- 1Leibniz Institute for Prevention Research and Epidemiology – BIPS, Bremen, Germany
- 2Faculty of Human and Health Sciences, University of Bremen, Bremen, Germany
- 3Institute of Medical Biostatistics, Epidemiology and Informatics (IMBEI), University Medical Center of the Johannes Gutenberg University Mainz, Mainz, Germany
- 4Institute of Organismic and Molecular Evolution, Molecular Genetics and Genome Analysis, Johannes Gutenberg University Mainz, Mainz, Germany
- 5Department of Radiation Oncology and Radiation Therapy, University Medical Center of the Johannes Gutenberg University Mainz, Mainz, Germany
- 6Radiation Biology and DNA Repair, Technical University of Darmstadt, Darmstadt, Germany
- 7Division of Childhood Cancer Epidemiology, German Childhood Cancer Registry, Institute of Medical Biostatistics, Epidemiology and Informatics (IMBEI), University Medical Center of the Johannes Gutenberg University Mainz, Mainz, Germany
Introduction: Long non-coding ribonucleic acids (lncRNAs) are involved in the cellular damage response following exposure to ionizing radiation as applied in radiotherapy. However, the role of lncRNAs in radiation response concerning intrinsic susceptibility to late effects of radiation exposure has not been examined in general or in long-term survivors of childhood cancer with and without potentially radiotherapy-related second primary cancers, in particular.
Methods: Primary skin fibroblasts (n=52 each) of long-term childhood cancer survivors with a first primary cancer only (N1), at least one second primary neoplasm (N2+), as well as tumor-free controls (N0) from the KiKme case-control study were matched by sex, age, and additionally by year of diagnosis and entity of the first primary cancer. Fibroblasts were exposed to 0.05 and 2 Gray (Gy) X-rays. Differentially expressed lncRNAs were identified with and without interaction terms for donor group and dose. Weighted co-expression networks of lncRNA and mRNA were constructed using WGCNA. Resulting gene sets (modules) were correlated to the radiation doses and analyzed for biological function.
Results: After irradiation with 0.05Gy, few lncRNAs were differentially expressed (N0: AC004801.4; N1: PCCA-DT, AF129075.3, LINC00691, AL158206.1; N2+: LINC02315). In reaction to 2 Gy, the number of differentially expressed lncRNAs was higher (N0: 152, N1: 169, N2+: 146). After 2 Gy, AL109976.1 and AL158206.1 were prominently upregulated in all donor groups. The co-expression analysis identified two modules containing lncRNAs that were associated with 2 Gy (module1: 102 mRNAs and 4 lncRNAs: AL158206.1, AL109976.1, AC092171.5, TYMSOS, associated with p53-mediated reaction to DNA damage; module2: 390 mRNAs, 7 lncRNAs: AC004943.2, AC012073.1, AC026401.3, AC092718.4, MIR31HG, STXBP5-AS1, TMPO-AS1, associated with cell cycle regulation).
Discussion: For the first time, we identified the lncRNAs AL158206.1 and AL109976.1 as involved in the radiation response in primary fibroblasts by differential expression analysis. The co-expression analysis revealed a role of these lncRNAs in the DNA damage response and cell cycle regulation post-IR. These transcripts may be targets in cancer therapy against radiosensitivity, as well as provide grounds for the identification of at-risk patients for immediate adverse reactions in healthy tissues. With this work we deliver a broad basis and new leads for the examination of lncRNAs in the radiation response.
1 Introduction
About 70% of the human genome is transcribed into ribonucleic acids (RNA) (1, 2), whereas only 2-3% are subsequently translated into proteins (1, 3, 4). The transcripts that are not coding for any proteins are called non-coding RNAs (ncRNAs) and can be divided into small (e.g., micro-RNAs (miRNAs) and small interfering RNAs, < 200 nucleotides in length) and long non-coding RNAs (lncRNAs, longer than 200 nucleotides (5)). Contrary to messenger RNAs (mRNAs), ncRNAs are direct effectors not necessitating prior translation into proteins (5). Recently, RNAs have been identified as important players in the cellular response to ionizing radiation (IR), particularly by affecting the deoxyribonucleic acid (DNA) damage response (6). Involvement has been documented for several lncRNAs in response to high doses of IR (HDIR, ≥ 2Gy) (7) as well as low doses of IR (LDIR, ≤ 0.5Gy, (8)). lncRNAs can directly act as part of the damage response by binding repair factors at the site of a radiation-induced double-strand break or indirectly by regulating the cell cycle, transcription and/or translation, as well as working of miRNAs through acting as miRNA sponges (6). Moreover, lncRNAs can influence chromatin organization and regulate gene expression (9). While the clinical application of IR procures a high benefit in radiology and radiotherapy, the involved health risks due to unavoidable exposure of healthy tissue is not to be underestimated. These include deterministic acute tissue toxicities and stochastic long-term effects associated with HDIR as well as LDIR (10). The latter include radiogenic tumors, which can occur as second primary malignancies after radiotherapy with latency periods of several years to decades in long-term survivors of cancer (11). In particular, long-term survivors of childhood cancer are at the highest risk for late sequelae of DNA-damaging tumor therapies, including the development of a second primary malignancy (12) In Germany, 8% of long-term survivors of a tumor before age 15 develop a second primary malignancy within 30 years after the first cancer diagnosis (13). HDIR applied to the tumor volume during radiotherapy as well as to normal tissue at its margins is an established risk factor for second primary malignancies (14, 15), but also LDIR is considered a risk factor for carcinogenic late effects of IR exposure (15, 16). LDIR occurs as out-of-field doses in radiotherapy, e.g., as peripheral leakage and scatter radiation (17), but on a much larger civilizational scale through radiological imaging procedures such as computed tomography showing a dramatic rise in application through recent decades (18). However, the basic molecular mechanisms and intrinsic susceptibility to various adverse effects of the medical use of IR, particularly the occurrence of second primary cancers, have not been unraveled yet. In this context, this also applies to the role of lncRNAs in the cellular response to IR. To date, lncRNA expression analyses have been performed in a very limited number of studies in peripheral blood lymphocytes after very high radiation doses [60 Gy (19)] or in human (cancer) cell lines (20–23), rarely investigating the response to LDIR (20, 21).
The present study is the first to investigate the expression of lncRNAs in primary fibroblasts from a large population-based nested case-control study comprising long-term survivors of childhood cancer without (N1) or with at least one second primary malignancy (N2+), and cancer-free controls (N0) after HDIR (2 Gy) and LDIR (0.05 Gy). In addition, a co-expression network analysis with protein-coding transcripts was performed to decipher novel gene signatures and functions of lncRNAs in the cellular radiation response.
2 Materials and methods
2.1 Study design and participants
To examine the functional network between hereditary dispositions for sporadic childhood cancer, subsequent iatrogenic second primary neoplasms, and the cellular reaction to IR, the KiKme study was established (24). For this purpose, 591 participants were recruited from 2013 to 2019 and included in this nested case-control study. To this date, a body of work has been published, outlining the overall design, including a detailed description of participants and the analysis plan (24), determination of the experimental conditions yielding the most differentially expressed genes and overall experimental design (25), as well as an in-depth functional analysis of the protein-coding transcripts (26). For the latter as well as the project at hand, 156 participants (52 cancer-free controls, 52 long-term survivors of childhood cancer without, and 52 with at least one second primary neoplasm) over 18 years of age were selected and grouped in triplets (one of each donor group), matching them by age at sampling and sex. The two long-term childhood cancer survivors per triplet were additionally matched by first neoplasm, as well as age at and year of diagnosis. The cancer-free controls were recruited from patients at the Department of Orthopedic Surgery at the University Medical Center Mainz that were subject to elective procedures not associated with cancer. The long-term cancer survivors were selected if the first primary neoplasm was among the three most common pediatric cancers (leukemia, lymphoma, or a tumor of the central nervous system). To ensure that the second primary neoplasm had a potential radiogenic origin, all included second primary neoplasm occurred at an anatomic site that may have been exposed during a potential radiotherapy of the first primary neoplasm. [e.g., thyroid carcinoma, breast cancer, skin carcinoma, malignant melanoma, leukemia, or ependymomas and choroid plexus tumors (27)].
2.2 Samples and experiments
For this project, primary skin fibroblasts were obtained from 156 donors selected among the participants of the KiKme study. These samples were collected as 3 mm punch biopsies from the inside of the cubital region among long-term childhood cancer survivors and from the scar region of the surgery among cancer-free controls. For exposure to IR, cells were cultured and synchronized in G1 by confluency. The exposure to 2 Gy was created with 140 kilovolt X-rays at a dose rate of 3.62 Gy per minute for 0.55 minutes, exposure to 0.05 Gy with 50 kilovolt X-rays at a dose rate of 0.34 Gy per minute for 0.15 minutes. Both experiments were done using the D3150 X-ray Therapy System (Gulmay Medical Ltd, Byfleet, UK). The exposure to 0 Gy (sham-irradiation) was achieved by keeping the cells in the radiation device control room. All experiments occurred at room temperature, processing the triplets together in order to avoid batch effects. RNA was then extracted 4 hours after the IR-exposure.
2.3 RNA-Sequencing and processing
After the RNA isolation, RNA integrity number was measured using an Agilent 2100 Bioanalyzer using an Agilent RNA 6000 pico and nano assay. RNA concentration was measured with Qubit 2 and Qubit 4 fluorometers (Invitrogen, Germany) using the RNA BR and HS assay kits. Samples with RIN values <7 were excluded from subsequent library preparation. For the lncRNA samples, ribosomal RNA (rRNA) depletion from total human RNA was carried out using the QIAseq FastSelect–rRNA HMR Kit (QIAGEN GmbH, Hilden, Germany) according to the FastSelect−rRNA protocol specific for NEBNext® libraries. For library preparations, we used the NEBNext® Ultra II Directional RNA Library Prep Kit (New England BioLabs®, Frankfurt am Main, Germany) according to the manufacturer’s recommendations. Different dual index adaptors were used for multiplexing samples in one sequencing run. Library concentrations and quality were measured using a Qubit double-strand DNA high-sensitivity kit and QIAxcel capillary electrophoresis system with QIAxcel ScreenGel software (QIAGEN GmbH, Hilden, Germany). For both, mRNA-sequencing (mRNA-Seq) and the lncRNA-sequencing (lncRNA-Seq), the libraries were processed on a HiSeq2500 instrument (Illumina, San Diego, California, USA) set to high-output mode (Nucleic Acids Core Facility, Faculty of Biology, University of Mainz). Reads were generated using TruSeq Single Read Cluster Kit v3 (Illumina, San Diego, California, USA) and TruSeq SBS Kit v3 (Illumina, San Diego, California, USA). Here, single-end reads had a length of 51 base pairs using single indices (8), and a length of 43 base pairs using dual indices (8/8), for mRNA- and lncRNA-Seq, respectively. Base calling was performed by Real-Time Analysis (Version 1.8.4) and the resulting data were converted into FASTQ format using bcl2fastq (Version 1.8.4, Illumina, San Diego, California, USA).
2.4 Processing of lncRNA- and mRNA-Seq data
For the detection of differentially expressed genes and subsequent functional analysis, the mRNA- and lncRNA-Seq data were processed first. Raw reads were separated from the adapter sequences using Trimmomatic (28). For the trimming, we used a quality threshold of three for the removal of bases. Moreover, reads with an average quality below 15 over the span of four bases were then trimmed. The resulting processed reads were aligned to the human reference genome (GRCh38) using STAR (29). The expression per gene was then computed as the number of aligned reads per gene, quantified using featureCounts (30). The data were then normalized using the voom method (31), and DESeq2 (32) for the differential expression and the weighted gene co-expression network analysis (WGCNA), respectively.
2.5 Analysis for differential gene expression
Differential gene expression of lncRNAs dependent on the radiation dose was computed with linear models implemented in the limma package (33). The individual donor was included as the block variable, additionally using donor group and radiation dose as factors of the model. The resulting log2 fold-changes (LFC) per gene and donor group after LDIR and HDIR were considered as the effect sizes on the individual transcriptome features, estimating the magnitude and direction of expression change between the respective groups (Supplementary Tables S1A–D, Supplementary Figure S1). For this purpose, we employed three different models: i.) considering the donor group (crude model), ii.) considering age and sex (model 1), and iii) considering age, sex, age at and year of diagnosis of the first neoplasm, and tumor type [(model 2), Figure 1]. Thus, we applied the specifications of model 2 to the subset of data including N1 and N2+ only. We additionally computed p-values for the interaction between the effect of the respective radiation dose and group to identify genes differentially expressed between the phenotype. Genes with a p-value adjusted at a false discovery rate [FDR, (34)] below 0.05 were reported as significant. The p-values from the separate analysis for each donor group have to be regarded as explorative since multiple pairwise comparisons were calculated. According to the best practice for reporting p-values originating from the R software, any p-values lower than 2.2x10-16 are reported as p-value<2.2x10-16 (35).
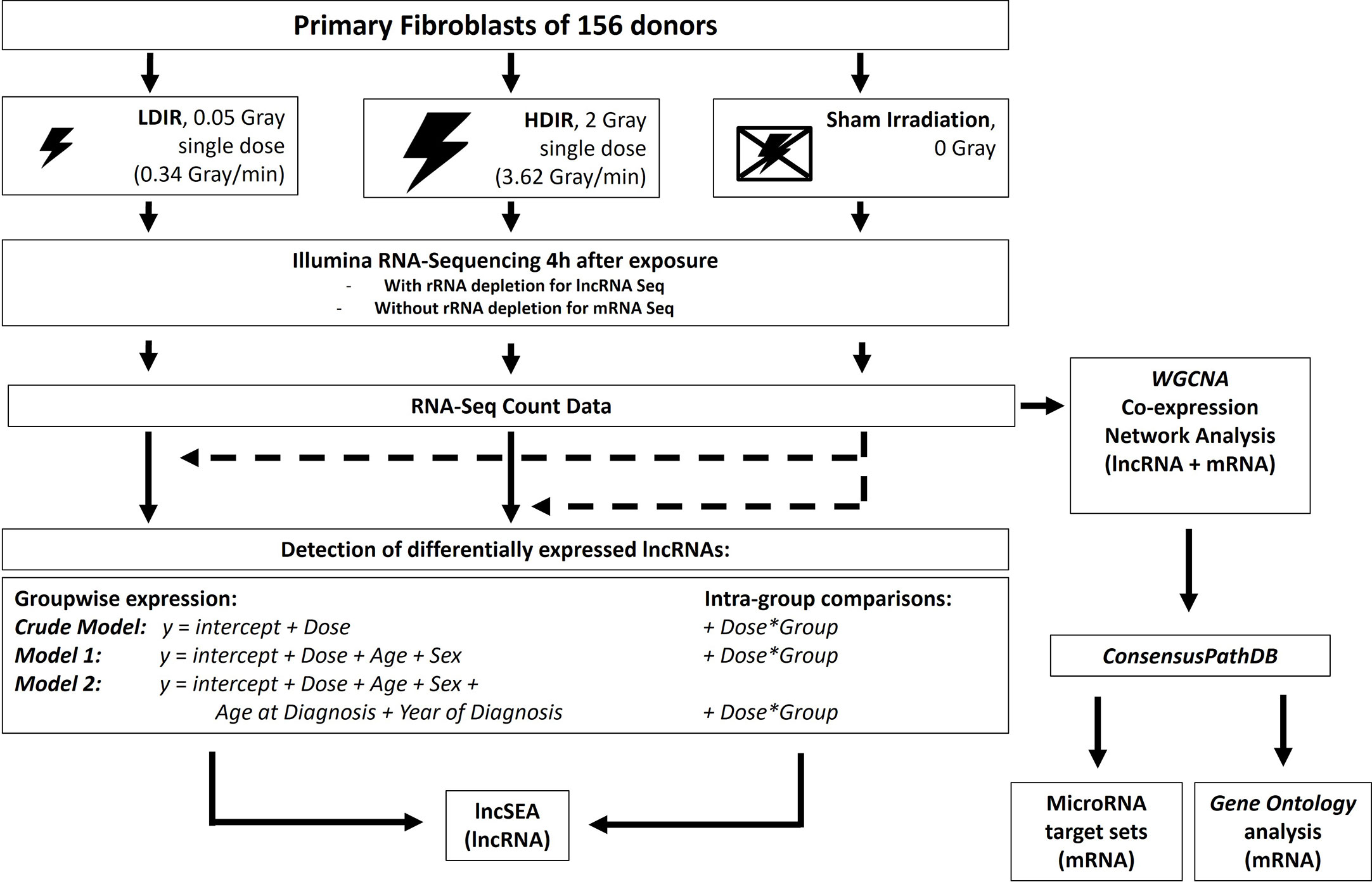
Figure 1 Experimental workflow: Primary fibroblasts of all donors were processed as matched triplets consisting of a donor with a first primary neoplasm only, a donor with a second primary neoplasm and a cancer-free control. The triplets were exposed to a low dose of ionizing radiation (LDIR, 0.05 Gray), a high dose of ionizing radiation (HDIR, 2 Gray), or were sham-irradiated (0 Gray). RNA was extracted 4h after exposure, sequenced on a HiSeq2500 Illumina device, and processed for the investigation of differentially expressed genes for each donor with regard to the regulation/level in sham-irradiated cells (dashed arrows). Differentially expressed lncRNAs as a result of groupwise-expression models, as well as data from the intra-group comparisons, were then subjected to the various analysis options of the lncSEA2.0 platform. DESeq2-normalized data from mRNA- and lncRNA-Seq were additionally filtered for protein-coding and lncRNA transcripts, respectively, and then jointly used to detect co-expressed sets of mRNAs and lncRNAs with WGCNA. The resulting gene sets correlated with the radiation doses were then subjected to the ConsensusPathDB and analyzed for miRNA targets and Gene Ontology enrichment.
2.6 Processing of RNA-seq data
2.6.1 Quality control
One sample (N2+ after HDIR) had to be removed due to a lack of appropriate quality. Thus, its samples and the samples from its matching group were not used for the identification of differentially expressed genes after HDIR. For the WGCNA, only the sample of concern was removed prior to network construction, as the donor group was later associated with a trait and not of relevance for the actual construction.
2.6.2 Filtering of sequencing data
In order to merge the results of mRNA- and lncRNA-Seq, both datasets were filtered first. The mRNA data set was filtered for protein-coding genes, and the lncRNA-Seq data for lncRNAs, using the functional Ensembl GRCh38 annotations (36) retrieved via BioMart v2.48.3 (37).
2.7 Weighted gene co-expression network
The WGCNA package allows for the examination of correlation patterns among a large set of genes. As result, it identifies clusters (called modules) of highly correlated transcripts. For the better legibility, these are then assigned colors that refer to each respective clustered group of co-expressed genes. All genes that were not clustered into a group of co-expressed transcripts were collected to the grey module. To construct robust weighted co-expression networks, we implemented the analysis with the WGCNA R package, following the instructions provided by its developers/creators. The mRNA- and lncRNA-Seq expression data were first merged and used to create a sample tree with the hclust function (method=“average”) in R (Supplemental Figure 2). Here, no further outliers were detected, and all 467 samples were used for the network construction. Next, we computed the powers (B) for a signed network to determine the power βi used to establish an approximate scale-free topology. This was necessary to determine clusters of genes with high connectivity (the sum of connection strengths) and identify the few genes that were connected to many others (hub-genes), additionally making the network more robust in the process. In short, we examined the correlation between log10 of the connectivity (k) and the log10 proportion of genes with connectivity k (p(k)), where we regarded scale-free topology as approximately satisfied if r2 ≥ 0.9. Thus, we chose β=24. The network was then constructed using the blockwisemodules() function with corType=“bicor”, minModuleSize=15, reassignThreshold=0, and mergeCutHeight=0.20. The hub-genes were then calculated for selected modules using the intramodularConnectivity() function. Details for the described analyses can be followed and reproduced by the notebooks included in the repository https://github.com/clg1990/KiKme_lncRNA.
2.7.1 Correlation of modules with traits
In order to correlate the colored modules with the available experimental metadata, each module was then assigned a single value that summarizes the expression direction, called eigengene. These eigengenes were then correlated to age, sex, donor group, and radiation dose, using the Pearson correlation coefficient. The belonging Student asymptotic p-values were computed for the correlation values and then adjusted at an FDR of 0.05.
2.8 Functional and interaction analyses of differentially expressed lncRNAs
Since lncRNAs can act as miRNA-sponges to suppress post-transcriptional regulation through miRNA-mRNA binding, we further examined prominently expressed lncRNAs for experimentally validated interactions with miRNAs stored in the DIANA lncBASEv3 (38). Next, we identified coding transcripts competing for regulatory mechanisms of miRNAs and lncRNAs by further examining information on miRNAs identified and stored in the lncBASEv3 for data on experimentally validated miRNA-mRNA-interactions in the ENCORI database (39). mRNAs associated with the previously retrieved miRNA-lncRNA data were finally integrated with the protein-coding genes present in the radiation modules from the WGCNA.
2.8.1 Over-representation and Gene Ontology analysis of co-expressed mRNA
Ensemble Gene IDs from the co-expression modules associated with the radiation dose were used as gene set input for the Gene Ontology (GO) (40, 41) overrepresentation analysis, using the ConsensusPathDB (42). According to best practices, the genes from the modules were compared to a given list of total genes detected in the experiment, called background (Supplementary Table S2A). The resulting GO terms (Supplementary Table S2B) were then summarized with an allowed semantic similarity of 0.9 with the organism set to Homo sapiens usingREVIGO (43), The results were then extracted as R script provided by the platform, translated into ggplot2 format for further modifications, and plotted as tree maps. In these, tile sizes of the tree maps were defined to represent the adjusted p-value of each respective GO term. Moreover, the genes from WGCNA modules associated with the radiation dose were examined for miRNAs (Supplementary Table S2C, miRTarBase v8.0 also provided by the ConsensusPathDB) known to regulate these transcripts, as well as joint transcription factors [Supplementary Table S2D (44)].
2.8.2 Candidate list of lncRNAs of interest
To examine differentially expressed lncRNAs that were detected in our data with the results of other researchers, we created a list containing 70 lncRNAs of interest, known to be involved in the modulation of radio sensitivity (45), damage and repair in cancer cells, as well as those proposed as biomarkers for radiation damage (46) (Supplementary Table S5, Supplementary Figure S6). We then filtered our data for the genes in the candidate list and analyzed them with regard to their status as being differentially expressed across the donor groups and models.
3 Results
3.1 LDIR
3.1.1 Differential expression of lncRNAs
After exposure to LDIR, only 1-3 lncRNAs were differentially expressed across the donor groups and models, with no lncRNA being differentially expressed in more than one donor group (Figure 2). In N0, the only differentially expressed lncRNA was AC004801.4 being detected as downregulated in all models (model 1: LFC=-0.84, p-value=0.02). In N1, LINC00691 was up- (model 1: LFC=-0.80, p-value=0.01) and Propionyl-CoA Carboxylase Subunit Alpha Divergent Transcript (PCCA-DT) downregulated (model 1: LFC=-0.33, p-value=0.01) in all models after LDIR. Additionally, AL158206.1 was upregulated in models 1 and 2 (model 1: LFC=0.36, p-value=0.04) and AF129075.3 was downregulated in model 1 (LFC=-0.94, p-value=0.04). In N2+, LINC02315 was upregulated in all models post-LDIR (model 1: LFC=0.85, p-value= 0.04). A detailed list of differentially expressed lncRNAs after LDIR is provided in Supplementary Table S1C. The additional analysis for interactions between the effect of radiation dose and the donor group did not identify lncRNAs with an adjusted p-value<0.05 in reaction to LDIR (Supplementary Table S1D). We thus applied a less conservative threshold of an unadjusted p-value<10-5. Application of this threshold resulted in the identification of three lncRNAs after LDIR. There were AC004801.4 for N2/N1 versus N0 and N1 versus N0, AC137932.3 for N2+ versus N0, and AC073591.1, for N2+ versus N1 post-LDIR (Table 1 and Supplementary Table S1E).
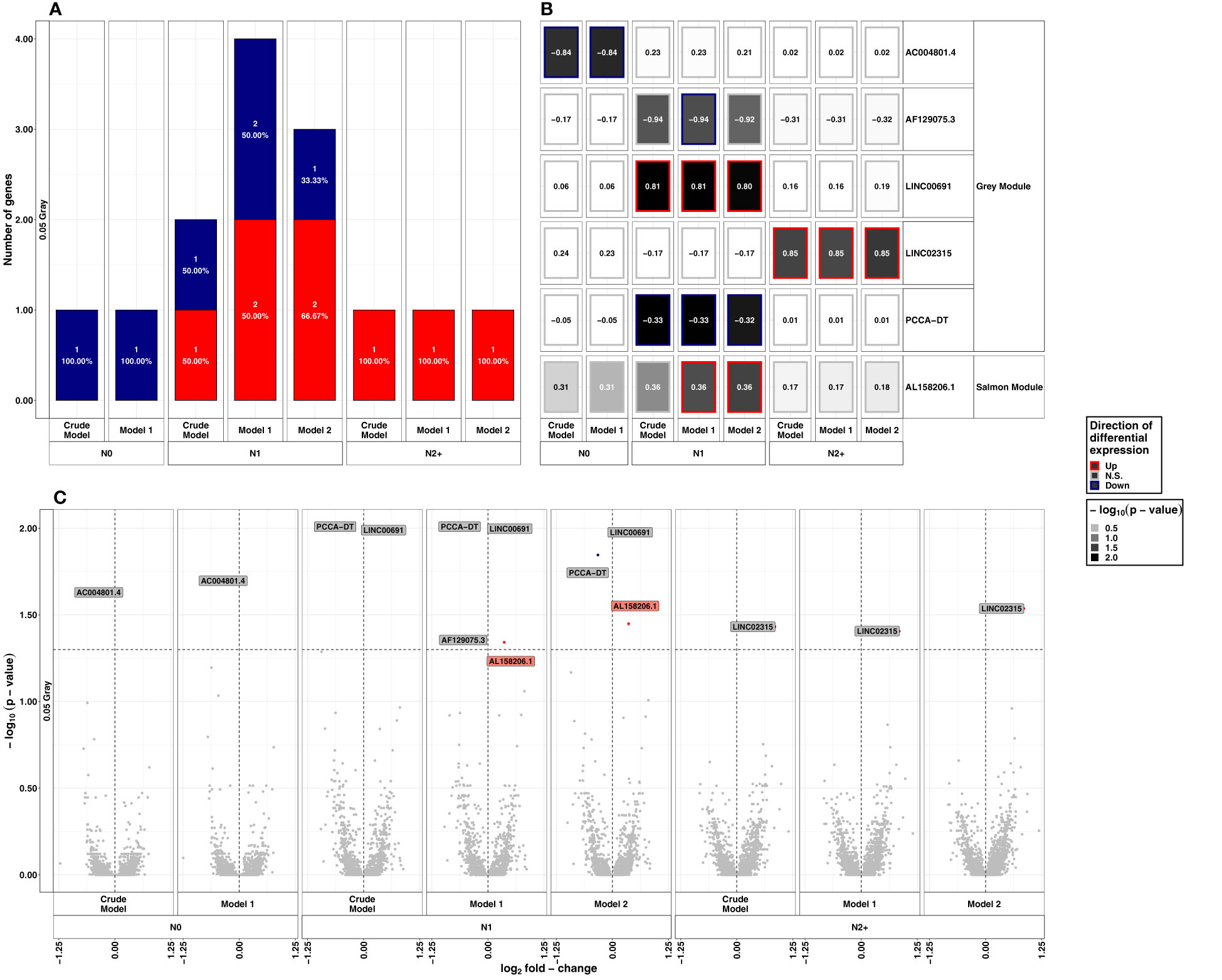
Figure 2 Summarized results on differential lncRNA expression after 0.05 Gray. Differentially expressed lncRNAs in irradiated compared to sham-irradiated fibroblasts from donors with a first primary neoplasm only (N1), donors with at least one second primary neoplasm (N2+), and cancer-free controls (N0) 4h after exposure to 0.05 Gray (false discovery rate adjusted p-value < 0.05). The data are presented for the crude model, model 1 (considering age at sampling and sex), and model 2 [considering age at sampling, sex, age at and year of diagnosis of the first neoplasm, and tumor type (not applicable for N0 data)]. In total 6225 lncRNAs were detected in the samples. Shown are (A) the proportion of up- (red) and downregulated (blue) genes stratified by radiation dose, group, and model, (B) a heat map of all differentially expressed lncRNAs, and (C) stratified by radiation dose, group, and model. The colors mentioned in the facets of (B) and used for the labels in (C) indicate the respective gene set the lncRNAs were assigned to (see Figure 4).
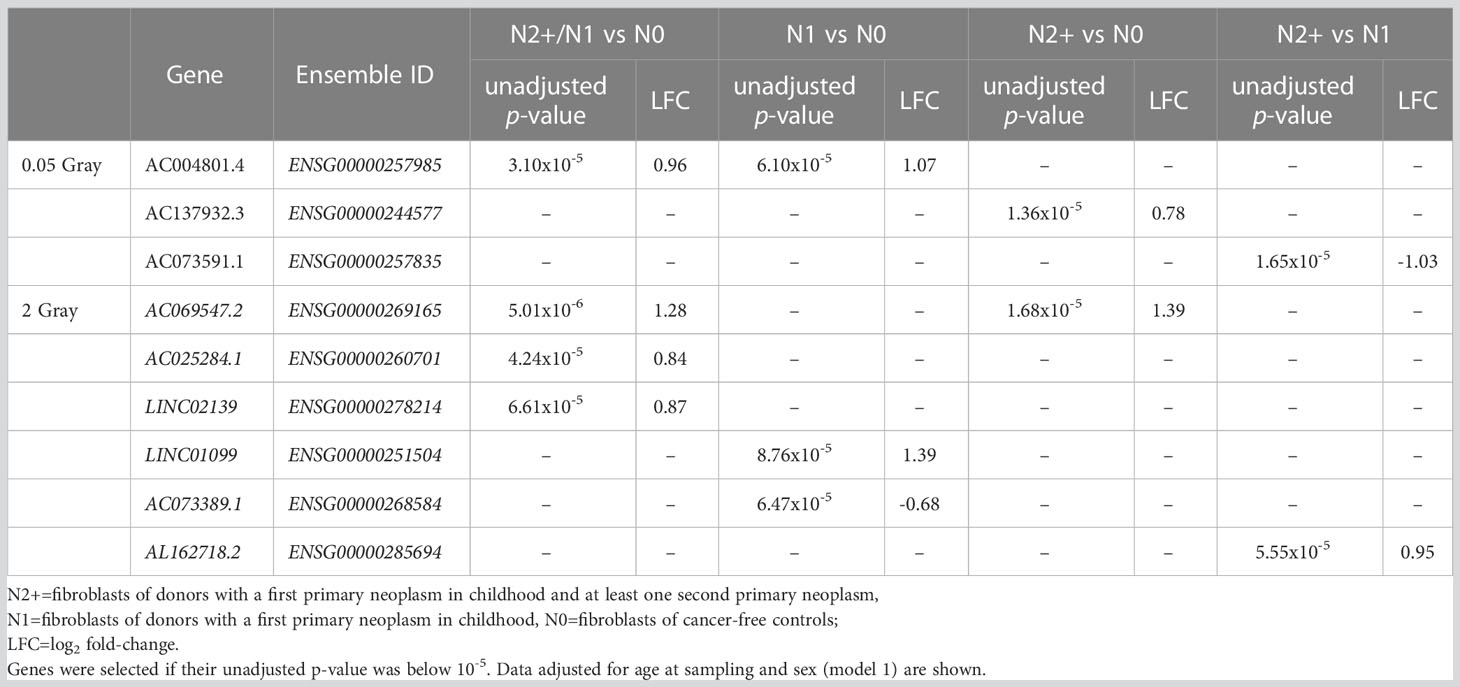
Table 1 Overview of lncRNAs found for the analysis of interactions between the effect of radiation dose and donor group.
3.1.1.1 Sensitivity analyses
After stratifying by sex, only LINC00642 was downregulated in N0 males after LDIR (LFC=-1.34, p-value=5.84x10-06). Moreover, LINC01909 (LFC=1.48, p-value=0.01), as well as AL121772.1 (LFC=0.87, p-value=0.04) were upregulated in N1 males (Supplementary Table S1E, Supplementary Figure S3A). None of these lncRNAs were differentially expressed in the main analysis and vice versa. In no female donors (N0/N1/N2+), nor male N2+ there were any differentially expressed lncRNAs after LDIR. The removal of self-reported non-Caucasian participants (n=1) did not change the results of the differential expression analysis after LDIR (data not shown).
3.2 HDIR
3.2.1 Differential expression of lncRNAs
After exposure to HDIR, the number of down- and upregulated lncRNAs was comparable in all donor groups and models with approximately 150 differentially expressed lncRNAs each (Figure 3A and Supplementary Table S1C). Among donor groups, the total number of differentially expressed lncRNAs was highest for N1 in all models (ncrude model: 167, nmodel 1: 169, nmodel 2:164). Upregulated lncRNAs after HDIR showed smaller p-values in the range of 10-10 to 10-64 compared to downregulated genes [smallest p-value>10-10, Figure 3C]. All of the highest ranking upregulated lncRNAs were found in all groups and models after HDIR (Figure 3B), while the top downregulated lncRNAs with regard to p-value differed across groups, some surpassing the threshold for significance (Figure 3B). The two downregulated lncRNAs sorted by p-value were present across all donor groups and models after HDIR. These were AC037459.2 (model 1: LFCN0: -0.16, p-valueN0: 4.41x10-04; LFCN1: -0.17, p-value N1: 2.48x10-04; LFCN2+: -0.27, p-valueN2+: 8.58x10-07) and AC125807.2 (model 1: LFCN0: - 0.31, p-valueN0: 5.29x10-07; LFCN1: - 0.32, p-valueN1: 2.28x10-07; LFCN2+: - 0.36, p-value N2+: 8.64x10-09; Figure 3C). Two lncRNAs were upregulated most strikingly with p-values below 10-45 in all donor groups and models. These were AL109976.1 (model 1: LFCN0: 1.15, p-valueN0: <2.2x10-16; LFCN1: 1.26, p-valueN1: <2.2x10-16; LFCN2+: 1.17, p-valueN2+: <2.2x10-16) and AL158206.1 (LFCN0: 1.67, p-valueN0: <2.2x10-16; LFCN1: 1.74, p-valueN1: <2.2x10-16; LFCN2+: 1.79, p-valueN2+: <2.2x10-16). As with LDIR, the analysis accounting for the interaction of donor group and HDIR resulted in no differentially expressed lncRNAs (Supplementary Table S1D). We thus again filtered for lncRNAs of interest below the threshold of the adjusted p-value but with an unadjusted p-value<10-5. Using this filter, we identified six lncRNAs after HDIR (AC069547.2, AC025284.1, LINC02139, LINC01099, AC073389.1, and AL162718.2; Table 1).
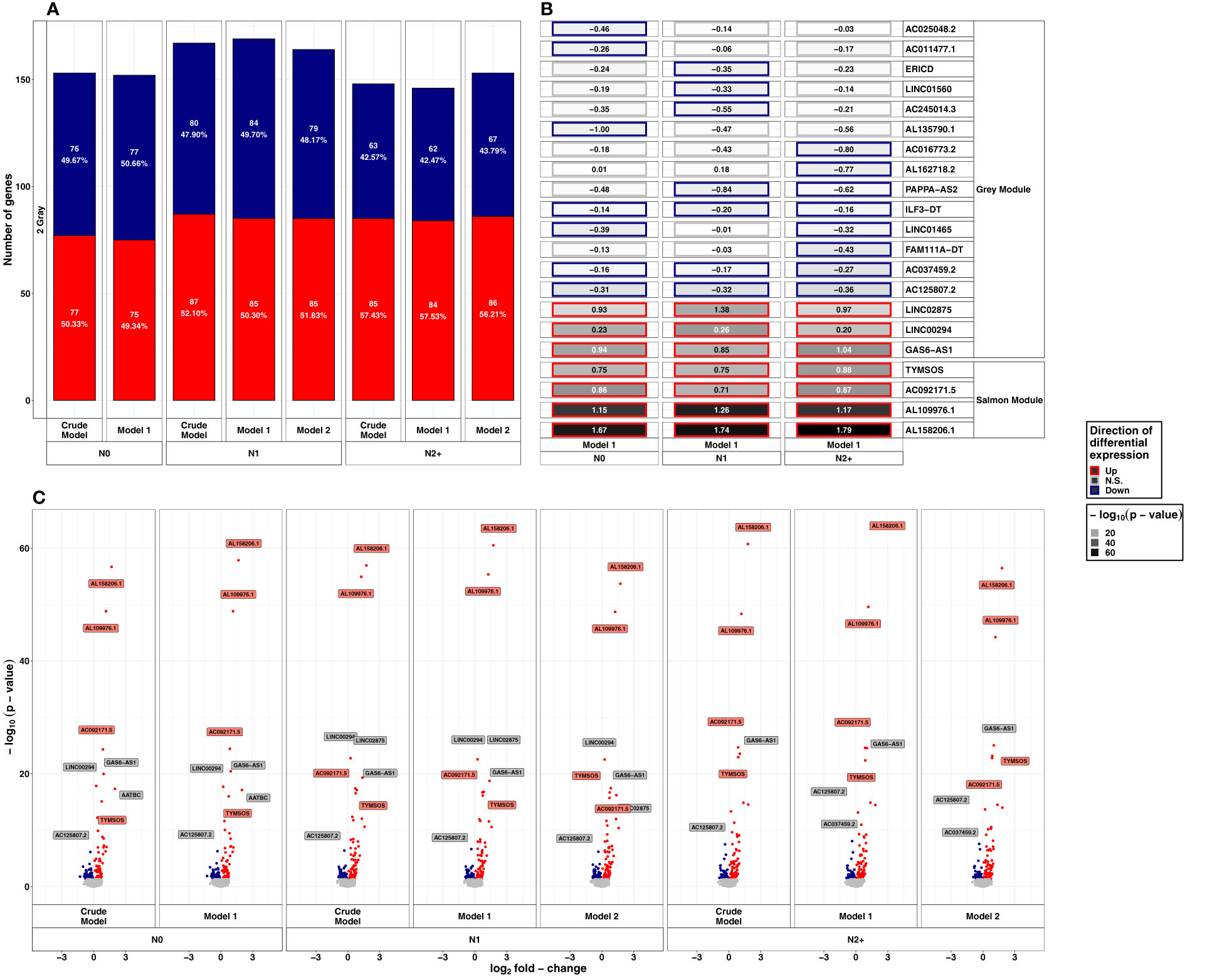
Figure 3 Summarized results on differential lncRNA expression after 2 Gray. Differentially expressed lncRNAs in irradiated compared to sham-irradiated fibroblasts from donors with a first primary neoplasm only (N1), donors with at least one second primary neoplasm (N2+), and cancer-free controls (N0) 4h after exposure to 2 Gray (false discovery rate adjusted p-value < 0.05). The data are presented for the crude model, model 1 (considering age at sampling and sex), and model 2 [considering age at sampling, sex, age at and year of diagnosis of the first neoplasm, and tumor type (not applicable for N0 data)]. In total 6225 lncRNAs were detected in the samples. Shown are (A) the proportion of up- and downregulated lncRNAs stratified by radiation dose, group, and model, (B) top 5 up- and downregulated lncRNAs with regard to the adjusted p-value of model 1, and, (C) volcano plots, stratified by radiation dose, group, and model. The colors mentioned in the facets of (B) and used for the labels in (C) indicate the respective gene set the lncRNAs were assigned to (see Figure 4).
3.2.1.1 Sensitivity analyses
After stratifying by sex, the most prominent lncRNAs after HDIR that were among the top upregulated lncRNAs with regard to the p-value in the main analyses were also present in the sensitivity analysis (AL158206.1, AL109976.1, TYMSOS, LINC00294, GAS6-AS1, AATBC, LINC02875; Supplementary Table S1D, Supplementary Figure S2B). The total number of differentially expressed lncRNA was halved compared to the number of the main analysis (N0Female: 68, N0Male: 66, N1Female: 63, N1Male: 59, N2+Female: 54, N2+Male: 78). Concerning downregulated lncRNAs, only AC125807.2 was downregulated in all male and female participants of all donor groups after HDIR. As with LDIR, the prior removal of self-reported non-Caucasian participants (n=1) did not change the results of the differential expression main analysis for exposure to HDIR (data not shown).
3.3 Weighted co-expression analysis of lncRNA and mRNA
Using WGCNA, we identified three modules that were significantly associated with HDIR (Figure 4A) and further examined the corresponding transcripts with regard to each modules’ intra-connectivity and the transcripts with the highest adjacency to the present lncRNAs. The visualization of the most important network features can be found in Supplementary Figure S3.
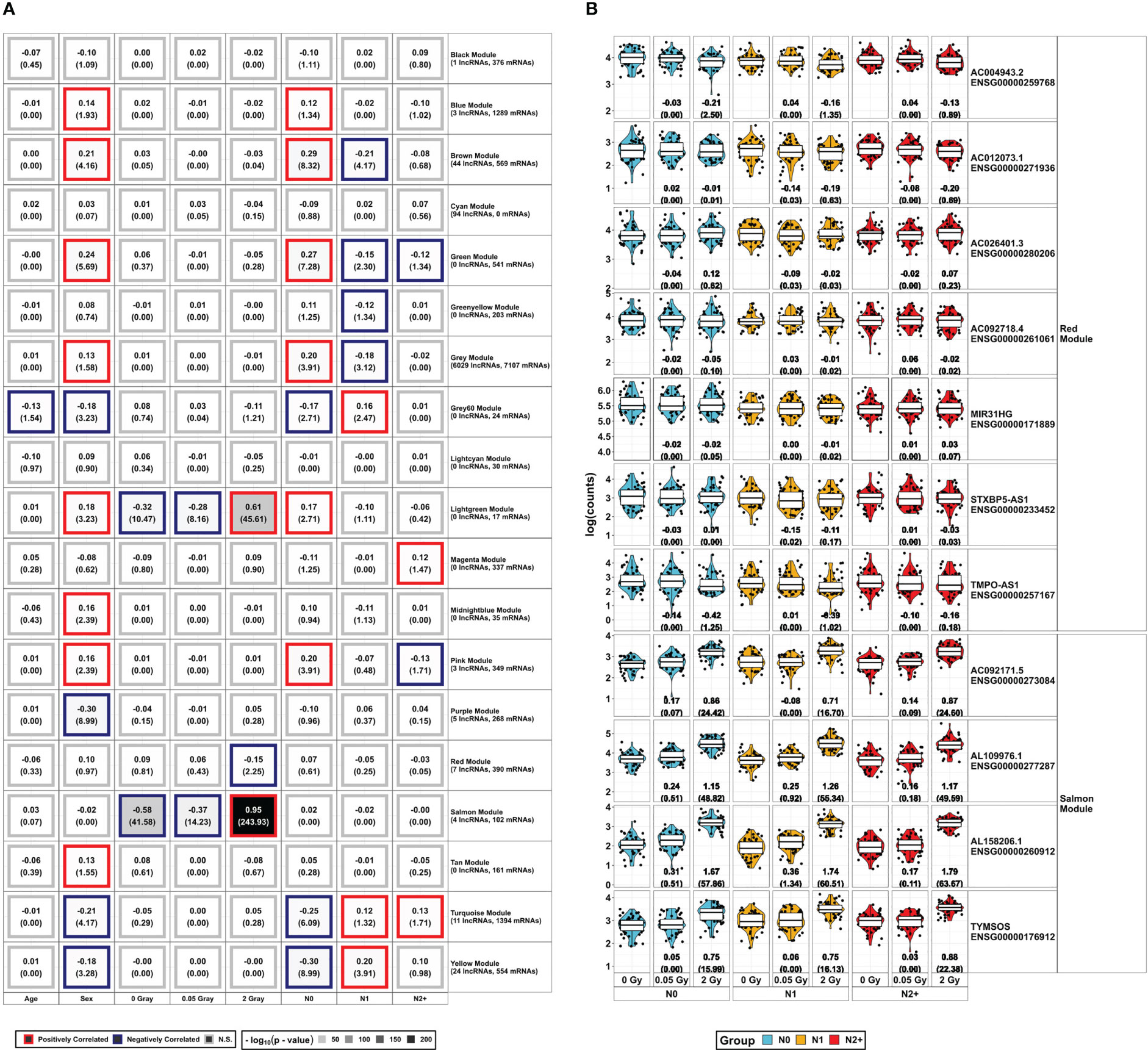
Figure 4 Overview of WGCNA Modules and lncRNAs found in modules associated with the radiation doses: (A) DESeq2-normalized protein-coding data from mRNA-Sequencing and lncRNAs from lncRNA-Sequencing were combined to construct a weighted co-expression network using WGCNA. The resulting gene sets (Modules) were assigned colors for better legibility. The respective expression of the lncRNAs in the radiation-associated modules is depicted in (B), showing log2 fold-change and the false discovery rate adjusted p-value in brackets below for all combinations of radiation dose and donor group [fibroblasts from donors with a first primary neoplasm only (N1), donors with at least one second primary neoplasm (N2+), and cancer-free controls (N0)]. These data are taken from the model 1 of the differential expression analysis, accounting for sex and age at sampling.
The salmon module was positively and strongly correlated with HDIR (r²=0.95, p-value<2.2x10-16) and negatively correlated to 0 Gray (r²=-0.58, p-value<2.2x10-16) and LDIR (r²=-0.37, p-value=10-14). Besides 102 coding genes, the salmon module encompassed four lncRNAs. These were AL109976.1, AL158206.1, TYMSOS, and AC092171.5, which were upregulated in differential expression analysis in all donor groups and models in response to HDIR (Figures 3, 4B). AL158206.1 was also upregulated by LDIR in N1 for models 1 and 2 (Figure 2). The five genes with the highest intra-modular connectivity were MDM2, SESN1, CDKN1A, PPM1D, and BTG2 (Supplementary Figure S2A). With regard to adjacency, i.) HSPA4L, BBC3, MDM2, IER5, and SESN1; ii.) CDKN1A, MDM2, TIGAR, BTG2, and HSPA4L; iii.) DDB2, BBC3, FDXR, IER5, and MRPL49; iv.) BLOC1S2, TIGAR, PPM1D, HSPA4L, and BTG2 were the top five genes to AL109976, AL158206.1, TYMSOS, and AC092171.5 respectively (Supplementary Tables S3B-E).
The red module was only correlated with HDIR (r²=-0.15, p-value=10-2). Besides 390 coding mRNAs, there were seven lncRNAs present in this module (AC004943.2, AC012073.1, AC026401.3, AC092718.4, MIR31HG, STXBP5-AS1, and TMPO-AS1). Contrary to the salmon module, AC004943.2 was the only differentially expressed lncRNA in the red module, downregulated only in N0 and N2+. The five genes with the highest intra-modular connectivity were ANLN, PRC1, KIF11, CEP55, and TPX2 (Supplementary Table S3F). With regard to adjacency, i.) KIF23, STIL, CENPO, DIAPH3, and MYBL1; ii.) TUBA1B, CCNA2, CENPO, STIL, and PRR11; iii.) PRR11, TOP2A, NCAPD2, KIF20B, and KNL1; iv.) CENPN, SHCBP1, DIAPH3, MYBL2, and CENPO; v.) ANP32E, DPYSL3, ITGB1, MYBL1, and TMPO; vi.) CKAP2, AURKA, ZWILCH, CDCA3, and PTTG1; vii.) CCNA2, CEP55, ANLN, KIF11, and KIF20B were the top five genes to AC004943.2, AC012073.1, AC026401.3, AC092718.4, MIR31HG, STXBP5-AS1, and TMPO-AS1, respectively (Supplementary Tables S3G-M).
The lightgreen module contained 17 protein-coding genes and was correlated with the radiation doses [positively correlated to HDIR (r²=0.61, p-value<2.2x10-16), negatively correlated to 0 Gray (r²=-0.28, p-value=3.39x10-11), and LDIR (r²=-0.32, - p-value=6.92x10-9)]. In addition, it was positively associated with sex (r2 = 0.18, - p-value=5.89x10-4) and N0 (r2 = 0.17, p-value=1.95x10-3). The five genes with the highest intra-modular connectivity were SUSD6, RIC1, TANC1, TCP11L1, and TNFRSF10D (Supplementary Table S3N).
3.3.1 Functional analysis of modules associated with exposure to radiation
In order to examine the co-expression modules for their functional implications, the protein-coding genes found in the salmon, red, and the lightgreen module were examined for the category biological process using the GO overrepresentation analysis in the ConsensusPathDB next (Supplementary Table S2B). Moreover, we additionally examined the mRNA of each module for the associated miRNA targets and transcription factors.
3.3.1.1 Gene Ontology overrepresentation analysis
The respective data were used in REVIGO and clustered for the best term representatives. For the salmon module positively correlated with HDIR and negatively with LDIR and sham-irradiation, these representatives were signal transduction by p53 class mediator (GO:0072331), positive regulation of cell death (GO:0010942), nucleotide-excision repair, DNA damage recognition (GO:0000715), regulation of cellular response to stress (GO:0080135), and cell death (GO:0008219) among others (Figure 5A). For the salmon module, the sole cellular component was the PCNA-p21-complex (GO:0070557, Supplementary Figure S4A), among the main representatives for the molecular functions were death receptor activity (GO:0005035), TRAIL binding (GO:0045569), damaged DNA binding (GO:0003684), and p53 binding [(GO:0002039), Supplementary Figure S4C].
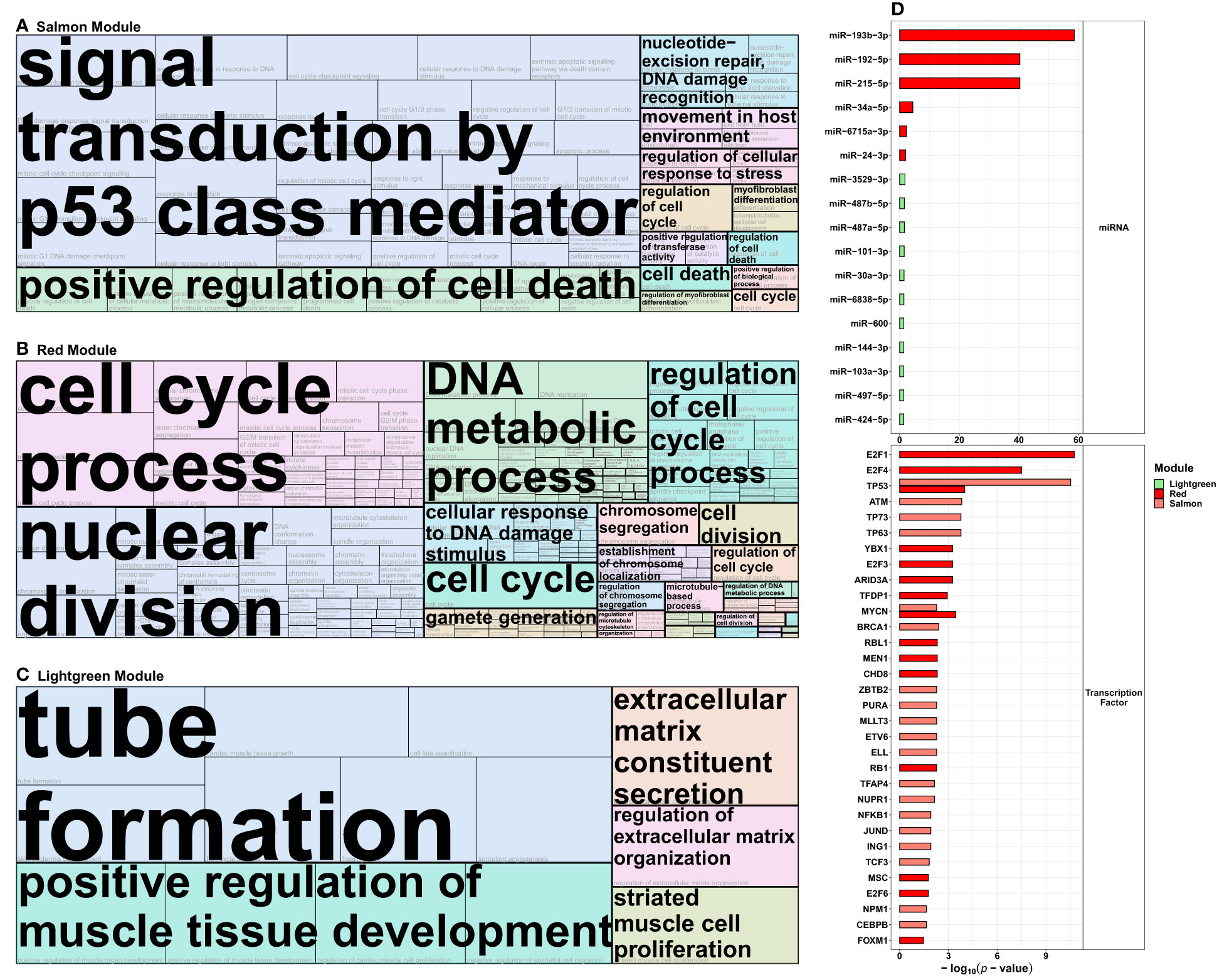
Figure 5 Functional analysis of the protein-coding transcripts from the radiation-modules identified in the co-expression network analysis. Protein-coding genes from the (A) salmon, (B) red, and (C) lightgreen (see Figure 4) WGCNA modules that were correlated with exposure to 2 Gray were used in Gene Ontology over-representation analysis via ConsensusPathDB and then summarized into tree maps via the REVIGO platform (allowed similarity 0.9, Homo sapiens, term category biological process). The term marked in large font indicates the representative term of each cluster of Gene Ontology terms, and the size of each tile represents the respective adjusted p-value. (D) depicts the miRNAs and transcription factors associated with the salmon, red, and lightgreen modules, respectively.
For the red module, only correlated with HDIR, the most prominent GO term-clusters concerning the p-value were various terms including the cell cycle and division [e.g., cell cycle process (GO:0022402), nuclear division (GO:0000280), regulation of cell cycle process (GO:0051726)], DNA metabolic process (GO:0055132), cellular response to DNA damage stimulus [(GO:0034984), Figure 5B]. For the cellular components or the molecular functions, chromosomal region (GO:0098687) and DNA replication preinitiation complex [(GO:0031261), Supplementary Figure S4B], single stranded DNA binding (GO:0003677) and catalytic activity, acting on DNA [(GO:0140097), Supplementary Figure S4D]. were among the main representatives, respectively.
For the lightgreen module, positively correlated with HDIR, sex, N0, as well as negatively correlated with LDIR and sham-irradiation, the most prominent GO term-clusters concerning p-value were tube formation (GO:0035148), positive regulation of muscle tissue development (GO:1901863), extracellular matrix constituent secretion (GO:0070278), regulation of extracellular matrix organization (GO:19030539), and striated muscle cell proliferation [(GO:0014855), Figure 5C]. No cellular components were identified for the lightgreen module, the sole representative for molecular function was transmembrane signaling receptor activity [(GO:0004888), Supplementary Figure S4E].
3.3.1.2 miRNA targets and transcription factors
We further used the ConsensusPathDB to extract information on miRNAs that are regulators of the protein-coding genes of the radiation-associated modules from the miRTarBase v8.0 (Figure 5D and Supplementary Table S2C). For the salmon module, no miRNAs were present in the overrepresentation analysis. The miRNAs concerning the adjusted p-value for the red module were miR-193b-3p (-log10(p-value)=58.60), miR-192-5p (-log10(p-value)=40.34), miR-215-5p (-log10(p-value)=40.33), miR-34a-5p (-log10(p-value)=4.44), miR-6715a-3p (-log10(p-value)=2.24), and miR-24-3p (-log10(p-value)=2.00). For the lightgreen module the top 5 miRNAs were miR-3529-3p (-log10(p-value)=1.76), miR-487b-5p (-log10(p-value)=1.57), miR-487a-5p (-log10(p-value)=1.57), miR-101-3p (-log10(p-value)=1.51), and miR-30a-3p [(-log10(p-value)=1.46).
We also computed associated transcription factors from TRRUST v2 for the radiation-responsive modules (Supplementary Table 2D). The top 5 transcription factors for the salmon module concerning adjusted p-value were TP53 (-log10(p-value)= 10.52), ATM (-log10(p-value)=3.82), TP63 (-log10(p-value)=3.77), TP73 (-log10(p-value)=3.77), and BRCA1 (-log10(p-value)=2.40); and E2F1 (-log10(p-value)=10.74), E2F4 (-log10(p-value)=7.50), TP53 (-log10(p-value)=4.00), MYCN (-log10(p-value)=3.46), and YBX1 (-log10(p-value)=3.26) for the red module. The analysis did not identify transcription factors for the lightgreen module, due to the small number (n=17) of transcripts in this module.
Our examination using the Diana lncBASEv3 returned 90 miRNAs known to interact with any of the 11 lncRNAs present in the radiation modules, as well as the 3 lncRNAs that were among the top downregulated lncRNAs in all donor groups after HDIR (ILF3-DT, AC037459.2, and AC125807.2) in healthy tissues. For these three, there were 24, 12, and 3 interacting miRNAs, respectively. With regard to the radiation modules, we identified 45, 20, 5, and one miRNA with experimentally validated interactions in normal tissue for AL158206.1, TYMSOS, AL109976.1, and AC092171.5 of the salmon module, respectively (Supplementary Table S4A). For the lncRNAs of red modules, the experimentally validated number of interacting miRNAs was: STXBP5-AS1 (n=47), TMPO-AS1 (n=14), AC092718.4 (n=12), AC004943.2 (n=10), AC012073.1 (n=8), MIR31HG (n=4), and AC026401.3 (n=1). Analysis for overlap identified hsa-miR-221-3p to interact with all lncRNAs from the salmon module (Supplementary Table S4B, Supplementary Figure S5). No miRNAs were found to interact with all lncRNAs of either the salmon or the red module.
Regarding the list with lncRNAs of interest, 39 of 70 lncRNAs were present in our data. Of these, only six were differentially expressed in any model and donor group combination after HDIR, none after LDIR, although especially PARTICLE has been proposed to be an important lncRNA in reaction to LDIR (O'Leary, Ovsepian et al., 2015). PVT1, DINOL, and NORAD were upregulated in all groups and models, PAPPA-AS1 was upregulated in all models of N1, LINC00963 was downregulated in all models of N0 and N1, and NKILA was only upregulated in the crude model of N0. All of these transcripts were assigned to the grey module (Supplementary Tables S1C, S8A, Supplementary Figure S6).
4 Discussion
In the present nested case-control study we examined the expression of lncRNA in primary skin fibroblasts of long-term survivors of childhood cancer without and with at least one subsequent second primary neoplasm, as well as of cancer-free controls after exposure to different doses of IR. We detected similar responses to a low and a high dose of ionizing radiation between all donor groups and identified the lncRNAs AL109976.1 and AL158206.1 to be strongly associated with HDIR among all donor groups for the first time.
4.1 Differentially expressed lncRNAs
The lncRNAs AC037459.2, AC125807.2, and ILF3-DT were downregulated across all models of all donor groups post-HDIR. AC037459.2 was previously associated with intracellular signal transduction and likely associated with carcinogenesis (47). Moreover, AC037459.2 might act jointly with PPP3CC, a neighboring gene, to be involved with osteosarcoma and further cancers by regulating apoptosis and the MAPK signaling pathway, with the need for further research, as the authors themselves stated (48). AC125807.2 was identified to be part of a network of competing endogenous RNA networks, also including TMPO-AS1 (red module; only correlated with HDIR), to regulate FAM82B expression in lung adenocarcinoma, leading to poor prognosis (49) and was overexpressed in cutaneous melanoma (50). Moreover, AC125807.2 was presented as part of a list of 14 immune-related lncRNAs which were associated with clinical outcomes in melanoma patients (51) and discussed as potentially involved in ferroptosis (52). Ferroptosis regulates cell death via lipid peroxidization, thus posing as a mechanism for tumor suppression. Recent data suggests that an increase in ferroptosis improved overall and progression-free survival after radiotherapy in cancer patients (53). In our radiation-responsive modules, however, no GO term comprising ferroptosis was found. ILF-DT is associated with autophagy and of prognostic value for cervical cancer together with 9 other lncRNAs, among those being AL109976.1 of the salmon module [positively correlated only with HDIR, as well as negatively with LDIR and sham-irradiation (54)].
Comparing our data with Ding and colleagues (55), none of the lncRNAs in their study (ITPK1-AS1, LINC00467, MIR22HG, DTX2P1-UPK3BP1-PMS2P11, OR2A1-AS1, LINC00173, TPTEP1, TINCR, LINC00336, C10orf111, TRHDE-AS1, MIR7-3HG, LINC00852, COLCA1, C20orf197, TTTY14, and MEG3) were differentially expressed after LDIR in our results. Only MIR22HG was downregulated in all donor groups after HDIR in our analysis. Additionally, RPP38-DT (In 2015 termed C10orf111) was upregulated only in N0 after HDIR in our results, in contrast to a downregulation reported by Ding et al. (55). This might be explained by the fact that we adjusted for multiple testing, while Ding and colleagues did not. They also examined human fibroblasts, but these were HSF42 cell lines and radiation doses were defined differently (LDIR as 2 cGy and HDIR as 4 Gy). Moreover, only six of the lncRNAs in our candidate list of 70 lncRNAs that were reported to be associated with the radiation response were differentially expressed in our analysis, underlining the strong variability and tissue specificity of lncRNAs (56, 57).
4.2 Co-expression analysis
None of the modules that contained lncRNAs were simultaneously correlated to any radiation dose and any donor group, which was in line with our results on differentially expressed lncRNA including interaction terms where we did not identify prominent differences between the three donor groups. However, we identified lncRNAs with a strong signal in the co-expression analysis in response to HDIR, which were additionally identified as upregulated in the differential expression analysis.
4.2.1 The salmon module
This module was positively correlated with HDIR but negatively correlated with LDIR and sham-irradiation. Its most prominent function was a plethora of GO-terms associated with the p53-mediated radiation response.
4.2.1.1 AL109976.1
AL109976.1 was previously reported to be associated with immune functions and used in the prediction of cervical cancer survival (58), among autophagy-related lncRNAs with prognostic value (54), as well as the top 20 downregulated lncRNAs in squamous cell tongue carcinoma (59).
4.2.1.2 AL158206.1
Upregulation of AL158206.1 was shown to act as a tumor suppressor by inhibiting proliferation and invasion in gastric cancer cells (60). Our finding of AL158206.1 being upregulated and strongly associated with the p53 pathway is supported by work on HepG2 cells exposed to cisplatin, a commonly used DNA damaging chemotherapeutic drug. Wang and colleagues associated this lncRNA with a comparable number of co-expressed transcripts (n=57) and identified p53 signaling as strongly affected pathways. They showed that CDKN1A, TP53I3, and PPM1D were upregulated by AL158206.1. Contrasting these findings, another work examining lncRNAs involved in the epithelial-mesenchymal-transition, associated with metastasis, identified AL158206.1 as one of four lncRNAs having the strongest negative impact on the survival of patients undergoing drug treatment of hepatocellular carcinoma (61). Interestingly, we observed upregulation of AL158206.1 and thus induction of protective radiation response after LDIR only in N1. This observation may suggest that in these donors, compared to N0 and N2+, there is an increased protective function in N1 in the case of low-dose genotoxic exposures of normal tissue, which occur, e.g., in the course of radiotherapy. This crucial difference in a lower-threshold cellular radiation response could therefore also reduce the risk of developing therapy-associated secondary malignancies in the N1 donors compared to N2+ donors. Regarding clinical relevance, AL158206.1 may pose as a potent biomarker for the identification of at-risk patients for long-term adverse reactions in healthy tissues.
4.2.1.3 TYMSOS
In gastric cancer, TYMSOS drives proliferation and migration through the sponging of miR-4739 (62) and in non-small cell lung carcinoma through the FOXM1/TYMSOS/miR-214-3p-axis (63). In our data neither miRNA was present the common regulators of the protein-coding transcripts of the radiation-responsive modules, very well keeping in mind that we examined healthy tissues, these patterns might be cancer-specific. Additionally, TYMSOS was used in a prognostic model as one of thirteen lncRNAs related to M6A regulators, a certain type of mRNA modification, that is also of importance in cancer (64), as one of 18 immune-related lncRNAs for clinical prediction in head and neck squamous cell carcinoma due to its potential to modulate tumor microenvironment (65).
4.2.1.4 AC125807.2
Similar to the potential involvement of AC125807.2 in ferroptosis, AC092171.5 was part of a ferroptosis signature, containing 10 lncRNAs that also showed involvement in immune pathways that were additionally associated with cancer, further implicating the success of immune- and chemotherapy in lung adenocarcinoma patients and part of another immune-related signature, comprising of nine lncRNAs, employed to predict overall survival in pancreatic cancer (66).
The role of these transcripts for the immune system and various cancers is in line with the functional involvement of the co-expressed protein-coding transcripts in this work. In particular, involvement in the p53 pathway and its associated functions such as cell death, response to stress, and recognition, as well as repair, of DNA damage are key drivers of carcinogenesis when not functioning properly (67), or too well in the case of cancerous tissue evading cell death through overly strong stress response pathways (68). However, current literature does not provide information on the expression of these transcripts in the radiation response of healthy tissues, which does not allow a more in-depth comparison to other works, but underlines the importance of our observations.
4.2.2 The red module
The red module was correlated with HDIR and associated with various processes of the cell cycle, as well as response to DNA damage. Interestingly, the analysis for differential expression did not determine six of the seven lncRNAs in this module to be differentially expressed post-LDIR or -HDIR. However, this module showed the weakest correlation of the three radiation-responsive modules, potentially implicating a signal very close to the detection limits of this analysis. Only AC004943.2 was downregulated in N0 and N1 and TMPO-AS1 was borderline significantly downregulated in N0 after HDIR.
4.2.2.1 AC004943.2
AC004943.2 was identified as one of three lncRNAs to be associated with BRCA1/2 that enabled prognosis prediction as well as prediction of response to chemotherapy in patients with ovarian cancer (69). Interestingly, AC004943.2 has been reported as part of a lncRNA set that is related to cuproptosis in head and neck squamous cell cancer (70). Such as the aforementioned ferroptosis, cuproptosis, is a novel pathway for programmed cell death, whereas this pathway involves excess intracellular copper, instead of iron (71).
4.2.2.2 TMPO-AS1
Due to its dysregulation in several malignancies, TMPO-AS1 has been a prominent transcript over the past years (72). TMPO-AS1 functions by binding FUS and recruiting p300 to the promoter of TMPO, thus activating its transcription (73) and being positively correlated (74). TMPO has been shown to induce proliferation and inducing cell cycle arrest and apoptosis in glioblastoma (75). Thus, unsurprisingly, the knockdown of TMPO-AS1 suppressed growth and increased apoptosis in thyroid cancer (76), glioma progression (77), and cell proliferation and motility in pancreatic carcinoma (72). Moreover, reduced expression of TMPO-AS1 increased overall survival and impaired growth of esophageal squamous cell carcinoma tumors (73). The expression of TMPO-AS1 itself may be activated by E2F1 (78) as being part of a positive regulatory loop, which was identified in the promotion of bladder cancer (79). Nevertheless, in our data N0 showed the lowest downregulation of E2F1 post-HDIR (LFCN0=-0.27, LFCN1=-0.39, LFCN2+=-0.31), while exhibiting the largest reduction in TMPO-AS1 expression and the only to be borderline significant (see Figure 4). Potentially, there are further mediators at play, that are not reflected in these data.
4.2.3 The lightgreen module
This module was the only identified module that was correlated with the radiation doses and with N0 as one of the donor groups. Nevertheless, no lncRNAs but 17 mRNAs were involved in this module. These were however not present among the top genes identified in a prior work that examined the differential mRNA expression including an interaction term for the donor group [see Table 2, (26)].
4.3 Interaction of lncRNAs with miRNAs and transcription factors
We have previously reported data from this donor collective showing that the transcription factors E2F1 and TP53 were involved in response to radiation, with E2F1 being enriched for N1 and N2+ post-HDIR, and TP53 being enriched post-LDIR and post-HDIR in all donor groups (26). Moreover, all of the further top transcription factors, such as E2F4 (80), ATM (81), TP63 and TP73 (82), MYCN (83), YBX1 (84), E2F3 (85), and BRCA1 (86) have been reported to be of relevance for many aspects of the radiation response. The associated lncRNAs, such as Linc01013, reported to affect DNA damage repair via YBX1 in endothelial cells (87) were not differentially expressed in our data. Noteworthy, hsa-miR-221-3p, the miRNA that was found to be interacting with all lncRNAs observed in the salmon module is also the only miRNA that is curated in the GO term DNA repair (GO:0006281) and prominently involved in cancer pathways (88, 89). This transcript was, however, not found in the analysis for involved miRNAs that regulate the protein-coding transcripts of the radiation responsive modules in our results.
4.4 Strengths and limitations
This is the first work to use primary skin fibroblasts from a large sample of a unique cohort of long-term survivors of childhood cancer with and without second primary neoplasms. Nevertheless, these results need to be interpreted bearing in mind some restrictions. lncRNAs are underlying a highly time- and tissue-specific expression and are present in far lower amounts compared to mRNA (90). Moreover, the largely unexplored intra-cellular cross-talk of long and further non-coding transcripts, as well as inter-cellular cross-talk of the DNA damage response after IR, known as the bystander effect (91), was not considered in this project. However, the high number of participants enabled us to facilitate differential expression analysis, with the appropriate statistical power, which, especially with lncRNA is heavily reliant on this fact, providing first insight on the topic and a potent basis to draw further projects from. Especially the interactions with miRNA are promising to elaborate on for further works (90), since it has been established that lncRNA and miRNA expression are important modulators for the radio sensitization of cancer cells (92), the findings of this work may facilitate new projects. The reported lncRNAs and their newly identified role may make them future targets in cancer therapy against radiosensitivity (92, 93). As resistance to the effect of applied ionizing radiation largely explains failure of cancer treatment (e.g., recurrence, metastasis, and survival), the molecular understanding and subsequent clinical modulation of such resistance to radiotherapy is at the core of facilitating future improvements in cancer treatment (94). Importantly, future projects of us and other are advised to complement their work by adding classical biological labwork (e.g., PCR validation of select candidates and design of further knockout experiments) to additionally support the findings, as well as to expand the analyses on tumor samples instead of healthy tissues to further examine implications on further aspects such as survival and metastasis, as others have done in similar work (95, 96).
5 Conclusion
We are the first to identify a set of lncRNAs, AL158206.1 and AL109976.1 most prominently, to be functionally involved in the radiation response through differential expression analysis of large-scale standardized radiation experiments and additional co-expression network analysis with protein-coding transcripts. These transcripts may pose as potent molecular targets, on the one hand for radio sensitization of tumors and on the other hand as predictive biomarkers of the individual response and risk of adverse effects of normal tissue to medical radiation exposures. Our work illustrates the relevance of investigating a broader spectrum and dimensions of transcriptomic cross-talk by miRNAs and non-coding transcripts in the context of IR exposures. Based on our findings, future investigation of the involvement of noncoding molecular units in the cellular radiation response and its associated health consequences is highly warranted to increase the efficiency of radiotherapy in tumor treatment as well as radiation protection.
Data availability statement
The individual level RNA-seq data presented in this article are not publicly available, as they contain sensitive patient data underlying data protection rules. As stated in the patient information and signed informed consent documents for the ISIBELa project, such data may only be analyzed by the study team. Due to the regulations to protect the participants’ data and to ensure that they remain pseudonymized, the datasets generated and analyzed in this study are only available upon reasonable request. Any request to access the datasets should be directed to dmwtaXNpYmVsQHVuaS1tYWluei5kZQ==.
Ethics statement
We certify that all applicable institutional and governmental regulations concerning the ethical use of human volunteers were followed during this research. Approval by the Ethics Committee of the Medical Association of Rhineland-Palatinate was obtained (no. 837.262.12 (8363-F) and no. 837.440.03 (4102); Supplementary Material (Documents 1-4). Study participants will not undergo any procedures unless they give consent for examinations, collection of samples, subsequent analysis, and storage of personal data and collected samples. Study subjects can consent to single components of the study while abstaining from others. The patients/participants provided their written informed consent to participate in this study.
Author contributions
MM developed the KiKme study and its design as the principal investigator. MM conceptualized the research idea on gene expression at different time points after exposure to high and low doses of ionizing radiation, designed the experiments, and developed the hypothesis on the role of lncRNAs in radiation response. LB and MM implemented the KiKme study. LB, CS, and MM conducted the participant recruitment, which was organized and planned by LB and MM. Former childhood cancer patients were identified, matched, and contacted by the German Childhood Cancer Registry. Doctors responsible for sample drawing were trained and supervised by MM and HS. The method of fibroblast sampling was established by DG and HS. HSZ takes care of the project ´s biobank and controls for the quality of all biosamples. Cell culture and radiation exposure of primary fibroblasts were established by SZ and DG. LB and SZ were responsible for the pseudonymization of all biosamples. The analysis pipeline for the project was developed by MM and AP. Analysis data of biosamples was processed by AP and TH. CG and AP conducted the statistical analysis. The final analysis of data concerning content was done by CG. All figures were designed and programmed by CG. CG, FM, and MM prepared the initial manuscript. All authors revised the manuscript critically for important intellectual content, approved the final version, and agreed to be accountable for all aspects of the work in ensuring that questions related to the accuracy or integrity of any part of the work are appropriately investigated and resolved. All authors contributed to the article and approved the submitted version.
Funding
The study was funded by the Federal Ministry of Education and Research in Germany under contracts No. 02NUK016A, 2NUK042A, 2NUK042B, 2NUK042C, and 2NUK042D and conducted among other research projects as part of the research consortia ISIMEP (Intrinsic radiation sensitivity: Identification, mechanisms and epidemiology, principal investigator: MB) and ISIBELa (Intrinsic radiation sensitivity: Identification of biological and epidemiological long-term effects, principal investigators: MB and HS).
Acknowledgments
The authors gratefully acknowledge the assistance from Peter Kaatsch, Franziska Himmelsbach, Cornelia Becker, Ilona Kerenyi, and Marianne Brömmel from the German Childhood Cancer Registry. For his work as the coordinator of the ISIBELa consortium, we thank Peter Scholz-Kreisel. We further thank Heiko Karle for his help in establishing the method for fibroblast irradiation. We are very thankful for the local support of all participating dermatologists in Germany, Austria, and Switzerland, and Ursula Disque-Kaiser for the excellent technical laboratory assistance as well as Willempje Hummel-Bartenschlager. The authors acknowledge resources and support from the Bioinformatics Core Facility at the University Medical Center Mainz. TH acknowledges the expert technical work of the staff of the Nucleic Acids Core Facility, Faculty of Biology, JGU Mainz. The study would not have been possible without the voluntary collaboration of all participants who participated in the extensive examinations. The authors acknowledge the help of Harald Binder and Moritz Hess in the establishment of the analysis pipeline.
Conflict of interest
The authors declare that the research was conducted in the absence of any commercial or financial relationships that could be construed as a potential conflict of interest.
Publisher’s note
All claims expressed in this article are solely those of the authors and do not necessarily represent those of their affiliated organizations, or those of the publisher, the editors and the reviewers. Any product that may be evaluated in this article, or claim that may be made by its manufacturer, is not guaranteed or endorsed by the publisher.
Supplementary material
The Supplementary Material for this article can be found online at: https://www.frontiersin.org/articles/10.3389/fonc.2023.1158176/full#supplementary-material
References
1. Dunham I, Kundaje A, Aldred SF, Collins PJ, Davis CA, Doyle F, et al. An integrated encyclopedia of DNA elements in the human genome. Nature (2012) 489(7414):57–74. doi: 10.1038/nature11247
2. DiStefano JK. The emerging role of long noncoding RNAs in human disease. Methods Mol Biol (2018) 1706:91–110. doi: 10.1007/978-1-4939-7471-9_6
3. ENCODE-Project Consortium, Birney E, Stamatoyannopoulos JA, Dutta A, Guigó R, Gingeras TR, et al. Identification and analysis of functional elements in 1% of the human genome by the ENCODE pilot project. Nature (2007) 447(7146):799–816. doi: 10.1038/nature05874
4. Sánchez Y, Huarte M. Long non-coding RNAs: challenges for diagnosis and therapies. Nucleic Acid Ther (2013) 23(1):15–20. doi: 10.1089/nat.2012.0414
5. Isin M, Dalay N. LncRNAs and neoplasia. Clin Chim Acta (2015) 444:280–8. doi: 10.3892/ol.2018.8513
6. Ketley RF, Gullerova M. Jack of all trades? the versatility of RNA in DNA double-strand break repair. Essays Biochem (2020) 64(5):721–35. doi: 10.1042/EBC20200008
7. Gao J, Zong X, Chen N, Lan T, Yu W, Long H, et al. Research progress on three different types of noncoding RNAs related to ionizing radiation. Radiat Med Prot (2021) 2(2):83–7. doi: 10.1016/j.radmp.2021.04.001
8. O'Leary VB, Ovsepian SV, Carrascosa LG, Buske FA, Radulovic V, Niyazi M, et al. PARTICLE, a triplex-forming long ncRNA, regulates locus-specific methylation in response to low-dose irradiation. Cell Rep (2015) 11(3):474–85. doi: 10.1016/j.celrep.2015.03.043
9. Statello L, Guo C-J, Chen L-L, Huarte M. Gene regulation by long non-coding RNAs and its biological functions. Nat Rev Mol Cell Biol (2021) 22(2):96–118. doi: 10.1038/s41580-020-00315-9
10. Barazzuol L, Coppes RP, van Luijk P. Prevention and treatment of radiotherapy-induced side effects. Mol Oncol (2020) 14(7):1538–54. doi: 10.1002/1878-0261.12750
11. Demoor-Goldschmidt C, de Vathaire F. Review of risk factors of secondary cancers among cancer survivors. Br J Radiol (2019) 92(1093):20180390. doi: 10.1259/bjr.20180390
12. Zahnreich S, Schmidberger H. Childhood cancer: Occurrence, treatment and risk of second primary malignancies. Cancers (Basel) (2021) 13(11). doi: 10.3390/cancers13112607
13. Scholz-Kreisel P, Kaatsch P, Spix C, Schmidberger H, Marron M, Grabow D, et al. Second malignancies following childhood cancer treatment in Germany from 1980 to 2014. Dtsch Arztebl Int (2018) 115(23):385–92. doi: 10.3238/arztebl.2018.0385
14. Dörr W, Herrmann T. Second primary tumors after radiotherapy for malignancies. treatment-related parameters. Strahlenther Onkol (2002) 178(7):357–62. doi: 10.1007/s00066-002-0951-6
15. Diallo I, Haddy N, Adjadj E, Samand A, Quiniou E, Chavaudra J, et al. Frequency distribution of second solid cancer locations in relation to the irradiated volume among 115 patients treated for childhood cancer. Int J Radiat Oncol Biol Phys (2009) 74(3):876–83. doi: 10.1016/j.ijrobp.2009.01.040
16. Brenner DJ, Hall EJ. Cancer risks from CT scans: now we have data, what next? Radiology (2012) 265(2):330–1. doi: 10.1148/radiol.12121248
17. Purdy JA. Dose to normal tissues outside the radiation therapy patient's treated volume: a review of different radiation therapy techniques. Health Phys (2008) 95(5):666–76. doi: 10.1097/01.HP.0000326342.47348.06
18. Hricak H, Brenner DJ, Adelstein SJ, Frush DP, Hall EJ, Howell RW, et al. Managing radiation use in medical imaging: a multifaceted challenge. Radiology (2011) 258(3):889–905. doi: 10.1148/radiol.10101157
19. Beer L, Nemec L, Wagner T, Ristl R, Altenburger LM, Ankersmit HJ, et al. Ionizing radiation regulates long non-coding RNAs in human peripheral blood mononuclear cells. J Radiat Res (2017) 58(2):201–9. doi: 10.1093/jrr/rrw111
20. Terradas M, Martin M, Repulles J, Huarte M, Genesca A. Distinct sets of lncRNAs are differentially modulated after exposure to high and low doses of X rays. Radiat Res (2016) 186(6):549–58. doi: 10.1667/RR14377.1
21. Borghini A, Andreassi MG. Non-coding RNAs in cellular response to ionizing radiation. Non-coding RNA Invest (2018) 2. doi: 10.21037/ncri.2018.06.10
22. Yang M, Sun Y, Xiao C, Ji K, Zhang M, He N, et al. Integrated analysis of the altered lncRNAs and mRNAs expression in 293T cells after ionizing radiation exposure. Int J Mol Sci (2019) 20(12):2968. doi: 10.3390/ijms20122968
23. Chopra S, Shankavaram U, Bylicky M, Dalo J, Scott K, Aryankalayil MJ, et al. Profiling mRNA, miRNA and lncRNA expression changes in endothelial cells in response to increasing doses of ionizing radiation. Sci Rep (2022) 12(1):19941. doi: 10.1038/s41598-022-24051-6
24. Marron M, Brackmann LK, Schwarz H, Hummel-Bartenschlager W, Zahnreich S, Galetzka D, et al. Identification of genetic predispositions related to ionizing radiation in primary human skin fibroblasts from survivors of childhood and second primary cancer as well as cancer-free controls: Protocol for the nested case-control study KiKme. JMIR Res Protoc (2021) 10(11):e32395. doi: 10.2196/32395
25. Brackmann LK, Poplawski A, Grandt CL, Schwarz H, Hankeln T, Rapp S, et al. Comparison of time and dose dependent gene expression and affected pathways in primary human fibroblasts after exposure to ionizing radiation. Mol Med (2020) 26(1):85. doi: 10.1186/s10020-020-00203-0
26. Grandt CL, Brackmann LK, Poplawski A, Schwarz H, Hummel-Bartenschlager W, Hankeln T, et al. Radiation-response in primary fibroblasts of long-term survivors of childhood cancer with and without second primary neoplasms: the KiKme study. Mol Med (2022) 28(1):105. doi: 10.1186/s10020-022-00520-6
27. Dracham CB, Shankar A, Madan R. Radiation induced secondary malignancies: a review article. Radiat Oncol J (2018) 36(2):85–94. doi: 10.3857/roj.2018.00290
28. Bolger AM, Lohse M, Usadel B. Trimmomatic: a flexible trimmer for illumina sequence data. Bioinformatics (2014) 30(15):2114–20. doi: 10.1093/bioinformatics/btu170
29. Dobin A, Davis CA, Schlesinger F, Drenkow J, Zaleski C, Jha S, et al. STAR: ultrafast universal RNA-seq aligner. Bioinformatics (2013) 29(1):15–21. doi: 10.1093/bioinformatics/bts635
30. Liao Y, Smyth GK, Shi W. featureCounts: an efficient general purpose program for assigning sequence reads to genomic features. Bioinformatics (2014) 30(7):923–30. doi: 10.1093/bioinformatics/btt656
31. Law CW, Chen Y, Shi W, Smyth GK. Voom: Precision weights unlock linear model analysis tools for RNA-seq read counts. Genome Biol (2014) 15(2):R29. doi: 10.1186/gb-2014-15-2-r29
32. Love MI, Huber W, Anders S. Moderated estimation of fold change and dispersion for RNA-seq data with DESeq2. Genome Biol (2014) 15(12):550. doi: 10.1186/s13059-014-0550-8
33. Ritchie ME, Phipson B, Wu D, Hu Y, Law CW, Shi W, et al. Limma powers differential expression analyses for RNA-sequencing and microarray studies. Nucleic Acids Res (2015) 43(7):e47. doi: 10.1093/nar/gkv007
34. Benjamini Y, Hochberg Y. Controlling the false discovery rate: A practical and powerful approach to multiple testing. J R Stat Society: Ser B (Methodological) (1995) 57(1):289–300. doi: 10.1111/j.2517-6161.1995.tb02031.x
36. Cunningham F, Allen JE, Allen J, Alvarez-Jarreta J, Amode MR, Armean IM, et al. Ensembl 2022. Nucleic Acids Res (2021) 50(D1):D988–95. doi: 10.1093/nar/gkab1049
37. Smedley D, Haider S, Ballester B, Holland R, London D, Thorisson G, et al. BioMart – biological queries made easy. BMC Genomics (2009) 10(1):22. doi: 10.1186/1471-2164-10-22
38. Karagkouni D, Paraskevopoulou MD, Tastsoglou S, Skoufos G, Karavangeli A, Pierros V, et al. DIANA-LncBase v3: indexing experimentally supported miRNA targets on non-coding transcripts. Nucleic Acids Res (2019) 48(D1):D101–10. doi: 10.1093/nar/gkz1036
39. Li JH, Liu S, Zhou H, Qu LH, Yang JH. starBase v2.0: decoding miRNA-ceRNA, miRNA-ncRNA and protein-RNA interaction networks from large-scale CLIP-seq data. Nucleic Acids Res (2014) 42(Database issue):D92–97.
40. The Gene Ontology Consortium. The gene ontology resource: 20 years and still GOing strong. Nucleic Acids Res (2018) 47(D1):D330–8.
41. Ashburner M, Ball CA, Blake JA, Botstein D, Butler H, Cherry JM, et al. Gene ontology: tool for the unification of biology. the gene ontology consortium. Nat Genet (2000) 25(1):25–9. doi: 10.1038/75556
42. Kamburov A, Stelzl U, Lehrach H, Herwig R. The ConsensusPathDB interaction database: 2013 update. Nucleic Acids Res (2012) 41(D1):D793–800. doi: 10.1093/nar/gks1055
43. Supek F, Bošnjak M, Škunca N, Šmuc T. REVIGO summarizes and visualizes long lists of gene ontology terms. PloS One (2011) 6(7):e21800. doi: 10.1371/journal.pone.0021800
44. Han H, Cho JW, Lee S, Yun A, Kim H, Bae D, et al. TRRUST v2: an expanded reference database of human and mouse transcriptional regulatory interactions. Nucleic Acids Res (2018) 46(D1):D380–d386. doi: 10.1093/nar/gkx1013
45. Wu W, Zhang S, He J. The mechanism of long non-coding RNA in cancer Radioresistance/Radiosensitivity: A systematic review. Front Pharmacol (2022) 13:879704. doi: 10.3389/fphar.2022.879704
46. May JM, Bylicky M, Chopra S, Coleman CN, Aryankalayil MJ. Long and short non-coding RNA and radiation response: a review. Trans Res (2021) 233:162–79. doi: 10.1016/j.trsl.2021.02.005
47. Dong S, Wu C, Song C, Qi B, Liu L, Xu Y. Identification of primary and metastatic lung cancer-related lncRNAs and potential targeted drugs based on ceRNA network. Front Oncol (2021) 10. doi: 10.3389/fonc.2020.628930
48. Xie L, Yao Z, Zhang Y, Li D, Hu F, Liao Y, et al. Deep RNA sequencing reveals the dynamic regulation of miRNA, lncRNAs, and mRNAs in osteosarcoma tumorigenesis and pulmonary metastasis. Cell Death Dis (2018) 9(7):772. doi: 10.1038/s41419-018-0813-5
49. Li X, Chen X, Yuan Y, Zhai R, Cho WC, Jiang X. Identifying immune cell infiltration and effective diagnostic biomarkers in lung adenocarcinoma by comprehensive bioinformatics analysis and in vitro study. Front Oncol (2022) 12. doi: 10.3389/fonc.2022.916947
50. Rong J, Wang H, Yao Y, Wu Z, Chen L, Jin C, et al. Identification of m7G-associated lncRNA prognostic signature for predicting the immune status in cutaneous melanoma. Aging (Albany NY) (2022) 14(12):5233–49. doi: 10.18632/aging.204151
51. Li FW, Luo SK. Identification and construction of a predictive immune-related lncRNA signature model for melanoma. Int J Gen Med (2021) 14:9227–35. doi: 10.2147/IJGM.S340025
52. Xu Y, Chen Y, Niu Z, Yang Z, Xing J, Yin X, et al. Ferroptosis-related lncRNA signature predicts prognosis and immunotherapy efficacy in cutaneous melanoma. Front Surg (2022) 9:860806. doi: 10.3389/fsurg.2022.860806
53. Lei G, Zhang Y, Koppula P, Liu X, Zhang J, Lin SH, et al. The role of ferroptosis in ionizing radiation-induced cell death and tumor suppression. Cell Res (2020) 30(2):146–62. doi: 10.1038/s41422-019-0263-3
54. Feng Q, Wang J, Cui N, Liu X, Wang H. Autophagy-related long non-coding RNA signature for potential prognostic biomarkers of patients with cervical cancer: a study based on public databases. Ann Transl Med (2021) 9(22):1668. doi: 10.21037/atm-21-5156
55. Ding X, Cheng L, Chen W, Zhou F, Dou X, Zhang B, et al. Integrative analysis of gene expression in response to low-dose ionizing radiation in a human skin model. Med Oncol (2015) 32(6):173. doi: 10.1007/s12032-015-0621-z
56. Jiang C, Li Y, Zhao Z, Lu J, Chen H, Ding N, et al. Identifying and functionally characterizing tissue-specific and ubiquitously expressed human lncRNAs. Oncotarget (2016) 7(6):7120–33. doi: 10.18632/oncotarget.6859
57. Assefa AT, De Paepe K, Everaert C, Mestdagh P, Thas O, Vandesompele J. Differential gene expression analysis tools exhibit substandard performance for long non-coding RNA-sequencing data. Genome Biol (2018) 19(1):96. doi: 10.1186/s13059-018-1466-5
58. Dai S, Yao D. An immune-associated ten-long noncoding RNA signature for predicting overall survival in cervical cancer. Transl Cancer Res (2021) 10(12):5295–306. doi: 10.21037/tcr-21-2390
59. Zhou R-S, Zhang E-X, Sun Q-F, Ye Z-J, Liu J-W, Zhou D-H, et al. Integrated analysis of lncRNA-miRNA-mRNA ceRNA network in squamous cell carcinoma of tongue. BMC Cancer (2019) 19(1):779. doi: 10.1186/s12885-019-5983-8
60. Chen C, Wang X, Liu T, Tang X, Liu Y, Liu T, et al. Overexpression of long non-coding RNA RP11-363E7.4 inhibits proliferation and invasion in gastric cancer. Cell Biochem Funct (2020) 38(7):921–31.
61. Huang S, Li D, Zhuang L, Zhang J, Wu J. Identification of an epithelial-mesenchymal transition-related long non-coding RNA prognostic signature to determine the prognosis and drug treatment of hepatocellular carcinoma patients. Front Med (Lausanne) (2022) 9:850343. doi: 10.3389/fmed.2022.850343
62. Gu Y, Wan C, Zhou G, Zhu J, Shi Z, Zhuang Z. TYMSOS drives the proliferation, migration, and invasion of gastric cancer cells by regulating ZNF703 via sponging miR-4739. Cell Biol Int (2021) 45(8):1710–9. doi: 10.1002/cbin.11610
63. Yuan Y, Jiang X, Tang L, Wang J, Zhang D, Cho WC, et al. FOXM1/lncRNA TYMSOS/miR-214-3p–mediated high expression of NCAPG correlates with poor prognosis and cell proliferation in non–small cell lung carcinoma. Front Mol Biosci (2022) 8. doi: 10.3389/fmolb.2021.785767
64. Huang J, Song J, Li x., Liu S, Huang W, Shen Z, et al. Analysis and prognostic significance of tumour immune infiltrates and immune microenvironment of m6A-related lncRNAs in patients with gastric cancer. BMC Med Genomics (2022) 15(1):164. doi: 10.1186/s12920-022-01318-5
65. Chen L, Cai Z, Lyu K, Cai Z, Lei W. A novel immune-related long non-coding RNA signature improves the prognosis prediction in the context of head and neck squamous cell carcinoma. Bioengineered (2021) 12(1):2311–25. doi: 10.1080/21655979.2021.1943284
66. Wei C, Liang Q, Li X, Li H, Liu Y, Huang X, et al. Bioinformatics profiling utilized a nine immune-related long noncoding RNA signature as a prognostic target for pancreatic cancer. J Cell Biochem (2019) 120(9):14916–27. doi: 10.1002/jcb.28754
67. Williams AB, Schumacher B. p53 in the DNA-Damage-Repair process. Cold Spring Harb Perspect Med (2016) 6(5). doi: 10.1101/cshperspect.a026070
68. O'Malley J, Kumar R, Inigo J, Yadava N, Chandra D. Mitochondrial stress response and cancer. Trends Cancer (2020) 6(8):688–701. doi: 10.1016/j.trecan.2020.04.009
69. Zhang M, Wang G, Zhu Y, Wu D. Characterization of BRCA1/2-directed ceRNA network identifies a novel three-lncRNA signature to predict prognosis and chemo-response in ovarian cancer patients with wild-type BRCA1/2. Front Cell Dev Biol (2020) 8:680. doi: 10.3389/fcell.2020.00680
70. Li YJ, Li HY, Zhang Q, Wei SL. The prognostic value and immune landscape of a cuproptosis-related lncRNA signature in head and neck squamous cell carcinoma. Front Genet (2022) 13:942785. doi: 10.3389/fgene.2022.942785
71. Wang Y, Zhang L, Zhou F. Cuproptosis: a new form of programmed cell death. Cell Mol Immunol (2022) 19(8):867–8. doi: 10.1038/s41423-022-00866-1
72. Xue F, Song X, Zhang S, Niu M, Cui Y, Wang Y, et al. Long non-coding RNA TMPO-AS1 serves as a tumor promoter in pancreatic carcinoma by regulating miR-383-5p/SOX11. Oncol Lett (2021) 21(4):255. doi: 10.3892/ol.2021.12517
73. Luo X-J, He M-M, Liu J, Zheng J-B, Wu Q-N, Chen Y-X, et al. LncRNA TMPO-AS1 promotes esophageal squamous cell carcinoma progression by forming biomolecular condensates with FUS and p300 to regulate TMPO transcription. Exp Mol Med (2022) 54(6):834–47. doi: 10.1038/s12276-022-00791-3
74. He YC, Bi YG, Jiang L. LncRNA TMPO-AS1 promotes proliferation and migration in bladder cancer. Eur Rev Med Pharmacol Sci (2020) 24(17):8740–6.
75. Zhang L, Wang G, Chen S, Ding J, Ju S, Cao H, et al. Depletion of thymopoietin inhibits proliferation and induces cell cycle arrest/apoptosis in glioblastoma cells. World J Surg Oncol (2016) 14(1):267. doi: 10.1186/s12957-016-1018-y
76. Li Z, Feng Y, Zhang Z, Cao X, Lu X. TMPO-AS1 promotes cell proliferation of thyroid cancer via sponging miR-498 to modulate TMPO. Cancer Cell Int (2020) 20(1):294. doi: 10.1186/s12935-019-1088-3
77. Liu G, Yang H, Cao L, Han K, Li G. LncRNA TMPO-AS1 promotes proliferation and invasion by sponging miR-383-5p in glioma cells. Cancer Manag Res (2020) 12:12001–9. doi: 10.2147/CMAR.S282539
78. Wei L, Liu Y, Zhang H, Ma Y, Lu Z, Gu Z, et al. TMPO-AS1, a novel E2F1-regulated lncRNA, contributes to the proliferation of lung adenocarcinoma cells via modulating miR-326/SOX12 axis. Cancer Manag Res (2020) 12:12403–14. doi: 10.2147/CMAR.S269269
79. Zhang Y, Zhu Y, Xiao M, Cheng Y, He D, Liu J, et al. The long non-coding RNA TMPO-AS1 promotes bladder cancer growth and progression via OTUB1-induced E2F1 deubiquitination. Front Oncol (2021) 11. doi: 10.3389/fonc.2021.643163
80. Crosby ME, Jacobberger J, Gupta D, Macklis RM, Almasan A. E2F4 regulates a stable G2 arrest response to genotoxic stress in prostate carcinoma. Oncogene (2007) 26(13):1897–909. doi: 10.1038/sj.onc.1209998
81. Bernstein JL, Haile RW, Stovall M, Boice JD Jr., Shore RE, Langholz B, et al. Radiation exposure, the ATM gene, and contralateral breast cancer in the women's environmental cancer and radiation epidemiology study. J Natl Cancer Inst (2010) 102(7):475–83. doi: 10.1093/jnci/djq055
82. Logotheti S, Richter C, Murr N, Spitschak A, Marquardt S, Pützer BM. Mechanisms of functional pleiotropy of p73 in cancer and beyond. Front Cell Dev Biol (2021) 9. doi: 10.3389/fcell.2021.737735
83. Zhang H, Liu T, Yi S, Gu L, Zhou M. Targeting MYCN IRES in MYCN-amplified neuroblastoma with miR-375 inhibits tumor growth and sensitizes tumor cells to radiation. Mol Oncol (2015) 9(7):1301–11. doi: 10.1016/j.molonc.2015.03.005
84. Kim A, Shim S, Kim YH, Kim MJ, Park S, Myung JK. Inhibition of y box binding protein 1 suppresses cell growth and motility in colorectal cancer. Mol Cancer Ther (2020) 19(2):479–89. doi: 10.1158/1535-7163.MCT-19-0265
85. Martinez LA, Goluszko E, Chen HZ, Leone G, Post S, Lozano G, et al. E2F3 is a mediator of DNA damage-induced apoptosis. Mol Cell Biol (2010) 30(2):524–36. doi: 10.1128/MCB.00938-09
86. Kan C, Zhang J. BRCA1 mutation: A predictive marker for radiation therapy? Int J Radiat Oncol Biol Phys (2015) 93(2):281–93. doi: 10.1016/j.ijrobp.2015.05.037
87. Pham TP, Bink DI, Stanicek L, van Bergen A, van Leeuwen E, Tran Y, et al. Long non-coding RNA aerrie controls DNA damage repair via YBX1 to maintain endothelial cell function. Front Cell Dev Biol (2020) 8:619079. doi: 10.3389/fcell.2020.619079
88. Garofalo M, Di Leva G, Romano G, Nuovo G, Suh SS, Ngankeu A, et al. miR-221&222 regulate TRAIL resistance and enhance tumorigenicity through PTEN and TIMP3 downregulation. Cancer Cell (2009) 16(6):498–509. doi: 10.1016/j.ccr.2009.10.014
89. Wang X, Liao X, Huang K, Zeng X, Liu Z, Zhou X, et al. Clustered microRNAs hsa-miR-221-3p/hsa-miR-222-3p and their targeted genes might be prognostic predictors for hepatocellular carcinoma. J Cancer (2019) 10(11):2520–33. doi: 10.7150/jca.29207
90. Derrien T, Johnson R, Bussotti G, Tanzer A, Djebali S, Tilgner H, et al. The GENCODE v7 catalog of human long noncoding RNAs: analysis of their gene structure, evolution, and expression. Genome Res (2012) 22(9):1775–89. doi: 10.1101/gr.132159.111
91. Shaw A, Gullerova M. Home and away: The role of non-coding RNA in intracellular and intercellular DNA damage response. Genes (Basel) (2021) 12(10). doi: 10.3390/genes12101475
92. Podralska M, Ciesielska S, Kluiver J, Berg Avd, Dzikiewicz-Krawczyk A, Slezak-Prochazka I. Non-coding RNAs in cancer radiosensitivity: MicroRNAs and lncRNAs as regulators of radiation-induced signaling pathways. Cancers (Basel) (2020) 12(6). doi: 10.3390/cancers12061662
93. Zhu J, Chen S, Yang B, Mao W, Yang X, Cai J. Molecular mechanisms of lncRNAs in regulating cancer cell radiosensitivity. Biosci Rep (2019) 39(8). doi: 10.1042/BSR20190590
94. Xie Y, Han J, Xie K, Gou Q. LncRNAs as biomarkers for predicting radioresistance and survival in cancer: a meta-analysis. Sci Rep (2022) 12(1):18494. doi: 10.1038/s41598-022-21785-1
95. Zhang Y, He W, Zhang S. Seeking for correlative genes and signaling pathways with bone metastasis from breast cancer by integrated analysis. Front Oncol (2019) 9:138. doi: 10.3389/fonc.2019.00138
96. Zhang Y, Huang X, Liu J, Chen G, Liu C, Zhang S, et al. New insight into long non-coding RNAs associated with bone metastasis of breast cancer based on an integrated analysis. Cancer Cell Int (2021) 21(1):372. doi: 10.1186/s12935-021-02068-7
Glossary
Abbreviation: Full
ANLN: Anillin Actin Binding Protein
ANP32E: Acidic Nuclear Phosphoprotein 32 Family Member E
ATM: ATM Serine/Threonine Kinase
BBC3: Bcl2 Binding Component 3
BRCA1: Brca1 DNA Repair Associated
BRCA2: Brca2 DNA Repair Associated
BTG2: BTG Anti-Proliferation Factor 2
CCNA2: Cyclin A2
CDCA3: Cell Division Cycle Associated 3
CDCA5: Cell Division Cycle Associated 5
CDKN1A: Cyclin Dependent Kinase Inhibitor 1a
CENPN: Centromere Protein N
CENPO: Centromere Protein O
CEP55: Centrosomal Protein 55
CKAP2: Cytoskeleton Associated Protein 2
COLCA1: Colorectal Cancer Associated 1
DEG: Differentially Expressed Gene
DIAPH3: Diaphanous Related Formin 3
DINOL: Damage Induced Long Noncoding Ribonucleic Acid
DNA: Deoxyribonucleic Acid
DPYSL3: Dihydropyrimidinase Like 3
DTX2P1-UPK3BP1-PMS2P11: Dtx2p1-Upk3bp1-Pms2p11 Readthrough, Transcribed Pseudogene
E2F1: E2f Transcription Factor 1
E2F3: E2f Transcription Factor 3
E2F4: E2f Transcription Factor 4
FAM82B: Family With Sequence Similarity 82, Member B
FANCD2: Fa Complementation Group D2
FDR: False Discovery Rate
FOXM1: Forkhead Box M1
FUS: Fus Ribonucleic Acid Binding Protein
GO: Gene Ontology
Gy: Gray
HDIR: High Dose of Ionizing Radiation
HSPA4L: Heat Shock Protein Family A (Hsp70) Member 4 Like
IER5: Immediate Early Response 5
ILF3-DT: Ilf3 Divergent Transcript
IR: Ionizing Radiation
ITGB1: Integrin Subunit Beta 1
ITPK1-AS1: Itpk1 Antisense Ribonucleic Acid 1
KIF11: Kinesin Family Member 11
KIF20B: Kinesin Family Member 20b
KIF23: Kinesin Family Member 23
KNL1: Kinetochore Scaffold 1
LDIR: Low Dose of Ionizing Radiation
LFC: Log2 Fold-Change
LINC00173: Long Intergenic Non-Protein Coding Ribonucleic Acid 173
LINC00336: Long Intergenic Non-Protein Coding Ribonucleic Acid 336
LINC00467: Long Intergenic Non-Protein Coding Ribonucleic Acid 467
LINC00852: Long Intergenic Non-Protein Coding Ribonucleic Acid 852
LINC00963: Long Intergenic Non-Protein Coding Ribonucleic Acid 963
lncRNA: Long Non-Coding Ribonucleic Acid
M6A: N6-Methyladenosine
MDM2: Mouse Double Minute Homolog 2 Proto-Oncogene
MEG3: Mate Ribonucleic Acidly Expressed 3
MIR22HG: Mir22 Host Gene
MIR31HG: Mir31 Host Gene
MIR7-3HG: Mir7-3 Host Gene
miRNA: Micro Ribonucleic Acid
mRNA: Messenger Ribonucleic Acid
MYBL1: Myb Proto-Oncogene Like 1
MYBL2: Myb Proto-Oncogene Like 2
MYCN: Mycn Proto-Oncogene, Bhlh Transcription Factor
N0: Fibroblasts of Cancer-Free Controls
N1: Fibroblasts of Donors with a First Primary Neoplasm in Childhood
N2+: Fibroblasts of Donors with a First Primary Neoplasm in Childhood and at least one Second Primary Neoplasm
NCAPD2: Non-Smc Condensin I Complex Subunit D2
ncRNA: Non-Coding Ribonucleic Acid
NORAD: Non-Coding Ribonucleic Acid Activated By DNA Damage
OR2A1-AS1: Or2a1 Antisense Ribonucleic Acid 1
p21: Cyclin-Dependent Kinase Inhibitor 1
PAPPA-AS1: Pappa Antisense Ribonucleic Acid 1
PARTICLE: Promoter Of MAT2A Antisense Radiation-Induced Circulating Long Non-Coding RNA
PCNA: Proliferating Cell Nuclear Antigen
PPM1D: Protein Phosphatase, Mg2+/Mn2+ Dependent 1d
PRC1: Protein Regulator Of Cytokinesis 1
PRR11: Proline Rich 11
PTTG1: Pttg1 Regulator Of Sister Chromatid Separation, Securin
PVT1: Pvt1 Oncogene
REVIGO: Reduce And Visualize Gene Ontology
RIC1: Ric1 Homolog, Rab6a Gef Complex Partner 1
RNA: Ribonucleic Acid
RPP38-DT: Rpp38 Divergent Transcript
rRNA: Ribosomal Ribonucleic Acid
SESN1: Sestrin 1
SHCBP1: Shc Binding And Spindle Associated 1
STIL: Stil Centriolar Assembly Protein
STXBP5-AS1: Stxbp5 Antisense Ribonucleic Acid 1
SUSD6: Sushi Domain Containing 6
TANC1: Tetratricopeptide Repeat, Ankyrin Repeat And Coiled-Coil Containing 1
TCP11L1: T-Complex 11 Like 1
TIGAR: Tp53 Induced Glycolysis Regulatory Phosphatase
TINCR: Tincr Ubiquitin Domain Containing
TMPO: Thymopoietin
TMPO-AS1: Tmpo Antisense Ribonucleic Acid 1
TNFRSF10D: Tnf Receptor Superfamily Member 10d
TOP2A: DNA Topoisomerase Ii Alpha
TP53: Tumor Protein P53
TP53I3: Tumor Protein P53 Inducible Protein 3
TP63: Tumor Protein P63
TP73: Tumor Protein P73
TPTEP1: Tpte Pseudogene 1
TPX2: Tpx2 Microtubule Nucleation Factor
TRAIL: TNF-related Apoptosis-Inducing Ligand
TRHDE-AS1: Trhde Antisense Ribonucleic Acid 1
TTTY14: Testis-Specific Transcript, Y-Linked 14
TUBA1B: Tubulin Alpha 1b
TYMSOS: Tyms Opposite Strand Ribonucleic Acid
WGCNA: Weighted Gene Co-Expression Network Analysis
YBX1: Y-Box Binding Protein 1
ZWILCH: Zwilch Kinetochore Protein
Keywords: weighted co-expression network analysis (WGCNA), differential gene expression analysis, RNA-Seq, radiation experiments, NGS - next generation sequencing, radiation response, KiKme Study
Citation: Grandt CL, Brackmann LK, Poplawski A, Schwarz H, Marini F, Hankeln T, Galetzka D, Zahnreich S, Mirsch J, Spix C, Blettner M, Schmidberger H and Marron M (2023) Identification of lncRNAs involved in response to ionizing radiation in fibroblasts of long-term survivors of childhood cancer and cancer-free controls. Front. Oncol. 13:1158176. doi: 10.3389/fonc.2023.1158176
Received: 03 February 2023; Accepted: 27 March 2023;
Published: 27 April 2023.
Edited by:
Tsair-Fwu Lee, National Kaohsiung University of Science and Technology, TaiwanReviewed by:
Renjun Peng, Central South University, ChinaSen Zhang, Chinese Academy of Medical Sciences and Peking Union Medical College, China
Zhirui Zeng, Guizhou Medical University, China
Copyright © 2023 Grandt, Brackmann, Poplawski, Schwarz, Marini, Hankeln, Galetzka, Zahnreich, Mirsch, Spix, Blettner, Schmidberger and Marron. This is an open-access article distributed under the terms of the Creative Commons Attribution License (CC BY). The use, distribution or reproduction in other forums is permitted, provided the original author(s) and the copyright owner(s) are credited and that the original publication in this journal is cited, in accordance with accepted academic practice. No use, distribution or reproduction is permitted which does not comply with these terms.
*Correspondence: Caine Lucas Grandt, c2VjLWVwaUBsZWlibml6LWJpcHMuZGU=