- 1Department of Medical Ultrasound, Tongji Hospital, Tongji Medical College, Huazhong University of Science and Technology, Wuhan, China
- 2Department of Ultrasonography, The First Hospital of Changsha, Changsha, China
- 3Health Medical Department, Dalian Municipal Central Hospital, Dalian, China
- 4Department of Medical Ultrasound, Minda Hospital of Hubei Minzu University, Enshi, China
- 5Department of Medical Ultrasound, The First Affiliated Hospital of Anhui Medical University, Hefei, China
- 6Department of Internal Medicine, Hirslanden Clinic, Bern, Switzerland
Ultrasound elastography (USE) provides complementary information of tissue stiffness and elasticity to conventional ultrasound imaging. It is noninvasive and free of radiation, and has become a valuable tool to improve diagnostic performance with conventional ultrasound imaging. However, the diagnostic accuracy will be reduced due to high operator-dependence and intra- and inter-observer variability in visual observations of radiologists. Artificial intelligence (AI) has great potential to perform automatic medical image analysis tasks to provide a more objective, accurate and intelligent diagnosis. More recently, the enhanced diagnostic performance of AI applied to USE have been demonstrated for various disease evaluations. This review provides an overview of the basic concepts of USE and AI techniques for clinical radiologists and then introduces the applications of AI in USE imaging that focus on the following anatomical sites: liver, breast, thyroid and other organs for lesion detection and segmentation, machine learning (ML) - assisted classification and prognosis prediction. In addition, the existing challenges and future trends of AI in USE are also discussed.
Introduction
Hardness or stiffness is an important biomarker of abnormal tissue. Changes in tissue hardness are often accompanied by common disease progression (1). It is also well known that cancerous tissue tends to be stiffer than benign and normal tissues (2). Ultrasound elastography (USE) is an emerging imaging technology sensitive to tissue stiffness. By adding the tissue stiffness as another measurement characteristic to the conventional ultrasound imaging system, USE can provide added power for improving the diagnostic performance in various diseases. USE has been gradually applied to the evaluation of diseases in some superficial organs, where tissue stiffness is closely related to the specific pathological process, such as characterizing breast masses (3) and thyroid nodules (4), assessing liver fibrosis (5) and detecting prostate lesions (6).
However, due to the great intra- and inter-observer variabilities and the instable diagnostic performance or even low accuracy with the manual interpretation of inexperienced radiologists, the analysis of medical images is challenging, especially for USE images in which the boundary of a lesion is usually implicit (7). The limitations mainly lie in the difficulty of identifying optimal stiffness cutoff values, the variability of the region of interest (ROI) selection and the lack of an image quality check (8). Besides, a wide disparity in diagnostic performance has been reported (9).
Artificial intelligence (AI) has been recognized as the Fourth Industrial Revolution because it is reshaping multiple fields worldwide, ranging from facial recognition to self-driving vehicles or natural language processing (10). Interpretating medical images is inherently a data processing step in which AI can be applied in the medical domain (11). Moreover, the availability of novel AI techniques, emerging imaging techniques and massive imaging datasets have made medical imaging a research field of AI (12). As a subset of AI, the rapid development of machine learning (ML) approaches, especially the advanced deep learning (DL) architectures, offer great potential to perform automatic medical image analysis tasks, such as segmentation, detection and classification (13).
Researches have already shown the significant value of ML- or DL-based analysis using conventional gray scale ultrasound images for diseases evaluation (14–17). As USE has gradually been used as a complement to conventional ultrasound by providing information on tissue elasticity, there is a growing trend in applications of AI-based USE images. By removing variability between examiners, these developed models have extensively enabled the accuracies of image interpretation and allowed the disease diagnosis go much further.
Although reference (18) was the first review on DL methods in the USE imaging, this article mainly reviews novel DL architectures applied to USE from an engineering perspective. Reference (19) covers a limited number of articles about the ML models applied to USE for breast tumor classification. Therefore, to our knowledge, there is no literature that provides radiologists with a comprehensive and clinically-oriented review of AI applied to USE imaging in a more easily-readable manner. In this review, a brief overview of USE and AI techniques (including ML, DL and radiomics) is provided. Then, the applications of AI-based USE for disease evaluation in several anatomical organs in the order of automatic analysis tasks are introduced. Finally, the existing challenges and future trends with the application of AI based on USE are discussed.
Overview of ultrasound elastography
In general, the USE technique can be classified into strain elastography (SE) applying constant stress and shear wave elastography (SWE) applying time-varying force. Figure 1 shows the category of the USE technique according to excitation methods. All of the approaches are based on the three-phase methodology: (a) tissue is compressed by static stress or shear wave propagation; (b) the displacements in tissues are tracked by ultrasound; and (c) tissue elasticity is estimated quantitatively or qualitatively from the measured displacements. Moreover, a physical property named as Young’s modulus (E) is calculated to estimate the tissue stiffness. Harder lesions have smaller deformations, lower strains and higher E values (1).
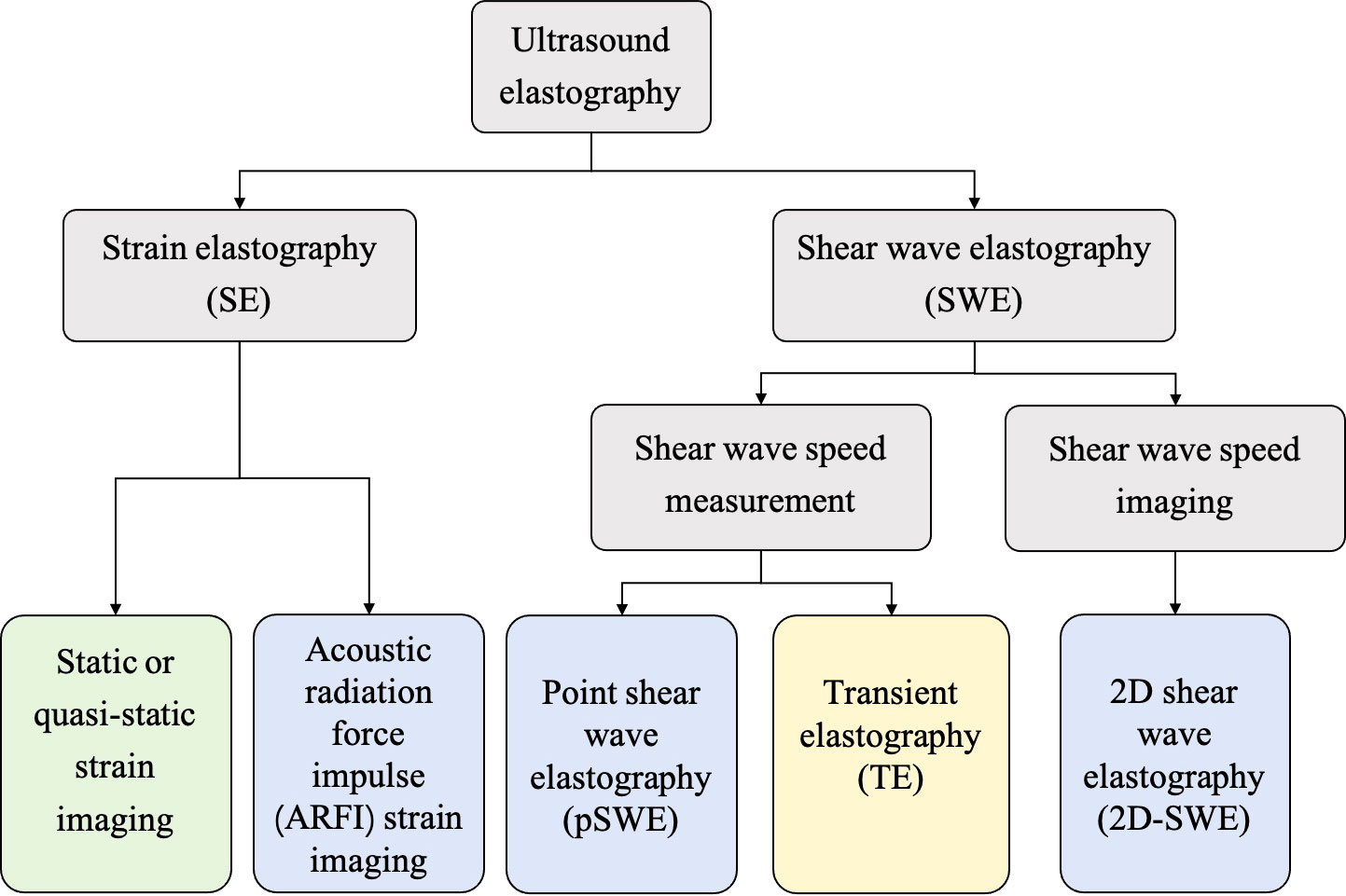
Figure 1 The category of ultrasound elastography (USE) technique according to the excitation methods, including external compression or internal physiologic motion (green), acoustic radiation force impulse (blue), and mechanical vibrating (yellow).
Strain elastography
SE was the first elastography imaging system introduced in the 1990s and can be divided into two methods (20): (a) static or quasi-static strain imaging: the operator manually compresses the tissue with an ultrasound transducer; and (b) acoustic radiation force impulse (ARFI) strain imaging: a short-duration high-intensity acoustic “pushing” pulse called ARFI can be used to displace the tissue (21).
According to Hooke’s law, E in strain imaging can be calculated by the equation E=σ/ϵ, where σ is the externally applied stress and ϵ is the strain, which equals the displacement (21). Due to the unknown applied stress to the tissue, the strain imaging system cannot provide the qualitative value of E. Therefore, in clinical practice, strain ratio (SR) is an often-used semiquantitative measurement. SR can be calculated as the ratio between the strain in the normal reference region and the strain in the region of interest. SR>1 indicates that the deformation of the target lesion is less than that of the normal reference tissue, indicating lower strain and greater hardness (1). There are some other common parameters: elasticity scores (ES) or grading systems, fat-to-lesion SR and elastography-to-B-mode size ratio (1).
Shear wave elastography
SWE, which is more quantitative and reproducible than SE, can be classified into two methods: (a) transient elastography: The first commercial SWE system Fibroscan™ is based on transient elastography, which is widely used to estimate liver fibrosis (5); (b) point shear wave elastography and 2D-SWE: ARFI is used as the external excitation.
Moreover, in contrast to nonimaging USE methods (transient elastography and point shear wave elastography), 2D-SWE is an emerging technology that can measure shear wave velocity (SWV) or E in real time and generate quantitative elastograms (21). The semitransparent color elastogram is usually overlayed on the corresponding B-mode sonogram, with red usually representing hard tissue and blue representing soft tissue. The SWV and E are related to the colors in the color bar along the image (22). The system will report E by using the equation E=3ρcs2, in which ρ represents the tissue density and cs represents the SWV (1). SWV is higher in hard tissue and lower in soft tissue.
Overview of artificial intelligence
Machine learning
As a branch of AI, ML enables the creation of algorithms that are able to learn from data and make predictions, thus enabling computers to learn like humans (23). It is an interdisciplinary field involving computer science, statistics and a variety of other disciplines concerning automatic improvement (24).
According to the types of labels utilized in the training dataset, ML techniques can be broadly classified by supervised, unsupervised and reinforcement learning (25) (Figure 2). Most ML algorithms related to radiology are supervised (11), which requires instances labeled with the desired classification outputs, named the “ground truth”. Examples of such algorithms include artificial neural networks (ANNs), support vector machine (SVM), random forest (RF), logistic regression, Naïve Bayes classifier, decision tree and K-nearest neighbor. In contrast, unsupervised learning is an algorithm in which instances are unlabeled and clusters of data need to be identified (26). Examples of such algorithms are K-means, fuzzy C-means clustering and Markov random fields. Since acquiring well-labeled databases is time-consuming, reinforcement learning, as a hybrid of supervised and unsupervised learning, uses less detailed information to train a model (27). It acquires data by learning from dynamic environment interaction (the computer will receive positive or negative reinforcement feedback) without being explicitly taught. The overview of ML strategies and their related algorithms and applications used in USE imaging has been presented in Table 1.
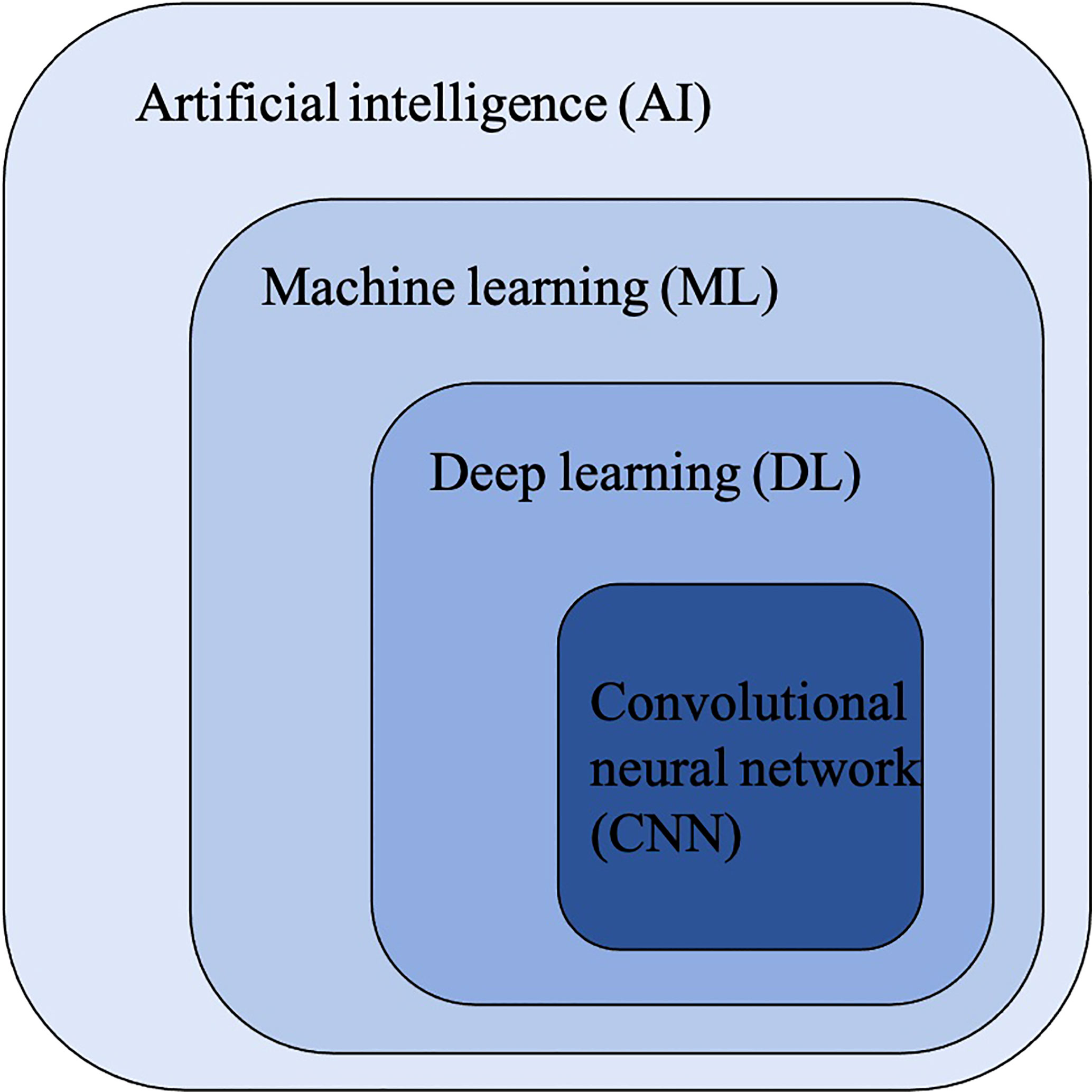
Figure 2 The relationship between artificial intelligence (AI), machine learning (ML), deep learning (DL) and convolutional neural networks (CNNs).
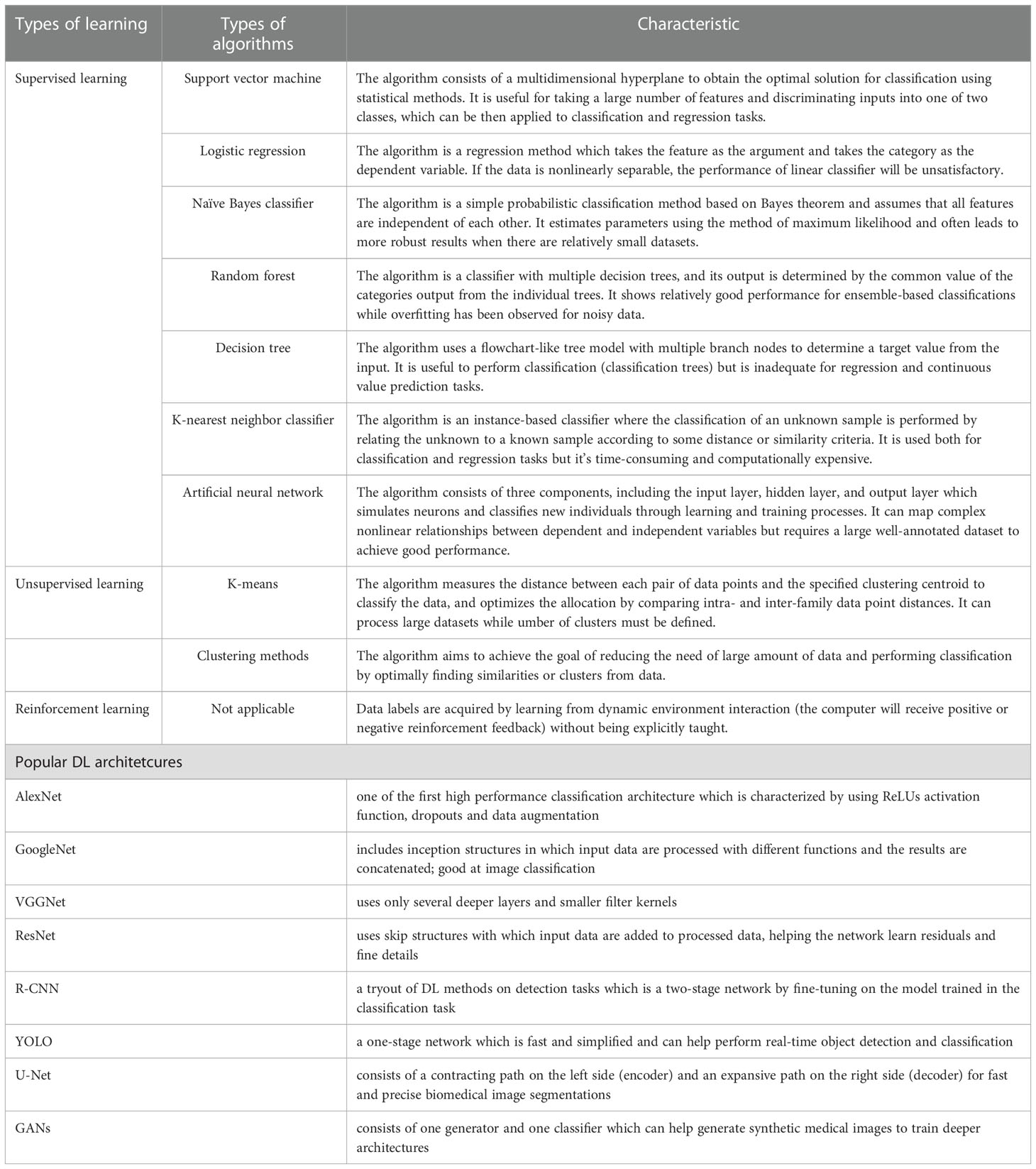
Table 1 Overview of machine learning strategies and their related algorithms and popular deep learning architectures.
In the field of radiology, ML algorithms often start with a set of available inputs (the image data, for example) and desired outputs (the classification of malignant and benign tumors, for example) (11). The input datasets are usually split into two sets: training and validation datasets. The training dataset serves to find the optimal weights and fit the model, while the validation dataset is used to optimize the parameters. After a model is developed, there is often an urgent need for an independent external test dataset to evaluate the performance and generalizability of the developed model (23).
Deep learning
The availability of large-scale labeled datasets, faster algorithms and more powerful parallel computing hardware, such as Graphics Processing Unit, have enabled the fast application of DL (28). DL methods enable to solve problems that have resisted the best attempts of the AI community for many years, since the use of large numbers of layers allow the improved universal approximation properties and the more features to be learned from the data with multiple levels of hierarchy and abstraction (29).
Convolutional Neural Networks (CNNs) have been considered as the state-of-art algorithms of DL methods in the field of radiology for computer vision tasks such as segmentation (30), detection (31, 32) and classification (33). There are four key ideas behind CNNs: local connections, shared weights, pooling and the use of many layers, resulting in the improved accuracy and efficiency of the whole system (29). The relationship between AI, ML, DL and CNNs is shown in Figure 3. CNNs are formed by a stack of one input layer, one output layer and multiple hidden layers, which consist of convolutional layers, pooling layers and fully-connected layers (34) (Figure 4). With convolution and pooling applied repeatedly, fully-connected layers are used to make classification or predictions (35). The combinations of layers are various and some deep neural network architectures have been successfully utilized in image analysis, such as GoogleNet (36), AlexNet (37), VGGNet (38) and ResNet (39).
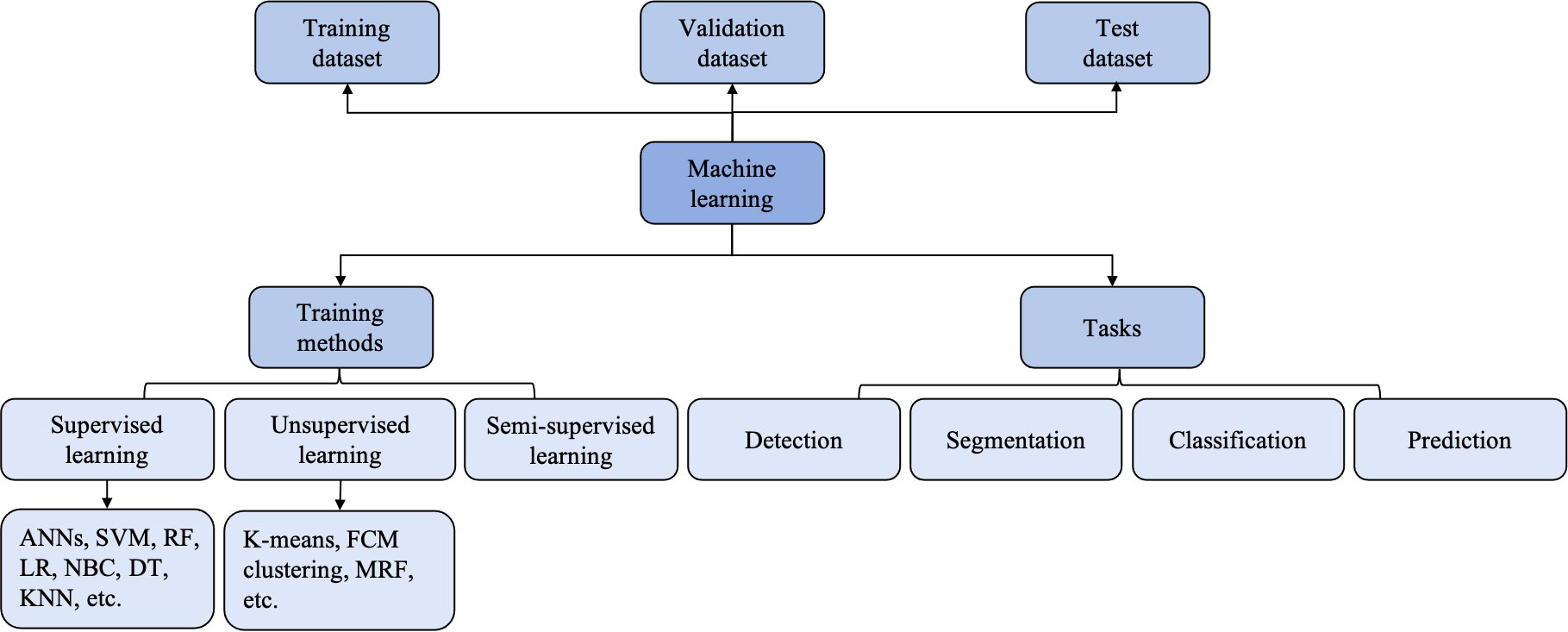
Figure 3 The classification and computer vision task of machine learning (ML). ANNs, artificial neural networks; DT, deciosin tree; FCM clustering, fuzzy C-means clustering; KNN, K-nearest neighbor; LR, logiastic regression; MRF, Markov random fields; NBC, Naïve Bayes classifier; RF, random forest; SVM, support vector machine.
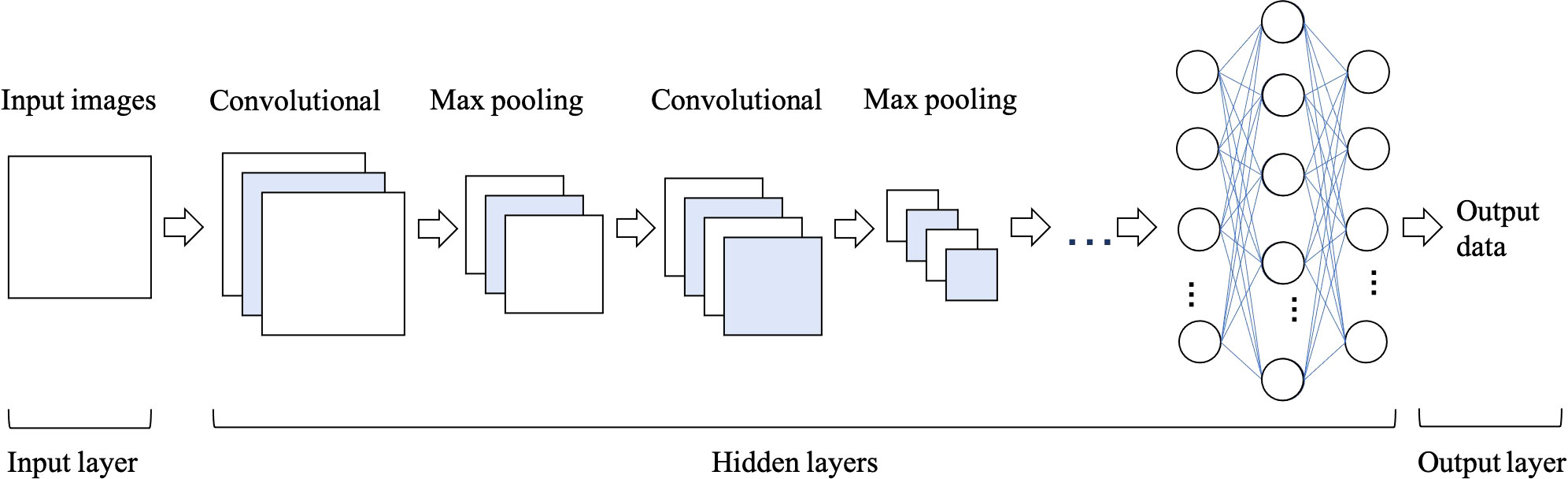
Figure 4 The architecture of convolutional neural network (CNN), which is formed of one input layer, multiple hidden layers and one output layer. Convolutional and max pooling layers can be stacked alternately until the network is deep enough to acquire optimal features of the images that are salient for classification task.
Transfer learning
Transfer learning (TL) strategies have recently been utilized in the medical world to avoid the overfitting problems caused by the lack of data. Within the TL method, knowledge can be shared and transferred between different tasks (40). The workflow involves two steps: pretrained on a large dataset (the ImageNet, for example) and fine-tuning on the target dataset (the limited ultrasound images, for example). In other words, by fine-tuning the DL architecture, the knowledge learned from one dataset can be transferred to another dataset obtained from another center.
Radiomics
Radiomics is defined as quantitative mapping, namely, to extract and analyze vast arrays of high-dimensional medical image features that are related to the prediction targets (41). Radiomics features, such as intensity, shape, texture or wavelet, reflect the underlying pathophysiology and provide the information on tumor phenotype and microenvironment (42). These features can be used solely or in combination with other relevant data sources (such as clinical reports, laboratory tests or genomic data) for robust evidence-based decision support to facilitate prediction, diagnosis, prognosis, or monitoring (41).
Radiomics features can be scored semantically by experienced radiologists, or can be mathematically computer-calculated to describe the size, shape and textures of the ROI, or can be created by DL algorithms (43). The calculated features are subsequently analyzed usually using traditional ML methods to build predictive models (44). Researches have already shown the capacity of radiomics analyses to increase diagnostic, prognostic, and predictive power (42).
Applications of AI in USE imaging
The primary automatic analysis tasks of AI-based USE models involve (13) (a) classification: to predict the target class labels of an image (to classify breast tumors as benign or malignant, for example); (b) detection: to predict the location of focal lesions, which is a prerequisite step for radiologists to characterize lesions; (c) segmentation: to distinguish the suspicious lesions from the surrounding normal tissues, that is, to acquire the ROI; and (d): prediction: to predict the status of disease or events that may happen.
The early developed AI-based models follow the traditional method: computing the handcrafted features, applying the feature selection algorithm to reduce the features in dimension, and training a classifier to acquire the best results (28). Overall, feature extraction and selection are the most important steps, helping to achieve the best diagnostic results (45).
Compared to conventional ML methods, the key aspect of DL algorithms is that these layers of features are not handcrafted with human expertise: they are automatically learned from data using a general-purpose learning procedure (29). It allows learning an end-to-end mapping from the input to the output, that is, the image-to-classification methods (13). In traditional ML methods, the accurate segmentation and choice of expert-designed features are keys to success. These limitations can be overcome by DL approaches, because these algorithms can identify the regions of the image that are most associated with the outcome by training themselves and can identify the features of the region that informed decision by multiple layers (43). The comparison of the traditional ML-based models and DL-based models is shown in Figure 5.

Figure 5 Conventional machine learning (ML)-based ultrasound elastography (USE) models vs deep learning (DL)-based USE models. Conventional ML-based USE models depend on carefully handcrafted features, while DL allows learning an end-to-end mapping from the input to the output.
The more influential literature on the applications of AI in USE images are subsequently summarized in terms of anatomical sites. USE is prone to be performed in most of the organs, including breast, thyroid, liver and so on.
Liver disease evaluation
Many etiologies of chronic liver diseases (CLDs) follow a common pathway to liver fibrosis, cirrhosis and hepatocellular carcinoma (HCC). HCC is the third highest cause of cancer mortality worldwide (46). Liver biopsy has traditionally served as the gold standard for staging liver fibrosis in CLD and diagnosing HCC. However, it is invasive and has some limitations, such as sampling error and postoperative complications (47). Compared to standard ultrasound, USE has become a popular non-invasive technique by providing additional information regarding liver tissue stiffness. AI-based SE models can help improve the diagnostic accuracy while reducing the unnecessary biopsies for either staging liver fibrosis in CLD patients or differentiating focal liver lesions (FLLs) from benign to malignant.
Staging liver fibrosis
Liver fibrosis is a principal factor in the development of CLD. Precise assessment of the amount and progression of liver fibrosis is of great value for the management and prognosis of CLD patients. Liver stiffness measured from USE has been regarded as the first-line noninvasive method; thus, AI-based USE models is proposed to reduce variability in stiffness measurements and to improve the diagnostic accuracy of liver fibrosis evaluation.
Earlier studies have extracted a series of hand-engineered features and then fed into various ML classifiers for predicting the different liver fibrosis levels. Textural features, liver stiffness and SWV have been proven to be the discriminant features, with ANN, SVM and RF being the most used classifiers (48–54).
More currently, AI with DL methods is expected to directly operate on the input images and reveal information that human experts cannot recognize. The better diagnostic performance of CNN than conventional ML classifiers for staging liver fibrosis has been demonstrated in the study of Brattain and coworkers (55). To obtain more standard SWE measurements and to detect significant fibrosis in patients with nonalcoholic fatty liver disease, they developed an automated framework called “SWE-Assist”. The model could automatically check the quality of SWE images, select a ROI, and classify the ROI with RF, SVM classifiers and CNNs. The CNN architecture yielded the largest classification improvement with an AUC of 0.89 in a large dataset of 3392 SWE images. A new method called DL radiomics of elastography (DLRE) has been proposed by Wang et al., which was expected to extract more radiomics features (56). In 398 patients with 1990 images from 12 hospitals, DLRE reached AUCs of 0.97 for F4, 0.98 for ≥ F3 and 0.85 for ≥ F2, which were significantly better than LSM and biomarkers. They also found that the model generated better performance as more images were acquired. As the DLRE model in Wang et al. showed limited accuracy in assessing ≥ F2, Lu et al. developed a new model named DLRE 2.0 based on the previous model by using the TL strategy for a more accurate evaluation of ≥ F2 liver fibrosis (57). They also developed three other DLRE-based models by gradually adding the tissue texture of the liver capsule and the liver parenchyma and serological results to DLRE 2.0. For evaluating ≥ F2, the performance of DLRE2.0 was significantly better than the previous DLRE model (AUCs: 0.84 vs 0.92, p < 0.05). However, no significant improvement was shown when adding more information. By using the TL strategy for classifying liver fibrosis, Xue et al. pretrained the InceptionV3 network on ImageNet to analyze multimodal BMUS and SWE images, generating higher diagnostic accuracy than non-TL with significantly higher AUCs (0.950, 0.932, and 0.930 for classifying F4, ≥ F3, and ≥ F2, respectively) (58). Furthermore, the model based on the multi-modal images yielded the highest diagnosis accuracy, outperformed the single-modality, LSM, and biomarkers.
How to define a reliable SWE image, especially a temporally stable SWE color box, is one of the limitations of USE. Gatos et al. tried to identify the temporally stable regions across SWE frames and to evaluate the impact of temporal stability for classifying various CLD combinations by means of a pretrained CNN scheme (59). The stability masked SWE images showed improved diagnostic performance compared to the unmasked ones. Therefore, in their study in 2020 (60), full and temporally stable masked SWE images were separately fed into GoogleNet, AlexNet, VGG16, ResNet50 and DenseNet201 with or without augmentation. All networks achieved maximum mean accuracies ranging from 87.2%–97.4% and AUCs ranging from 0.979–0.990.
Classification and evaluation of focal liver lesions
Early detection and precise diagnosis of HCC are crucial for treatment selection and patient prognosis, making the differentiation of FLLs an important task. Since structural alterations can be reflected by changes in tissue stiffness, USE, especially SWE, has been widely used for differentiating benign from malignant FLLs (61, 62).
For different diagnostic purposes (all including FLL classification), radiomics approaches have been proposed with an emphasis on the extraction of high-dimensional features from different modality ultrasound images and the integration of the high-dimensional features with the low-dimensional data, such as expert-designed SWE parameters or serological clinical data (63, 64). Specifically, to classify FLLs, Wang et al. established two radiomics models: the ultrasomics score (based on radiomics features only) and the combined score (based on radiomics features and quantitvative SWE measurements) (63). With 1044 features extracted by ultrasomics, they found that the combined score had the best performance, yielding an AUC of 0.92. Chronic hepatitis B infection is the main risk factor for HCC in China. Early and accurate prediction of HCC occurrence in chronic hepatitis B patients is of great benefit for individual treatment and prognosis. Jin et al. established different HCC prediction models by combining high-throughput radiomics features using DL radiomics with serological and clinical information (64). Finally, the model including SWE, BMUS radiomics features, sex and age showed the best prediction performance (AUCs: 0.981, 0.942 and 0.900 in training, validation and testing cohorts for predicting 5-year prognosis of HCC).
The prediction of malignant subtype and clinical prognosis are also important for the decision-making and treatment of HCC patients. Yao et al. have demonstrated the value of the radiomics analysis system based on multi-modal ultrasound images (BMUS, SWE and SWV imaging) for benign and malignant classification, malignant subtyping, programmed cell death protein 1 prediction, Ki-67 prediction, and microvascular invasion prediction, with the AUCs ranging from 0.94-0.98 (65). The more detailed performance of AI based on USE for the evaluation of liver diseases is summarized in Table 2.
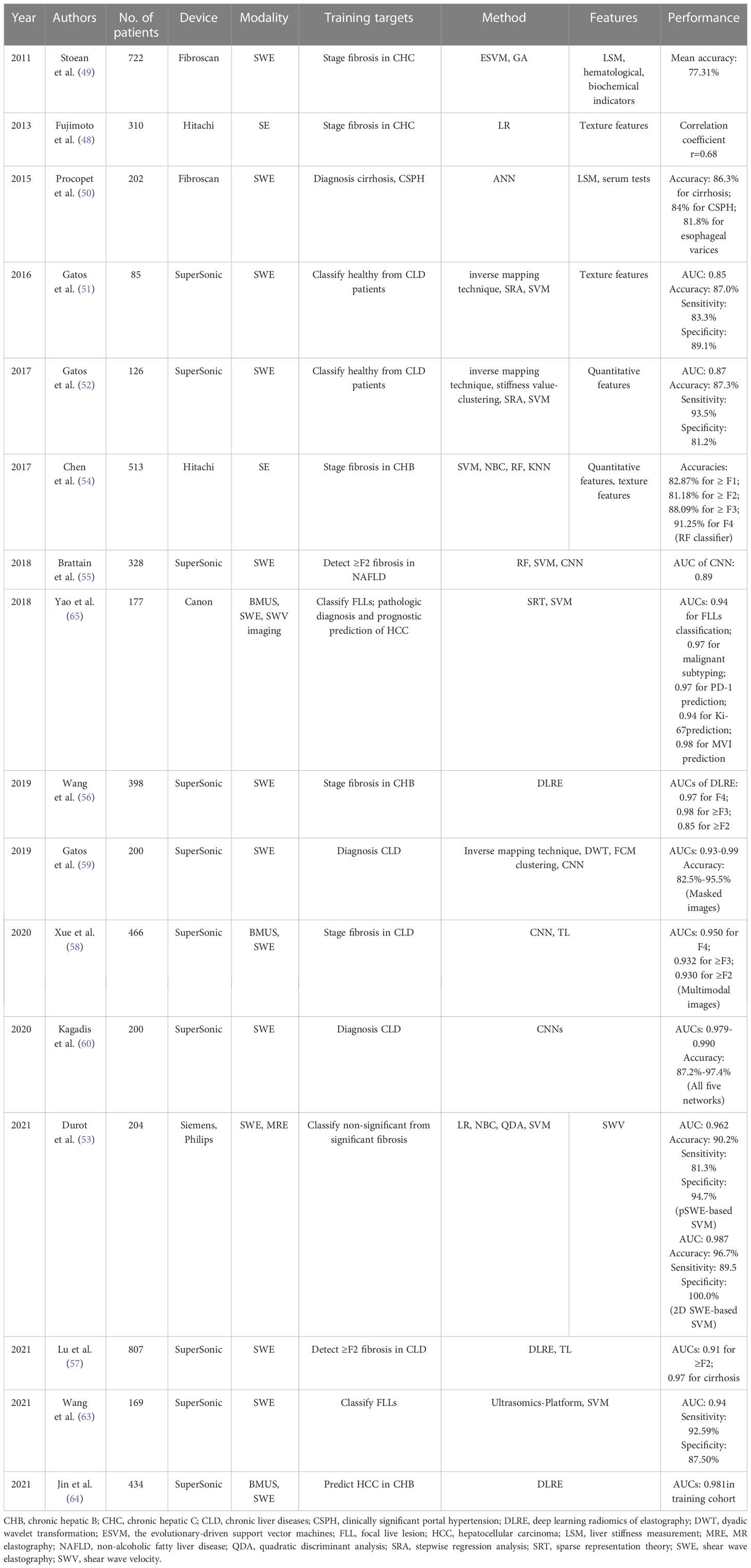
Table 2 The performance summary of artificial intelligence applied to ultrasound elastography in the evaluation of liver diseases.
Breast mass evaluation
Breast cancer is the most common cancer diagnosed in women (66). Accurate breast mass evaluations, including segmentation and detection, differentiation of benign from malignant breast masses, and prediction of axillary lymph node (ALN) status and treatment response, can lead to individualized treatment and favorable prognosis. AI methods applied to USE are expected to provide a more objective and reproducible evaluation for breast masses.
Segmentation and delineation of breast masses
Accurate delineation of breast masses on ultrasound images is an indispensable first step for image interpretation. Manual segmentation of breast masses is labor intensive and time-consuming (67). The various artifacts, uneven intensities and blurred boundaries in USE images have made automated segmentation of breast mass still a tough task (68). Sergiu and coworkers developed a probabilistic model for every pixel derived by a video sequence, followed by a Deterministic Annealing Expectation Maximization method used for automatic image segmentation of breast elastographic images (69). Only an error of 5% on the phantom test images was provided.
Classification and diagnosis of breast masses
Early detection and treatment of breast cancer can significantly decrease mortality. Therefore, the differential diagnosis of benign and malignant breast masses is of great value in breast mass evaluation. Although the American College of Radiology Breast Imaging Reporting and Data System (ACR BI-RADS) can provide a standardized and systemic interpretation of breast ultrasound, the problems of inter- and intra-observer variability can be enormously addressed by AI solutions (70).
Early AI-based USE models for breast mass classification focused on the extraction of discriminant features and the utilization of various classifiers. The elasticity indices, such as SR and quantitative tissue elasticity, as well as texture features have served as the most commonly used handcrafted features (71–77). ANN and SVM classifiers are the most commonly used ML algorithms, all yielding satisfying performance. The fused ultrasound B-mode and elastographic features, have all been proven to generate better results than single-modal imaging (71, 72, 75, 77–83). It is also proved that the elasticity features along the rim surrounding the lesion is valuable for the classification of breast masses (84).
However, it is difficult to extract human-crafted features from USE images since they often contain irrelevant patterns (28), and the classification performance is greatly influenced by the selection of particular features. Recently, DL algorithms, especially CNNs, have made great strides in establishing AI-based USE models for breast mass classification (85–88). Zhang et al. developed a two-layer DL architecture based on SWE, comprising a pointwise gated Boltzmann machine and a restricted Boltzmann machine (85). The pointwise gated Boltzmann machine, restricted Boltzmann machine and SVM classifiers were used for automated feature learning, distinct representation learning and classification of breast tumors, respectively. Compared with the handcrafted statistical features, an accuracy of 93.4%, a sensitivity of 88.6%, a specificity of 97.1%, and an AUC of 0.947 were achieved. Fujioka et al. developed a DL model based on SWE images using six CNN architectures with different epochs for breast mass classification (86). The developed DL model reached a mean AUC of 0.870, showing equal or better diagnostic performance compared with the radiologists who analyzed the images using the 5-point visual color assessment and the mean elasticity value. Li et al. found that the CNN-based model using dual modal ultrasound images could provide a more pronounced improvement in diagnostic performance for inexperienced radiologists, with the AUC increasing from 0.794 to 0.830 (87).
Radiomics can provide automated quantification of high-throughput image features. It is expected to reveal disease characteristics that are invisible to the naked eye (42). Generally, the proposed ML-based radiomics models focused on the extraction of low- and high-order features and the utilization of various feature selection algorithms (89–91). The radiomics features involve shape, intensity, texture or wavelet features from different modality ultrasound images, such as SE (89), SWE (90), contrast-enhanced ultrasound (CEUS) (91) and B-mode ultrasound (BMUS) (90, 91). The frequently used feature selection algorithms include hierarchical clustering (89), the least absolute shrinkage and selection operator regression algorithm (90) and the genetic algorithm (91). The selected discriminant features are used alone or in combination with clinical data to train the ML classifiers for breast mass classification. More recently, the potential of using DL radiomics to facilitate the classification of breast masses has also been confirmed, which was expected to identify vast arrays of quantitative features (92, 93). Zhang et al. used a CNN to extract 768 radiomic features from segmented BMUS and SWE images to further build radiomics scores, which was then confirmed to have a better performance than the radiologist assessment using BI-RADS and quantitative SWE features for discriminating benign from malignant breast masses (92). However, this study has the limitation of complicated segmentation tasks. Zhou et al. utilized a CNN for both radiomics feature extraction and breast masses classification, and 4224 low-level and high-abstract features were extracted directly from 540 SWE images that does not need object segmentation (93). The model reached a classification accuracy of 95.8%, a sensitivity of 96.2% and a specificity of 95.7%.
Since TL is an effective strategy to augment accuracy and to reduce training time by transferring the knowledge learned from a source domain to a target domain, Fei et al. proposed a projective model-based multilayer kernel extreme learning machine to transfer parameters (94). The SE-based diagnosis with BMUS imaging used as the source domain generated the best performance, with an accuracy, sensitivity, and specificity of 87.12, 86.06, and 88.15, respectively. Liao et al. applied the VGG-19 network pretrained on the ImageNet dataset based on either single-modal or multi-modal images for breast tumor classification (95). The combination feature model based on BMUS and SE images yielded a correct recognition rate of 92.95% and an AUC of 0.98.
Prediction of ALN status and treatment response
Accurate preoperative evaluation of ALN status in patients with breast tumors is important for surgical decisions and prognosis (96). To analyze ALN status, several AI models have been proposed based on the ultrasound images of ALNs (97) or of primary breast tumors (98, 99). For the differentiation of disease-free axilla and any axillary metastasis in early-stage breast cancer, Our team (98) and Zheng et al. (99) proposed to combine the radiomics features from BMUS and SWE with independent clinical risk factors, all generating satisfactory results. In addition, the DL radiomics model of Zheng et al. could also discriminate between a low and heavy metastatic axillary nodal burden, with an AUC of 0.905 (99).
Knowing how the tumor responds to treatment can be helpful for subsequent treatment selections. Furthermore, it is important to evaluate the pathological complete response (pCR) in breast cancer, since it is related to the rates of long-term survival. Fernandes et al. found that the SR could be predictive of the neoadjuvant chemotherapy response in locally advanced breast cancer (100). A significant difference in tumor stiffness was observed as early as 2 weeks into treatment. By using the preoperative data, the Naïve Bayes classifier achieved a classification of pCR and npCR with a sensitivity of 84%, a specificity of 85%, and AUC of 81%. The detailed results are summarized in Table 3.
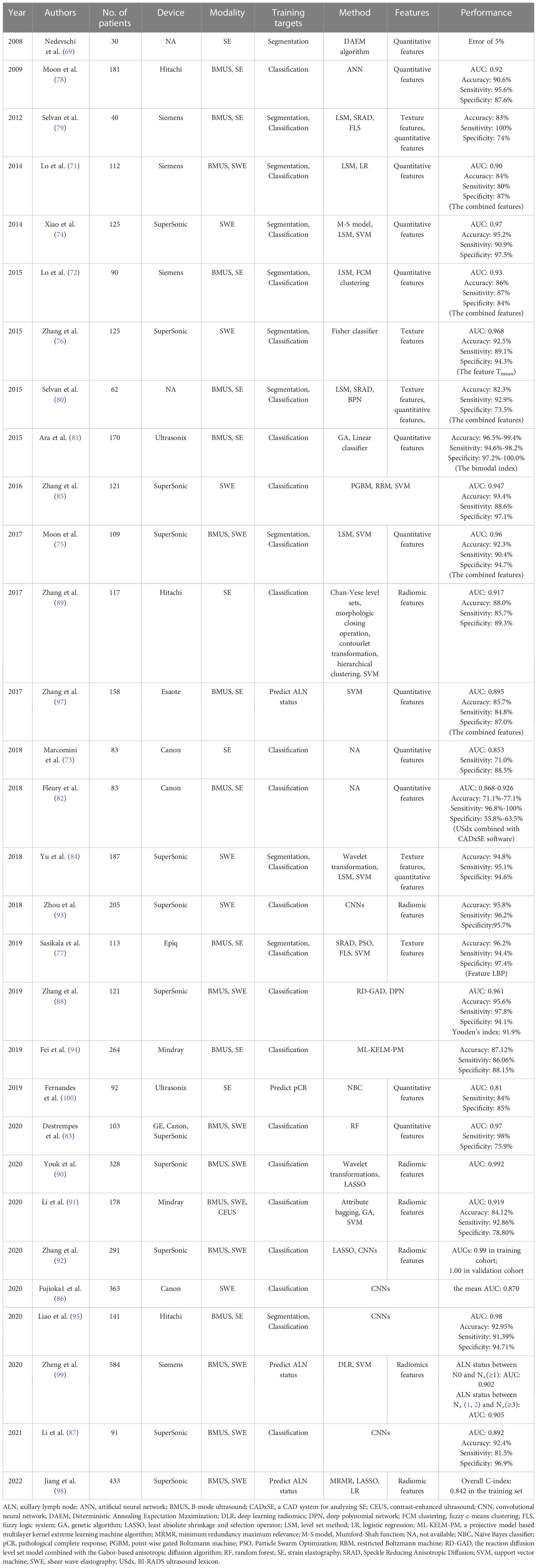
Table 3 The performance summary of artificial intelligence applied to ultrasound elastography in the evaluation of breast masses.
Thyroid nodule evaluation
Thyroid nodular diseases are very common, and the incidence of thyroid cancer has increased worldwide year by year (101). However, only 5%-15% of thyroid nodules are malignant and the majority of thyroid nodules selected for fine-needle aspiration biopsy are benign (102, 103). The current clinical challenge is to discriminate the few clinically significant malignant nodules from the many benign nodules and thus identify patients who warrant surgical excision, hence to decrease medical costs and patient suffering. Moreover, lymph node (LN) metastasis is highly associated with local recurrence, distant metastasis and thyroid cancer staging, which will further guide the surgical plan. Therefore, a credible and noninvasive method is highly desirable for evaluation of thyroid nodules, including classification of thyroid nodules or prediction of the lymph node metastasis.
Segmentation and delineation of thyroid nodules
Segmentation plays an essential role in AI-based USE models, for that malignant thyroid nodules can be accurately diagnosed by using features of well-segmented nodules. However, USE images often have low image quality due to the existing high noise, making automated segmentation a tough task. To segment the thyroid nodules in a noisy environment, Huang et al. proposed a new segmentation method based on the adaptive fast generalized fuzzy clustering algorithm by utilizing the gray level and spatial position information of the original image (104). The proposed method obtained segmentation accuracies of 0.9981 in Gauss noise (0.03) and 0.9986 in Gauss noise (0.05), indicating that it had a strong ability to suppress noise and obtained more accurate results when clustering images with high noise.
Classification and diagnosis of thyroid nodules
Although the indices such as ES and SE have been introduced, SE is still a quantitative and subjective imaging method. Ding et al. computed a quantitative metric “hard area ratio” by transferring the original color thyroid elastograms from the red–green-blue color space to the hue-saturation-value color space (105). The SVM classifier obtained an accuracy of 93.6% when the hard area ratio and textural feature were used. Two studies used logistic regression analysis to investigate which sonographic features were associated with the malignancy of thyroid nodules and established formulas for predicting whether thyroid nodules were malignant or benign (106, 107).
Whether the ML-based diagnostic pattern can provide a more effective and accurate diagnosis than human experts for thyroid nodule classification still remains unknown. In a large study including 2064 thyroid nodules, Zhang et al. compared the diagnostic performance of nine ML classifiers trained on 11 BMUS features and 1 SE feature with experienced radiologists for thyroid nodule discrimination (108). The RF classifier generated the highest AUC of 0.938, performing better than radiologist diagnosis based on BMUS only (AUC= 0.924 vs. 0.834) and based on both BMUS and SE (AUC= 0.938 vs. 0.843). Recently, both ML-based visual and radiomics are popular methods used to diagnose thyroid nodules, Zhao et al. found that the ML-assisted US visual approach had the best diagnostic capability compared with radiomics approach and American College of Radiology Thyroid Imaging Reporting and Data System (ACR TI-RADS) (AUCs: 0.900 vs. 0.789 vs. 0.689 for the validation dataset, 0.917 vs. 0.770 vs. 0.681 for the test dataset) (109). When employing the ML-assisted US+SWE visual approach, the unnecessary fine-needle aspiration biopsy rate decreased from 30.0% to 4.5% for the validation dataset and from 37.7% to 4.7% for the test dataset.
Since DL is data-hungry, and the lack of standardized image data may lead to the overfitting problem, the TL strategy has also been applied to a few studies for thyroid nodule classification (110, 111). Qin et al. proposed to transferring feature parameters learned from VGG16, which was pretrained on ImageNet, to ultrasound images and used the hybrid features of BMUS and SE images to build an end-to-end CNN model (110). The proposed AI-based method yielded an accuracy of 0.9470, which was better than other single data-source methods. Pereira et al. compared the performance of conventional feature extraction-based ML approaches, fully trained CNNs, and TL-based pretrained CNNs for the detection of malignant thyroid nodules (111). The results showed that the pretrained network yielded the best classification performance with an accuracy of 0.83, which was better than that of fully trained CNNs. This may be caused by the relatively limited sample size used to train the fully trained network.
Prediction of CLN status
Although papillary thyroid cancer (PTC) is an indolent type of cancer, 20-90% of PTC patients are diagnosed with cervical lymph node metastasis (CLNM) (112), which is highly related to recurrence and a poor survival rate. Accurate CLNM estimations of PTC patients are clinically important. Liu et al. built a radiomics-based model, which extracted 684 radiomic features from both BMUS and SE images to estimate LN metastasis for PTC patients, yielding an AUC of 0.90, which was better than using features extracted from BMUS or SE separately (113). However, it only utilized the radiomics features, with no consideration on other clinical information. Our team developed a radiomics nomogram by incorporating SWE radiomics features as well as clinicopathological risk factors for predicting CLNM in PTC patients, which showed good diagnostic performance in the training set (AUC of 0.851) and the validation set (AUC of 0.832) (114). The detailed performance of AI based on USE for the evaluation of thyroid nodules is summarized in Table 4.
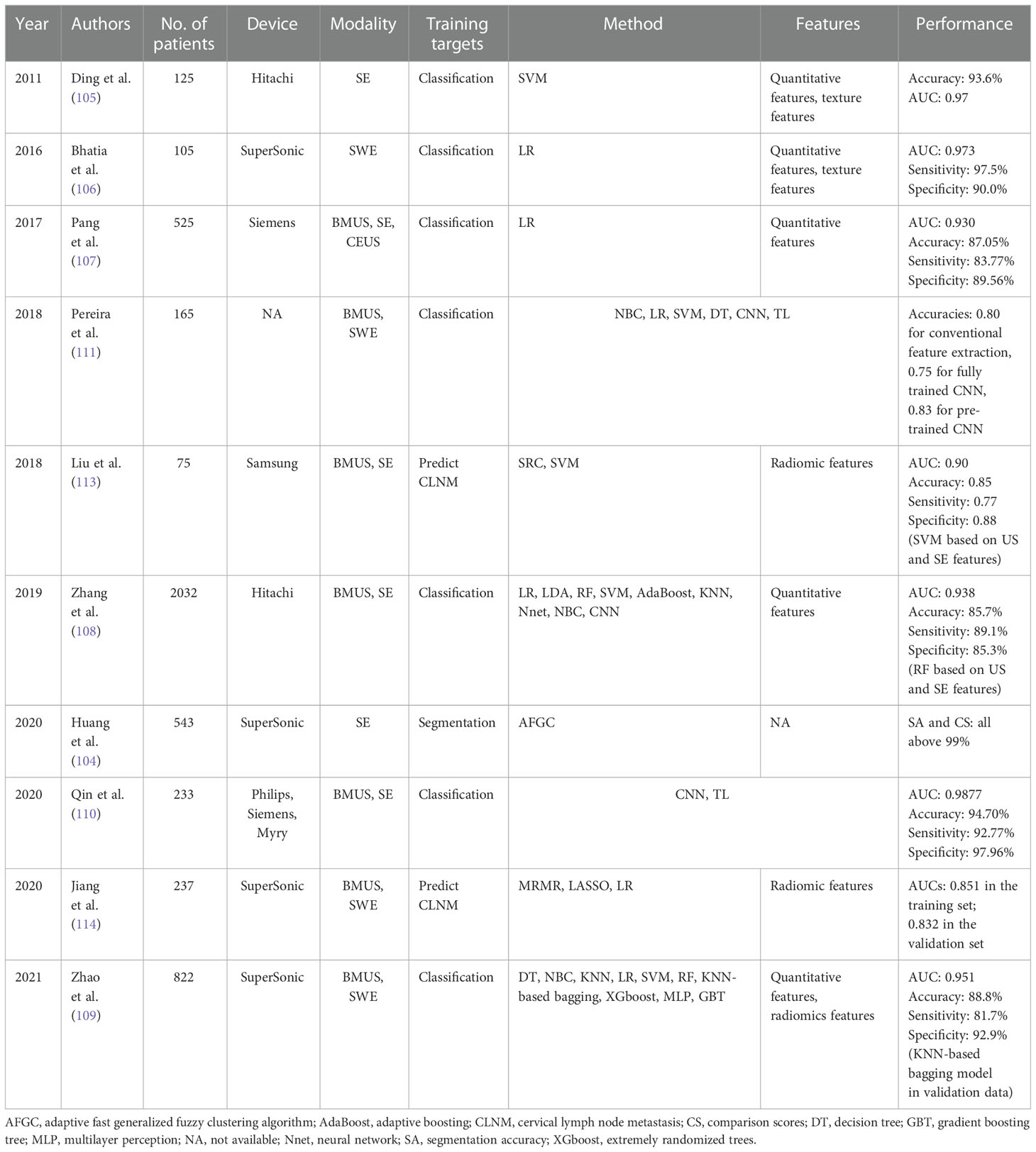
Table 4 The performance summary of artificial intelligence applied to ultrasound elastography in the evaluation of thyroid nodules.
Others
In addition to the applications mentioned above, there are some applications of AI in USE to other organs, such as the diagnosis of prostate cancer (115, 116), the prediction of LN metastasis (117, 118) and tumor deposits (119) in rectal cancer, the prediction of lung mass density lung (120–122), the evaluation of plantar fasciitis (123), as well as the differential diagnosis of brain tumors (124, 125) or the prediction of the overall survival in glioblastomas (126).
Discussion and conclusion
In conclusion, the diagnostic abilities will be extensively enhanced with the AI methods applied to USE images, especially to the multi-modal images, including ultrasound imaging methods (BMUS, USE and CEUS) and other medical imaging techniques (MRI and CT). Although the available studies have all revealed that the models based on multimodal images had superior performance to those based on single modality, the actual selection in the model development depends on the availability of datasets. One the one hand, an additional imaging modality can help provide more effective and comprehensive information. If the multi-modal imaging data is available and acquired standardized, USE data in combination with BMUS or other modalities is expected to improve the diagnostic accuracy. On the other hand, the model based on unimodal images yielded acceptable performance and the standardized and curated unimodal images are usually more easily-obtained. AI models based on them are also easier to use and generalize in the clinic, especially in primary hospital. Thus, higher accuracy should not be the only factor taken into account when selecting a unimodal or multimodal prediction model; the model’s applicability at various institutions should also be considered.
Overall, according to the available studies, AI technology is a powerful tool to assist different clinical tasks of different diseases with a comparable consistency. Although the diagnostic performance varies in different diseases, AI methods applied to USE imaging demonstrated remarkable capability for the differentiation of malignant or benign breast masses, focal liver lesions and thyroid nodules, the staging of liver fibrosis and the prediction of lymph node metastasis and so on. The performance of many applications has been shown to be comparable or even better than that of experienced radiologists. This might be facilitated by the increasing availability of curated datasets and the optimized AI architectures. However, further validations with extensive datasets are still needed to affirm the performance. In addition, the nonuniform acquisition methods and variability of ultrasound data are major challenges that restrict the comparison and generalization of different methods in different tasks. The construction of standard databases for different ultrasound applications is a future direction in further studies.
In general, early ML-based USE models have an emphasis on the extraction of discriminant features from the USE images alone or the combined BMUS and USE images, with texture features and elasticity indices mostly utilized. Then, these features will be input into classical ML classifiers, such as SVM, RF or ANN etc., for a more accurate and effective diagnosis. Since feature calculations and image segmentation are not required and hierarchical features can be automatically learned, some DL architectures, including CNNs and other popular DL architectures based on different training strategy such as GoogleNet, AlexNet, VGG, ResNet and DenseNet, are also increasingly being applied to mitigate the limitations of traditional ML processes. Furthermore, dozens of notable radiomics studies have been enabled since radiomics allows quantitative extraction of high-throughput features from medical images that are not directly visible to the naked eyes, and then ML methods are applied to build classification or prediction models based on the radiomics features alone or the incorporation of disease-correlated clinical information.
However, there are still some challenges to generalizing AI methods applied to USE in the clinical practice. The lack of large curated data has been considered as the one of the main challenges. Given that DL algorithms are “data hungry”, large-scale multicenter studies with well-annotated datasets are needed to further determine the diagnostic values of AI in USE imaging. The lack of a dataset will increase the risk of overfitting, which will occur when a model has too many parameters and remembers the training data but cannot be generalized to new independent data. One common solution is the data augmentation method. Data can be artificially augmented by random transformations, including flipping, rotation, translation and zooming (13). Another common strategy is the TL, which can transfer the parameters learned from one dataset to another target dataset. Recent advances of novel DL architectures, including the unsupervised learning, Generative Adversarial Networks (GANs) (127) for example, and federated learning (128) have shown great promise to circumvent the obstacle of scarce data. GANs, consisting of one generator and one classifier, can help generate synthetic medical images to train deeper architectures. GANs have been shown to be very effective at medical image synthesis between magnetic resonance imaging (MRI) and computed tomography (CT) images (129, 130). Federated learning enables multiple parties to collaboratively construct a ML model based on datasets that are distributed across multiple devices while keeping their private training data private. It can be useful to help address the problems related to privacy and ethical when patients’ data are sharing among different centers. It’s also worth mentioning the Graph Neural Networks (GNNs), which concentrates on learning the data represented in the form of graphs (131). GNNs have been applied to several computer vision tasks, including few-shot image classification (132) and image segmentation (133). Nevertheless, these advancements have not yet been explored in USE imaging and are likely to be promising approaches. The non-interpretability of DL approaches is another challenge. It is also known as the “black box”, which means that it is difficult for radiologists to explain the results given by DL architectures. Since there is a lack of understanding of the relationship between the input and output, identifying the features actually used for interpretation seems to be impossible, and radiologists may not accept the conclusions derived from such an AI architecture. The advance of heatmap may help to address this problem.
In addition to the above impediments, USE presents unique challenges. First, irrelevant patterns such as noises, artifacts and regions lacking USE information can often be detected, which will increase the difficulty for manual or automatic feature extraction. The image quality of USE still needs to be improved. Secondly, because ultrasound is often used as a first-line imaging modality, there is often an imbalance with an excess of normal or healthy images than abnormal or unhealthy ones, which will reduce the diagnostic accuracy of AI models. In addition, the generalizability of the developed AI models is another challenge. Most datasets are generated from a single device type and a single collection center, and most of the present AI has concentrated on single task within an overall system, such segmentation or classification, all limiting the generalizability of the developed AI models.
AI is unlikely to replace radiologists in the near or far future, owing to the complexity of creating and training an AI architecture. What is imperative is that radiologists should understand the basic working principles of AI and better apply it to medical image interpretation and analysis. Although a second opinion can be provided by AI based on USE models using ML or DL techniques, the final diagnosis decision should be made by the radiologists.
Author contributions
All authors helped in writing and revising the manuscript and drafting the figures and tables. All authors contributed to the article and approved the submitted version.
Funding
This work was supported by the National Natural Science Foundation of China [grant numbers 82071953] of X-WC, who have helped to design the study and decide to submit the article for publication.
Conflict of interest
All authors have completed the ICMJE uniform disclosure form.
The authors declare that the research was conducted in the absence of any commercial or financial relationships that could be construed as a potential conflict of interest.
Publisher’s note
All claims expressed in this article are solely those of the authors and do not necessarily represent those of their affiliated organizations, or those of the publisher, the editors and the reviewers. Any product that may be evaluated in this article, or claim that may be made by its manufacturer, is not guaranteed or endorsed by the publisher.
Glossary
References
1. Ozturk A, Grajo JR, Dhyani M, Anthony BW. Principles of ultrasound and elastography. Physiol Behav (2016) 176:139–48. doi: 10.1007/s00261-018-1475-6
2. Park AY, Son EJ, Han K, Youk JH, Kim JA, Park CS. Shear wave elastography of thyroid nodules for the prediction of malignancy in a large scale study. Eur J Radiol (2015) 84:407–12. doi: 10.1016/j.ejrad.2014.11.019
3. Fujioka T, Mori M, Kubota K, Kikuchi Y, Katsuta L, Kasahara M, et al. Simultaneous comparison between strain and shear wave elastography of breast masses for the differentiation of benign and malignant lesions by qualitative and quantitative assessments. Breast Cancer (2019) 26:792–8. doi: 10.1007/s12282-019-00985-0
4. Cosgrove D, Barr R, Bojunga J, Cantisani V, Chammas MC, Dighe M, et al. WFUMB guidelines and recommendations on the clinical use of ultrasound elastography: part 4. Thyroid Ultrasound Med Biol (2017) 43:4–26. doi: 10.1016/j.ultrasmedbio.2016.06.022
5. Barr RG, Ferraioli G, Palmeri ML, Goodman ZD, Garcia-Tsao G, Rubin J, et al. Elastography assessment of liver fibrosis: society of radiologists in ultrasound consensus conference statement. Radiology (2015) 276:846–61. doi: 10.1148/radiol.2015150619
6. Barr RG, Cosgrove D, Brock M, Cantisani V, Correas JM, Postema AW, et al. WFUMB guidelines and recommendations on the clinical use of ultrasound elastography: part 5. Prostate. Ultrasound Med Biol (2017) 43:27–48. doi: 10.1016/j.ultrasmedbio.2016.06.020
7. Gao J, Jiang Q, Zhou B, Chen D. Convolutional neural networks for computer-aided detection or diagnosis in medical image analysis: an overview. Math Biosci Eng (2019) 16:6536–61. doi: 10.3934/mbe.2019326
8. Milas M, Mandel SJ, Langer JE. Elastography: applications and limitations of a new technology. Adv Thyroid Parathyr Ultrasound (2017), 67–73. doi: 10.1007/978-3-319-44100-9_8
9. Bhatia KSS, Tong CSL, Cho CCM, Yuen EHY, Lee YYP, Ahuja AT. Shear wave elastography of thyroid nodules in routine clinical practice: preliminary observations and utility for detecting malignancy. Eur Radiol (2012) 22:2397–406. doi: 10.1007/s00330-012-2495-1
10. Yoon D. What we need to prepare for the fourth industrial revolution. Healthc Inform Res (2017) 23:75–6. doi: 10.4258/hir.2017.23.2.75
11. Kohli M, Prevedello LM, Filice RW, Geis JR. Implementing machine learning in radiology practice and research. Am J Roentgenol (2017) 208:754–60. doi: 10.2214/AJR.16.17224
12. Giger ML. Machine learning in medical imaging. J Am Coll Radiol (2018) 15:512–20. doi: 10.1016/j.jacr.2017.12.028
13. Chartrand G, Cheng PM, Vorontsov E, Drozdzal M, Turcotte S, Pal CJ, et al. Deep learning: a primer for radiologists. Radiographics (2017) 37:2113–31. doi: 10.1148/rg.2017170077
14. Shen YT, Chen L, Yue WW, Xu HX. Artificial intelligence in ultrasound. Eur J Radiol (2021) 139:109717. doi: 10.1016/j.ejrad.2021.109717
15. Nguyen DT, Kang JK, Pham TD, Batchuluun G, Park KR. Ultrasound image-based diagnosis of malignant thyroid nodule using artificial intelligence. Sensors (Switzerland) (2020) 20:1822. doi: 10.3390/s20071822
16. Qi X, Zhang L, Chen Y, Pi Y, Chen Y, Lv Q, et al. Automated diagnosis of breast ultrasonography images using deep neural networks. Med Image Anal (2019) 52:185–98. doi: 10.1016/j.media.2018.12.006
17. Lee JH, Joo I, Kang TW, Paik YH, Sinn DH, Ha SY, et al. Deep learning with ultrasonography: automated classification of liver fibrosis using a deep convolutional neural network. Eur Radiol (2020) 30:1264–73. doi: 10.1007/s00330-019-06407-1
18. Li H, Bhatt M, Qu Z, Zhang S, Hartel MC, Khademhosseini A, et al. Deep learning in ultrasound elastography imaging: a review. Med Phys (2022) 49:5993–6018. doi: 10.1002/mp.15856
19. Mao YJ, Lim HJ, Ni M, Yan WH, Wong DWC, Cheung JCW. Breast tumour classification using ultrasound elastography with machine learning: a systematic scoping review. Cancers (Basel) (2022) 14:1–18. doi: 10.3390/cancers14020367
20. Ophir J, Céspedes I, Ponnekanti H, Yazdi Y, li X. Elastography: a quantitative method for imaging the elasticity of biological tissues. Ultrason Imaging (1991) 13:111–34. doi: 10.1177/016173469101300201
21. Sigrist RMS, Liau J, El KA, MC C, Willmann JK. Ultrasound elastography: review of techniques and clinical applications. Theranostics (2017) 7:1303–29. doi: 10.7150/thno.18650
22. Garra BS. Elastography: history, principles, and technique comparison. Abdom Imaging (2015) 40:680–97. doi: 10.1007/s00261-014-0305-8
23. Choy G, Khalilzadeh O, Michalski M, Do S, Samir AE, Pianykh OS, et al. Current applications and future impact of machine learning in radiology. Radiology (2018) 288:318–28. doi: 10.1148/radiol.2018171820
24. Jordan MI, Mitchell TM. Machine learning: trends, perspectives, and prospects. Sci (80- ) (2015) 349:255–60. doi: 10.1126/science.aaa8415
25. Wang S, Summers RM. Machine learning and radiology. Med Image Anal (2012) 16:933–51. doi: 10.1016/j.media.2012.02.005
26. Jain AK, Murty MN, Flynn PJ. Data clustering: a review. ACM Comput Surv (1999) 31:264–323. doi: 10.1145/331499.331504
27. Le EPV, Wang Y, Huang Y, Hickman S, Gilbert FJ. Artificial intelligence in breast imaging. Clin Radiol (2019) 74:357–66. doi: 10.1016/j.crad.2019.02.006
28. Brattain LJ, Telfer BA, Dhyani M, Grajo JR, Samir AE. Machine learning for medical ultrasound: status, methods, and future opportunities. Abdom Radiol (2018) 43:786–99. doi: 10.1007/s00261-018-1517-0
30. Abdelhafiz D, Bi J, Ammar R, Yang C, Nabavi S. Convolutional neural network for automated mass segmentation in mammography. BMC Bioinf (2020) 21:1–19. doi: 10.1186/s12859-020-3521-y
31. Kooi T, Litjens G, van Ginneken B, Gubern-Mérida A, Sánchez CI, Mann R, et al. Large Scale deep learning for computer aided detection of mammographic lesions. Med Image Anal (2017) 35:303–12. doi: 10.1016/j.media.2016.07.007
32. Khan AI, Shah JL, Bhat M. CoroNet: a deep neural network for detection and diagnosis of covid-19 from chest X-ray images. Comput Methods Programs BioMed (2020) 196:105581. doi: 10.1016/j.cmpb.2020.105581
33. Choi B-K, Madusanka N, Choi H-K, So J-H, Kim C-H, Park H-G, et al. Convolutional neural network-based MR image analysis for alzheimer’s disease classification. Curr Med Imaging Rev (2019) 16:27–35. doi: 10.2174/1573405615666191021123854
34. Yasaka K, Akai H, Kunimatsu A, Kiryu S, Abe O. Deep learning with convolutional neural network in radiology. Jpn J Radiol (2018) 36:257–72. doi: 10.1007/s11604-018-0726-3
35. Erickson BJ, Korfiatis P, Kline TL, Akkus Z, Philbrick K, Weston AD. Deep learning in radiology: does one size fit all? J Am Coll Radiol (2018) 15:521–6. doi: 10.1016/j.jacr.2017.12.027
36. Szegedy C, Liu W, Jia Y, Sermanet P, Reed S, Anguelov D, et al. Going deeper with convolutions, in: 2015 IEEE Conference on Computer Vision and Pattern Recognition (CVPR) (2015). Boston, MA, USA: IEEE p. 1–9. doi: 10.1109/CVPR.2015.7298594
37. Krizhevsky BA, Sutskever I, Hinton GE. ImageNet classification with deep convolutional neural networks. Commun ACM (2017) 60:84–90. doi: 10.1145/3065386
38. Simonyan K, Zisserman A. Very deep convolutional networks for large-scale image recognition. Computer Science (2014). doi: 10.48550/arXiv.1409.1556
39. He K, Zhang X, Ren S, Sun J. Deep residual learning for image recognition. 2016 IEEE Conference on Computer Vision and Pattern Recognition (CVPR) (2016). Las Vegas, NV, USA: IEEE. p. 770–8. doi: 10.1109/CVPR.2016.90
40. Bengio Y, Courville A, Vincent P. Representation learning: a review and new perspectives. IEEE Trans Pattern Anal Mach Intell (2013) 35:1798–828. doi: 10.1109/TPAMI.2013.50
41. Lambin P, Leijenaar RTH, Deist TM, Peerlings J, De Jong EEC, Van Timmeren J, et al. Radiomics: the bridge between medical imaging and personalized medicine. Nat Rev Clin Oncol (2017) 14:749–62. doi: 10.1038/nrclinonc.2017.141
42. Gillies RJ, Kinahan PE, Hricak H. Radiomics: images are more than pictures, they are data. Radiology (2016) 278:563–77. doi: 10.1148/radiol.2015151169
43. Gillies RJ, Schabath MB. Radiomics improves cancer screening and early detection. Cancer Epidemiol Biomarkers Prev (2020) 29:2556–67. doi: 10.1158/1055-9965.EPI-20-0075
44. Bi Q, Goodman KE, Kaminsky J, Lessler J. What is machine learning? a primer for the epidemiologist. Am J Epidemiol (2019) 188:2222–39. doi: 10.1093/aje/kwz189
45. Jalalian A, Mashohor SBT, Mahmud HR, Saripan MIB, Ramli ARB, Karasfi B. Computer-aided detection/diagnosis of breast cancer in mammography and ultrasound: a review. Clin Imaging (2013) 37:420–6. doi: 10.1016/j.clinimag.2012.09.024
46. Altekruse SF, McGlynn KA, Reichman ME. Hepatocellular carcinoma incidence, mortality, and survival trends in the united states from 1975 to 2005. J Clin Oncol (2009) 27:1485–91. doi: 10.1200/JCO.2008.20.7753
47. Bravo A, Sheth S, Chopra S. Current concepts: liver biopsy. N Engl J Med (2001) 344:495–500. doi: 10.1056/NEJM200102153440706
48. Fujimoto K, Kato M, Kudo M, Yada N, Shiina T, Ueshima K, et al. Novel image analysis method using ultrasound elastography for noninvasive evaluation of hepatic fibrosis in patients with chronic hepatitis c. Oncology (2013) 84 Suppl 1:3–12. doi: 10.1159/000345883
49. Stoean R, Stoean C, Lupsor M, Stefanescu H, Badea R. Evolutionary-driven support vector machines for determining the degree of liver fibrosis in chronic hepatitis c. Artif Intell Med (2011) 51:53–65. doi: 10.1016/j.artmed.2010.06.002
50. Procopet B, Cristea VM, Robic MA, Grigorescu M, Agachi PS, Metivier S, et al. Serum tests, liver stiffness and artificial neural networks for diagnosing cirrhosis and portal hypertension. Dig Liver Dis (2015) 47:411–6. doi: 10.1016/j.dld.2015.02.001
51. Gatos I, Tsantis S, Spiliopoulos S, Karnabatidis D, Theotokas I, Zoumpoulis P, et al. A new computer aided diagnosis system for evaluation of chronic liver disease with ultrasound shear wave elastography imaging. Med Phys (2016) 43:1428–36. doi: 10.1118/1.4942383
52. Gatos I, Tsantis S, Spiliopoulos S, Karnabatidis D, Theotokas I, Zoumpoulis P, et al. A machine-learning algorithm toward color analysis for chronic liver disease classification, employing ultrasound shear wave elastography. Ultrasound Med Biol (2017) 43:1797–810. doi: 10.1016/j.ultrasmedbio.2017.05.002
53. Durot I, Akhbardeh A, Sagreiya H, Loening AM, Rubin DL. A new multi-model machine learning framework to improve hepatic fibrosis grading using ultrasound elastography systems from different vendors. Ultrasound Med Biol (2021) 46:26–33. doi: 10.1016/j.ultrasmedbio.2019.09.004.A
54. Chen Y, Luo Y, Huang W, Hu D, Zheng RQ, Cong SZ, et al. Machine-learning-based classification of real-time tissue elastography for hepatic fibrosis in patients with chronic hepatitis b. Comput Biol Med (2017) 89:18–23. doi: 10.1016/j.compbiomed.2017.07.012
55. Brattain LJ, Telfer BA, Dhyani M, Grajo JR, Samir AE. Objective liver fibrosis estimation from shear wave elastography. Annu Int Conf IEEE Eng Med Biol Soc (2018) 2018:1–5. doi: 10.1109/EMBC.2018.8513011
56. Wang K, Lu X, Zhou H, Gao Y, Zheng J, Tong M, et al. Deep learning radiomics of shear wave elastography significantly improved diagnostic performance for assessing liver fibrosis in chronic hepatitis b: a prospective multicentre study. Gut (2019) 68:729–41. doi: 10.1136/gutjnl-2018-316204
57. Lu X, Zhou H, Wang K, Jin J, Meng F, Mu X, et al. Comparing radiomics models with different inputs for accurate diagnosis of significant fibrosis in chronic liver disease. Eur Radiol (2021) 31:8743–54. doi: 10.1007/s00330-021-07934-6
58. Xue L-Y, Jiang Z-Y, Fu T-T, Wang Q-M, Zhu Y-L, Dai M, et al. Transfer learning radiomics based on multimodal ultrasound imaging for staging liver fibrosis. Eur Radiol (2020) 30:2973–83. doi: 10.1007/s00330-019-06595-w
59. Gatos I, Tsantis S, Spiliopoulos S, Karnabatidis D, Theotokas I, Zoumpoulis P, et al. Temporal stability assessment in shear wave elasticity images validated by deep learning neural network for chronic liver disease fibrosis stage assessment. Med Phys (2019) 46:2298–309. doi: 10.1002/mp.13521
60. Kagadis GC, Drazinos P, Gatos I, Tsantis S, Papadimitroulas P, Spiliopoulos S, et al. Deep learning networks on chronic liver disease assessment with fine-tuning of shear wave elastography image sequences. Phys Med Biol (2020) 65:215027. doi: 10.1088/1361-6560/abae06
61. Tian WS, Lin MX, Zhou LY, Pan FS, Huang GL, Wang W, et al. Maximum value measured by 2-d shear wave elastography helps in differentiating malignancy from benign focal liver lesions. Ultrasound Med Biol (2016) 42:2156–66. doi: 10.1016/j.ultrasmedbio.2016.05.002
62. Ronot M, Di Renzo S, Gregoli B, Duran R, Castera L, Van Beers BE, et al. Characterization of fortuitously discovered focal liver lesions: additional information provided by shearwave elastography. Eur Radiol (2015) 25:346–58. doi: 10.1007/s00330-014-3370-z
63. Wang W, Zhang JC, Tian WS, Chen LD, Zheng Q, Hu HT, et al. Shear wave elastography-based ultrasomics: differentiating malignant from benign focal liver lesions. Abdom Radiol (2021) 46:237–48. doi: 10.1007/s00261-020-02614-3
64. Jin J, Yao Z, Zhang T, Zeng J, Wu L, Wu M, et al. Deep learning radiomics model accurately predicts hepatocellular carcinoma occurrence in chronic hepatitis b patients: a five-year follow-up. Am J Cancer Res (2021) 11:576–89.
65. Yao Z, Dong Y, Wu G, Zhang Q, Yang D, Yu JH, et al. Preoperative diagnosis and prediction of hepatocellular carcinoma: radiomics analysis based on multi-modal ultrasound images. BMC Cancer (2018) 18:1–11. doi: 10.1186/s12885-018-5003-4
66. Siegel RL, Miller KD, Fuchs HE, Jemal A. Cancer statistics, 2021. CA Cancer J Clin (2021) 71:7–33. doi: 10.3322/caac.21654
67. Xu Y, Wang Y, Yuan J, Cheng Q, Wang X, Carson PL. Medical breast ultrasound image segmentation by machine learning. Ultrasonics (2019) 91:1–9. doi: 10.1016/j.ultras.2018.07.006
68. Guo Y, Duan X, Wang C, Guo H. Segmentation and recognition of breast ultrasound images based on an expanded U-net. PloS One (2021) 16:e0253202. doi: 10.1371/journal.pone.0253202
69. Nedevschi S, Pantilie C, Mariţa T, Dudea S. Statistical methods for automatic segmentation of elastographic images. 2008 4th International Conference on Intelligent Computer Communication and Processing (2008). Cluj-Napoca: IEEE. p. 287–90. doi: 10.1109/ICCP.2008.4648388
70. Kim J, Kim HJ, Kim C, Kim WH. Artificial intelligence in breast ultrasonography. Ultrasonography (2021) 40:183–90. doi: 10.14366/usg.20117
71. Lo CM, Chen YP, Chang YC, Lo C, Huang CS, Chang RF. Computer-aided strain evaluation for acoustic radiation force impulse imaging of breast masses. Ultrason Imaging (2014) 36:151–66. doi: 10.1177/0161734613520599
72. Lo CM, Chang YC, Yang YW, Huang CS, Chang RF. Quantitative breast mass classification based on the integration of b-mode features and strain features in elastography. Comput Biol Med (2015) 64:91–100. doi: 10.1016/j.compbiomed.2015.06.013
73. Marcomini KD, Fleury EFC, Oliveira VM, Carneiro AAO, Schiabel H, Nishikawa RM. Evaluation of a computer-aided diagnosis system in the classification of lesions in breast strain elastography imaging. Bioengineering (2018) 5:62. doi: 10.3390/bioengineering5030062
74. Xiao Y, Zeng J, Niu L, Zeng Q, Wu T, Wang C, et al. Computer-aided diagnosis based on quantitative elastographic features with supersonic shear wave imaging. Ultrasound Med Biol (2014) 40:275–86. doi: 10.1016/j.ultrasmedbio.2013.09.032
75. Moon WK, Huang YS, Lee YW, Chang SC, Lo CM, Yang MC, et al. Computer-aided tumor diagnosis using shear wave breast elastography. Ultrasonics (2017) 78:125–33. doi: 10.1016/j.ultras.2017.03.010
76. Zhang Q, Xiao Y, Chen S, Wang C, Zheng H. Quantification of elastic heterogeneity using contourlet-based texture analysis in shear-wave elastography for breast tumor classification. Ultrasound Med Biol (2015) 41:588–600. doi: 10.1016/j.ultrasmedbio.2014.09.003
77. Sasikala S, Bharathi M, Ezhilarasi M, Senthil S. Reddy MR. particle swarm optimization based fusion of ultrasound echographic and elastographic texture features for improved breast cancer detection. Australas Phys Eng Sci Med (2019) 42:677–88. doi: 10.1007/s13246-019-00765-2
78. Moon WK, Huang CS, Shen WC, Takada E, Chang RF, Joe J, et al. Analysis of elastographic and b-mode features at sonoelastography for breast tumor classification. Ultrasound Med Biol (2009) 35:1794–802. doi: 10.1016/j.ultrasmedbio.2009.06.1094
79. Selvan S, Kavitha M, Devi SS, Suresh S. Fuzzy-based classification of breast lesions using ultrasound echography and elastography. Ultrasound Q (2012) 28:159–67. doi: 10.1097/RUQ.0b013e318262594a
80. Selvan S, Shenbagadevi S, Suresh S. “Computer-Aided Diagnosis of Breast Elastography and B-Mode Ultrasound.,” In: Suresh LP, Dash SS, Panigrahi BK, editors. Artificial Intelligence and Evolutionary Algorithms in Engineering Systems. Advances in Intelligent Systems and Computing (2015). New Delhi: Springer India. p. 213–23. doi: 10.1007/978-81-322-2135-7_24
81. Ara SR, Alam F, Rahman MH, Akhter S, Awwal R, Hasan MK. Bimodal multiparameter-based approach for benign-malignant classification of breast tumors. Ultrasound Med Biol (2015) 41:2022–38. doi: 10.1016/j.ultrasmedbio.2015.01.023
82. Fleury EFC, Gianini AC, Marcomini K, Oliveira V. The feasibility of classifying breast masses using a computer-assisted diagnosis (CAD) system based on ultrasound elastography and BI-RADS lexicon. Technol Cancer Res Treat (2018) 17:1533033818763461. doi: 10.1177/1533033818763461
83. Destrempes F, Trop I, Allard L, Chayer B, Garcia-Duitama J, El Khoury M, et al. Added value of quantitative ultrasound and machine learning in BI-RADS 4–5 assessment of solid breast lesions. Ultrasound Med Biol (2020) 46:436–44. doi: 10.1016/j.ultrasmedbio.2019.10.024
84. Yu Y, Xiao Y, Cheng J, Chiu B. Breast lesion classification based on supersonic shear-wave elastography and automated lesion segmentation from b-mode ultrasound images. Comput Biol Med (2018) 93:31–46. doi: 10.1016/j.compbiomed.2017.12.006
85. Zhang Q, Xiao Y, Dai W, Suo J, Wang C, Shi J, et al. Deep learning based classification of breast tumors with shear-wave elastography. Ultrasonics (2016) 72:150–7. doi: 10.1016/j.ultras.2016.08.004
86. Fujioka T, Katsuta L, Kubota K, Mori M, Kikuchi Y, Kato A, et al. Classification of breast masses on ultrasound shear wave elastography using convolutional neural networks. Ultrason Imaging (2020) 42:213–20. doi: 10.1177/0161734620932609
87. Li C, Li J, Tan T, Chen K, Xu Y, Wu R. Application of ultrasonic dual-mode artificially intelligent architecture in assisting radiologists with different diagnostic levels on breast masses classification. Diagn Interv Radiol (2021) 27:315–22. doi: 10.5152/DIR.2021.20018
88. Zhang Q, Song S, Xiao Y, Chen S, Shi J, Zheng H. Dual-mode artificially-intelligent diagnosis of breast tumours in shear-wave elastography and b-mode ultrasound using deep polynomial networks. Med Eng Phys (2019) 64:1–6. doi: 10.1016/j.medengphy.2018.12.005
89. Zhang Q, Xiao Y, Suo J, Shi J, Yu J, Guo Y, et al. Sonoelastomics for breast tumor classification: a radiomics approach with clustering-based feature selection on sonoelastography. Ultrasound Med Biol (2017) 43:1058–69. doi: 10.1016/j.ultrasmedbio.2016.12.016
90. Youk JH, Kwak JY, Lee E, Son EJ, Kim J-A. Grayscale ultrasound radiomic features and shear-wave elastography radiomic features in benign and malignant breast masses. Ultraschall der Med (2020) 41:390–6. doi: 10.1055/a-0917-6825
91. Li Y, Liu Y, Zhang M, Zhang G, Wang Z, Luo J. Radiomics with attribute bagging for breast tumor classification using multimodal ultrasound images. J Ultrasound Med (2020) 39:361–71. doi: 10.1002/jum.15115
92. Zhang X, Liang M, Yang Z, Zheng C, Wu J, Ou B, et al. Deep learning-based radiomics of b-mode ultrasonography and shear-wave elastography: improved performance in breast mass classification. Front Oncol (2020) 10:1621. doi: 10.3389/fonc.2020.01621
93. Zhou Y, Xu J, Liu Q, Li C, Liu Z, Wang M, et al. A radiomics approach with CNN for shear-wave elastography breast tumor classification. IEEE Trans BioMed Eng (2018) 65:1935–42. doi: 10.1109/TBME.2018.2844188
94. Fei X, Zhou W, Shen L, Chang C, Zhou S, Shi J. Ultrasound-based diagnosis of breast tumor with parameter transfer multilayer kernel extreme learning machine. IEEE engineering in medicine and biology society conference proceedings (2019). Berlin, GERMANY: IEEE. p. 933–6. doi: 10.1109/EMBC.2019.8857280
95. Liao WX, He P, Hao J, Wang XY, Yang RL, An D, et al. Automatic identification of breast ultrasound image based on supervised block-based region segmentation algorithm and features combination migration deep learning model. IEEE J BioMed Heal Inf (2020) 24:984–93. doi: 10.1109/JBHI.2019.2960821
96. Ahmed M, Purushotham AD, Douek M. Novel techniques for sentinel lymph node biopsy in breast cancer: a systematic review. Lancet Oncol (2014) 15:e351–62. doi: 10.1016/S1470-2045(13)70590-4
97. Zhang Q, Suo J, Chang W, Shi J, Chen M. Dual-modal computer-assisted evaluation of axillary lymph node metastasis in breast cancer patients on both real-time elastography and b-mode ultrasound. Eur J Radiol (2017) 95:66–74. doi: 10.1016/j.ejrad.2017.07.027
98. Jiang M, Li CL, Luo XM, Chuan ZR, Chen RX, Tang SC, et al. Radiomics model based on shear-wave elastography in the assessment of axillary lymph node status in early-stage breast cancer. Eur Radiol (2022) 32:2313–25. doi: 10.1007/s00330-021-08330-w
99. Zheng X, Yao Z, Huang Y, Yu Y, Wang Y, Liu Y, et al. Deep learning radiomics can predict axillary lymph node status in early-stage breast cancer. Nat Commun (2020) 11:1–9. doi: 10.1038/s41467-020-15027-z
100. Fernandes J, Sannachi L, Tran WT, Koven A, Watkins E, Hadizad F, et al. Monitoring breast cancer response to neoadjuvant chemotherapy using ultrasound strain elastography. Transl Oncol (2019) 12:1177–84. doi: 10.1016/j.tranon.2019.05.004
101. Cooper DS, Doherty GM, Haugen BR, Kloos RT, Lee SL, Mandel SJ, et al. Revised American thyroid association management guidelines for patients with thyroid nodules and differentiated thyroid cancer. Thyroid (2009) 19:1167–214. doi: 10.1089/thy.2009.0110
102. Guille JT, Opoku-Boateng A, Thibeault SL, Chen H. Evaluation and management of the pediatric thyroid nodule. Oncologist (2015) 20:19–27. doi: 10.1634/theoncologist.2014-0115
103. Hambly NM, Gonen M, Gerst SR, Li D, Jia X, Mironov S, et al. Implementation of evidence-based guidelines for thyroid nodule biopsy: a model for establishment of practice standards. Am J Roentgenol (2011) 196:655–60. doi: 10.2214/AJR.10.4577
104. Huang W. Segmentation and diagnosis of papillary thyroid carcinomas based on generalized clustering algorithm in ultrasound elastography. J Med Syst (2020) 44:13. doi: 10.1007/s10916-019-1462-7
105. Ding J, Cheng H, Ning C, Huang J, Zhang Y. Quantitative measurement for thyroid cancer characterization based on elastography. J Ultrasound Med (2011) 30:1259–66. doi: 10.7863/jum.2011.30.9.1259
106. Bhatia KSS, Lam ACL, Pang SWA, Wang D, Ahuja AT. Feasibility study of texture analysis using ultrasound shear wave elastography to predict malignancy in thyroid nodules. Ultrasound Med Biol (2016) 42:1671–80. doi: 10.1016/j.ultrasmedbio.2016.01.013
107. Pang T, Huang L, Deng Y, Wang T, Chen S, Gong X, et al. Logistic regression analysis of conventional ultrasonography, strain elastosonography, and contrast-enhanced ultrasound characteristics for the differentiation of benign and malignant thyroid nodules. PloS One (2017) 12:1–11. doi: 10.1371/journal.pone.0188987
108. Zhang B, Tian J, Pei S, Chen Y, He X, Dong Y, et al. Machine learning-assisted system for thyroid nodule diagnosis. Thyroid (2019) 29:858–67. doi: 10.1089/thy.2018.0380
109. Zhao C-K, Ren T-T, Yin Y-F, Shi H, Wang H-X, Zhou B-Y, et al. A comparative analysis of two machine learning-based diagnostic patterns with thyroid imaging reporting and data system for thyroid nodules: diagnostic performance and unnecessary biopsy rate. Thyroid (2021) 31:470–81. doi: 10.1089/thy.2020.0305
110. Qin P, Wu K, Hu Y, Zeng J, Chai X. Diagnosis of benign and malignant thyroid nodules using combined conventional ultrasound and ultrasound elasticity imaging. IEEE J BioMed Heal Inf (2020) 24:1028–36. doi: 10.1109/JBHI.2019.2950994
111. Pereira C, Dighe MK, Alessio AM. Comparison of machine learned approaches for thyroid nodule characterization from shear wave elastography images. In: Mori K, Petrick N, editors. Medical Imaging 2018: Computer-Aided Diagnosis (2018). Houston, United States: SPIE. p. 68. doi: 10.1117/12.2294572
112. Kim SK, Chai YJ, Park I, Woo JW, Lee JH, Lee KE, et al. Nomogram for predicting central node metastasis in papillary thyroid carcinoma. J Surg Oncol (2017) 115:266–72. doi: 10.1002/jso.24512
113. Liu T, Ge X, Yu J, Guo Y, Wang Y, Wang W, et al. Comparison of the application of b-mode and strain elastography ultrasound in the estimation of lymph node metastasis of papillary thyroid carcinoma based on a radiomics approach. Int J Comput Assist Radiol Surg (2018) 13:1617–27. doi: 10.1007/s11548-018-1796-5
114. Jiang M, Li C, Tang S, Lv W, Yi A, Wang B, et al. Nomogram based on shear-wave elastography radiomics can improve preoperative cervical lymph node staging for papillary thyroid carcinoma. Thyroid (2020) 30:885–97. doi: 10.1089/thy.2019.0780
115. Wildeboer RR, Mannaerts CK, van Sloun RJG, Budäus L, Tilki D, Wijkstra H, et al. Automated multiparametric localization of prostate cancer based on b-mode, shear-wave elastography, and contrast-enhanced ultrasound radiomics. Eur Radiol (2020) 30:806–15. doi: 10.1007/s00330-019-06436-w
116. Shi J, Zhou S, Liu X, Zhang Q, Lu M, Wang T. Stacked deep polynomial network based representation learning for tumor classification with small ultrasound image dataset. Neurocomputing (2016) 194:87–94. doi: 10.1016/j.neucom.2016.01.074
117. Xian MF, Zheng X, Xu JB, Li X, Da CL, Wang W. Prediction of lymph node metastasis in rectal cancer: comparison between shear-wave elastography based ultrasomics and mri. Diagn Interv Radiol (2021) 27:424–31. doi: 10.5152/DIR.2021.20031
118. Chen LD, Liang JY, Wu H, Wang Z, Li SR, Li W, et al. Multiparametric radiomics improve prediction of lymph node metastasis of rectal cancer compared with conventional radiomics. Life Sci (2018) 208:55–63. doi: 10.1016/j.lfs.2018.07.007
119. Chen LD, Li W, Xian MF, Zheng X, Lin Y, Liu BX, et al. Preoperative prediction of tumour deposits in rectal cancer by an artificial neural network–based US radiomics model. Eur Radiol (2020) 30:1969–79. doi: 10.1007/s00330-019-06558-1
120. Zhou B, Zhang X. Lung mass density analysis using deep neural network and lung ultrasound surface wave elastography. Ultrasonics (2018) 89:173–7. doi: 10.1016/j.ultras.2018.05.011
121. Zhou B, Bartholmai BJ, Kalra S, Zhang X. Predicting lung mass density of patients with interstitial lung disease and healthy subjects using deep neural network and lung ultrasound surface wave elastography. J Mech Behav BioMed Mater (2020) 104:103682. doi: 10.1016/j.jmbbm.2020.103682
122. Zhou B, Bartholmai BJ, Kalra S, Osborn T, Zhang X. Lung mass density prediction using machine learning based on ultrasound surface wave elastography and pulmonary function testing. J Acoust Soc Am (2021) 149:1318–23. doi: 10.1121/10.0003575
123. Gao J, Xu L, Bouakaz A, Wan M. A deep Siamese-based plantar fasciitis classification method using shear wave elastography. IEEE Access (2019) 7:130999–1007. doi: 10.1109/ACCESS.2019.2940645
124. Cepeda S, García-García S, Arrese I, Velasco-Casares M, Sarabia R. Advantages and limitations of intraoperative ultrasound strain elastography applied in brain tumor surgery: a single-center experience. Oper Neurosurg (Hagerstown Md) (2022) 22:305–14. doi: 10.1227/ons.0000000000000122
125. Cepeda S, García-García S, Arrese I, Fernández-Pérez G, Velasco-Casares M, Fajardo-Puentes M, et al. Comparison of intraoperative ultrasound b-mode and strain elastography for the differentiation of glioblastomas from solitary brain metastases. an automated deep learning approach for image analysis. Front Oncol (2021) 10:590756. doi: 10.3389/fonc.2020.590756
126. Cepeda S, García-García S, Arrese I, Velasco-Casares M, Sarabia R. Relationship between the overall survival in glioblastomas and the radiomic features of intraoperative ultrasound: a feasibility study. J Ultrasound (2022) 25:121–8. doi: 10.1007/s40477-021-00569-9
127. Goodfellow BI, Pouget-abadie J, Mirza M, Xu B, Warde-farley D, Ozair S, et al. Generative adversarial networks. Commun ACM (2020) 63:139–44. doi: 10.1145/3422622
128. Yang Q, Liu Y, Chen T, Tong Y. Federated machine Learning: concept and applications. ACM Trans Intell Syst Technol (2019) 10:1–19. doi: 10.1145/3298981
129. Li Y, Li W, Xiong J, Xia J, Xie Y. Comparison of supervised and unsupervised deep learning methods for medical image synthesis between computed tomography and magnetic resonance images. BioMed Res Int (2020) 2020:5193707. doi: 10.1155/2020/5193707
130. Nie D, Trullo R, Lian J, Petitjean C, Ruan S, Wang Q, et al. “Medical Image Synthesis with Context-Aware Generative Adversarial Networks.,” In: Descoteaux M, Maier-Hein L, Franz A, Jannin P, Collins DL, Duchesne S, editors. Medical Image Computing and Computer Assisted Intervention − MICCAI 2017. Lecture Notes in Computer Science (2017). Cham: Springer International Publishing. p. 417–25. doi: 10.1007/978-3-319-66179-7_48
131. Wu Z, Pan S, Chen F, Long G, Zhang C, Member S, et al. A comprehensive survey on graph neural networks. IEEE Trans Neural Networks Learn Syst (2021) 32:4–24. doi: 10.1109/TNNLS.2020.2978386
132. Garcia V, Bruna J. Few-Shot Learning with Graph Neural Networks. (2018), 1–13. doi: 10.48550/arXiv.1711.04043
Keywords: ultrasound, elastography, artificial intelligence, machine learning, deep learning, radiomics
Citation: Zhang X-Y, Wei Q, Wu G-G, Tang Q, Pan X-F, Chen G-Q, Zhang D, Dietrich CF and Cui X-W (2023) Artificial intelligence - based ultrasound elastography for disease evaluation - a narrative review. Front. Oncol. 13:1197447. doi: 10.3389/fonc.2023.1197447
Received: 31 March 2023; Accepted: 22 May 2023;
Published: 02 June 2023.
Edited by:
Jakub Nalepa, Silesian University of Technology, PolandCopyright © 2023 Zhang, Wei, Wu, Tang, Pan, Chen, Zhang, Dietrich and Cui. This is an open-access article distributed under the terms of the Creative Commons Attribution License (CC BY). The use, distribution or reproduction in other forums is permitted, provided the original author(s) and the copyright owner(s) are credited and that the original publication in this journal is cited, in accordance with accepted academic practice. No use, distribution or reproduction is permitted which does not comply with these terms.
*Correspondence: Qi Tang, MjUwNzE3NjczMUBxcS5jb20=; Xin-Wu Cui, Y3VpeGlud3VAbGl2ZS5jbg==