- 1Henan Provincial People’s Hospital, Zhengzhou University, Henan, China
- 2College of Public Health, Zhengzhou University, Henan, China
Objective: Whether fasting insulin (FI) plays a role in cancer risk remains unclear. This study aimed to investigate the association between FI and cancer risk and to explore its potential mediator role in the association between type 2 diabetes mellitus (T2DM) and cancer.
Methods: Two-sample Mendelian randomization (TSMR) analysis was performed to evaluate the effect of FI on overall and 14 site-specific cancers using genome-wide association study (GWAS) summary-level data from Meta-Analyses of Glucose and Insulin-related traits Consortium (MAGIC) and consortia of 14 site-specific cancers. The primary MR approach was conducted by using the random-effect inverse-variance weighted (IVW) method, and sensitivity analyses were implemented by adopting weighted-median, weighted-mode, MR-Egger, and MR-PRESSO tests. Polygenic risk score analysis was executed by using individual-level data from UK Biobank to validate the findings from TSMR analyses. Multivariable Mendelian randomization (MVMR) was carried out to estimate the mediation effect of FI on the association between T2DM and cancer.
Results: TSMR study suggested that genetically determined high FI levels were associated with increased risk of colorectal cancer (odds ratio (OR) = 1.87, 95% CI: 1.23–2.84, p = 0.003) and endometrial cancer (OR = 1.89, 95% CI: 1.08–3.01, p = 0.008), but not associated with overall cancer risk or the other 12 studied cancer sites. Polygenic risk score analysis successfully replicated the association between genetic liability to high FI levels and the increased risk of colorectal and endometrial cancers. MVMR and MR mediation analyses detected an intermediary effect of FI and quantified that FI mediated 21.3% of the association between T2DM and endometrial cancer.
Conclusions: This study demonstrated that FI levels are associated with the risk of colorectal and endometrial cancers, and FI was found to play an intermediary role in the association between T2DM and endometrial cancer. The associations between FI and other cancers need to be further studied.
Introduction
Fasting insulin (FI), a peptide hormone, plays an indispensable role in regulating blood glucose and energy metabolism. Evidence from observational studies has indicated that elevated FI levels may be related to increased risk of overall cancer (1) and site-specific cancers, such as breast (2), colorectal (3), prostate (4, 5), pancreatic (6, 7), kidney (8), uterine (9), gastric (10), lung (11), and liver cancers (12). Besides, a comprehensive meta-analysis of observational studies has demonstrated that type 2 diabetes mellitus (T2DM) was associated with developing several cancers (13), including hepatocellular, hepatobiliary, pancreas, breast, ovarian, endometrial, and gastrointestinal cancers. However, because of the possible residual confounding and reverse association of these observational studies, the effects of FI and T2DM on cancer risk are not fully established. Furthermore, although several studies have reported possible mechanisms underlying diabetes and cancer, such as hyperglycemia (14), hyperinsulinemia (15), and increased activity of insulin-like growth factor 1(IGF-1) (16), the role of FI in the association between T2DM and cancer remains unclear. Considering that FI levels can be modified by diet control (17, 18) and medical therapy (19), establishing the association between FI and cancer risk and exploring its effect on the association between T2DM and associated cancer are crucial from clinical and public health perspectives.
Compared with conventional observational studies, Mendelian randomization (MR) study, by exploiting genetic variants (usually single-nucleotide polymorphisms (SNPs)) as instrumental variables (IVs) of the exposure, can enhance the exposure–outcome inference by diminishing possible confounding and avoiding the reverse correlation (20, 21). This method minimizes confounding because genetic variants are randomly assorted at conception and therefore have nothing to do with self-adapted lifestyle and environmental factors. Since allelic randomization precedes the occurrence of diseases, the MR framework can also overcome inverse association. Recently, this method has been extended to multivariable MR (MVMR) (22, 23), which can be used to evaluate the independent direct effects of multiple and potentially related exposures, as well as to estimate the proportion of the exposure–outcome effect mediated by a mediator or set of mediators when combined with MR (24).
Given the inconclusive evidence and natural methodological limitations of previous observational studies on FI levels and cancer risk, the primary objective of this study is to determine the association between FI levels and the risk of overall and 14 site-specific cancers by conducting two-sample MR (TSMR) framework and polygenic risk score (PRS) analyses. Furthermore, we performed MVMR and MR mediation analyses to explore the mediation effect of FI on the association between T2DM and cancer risk.
Methods
Study Design
To assess the associations between FI and the risk of overall cancer and 14 site-specific cancers as well as the possibly intermediary effect of FI on the association between T2DM and cancer, a TSMR framework was first performed based on genome-wide association study (GWAS) summary-level data from several consortia for overall cancer and site-specific cancers. Then PRS analysis using individual-level data from UK Biobank was conducted to further validate the associations between genetically determined FI levels and cancer risk. Finally, the MVMR and mediation analyses were carried out to explore the potential intermediary effect of FI on the association between T2DM and cancer. Individual-level UK Biobank data were accessed under the approved project application (ID: 66354). The study design is presented in Figure 1.
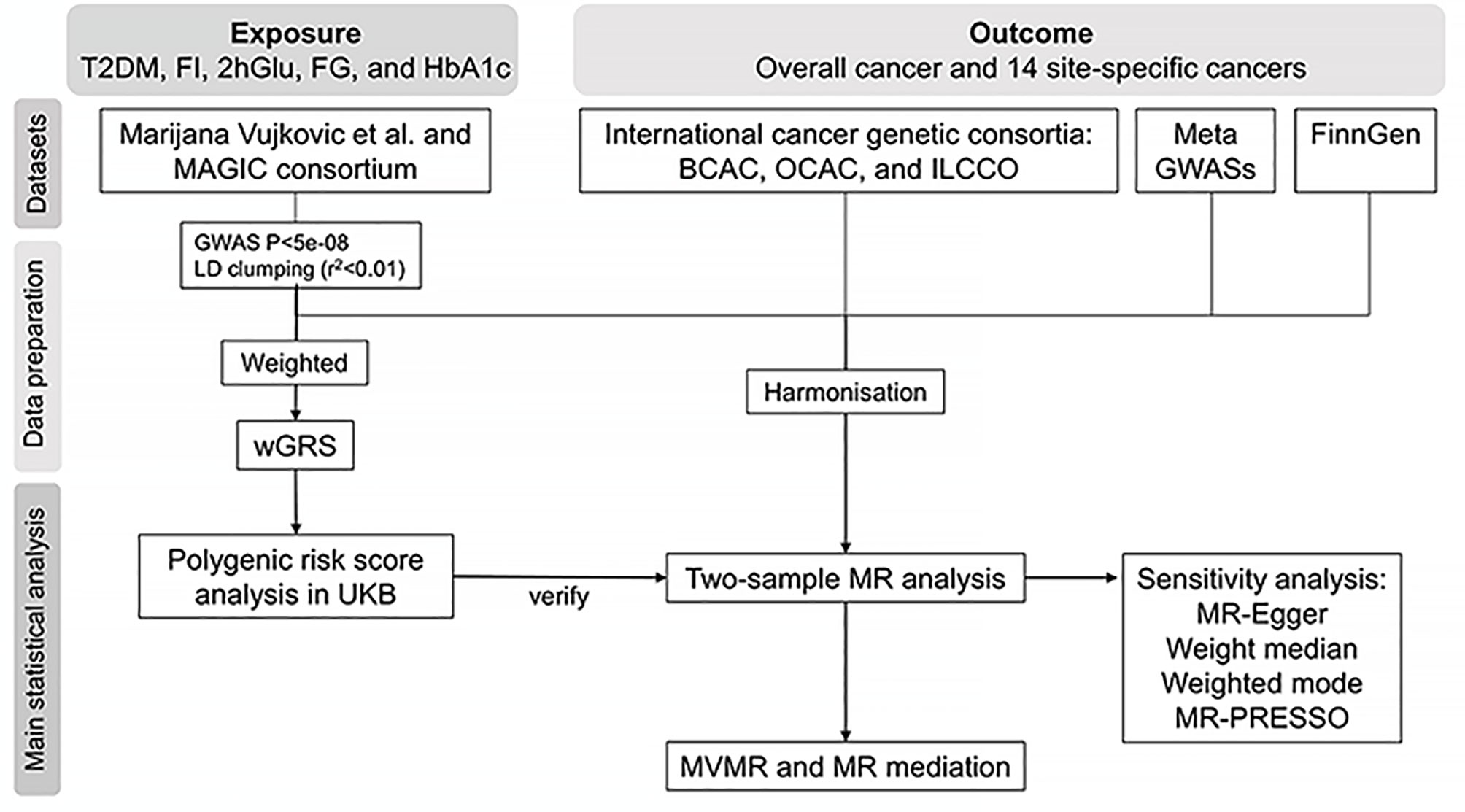
Figure 1 Flowchart of the study. T2DM, type 2 diabetes; FI, fasting insulin; 2hGlu, 2-h glucose after an oral glucose challenge; FG, fasting glucose; HbA1c, glycated hemoglobin; LD, linkage disequilibrium; wGRS, weighted genetic risk score; UKB, UK Biobank; BCAC, the Breast Cancer Association Consortium; OCAC, the Ovarian Cancer Association Consortium; ILCCO, the International Lung Cancer Consortium; MR, Mendelian randomization; MVMR, multivariable MR.
Genetic Instruments of Glycemic Traits and Type 2 Diabetes Mellitus
Single-nucleotide polymorphisms (SNPs) for glycemic traits [including FI, fasting glucose (FG), 2-h glucose after an oral glucose challenge (2hGlu), and glycated hemoglobin (HbA1c)] were derived from the Meta-Analyses of Glucose and Insulin-related traits Consortium (MAGIC) including 281,146 participants (25). For T2DM, the genetic instruments were extracted from a multi-ethnic meta-analysis among 1.4 million participants (26). For the SNPs with a genome-wide significance among those of European descent (p < 5×10−8), linkage disequilibrium (LD) was evaluated among these SNPs, and the F-statistic for each of them was calculated. Genetic instruments with a low LD level (r2 < 0.01) and high F-statistics (F > 10) were finally included in the MR analysis. Briefly, a total of 380, 36, 69, 14, and 50 SNPs were selected as IVs for T2DM, FI, FG, 2hGlu, and HbA1c, respectively. Trait variance explained by genetic IVs for T2DM, FI, FG, 2hGlu, and HbA1c was 35.5%, 0.8%, 3.9%, 0.9%, and 5.2% (25, 26), respectively. The details of the used SNPs are displayed in Supplementary Table 1.
Data Sources for Overall Cancer and Site-Specific Cancers
Summary-level genetic data of overall cancer and 14 site-specific cancers were taken from the FinnGen Biobank, the Breast Cancer Association Consortium (BCAC), the UKB and the Kaiser Permanente Genetic Epidemiology Research on Adult Health and Aging (GERA) cohorts, the Ovarian Cancer Association Consortium (OCAC), the International Lung Cancer Consortium (ILCCO), a meta-analysis of 13 studies of endometrial cancer and the Epidemiology of Endometrial Cancer Consortium (E2C2), and a meta-analysis of 11 previous colorectal cancer (CRC) GWASs (Supplementary Table 2). To enhance the statistical power, only cancer types with more than 400 cases were included in the TSMR analysis. The details of the outcome data source are described in the Supplementary Material.
Two-Sample Mendelian Randomization Analysis and Sensitivity Analysis
The inverse-variance weighted (IVW) approach was adopted as the primary analysis to determine the associations between FI levels and different types of cancer. Model-based estimates (27), weighted median (WM) method (28), and MR pleiotropy residual sum and outlier (MR-PRESSO) test (29) were also performed to further assess the robustness of the associations. Cochrane’s Q statistic was calculated to measure the heterogeneity, and the MR-Egger test (intercept p ≤ 0.05) was conducted to detect possible pleiotropy. If more than 50% of the weight comes from valid instruments, the weighted-median method provides consistent estimates of associations even when horizontal pleiotropy exists (30). Since the MR-PRESSO test can detect possible outliers and provide estimates after the removal of outliers, it was implemented to correct for possible pleiotropic effects (29). In addition, scatter plots were employed to compare MR models, and “leave-one-out” analyses were also used to detect outliers for SNPs (31). The “TwoSampleMR” R package and “MR-PRESSO” package were applied for these analyses in R4.1.2 software. All statistical analyses were two-tailed.
Polygenic Risk Score Analysis in UK Biobank
PRS analysis was conducted by using individual-level data from the UK Biobank (32). The cancer cases were defined by corresponding codes in International Classification of Diseases (ICD) versions 9 and 10 with information from national medical records, which included inpatient hospital episode records, cancer registry, and death registry. The PRSs were constructed by summing up the number of risk-increasing alleles for each SNP weighted by their effect size (beta) on FI levels and then adding this weighted score for all used SNPs. The details of the used SNPs are displayed in Supplementary Table 3. Multivariate logistic regression was performed subsequently to explore the association between the PRS of FI and cancer risk with adjustment for age, sex, BMI, drinking, smoking, and the first 10 genetic principal components. Bonferroni correction was applied to correct the threshold of statistical significance for multiple comparisons, and the p-value below 0.0036 (where p = 0.05/14) in PRS analysis was considered to be strong evidence of significant association, while associations with a p-value below 0.05 were considered as suggestive significance. The PRS analysis was conducted by using R4.1.2 software.
Multivariable Mendelian Randomization and Mediation Analysis
Given the overlap in the genetic variables of T2DM with FI, whether FI may play an intermediary role in the association between T2DM and a certain type of cancer was assessed by using the MVMR and MR mediation analyses. In this stage, MVMR analysis was applied in a two-sample setting to adjust for the genetic association of the instruments with FI. Subsequently, network MR analysis was carried out to estimate the proportion of the effect of T2DM on certain cancer mediated by FI (33). Analyses at this stage were also implemented through the “TwoSampleMR” package in R4.1.2 software.
Results
Two-Sample Mendelian Randomization Analysis
MR IVW approach suggested that genetically determined high FI levels were associated with increased risk of colorectal (odds ratio (OR) = 1.87, 95% CI: 1.23–2.84, p = 0.003) (Figure 2A) and endometrial cancer (OR = 1.89, 95% CI: 1.08–3.01, p = 0.008) (Figure 3A). Sensitivity analysis comprising MR-Egger, WM, and MR-PRESSO showed a similar association pattern (all OR > 1). The p-values of MR-Egger interception for colorectal and endometrial cancers were 0.616 and 0.203, respectively, indicating there was no horizontal pleiotropy. Although outlier (n = 1) was detected in the MR-PRESSO test for endometrial cancer, the effect estimate (β = 0.71, p = 0.002) with the removal of outliers was similar to the effect estimate (β = 0.62, p = 0.012) without removal (p-value of distortion test > 0.05, as shown in Supplementary Table 4). Scatter plots illustrated consistent estimate trends among three MR methods (Figures 2B and 3B), indicating the robustness of these results. The “leave-one-out” MR analysis also reported consistent significant associations between FI level and the risk of colorectal and endometrial cancers (Supplementary Figure 1).
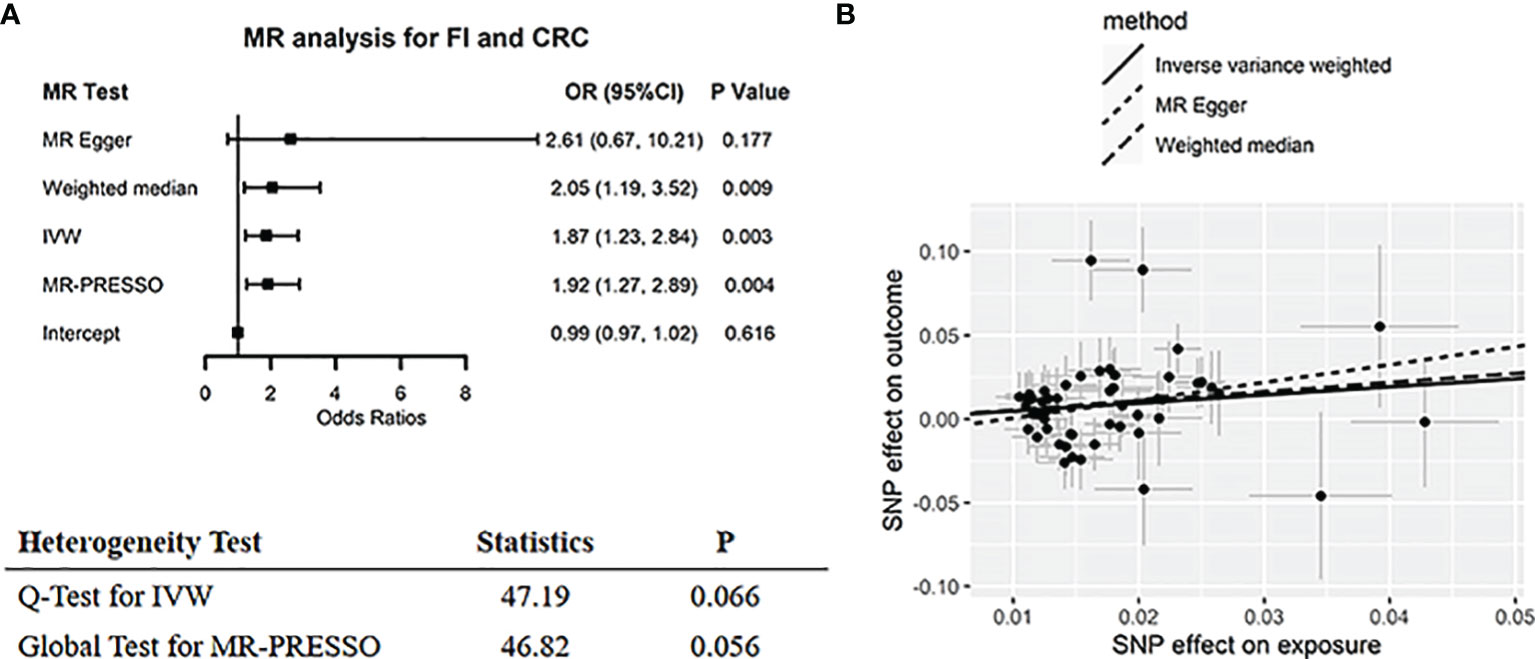
Figure 2 Two-sample MR analysis results of FI on colorectal cancer. (A) Forest plot for summarizing the results of all MR methods. The black box represents the effect estimates, and the error line represents the 95% CI. (B) Scatter plot for comparison of MR methods. The black circle represents the point effect estimate, and the slope represents the correlation trend. FI, fasting insulin; CRC, colorectal cancer; MR, Mendelian randomization; IVW, inverse-variance weighted; MR-PRESSO, MR pleiotropy residual sum and outlier test; OR, odds ratio.
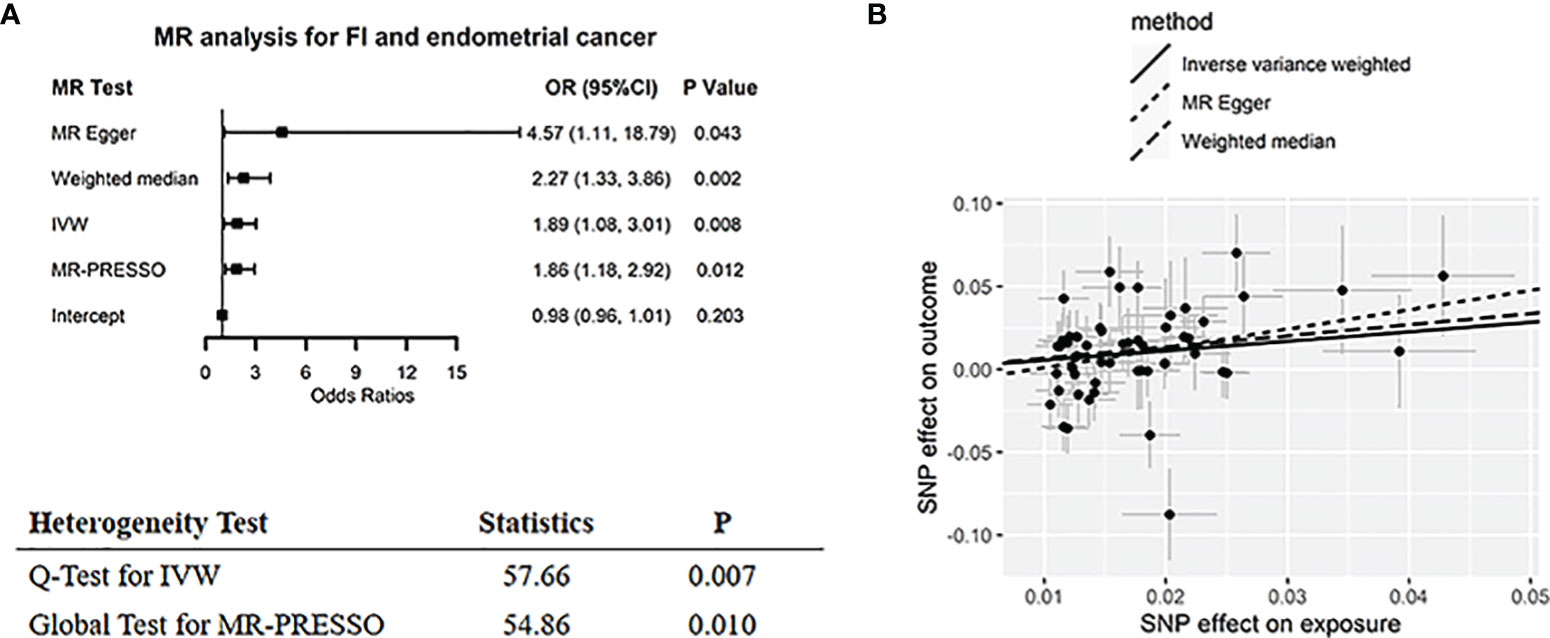
Figure 3 Two-sample MR analysis results of FI on endometrial cancer. (A) Forest plot for summarizing the results of all MR methods. The black box represents the effect estimates, and the error line represents the 95% CI. (B) Scatter plot for comparison of MR methods. The black circle represents the point effect estimate, and the slope represents the correlation trend. FI, fasting insulin; CRC, colorectal cancer; MR, Mendelian randomization; IVW, inverse-variance weighted; MR-PRESSO, MR pleiotropy residual sum and outlier test; OR, odds ratio.
However, there was no evidence supporting an association of FI with other cancers and overall cancer (all peffect > 0.05) (Supplementary Table 5). The results of sensitivity analyses for FI and other cancers showed consistent estimates (Supplementary Table 3 and Supplementary Figure 1).
Table 1 depicts the TSMR results of other glycemic traits and T2DM on colorectal and endometrial cancers. There was no association of other glycemic traits with colorectal cancer (all peffect > 0.05), and the sensitivity analyses presented consistently null estimates (all peffect > 0.05) (Supplementary Figure 2). However, we found a significant association between genetic liability to T2DM and increased endometrial cancer risk (OR = 1.10, 95% CI: 1.04–1.16, p = 0.001). Although the Q-test of the IVW approach (pheterogeneity < 0.001) showed obvious heterogeneity, the sensitivity analyses illustrated consistent results with the primary analysis (Supplementary Figure 3), and there was no horizontal pleiotropy (pintercept = 0.097).
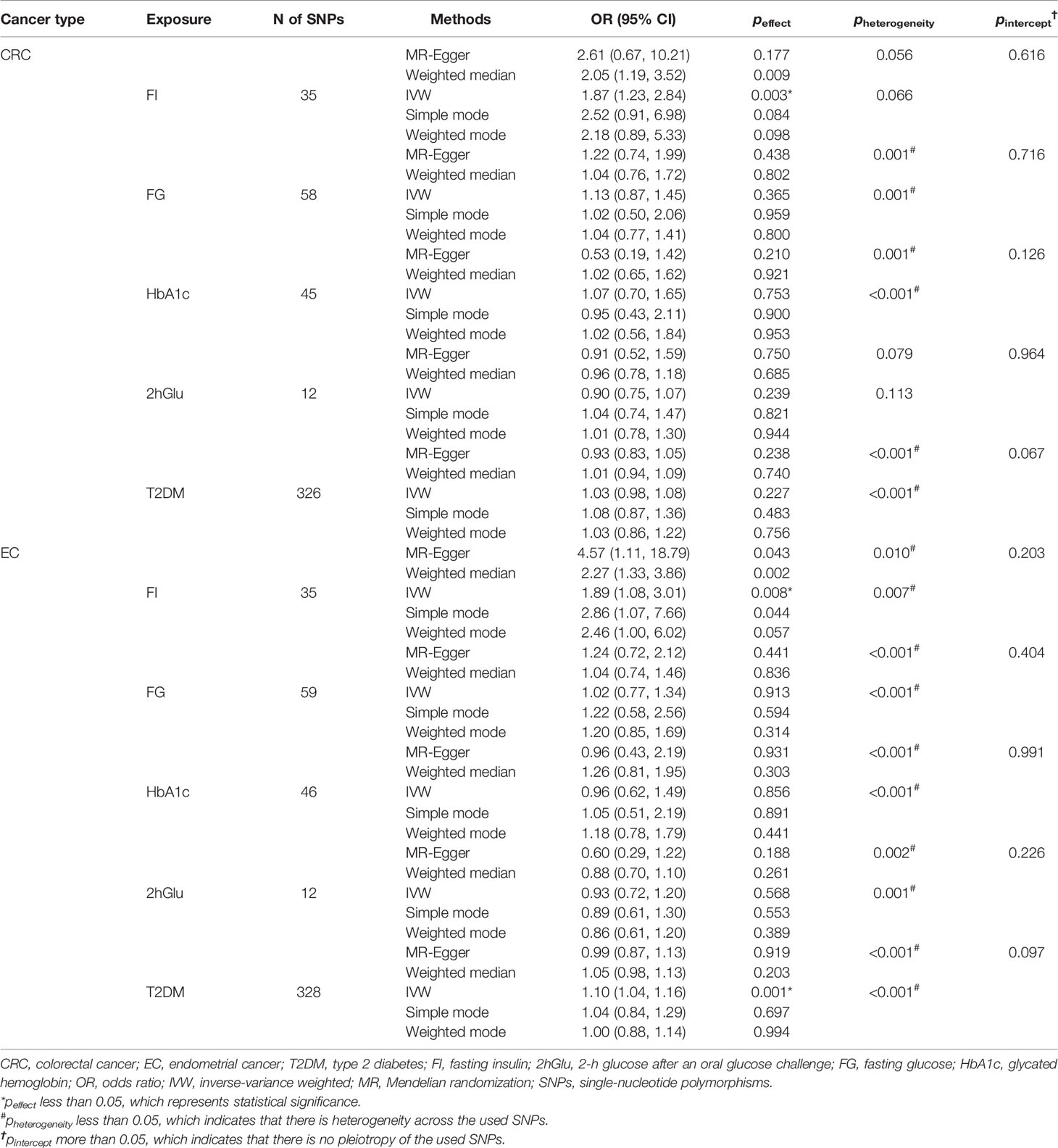
Table 1 Two-sample MR analysis results of glycemic traits and T2DM on colorectal and endometrial cancers.
Polygenic Risk Score Analysis in UK Biobank
With adjustment for age, sex, BMI, drinking, smoking, and the first 10 genetic principal components in a multivariable logistic regression model, a significant association was observed between genetically determined high levels of FI and the increased risk of endometrial cancer (OR = 2.83, 95% CI: 1.51–5.33, p = 0.001) (Figure 4). There was suggestive evidence supporting the association between genetically determined high FI levels and increased risk of colorectal cancer (OR = 1.48, 95% CI: 1.05–2.09, p = 0.026). As displayed in Figure 4, we did not find any significant association between genetically related FI levels with other site-specific cancers (all p > 0.05).
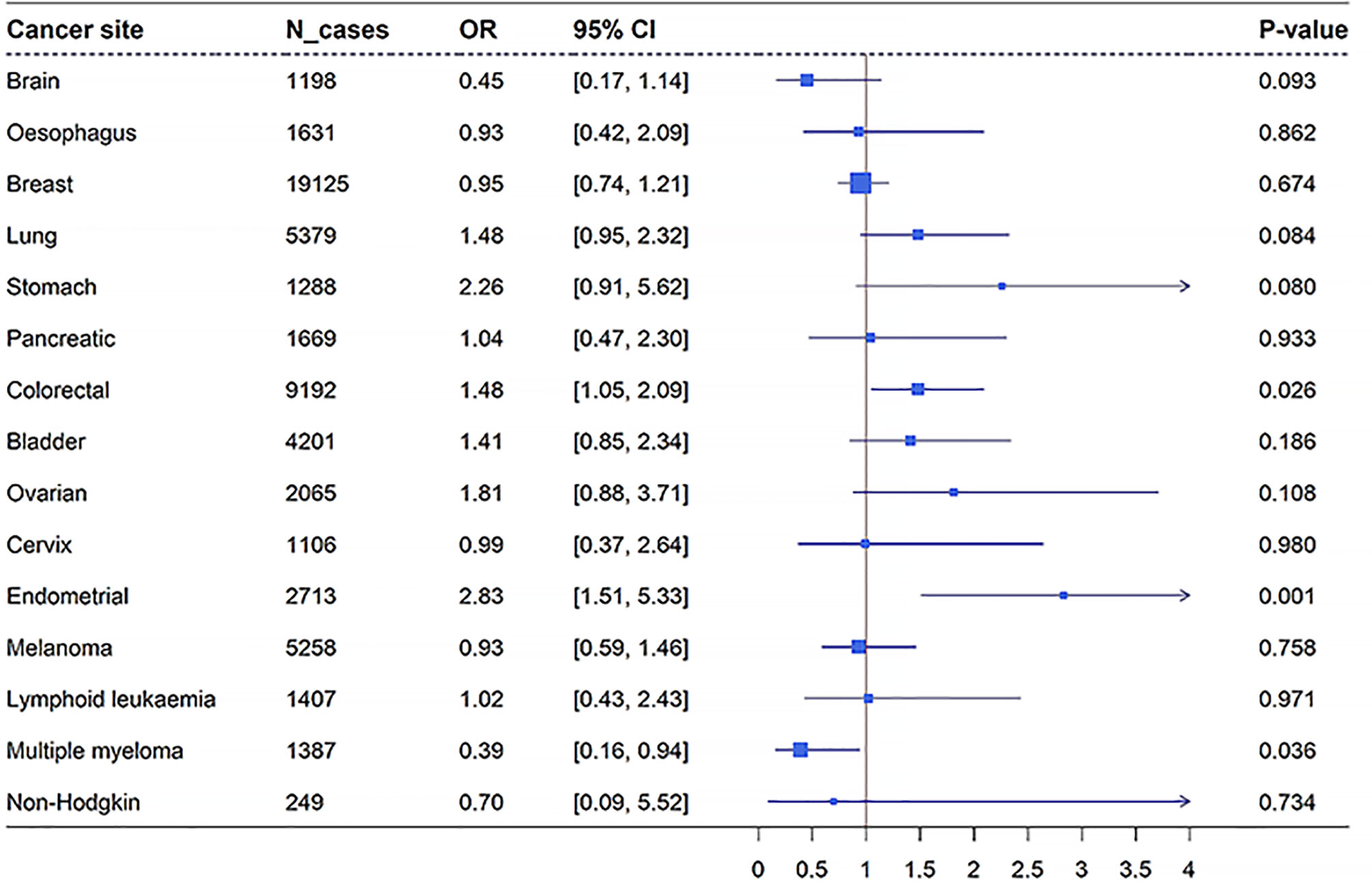
Figure 4 Forest plot for the results of PRS analysis of FI on overall cancer and site-specific cancers in UK Biobank. The blue box represents the effect estimates, and the blue error line represents the 95% CI. OR, odds ratio; PRS, polygenic risk score; FI, fasting insulin.
Multivariable Mendelian Randomization and Mediation Analysis
Based on the results from TSMR, we performed MVMR analysis to adjust for genetically determined FI and T2DM in the same model. We found that the effect estimates of T2DM on endometrial cancer (OR = 1.04, 95% CI: 0.98–1.10, p = 0.224) were obviously attenuated when compared with the primary univariable MR analysis (OR = 1.10, 95% CI: 1.04–1.16, p < 0.001) (Figure 5). The residual MVMR method illustrated similar results (Supplementary Table 6). The results of network MR analysis are presented in Supplementary Table 7; the ORs of T2DM and FI on endometrial cancer were 1.04 (95% CI: 0.98–1.10, p = 0.224) and 2.75 (95% CI: 1.60–4.73, p < 0.001), respectively. Moreover, increased risk of T2DM was also associated with increased FI levels (OR = 1.02, 95% CI: 1.01–1.03, p = 0.001). These results indicated that the effect of T2DM on endometrial cancer may be mediated through FI. Subsequently, we performed MR mediation analysis and quantified the intermediary effect of FI on the association between T2DM and endometrial cancer as 21.3%.
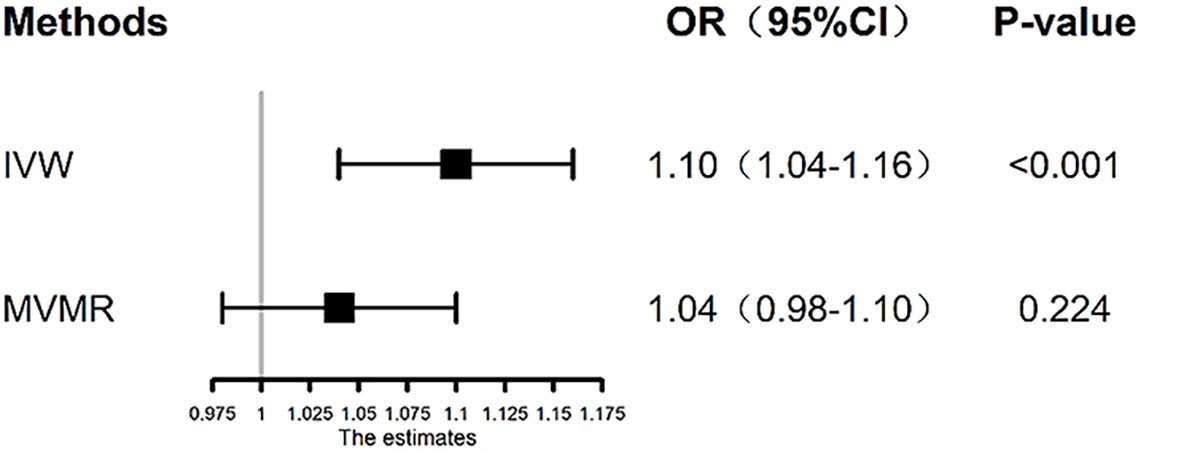
Figure 5 Inverse-variance weighted (IVW) and multivariable Mendelian randomization (MVMR) estimates for genetic liability to T2DM on risk of endometrial cancer. The MVMR analysis adjusts for the associations of the genetic instruments with fasting insulin. The black box represents the effect estimates, and the black error line represents the 95% CI. IVW, inverse-variance weighted; OR, odds ratio; T2DM, type 2 diabetes mellitus.
Discussion
In this study, we found strong evidence of positive associations between FI levels and the risk of colorectal and endometrial cancers. Furthermore, MVMR and MR mediation analyses demonstrated that FI plays an intermediary role in the association between T2DM and endometrial cancer.
Several observational studies have found that higher FI levels were related to increased colorectal cancer risk (34–36). Consistent with our finding, a case–control study reported that fasting blood insulin levels were higher in non-diabetic colorectal cancer patients compared with obesity-matched controls and that FI levels were associated with increased colorectal cancer risk (34). Similarly, a case–control study from Korea with 3,606 cases and 6,019 controls indicated that increased serum insulin levels and insulin insistence were significantly associated with the presence of colorectal cancer (36). A meta-analysis of 35 studies involving 25,566 cases showed that higher FI levels were significantly associated with an increased risk of colorectal cancer (37). A recent MR study also validated the association between FI and colorectal cancer risk (38). In contrast, a longitudinal study with a median follow-up period of 11.9 years demonstrated that serum insulin was not related to the risk of colorectal cancer in postmenopausal women (39). Furthermore, a previous MR study found no evidence supporting the association between FI levels and colorectal cancer risk (8). The discrepancy may be due to the small number of genetic instruments used and the small proportion of variance explained by IVs in the previous MR study.
The results of the current study are consistent with previous findings supporting a harmful role of high FI levels in endometrial cancer. Increased serum insulin has been reported to be associated with roughly a doubling of endometrial cancer risk in postmenopausal women (40). A systematic review and meta-analysis (13 studies, n = 4,088) provided suggestive evidence that high FI was related to an increased risk of endometrial cancer (41). An MR study also reported a positive association between genetically determined higher FI levels and greater risk of endometrial cancer (42). In addition, observational and meta-analysis studies have illustrated a positive association between T2DM and endometrial cancer (43–45). An umbrella review of observational and MR studies also identified potential associations for genetic predisposition to T2DM and FI concentrations and risk of endometrial cancer (46). However, the intermediary role of FI in the association between T2DM and endometrial cancer was not reported. Our study observed that FI mediated around 23.1% of the effect of T2DM on endometrial cancer.
In line with our findings, a TSMR study found limited evidence of the association of genetically determined FI levels with overall cancer (8). However, a prospective cohort study showed higher cancer mortality in patients with hyperinsulinemia regardless of obesity (47). Another observational study also has proposed that extremely high FI levels were probably an independent risk of cancer mortality among men rather than women (48). Since the present study mainly focused on the overall cancer risk, we could not conclude that the results of this work were inconsistent with those observational studies.
The advantage of our study is the MR study design, which can diminish confounding and reverse correlation, potentially biasing the findings of observational studies. Secondly, considering that many metabolism-related traits may affect each other, we also performed MVMR and MR mediation analyses to rule out the possible influence and to explore the potential effect of FI on the association between T2DM and endometrial cancer. To our knowledge, the IVs for glycemic traits used in this study are the most up to date and comprehensive, and it can enhance the statistical power of this work. We performed the analysis only among European populations, which largely avoided bias caused by population stratification. However, it also confined the transferability of our results to other populations at the same time. Another major limitation of the study is that the number of cases was few for certain site-specific cancers, which would reduce precisely the estimates. Additionally, given the relatively small proportion of variance explained by FI-related genetic variants, our study might lack the power to identify weak or moderate associations. It should be acknowledged that although MR design is advantageous in the association inference, the claim of causality should be taken with caution given the complexity of association and the limitations of the MR study. In order to increase the reliability of the results, we conducted PRS analysis in UK Biobank to validate the findings of the TSMR study. However, given that UK Biobank did not have data on circulating FI levels, we were therefore not able to estimate the variance of FI levels explained by the PRS. In the future, larger observational and well-designed experimental studies are warranted to explore the complex role and mechanism of FI in cancer progression.
In summary, this MR study provided strong evidence of positive associations of FI with colorectal and endometrial cancer risk. Our study also demonstrated an intermediary effect of FI on the association between T2DM and endometrial cancer. However, there was limited evidence in support of associations between FI and overall cancer and other site-specific cancers. In addition to the benefits of insulin for diabetes treatment, it is suggested that regular monitoring of insulin levels and screening and treatment for subclinical insulinemia may be an efficient colorectal and endometrial cancer prevention strategy.
Data Availability Statement
The original contributions presented in the study are included in the article/Supplementary Material. Further inquiries can be directed to the corresponding authors.
Author Contributions
Conceptualization: XL and YW. Methodology: XL. Software: HZ. Validation: HZ, DL, and XZL. Formal analysis: HZ. Investigation: DL and XZL. Resources: XZL. Data curation: ZY and YW. Writing—original draft preparation: HZ. Writing—review and editing: ZW, XL, YW, and ZY. Visualization: HZ. Supervision: XL and YW. Project administration: XL. All authors listed have made a substantial, direct, and intellectual contribution to the work and approved it for publication.
Conflict of Interest
The authors declare that the research was conducted in the absence of any commercial or financial relationships that could be construed as a potential conflict of interest.
Publisher’s Note
All claims expressed in this article are solely those of the authors and do not necessarily represent those of their affiliated organizations, or those of the publisher, the editors and the reviewers. Any product that may be evaluated in this article, or claim that may be made by its manufacturer, is not guaranteed or endorsed by the publisher.
Supplementary Material
The Supplementary Material for this article can be found online at: https://www.frontiersin.org/articles/10.3389/fonc.2022.863340/full#supplementary-material
References
1. Ahn KY, Lee MK, Kim DI, Park J, Min J, Yang H, et al. Cardiopulmonary Fitness, Adiponectin, Chemerin Associated Fasting Insulin Level in Colorectal Cancer Patients. Supportive Care Cancer Off J Multinational Assoc Supportive Care Cancer (2016) 24(7):2927–35. doi: 10.1007/s00520-016-3095-4
2. Goodwin PJ, Ennis M, Pritchard KI, Trudeau ME, Koo J, Madarnas Y, et al. Fasting Insulin and Outcome in Early-Stage Breast Cancer: Results of a Prospective Cohort Study. J Clin Oncol Off J Am Soc Clin Oncol (2002) 20(1):42–51. doi: 10.1200/jco.2002.20.1.42
3. Wolpin BM, Meyerhardt JA, Chan AT, Ng K, Chan JA, Wu K, et al. Insulin, the Insulin-Like Growth Factor Axis, and Mortality in Patients With Nonmetastatic Colorectal Cancer. J Clin Oncol Off J Am Soc Clin Oncol (2009) 27(2):176–85. doi: 10.1200/jco.2008.17.9945
4. Albanes D, Weinstein SJ, Wright ME, Männistö S, Limburg PJ, Snyder K, et al. Serum Insulin, Glucose, Indices of Insulin Resistance, and Risk of Prostate Cancer. J Natl Cancer Institute (2009) 101(18):1272–9. doi: 10.1093/jnci/djp260
5. Hsing AW, Chua S Jr., Gao YT, Gentzschein E, Chang L, Deng J, et al. Prostate Cancer Risk and Serum Levels of Insulin and Leptin: A Population-Based Study. J Natl Cancer Institute (2001) 93(10):783–9. doi: 10.1093/jnci/93.10.783
6. Stolzenberg-Solomon RZ, Graubard BI, Chari S, Limburg P, Taylor PR, Virtamo J, et al. Insulin, Glucose, Insulin Resistance, and Pancreatic Cancer in Male Smokers. Jama (2005) 294(22):2872–8. doi: 10.1001/jama.294.22.2872
7. Tsilidis KK, Dai JY, Peters U. Editorial: Mendelian Randomization Analysis Identifies Body Mass Index and Fasting Insulin as Potential Causal Risk Factors for Pancreatic Cancer Risk. J Natl Cancer Institute (2017) 109(9):djx043. doi: 10.1093/jnci/djx043
8. Yuan S, Kar S, Carter P, Vithayathil M, Mason AM, Burgess S, et al. Is Type 2 Diabetes Causally Associated With Cancer Risk? Evidence From a Two-Sample Mendelian Randomization Study. Diabetes (2020) 69(7):1588–96. doi: 10.2337/db20-0084
9. Gunter MJ, Hoover DR, Yu H, Wassertheil-Smoller S, Manson JE, Li J, et al. A Prospective Evaluation of Insulin and Insulin-Like Growth Factor-I as Risk Factors for Endometrial Cancer. Cancer epidemiology Biomarkers Prev Publ Am Assoc Cancer Research cosponsored by Am Soc Prev Oncol (2008) 17(4):921–9. doi: 10.1158/1055-9965.epi-07-2686
10. Hidaka A, Sasazuki S, Goto A, Sawada N, Shimazu T, Yamaji T, et al. Plasma Insulin, C-Peptide and Blood Glucose and the Risk of Gastric Cancer: The Japan Public Health Center-Based Prospective Study. Int J Cancer (2015) 136(6):1402–10. doi: 10.1002/ijc.29098
11. Argirion I, Weinstein SJ, Männistö S, Albanes D, Mondul AM. Serum Insulin, Glucose, Indices of Insulin Resistance, and Risk of Lung Cancer. Cancer epidemiology Biomarkers Prev Publ Am Assoc Cancer Research cosponsored by Am Soc Prev Oncol (2017) 26(10):1519–24. doi: 10.1158/1055-9965.epi-17-0293
12. Loftfield E, Freedman ND, Lai GY, Weinstein SJ, McGlynn KA, Taylor PR, et al. Higher Glucose and Insulin Levels Are Associated With Risk of Liver Cancer and Chronic Liver Disease Mortality Among Men Without a History of Diabetes. Cancer Prev Res (Philadelphia Pa) (2016) 9(11):866–74. doi: 10.1158/1940-6207.capr-16-0141
13. Tsilidis KK, Kasimis JC, Lopez DS, Ntzani EE, Ioannidis JP. Type 2 Diabetes and Cancer: Umbrella Review of Meta-Analyses of Observational Studies. BMJ (Clinical Res ed) (2015) 350:g7607. doi: 10.1136/bmj.g7607
14. Ryu TY, Park J, Scherer PE. Hyperglycemia as a Risk Factor for Cancer Progression. Diabetes Metab J (2014) 38(5):330–6. doi: 10.4093/dmj.2014.38.5.330
15. Noto H. Unfolding Link Between Diabetes and Cancer. J Diabetes Invest (2017) 9(3):473–4. doi: 10.1111/jdi.12725
16. Hua F, Yu JJ, Hu ZW. Diabetes and Cancer, Common Threads and Missing Links. Cancer Lett (2016) 374(1):54–61. doi: 10.1016/j.canlet.2016.02.006
17. Fatahi S, Nazary-Vannani A, Sohouli MH, Mokhtari Z, Kord-Varkaneh H, Moodi V, et al. The Effect of Fasting and Energy Restricting Diets on Markers of Glucose and Insulin Controls: A Systematic Review and Meta-Analysis of Randomized Controlled Trials. Crit Rev Food Sci Nutr (2021) 61(20):3383–94. doi: 10.1080/10408398.2020.1798350
18. Gower BA, Pearson K, Bush N, Shikany JM, Howard VJ, Cohen CW, et al. Diet Pattern May Affect Fasting Insulin in a Large Sample of Black and White Adults. Eur J Clin Nutr (2021) 75(4):628–35. doi: 10.1038/s41430-020-00762-9
19. Thomas MK, Nikooienejad A, Bray R, Cui X, Wilson J, Duffin K, et al. Dual Gip and Glp-1 Receptor Agonist Tirzepatide Improves Beta-Cell Function and Insulin Sensitivity in Type 2 Diabetes. J Clin Endocrinol Metab (2021) 106(2):388–96. doi: 10.1210/clinem/dgaa863
20. Davey Smith G, Hemani G. Mendelian Randomization: Genetic Anchors for Causal Inference in Epidemiological Studies. Hum Mol Genet (2014) 23(R1):R89–98. doi: 10.1093/hmg/ddu328
21. Smith GD, Ebrahim S. 'Mendelian Randomization': Can Genetic Epidemiology Contribute to Understanding Environmental Determinants of Disease? Int J Epidemiol (2003) 32(1):1–22. doi: 10.1093/ije/dyg070
22. Burgess S, Thompson SG. Multivariable Mendelian Randomization: The Use of Pleiotropic Genetic Variants to Estimate Causal Effects. Am J Epidemiol (2015) 181(4):251–60. doi: 10.1093/aje/kwu283
23. Sanderson E, Davey Smith G, Windmeijer F, Bowden J. An Examination of Multivariable Mendelian Randomization in the Single-Sample and Two-Sample Summary Data Settings. Int J Epidemiol (2019) 48(3):713–27. doi: 10.1093/ije/dyy262
24. Sanderson E. Multivariable Mendelian Randomization and Mediation. Cold Spring Harbor Perspect Med (2021) 11(2):a038984. doi: 10.1101/cshperspect.a038984
25. Chen J, Spracklen CN, Marenne G, Varshney A, Corbin LJ, Luan J, et al. The Trans-Ancestral Genomic Architecture of Glycemic Traits. Nat Genet (2021) 53(6):840–60. doi: 10.1038/s41588-021-00852-9
26. Vujkovic M, Keaton JM, Lynch JA, Miller DR, Zhou J, Tcheandjieu C, et al. Discovery of 318 New Risk Loci for Type 2 Diabetes and Related Vascular Outcomes Among 1.4 Million Participants in a Multi-Ancestry Meta-Analysis. Nat Genet (2020) 52(7):680–91. doi: 10.1038/s41588-020-0637-y
27. Hartwig FP, Davey Smith G, Bowden J. Robust Inference in Summary Data Mendelian Randomization Via the Zero Modal Pleiotropy Assumption. Int J Epidemiol (2017) 46(6):1985–98. doi: 10.1093/ije/dyx102
28. Bowden J, Davey Smith G, Haycock PC, Burgess S. Consistent Estimation in Mendelian Randomization With Some Invalid Instruments Using a Weighted Median Estimator. Genet Epidemiol (2016) 40(4):304–14. doi: 10.1002/gepi.21965
29. Verbanck M, Chen CY, Neale B, Do R. Detection of Widespread Horizontal Pleiotropy in Causal Relationships Inferred From Mendelian Randomization Between Complex Traits and Diseases. Nat Genet (2018) 50(5):693–8. doi: 10.1038/s41588-018-0099-7
30. Burgess S, Bowden J, Fall T, Ingelsson E, Thompson SG. Sensitivity Analyses for Robust Causal Inference From Mendelian Randomization Analyses With Multiple Genetic Variants. Epidemiol (Cambridge Mass) (2017) 28(1):30–42. doi: 10.1097/ede.0000000000000559
31. Zheng J, Baird D, Borges MC, Bowden J, Hemani G, Haycock P, et al. Recent Developments in Mendelian Randomization Studies. Curr Epidemiol Rep (2017) 4(4):330–45. doi: 10.1007/s40471-017-0128-6
32. Sudlow C, Gallacher J, Allen N, Beral V, Burton P, Danesh J, et al. UK Biobank: An Open Access Resource for Identifying the Causes of a Wide Range of Complex Diseases of Middle and Old Age. PloS Med (2015) 12(3):e1001779. doi: 10.1371/journal.pmed.1001779
33. Carter AR, Sanderson E, Hammerton G, Richmond RC, Davey Smith G, Heron J, et al. Mendelian Randomisation for Mediation Analysis: Current Methods and Challenges for Implementation. Eur J Epidemiol (2021) 36(5):465–78. doi: 10.1007/s10654-021-00757-1
34. Ferroni P, Formica V, Della-Morte D, Lucchetti J, Spila A, D'Alessandro R, et al. Prognostic Value of Glycated Hemoglobin in Colorectal Cancer. World J Gastroenterol (2016) 22(45):9984–93. doi: 10.3748/wjg.v22.i45.9984
35. Flood A, Mai V, Pfeiffer R, Kahle L, Remaley AT, Lanza E, et al. Elevated Serum Concentrations of Insulin and Glucose Increase Risk of Recurrent Colorectal Adenomas. Gastroenterology (2007) 133(5):1423–9. doi: 10.1053/j.gastro.2007.08.040
36. Kim EH, Kim HK, Bae SJ, Chang HS, Park HW, Do MY, et al. Fasting Serum Insulin Levels and Insulin Resistance Are Associated With Colorectal Adenoma in Koreans. J Diabetes Invest (2014) 5(3):297–304. doi: 10.1111/jdi.12178
37. Xu J, Ye Y, Wu H, Duerksen-Hughes P, Zhang H, Li P, et al. Association Between Markers of Glucose Metabolism and Risk of Colorectal Cancer. BMJ Open (2016) 6(6):e011430. doi: 10.1136/bmjopen-2016-011430
38. Murphy N, Song M, Papadimitriou N, Carreras-Torres R, Langenberg C, Martin RM, et al. Associations Between Glycemic Traits and Colorectal Cancer: A Mendelian Randomization Analysis. J Natl Cancer Institute (2022) djac011. doi: 10.1093/jnci/djac011
39. Kabat GC, Kim MY, Strickler HD, Shikany JM, Lane D, Luo J, et al. A Longitudinal Study of Serum Insulin and Glucose Levels in Relation to Colorectal Cancer Risk Among Postmenopausal Women. Br J Cancer (2012) 106(1):227–32. doi: 10.1038/bjc.2011.512
40. Kabat GC, Kim MY, Lane DS, Zaslavsky O, Ho GYF, Luo J, et al. Serum Glucose and Insulin and Risk of Cancers of the Breast, Endometrium, and Ovary in Postmenopausal Women. Eur J Cancer Prev Off J Eur Cancer Prev Organisation (ECP) (2018) 27(3):261–8. doi: 10.1097/cej.0000000000000435
41. Hernandez AV, Pasupuleti V, Benites-Zapata VA, Thota P, Deshpande A, Perez-Lopez FR. Insulin Resistance and Endometrial Cancer Risk: A Systematic Review and Meta-Analysis. Eur J Cancer (Oxford Engl 1990) (2015) 51(18):2747–58. doi: 10.1016/j.ejca.2015.08.031
42. Nead KT, Sharp SJ, Thompson DJ, Painter JN, Savage DB, Semple RK, et al. Evidence of a Causal Association Between Insulinemia and Endometrial Cancer: A Mendelian Randomization Analysis. J Natl Cancer Institute (2015) 107(9):djv178. doi: 10.1093/jnci/djv178
43. Ling S, Brown K, Miksza JK, Howells L, Morrison A, Issa E, et al. Association of Type 2 Diabetes With Cancer: A Meta-Analysis With Bias Analysis for Unmeasured Confounding in 151 Cohorts Comprising 32 Million People. Diabetes Care (2020) 43(9):2313–22. doi: 10.2337/dc20-0204
44. Liu X, Hemminki K, Försti A, Sundquist K, Sundquist J, Ji J. Cancer Risk in Patients With Type 2 Diabetes Mellitus and Their Relatives. Int J Cancer (2015) 137(4):903–10. doi: 10.1002/ijc.29440
45. Luo J, Beresford S, Chen C, Chlebowski R, Garcia L, Kuller L, et al. Association Between Diabetes, Diabetes Treatment and Risk of Developing Endometrial Cancer. Br J Cancer (2014) 111(7):1432–9. doi: 10.1038/bjc.2014.407
46. Pearson-Stuttard J, Papadimitriou N, Markozannes G, Cividini S, Kakourou A, Gill D, et al. Type 2 Diabetes and Cancer: An Umbrella Review of Observational and Mendelian Randomization Studies. Cancer Epidemiology Biomarkers Prev Publ Am Assoc Cancer Research cosponsored by Am Soc Prev Oncol (2021) 30(6):1218–28. doi: 10.1158/1055-9965.epi-20-1245
47. Tsujimoto T, Kajio H, Sugiyama T. Association Between Hyperinsulinemia and Increased Risk of Cancer Death in Nonobese and Obese People: A Population-Based Observational Study. Int J Cancer (2017) 141(1):102–11. doi: 10.1002/ijc.30729
Keywords: fasting insulin, type 2 diabetes, cancer, Mendelian randomization study, polygenic risk score analysis
Citation: Zhang H, Li D, Liu X, Wan Z, Yu Z, Wang Y and Li X (2022) Fasting Insulin and Risk of Overall and 14 Site-Specific Cancers: Evidence From Genetic Data. Front. Oncol. 12:863340. doi: 10.3389/fonc.2022.863340
Received: 27 January 2022; Accepted: 22 March 2022;
Published: 21 April 2022.
Edited by:
Jun Zhong, National Cancer Institute (NIH), United StatesReviewed by:
Shu-Chun Chuang, National Health Research Institutes, TaiwanJerry Polesel, Aviano Oncology Reference Center (IRCCS), Italy
Copyright © 2022 Zhang, Li, Liu, Wan, Yu, Wang and Li. This is an open-access article distributed under the terms of the Creative Commons Attribution License (CC BY). The use, distribution or reproduction in other forums is permitted, provided the original author(s) and the copyright owner(s) are credited and that the original publication in this journal is cited, in accordance with accepted academic practice. No use, distribution or reproduction is permitted which does not comply with these terms.
*Correspondence: Xue Li, 279433473@qq.com; Yuming Wang, henanyuming@sina.com