- 1School of Chinese Medicine, Shandong University of Traditional Chinese Medicine, Jinan, China
- 2Department of Obstetrics and Gynecology, Shandong Provincial Third Hospital, Jinan, China
- 3Department of Pathology, Shandong University of Traditional Chinese Medicine, Jinan, China
Background: The role of drug repositioning in the treatment of ovarian cancer has received increasing attention. Although promising results have been achieved, there are also major controversies.
Methods: In this study, we conducted a drug-target Mendelian randomisation (MR) analysis to systematically investigate the reported effects and relevance of traditional drugs in the treatment of ovarian cancer. The inverse-variance weighted (IVW) method was used in the main analysis to estimate the causal effect. Several MR methods were used simultaneously to test the robustness of the results.
Results: By screening 31 drugs with 110 targets, FNTA, HSPA5, NEU1, CCND1, CASP1, CASP3 were negatively correlated with ovarian cancer, and HMGCR, PLA2G4A, ITGAL, PTGS1, FNTB were positively correlated with ovarian cancer.
Conclusion: Statins (HMGCR blockers), lonafarnib (farnesyltransferase inhibitors), the anti-inflammatory drug aspirin, and the anti-malarial drug adiponectin all have potential therapeutic roles in ovarian cancer treatment.
Highlights
● The treatment outcomes for ovarian cancer remain unsatisfactory.
● Drug repositioning is a superior therapeutic strategy to investigate.
● Fat-soluble statins may be more effective than water-soluble statins.
● Statins (HMGCR blockers), lonafarnib (farnesyltransferase inhibitors), the anti-inflammatory drug aspirin, and the anti-malarial drug adiponectin all have a therapeutic role in ovarian cancer.
Introduction
Ovarian cancer is the second most common gynaecological cancer and the gynaecological malignancy with the highest mortality rate worldwide (1). Due to the concealed location of the disease, most cases are found at an advanced stage. Although the effect of surgery or chemotherapy is better in early stage patients, they are prone to relapse and drug resistance. Therefore, there is a need to develop new therapeutic agents (2). But drug discovery and development is an expensive, time-consuming, and risky business. In this context, drug repositioning strategies are becoming increasingly attractive. Drug repositioning aims to identify new indications for old drugs, with the advantage that data on pharmacokinetic properties and toxicity are already available. Thus, in principle, it is possible to reduce research costs and accelerate drug use/distribution (3).
Currently, there are many related reports on the role of drug repositioning in ovarian cancer treatment (4, 5), such as lipid-lowering drug statins (6), glucose-lowering drug metformin (7), antiarrhythmic drug amiodarone (8), neuroprotective drugs (9), ACEI for hypertension (10), disulfiram for chronic alcoholism anti-inflammatory (11, 12), anti-bacterial (13, 14), anti-viral (15), and anti-parasitic drugs (16, 17) as well as calcium-channel blockers (18), have all shown different anti-ovarian cancer effects. However, there were huge differences in the specific results. This discrepancy may be related to being affected by different confounding factors (19).
Mendelian randomization studies are an etiological research method based on Mendel’s law of independent assignment, using genetic factors as instrumental variables for risk factors to make causal inferences about disease or health-related outcomes. Its advantage is that it can effectively avoid the influence of various confounding factors (20). In this study, we used the Mendelian randomization study method to analyse the previously reported therapeutic effects of conventional drugs on ovarian cancer.
Materials and methods
All analyses used publicly available database resources and therefore did not require ethical approval from an institutional review board. The entire MR analysis process illustrated in Figure 1.
GWAS data source of ovarian cancer
Genetic association data for ovarian cancer (ieu-a-1120) were obtained from the Ovarian Cancer Association Consortium (OCAC), which performed a GWAS analysis of 22,406 cases of invasive epithelial ovarian cancer pathology and 40,941 healthy population controls, and through genetic analysis identified 17273705 SNP loci (21) (Table 1).
We additionally selected a dataset on ovarian cancer (ieu-b-4963) from the UKB database, which contains 1,218 ovarian cancer cases and 198,523 controls from healthy populations, and identified 9,822,229 SNP loci by genetic analysis. Both data sets were from European populations. (Table 1) (https://gwas.mrcieu.ac.uk).
Selection of drugs and targets
All publicly available literatures (published in English) were retrieved from China National Knowledge Infrastructure (CNKI) and Pubmed database from inception to December 2023 with the following keywords as search terms: “Drug repositioning” and “ovarian cancer”, and drugbank database was used to search the targets of related drugs. Drugs with the same mechanism of action and target were grouped into one group, and drugs with unclear targets were excluded.
Selection of drug targeting sites and exposure factors
We used relevant drug targets as proxies for each drug exposure at 100 kb loci downstream of each of the drug target gene regions above and below the drug target gene region. p<5×10-8, r2<0.3 were obtained to screen for SNP loci closely related to the drug target as exposure factors, and targets for which no SNP loci were retrieved were excluded. To reduce the weak instrumental variable bias, the F-statistic was calculated separately for each SNP, and then weak instruments with an F-statistic < 10 were filtered.
MR analysis
In univariate MR analysis, IVW is often used as the primary statistical method for MR analysis-. When horizontal pleiotropy is not present, inverse variance weighted (IVW) can provide relatively stable and accurate causality by using meta-analytical methods in combination with estimates of the causal effects of each SNP. The results of IVW were supplemented using the MR Egger, (weighted median, WME) method. Heterogeneity was estimated using Cochran’s Q statistic and p < 0.05 was used as the threshold parameter for identifying heterogeneity. Directional pleiotropy was detected using and MR-Egger test and outliers, and p < 0.05 was used as the threshold parameter for identifying horizontal pleiotropy. Leave-one-out sensitivity analyses were used to evaluate the magnitude of the effect of SNPs on the estimation results of causal associations.
Result
After removing the drugs with completely consistent targets and those without clear targets, 31 drugs and 110 targets were obtained. (see Supplementary Table 1) The causal associations between different kinds of drug targets and ovarian cancer were analysed by MR, and there were 77 instrumental variable SNPs with F-statistics >10, suggesting that there was a low possibility of weak instrumental variable bias. The information of the 77 SNPs is shown in Table 2.
MR analysis was performed using IVW, MR Egger and weighted median methods. IVW analysis of target genes in relation to ovarian cancer showed that HMGCR, PLA2G4A, ITGAL, PTGS1, FNTA, FNTB, HSPA5, NEU1, CCND1, CASP1, CASP3 were significantly associated in ovarian cancer had significant correlation. Among them, FNTA, HSPA5, NEU1, CCND1, CASP1, CASP3 had a negative causal association with ovarian cancer development, and HMGCR, PLA2G4A, ITGAL, PTGS1, FNTB had a positive causal association with ovarian cancer development.
FNTA, HSPA5, NEU1, CCND1, CASP1, CASP3 were included in the analyses of 4, 4, 4, 9, 7 and 11 SNPs respectively, with statistically significant IVW results for FNTA (ORIVW = 0.714571216, 95% CI = 0.597682908 - 0.854319265; PIVW = 0.000226306), HSPA5 (ORIVW = 0.997892376, 95% CI = 0.995974116- 0.999814331; PIVW = 0.031622971), NEU1 (ORIVW = 0.996386436, 95% CI = 0.993805213- 0.998974362; PIVW = 0.006230918), CCND1 (ORIVW = 0.996798602, 95% CI = 0.995203219- 0.998396544; PIVW = 8.72E-05), CASP1 ORIVW = 0.914123044, 95% CI = 0.836059318 - 0.999475662; PIVW= 0.048664899), CASP3 (ORIVW = 0.999417702, 95% CI = 0.998700179- 0.999846044; PIVW = 0.012906474), the statistical results showed a negative correlation between these targets and ovarian cancer risk. (Figure 2; Supplementary Table 2).
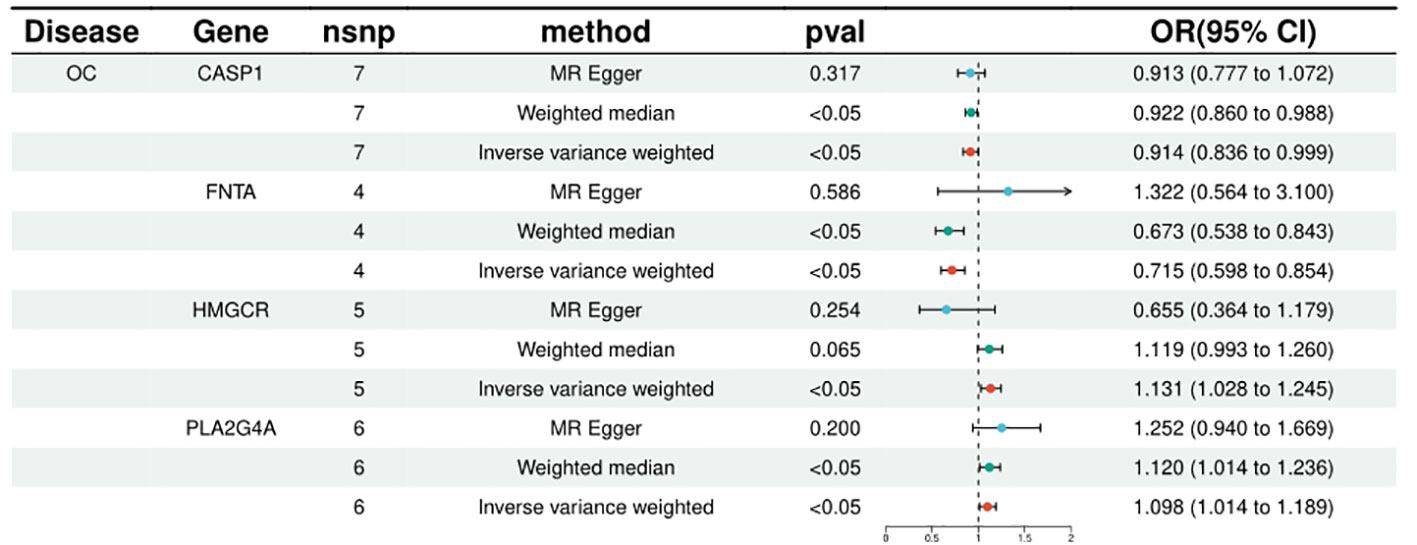
Figure 2 Summary of the univariable MR analysis results for the association between drug-related targets and the risk of Ovarian cancer (ieu-a-1120) using the IVW, MR Egger, and Weighted median methods.
HMGCR, PLA2G4A, ITGAL, PTGS1, and FNTB were included in the analysis of 5, 6, 10, 8, and 9, respectively, and the statistical results of IVW were, respectively, HMGCR (ORIVW = 1.131314709, 95% CI = 1.027776107 - 1.027776107; PIVW = 0.011753459), PLA2G4A (ORIVW = 1.097906571, 95% CI = 1.013595152- 1.189231062; PIVW = 0.021949277), ITGAL (ORIVW = 1.001353198, 95% CI = 1.000217005 - 1.002490683; PIVW = 0.000579236), PTGS1 (ORIVW = 1.002096738, 95% CI = 1.000537301-1.003658604; PIVW = 0.008388408), FNTB (ORIVW = 1.000560816, 95% CI = 1.000092211 - 1.001029641; PIVW = 0.018986672). It is suggested that increased expression of these targets may promote ovarian carcinogenesis, while targeted inhibition of these targets may prevent or reduce ovarian carcinogenesis. (Figure 3; Supplementary Table 2).
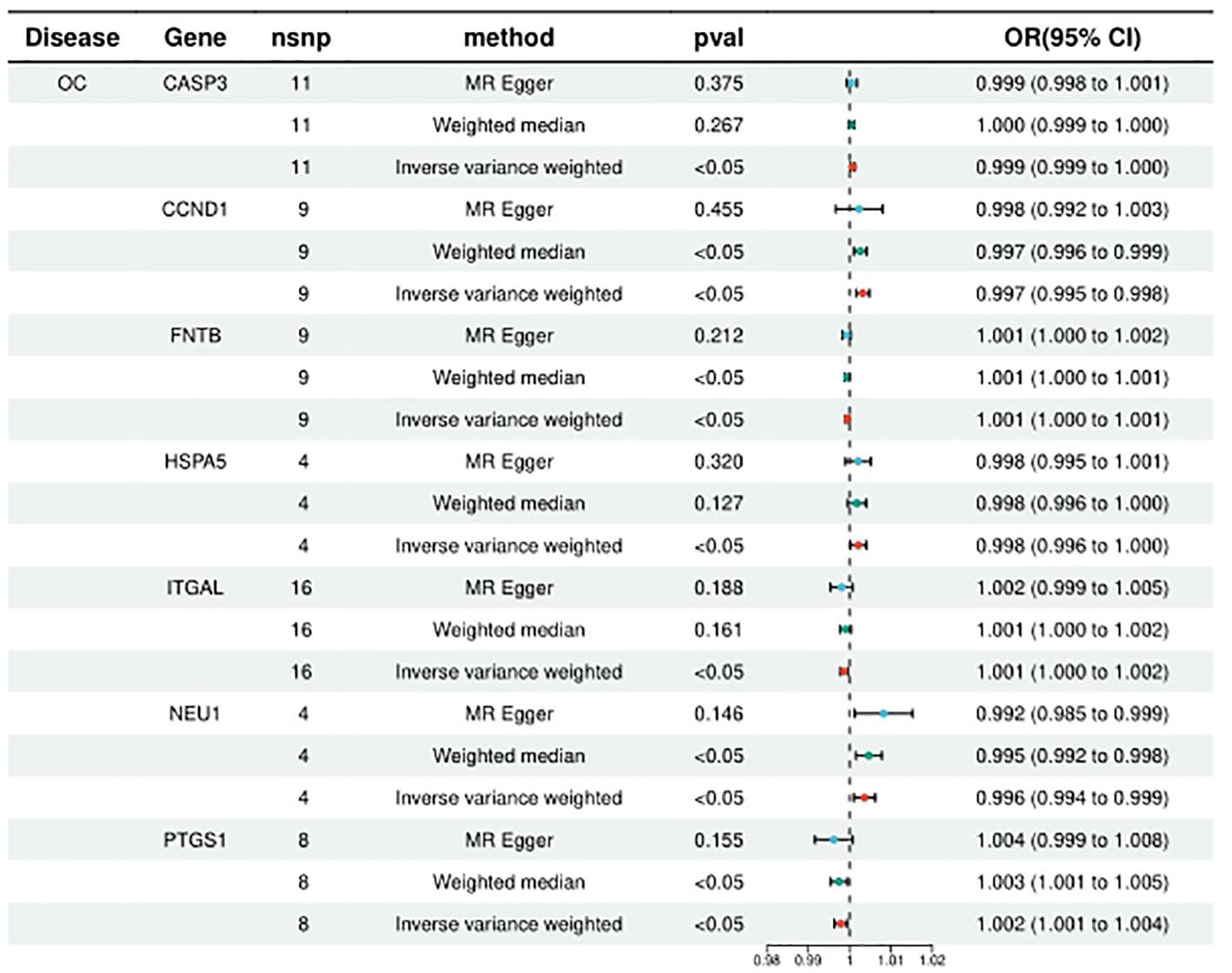
Figure 3 Summary of the univariable MR analysis results for the association between drug-related targets and the risk of Ovarian cancer (ieu-b-4963) using the IVW, MR Egger, and Weighted median methods.
Results of sensitivity analyses
The results of MR-PRESSO analysis showed that there were no outliers for all SNPs included in this study. Heterogeneity test was conducted using the IVW method and MR-Egger regression, when the test P < 0.05, heterogeneity exists among SNPs; when the P value > 0.05, heterogeneity does not exist among SNPs (Supplementary Figure 1). MR-Egger regression was used to test for polytropy, when the test P < 0.05, it means that there is polytropy; when the P value > 0.05, it means that there is no polytropy. (Supplementary Figure 2) Leave-one-out analysis was used to remove one SNP at a time, and the remaining SNPs were subjected to IVW analysis. (Supplementary Figure 3,Supplementary Table 3).
Discussion
Currently, the role of drug repositioning in the treatment of ovarian cancer is gradually attracting attention, but there is a lack of large-scale, multicentre, prospective studies, and the retrospective studies are affected by a variety of confounding factors, leading to huge discrepancies in the results of current studies. Drug-targeted Mendelian randomisation studies are based on the downstream products of target proteins (biomarker), SNPs (pQTL or eQTL) with significant effects on biomarker near the gene encoding the target protein are used as instrumental variables, and Mendelian randomisation analyses are performed with disease as the endpoint to validate the effects of the target proteins on the disease under study.
By analysis, we found that HMGCR, PLA2G4A, ITGAL, NR3C1, PTGS1, FNTA, FNTB, HSPA5, NEU1, CCND1, CASP1, CASP3 were significantly correlated with ovarian carcinogenesis. Among them, HMGCR is an important target of various statins, ITGAL is mainly a target of fat-soluble statins (e.g., lovastatin, pitavastatin), and FNTA and FNTB are targets of the MVA pathway-related enzyme inhibitor lonafarnib (22). PLA2G4A is a target of the anti-malarial drug adiponectin, PTGS1, HSPA5, NEU1, CCND1, CASP1, CASP3 is an important target of aspirin.
The main target of action of statins is HMGCR, but they can also act on other targets, such as HDAC2, DPP4, ITGAL and so on. Among these, ITGAL encodes integrin α, which is the target of fat-soluble statins. Previously, it was thought that only fat-soluble statins have an anti-ovarian cancer effect and can prolong the generation time of patients (23). It has been suggested that this may be due to the fact that lipid-soluble drugs can cross the cell membrane more easily and thus exert anti-tumour effects (24). The results of the present study suggest that lipid-soluble statins may exert anti-tumour effects not only by interfering with HMGCR, but also, possibly, by inhibiting ITGAL.
As statins are key enzymes in the MVA pathway, it has long been questioned whether similar anti-tumour effects could be achieved by inhibiting other enzymes in the pathway (25), or whether better therapeutic effects could be achieved by combining drugs (26). It has also been suggested that the anti-tumour effects of statins may not be related to lipid lowering but to their intermediate metabolites such as FPP and GGPP, which provide isoprenyl groups to isoprenylate a variety of small GTPase-binding proteins such as Ras, MEK, PI3K, and anchor them to the cell membrane. This is how it works, and some studies suggest that zoledronic acid and lonafarnib may reduce the production of FPP and GGPP, thereby exerting an anti-ovarian cancer effect. However, it has also been suggested that the anti-tumour effect of statins can only be reversed by the addition of GGPP (24). In this study, we also found that the main targets of zoledronic acid, GGPS1 and FDPS, were not associated with the development of ovarian cancer, but the targets of action of lonafarnib, the two subunits of farnesyltransferase, FNTA and FNTB, were both associated with the development of ovarian cancer, which seems to support this view. However, it is difficult to explain why FNTA is negatively associated with ovarian cancer prognosis, while FNTB is positively associated with ovarian carcinogenesis.
The mechanism by which aspirin improves the prognosis of ovarian cancer is usually thought to be related to COX inhibition, with low-dose aspirin primarily inhibiting COX1 and higher doses inhibiting COX2 activity. In the past, the literature has suggested that high doses of aspirin reduced mortality in patients of ovarian cancer (19). More recent literature suggests that the source of the statistics may amplify this dose-dependent difference (27). Our study shows that PTGS1 (COX1) is significantly positively correlated with ovarian cancer prognosis, while PTGS2 is not significantly correlated with ovarian cancer prognosis. In addition aspirin may inhibit several targets such as HSPA5, NEU1, CCND1, CASP1, CASP3, which are negatively correlated with ovarian cancer prognosis. Therefore, whether simply acting on PTGS1 targets can obtain better anti-ovarian cancer effects will be a question we need to verify in the future.
Originally used in antimalarial therapy, quinacrine has now been shown to have anti-tumour effects (28, 29), particularly in inducing apoptosis in P53-deficient or mutated tumour cells (30). PLA2G4A encodes phospholipase A2, and quinacrine may inhibit ovarian cancer by inhibiting PLA2G4A which in turn reduces arachidonic acid metabolites and thus inhibits ovarian cancer development.
Limitation of the study
Currently, drug target Mendelian randomisation analysis usually selects single-target drugs, such as PCSK9 inhibitors, while statins are considered to be single-target drugs for HMGCR (20), but statins are not actually single-target drugs, and their targets of action are not limited to HMGCR. there are also drugs for which it is difficult to specify which target is the main target of their action. Therefore, in this study, all known targets were screened for target selection. However, whether this affects the results is also a problem that needs to be addressed in the current Mendelian randomization analysis of drug targeting.
In addition, when selecting genetic association data for ovarian cancer, we selected two datasets, but found that the conclusions drawn from the two databases were not consistent. The number of ovarian cancer patients and SNP loci found in ieu-a-1120 data far exceeds that in ieu-b-4963. It suggests that the results of drug-targeted Mendelian randomisation analysis are affected by multiple factors (sequencing technology, sequencing depth and number of cases).
Third, the genetic information related to ovarian cancer is mainly from European populations, but the results of drug repositioning may be affected by regional and racial differences as reported in the relevant literature. Further studies are needed to clarify the differences between different races.
Therefore, the results of this study are only for reference, and we hope to provide ideas for the selection of therapeutic targets for ovarian cancer in the future.
Conclusion
This study provides MR evidence for the potential value of conventional drugs in preventing ovarian cancer. Genetic links between drug targets and ovarian cancer may accelerate drug development prioritisation and reduce the failure rate of clinical trials and provide new ideas for future drug development.
Data availability statement
The original contributions presented in the study are included in the article/Supplementary Material. Further inquiries can be directed to the corresponding authors.
Ethics statement
Ethical approval was not required for the study involving humans in accordance with the local legislation and institutional requirements. Written informed consent to participate in this study was not required from the participants or the participants’ legal guardians/next of kin in accordance with the national legislation and the institutional requirements.
Author contributions
LZ: Writing – original draft, Data curation, Software. HZ: Writing – original draft, Conceptualization, Investigation, Software. XZ: Data curation, Software, Writing – original draft. RC: Data curation, Investigation, Writing – original draft. LX: Supervision, Validation, Writing – review & editing.
Funding
The author(s) declare that no financial support was received for the research, authorship, and/or publication of this article.
Conflict of interest
The authors declare that the research was conducted in the absence of any commercial or financial relationships that could be construed as a potential conflict of interest.
Publisher’s note
All claims expressed in this article are solely those of the authors and do not necessarily represent those of their affiliated organizations, or those of the publisher, the editors and the reviewers. Any product that may be evaluated in this article, or claim that may be made by its manufacturer, is not guaranteed or endorsed by the publisher.
Supplementary material
The Supplementary Material for this article can be found online at: https://www.frontiersin.org/articles/10.3389/fonc.2024.1376515/full#supplementary-material
Supplementary Figure 1 | IVW and MR-Egger regression heterogeneity test chart.
Supplementary Figure 2 | Scatter plot of Mendelian randomization analysis.
Supplementary Figure 3 | Analysis diagram of leave-one-out method.
References
1. Siegel RL, Miller KD, Fuchs HE, Jemal A. Cancer statistics, 2022. CA: Cancer J Clin. (2022) 72:7–33. doi: 10.3322/caac.21708
2. Konstantinopoulos PA, Matulonis UA. Clinical and translational advances in ovarian cancer therapy. Nat Cancer. (2023) 4:1239–57. doi: 10.1038/s43018-023-00617-9
3. Hua Y, Dai X, Xu Y, Xing G, Liu H, Lu T, et al. Drug repositioning: Progress and challenges in drug discovery for various diseases. Eur J Med Chem. (2022) 234:114239. doi: 10.1016/j.ejmech.2022.114239
4. Banno K, Iida M, Yanokura M, Irie H, Masuda K, Kobayashi Y, et al. Drug repositioning for gynecologic tumors: a new therapeutic strategy for cancer. TheScientificWorldJournal. (2015) 2015:341362. doi: 10.1155/2015/341362
5. Pushpakom S, Iorio F, Eyers PA, Escott KJ, Hopper S, Wells A, et al. Drug repurposing: progress, challenges and recommendations. Nat Rev Drug Discovery. (2019) 18:41–58. doi: 10.1038/nrd.2018.168
6. Abdullah MI, Abed MN, Khanim F, Richardson A. Screening a library of approved drugs reveals that prednisolone synergizes with pitavastatin to induce ovarian cancer cell death. Sci Rep. (2019) 9:9632. doi: 10.1038/s41598-019-46102-1
7. Tossetta G. Metformin improves ovarian cancer sensitivity to paclitaxel and platinum-based drugs: A review of in vitro findings. Int J Mol Sci. (2022) 23(21):12893. doi: 10.3390/ijms232112893
8. Belur Nagaraj A, Joseph P, Kovalenko O, Wang Q, Xu R, DiFeo A. Evaluating class III antiarrhythmic agents as novel MYC targeting drugs in ovarian cancer. Gynecol Oncol. (2018) 151:525–32. doi: 10.1016/j.ygyno.2018.09.019
9. Lee JY, Ham J, Lim W, Song G. Apomorphine facilitates loss of respiratory chain activity in human epithelial ovarian cancer and inhibits angiogenesis in vivo. Free Radical Biol Med. (2020) 154:95–104. doi: 10.1016/j.freeradbiomed.2020.05.001
10. Regulska K, Michalak M, Kolenda T, Kozłowska-Masłoń J, Guglas K, Stanisz B. Angiotensin-converting enzyme inhibitors for ovarian cancer? - a new adjuvant option or a silent trap. Rep Pract Oncol Radiother. (2023) 28:551–64. doi: 10.5603/RPOR.a2023.0059
11. Papaioannou M, Mylonas I, Kast RE, Brüning A. Disulfiram/copper causes redox-related proteotoxicity and concomitant heat shock response in ovarian cancer cells that is augmented by auranofin-mediated thioredoxin inhibition. Oncoscience. (2014) 1:21–9. doi: 10.18632/oncoscience.v1i1
12. Gan Y, Liu T, Feng W, Wang L, Li LI, Ning Y. Drug repositioning of disulfiram induces endometrioid epithelial ovarian cancer cell death via the both apoptosis and cuproptosis pathways. Oncol Res. (2023) 31:333–43. doi: 10.32604/or.2023.028694
13. Ortore G, Poli G, Martinelli A, Tuccinardi T, Rizzolio F, Caligiuri I. From anti-infective agents to cancer therapy: A drug repositioning study revealed a new use for nitrofuran derivatives. Med Chem (Shariqah (United Arab Emirates)). (2022) 18:249–59. doi: 10.2174/1573406417666210511001241
14. Fawzy MA, Abu-Baih RH, Abuo-Rahma GEA, Abdel-Rahman IM, El-Sheikh AAK, Nazmy MH. In vitro anticancer activity of novel ciprofloxacin mannich base in lung adenocarcinoma and high-grade serous ovarian cancer cell lines via attenuating MAPK signaling pathway. Molecules (Basel Switzerland). (2023) 28(3):1137. doi: 10.3390/molecules28031137
15. Subeha MR, Goyeneche AA, Bustamante P, Lisio MA, Burnier JV, Telleria CM. Nelfinavir induces cytotoxicity towards high-grade serous ovarian cancer cells, involving induction of the unfolded protein response, modulation of protein synthesis, DNA damage, lysosomal impairment, and potentiation of toxicity caused by proteasome inhibition. Cancers. (2021) 14(1):99. doi: 10.1101/2021.10.25.465799
16. Deng Y, Wang Z, Zhang F, Qiao M, Yan Z, Wei Q, et al. A blockade of IGF signaling sensitizes human ovarian cancer cells to the anthelmintic niclosamide-induced anti-proliferative and anticancer activities. Cell Physiol Biochem. (2016) 39:871–88. doi: 10.1159/000447797
17. Huang L, Zhao L, Zhang J, He F, Wang H, Liu Q, et al. Antiparasitic mebendazole (MBZ) effectively overcomes cisplatin resistance in human ovarian cancer cells by inhibiting multiple cancer-associated signaling pathways. Aging. (2021) 13:17407–27. doi: 10.18632/aging.v13i13
18. Lee H, Kim JW, Kim DK, Choi DK, Lee S, Yu JH, et al. Calcium channels as novel therapeutic targets for ovarian cancer stem cells. Int J Mol Sci. (2020) 21(7):2327. doi: 10.3390/ijms21072327
19. Kobayashi Y, Banno K, Kunitomi H, Tominaga E, Aoki D. Current state and outlook for drug repositioning anticipated in the field of ovarian cancer. J Gynecol Oncol. (2019) 30:e10. doi: 10.3802/jgo.2019.30.e10
20. Schmidt AF, Finan C, Gordillo-Marañón M, Asselbergs FW, Freitag DF, Patel RS, et al. Genetic drug target validation using Mendelian randomisation. Nat Commun. (2020) 11:3255. doi: 10.1038/s41467-020-16969-0
21. Phelan CM, Kuchenbaecker KB, Tyrer JP, Kar SP, Lawrenson K, Winham SJ, et al. Identification of 12 new susceptibility loci for different histotypes of epithelial ovarian cancer. Nat Genet. (2017) 49:680–91. doi: 10.1038/ng.3826
22. Kobayashi Y, Kashima H, Rahmanto YS, Banno K, Yu Y, Matoba Y, et al. Drug repositioning of mevalonate pathway inhibitors as antitumor agents for ovarian cancer. Oncotarget. (2017) 8:72147–56. doi: 10.18632/oncotarget.v8i42
23. Vogel TJ, Goodman MT, Li AJ, Jeon CY. Statin treatment is associated with survival in a nationally representative population of elderly women with epithelial ovarian cancer. Gynecol Oncol. (2017) 146:340–5. doi: 10.1016/j.ygyno.2017.05.009
24. Torralba M, Farra R, Maddaloni M, Grassi M, Dapas B, Grassi G. Drugs repurposing in high-grade serous ovarian cancer. Curr Med Chem. (2020) 27:7222–33. doi: 10.2174/0929867327666200713190520
25. Göbel A, Zinna VM, Dell’endice S, Jaschke N, Kuhlmann JD, Wimberger P, et al. Anti-tumor effects of mevalonate pathway inhibition in ovarian cancer. BMC Cancer. (2020) 20:703. doi: 10.1186/s12885-020-07164-x
26. Xia L, Ding S, Wang X, Zhang X, Zhu L, Zhang H, et al. Advances in ovarian cancer treatment using a combination of statins with other drugs. Front Pharmacol. (2022) 13:1048484. doi: 10.3389/fphar.2022.1048484
27. Majidi A, Na R, Jordan SJ, DeFazio A, Obermair A, Friedlander M, et al. Common analgesics and ovarian cancer survival: the Ovarian cancer Prognosis And Lifestyle (OPAL) Study. J Natl Cancer Inst. (2023) 115:570–7. doi: 10.1093/jnci/djac239
28. Marastoni S, Madariaga A, Pesic A, Nair SN, Li ZJ, Shalev Z, et al. Repurposing itraconazole and hydroxychloroquine to target lysosomal homeostasis in epithelial ovarian cancer. Cancer Res Commun. (2022) 2:293–306. doi: 10.1158/2767-9764.CRC-22-0037
29. Jung D, Khurana A, Roy D, Kalogera E, Bakkum-Gamez J, Chien J, et al. Quinacrine upregulates p21/p27 independent of p53 through autophagy-mediated downregulation of p62-Skp2 axis in ovarian cancer. Sci Rep. (2018) 8:2487. doi: 10.1038/s41598-018-20531-w
Keywords: Mendelian randomisation (MR) analysis, ovarian cancer, drug repositioning, statins, aspirin
Citation: Zhu L, Zhang H, Zhang X, Chen R and Xia L (2024) Drug repositioning and ovarian cancer, a study based on Mendelian randomisation analysis. Front. Oncol. 14:1376515. doi: 10.3389/fonc.2024.1376515
Received: 25 January 2024; Accepted: 25 March 2024;
Published: 08 April 2024.
Edited by:
Eswar Shankar, The Ohio State University, United StatesReviewed by:
Shiv Verma, Case Western Reserve University, United StatesRadha Vaddavalli, The Ohio State University, United States
Copyright © 2024 Zhu, Zhang, Zhang, Chen and Xia. This is an open-access article distributed under the terms of the Creative Commons Attribution License (CC BY). The use, distribution or reproduction in other forums is permitted, provided the original author(s) and the copyright owner(s) are credited and that the original publication in this journal is cited, in accordance with accepted academic practice. No use, distribution or reproduction is permitted which does not comply with these terms.
*Correspondence: Lei Xia, pathology001@sina.com; Hairong Zhang, sdzhhr7211@163.com
†These authors have contributed equally to this work and share first authorship