each subject were converted to NIFTI data. The preprocessing included the following steps: correction of motion, stripping of the skull, transformation of coordinate, segmentation of gray–white matter, reconstruction of cortical surface models, labeling of a region, registration, and statistical analysis (22). Then the entire cortex was separated by the Destrieux atlas (23). Finally, eight brain cortex features from each brain region were obtained involving gray matter volume, surface area, SD of thickness, average thickness, folding index, intrinsic curvature index, integrated rectified mean curvature, and integrated rectified Gaussian curvature. A total of 1,184 (8 × 148) features were acquired.
Feature Dimension Reduction, Model Construction, and Evaluation
The processes of radiomics feature reduction and diagnosis model construction were performed with R software (24). First, the abnormal values were replaced by the median, and then standardization was applied to get all data on the same scale (25). The feature reduction included the following steps: univariate logistic regression (LR), Spearman’s correlation, general univariate analysis, gradient boosting decision tree (GBDT), least absolute shrinkage and selection operator (LASSO), and variance analysis (22). Then, three machine learning models of LR, random forest (RF), and support vector machine (SVM) were constructed. These machine learning models and feature selection methods were selected because of their practicability and experimental efficiency (11). Each model was trained and validated independently employing the 5-fold cross-validation, and 10 times 10-fold cross-validation was used to evaluate the generalization performance (11). The accuracy, specificity, sensitivity, and area under the receiver operator characteristic (ROC) curve (AUC) were employed to assess the diagnosis capability. The framework of the radiomics workflow is summarized in Figure 1.
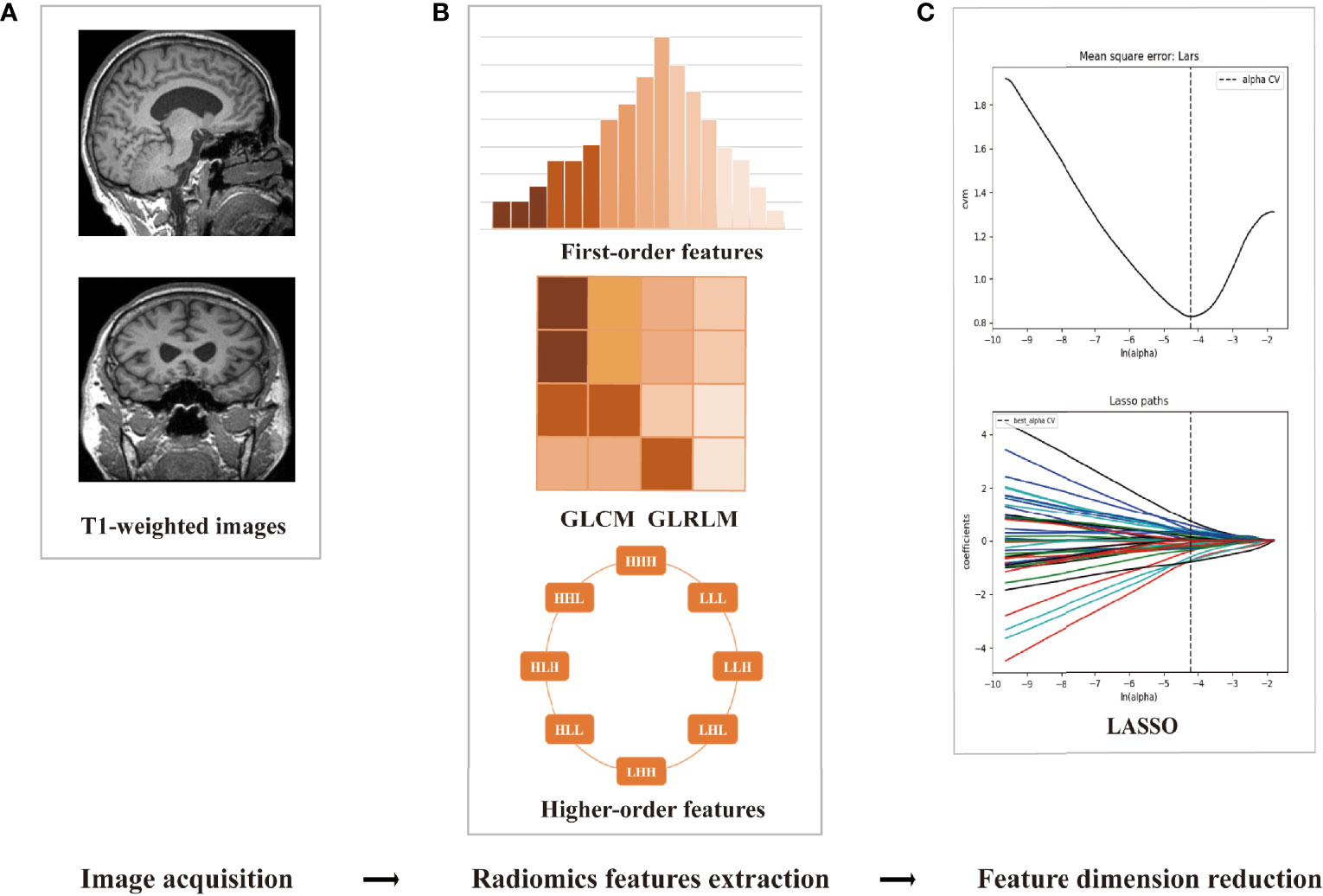
Figure 1 The framework of the radiomics workflow. (A) Data preprocessing and cortex/subcortical brain region segmentation. (B) Three categories of radiomics feature extraction. (C) Employing the least absolute shrinkage and selection operator (LASSO) algorithm to reduce the redundancy feature.
Correlation Analysis
Correlation analysis was performed between the optimal features and the MoCA, MMSE, and ADL scores of the SIVCIND patients using Pearson’s correlation test (for normally distributed features) or Spearman’s rank correlation analysis (for abnormally distributed features), respectively. A two-tailed p < 0.05 was regarded as statistically significant. SPSS (version 21.0) was used for correlation tests.
Results
Patient Cohort Characteristics
The SIVCIND patients showed significantly lower MoCA and MMSE scores than the normal controls (p < 0.001). There was no significant difference between the SIVCIND cohort and normal control cohort in gender (p = 0.589), age (p = 0.696), and educational experience (p = 0.773) (Table 1).
Feature Dimension Reduction and Optimal Subset Selection
During the feature extraction, a total of 7,106 (5,922 + 1,184) features were acquired. A total of 5,922 features were from subcortical brain regions, and 1,184 features were from the brain cortex. First, 925 features were selected by employing the univariate LR. Then, with Spearman’s correlation, 413 features were selected. After general univariate analysis, 357 features were retained. Next, GBDT was employed, and 57 features remained. Then, 27 features were chosen through the LASSO method. Finally, 13 features were selected as the optimal subset with variance analysis. All these features are illustrated in Table 2.
For the diagnosis of SIVCIND, the RF model, LR model, and SVM model showed an AUC of 0.990, 0.934, and 0.969; accuracy of 0.948, 0.888, and 0.888; sensitivity of 0.875, 0.675, and 0.700; and specificity of 0.987, 1.00, and 0.987, respectively. Among all the three models, the RF model yielded the best diagnosis performance (Figure 2). All of the models have robust generalization performance, and the results are expressed by box plots (Figure 3).
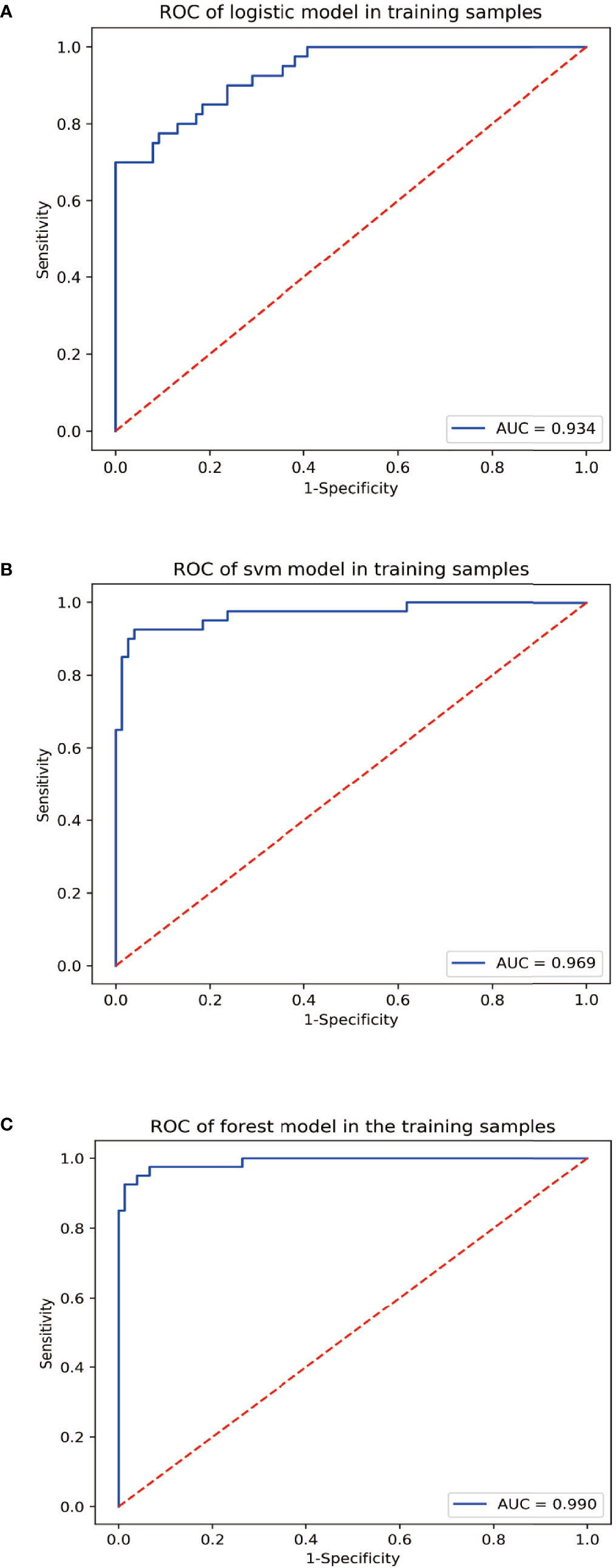
Figure 2 The receiver operator characteristic (ROC) curves of the radiomics models for discriminating the normal controls (NM) and subcortical ischemic vascular cognitive impairment with no dementia (SIVCIND) subjects. (A) ROC curve of logistic regression (LR) (area under the ROC curve (AUC) = 0.934). (B) ROC curve of support vector machine (SVM) (AUC = 0.969). (C) ROC curve of random forest (RF) (AUC = 0.990).
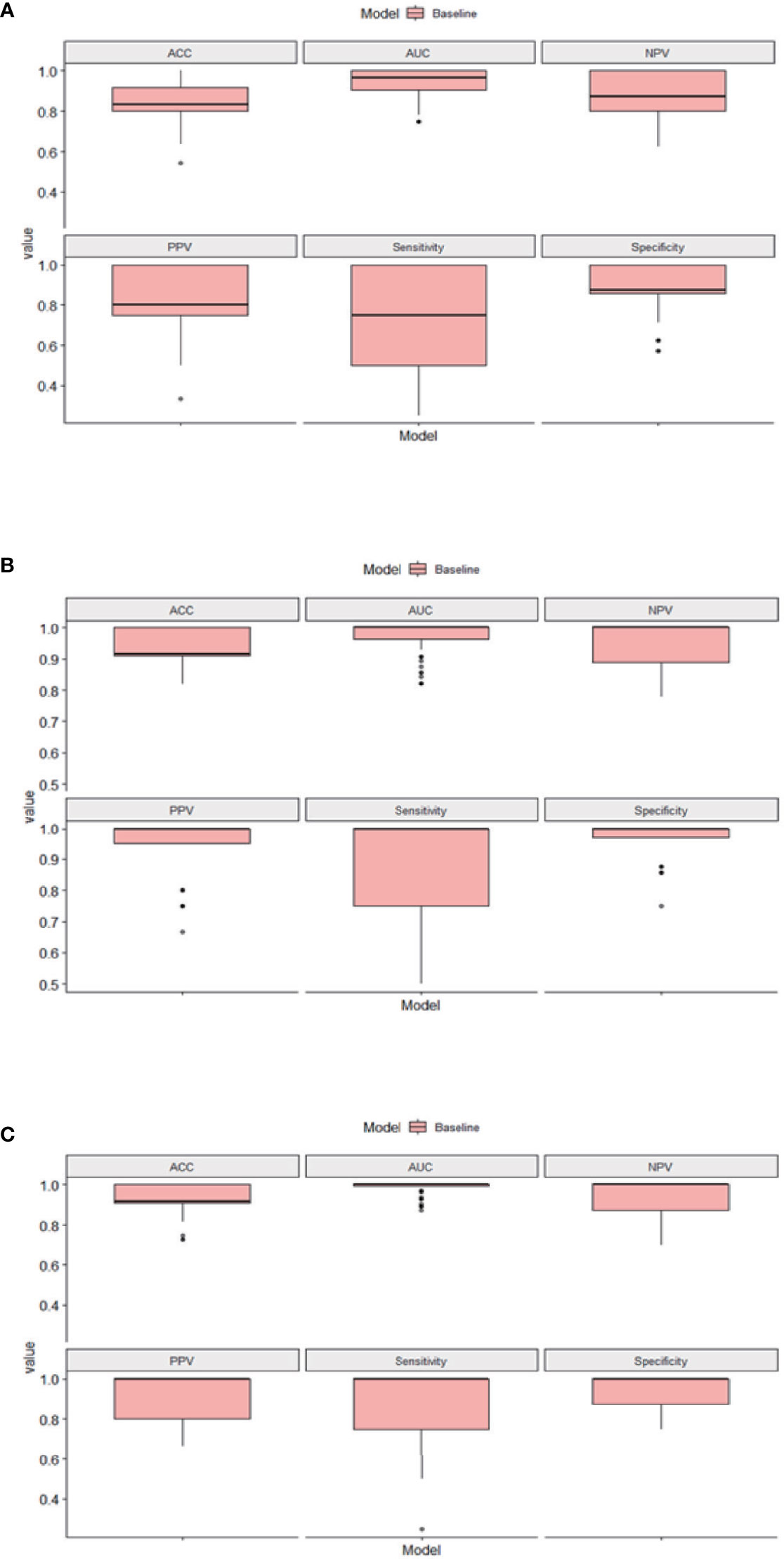
Figure 3 The results of the 10 times 10-fold cross-validation of our models. (A) Box plots of logistic regression (LR). (B) Box plots of support vector machine (SVM). (C) Box plots of random forest (RF). ACC, accuracy; AUC, area under the curve; NPV, negative predictive value; PPV, positive predictive value.
Correlation Analysis
The High Gray Level Run Emphasis (HGLRE)-LHL of the right amygdala, Short Run Emphasis (SRE)-HLH of the left caudate nucleus and SRE-HHH of the left thalamus were found significantly correlated (p<0.05) with the MoCA scores of SIVCIND patients. The Cluster Shade (CS) of the left putamen and SRE-HLH of the left caudate nucleus were found significantly correlated (p<0.05) with the MMSE scores of SIVCIND patients. The SRE-HLH of the left caudate nucleus was found significantly correlated (p<0.05) with the ADL scores of SIVCIND patients. The detail results of the correlation test are shown in Table 3.
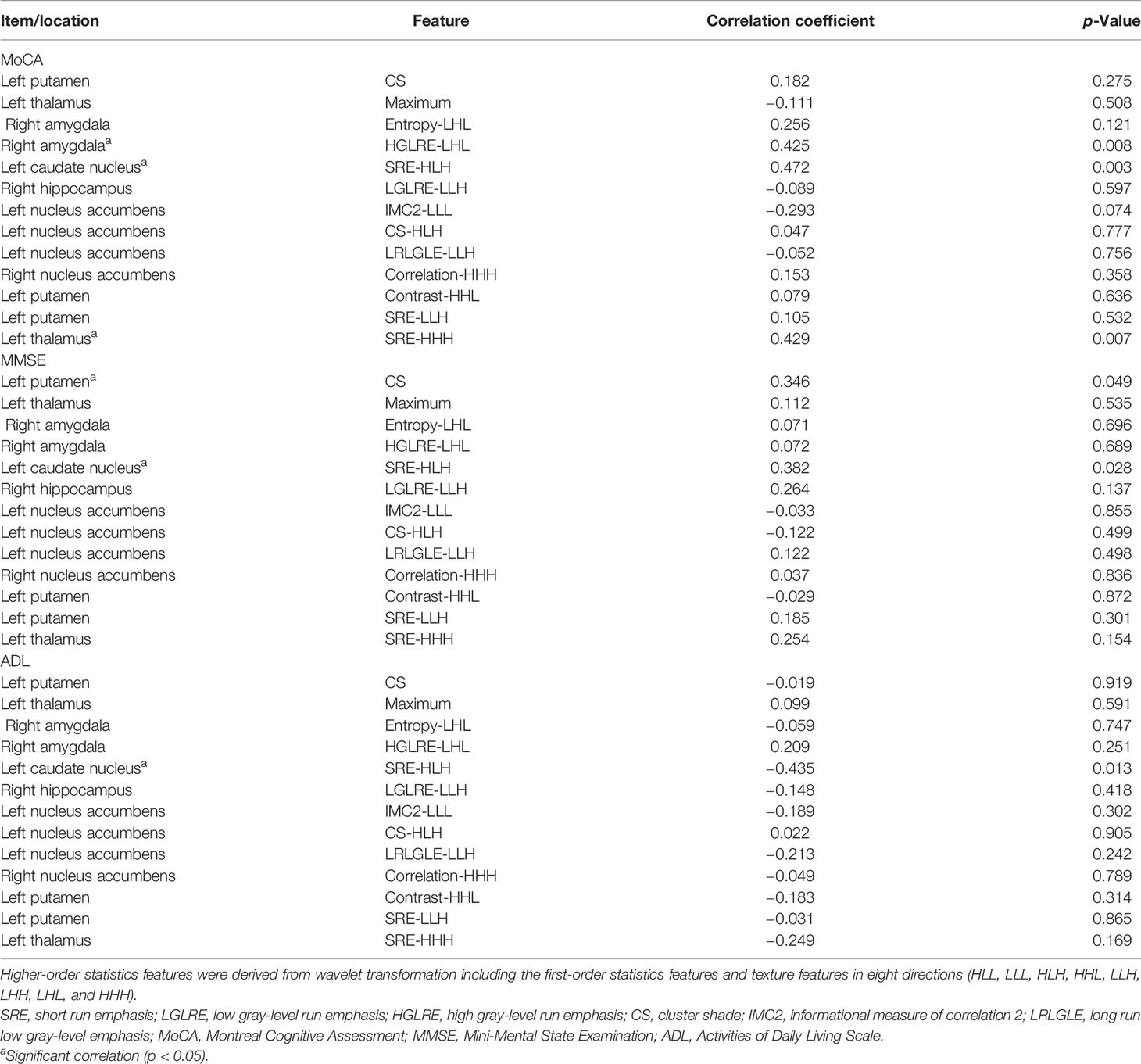
Table 3 Correlation tests between radiomics features of optimal subset and MoCA, MMSE, and ADL cores.
Discussion
The early diagnosis of SIVCIND is very important for timely treatment and prognosis improvement. Neuropsychological scale testing, electrophysiology examination, and medical imaging of structural or functional approaches all have various defects that limit their clinical use. Radiomics can automatically extract a large number of high-throughput quantitative features from neuroimaging images using data representation algorithms and provide additional potential information far beyond visual range analysis. Appropriate feature reduction and machine learning methods can obtain the optimal subset of radiographic features and establish a robust and effective diagnostic model. Previously, radiomics analysis of brain MR images was utilized to diagnose mild cognitive impairment (MCI), Alzheimer’s disease (AD), and schizophrenia and differentiate Parkinson’s disease motor subtypes successfully (26). On the other hand, the radiomics of brain MR images could predict the time to progression from MCI to AD (22).
So far, the research on machine learning and radiomics in the diagnosis of SIVCIND has not been reported. In this study, we found that the LR, SVM, and RF models based on the optimal features all yielded excellent performance in terms of SIVCIND diagnosis. Among them, the RF model showed the highest AUC of 0.990 and the highest accuracy of 0.948. These results suggested that the combination of machine learning and radiomics could diagnose SIVCIND accurately. Because of the convenience, objectivity, and non-invasiveness, it has important clinical application value. Previously, graph theory and network-based statistics (NBS) have been employed to analyze the whole-brain mean factional anisotropy matrix, and the accuracy for diagnosing SIVCIND is 0.780 (27). Another study used an SVM-based machine learning strategy to discriminate between different cognitive stages of SIVCI patients with predefined features extracted from diffusion tensor imaging (DTI) and got an accuracy of 0.775–0.800 (28). Wang et al. had used a convolutional neural network (CNN) and T2-weighted sequence to diagnose SIVCIND. The accuracy of the 2D model was 0.540, and that of the 3D model was 0.900 (29). Compared with these previous studies, our study obtained higher AUC and accuracy. The possible reason may be that we analyzed the high-resolution images of the whole cerebral cortex and subcortical nucleus. A large number of high-throughput radiological features can provide more neuropathological features and improve diagnostic accuracy.
In this study, after feature reduction, 13 features from subcortical nuclei of the thalamus, caudate nucleus, nucleus accumbens, putamen, amygdala, and hippocampus were selected as the optimal subset. The radiomics features can reveal hidden changes of brain microstructure that are difficult to be quantitatively recognized by the naked eye. All of the 13 features were intensity features and texture features, which can reflect the heterogeneity of brain tissue images. The heterogeneity of the SIVCIND might be due to the neuron degeneration, lacunar infarcts, and latent lesions originating from subcortical ischemic vascular disease, which often occurs in the area of the basal ganglia and thalamus (30, 31). Among all the 13 features, 11 of them were higher-order statistics features of wavelet conversion. This indicated that higher-order statistics features could disclose the latent changes of brain tissue more explicitly. Wavelet conversion can lessen noise and sharpen the image and does not change the semantic meaning of the radiomics parameters (11). According to the correlation analysis, the radiomics features of the right amygdala, left caudate nucleus, left putamen, and left thalamus were found to be significantly correlated with the neuropsychological scores of the SIVCIND patients. This result suggested that the concealed changes of the above regions may be early biological markers of SIVCIND. The putamen is the area of the brain responsible for emotion and motivation (32, 33). The thalamus and caudate nucleus have been proved to be related to consciousness and cognition (33, 34). The amygdala is associated with exercise and emotion (32, 35).
In conclusion, in this study, we found that the integration of radiomics derived from brain high-resolution T1WI and machine learning could diagnose SIVCIND accurately and automatically. The optimal radiomics features are mostly located in the right amygdala, left caudate nucleus, left putamen, and left thalamus, which might be the new biomarkers of SIVCIND. The high-resolution T1-weighted MR imaging was generated by MP-RAGE sequence, which was easily obtained and widely applied in clinical practice. It can provide images with high spatial resolution and a high signal-to-noise ratio (36). The combination of radiomics derived from brain high-resolution T1WI and automatic machine learning to diagnose SIVCIND is a new technology. This method is independent of the traditional clinical evaluation and can be used as an effective supplement to the traditional neuropsychological scale test.
This study has several limitations. First, the sample size is relatively small due to strict inclusion/exclusion criteria. Second, this research only focused on the structural data of the SIVCIND subjects but did not study the functional data. Third, in the model construction, only MRI-derived radiological features were used. The laboratory measurements and clinical information were not taken into consideration. In future work, different imaging technologies, including CT, PET, and MRI, and clinical and laboratory parameters should be combined to further improve the diagnostic efficiency of SIVCIND.
Data Availability Statement
The raw data supporting the conclusions of this article will be made available by the authors, without undue reservation.
Ethics Statement
The studies involving human participants were reviewed and approved by The Second Affiliated Hospital of Chongqing Medical University. The patients/participants provided their written informed consent to participate in this study.
Author Contributions
BL and SM analyzed the data and wrote the manuscript. JC and HL analyzed the data. YZ, DZ, XD, LK, XW, LT, and HW collected the relevant data. CML and CL put forward the study topic and revised the manuscript. All authors read and approved the final manuscript.
Funding
This study has received funding from the Chongqing Natural Science Foundation (grant number cstc2020jcyj-msxmX0044), Chongqing Science and Health Joint Medical Research Project of China (grant number 2018ZDXM005), Intelligent Medicine Research Project of Chongqing Medical University (grant number ZHYX202004), Special Project for Technological Innovation and Application Development of Chongqing City (grant number cstc2019jscx-msxmX0104), and Kuanren Talents Program of the Second Affiliated Hospital of Chongqing Medical University.
Conflict of Interest
Author HL was employed by GE Healthcare (China).
The remaining authors declare that the research was conducted in the absence of any commercial or financial relationships that could be construed as a potential conflict of interest.
Publisher’s Note
All claims expressed in this article are solely those of the authors and do not necessarily represent those of their affiliated organizations, or those of the publisher, the editors and the reviewers. Any product that may be evaluated in this article, or claim that may be made by its manufacturer, is not guaranteed or endorsed by the publisher.
Acknowledgments
The authors thank GE Healthcare for their kind support.
Footnotes
References
1. Prince M, Bryce R, Albanese E, Wimo A, Ribeiro W, Ferri CP. The Global Prevalence of Dementia: A Systematic Review and Metaanalysis. Alzheimers Dement (2013) 9(1):63–75.e2. doi: 10.1016/j.jalz.2012.11.007
2. Gale SA, Acar D, Daffner KR. Dementia. Am J Med (2018) 131(10):1161–9. doi: 10.1016/j.amjmed.2018.01.022
3. Seo SW, Ahn J, Yoon U, Im K, Lee JM, Tae Kim S, et al. Cortical Thinning in Vascular Mild Cognitive Impairment and Vascular Dementia of Subcortical Type. J Neuroimaging (2010) 20(1):37–45. doi: 10.1111/j.1552-6569.2008.00293.x
4. Jak AJ, Bondi MW, Delano-Wood L, Wierenga C, Corey-Bloom J, Salmon DP, et al. Quantification of Five Neuropsychological Approaches to Defining Mild Cognitive Impairment. Am J Geriatr Psychiatry (2009) 17(5):368–75. doi: 10.1097/JGP.0b013e31819431d5
5. Hsu YH, Huang CF, Lo CP, Wang TL, Yang CC, Tu MC. Frontal Assessment Battery as a Useful Tool to Differentiate Mild Cognitive Impairment Due to Subcortical Ischemic Vascular Disease From Alzheimer Disease. Dement Geriatr Cognit Disord (2016) 42(5-6):331–41. doi: 10.1159/000452762
6. Xu J, Sheng H, Lou W, Zhao S. Approximate Entropy Analysis of Event-Related Potentials in Patients With Early Vascular Dementia. J Clin Neurophysiol (2012) 29(3):230–6. doi: 10.1097/WNP.0b013e318257ca9d
7. Jung WB, Mun CW, Kim YH, Park JM, Lee BD, Lee YM, et al. Cortical Atrophy, Reduced Integrity of White Matter and Cognitive Impairment in Subcortical Vascular Dementia of Binswanger Type. Psychiatry Clin Neurosci (2014) 68(12):821–32. doi: 10.1111/pcn.12196
8. Li M, Meng Y, Wang M, Yang S, Wu H, Zhao B, et al. Cerebral Gray Matter Volume Reduction in Subcortical Vascular Mild Cognitive Impairment Patients and Subcortical Vascular Dementia Patients, and Its Relation With Cognitive Deficits. Brain Behav (2017) 7(8):e00745. doi: 10.1002/brb3.745
9. Sang L, Chen L, Wang L, Zhang J, Zhang Y, Li P, et al. Progressively Disrupted Brain Functional Connectivity Network in Subcortical Ischemic Vascular Cognitive Impairment Patients. Front Neurol (2018) 9:94. doi: 10.3389/fneur.2018.00094
10. Lambin P, Rios-Velazquez E, Leijenaar R, Carvalho S, van Stiphout RG, Granton P, et al. Radiomics: Extracting More Information From Medical Images Using Advanced Feature Analysis. Eur J Cancer (2012) 48(4):441–6. doi: 10.1016/j.ejca.2011.11.036
11. Liu B, Cheng J, Guo DJ, He XJ, Luo YD, Zeng Y, et al. Prediction of Prostate Cancer Aggressiveness With a Combination of Radiomics and Machine Learning-Based Analysis of Dynamic Contrast-Enhanced Mri. Clin Radiol (2019) 74(11):896.e1–896.e8. doi: 10.1016/j.crad.2019.07.011
12. Betrouni N, Yasmina M, Bombois S, Pétrault M, Dondaine T, Lachaud C, et al. Texture Features of Magnetic Resonance Images: An Early Marker of Post-Stroke Cognitive Impairment. Transl Stroke Res (2020) 11(4):643–52. doi: 10.1007/s12975-019-00746-3
13. Betrouni N, Lopes R, Defebvre L, Leentjens AFG, Dujardin K. Texture Features of Magnetic Resonance Images: A Marker of Slight Cognitive Deficits in Parkinson’s Disease. Mov Disord (2020) 35(3):486–94. doi: 10.1002/mds.27931
14. Tozer DJ, Zeestraten E, Lawrence AJ, Barrick TR, Markus HS. Texture Analysis of T1-Weighted and Fluid-Attenuated Inversion Recovery Images Detects Abnormalities That Correlate With Cognitive Decline in Small Vessel Disease. Stroke (2018) 49(7):1656–61. doi: 10.1161/strokeaha.117.019970
15. Nasreddine ZS, Phillips NA, Bédirian V, Charbonneau S, Whitehead V, Collin I, et al. The Montreal Cognitive Assessment, Moca: A Brief Screening Tool for Mild Cognitive Impairment. J Am Geriatr Soc (2005) 53(4):695–9. doi: 10.1111/j.1532-5415.2005.53221.x
16. Morris JC. The Clinical Dementia Rating (Cdr): Current Version and Scoring Rules. Neurology (1993) 43(11):2412–4. doi: 10.1212/wnl.43.11.2412-a
17. Cummings JL, Mega M, Gray K, Rosenberg-Thompson S, Carusi DA, Gornbein J. The Neuropsychiatric Inventory: Comprehensive Assessment of Psychopathology in Dementia. Neurology (1994) 44(12):2308–14. doi: 10.1212/wnl.44.12.2308
18. Galluzzi S, Sheu CF, Zanetti O, Frisoni GB. Distinctive Clinical Features of Mild Cognitive Impairment With Subcortical Cerebrovascular Disease. Dement Geriatr Cognit Disord (2005) 19(4):196–203. doi: 10.1159/000083499
19. Sachdev P, Kalaria R, O’Brien J, Skoog I, Alladi S, Black SE, et al. Diagnostic Criteria for Vascular Cognitive Disorders: A Vascog Statement. Alzheimer Dis Assoc Disord (2014) 28(3):206–18. doi: 10.1097/wad.0000000000000034
20. Aerts HJ, Velazquez ER, Leijenaar RT, Parmar C, Grossmann P, Carvalho S, et al. Decoding Tumour Phenotype by Noninvasive Imaging Using a Quantitative Radiomics Approach. Nat Commun (2014) 5:4006. doi: 10.1038/ncomms5006
21. Huynh E, Coroller TP, Narayan V, Agrawal V, Hou Y, Romano J, et al. Ct-Based Radiomic Analysis of Stereotactic Body Radiation Therapy Patients With Lung Cancer. Radiother Oncol (2016) 120(2):258–66. doi: 10.1016/j.radonc.2016.05.024
22. Tang L, Wu X, Liu H, Wu F, Song R, Zhang W, et al. Individualized Prediction of Early Alzheimer’s Disease Based on Magnetic Resonance Imaging Radiomics, Clinical, and Laboratory Examinations: A 60-Month Follow-Up Study. J Magn Reson Imaging (2021) 54(5):1647–57. doi: 10.1002/jmri.27689
23. Destrieux C, Fischl B, Dale A, Halgren E. Automatic Parcellation of Human Cortical Gyri and Sulci Using Standard Anatomical Nomenclature. Neuroimage (2010) 53(1):1–15. doi: 10.1016/j.neuroimage.2010.06.010
24. Kolossváry M, Karády J, Szilveszter B, Kitslaar P, Hoffmann U, Merkely B, et al. Radiomic Features Are Superior to Conventional Quantitative Computed Tomographic Metrics to Identify Coronary Plaques With Napkin-Ring Sign. Circ Cardiovasc Imaging (2017) 10(12):e006843. doi: 10.1161/circimaging.117.006843
25. Yin P, Mao N, Liu X, Sun C, Wang S, Chen L, et al. Can Clinical Radiomics Nomogram Based on 3d Multiparametric Mri Features and Clinical Characteristics Estimate Early Recurrence of Pelvic Chondrosarcoma? J Magn Reson Imaging (2020) 51(2):435–45. doi: 10.1002/jmri.26834
26. Sun D, Wu X, Xia Y, Wu F, Geng Y, Zhong W, et al. Differentiating Parkinson’s Disease Motor Subtypes: A Radiomics Analysis Based on Deep Gray Nuclear Lesion and White Matter. Neurosci Lett (2021) 760:136083. doi: 10.1016/j.neulet.2021.136083
27. Sang L, Liu C, Wang L, Zhang J, Zhang Y, Li P, et al. Disrupted Brain Structural Connectivity Network in Subcortical Ischemic Vascular Cognitive Impairment With No Dementia. Front Aging Neurosci (2020) 12:6. doi: 10.3389/fnagi.2020.00006
28. Ciulli S, Citi L, Salvadori E, Valenti R, Poggesi A, Inzitari D, et al. Prediction of Impaired Performance in Trail Making Test in Mci Patients With Small Vessel Disease Using Dti Data. IEEE J BioMed Health Inform (2016) 20(4):1026–33. doi: 10.1109/jbhi.2016.2537808
29. Wang Y, Tu D, Du J, Han X, Sun Y, Xu Q, et al. Classification of Subcortical Vascular Cognitive Impairment Using Single Mri Sequence and Deep Learning Convolutional Neural Networks. Front Neurosci (2019) 13:627. doi: 10.3389/fnins.2019.00627
30. Feekes JA, Hsu SW, Chaloupka JC, Cassell MD. Tertiary Microvascular Territories Define Lacunar Infarcts in the Basal Ganglia. Ann Neurol (2005) 58(1):18–30. doi: 10.1002/ana.20505
31. Lim JS, Kwon HM, Lee YS. Effect of Cholinergic Pathway Disruption on Cortical and Subcortical Volumes in Subcortical Vascular Cognitive Impairment. Eur J Neurol (2020) 27(1):210–2. doi: 10.1111/ene.14073
32. Eimontaite I, Schindler I, De Marco M, Duzzi D, Venneri A, Goel V. Left Amygdala and Putamen Activation Modulate Emotion Driven Decisions in the Iterated Prisoner’s Dilemma Game. Front Neurosci (2019) 13:741. doi: 10.3389/fnins.2019.00741
33. Huijgens PT, Snoeren EMS, Meisel RL, Mermelstein PG. Effects of Gonadectomy and Dihydrotestosterone on Neuronal Plasticity in Motivation and Reward Related Brain Regions in the Male Rat. J Neuroendocrinol (2021) 33(1):e12918. doi: 10.1111/jne.12918
34. Jia X, Pan Z, Chen H, Wang Z, Li K, Wang X, et al. Differential Functional Dysconnectivity of Caudate Nucleus Subdivisions in Parkinson’s Disease. Aging (Albany NY) (2020) 12(16):16183–94. doi: 10.18632/aging.103628
35. Schönfeld LM, Wojtecki L. Beyond Emotions: Oscillations of the Amygdala and Their Implications for Electrical Neuromodulation. Front Neurosci (2019) 13:366. doi: 10.3389/fnins.2019.00366
Keywords: subcortical ischemic vascular cognitive impairment with no dementia, diagnosis, radiomics, high-resolution T1-weighted imaging, machine learning
Citation: Liu B, Meng S, Cheng J, Zeng Y, Zhou D, Deng X, Kuang L, Wu X, Tang L, Wang H, Liu H, Liu C and Li C (2022) Diagnosis of Subcortical Ischemic Vascular Cognitive Impairment With No Dementia Using Radiomics of Cerebral Cortex and Subcortical Nuclei in High-Resolution T1-Weighted MR Imaging. Front. Oncol. 12:852726. doi: 10.3389/fonc.2022.852726
Received: 11 January 2022; Accepted: 15 March 2022;
Published: 08 April 2022.
Edited by:
Bo Gao, Affiliated Hospital of Guizhou Medical University, ChinaReviewed by:
S. Senthil Kumaran, All India Institute of Medical Sciences, IndiaWellington Pinheiro dos Santos, Federal University of Pernambuco, Brazil
Copyright © 2022 Liu, Meng, Cheng, Zeng, Zhou, Deng, Kuang, Wu, Tang, Wang, Liu, Liu and Li. This is an open-access article distributed under the terms of the Creative Commons Attribution License (CC BY). The use, distribution or reproduction in other forums is permitted, provided the original author(s) and the copyright owner(s) are credited and that the original publication in this journal is cited, in accordance with accepted academic practice. No use, distribution or reproduction is permitted which does not comply with these terms.
*Correspondence: Chen Liu, liuchen@aifmri.com; Chuanming Li, lichuanming@hospital.cqmu.edu.cn
†These authors contributed equally to this work and share first authorship