- 1The Fourth School of Clinical Medicine, Zhejiang Chinese Medical University, Hangzhou, China
- 2School of Public Health, Zhejiang Chinese Medical University, Hangzhou, China
Introduction: Observational studies have reported a relationship between iron status and the risk of prostate cancer. However, it remains uncertain whether the association is causal or due to confounding or reverse causality. To further clarify the underlying causal relationship, we conducted a Mendelian randomization (MR) analysis.
Methods: We selected three genetic variants (rs1800562, rs1799945, and rs855791) closely correlated with four iron status biomarkers (serum iron, log-transformed ferritin, transferrin saturation, and transferrin) as instrumental variables. Summary statistics for prostate cancer were obtained from the Prostate Cancer Association Group to Investigate Cancer Associated Alterations in the Genome consortium including 79,148 cases and 61,106 controls of European ancestry. The inverse-variance weighted (IVW) method was conducted primarily to estimate the association of genetically predicted iron status and the risk of prostate cancer, supplemented with simple-median, weighted-median and maximum-likelihood methods as sensitivity analysis. MR-Egger regression was used to detect directional pleiotropy. We also conducted a meta-analysis of observational studies to assess the associations between iron status and the risk of prostate cancer.
Results: Genetically predicted increased iron status was associated with the decreased risk of prostate cancer, with odds ratio of 0.91 [95% confidence interval (CI): 0.84, 0.99; P = 0.035] for serum iron, 0.81 (95% CI: 0.65, 1.00; P = 0.046) for log- transformed ferritin, 0.94 (95% CI: 0.88, 0.99; P = 0.029) for transferrin saturation, and 1.15 (95% CI: 0.98, 1.35; P = 0.084) for transferrin (with higher transferrin levels representing lower systemic iron status), using the inverse-variance weighted method. Sensitivity analyses produced consistent associations, and MR-Egger regression indicated no potential pleiotropy. Our replication analysis based on FinnGen research project showed compatible results with our main analysis. Results from our meta-analysis similarly showed that serum ferritin [standardized mean difference (SMD): −1.25; 95% CI: −2.34, −0.16; P = 0.024] and transferrin saturation (SMD: −1.19; 95% CI: −2.34, −0.05; P = 0.042) were lower in patients with prostate cancer compared with that in controls.
Discussion: Our study suggests a protective role of iron in the risk of prostate cancer, further investigations are required to clarify the underlying mechanisms.
1 Introduction
Prostate cancer is the second most frequently diagnosed cancer and the fifth leading cause of cancer death in men, with 1.4 million new cases and 375,000 deaths worldwide in 2020 (1). Because aggressive forms of prostate cancer could commonly metastasize to bone and other parts of the body (2–4), the overall survival rate in cases with advanced and metastatic prostate cancer decreases markedly than those with localized prostate cancer, ranging from 26% to 30% at 5 years (5). To date, well-established risk factors of prostate cancer included advanced age, ethnicity, family history, and certain genetic mutations (1), whereas other risk factors remain undefined, such as nutritional status.
Iron is an essential element in basic biological processes and tightly linked to the utilization of oxygen and DNA synthesis (6). Accumulating observational studies have explored the associations of serum iron biomarkers (iron, ferritin, transferrin saturation, and transferrin) with the risk of prostate cancer. However, findings are conflicting. For example, a case-control study in America including 34 prostate cancer cases and 84 controls suggested that serum iron, ferritin, and transferrin saturation levels were lower in patients with prostate cancer than that in controls (7). However, a cohort study based on 114,847 Swedish populations including 1,505 patients with prostate cancer did not find statistically significant associations between serum iron and risk of prostate cancer (8). The inconsistent results from these studies may be due to differences in the study population, sample size, study design, and so on. In addition, the associations derived from conventional observational studies are susceptible to reverse causation and residual confounding (9). Therefore, it remains unclear whether the observed association was causal or not.
Mendelian randomization (MR) is a type of instrumental variable (IV) analysis for causal inference by utilizing genetic variants, such as single-nucleotide polymorphisms (SNPs) (10). SNPs are assumed to be randomly distributed in the general population in terms of Mendel’s laws of inheritance, which simulates a randomization process and thus enables MR analysis to minimize the confounding bias (11). As the genotype is randomly allocated at conception and cannot be changed by disease status, reverse causality could be eliminated in MR analysis. Moreover, a two-sample MR study design is harnessed for greater statistical power by obtaining genetic data in different populations (12).
In the current study, we conducted a two-sample MR study to assess the associations between iron biomarkers and the risk of prostate cancer. We also conducted a meta-analysis of observational studies to orient on the associations between iron status and the risk of prostate cancer.
2 Materials and methods
2.1 Mendelian randomization
The overall design for the current study is shown in Figure 1. We conducted a two-sample MR study to assess the associations between iron status (respectively reflecting serum iron, log-transformed ferritin, transferrin saturation, and transferrin) and the risk of prostate cancer based on summary data from genome-wide association studies (GWASs).
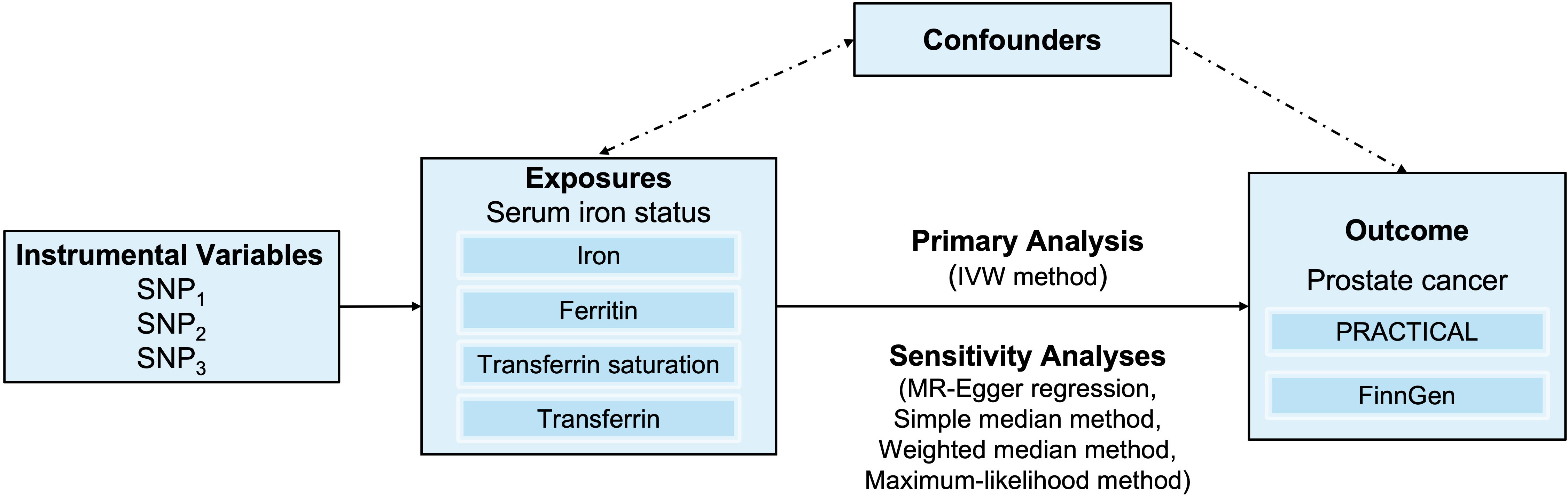
Figure 1 An overview of the study design. Abbreviations: IVW, inverse-variance weighted; SNP, single-nucleotide polymorphism. PRACTICAL, Prostate Cancer Association Group to Investigate Cancer Associated Alterations in the Genome.
2.1.1 Data sources
Summary statistics of prostate cancer in main analysis were obtained from a GWAS meta-analysis involving 79,148 cases and 61,106 controls of European ancestry conducted by Prostate Cancer Association Group to Investigate Cancer Associated Alterations in the Genome (PRACTICAL) consortium (13). The study performed a meta-analysis combining the summary statistics from a custom high-density array-OncoArray and seven previous prostate cancer GWAS or high-density SNP panels imputed to the 1000 Genomes Project of European ancestry. The summary statistics for replication were obtained from FinnGen database, containing 10,414 prostate cancer cases and 124,994 controls (14). The detailed information of the two projects (PRACTICAL and FinnGen) is listed in Supplementary Table 2. Because summary statistics of published studies were used, no additional ethical approval from an institutional review board was required.
2.1.3 Selection of instrumental SNPs
Instrumental SNPs of iron status were derived from a meta-analysis of 19 GWASs performed by the Genetics of Iron Status Consortium with a total sample size of 48,972 European individuals (15). Three SNPs (rs1800562, rs1799945, and rs855791) tightly related to four iron status biomarkers (all P-values< 5 × 10−8 and pairwise r2< 0.01) and consistent with an effect on systemic iron status (i.e., concordantly associated with increased levels of serum iron, log-transformed ferritin and transferrin saturation, and decreased levels of transferrin) were selected as IVs (Supplementary Table 1). The details of GWAS studies and datasets used in the present study are listed in Supplementary Table 2.
We calculated the proportion of variance (R2) explained by SNPs, using the formula 2×MAF×(1−MAF)×β2 (16). F-statistic was performed to assess the strength of the instrument (17). The minimum detectable odds ratios (ORs) to provide 80% statistical power at a significance level of 5% were calculated using an online tool (https://shiny.cnsgenomics.com/mRnd/) (18).
2.1.4 Statistical analysis
We first used the inverse-variance weighted (IVW) method to estimate the potential causal associations between genetically predicted iron status and the risk of prostate cancer. The IVW method combines the causal effect estimates of each IV by Wald ratio, having the greatest statistical power when all IVs are valid or the average pleiotropic is zero (balanced pleiotropy) (19, 20). A random-effects model was used to pool the Wald estimates in consideration of heterogeneity between SNPs. Cochran’s Q test was used to assess the heterogeneity from IVs due to pleiotropy or other causes. We further conducted several sensitivity analyses to evaluate the robustness of the results from IVW method. The simple median estimator can be thought of as a weighted median estimator with equal weights, which provides a consistent estimate of causal effect if at least 50% of IVs are valid, and the weighted median provides a robust estimate if at least 50% of the weight comes from valid IVs (21). Moreover, we performed MR-Egger regression to detect violations of the IV assumptions and assess potential directional pleiotropy. The intercept term was calculated as part of the analysis in MR-Egger method and could be regarded as the average pleiotropic effect across the genetic variants. The test on whether the intercept term differs from zero is so-called the MR-Egger intercept test (22).
All statistical analysis were performed using the “Mendelian Randomization” package in R version 4.1.0. P-values less than 0.05 were considered statistically significant, unless otherwise noted.
2.2 Meta-analysis
2.2.1 Search strategy
We conducted a systematic search through PubMed and Web of Science for publications on the associations between iron status and prostate cancer until 12 March 2022. The review protocol of this meta-analysis was registered in PROSPERO (CRD42022316784). The search terms used were as follows: (iron OR Fe OR ferritin OR hyperferritinemia OR transferrin OR transferrin saturation) AND (prostate cancer OR prostatic cancer OR prostate neoplasms OR prostatic neoplasms).
2.2.2 Literature inclusion and exclusion criteria
The inclusion criteria were as follows (1): study design of cross-sectional, case-control, or cohort; (2) prostate cancer cases and controls were distinguished according to clear diagnostic definition; and (3) the effect size of the association between iron status and prostate cancer was available, or there were sufficient data to calculate. The exclusion criteria were as follows: (1) article type of conference paper, protocol, abstract, review, or meta-analysis; (2) duplicate studies from different databases; and (3) if the population of individual studies overlapped, then the study with a smaller sample size was excluded.
2.2.3 Data extraction and quality assessment
Two authors (Song Jie and Ying Jiacheng) separately extracted data from each eligible study onto a form with standard specifications, including first author, publication year, region, type of study design, sample size, and effect size. All the extracted information was checked, and the differences were solved by discussion. Quality assessments were conducted according to the modified Newcastle-Ottawa Scale (NOS) with scores ranging from 0 to 9 points (23). Studies with a quality score of 7–9 were regarded as high quality, 5–6 as moderate quality, and ≤4 as low quality.
2.2.4 Statistical analysis
The mean and standard deviations of iron status and sample sizes between patients with prostate cancer and controls were used to calculate standardized mean differences (SMDs) and 95% confidence intervals (CIs) (24). For studies that provided OR, we converted OR to SMD by the formulae of (25) , and relative risk (RR) and hazard ratio (HR) approximate OR because the incidence of prostate cancer is relatively low (26). If the exposure category (iron status) was equal or greater than 3, then we used the lowest category as the reference and combined the risk estimates into a single estimate.
Cochrane’s Q test and I2 value were used to assess the heterogeneity (I2< 50% and P > 0.10, a fixed-effects model was used; otherwise, a random-effects model was applied). I² values of 25%, 50%, and 75% were considered low, moderate, and high heterogeneity, respectively. Publication bias was assessed by Egger’s test (27) and Begg’s test (28).
All statistical analyses were performed using STATA (version 15.1). Two-sided P-values less than 0.05 were considered statistically significant, unless otherwise noted.
3 Results
3.1 MR analysis
The F statistics for the instrumental SNPs ranged from 46.90 to 2,126.74 across the four biomarkers of iron status, all of which reached the conventional threshold of 10 (known as a rule of thumb to identify strong or weak instruments). The proportion of variance explained by the selected instrumental SNPs was about 3.9%, 0.8%, 7.3%, and 3.3% for serum iron, log-transformed ferritin, transferrin saturation, and transferrin, respectively. The minimum detectable ORs were 1.08, 1.19, 1.06, and 1.09, respectively, with 80% statistical power. Detailed information is listed in Supplementary Table 3.
Forest plot of the MR estimates from the three instruments for the four iron biomarkers on the risk of prostate cancer is shown in Figure 2. Cochran’s Q test showed that no significant heterogeneity existed among the three instrumental SNPs for the association between iron status and risk of prostate cancer (P = 0.133 for serum iron, P = 0.135 for log-transformed ferritin, P = 0.169 for transferrin saturation, and P = 0.109 for transferrin). The effect estimates by IVW method suggested the protective role of increased iron status on prostate cancer, with ORs of 0.91 (95% CI: 0.84, 0.99; P = 0.035), 0.81 (95% CI: 0.65, 1.00; P = 0.046), 0.94 (95% CI: 0.88, 0.99; P = 0.029), and 1.15 (95% CI: 0.98, 1.35; P = 0.084) for genetically predicted increased serum iron, log-transformed ferritin, transferrin saturation, and transferrin (Table 1). As shown in Table 1 and Supplementary Figure 2, alternative MR methods using simple-median method, weighted-median method, and likelihood-based method produced similar results. The MR-Egger regression did not find potential directional pleiotropy (serum iron: intercept = 0.011, P = 0.829; log-transformed ferritin: intercept = −0.006, P = 0.805; transferrin saturation: intercept = −0.001, P = 0.955; transferrin: intercept = 0.009, P = 0.533).
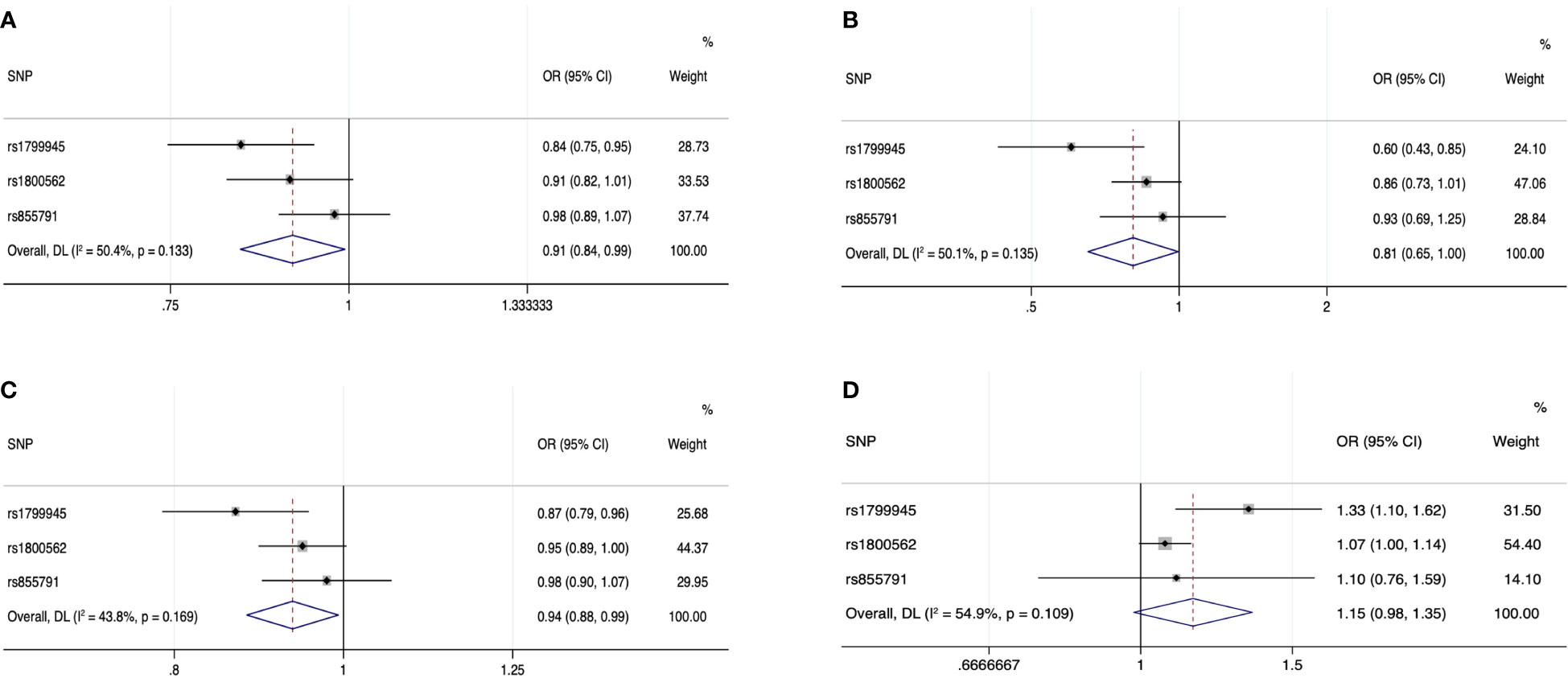
Figure 2 Forest plot of the MR estimates from the three instruments among European populations for serum iron (A), log-transformed ferritin (B), transferrin saturation (C), and transferrin (D) on prostate cancer risk. The size of the squares is proportional to the precision of the MR estimates for each SNP, with the horizontal lines indicating their 95% CIs. The combined MR estimate is represented by the center of the diamond, with the lateral tips indicating its 95% CI. The solid vertical line is the line of no effect. Abbreviations: CI, confidence interval; MR, Mendelian randomization; SNP, single-nucleotide polymorphism.
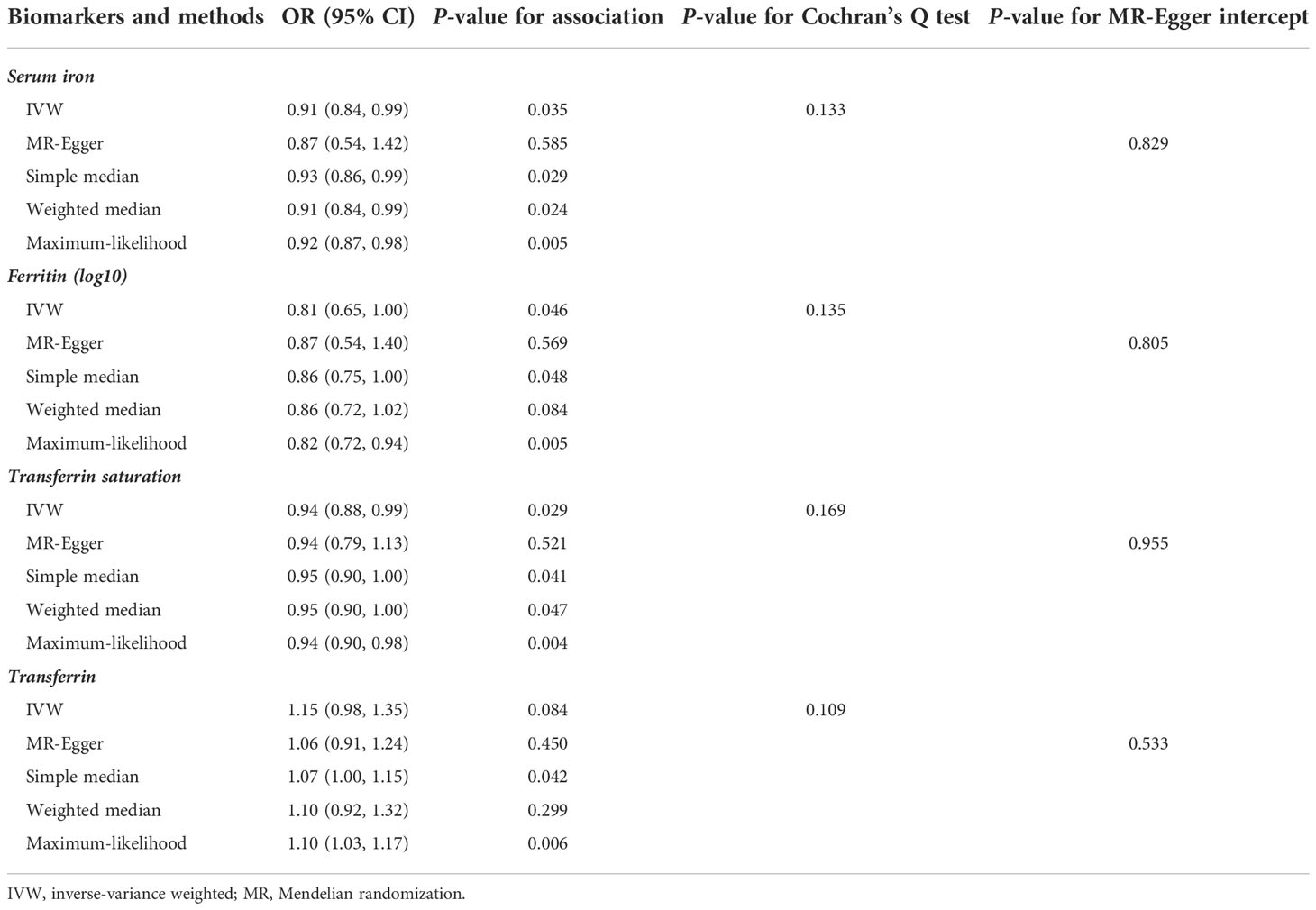
Table 1 MR analysis estimates for each iron biomarkers on the risk of prostate cancer among European populations.
The MR analysis was validated using summary statistic generated by FinnGen research project (Figure 3). The point estimates in FinnGen gave the compatible directions with our main analysis in PRACTICAL, although the results were not statistically significant. The combined effect sizes were also consistent with our findings in main analysis for iron (OR: 0.91; 95% CI: 0.84, 0.98; P = 0.009), ferritin (OR: 0.80; 95% CI: 0.65, 0.98; P = 0.028), transferrin saturation (OR: 0.94; 95% CI: 0.89, 0.99; P = 0.020), and transferrin (OR: 1.17; 95% CI: 1.00, 1.35; P = 0.046).
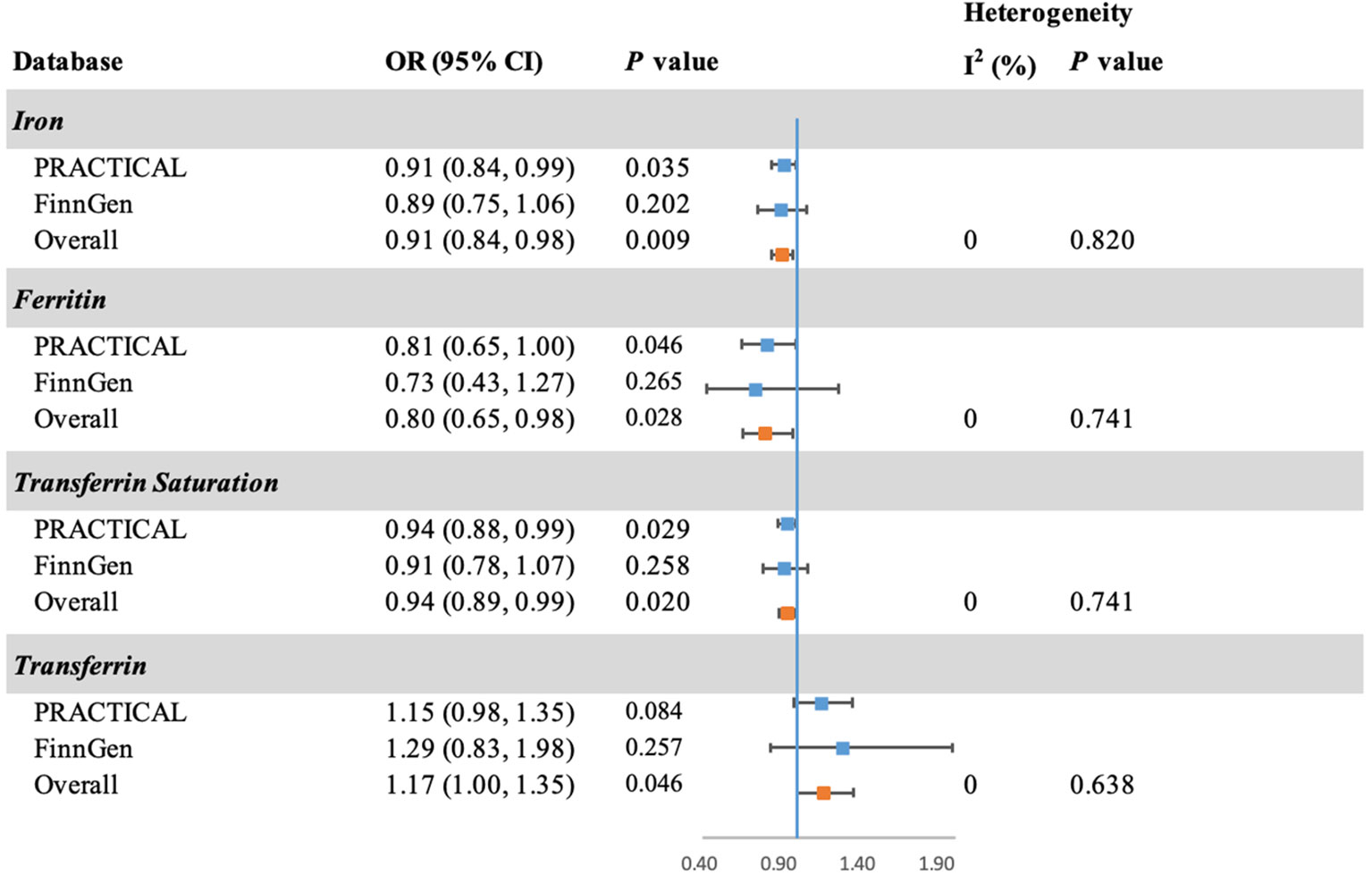
Figure 3 Forest plot of the MR estimates of iron status on prostate cancer in PRACTICAL and FinnGen. The overall odds ratios with 95% CIs were generated using random-effects model. Abbreviations: PRACTICAL, Prostate Cancer Association Group to Investigate Cancer Associated Alterations in the Genome; CI, confidence interval.
3.2 Meta-analysis
Supplementary Figure 1 presents the process of study inclusion and exclusion of the meta-analysis. A total of 10 articles (six retrospective studies and four prospective studies) were identified, which investigated the association of serum iron, ferritin, and transferrin saturation with the risk of prostate cancer. We did not perform meta-analysis for transferrin because the number of eligible studies was one. Detailed information of the included studies is displayed in Table 2.
Forest plots of the three iron status biomarkers in patients with prostate cancer relative to controls are displayed in Supplementary Figure 3. Consistently, we found lower serum ferritin (SMD: −1.25; 95% CI: −2.34, −0.16; P = 0.024) and transferrin saturation (SMD: −1.19; 95% CI: –2.34, −0.05; P = 0.042) levels in patients with prostate cancer compared with that in controls. However, we did not find statistically significant differences in serum iron levels between cases and controls (SMD: −0.09; 95% CI: −0.35, 0.18; P = 0.503). In addition, no evidence of publication bias was detected by Begg’s test (iron: P = 0.858, ferritin: P = 1.000, transferrin saturation: P = 1.000) or Egger’s test (iron: P = 0.790, ferritin: P = 0.347, transferrin saturation: P = 0.396).
4 Discussion
In the current study, we performed a MR and meta-analysis to investigate the association of iron status with the risk of prostate cancer. Our results suggested a protective role of iron in the development of prostate cancer.
Several observational studies have found that serum iron biomarkers were higher in patients with prostate cancer than that in the control group. For example, a case-control study of 74 patients with prostate cancer and 66 healthy controls found that the serum iron was significantly higher in patients with prostate cancer compared with that in healthy controls (mean ± standard error: 850.8 ± 46.52 vs. 464.3 ± 27.38; P< 0.001) (29). Similarly, a recent study conducted by Saleh et al. (30) observed similar result for serum iron (1.96 ± 0.58 vs. 1.21 ± 0.27; P< 0.001, cases vs. controls). On the contrary, a case-control study in America including 34 patients with prostate cancer and 84 controls reported lower serum iron, ferritin, and transferrin saturation in patients with prostate cancer than that in controls (7). Kaba et al. (31) found that the serum iron was lower in the sera samples of 30 patients with prostate cancer than those in 32 healthy controls (0.76 ± 0.43 vs. 2.42 ± 0.19; P< 0.001). However, the other six studies (two case-control, one case-cohort, and three cohort studies) did not find statistically significant associations between iron status and risk of prostate cancer (8, 32–36). To our knowledge, the current study is the first to systematically review and perform a meta-analysis of the relationship between iron status and the risk of prostate cancer. A total of 260,189 participants (including 2,906 cases) were included in our meta-analysis, which revealed statistically significant associations of increased serum ferritin and transferrin saturation levels with the decreased risk of prostate cancer. However, we did not find associations between serum iron and the risk of prostate cancer, and there was only one study investigating the association of transferrin and prostate cancer, which reported that transferrin was not significantly associated with the risk of prostate cancer.
It is a fact that there were limitations in the meta-analysis. First, because of the limited number of included studies, the result of ferritin and transferrin saturation may not be stable. Second, heterogeneity was at a relatively high level across included observational studies in our meta-analysis. It may result from the study designs, various regions, study quality, baseline characteristics of participants, and potential measurement error. However, stratified analysis was not available for the current study, which needs to be further explored in the future research. Moreover, six of the 10 studies on serum iron were case-control studies with small sample size, which might be biased by reverse causality and confounding. Therefore, the causal direction remains uncertain. Furthermore, we applied a two-sample MR approach to investigate the associations between genetically predicted iron status and the risk of prostate cancer.
To ensure a credible conclusion in the MR study, the selected IVs must satisfy the following three assumptions. The first assumption is that the IV is strongly associated with the exposure. In the current study, all IVs were selected from a GWAS meta-analysis at a genome-wide significance threshold (P-value< 5 × 10−8). The second assumption is that the IV is not associated with the outcome via a confounding pathway. This assumption is like an assurance that all variables except the exposure (which refers to iron status in our study) are distributed equally between the subgroups; hence, no confounders would interfere with the exposure-outcome association. Because genotype is presumed to be randomly allocated at conception, covariates are anticipated to be randomly distributed with respect to genotypes. The third assumption is that the IV affects the outcome through their effects on exposure directly but not through any alternative pathways. Accordingly, we conducted Cochran’s Q test and MR-Egger intercept test, and no potential pleiotropy was detected. Moreover, to prove the stability of the results from MR study, we performed alternative MR methods, i.e., simple-median method, weighted-median method, and likelihood-based method, which gave consistent results on the associations between iron status and the risk of prostate cancer and hence proved the robustness of our findings.
To date, the effect of iron on cancer risk is controversial, and there might be discrepancies in the association of iron with different types of cancer. For example, a cohort study conducted by Ellervik et al. found that participants with higher transferrin saturation levels were more likely to develop liver cancer than those with lower transferrin saturation levels (37). Conversely, a nested case-control study conducted by Fonseca-Nunes et al. found a statistically significant inverse association of ferritin and transferrin with gastric cancer (38). Moreover, rising evidence showed that low systemic iron status and reduced iron intake were associated with the pathogenesis of colorectal cancer (39, 40). Although the mechanism for how serum iron status alters the risk of prostate cancer remains unknown, there are possible hypotheses in support of the protective role of iron in prostate cancer. Iron may protect against prostate cancer through ferroptosis, a form of regulated cell death that is recently found to act in inhibiting some types of cancers including prostate cancer (41). Although ferroptosis is only confirmed to function in pathological cells, a recent study suggested that it may also be triggered in normal cells by accumulation of iron (42). Furthermore, a recent experimental study proved the inhibition of iron toxicity on the proliferation of prostate cancer cells, which primarily affected lipids, promoting ferroptosis (43). Moreover, ferritin is a hollow protein shell composed of two functionally distinct subunits, namely, H-subunit and L-subunit and capable of storing up to 4500 Fe (III) atoms within a single molecule (44). H-subunit acts as a ferroxidase that transforms highly toxic Fe (II) to the mild Fe (III) form, thereby enabling ferritin to sequester it in its cavity (45). Its capability of sequestering free Fe gives ferritin the function of iron detoxification and thus may reduce the incidence of iron overload, causing a decreased risk of prostate cancer. Further investigation is warranted to elucidate the mechanisms behind the protective role of high iron status on prostate cancer.
There are several strengths in our study. First, we used the largest summary statistics of prostate cancer that we know of, which included 79,148 cases and 61,106 controls of European ancestry (13). The large sample size gave us adequate power to estimate the potential causal relationship between iron status and risk of prostate cancer, with 0.87 for serum iron, 0.88 for log-transformed ferritin, 0.89 for transferrin saturation, and 0.86 for transferrin, respectively. Second, our study is the first meta-analysis on the relationship between iron status and risk of prostate cancer, which provides a comprehensive comparison with our MR findings.
There are limitations in our study. First, although the selected IVs reached the genome-wide significant threshold to satisfy the first assumption, the proportion of the variance explained by these selected SNPs for the four biomarkers was limited, with R2 of 3.87%, 0.78%, 7.31%, and 3.30% for serum iron, log-transformed ferritin, transferrin saturation, and transferrin, respectively. Therefore, more strong IVs are warranted to improve the power. Second, there can still be potential residual bias as the biological functions of the selected SNPs remains unknown, although our analysis did not find potential pleiotropy of selected SNPs by MR-Egger analysis. Third, because the region of participants was limited to Europe in MR study, whether our finds can be extrapolated to other populations remains unknown. Fourth, our analysis could only detect the linear relation of iron status and risk of prostate cancer due to the limitation of summary-level data used in the MR study. Further analysis with individual-level data is required to evaluate the potential dose-response relationship of iron status and risk of prostate cancer. Finally, our study evaluates the lifelong effect of genetically predicted iron status on the risk of prostate cancer, which does not take the effect of acute changes in iron status into consideration.
In conclusion, our study provided evidence of a potential protective role of iron in the development of prostate cancer. Further studies are warranted to identify the mechanisms, as well as the application prospect of iron supplement in clinical cancer prevention and treatment.
Data availability statement
Publicly available datasets were analyzed in this study. This data can be found here: PRACTICAL: http://practical.icr.ac.uk, FinnGen: https://finngen.gitbook.io/documentation/.
Author contributions
DY and XS conceived the idea for the study. SH and JS obtained the genetic data. JY, SH, KL, and WC performed the data analyses. JY, BW, and JS interpreted the results of the data analyses. JY wrote the manuscript, and YM and DY revised the manuscript. All authors contributed to the article and approved the submitted version.
Funding
This work was jointly supported by grants from Natural Science Foundation of Zhejiang Province (LQ20H260008 and LQ21H260001), National Natural Science Foundation of China (82103936), and Zhejiang Chinese Medical University Foundation (2020ZG01 and 2020ZG16).
Acknowledgments
We thank all the researchers and participants of the studies involved in the meta-analysis and Mendelian randomization. We want to acknowledge the participants and investigators of PRACTICAL and FinnGen.
Conflict of interest
The authors declare that the research was conducted in the absence of any commercial or financial relationships that could be construed as a potential conflict of interest.
Publisher’s note
All claims expressed in this article are solely those of the authors and do not necessarily represent those of their affiliated organizations, or those of the publisher, the editors and the reviewers. Any product that may be evaluated in this article, or claim that may be made by its manufacturer, is not guaranteed or endorsed by the publisher.
Supplementary material
The Supplementary Material for this article can be found online at: https://www.frontiersin.org/articles/10.3389/fonc.2022.959892/full#supplementary-material
References
1. Sung H, Ferlay J, Siegel RL, Laversanne M, Soerjomataram I, Jemal A, et al. Global cancer statistics 2020: GLOBOCAN estimates of incidence and mortality worldwide for 36 cancers in 185 countries. CA: Cancer J Clin (2021) 71(3):209–49. doi: 10.3322/caac.21660
2. Della Pepa C, Cavaliere C, Rossetti S, Di Napoli M, Cecere SC, Crispo A, et al. Predictive comprehensive geriatric assessment in elderly prostate cancer patients: the prospective observational scoop trial results. Anti-cancer Drugs (2017) 28(1):104–9. doi: 10.1097/CAD.0000000000000428
3. Loberg RD, Logothetis CJ, Keller ET, Pienta KJ. Pathogenesis and treatment of prostate cancer bone metastases: targeting the lethal phenotype. J Clin Oncol (2005) 23(32):8232–41. doi: 10.1200/JCO.2005.03.0841
4. Veccia A, Caffo O, De Giorgi U, Di Lorenzo G, Ortega C, Scognamiglio F, et al. Clinical outcomes in octogenarians treated with docetaxel as first-line chemotherapy for castration-resistant prostate cancer. Future Oncol (London England) (2016) 12(4):493–502. doi: 10.2217/fon.15.302
5. Steele CB, Li J, Huang B, Weir HK. Prostate cancer survival in the united states by race and stage (2001-2009): Findings from the CONCORD-2 study. Cancer (2017) 123(Suppl 24):5160–77. doi: 10.1002/cncr.31026
6. Toyokuni S. Role of iron in carcinogenesis: Cancer as a ferrotoxic disease. Cancer Sci (2009) 100(1):9–16. doi: 10.1111/j.1349-7006.2008.01001.x
7. Kuvibidila SR, Gauthier T, Rayford W. Serum ferritin levels and transferrin saturation in men with prostate cancer. J Natl Med Assoc (2004) 96(5):641–9.
8. Gaur A, Collins H, Wulaningsih W, Holmberg L, Garmo H, Hammar N, et al. Iron metabolism and risk of cancer in the Swedish AMORIS study. Cancer causes Control (2013) 24(7):1393–402. doi: 10.1007/s10552-013-0219-8
9. Smith GD, Ebrahim S. 'Mendelian randomization': Can genetic epidemiology contribute to understanding environmental determinants of disease? Int J Epidemiol (2003) 32(1):1–22. doi: 10.1093/ije/dyg070
10. Burgess S, Small DS, Thompson SG. A review of instrumental variable estimators for mendelian randomization. Stat Methods Med Res (2017) 26(5):2333–55. doi: 10.1177/0962280215597579
11. Burgess S, Scott RA, Timpson NJ, Davey Smith G, Thompson SG. Using published data in mendelian randomization: a blueprint for efficient identification of causal risk factors. Eur J Epidemiol (2015) 30(7):543–52. doi: 10.1007/s10654-015-0011-z
12. Bentham J, Morris DL, Graham DSC, Pinder CL, Tombleson P, Behrens TW, et al. Genetic association analyses implicate aberrant regulation of innate and adaptive immunity genes in the pathogenesis of systemic lupus erythematosus. Nat Genet (2015) 47(12):1457–64. doi: 10.1038/ng.3434
13. Schumacher FR, Al Olama AA, Berndt SI, Benlloch S, Ahmed M, Saunders EJ, et al. Association analyses of more than 140,000 men identify 63 new prostate cancer susceptibility loci. Nat Genet (2018) 50(7):928–36. doi: 10.1038/s41588-018-0142-8
14. Kurki MI, Karjalainen J, Palta P, Sipilä TP, Kristiansson K, Donner K, et al. Finngen research project is an expedition to the frontier of genomics and medicine [Internet]. In: FinnGen (2022). Available at: https://www.finngen.fi/fi/finngen_tutkimushanke_vie_suomalaiset_loytoretkelle_genomitietoon.
15. Benyamin B, Esko T, Ried JS, Radhakrishnan A, Vermeulen SH, Traglia M, et al. Novel loci affecting iron homeostasis and their effects in individuals at risk for hemochromatosis. Nat Commun (2014) 5:4926. doi: 10.1038/ncomms5926
16. Park JH, Wacholder S, Gail MH, Peters U, Jacobs KB, Chanock SJ, et al. Estimation of effect size distribution from genome-wide association studies and implications for future discoveries. Nat Genet (2010) 42(7):570–5. doi: 10.1038/ng.610
17. Palmer TM, Lawlor DA, Harbord RM, Sheehan NA, Tobias JH, Timpson NJ, et al. Using multiple genetic variants as instrumental variables for modifiable risk factors. Stat Methods Med Res (2012) 21(3):223–42. doi: 10.1177/0962280210394459
18. Brion MJ, Shakhbazov K, Visscher PM. Calculating statistical power in mendelian randomization studies. Int J Epidemiol (2013) 42(5):1497–501. doi: 10.1093/ije/dyt179
19. Lawlor DA, Harbord RM, Sterne JA, Timpson N, Davey Smith G. Mendelian randomization: using genes as instruments for making causal inferences in epidemiology. Stat Med (2008) 27(8):1133–63. doi: 10.1002/sim.3034
20. Burgess S, Butterworth A, Thompson SG. Mendelian randomization analysis with multiple genetic variants using summarized data. Genet Epidemiol (2013) 37(7):658–65. doi: 10.1002/gepi.21758
21. Bowden J, Davey Smith G, Haycock PC, Burgess S. Consistent estimation in mendelian randomization with some invalid instruments using a weighted median estimator. Genet Epidemiol (2016) 40(4):304–14. doi: 10.1002/gepi.21965
22. Burgess S, Thompson SG. Interpreting findings from mendelian randomization using the MR-egger method. Eur J Epidemiol (2017) 32(5):377–89. doi: 10.1007/s10654-017-0255-x
23. Stang A. Critical evaluation of the Newcastle-Ottawa scale for the assessment of the quality of nonrandomized studies in meta-analyses. Eur J Epidemiol (2010) 25(9):603–5. doi: 10.1007/s10654-010-9491-z
24. Hozo SP, Djulbegovic B, Hozo I. Estimating the mean and variance from the median, range, and the size of a sample. BMC Med Res Methodol (2005) 5:13. doi: 10.1186/1471-2288-5-13
25. Chinn S. A simple method for converting an odds ratio to effect size for use in meta-analysis. Stat Med (2000) 19(22):3127–31. doi: 10.1002/1097-0258(20001130)19:22<3127::AID-SIM784>3.0.CO;2-M
26. Egger M, Smith GD, Phillips AN. Meta-analysis: principles and procedures. Bmj (1997) 315(7121):1533–7. doi: 10.1136/bmj.315.7121.1533
27. Egger M, Davey Smith G, Schneider M, Minder C. Bias in meta-analysis detected by a simple, graphical test. Bmj (1997) 315(7109):629–34. doi: 10.1136/bmj.315.7109.629
28. Begg CB, Mazumdar M. Operating characteristics of a rank correlation test for publication bias. Biometrics (1994) 50(4):1088–101. doi: 10.2307/2533446
29. Qayyum MA, Shah MH. Comparative study of trace elements in blood, scalp hair and nails of prostate cancer patients in relation to healthy donors. Biol Trace element Res (2014) 162(1-3):46–57. doi: 10.1007/s12011-014-0123-4
30. Saleh SAK, Adly HM, Abdelkhaliq AA, Nassir AM. Serum levels of selenium, zinc, copper, manganese, and iron in prostate cancer patients. Curr Urol (2020) 14(1):44–9. doi: 10.1159/000499261
31. Kaba M, Pirincci N, Yuksel MB, Gecit I, Gunes M, Ozveren H, et al. Serum levels of trace elements in patients with prostate cancer. Asian Pacific J Cancer Prev (2014) 15(6):2625–9. doi: 10.7314/APJCP.2014.15.6.2625
32. Quintana Pacheco DA, Sookthai D, Graf ME, Schübel R, Johnson T, Katzke VA, et al. Iron status in relation to cancer risk and mortality: Findings from a population-based prospective study. Int J Cancer. (2018) 143(3):561–9. doi: 10.1002/ijc.31384
33. Chua AC, Knuiman MW, Trinder D, Divitini ML, Olynyk JK. Higher concentrations of serum iron and transferrin saturation but not serum ferritin are associated with cancer outcomes. Am J Clin Nutr (2016) 104(3):736–42. doi: 10.3945/ajcn.115.129411
34. Wen CP, Lee JH, Tai YP, Wen C, Wu SB, Tsai MK, et al. High serum iron is associated with increased cancer risk. Cancer Res (2014) 74(22):6589–97. doi: 10.1158/0008-5472.CAN-14-0360
35. Adedapo KS, Arinola OG, Shittu OB, Kareem OI, Okolo CA, Nwobi LN. Diagnostic value of lipids, total antioxidants, and trace metals in benign prostate hyperplasia and prostate cancer. Nigerian J Clin Practice (2012) 15(3):293–7. doi: 10.4103/1119-3077.100623
36. Ozmen H, Erulas FA, Karatas F, Cukurovali A, Yalcin O. Comparison of the concentration of trace metals (Ni, zn, Co, Cu and Se), fe, vitamins a, c and e, and lipid peroxidation in patients with prostate cancer. Clin Chem Lab Med (2006) 44(2):175–9. doi: 10.1515/CCLM.2006.032
37. Ellervik C, Tybjaerg-Hansen A, Nordestgaard BG. Risk of cancer by transferrin saturation levels and haemochromatosis genotype: population-based study and meta-analysis. J Intern Med (2012) 271(1):51–63. doi: 10.1111/j.1365-2796.2011.02404.x
38. Fonseca-Nunes A, Agudo A, Aranda N, Arija V, Cross AJ, Molina E, et al. Body iron status and gastric cancer risk in the EURGAST study. Int J Cancer (2015) 137(12):2904–14. doi: 10.1002/ijc.29669
39. Phipps O, Brookes MJ, Al-Hassi HO. Iron deficiency, immunology, and colorectal cancer. Nutr Rev (2021) 79(1):88–97. doi: 10.1093/nutrit/nuaa040
40. Cross AJ, Gunter MJ, Wood RJ, Pietinen P, Taylor PR, Virtamo J, et al. Iron and colorectal cancer risk in the alpha-tocopherol, beta-carotene cancer prevention study. Int J Cancer (2006) 118(12):3147–52. doi: 10.1002/ijc.21780
41. Tang M, Chen Z, Wu D, Chen L. Ferritinophagy/ferroptosis: Iron-related newcomers in human diseases. J Cell Physiol (2018) 233(12):9179–90. doi: 10.1002/jcp.26954
42. Stockwell BR, Friedmann Angeli JP, Bayir H, Bush AI, Conrad M, Dixon SJ, et al. Ferroptosis: A regulated cell death nexus linking metabolism, redox biology, and disease. Cell (2017) 171(2):273–85. doi: 10.1016/j.cell.2017.09.021
43. Bordini J, Morisi F, Elia AR, Santambrogio P, Pagani A, Cucchiara V, et al. Iron induces cell death and strengthens the efficacy of antiandrogen therapy in prostate cancer models. Clin Cancer Res (2020) 26(23):6387–98. doi: 10.1158/1078-0432.CCR-20-3182
44. Ford GC, Harrison PM, Rice DW, Smith JM, Treffry A, White JL, et al. Ferritin: design and formation of an iron-storage molecule. Philos Trans R Soc Lond B Biol Sci (1121) 1984:551–65:304. doi: 10.1098/rstb.1984.0046
Keywords: iron, ferritin, transferrin saturation, transferrin, prostate cancer, Mendelian randomization, meta-analysis
Citation: Ying J, Wang B, Han S, Song J, Liu K, Chen W, Sun X, Mao Y and Ye D (2022) Genetically predicted iron status was associated with the risk of prostate cancer. Front. Oncol. 12:959892. doi: 10.3389/fonc.2022.959892
Received: 02 June 2022; Accepted: 08 November 2022;
Published: 06 December 2022.
Edited by:
W. Nathaniel Brennen, Johns Hopkins Medicine, United StatesReviewed by:
Jungeun Lim, National Cancer Institute (NIH), United StatesChristina Ellervik, Harvard Medical School, United States
Copyright © 2022 Ying, Wang, Han, Song, Liu, Chen, Sun, Mao and Ye. This is an open-access article distributed under the terms of the Creative Commons Attribution License (CC BY). The use, distribution or reproduction in other forums is permitted, provided the original author(s) and the copyright owner(s) are credited and that the original publication in this journal is cited, in accordance with accepted academic practice. No use, distribution or reproduction is permitted which does not comply with these terms.
*Correspondence: Ding Ye, eWVkaW5nQHpjbXUuZWR1LmNu; Yingying Mao, bXl5QHpjbXUuZWR1LmNu
†These authors have contributed equally to this work and share first authorship