- 1Myeloma Lymphoma Research Group, Champalimaud Experimental Clinical Research Programme, Champalimaud Foundation, Lisbon, Portugal
- 2NOVA Medical School (NMS), NOVA University Lisbon, Lisbon, Portugal
- 3Hemato-Oncology Unit, Champalimaud Clinical Centre, Champalimaud Foundation, Lisbon, Portugal
- 4Centre of Statistics and its Applications, Faculty of Sciences, University of Lisbon, Lisbon, Portugal
- 5Faculty of Medicine, University of Lisbon, Lisbon, Portugal
- 6Centre for Clinical Proteomics, Clinical Biochemistry and Pharmacology, Odense University Hospital, Odense, Denmark
- 7NOVA Medical School (NMS), Faculdade de Ciências Médicas (FCM), Universidade Nova de Lisboa, Lisboa, Portugal
- 8Systems Oncology Group, Champalimaud Physiology and Cancer Programme, Champalimaud Foundation, Lisbon, Portugal
Multiple myeloma (MM) is a hematological malignancy of clonal antibody–secreting plasma cells (PCs). MM diagnosis and risk stratification rely on bone marrow (BM) biopsy, an invasive procedure prone to sample bias. Liquid biopsies, such as extracellular vesicles (EV) in peripheral blood (PB), hold promise as new minimally invasive tools. Real-world studies analyzing patient-derived EV proteome are rare. Here, we characterized a small EV protein content from PB and BM samples in a cohort of 102 monoclonal gammopathies patients routinely followed in the clinic and 223 PB and 111 BM samples were included. We investigated whether EV protein and particle concentration could predict an MM patient prognosis. We found that a high EV protein/particle ratio, or EV cargo >0.6 µg/108 particles, is related to poorer survival and immune dysfunction. These results were supported at the protein level by mass spectrometry. We report a set of PB EV-proteins (PDIA3, C4BPA, BTN1A1, and TNFSF13) with a new biomarker potential for myeloma patient outcomes. The high proteomic similarity between PB and BM matched pairs supports the use of circulating EV as a counterpart of the BM EV proteome. Overall, we found that the EV protein content is related to patient outcomes, such as survival, immune dysfunction, and possibly treatment response.
1 Introduction
MM is a hematological malignancy characterized by the accumulation of clonal plasma cells (PCs) within the bone marrow (BM) and progressive immune dysfunction. The natural progression of myeloma includes the monoclonal gammopathies of uncertain significance (MGUS) and smoldering multiple myeloma (MM) asymptomatic phases, characterized by clonal PCs in the BM without organ damage or a myeloma-defining event (1). Current risk stratification requires BM PC phenotypic/genotypic characterization. Given clonal PC patchy distribution, BM biopsies may not reflect disease heterogeneity (2) while submitting patients to invasive procedures. Finally, scoring systems can be improved as patients within the same prognostic categories may still have very different outcomes. Liquid biopsies may overcome these limitations since they are minimally invasive and less prone to spatial bias and allow for serial sampling. They encompass collecting biofluids (e.g., PB) to obtain a subset of circulating tumor components such as extracellular vesicles (EV), which are bilayer lipid particles naturally released from all cells (3). In recent years, their value as key players in precision medicine has been demonstrated (4). In cancer, EV are emergent actors in intercellular communication transferring cargo molecules (e.g., microRNA and proteins) influencing the phenotype and function of target cells (5). In MM, there is evidence that EV intervenes in key processes such as tumor progression (6), immunosuppression (7), and drug resistance (8). Most studies analyzing MM EV have been focused on the genome, and real-world studies on the EV proteome are scarce. Here, we assessed MM patient EV across routine hemato-oncology clinical practice. Our results demonstrated that the EV protein content is potentially associated to MM patient outcomes such as immune dysfunction, survival, and response to treatment.
2 Materials and Methods
Clinical study: Healthy donors (HDs), MGUS, and MM patients followed at two hematology departments were included from May 2016 to July 2020, after study approvals. All procedures followed the Helsinki Declaration and were approved by the Institutions’ Ethics Committee, and participants gave written informed consent before inclusion. Disease and response assessments followed the International Myeloma Working Group guidelines (9). Patients’ PB and/or BM samples were collected prospectively according to the clinical follow-up, meaning that MM patients were included before treatment and/or at disease evaluation (all lines). The MM status at the sample collection was defined as smoldering (SMM), newly diagnosed (MM-ND), responders (MM-R) if in partial response or better, or non-responders (MM-NR) if a stable or progressive disease. HDs only collected PB. The patient and sample distribution for each analysis is described in Figure S1. Overall survival (OS) refers to the time from inclusion to death by any cause.
Small EV purification: PB and BM samples were centrifuged at 500 g for 10 min. The collected supernatant was centrifuged at 3,000 g for 20 min at 4°C, and plasma was stored at -80°C. Sequential ultracentrifugation with gradient density was performed as previously described (10), permitting intermediate EV recovery with intermediate specificity. Detailed protocols can be found in supplementary material.
Transmission electron-microscopy (TEM): Purified EV were absorbed onto formvar/carbon-coated glow-discharged copper EM grids (5 μl on each grid) for 20 min; then fixed with 2% formaldehyde, 20min. Grids were stained with 2% uranyl acetate for 5 min. TEM was performed using FEI Tecnai G2 Spirit BioTWIN TEM operating at an accelerating voltage of 120 keV. Images were acquired using an Olympus-SIS Veleta CCD Camera.
Western blot: The presence and purity of EV were assessed by western blot using 5ug of protein per sample. CD9 was used as an EV-positive biomarker and APOA1 as a purity biomarker (3).
EV characteristics: EV protein concentration was determined by the colorimetric bicinchoninic acid protein assay (BCA) according to the manufacturer’s instructions (Sigma, #B9643). The size and EV particle concentration were quantified by the NS300 Nanoparticle Tracking Analysis (NTA) system. Both measures were normalized by the initial volume of plasma per sample. The protein/particle ratio was determined as EV cargo (EVc).
Mass spectrometry: LC-MS/MS was used to characterize EV protein content. Peptides were analyzed by nano-LC-MS/MS using a Q-Exactive mass spectrometer coupled to an EASY-nLC 1000 liquid chromatography system (Thermo Fisher Scientific, Waltham, Massachusetts, United States) via the Nanospray Flex Ion Source.
Statistics: Differences in EV characteristics were tested with the linear mixed-effect model (“lme4” package) (11, 12). The EV sample correlation used the Spearman rank-order correlation (r) test; its strength was considered: r < 0.3, poor; 0.3 ≤ r <0.6, fair; 0.6 ≤ r <0.8, moderately strong, and 0.8 ≤ r, very strong (13). The variable association was tested through Pearson’s chi-squared test/Fisher’s exact test. Differently expressed EV proteins and protein expression correlations (duplicateCorrelation function) were analyzed using the “limma” package. The unadjusted hypergeometric p-value was used to compare functional protein enrichment between the groups of differentially expressed proteins. The surv_cutpoint function from the “survminer” package (14) was used to obtain the optimal cut-off point with the most significant relation to survival for EV characteristics (min. 0.25 observations/group). The multivariable logistic regression model for binary longitudinal data and Cox proportional hazards models used the “bild” (15) and “survival” (16) packages, respectively, with the inclusion/exclusion p-value criterion of 0.2 (17). The Wald test was used for parameter significance testing. “Survival” (16) and “survminer” (14) packages estimated the survival functions and computed the Kaplan–Meier survival curves. For the differences between survival estimates, the log-rank test was used. Unless otherwise stated, the significance is at the 5% level. Two-tailed p-value ≤ 0.05*, <0.01** and < 0.001*** were considered significant.. R software was used for analysis (18).
3 Results
3.1 Cohort Description
A total of 102 patients (MGUS=38, SMM=13, and MM=51) and 19 HDs were included. Baseline characteristics are summarized in Table 1. The cohort median follow-up time was 25.18 (95% CI: 21.0–31.13) months: 27.12 (95% CI: 19.82–31.20) months for MGUS, 23.54 (95% CI: 18.0–38.0 months for SMM, and 25.18 (95% CI: 20.8–35.4) months for MM patients. At the study inclusion, 21 patients were classified as MM-ND (before first-line treatment), 24 were classified as MM-NR, and 6 were classified as MM-R. According to the approved regimens, patients could have received a proteasome inhibitor (bortezomib, carfilzomib, or ixazomib), an immunomodulatory agent (lenalidomide or pomalidomide), or anti-CD38 monoclonal antibodies (daratumumab).
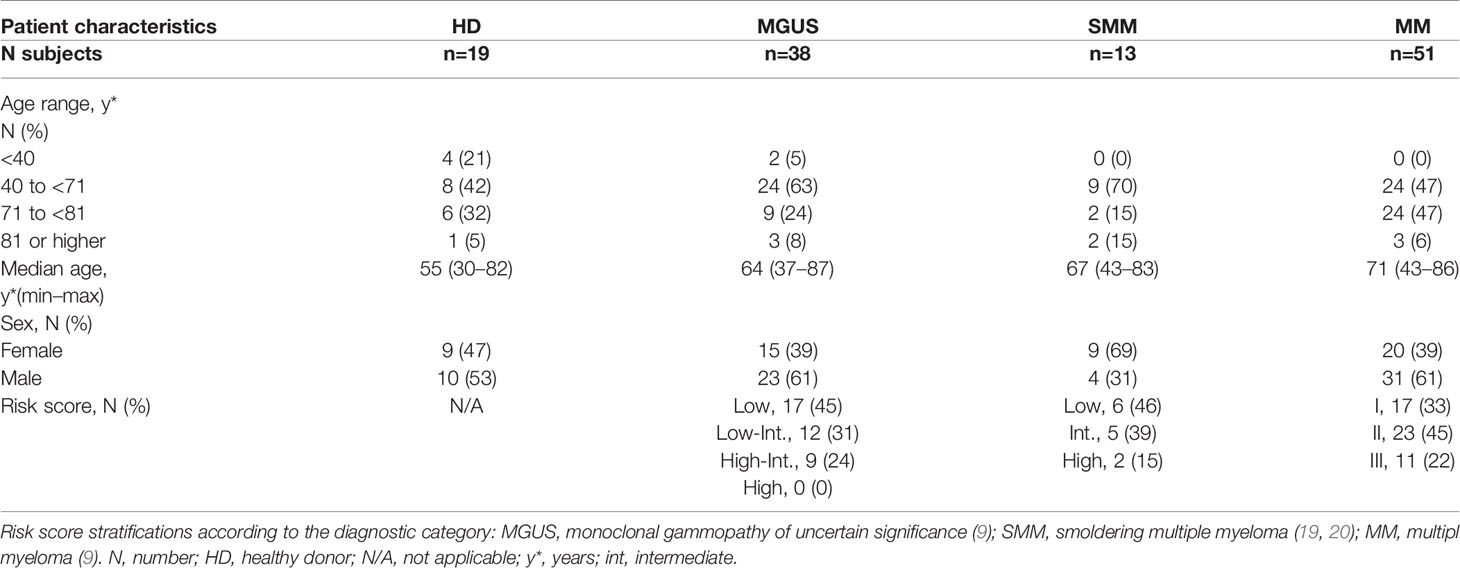
Table 1 Patient characteristics and distribution according to diagnostic category at study entrance.
3.2 Circulating EV Characterization
EV were isolated from 223 PB (circulating EV) and from 111 BM samples, and all were analyzed by NTA and BCA. Samples and EV characteristics according to MGUS, SMM, and MM (ND, NR, R) subgroups are described in Figure S1 and Table S1. The EV morphology and size were confirmed by TEM (Figure 1A). The specificity of isolated EV was confirmed by the presence of several conventional EV markers (3) by MS (Figure 1B). The purity of EV was confirmed by Western blot in representative samples, where higher CD9 and lower APOA1 expressions were observed in EV samples compared to the unpurified plasma samples of origin (Figure 1C). The average modal size of EV was <150 nm in all groups (Figure 1D), showing a specific enrichment of the samples in small EV. Using a mixed-effect model, no significant differences in EVc, EV protein, or particle concentrations were found, either for PB or BM samples (Figures 1E and S2A–F) between MGUS, SMM, MM-ND, MM-NR, and MM-R subgroups. There were no significant associations between the EVc level and age or sex (Pearson’s chi-squared test, p-value=0.139 and 0.527, respectively).
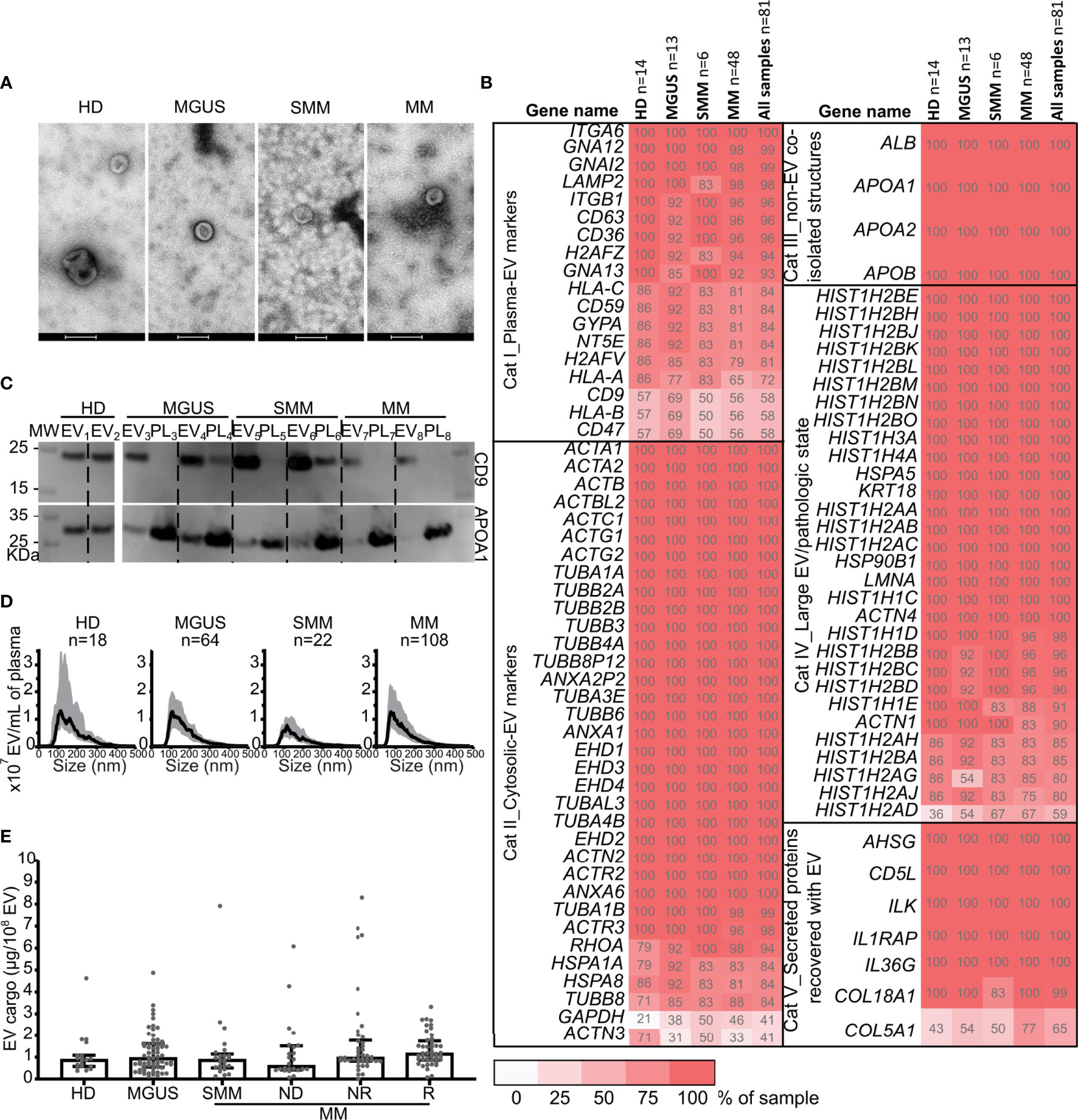
Figure 1 Characterization of extracellular vesicles from patient peripheral blood according to diagnostic category. (A) Transmission electron microscopy of circulating EV from representative samples from HD, MGUS, SMM, and MM samples. Scale bar, 200 nm. (B) Positivity for conventional protein markers identified by mass spectrometry across patients’ groups according to MISEV (3).The percentage of the samples expressing the specified protein is noted in each box. Dark red depicts a higher frequency. (C) Western blot analysis of CD9 and APOA-1 EV markers in EV samples and the source plasma (PL) sample. Two representative samples per group of diagnosis were tested. (D) Particle concentrations in plasma according to the size distribution from peripheral blood samples used in the present study (median and interquartile range). (E) EV cargo data distribution from peripheral blood samples according to the disease category at sample collection, HD (n=19 subjects, 19 samples), MGUS (n=38 patients, 67 samples), and MM (n=65 patients,137 samples): SMM (n=13 patients, 25 samples); ND (n=24 patients, 24 samples), NR, non-responder (n=32 patients, 42 samples), R, responder (n=27 patients, 46 samples). Using a linear mixed-effect model analysis, no significant differences between diagnostic categories (HD, MGUS, SMM, ND, NR, and R) were observed (p=0.39). HD, healthy donor; MGUS, monoclonal gammopathy of uncertain significance; SMM, smoldering multiple myeloma; NR, non-responder; R, responder, MM, multiple myeloma; EV, extracellular vesicle; PL, plasma; MW, molecular weight marker.
3.3 EV Protein Content
3.3.1 EV in PB as “Circulating” Counterpart of the BM
Direct comparisons were made between EV-PB and EV-BM from paired samples retrieved from the same patient. Among the 93 patients with paired PB and BM samples at study inclusion, a significant positive linear correlation was found in the EV protein concentration between PB and BM samples in MGUS (Spearman correlation r=0.63, p<0.001, n=37, Figure S2G) and MM patients (Spearman correlation r=0.77, p<0.001, n=56, Figure S2H). This means that a high protein concentration within circulating EV was associated with a high EV protein concentration in BM for both MGUS and MM patients, however, to a lesser extent in MGUS compared to MM.
Comparative EV protein profile analysis using LC-MS/MS results was performed in paired PB and BM samples from five patients (1 MGUS and 4 MM). An overall correlation of protein expression levels between the PB/BM pairs of 0.4 was found (Figure 2B). Then, the PB EV protein profile was compared to PB EV samples from other patients, matched for MM ND, R, and NR to understand the potential influence of the disease status in protein expression. Here, no correlation of protein expression levels between PB/PB-matched samples was observed (Figure 2A). This suggests a higher PB/BM proteomic expression similarity and supports the potential use of circulating EV as a personalized counterpart of the BM EV proteome. Interestedly, the major difference in proteomic expression between PB/BM-paired samples was in ferritin heavy chain 1 (FTH1), which was significantly more abundant in BM versus PB samples (T-test p-value=0.03, log2 FC=2.2, Figure 2C), corroborating the sample origin.
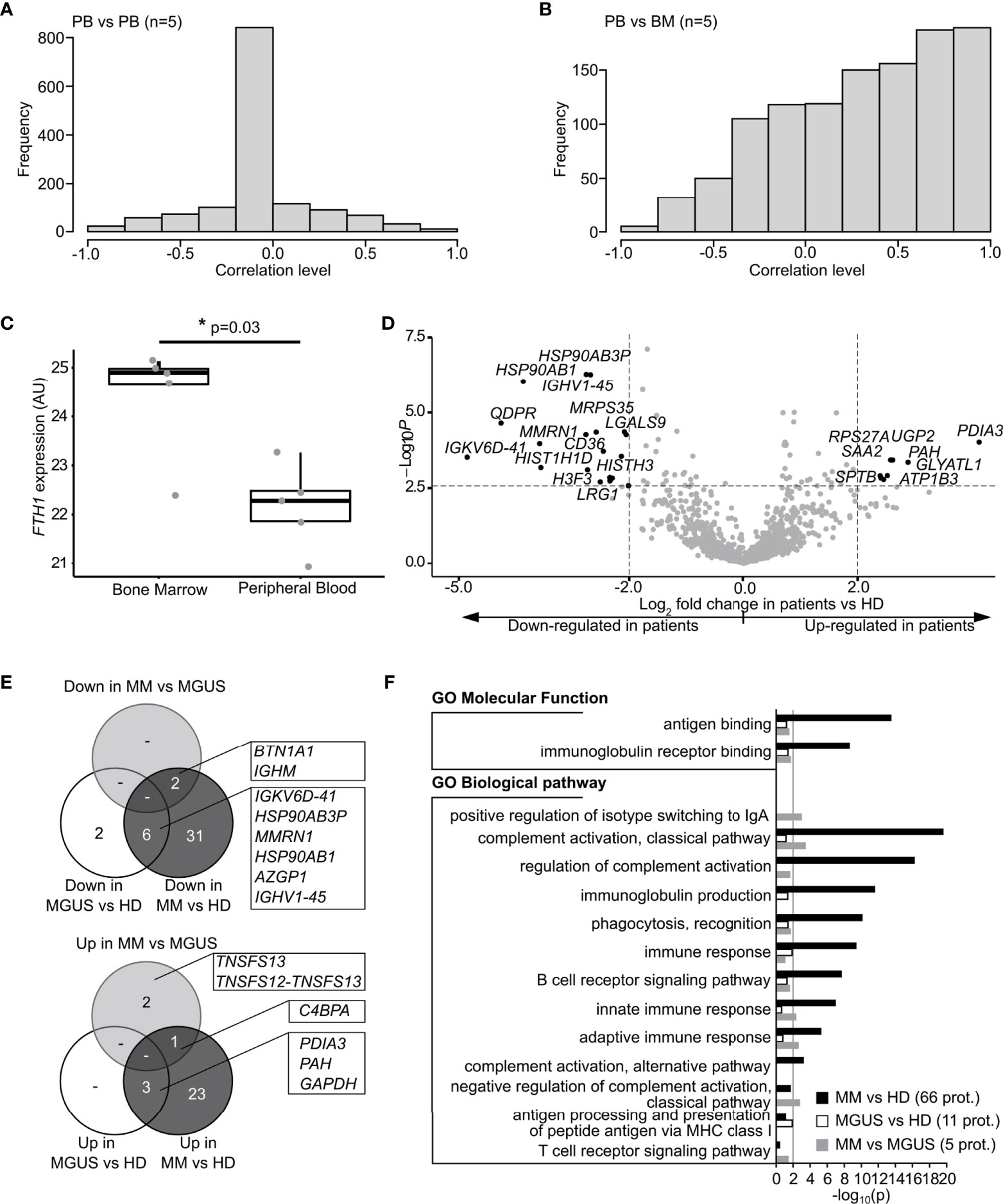
Figure 2 Characterization of proteins identified in EV from peripheral blood and bone marrow according to the diagnostic group. (A) Correlations between PB-matched samples from 5 pairs of patients (10 samples). Samples were matched considering the myeloma status at sample collection, existence of extramedullary disease, R-ISS status, number of previous treatment lines, and depth of response to treatment. The correlation for each protein is stored on as hyperbolic arctan (correlation). In this case, the correlations are equally distributed between positive and negative values but present a mode around 0. In the “limma” package, the consensus correlation is computed by discarding the most extreme outliers, averaging the remainder on the hyperbolic arctan scale, and then transforming back to a correlation. (B) Correlations between PB and BM samples collected from the same 5 patients at matched time points (10 samples). Correlation was computed as described in (A). For this case, the correlations are mainly positive and have a mode around 0.8. (C) Boxplot of iBAQ values obtained for Ferritin Heavy Chain 1 (FTH1). Matched PB and BM samples from 5 patients were compared (10 samples). The LC-MS/MS data were quantified by three different computational methods to ensure a consistent computational analysis: spectral counting, iBAQ quantitation based on ion counts, and iBAQ quantification based on ion counts using the match between runs. For all three analyses, FTH1 was significantly more abundant in BM samples, even after correction for multiple testing. (D) Volcano plot representation of differentially expressed proteins between all monoclonal gammopathy patients analyzed together and HD: differential expression of proteins above the horizontal line is significant with p-adjusted <0.05; differential expression of proteins outside the vertical dotted lines indicates a 2-logarithimical fold change (log2 FC) <-2 or >2. (E) Venn diagrams representing significant differentially expressed proteins of downregulated (top panel) and upregulated (bottom panel) proteins in peripheral blood-derived EV between patient subgroups (MM and MGUS) and HD. Proteins with overlap between the 3 comparisons (MM vs. MGUS, MGUS vs. HD, and MM vs. HD) are described in boxes. (F) Functional enrichment analysis of differentially expressed proteins using the FunRich software. Enrichment of immune-related molecular function and biological process was ranked for all deregulated proteins in MGUS and MM patient subgroups compared to healthy donors’ EV. PB, peripheral blood; R-ISS, revised international staging system; BM, bone marrow; AU, arbitrary unit; HD, healthy donor; MGUS, monoclonal gammopathy of uncertain significance; MM, multiple myeloma; EV, extracellular vesicle; prot, proteins.
3.3.2 EV Proteomic Expression in PB
EV proteomic expression was analyzed in a total of 51 patients with monoclonal gammopathies (MGs) including 13 MGUS, 5 SMM, and 33 MM.
First, a “disease”-blinded approach was performed by comparing EV proteomic expression in the PB of all the 51 MG patients together to HDs (n=14). Functional enrichment analysis using FunRich software (version3.1.3_March 2017) (21) against the Vesiclepedia database showed that 287 proteins were not annotated in this database. Interestingly, a higher enrichment in biological processes related to the immune response was observed in EV from all MG cohorts compared to the database. Within the 1,518 proteins identified, 82 were differently expressed in all MG patients compared to HDs (Benjamini–Hochberg-adjusted p-value <0.05) with 35 upregulated and 47 downregulated in patients. From these, the disulfide isomerase A3 precursor (PDIA3) required for protein folding in the endoplasmatic reticulum (22) had the highest positive fold change in MG patients, compared to HDs (Figure 2D). Comparisons between SMM and other disease status subgroups (MGUS, MM-ND, MM-NR, and MM-R) showed only the upregulation of collectin-11 (COLEC11) protein (log2 FC= 2.29) in patients responding to treatment, with no additional differences.
Considering this, we analyzed possible differences between HDs, MGUS, and MM (including SMM) and found that from the total of 5 proteins differentially expressed between MM and MGUS, Complement component 4 binding protein Alpha (C4BPA) protein was upregulated in MM patients (Figure 2E, bottom). C4bp is a regulator of complement activation that accelerates the decay of the classical pathway (23). Regarding the alternative pathway, Complement C3 (C3) and Complement factor H (CFH) were upregulated in MM vs. HDs, with a lower fold-change compared to the classical pathway. On the other hand, immunoglobulin heavy constant mu (IGHM) and butyrophilin subfamily 1 member A1 (BTN1A1) were downregulated in myeloma patients (Figure 2E, top). BTN1A1 contains immunomodulatory functions as a B7 family member (24). The tumor necrosis factor ligand superfamily member 13 or APRIL (TNFSF13) and TNFSF12-TNFSF13 were significantly upregulated in EV from MGUS patients when compared to myeloma patient EV. APRIL is usually secreted as a soluble molecule and binds with high affinity to the B-cell maturation antigen (BCMA), with higher serum levels in MM (25). Functional enrichment analysis was performed on differentially expressed proteins between groups (HD, MGUS, MM) focusing on molecular functions and biological processes related to immune functions as the most enriched categories. Differentially expressed proteins between HD, MGUS, and MM groups are strongly related to innate and adaptive immune responses. These enrichments were more pronounced when comparing MM vs. HDs, than MGUS vs. HDs, supporting a higher number of immune function alterations in MM patients (Figure 2F).
3.4 Circulating EV Cargo and Patient Outcomes
3.4.1 High EVc in Myeloma Patients Is Related to Lower Survival
The potential prognostic function of EV characteristics was investigated in the entire MG population with the intent of exploring them as new biomarkers independently of the disease category. These included the protein concentration, particle concentration, and protein/particle ratio (EVc). EV protein and particle concentrations had no impact in patient OS (Figures S3A, B, respectively). However, patients with EVc > 0.6 µg/108 particles (high EVc) had a significantly shorter OS compared to patients with EVc ≤ 0.6 µg/108 particles (low EVc), with survival probability at 25 months of 84% (95% CI: 0.75–0.94) vs. 97% (95% CI: 0.91–1), respectively (log-rank test, p-value=0.032, Figure 3A). Moreover, at 10% confidence level, the proposed cut-off point significantly differentiates MM patients with a better (low EVc) vs. poorer (high EVc) prognosis (Figure 3B, log-rank test, p-value=0.071). In detail, MM patients with high EVc presented a shorter probability of surviving beyond 25 months when compared to patients with low EVc (76% vs. 94%, respectively) with 90% CI equals to 0.66–0.88 and 0.84–1, respectively. Univariable and multivariable Cox proportional hazards models were developed to understand the role of EVc in the MM patient survival (Table S2). In addition to increased age, high β2-microglobulin, and MM-NR, patients with high EVc have a higher risk of death when compared to patients with low EVc (HR 12.23, p-value=0.028).
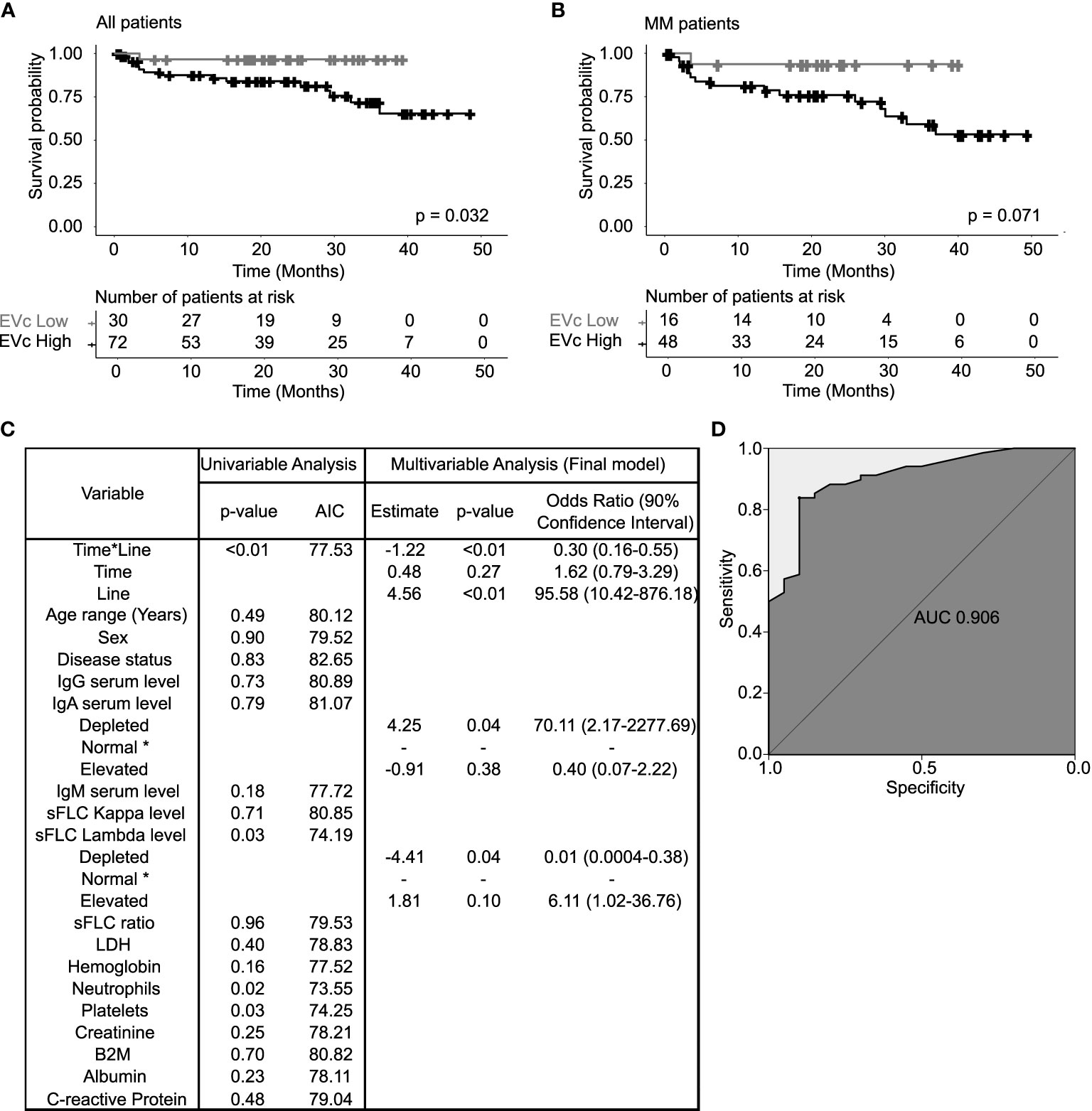
Figure 3 Extracellular vesicle cargo (EVc) characterization in terms of patients’ survival and longitudinal assessment. EVc, or protein-to-particle ratio, was defined by the ratio between the EV protein concentration and the EV particle concentration, for each EV sample. (A) Kaplan–Meier curves of patients’ overall survival (OS) according to the EVc level and diagnostic category. Applying the same method for optimal cut-off assessment as used for EV protein and particle concentration, MG patients (MGUS, SMM, and MM) with high EVc (>0.6 µg/108 EV) had a statistically significant lower OS when compared to patients with low EVc (≤0.6 µg/108 EV; log-rank test, p-value=0.032). (B) Comparing the specific EVc levels for MM (with SMM) patients, one can state that EVc High (>0.6 µg/108 particles) patients always present a worse prognosis than EVc Low (≤0.6 µg/108 particles) (considering a significance level of 10%: log-rank test, p-value=0.071). (C) Description of the variables tested to predict the odds of patients having a high EV cargo over time (SMM, MM-ND, MM-NR, and MM-R patients). Patient laboratory parameters obtained between 30 days before and 1 week after EV collection were used. Time—the chronological sequence of sample collection in each patient; line—the number of previous treatment lines. A variable of the interactions between time and line variables (time*line) was used to infer time in response (number of samples collected within the same line of treatment). The final model (multivariable analysis) resulted from a stepwise selection procedure, where variables were added one at a time and singularly tested in a univariable model. Wald test was used as a method for parameter significance testing. Model selection was performed through likelihood ratio test (LRT) computation, considering an inclusion/exclusion p-value set at 0.2 as described by Bendel and Afifi (17). IgA serum level, sFLC lambda level, and time*line were significant in the final model (p<0.1). (D) Final model goodness-of-fit graphical representation by the receiver operating characteristic (ROC) curve with an area under the curve (AUC) of 0.906. AIC (Akaike information criterion); * (reference class). sFLC, serum free light chain; B2M, β2microglobulin; LDH, lactate dehydrogenase.
3.4.2 High EVc in Myeloma Patients Can Be Predicted by Immunoparesis, High Serum Free Light Chain Level, and Shorter Time in Response
According to the results from the previous section, a multivariable longitudinal logistic regression model, estimating the odds of MM patients having a high EVc across the study time (for SMM, MM-ND, MM-NR, and MM-R) was built to determine if common myeloma-related blood parameters can explain the predictive value of EVc on MM OS (Figure 3C) in our cohort. Patient characteristics according to the EVc level are described in Table S3. IgA and sFLC lambda serum levels together with the time*line interaction were associated with high EVc, with an area under the ROC curve of 0.91 (Figure 3D). Patients with immunoparesis (IgA <50 mg/dl) had an increase of 70 times in the odds of high EVc compared to patients with normal IgA. Interestingly, among the 26 patients included in the model who presented IgA depletion, 22 were of the IgG subtype, suggesting that the immunoparesis of IgA was mostly associated to uninvolved Ig. Patients with elevated sFLC lambda (>27 mg/L) had an increase of 6 times in the odds of high EVc compared to patients with normal levels. Patients with low levels of sFLC lambda (<8.3 mg/L) presented an average reduction of 98% in the odds of having a high EVc compared to patients with normal levels. Patients with longer time in response showed an average decrease of 70% in the odds of having a high EVc compared to patients with a shorter response period. Furthermore, EV LC-MS/MS analysis was performed in a subgroup of 38 myeloma patients with high and low EVc (38 patients/51 samples) to explore the influence of patient serum IgA depletion and sFLC lambda elevation in the EV protein content. Interestingly, myeloma patients with IgA depletion had a significant downregulation of immunoglobulin heavy constant alpha 1 and 2 (IGHA1 and IGHA2) when compared to patients with normal and elevated levels of IgA. Furthermore, patients with sFLC lambda elevation had an upregulation of proteins related to the immunoglobulin lambda constant (IGLC1, IGLC2, IGLC3, and IGLC6); immunoglobulin lambda-like polypeptides (IGLL1 and IGLL5) and ferritin heavy chain 1 (FTH1), when compared to patients with the depletion of sFLC lambda.
4 Discussion
4.1 EV in a Real-World Study
To our knowledge, this is the first time that the EV protein content from an entire real-world MG patient cohort, prospectively followed in clinical practice for more than 2 years, is presented. The descriptive and comprehensive nature of our study generated a large set of real-world data from PB and BM EV derived from MG patients. In the 223 PB and 111 BM samples collected, the size and shape of the isolated particles were analyzed, and enrichment in EV markers was confirmed. Samples were tested for EV contaminants and were all coherent with purified-EV samples. The EV protein content from patient PB samples revealed a strong positive correlation with matched BM samples, as confirmed by proteomic expression. This is in line with the description of the BM proteome being more related to the blood proteome than to other tissues (26). However, in our study, BM samples were only collected from patients and therefore, this observation cannot be inferred to HDs. FTH1, the heavy chain of ferritin, a major intracellular iron storage protein, was found with higher expression in BM compared to PB samples, supporting the circulating EV protein content as liquid biopsies in MM. Considering this, we analyzed differences in PB EV-protein expression in a larger set of MG patients.
4.2 High EVc Level in Myeloma Patients Is Related to Poor Outcomes
In this work, we examined the extent to which EV characteristics, such as protein concentration, particle concentration, and the protein/particle ratio (EVc), could be a predictor of survival in patients with MG, regardless of the disease status allocation at study inclusion. Since the study of the EV characteristics in MG had not been conducted so far, there was no prior knowledge of their influence in combination with the disease status regarding the time to death. For that reason, we first investigated the optimal cutoff point better defining an MG patients’ prognosis by applying an outcome-oriented statistical method. We demonstrated for the first time that the EVc level is significantly associated with OS. We identified the value of 0.6 µg/108 particles as the optimal cut-off above which high EVc myeloma patients had a significantly shorter OS compared to patients with low EVc (≤0.6 µg/108 particles). As patients were included at any point in their disease history, the samples at study entry reflected the real-world heterogeneity of myeloma patients followed in clinical practice. Despite this cohort clinical diversity, circulating EVc alone was able to accurately identify patients with poor outcomes. This result was confirmed in a multivariable Cox regression model analysis accounting for age, β2-microglobulin, and disease status, ultimately indicating that myeloma patients with high EVc had 12 times increased risk of dying. Until now, the EVc has been typically described as quality control for EV purification (27) and, to our knowledge, this has not been associated with patient outcomes.
A multivariable logistic regression model fitted for longitudinal data was developed to determine whether common myeloma-related blood parameters could explain high EVc. Our model associated high EVc in MM patients with high sFLC lambda levels (>27 mg/L), immunoparesis (IgA <50 mg/dl), and shorter time in response. The utility of sFLC for the diagnosis, prognosis, and monitoring of MG is recognized in international guidelines (28). Extreme elevations of sFLC values and highly abnormal κ/λ ratios at the baseline are associated with refractory disease (29, 30) and are used to stratify SMM (19, 20). Our results confirmed the previously reported association of sFLC and EV (31–33). Interestingly, Di Noto et al. showed that endothelial and heart muscle cell lines reroute FLCs via EV (32) and that EV pre-treatment with anti-FLCs antibodies block MM EV uptake (31). Concerning IgA depletion, if immunoparesis was already associated with MM patient–reduced survival (34), its relation with circulating EV has not been described so far. Our results support EVc as a promising prognostic biomarker for MM patients, as it is associated with important prognostic features such as immunoparesis, sFLC, or the duration of the treatment response. To explore the potential biological link between high EVc and these immune alterations, LC-MS/MS was performed in circulating EV. The downregulation of IGHA1/IGHA confirmed IgA depletion in patients with immunoparesis. The upregulation of proteins related to Ig lambda production in patients with elevated sFLC lambda levels confirmed the increased presence of sFLC in these patients’ EV. EVc is an indirect and more affordable measure to infer EV protein load compared to mass spectrometry. After validation in larger and independent cohorts, EVc could be used as a first approach to predict patients with poor survival and select those at higher risk to be further analyzed by LC MS/MS.
4.3 Circulating EV as Source of New Myeloma Biomarkers
Eighty-two proteins were differentially expressed in MG patients compared to HDs. The functional analysis of differentially expressed proteins unraveled circulating EV as potential new tools for the dynamic interception of cellular communications in MM. Most downregulated proteins were involved in innate and adaptive immune responses, as proteins expressed in physiological conditions are lost in immune impaired myeloma patients (35, 36). EV are known to play important roles in immune phenomena (37), such as those from dendritic and B cells carrying major histocompatibility complex class (MHC), co-stimulatory, and adhesion molecules (38–40). In MM, the proteomic analyses of EV from MM cell lines and patient serum showed that MHC-I and β2-microglobulin were the most abundant enriched proteins (41).
Here, we describe a set of specific proteins from MG patients circulating EV. We propose PDIA3 as a potential disease biomarker for MG, present since the MGUS stage. PDIA3 codes the protein disulfide isomerase (PDI)–like family (22, 42). In cancer, PDI expression is upregulated in various tumors with poor outcomes (43). PDIA3 is involved in the antigen presentation pathway of folding, assembly, and peptide loading of MHC-I (42). With the inhibition of PDI in the endoplasmic reticulum (ER), increasing ER stress could be a new strategy for MM treatment (44). A recent pan-PDI inhibitor enhanced the cytotoxic effects of proteasome inhibitors in MM models (45). Together with our results, circulating EV-derived PDIA3 could be potentially used for PDI inhibition treatment monitoring. Additionally, we showed that immunomodulatory functions were associated to MG-specific disease stages. BTN1A1 codes a protein related to the B7 family of costimulatory molecules and was significantly downregulated in myeloma patients, compared to HDs and MGUS. Conversely, myeloma patients presented a significant and consistent upregulation of C4BPA, a regulator of complement activation that accelerates the decay of the classical pathway. The sequestration of plasma regulators, such as C4BPA, by tumor cells has been shown as a mechanism to prevent complement-dependent cytotoxicity (23, 46). Complement activation is an important cause of inflammation involved in tumor progression (47). The expression of EV proteins such as IGHM and C4BPA, related to the classical pathway, suggest an alteration of this pathway in MGUS and to a greater extent in MM, compared to HDs. A proteomic analysis of MM patient serum samples reported the upregulation of complement activation proteins, notably C4B (48), which is also upregulated in our study. Surprisingly, we found a significant upregulation of APRIL, the natural ligand for BCMA, and TWE-PRIL proteins in MGUS patients’ EV compared to MM EV. This suggests a potential loss of expression in EV during disease progression, which may be related to APRIL higher increase in the serum of MM patients. Indeed, other studies have shown an increase of serum APRIL levels in patients with MM (49). In our study, only the EV protein content was analyzed, as opposed to the entire patient serum. Based on our results, one can hypothesize that “richer” EV-derived APRIL in MGUS patients can be shed off from EV upon disease progression to MM (with “poorer” EV-derived APRIL). This hypothetical release of APRIL in MM from EV to serum could be directly related to the increase of malignant plasma cells and microenvironment cells that overexpress APRIL receptors upon disease progression. If confirmed, this has the potential to be an early biomarker of disease evolution. Our findings show that EV-derived proteins (PDIA3, BTN1A1, APRIL) and complement-associated proteins could be further explored in myeloma, as biomarkers across disease natural history and as potential new drug targets that could be monitored by PB sampling. To identify tumor-specific proteins as previously done (50), we selected the proteins that were commonly differentially expressed in matched pairs of BM and PB of MM patients vs. the PB of HDs. A total of 228 upregulated and 266 downregulated proteins in MM vs. HDs, that are potentially tumor specific were identified. Among them, we could find C4BPA, IGHM, and BTN1A1 that we previously mentioned as being specifically related to MM patients, suggesting that they could be directly produced by the tumor. However, due to the limited sample size, this needs to be confirmed.
Of note, preliminary results on a small number of patients suggest the presence of upregulated proteins in MM patients resistant to the bortezomib–lenalidomide–dexamethasone (VRD) regimen when compared to patients resistant to autologous stem cell transplant or to MM patients resistant to daratumumab-based regimens (data not shown). Among these, 17 proteins were constantly upregulated in patients resistant to VRD, including the proteasome subunit beta proteins (PSM8 and PSMB8). In patients resistant to the daratumumab regimen the high-affinity Camp-specific and IBMX-insensitive 3’,5’-cyclic phosphodiesterase 8B (PDE8B) was also significantly upregulated when compared to patients resistant to VRD. Despite being preliminary, these observations suggest that EV proteins have the potential to be used as the biomarkers of drug resistance and that further investigation is worthy. In the literature, the replacement of PSMB5 by PSMB8 is described as increasing the cleavage capacity of the immunoproteasome peptides (51). It is also known that daratumumab treatment modulates the enzymatic activity of CD38 by reducing the adenosine levels and that PDE8B is a regulator of cyclic adenosine monophosphate. Therefore, the upregulation of PDE8B as a potential via the resistance to the immunomodulatory effect of daratumumab through adenosine reduction (52) is worthy of further study.
5 Conclusions
In this work, we thoroughly describe EV protein content from real-world MG patients. We found that the level of circulating EVc (protein/particle ratio, easily obtained by BCA and NTA) can be related to patients’ poorer outcomes including OS, high sFLC, immunoparesis, and shorter time in response. By mass spectrometry analysis, we also report new candidate biomarkers that can be associated with different disease stages. Altogether, our results corroborate the pursuit of EV as new liquid biopsies in myeloma and future validation in independent clinical settings is urged.
Data Availability Statement
The original contributions presented in the study are publicly available. This data can be found here: https://www.ebi.ac.uk/pride/archive/projects/PXD024121.
Ethics Statement
The studies involving human participants were reviewed and approved by Champalimaud Foundation and Centro Hospitalar de Lisboa Ocidental Ethics Committees, respectively and by the Portuguese National Committee for Data Protection. The patients/participants provided their written informed consent to participate in this study.
Author Contributions
Conceptualization, BF, CJ, and BC-S. Methodology, BF, EC, CP, RM, and CJ. Formal analysis, BF, EC, CP, FB, RL, PL, MN, BC-S, and CJ. Investigation, BF, EC, FB, JC, RL, HB, AC, and RM. Resources, HB, RM, and AC. Data curation, EC, FB, PL, MN, and CJ. Writing—original draft preparation, BF, EC, CP, MN, PL, BC-S, and CJ. Writing—review and editing, BF, EC, BC-S, and C.J. Supervision, BF and CJ. Project administration, CJ. Funding acquisition, BF and CJ. All authors have read and agreed to the published version of the manuscript.
Funding
This research was funded by the Champalimaud Foundation; by the Fundação para a Ciência e Tecnologia – FCT (Research Grant PTDC/MEC-HEM/30315/2017) and by Sociedade Portuguesa de Hematologia -SPH (Initiation to Investigation Grant 2018).
Conflict of Interest
The authors declare that the research was conducted in the absence of any commercial or financial relationships that could be construed as a potential conflict of interest.
Publisher’s Note
All claims expressed in this article are solely those of the authors and do not necessarily represent those of their affiliated organizations, or those of the publisher, the editors and the reviewers. Any product that may be evaluated in this article, or claim that may be made by its manufacturer, is not guaranteed or endorsed by the publisher.
Acknowledgments
The authors want to first acknowledge the contribution of all study participants, patients, and their families to this research. We thank the nurses, staff, and investigators from the clinical centers who participated in the care of these patients. We also acknowledge the collaboration of Professor Salomé Cabral from the Faculdade de Ciências, Universidade de Lisboa for the support with the multivariable logistic regression model for binary longitudinal data construction, and M.J. Almeida and E.M. Tranfield from the Electron Microscopy Facility at the Instituto Gulbenkian de Ciência for the technical expertise, sample processing, and imaging of our extracellular vesicles.
Supplementary Material
The Supplementary Material for this article can be found online at: https://www.frontiersin.org/articles/10.3389/fonc.2022.860849/full#supplementary-material
References
1. Rajkumar SV, Dimopoulos MA, Palumbo A, Blade J, Merlini G, Mateos MV, et al. International Myeloma Working Group Updated Criteria for the Diagnosis of Multiple Myeloma. Lancet Oncol (2014) 15(12):e538–48. doi: 10.1016/S1470-2045(14)70442-5
2. Rasche L, Chavan SS, Stephens OW, Patel PH, Tytarenko R, Ashby C, et al. Spatial Genomic Heterogeneity in Multiple Myeloma Revealed by Multi-Region Sequencing. Nat Commun (2017) 8(1):268. doi: 10.1038/s41467-017-00296-y
3. Théry C, Witwer KW, Aikawa E, Alcaraz MJ, Anderson JD, Andriantsitohaina R, et al. Minimal Information for Studies of Extracellular Vesicles 2018 (MISEV2018): A Position Statement of the International Society for Extracellular Vesicles and Update of the MISEV2014 Guidelines. J Extracell Vesicles (2018) 7(1):1535750. doi: 10.1080/20013078.2018.1535750
4. Ferreira B, Caetano J, Barahona F, Lopes R, Carneiro E, Costa-Silva B, et al. Liquid Biopsies for Multiple Myeloma in a Time of Precision Medicine. J Mol Med (2020) 98(4):513–25. doi: 10.1007/s00109-020-01897-9
5. Maia J, Caja S, Carolina M, Moraes S, Couto N, Costa-silva B. Exosome-Based Cell-Cell Communication in the Tumor Microenvironment. Front Cell Dev Biol (2018) 6:1–19. doi: 10.3389/fcell.2018.00018
6. Roccaro AM, Sacco A, Maiso P, Azab AK, Tai YT, Reagan M, et al. BM Mesenchymal Stromal Cell-Derived Exosomes Facilitate Multiple Myeloma Progression. J Clin Invest (2013) 123(4):1542–55. doi: 10.1172/JCI66517
7. Wang J, De Veirman K, De Beule N, Maes K, De Bruyne E, Valckenborgh EV, et al. The Bone Marrow Microenvironment Enhances Multiple Myeloma Progression by Exosome-Mediated Activation of Myeloid-Derived Suppressor Cells. Oncotarget (2015) 6(41):43992–4004. doi: 10.18632/oncotarget.6083
8. Zhang L, Pan L, Xiang B, Zhu H, Wu Y, Chen M, et al. Potential Role of Exosome-Associated microRNA Panels and In Vivo Environment to Predict Drug Resistance for Patients With Multiple Myeloma. Oncotarget (2014) 7(21):30876–91. doi: 10.18632/oncotarget.9021
9. Rajkumar SV. Multiple Myeloma: 2020 Update on Diagnosis, Risk-Stratification and Management. Am J Hematol (2020) 95(5):548–67. doi: 10.1002/ajh.25791
10. Maia J, Batista S, Couto N, Gregório AC, Bodo C, Elzanowska J, et al. Employing Flow Cytometry to Extracellular Vesicles Sample Microvolume Analysis and Quality Control. Front Cell Dev Biol (2020) 8:593750. doi: 10.3389/fcell.2020.593750
11. de Boeck P, Bakker M, Zwitser R, Nivard M, Hofman A, Tuerlinckx F, et al. The Estimation of Item Response Models With the Lmer Function From the Lme4 Package in R. J Stat Software (2011) 39(12):1–28. doi: 10.18637/jss.v039.i12
12. Bates D, Maechler M, Bolker B, Walker S. Fitting Linear Mixed-Effects Models Using lme4. J Stat Software (2015) 67(1):1–48. doi: 10.18637/jss.v067.i01
14. Kassambara A, Kosinski M, Biecek P. Survminer: Drawing Survival Curves Using “Ggplot2”. In: R Package Version 0.4.9 (2021). Available at: https://CRAN.R-project.org/package=survminer.
15. Gonçalves MH, Cabral MS, Azzalini A. A Package for BInary Longitudinal Data, Version 1.2-0 (2020). Available at: https://CRAN.R-project.org/package=bild.
16. Therneau T. A Package for Survival Analysis in R. In: R Package Version 3.2-10 (2021). Available at: https://CRAN.R-project.org/package=survival.
17. Bendel RB, Afifi AA. Comparison of Stopping Rules in Forward “Stepwise” Regression. J Am Stat Assoc Taylor Francis Group (1977) 72(357):46–53. doi: 10.1080/01621459.1977.10479905
18. R Core Team. R: A Language and Environment for Statistical Computing. Vienna, Austria: R Foundation for Statistical Computing. (2021).
19. Lakshman A, Vincent Rajkumar S, Buadi FK, Binder M, Gertz MA, Lacy MQ, et al. Risk Stratification of Smoldering Multiple Myeloma Incorporating Revised IMWG Diagnostic Criteria. Blood Cancer J (2018) 8(6):59. doi: 10.1038/s41408-018-0077-4
20. Mateos M-V, Kumar S, Dimopoulos MA, González-Calle V, Kastritis E, Hajek R, et al. International Myeloma Working Group Risk Stratification Model for Smoldering Multiple Myeloma (SMM). Blood Cancer J (2020) 10(10):102. doi: 10.1038/s41408-020-00366-3
21. Pathan M, Keerthikumar S, Chisanga D, Alessandro R, Ang CS, Askenase P, et al. A Novel Community Driven Software for Functional Enrichment Analysis of Extracellular Vesicles Data. J Extracell Vesicles (2017) 6(1):1321455. doi: 10.1080/20013078.2017.1321455
22. Galligan JJ, Petersen DR. The Human Protein Disulfide Isomerase Gene Family. Hum Genomics Henry Stewart Publications (2012) 6(1):6. doi: 10.1186/1479-7364-6-6
23. Reis ES, Mastellos DC, Ricklin D, Mantovani A, Lambris JD. Complement in Cancer: Untangling an Intricate Relationship. Nat Rev Immunol (2018) 18(1):5–18. doi: 10.1038/nri.2017.97
24. Arnett HA, Viney JL. Immune Modulation by Butyrophilins. Nat Rev Immunol (2014) 14(8):559–69. doi: 10.1038/nri3715
25. Rennert P, Schneider P, Cachero TG, Thompson J, Trabach L, Hertig S, et al. A Soluble Form of B Cell Maturation Antigen, a Receptor for the Tumor Necrosis Factor Family Member April, Inhibits Tumor Cell Growth. J Exp Med (2000) 192(11):1677–84. doi: 10.1084/jem.192.11.1677
26. Available at: https://www.proteinatlas.org/humanproteome/tissue/bone+marrow.
27. Webber J, Clayton A. How Pure are Your Vesicles? J Extracell Vesicles (2013) 2(1). doi: 10.3402/jev.v2i0.19861
28. Dispenzieri A, Kyle R, Merlini G, Miguel JS, Ludwig H, Hajek R, et al. International Myeloma Working Group Guidelines for Serum-Free Light Chain Analysis in Multiple Myeloma and Related Disorders. Leukemia (2009) 23(2):215–24. doi: 10.1038/leu.2008.307
29. Chilkulwar A, Mewawalla P, Miller A, Berteotti G, Sahovic E, Lister J. Serum Free Light Chain Concentration (>1000mg/Dl) at Diagnosis and at Relapse Predicts for Very Poor Prognosis in Multiple Myeloma. Blood. Am Soc Hematol (2016) 128(22):5698–8. doi: 10.1182/blood.V128.22.5698.5698
30. Du J, Lu J, Gao W, Liu J, He H, Li L, et al. Serum-Free Light Chains Combined With the Revised International Staging System Could Further Distinguish the Superior and Inferior Clinical Outcome of Multiple Myeloma Patients. Ann Hematol Springer (2020) 99(8):1779–91. doi: 10.1007/s00277-020-04162-8
31. Di Noto GG, Chiarini M, Paolini LL, Mazzoldi EL, Giustini V, Radeghieri A, et al. Immunoglobulin Free Light Chains and GAGs Mediate Multiple Myeloma Extracellular Vesicles Uptake and Secondary Nfκb Nuclear Translocation. Front Immunol (2014) 5. doi: 10.3389/fimmu.2014.00517
32. Di Noto G, Paolini L, Zendrini A, Radeghieri A, Caimi L, Ricotta D. C-Src Enriched Serum Microvesicles Are Generated in Malignant Plasma Cell Dyscrasia. PloS One (2013) 8(8):e70811. doi: 10.1371/journal.pone.0070811
33. Ramirez-Alvarado M, Ward CJ, Huang BQ, Gong X, Hogan MC, Madden BJ, et al. Differences in Immunoglobulin Light Chain Species Found in Urinary Exosomes in Light Chain Amyloidosis (Al). PloS One (2012) 7(6):e38061. doi: 10.1371/journal.pone.0038061
34. Ludwig H, Milosavljevic D, Berlanga O, Zojer N, Hübl W, Fritz V, et al. Suppression of the Noninvolved Pair of the Myeloma Isotype Correlates With Poor Survival in Newly Diagnosed and Relapsed/Refractory Patients With Myeloma. Am J Hematol Wiley-Liss Inc. (2016) 91(3):295–301. doi: 10.1002/ajh.24268
35. de Magalhães RJP, Vidriales MB, Paiva B, Fernandez-Gimenez C, García-Sanz R, Mateos MV, et al. Analysis of the Immune System of Multiple Myeloma Patients Achieving Long-Term Disease Control by Multidimensional Flow Cytometry. Haematologica Haematologica (2013) 98(1):79–86. doi: 10.3324/haematol.2012.067272
36. Racanelli V, Leone P, Frassanito MA, Brunetti C, Perosa F, Ferrone S, et al. Alterations in the Antigen Processing-Presenting Machinery of Transformed Plasma Cells are Associated With Reduced Recognition by CD8+ T Cells and Characterize the Progression of MGUS to Multiple Myeloma. Blood. Blood (2010) 115(6):1185–93. doi: 10.1182/blood-2009-06-228676
37. Li XB, Zhang ZR, Schluesener HJ, Xu SQ. Role of Exosomes in Immune Regulation. J Cell Mol Med J Cell Mol Med (2006) 10(2):364–75. doi: 10.1111/j.1582-4934.2006.tb00405.x
38. Clayton A, Court J, Navabi H, Adams M, Mason MD, Hobot JA, et al. Analysis of Antigen Presenting Cell Derived Exosomes, Based on Immuno-Magnetic Isolation and Flow Cytometry. J Immunol Methods J Immunol Methods (2001) 247(1–2):163–74. doi: 10.1016/S0022-1759(00)00321-5
39. Escola JM, Kleijmeer MJ, Stoorvogel W, Griffith JM, Yoshie O, Geuze HJ. Selective Enrichment of Tetraspan Proteins on the Internal Vesicles of Multivesicular Endosomes and on Exosomes Secreted by Human B-Lymphocytes. J Biol Chem J Biol Chem (1998) 273(32):20121–7. doi: 10.1074/jbc.273.32.20121
40. Raposo G, Nijman HW, Stoorvogel W, Leijendekker R, Harding CV, Melief CJM, et al. B Lymphocytes Secrete Antigen-Presenting Vesicles. J Exp Med J Exp Med (1996) 183(3):1161–72. doi: 10.1084/jem.183.3.1161
41. Harshman SW, Canella A, Ciarlariello PD, Agarwal K, Branson OE, Rocci A, et al. Proteomic Characterization of Circulating Extracellular Vesicles Identifies Novel Serum Myeloma Associated Markers. J Proteomics (2016) 136:89–98. doi: 10.1016/j.jprot.2015.12.016
42. Zhang Y, Baig E, Williams DB. Functions of ERp57 in the Folding and Assembly of Major Histocompatibility Complex Class I Molecules. J Biol Chem J Biol Chem (2006) 281(21):14622–31. doi: 10.1074/jbc.M512073200
43. Xu S, Sankar S, Neamati N. Protein Disulfide Isomerase: A Promising Target for Cancer Therapy. Drug Discov Today (2014) 19(3):222–40. doi: 10.1016/j.drudis.2013.10.017
44. Vatolin S, Phillips JG, Jha BK, Govindgari S, Hu J, Grabowski D, et al. Novel Protein Disulfide Isomerase Inhibitor With Anticancer Activity in Multiple Myeloma. Cancer Res (2016) 76(11):3340–50. doi: 10.1158/0008-5472.CAN-15-3099
45. Robinson RM, Reyes L, Duncan RM, Bian H, Strobel ED, Hyman SL, et al. Tuning Isoform Selectivity and Bortezomib Sensitivity With a New Class of Alkenyl Indene PDI Inhibitor. Eur J Med Chem (2020) 186:111906. doi: 10.1016/j.ejmech.2019.111906
46. Holmberg MT, Blom AM, Meri S. Regulation of Complement Classical Pathway by Association of C4b-Binding Protein to the Surfaces of SK-OV-3 and Caov-3 Ovarian Adenocarcinoma Cells. J Immunol (2001) 167(2):935 LP–939. doi: 10.4049/jimmunol.167.2.935
47. Bonavita E, Gentile S, Rubino M, Maina V, Papait R, Kunderfranco P, et al. PTX3 Is an Extrinsic Oncosuppressor Regulating Complement-Dependent Inflammation in Cancer. Cell (2015) 160(4):700–14. doi: 10.1016/j.cell.2015.01.004
48. Chanukuppa V, Taware R, Taunk K, Chatterjee T, Sharma S, Somasundaram V, et al. Proteomic Alterations in Multiple Myeloma: A Comprehensive Study Using Bone Marrow Interstitial Fluid and Serum Samples. Front Oncol (2021) 10:566804. doi: 10.3389/fonc.2020.566804
49. Lemancewicz D, Bolkun L, Jablonska E, Kulczynska A, Bolkun-Skornicka U, Kloczko J, et al. Evaluation of TNF Superfamily Molecules in Multiple Myeloma Patients: Correlation With Biological and Clinical Features. Leuk Res (2013) 37(9):1089–93. doi: 10.1016/j.leukres.2013.05.014
50. Hoshino A, Kim HS, Bojmar L, Gyan KE, Cioffi M, Hernandez J, et al. Extracellular Vesicle and Particle Biomarkers Define Multiple Human Cancers. Cell (2020) 182(4):1044–61. doi: 10.1016/j.cell.2020.07.009
51. National Center for Biotechnology Information. PubChem Protein Summary for NCBI Protein P28062, Proteasome Subunit Beta Type-8 (2021). Available at: https://pubchem.ncbi.nlm.nih.gov/protein/P28062.
52. Saltarella I, Desantis V, Melaccio A, Solimando AG, Lamanuzzi A, Ria R, et al. Mechanisms of Resistance to Anti-CD38 Daratumumab in Multiple Myeloma. Cells (2020). doi: 10.3390/cells9010167
53. Deutsch EW, Csordas A, Sun Z, Jarnuczak A, Perez-Riverol Y, Ternent T, et al. The ProteomeXchange Consortium in 2017: Supporting the Cultural Change in Proteomics Public Data Deposition. Nucleic Acids Res (2017) 45(D1):D1100–6. doi: 10.1093/nar/gkw936
Keywords: extracellular vesicles (EV), multiple myeloma, biomarkers, protein, liquid biopsy
Citation: Ferreira BV, Carneiro EA, Pestana C, Barahona F, Caetano J, Lopes R, Lúcio P, Neves M, Beck HC, Carvalho AS, Matthiesen R, Costa-Silva B and João C (2022) Patient-Derived Extracellular Vesicles Proteins as New Biomarkers in Multiple Myeloma - A Real-World Study. Front. Oncol. 12:860849. doi: 10.3389/fonc.2022.860849
Received: 23 January 2022; Accepted: 16 May 2022;
Published: 21 June 2022.
Edited by:
J. Luis Espinoza, Kanazawa University, JapanReviewed by:
Antonia Reale, Monash University, AustraliaXiaoxing Kou, Sun Yat-sen University, China
Denise Toscani, University of Parma, Italy
Copyright © 2022 Ferreira, Carneiro, Pestana, Barahona, Caetano, Lopes, Lúcio, Neves, Beck, Carvalho, Matthiesen, Costa-Silva and João. This is an open-access article distributed under the terms of the Creative Commons Attribution License (CC BY). The use, distribution or reproduction in other forums is permitted, provided the original author(s) and the copyright owner(s) are credited and that the original publication in this journal is cited, in accordance with accepted academic practice. No use, distribution or reproduction is permitted which does not comply with these terms.
*Correspondence: Bruno Costa-Silva, YnJ1bm8uY29zdGEtc2lsdmFAcmVzZWFyY2guZmNoYW1wYWxpbWF1ZC5vcmc=; Cristina João, Y3Jpc3RpbmEuam9hb0ByZXNlYXJjaC5mY2hhbXBhbGltYXVkLm9yZw==