- 1Pharmacology Laboratory, National Institute of Pediatrics, Mexico City, Mexico
- 2Neuroscience Laboratory, National Institute of Pediatrics, Mexico City, Mexico
- 3Oncology Service, National Institute of Pediatrics, Mexico City, Mexico
- 4Tissue Engineering, Cell Therapy and Regenerative Medicine Unit, National Institute of Rehabilitation, Mexico City, Mexico
- 5Pathology Department, National Institute of Neurology and Neurosurgery, Mexico City, Mexico
- 6Biomedical Oncology Laboratory, National Institute of Respiratory Diseases, Mexico City, Mexico
- 7Epidemiological Surveillance Unit, National Institute of Respiratory Diseases, Mexico City, Mexico
Purpose: The objective of this study was to determine whether a comorbidity index could be used to predict mortality in pediatric patients with chemotherapy-treated solid tumors.
Methods: Pediatric patients who underwent chemotherapy treatment for solid tumors were included, and demographic, clinical, and comorbidity data were obtained from patient electronic records.
Results: A total of 196 pediatric patients with embryonic solid tumors were included. Metastatic tumors were the most frequently observed (n = 103, 52.6%). The most common comorbidities encountered for the Charlson comorbidity index (CCI) were cellulitis (n = 24, 12.2%) and acute renal failure (n = 15, 7.7%). For the Pediatric Comorbidity Index (PCI), the most frequent comorbidities were pneumonia and sepsis, with n = 64 (32.7%) for each. We evaluated established the prognostic values for both indexes using Kaplan-Meier curves, finding that the CCI and PCI could predict mortality with p < 0.0001.
Conclusion: Using the PCI, we observed 100% survival in patients without comorbidities, 70% survival in patients with a low degree of comorbidity, and 20% survival in patients with a high degree of comorbidity. Greater discrimination of probability of survival could be achieved using degrees of comorbidity on the PCI than using degrees of comorbidity on the CCI. The application of the PCI for assessing the hospitalized pediatric population may be of importance for improving clinical evaluation.
Introduction
Childhood cancer is a health problem worldwide, and with a reduction of infectious diseases in low- and middle-income countries, this pathology has become the principal cause of mortality for this population (1). Cancer is the second leading cause of death globally and was responsible for 8.8 million deaths in 2015. Globally, nearly 1 in 6 deaths are due to cancer. It is estimated that 70% of deaths from cancer occur in low- and middle-income countries (2).
Mortality in the pediatric population varies considerably according to tumor type, tumor site, nutritional status, patient age, and tumor stage. In adults, there is an effect of gender on mortality; for example, women with pulmonary cancer have a better prognosis than men with the same diagnosis (3, 4), and the underlying reasons are still debated. Several epidemiological studies have established mortality differences according to the socioeconomic status and/or ethnic group (5–8), but just a few have evaluated the effect of any comorbidity on the prognosis of these patients.
Comorbidity is defined as the presence of one or more diseases among patients with the same disease index, and it is measured with the sum of the number of diseases present in one individual through comorbidity scales that combine the number and severity of the concomitant diseases (9). Increased comorbidity scores are associated with (a) an increased risk of hospitalization due to treatment-associated toxicity, (b) increased hospitalization costs and (c) decreased patient quality of life. These effects increase mortality risk (3–7), even without considering impact on the patient's family or treatment cost.
Recently, epidemiological research studies have started to focus on the evaluation of comorbidities in an attempt to measure associations of these comorbidities with treatment outcomes and patient recovery. This research has shown that comorbidities are related to disease prognosis for patients with cancer and may help to predict patient survival (10–14). The Charlson comorbidity index (CCI), which has been validated in adult populations, is the most frequently used comorbidity index in adult populations and overall (15). This method predicts 1-year mortality for a patient based on multiple (22 in total) comorbid conditions, including cardiac disease, AIDS, and cancer (16, 17). Currently, there are no standardized methods for giving an exact morbimortality percentage in a pediatric, which could be used for chronic or lethal pathologies to improve hope and cope with their disease. The frequent practice of excluding patients with common comorbidities and the lack of systemic measurement in studies of pediatric cancer patients limit the evidence for informed decision making; therefore, baseline measurements using a prognostic approach for clinical management and decision making in pediatric populations with comorbidities undergoing chemotherapy treatment remain unclear. The aim of this study was to evaluate if the comorbidity indexes can predict the mortality in pediatric patients with chemotherapy-treated solid tumors.
Materials and Methods
A retrospective study was conducted by reviewing electronic clinical records of patients diagnosed with the International Classification of Diseases (ICD)-10-compatible solid tumors diagnosis. The study was approved by the Research and Ethics Committees of our institute (National Institute of Pediatrics, NIP) with the registry number 068/2013. One hundred ninety-six pediatric patients (1 month to 17 years old) treated in a tertiary-level hospital (from August 2013 to May 2017) with chemotherapy according to the Mexican Cancer Technical Protocols for Children (18) were analyzed.
Data Information Source
Data were collected from a survey through the electronic clinical record system and included information regarding demographics, clinical data, and comorbidities presented by the patients at diagnosis, such as acute myocardial infarction, heart failure, peripheral arterial disease, cerebrovascular disease, dementia, chronic respiratory failure, gastroduodenal ulcer, rheumatic disease, hemiplegia, mild chronic hepatopathy, moderate/severe hepatopathy, diabetes without damage to the target organs, diabetes with damage to the target organs, solid tumor, or neoplasia with metastasis, leukemia, and AIDS. Follow-up information was documented during chemotherapy cycles. A database was designed to include living patients and for deceased patients.
Charlson Comorbidity Index (CCI)
Twenty-three diseases were studied using the CCI; each condition was given a score of 1, 2, 3, or 6 according to the risk of death associated with this condition. The sum of the scores provided a total score that predicts mortality, and is classified as low comorbidity for a score of 0–2 points or high comorbidity for a score ≥ 3 points (17).
Pediatric Comorbidity Index (PCI)
For the PCI, 30 diseases were evaluated. For the conceptualization of this new index, the relative risks for prediction of 1-year mortality reported for the most common pathologies were used according to Tai et al. (19), and we added some other comorbidities of prognostic importance to the NIP, such as renal disease, lymphoma, HIV/AIDS and associated genetic syndromes. A panel of experts in pediatric oncology (doctors and researchers) from the NIP granted a weighted score of 1, 2, or 4 according to the severity of the comorbidity, assigning a score of 1 for granulocytosis, pneumonia, hydrocephalus, pyrexia, coagulopathy, septicaemia, type 1 diabetes, arrhythmia, mild hepatopathy, pulmonary contusion, obesity, and short size [those with an odds ratio (OR) between 1 and 5]; a score of 2 was assigned for pneumonitis, acidosis, hypertension, malnutrition, cerebrovascular events, traumatic brain injury, candidiasis, renal disease, lymphoma, solid tumor without metastasis, and congenital heart disease (adapted by putting together a ventricular septal defect and a congenital subaortic stenosis encompassing any congenital heart disease), which had an (OR between 6 and 20); and finally a score of 4 was given for heart failure, leukemia, shock state, asphyxia, associated genetic syndromes, HIV/AIDS, solid tumor with metastasis, insipid diabetes and brain cancer because they had an (OR higher than 20) (19).
To further classify the burden of comorbidity, three categories were created according to the scores obtained from the total sum of each patient: 0–2 points (absent), 3–5 points (low) and 6 or more points (high). This index was applied from 0 to 17 years; comorbidities present were recorded at diagnosis and patient admission.
Statistical Analysis
Univariate Analysis
A summary of descriptive statistics was created according to the operationalization of the variables defined in the protocol. Each variable was analyzed separately by means of frequency measurements and percentages for qualitative variables, and measures of central tendency, as well as their corresponding dispersion, were used for quantitative variables.
Bivariate Analysis
Results for the CCI and PCI were compared via non-parametric tests of medians using the Mann-Whitney U. A value of p < 0.05 was considered statistically significant.
Survival Analysis
Kaplan-Meier (KM) curves were plotted to determine existence of differences in survival probability according to the calculated comorbidity category (absent, low, or high) presented by the patients. In the log-rank test, a value of p < 0.05 was considered statistically significant.
Cox Regression
Multivariate analysis by Cox's proportional hazards ratio (HR) model was used to test the significance of prognostic factors including gender, age, CCI, PCI, with the relative risks estimated in two models. The HR and 95% confidence intervals (95% CI) of the prognostic factors were calculated, and the results were considered significant if the 95% CI excluded the null value. Data was analyzed using the IBM SPSS Statistics 20 statistical package.
Results
General Description of the Cohort
From the 196 patients with embryonic solid tumors included in the study, 78 (39.8%) were female and 118 (60.2%) were male, with a median of 8 years old (range, 3–13 years). Seven types of embryonic solid tumors were analyzed. Medulloblastoma, neuroblastoma, glioblastoma, ependymoma, pineal tumor, neuroepithelial tumor, astrocytoma, and neuroectodermal tumor were grouped into central nervous system (CNS) tumors and were the most frequent (n = 53, 27%). Retinoblastomas were the second most common (n = 35, 17.8%) followed by soft tissue sarcomas (n = 34, 17.3%).
Comorbidity Frequencies in Patients With Embryonic Solid Tumors
Metastatic tumors were the most frequent for both indexes (n = 103, 56.2%). Table 1 shows comorbidities evaluated with both indexes; for CCI, the most frequent were cellulitis (n = 24, 12.2%), followed by acute renal failure (n = 15, 7.7%) and hypertension (n = 15, 7.7%). For PCI, the most frequent comorbidities were pneumonia (n = 64, 32.7%), septicaemia (n = 64, 32.7%) and malnutrition (n = 61, 31.1%).
Mortality Differences Associated to Comorbidity Burden
Comparison of CCI scores between deceased and living patients, showed significant differences, with median scores of 6 points for deceased patients and 4 points for living patients (p < 0.001; Figure 1A); PCI also showed significant differences, with median scores of 9 points for deceased patients and 5 points for living patients (p < 0.001; Figure 1B). During follow-up, 70 patients died (35.7%); the most common causes were disease progression (n = 23, 11.7%) followed by septic shock (n = 14, 7.1%) and cardiorespiratory arrest (n = 11, 5.6%).
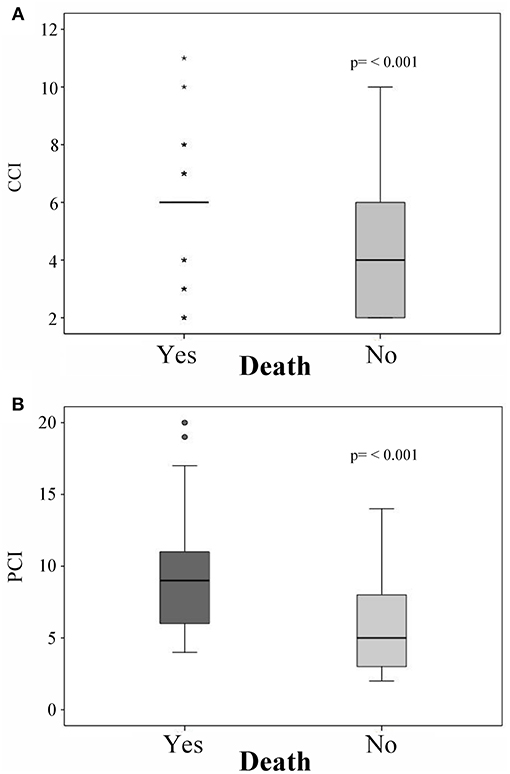
Figure 1. Mortality predictions with the (A) Charlson comorbidity index (CCI) and (B) Pediatric Comorbidity Index (PCI). *Represents an outlier above the 99th percentile or below the 1th percentile.
Mortality According to the Diagnosis
The highest number of deceased patients was found in the group of CNS tumors (27.57%), followed by retinoblastomas (21.4%), sarcomas (14.2%), osteosarcomas (12.86%), and Ewing sarcomas (10%).
Comorbidity Indexes as Prognostic Factors for Mortality
Differences in survival were found by using the two comorbidity indexes; 5-year survival for the CCI was 80% for patients with a low comorbidity category and 24% for those with a high comorbidity category. The 5-year survival for the PCI was 100% in the absence of comorbidity, 68% for low comorbidity, and 18% for high comorbidity. When survival curves according to CCI group (high comorbidity = 1 vs. low comorbidity = 2) within the months from diagnosis to the end of follow-up were compared, a statistically significant difference was found (p = 0.000066; Figure 2A). Similarly, a comparison of survival curves according to PCI group (high comorbidity = 1 vs. low comorbidity = 2 vs. absent comorbidity = 3) revealed a statistically significant difference with p = 0.000044 (Figure 2B).
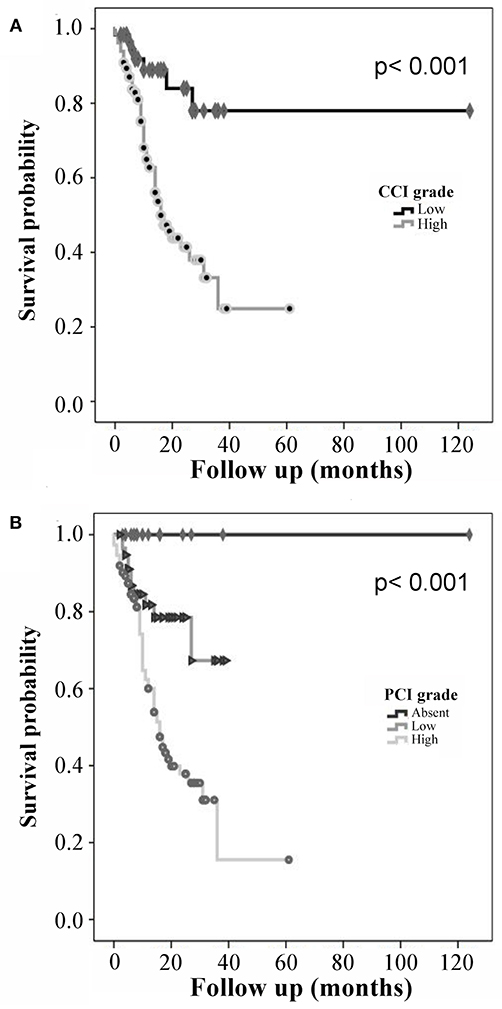
Figure 2. Kaplan-Meier curves for prediction of mortality: (A) Charlson comorbidity index (CCI) and (B) Pediatric Comorbidity Index (PCI).
Multivariate Cox Regression Models for Mortality
We found an increase in the number of deaths in patients who presented high comorbidity for both indexes. In a Cox survival model adjusted for gender and age, for every additional unit of PCI, the risk of death increased up to 20% (HR: 1.202; 95% CI: 1.078–1.341, p < 0.001; Table 2). We also observed a similar mortality risk for each unit added of CCI (HR: 1.152, 95% CI: 1.088–1.220, p = 0.001).
Discussion
This study found that high comorbidity burden acts as a mortality prognostic factor in a cohort of pediatric patients with solid tumors. To avoid possible interference or confusion from other factors, we chose patients who were treated with only chemotherapy. Interestingly, the results showed that the highest scores for both comorbidity indexes (PCI ≥ 6 and CCI ≥ 3) are associated with an increase in mortality when adjusted by gender and age.
The most frequent comorbidities found in patients with solid tumors were cellulitis, acute renal failure, and hypertension for the CCI; meanwhile, pathologies such as pneumonia, septicaemia, and malnutrition were the most frequent for the PCI. An important observation in our study is the fact that the CCI was categorized as two comorbidity groups (high and low), while the PCI as 3 (absent, low, and high).
The presence of comorbidities in a disease increases the risk of complications, including death, impacting on clinical practice and hospital care costs. Williams et al. (20) showed that the incidence of comorbidities increases with age, and this is a subject of growing interest in the adult populations. Extermann (21) published that older cancer patients have an average of 3 comorbidities. Comorbidities have an impact on survival, disease progression and treatment in cancer patients, which proves that comorbidities negatively affect patient survival (22). It has been observed in adults that the older the patient is, the greater the comorbidity burden, and the most frequent comorbidities in adult cancer patients are cardiovascular diseases, infections, liver disorders and early malignancy (23, 24).
Currently, there are several indexes available to measure comorbidity conditions as mortality prognosis; however, none of these are designed for pediatric patients. In a retrospective study by Savic et al. (24) that included 233 adult patients with acute myeloid leukemia (AML) who were treated with intensive chemotherapy, the comorbidity index (comorbidity evaluation in adults-27-ACE-27) was able to predict survival in patients with several hematologic malignancies. Additionally, Wass et al. (25) confirmed in a study of 194 adult patients with AML, that comorbidities have a significant impact on the survival of patients and that a pre-treatment evaluation of comorbidities can help to identify those patients with unfavorable outcomes.
The comorbidity evaluation is considered of value to predict mortality in different groups of patients (26–29), and it may aid in decision- making, which can potentially lead to lower costs of care in pediatric patients with solid tumors.
CCI is the most commonly used comorbidity index validated in adult patients, but in our opinion, it does not appropriately represent the comorbidity burden found in pediatric patients. PCI based evaluation of comorbidity introduces 3 risk-group categories. While we acknowledge that this result is debatable, given that it is based on a subjective weighted score granted by an expert panel, it is significant that this method evaluates comorbidity burden in the early ages of life. This is done by taking into account the pathologies associated with mortality risk in hospitalized pediatric patients upon diagnosis.
We found similar results for both indexes, since for each comorbidity, the risk of mortality significantly increases by 15% (PCI) to 20% (CCI) when adjusting for age and gender. Age has a significant impact on the survival of patients, as reported in previous studies (30–32). Interestingly, in our study, the one-year increment in age was a statistically significant protective factor against mortality in the PCI, while gender showed no significance in either index.
The limitations for this study include aspects related to some retrospective data collection. We cannot exclude the possibility that the observed differences among categories in our analysis could be enhanced by differences between scoring methods for the PCI and CCI; however, we consider our findings valid because the evaluation of the comorbidity burden was strictly realized in both groups of the population studied. Sample size may also be considered a limitation, due to low prevalence of pediatric tumors; however, we consider it adequate and representative of patients managed at our tertiary pediatric hospital, who met the inclusion criteria during the study period. We acknowledge our findings require external replication in other pediatric populations.
Conclusions
Comorbidities may affect treatment decisions, prognosis, and the evaluation of the quality of the patients' care. The comorbidity degrees of the PCI seem to facilitate better discrimination of the survival probability than the comorbidity degrees established in the CCI for pediatric cancer patients. The application of this index to the hospitalized pediatric population can be very useful for a baseline clinical evaluation that allows better decision-making during clinical follow-up.
Data Availability
The datasets for this manuscript are not publicly available because of data confidentiality. Requests to access the datasets should be directed tobWNhc3RpbGxlam9zQGdtYWlsLmNvbQ==.
Author Contributions
LT-E and MC-L designed study. JD-R, CG-D, and MP-G performed follow-up of the patients and recorded the information. LT-E, AA-G, and MC-L analyzed the data, and wrote the manuscript. JG-M, LC-A and IT-R the manuscript and revised it. LT-E, MC-L, JD-R, CG-D, MP-G, AA-G, JG-M, IT-R, LC-A and JC-P reviewed the final version of the manuscript.
Funding
This study was funded by National Council for Science and Technology (CONACYT), with grant number FOSISS/262406.
Conflict of Interest Statement
The authors declare that the research was conducted in the absence of any commercial or financial relationships that could be construed as a potential conflict of interest.
Acknowledgments
The authors would like to thank social service student Paulina Serrano Licea for helping to review records.
References
1. Gupta S, Rivera-Luna R, Ribeiro RC, Howard SC. Pediatric oncology as the next global child health priority: the need for national childhood cancer strategies in low- and middle-income countries. PLoS Med. (2014) 11:e1001656. doi: 10.1371/journal.pmed.1001656
2. Rivera-Luna R, Cárdenas-Cardós R, Olaya-Vargas A, Shalkow-Klincovstein J, Pérez-García M, Pérez-González OA, et al. El niño de población abierta con cáncer en México. Consideraciones epidemiológicas. Anales Médicos (2015) 60:91–7.
3. Wheatley-Price P, Blackhall F, Thatcher N. The influence of sex in non-small cell lung cancer. Onkologie (2009) 32:547–8. doi: 10.1159/000235609
4. Shugarman LR, Mack K, Sorbero ME, Tian H, Jain AK, Ashwood JS, et al. Race and sex differences in the receipt of timely and appropriate lung cancer treatment. Med Care (2009) 47:774–81. doi: 10.1097/MLR.0b013e3181a393fe
5. Aarts MJ, Voogd AC, Duijm LE, Coebergh JW, Louwman WJ. Socioeconomic inequalities in attending the mass screening for breast cancer in the south of the Netherlands–associations with stage at diagnosis and survival. Breast Cancer Res Treat (2011) 128:517–25. doi: 10.1007/s10549-011-1363-z
6. Hill S, Sarfati D, Blakely T, Robson B, Purdie G, Chen J, et al. Survival disparities in Indigenous and non-indigenous New Zealanders with colon cancer: the role of patient comorbidity, treatment and health service factors. J Epidemiol Community Health (2010) 64:117–23. doi: 10.1136/jech.2008.083816
7. Frederiksen BL, Osler M, Harling H, Ladelund S, Jorgensen T. Do patient characteristics, disease, or treatment explain social inequality in survival from colorectal cancer? Soc Sci Med. (2009) 69:1107–15. doi: 10.1016/j.socscimed.2009.07.040
8. Dalton SO, Ross L, During M, Carlsen K, Mortensen PB, Lynch J, et al. Influence of socioeconomic factors on survival after breast cancer–a nationwide cohort study of women diagnosed with breast cancer in Denmark 1983-1999. Int J Cancer (2007) 121:2524–31. doi: 10.1002/ijc.22979
9. Gijsen R, Hoeymans N, Schellevis FG, Ruwaard D, Satariano WA, van den Bos GA. Causes and consequences of comorbidity: a review. J Clin Epidemiol. (2001) 54:661–74. doi: 10.1016/S0895-4356(00)00363-2
10. Newschaffer CJ, Bush TL, Penberthy LT. Comorbidity measurement in elderly female breast cancer patients with administrative and medical records data. J Clin Epidemiol. (1997) 50:725-33. doi: 10.1016/S0895-4356(97)00050-4
11. Kieszak SM, Flanders WD, Kosinski AS, Shipp CC, Karp H. A comparison of the Charlson comorbidity index derived from medical record data and administrative billing data. J Clin Epidemiol. (1999) 52:137–42. doi: 10.1016/S0895-4356(98)00154-1
12. Yancik R, Wesley MN, Ries LA, Havlik RJ, Long S, Edwards BK, et al. Comorbidity and age as predictors of risk for early mortality of male and female colon carcinoma patients: a population-based study. Cancer (1998) 82:2123–34.
13. Rochon PA, Katz JN, Morrow LA, McGlinchey-Berroth R, Ahlquist MM, Sarkarati M, et al. Comorbid illness is associated with survival and length of hospital stay in patients with chronic disability. A prospective comparison of three comorbidity indices. Med Care (1996) 34:1093–101. doi: 10.1097/00005650-199611000-00004
14. Liu M, Domen K, Chino N. Comorbidity measures for stroke outcome research: a preliminary study. Arch Phys Med Rehabil. (1997) 78:166–72. doi: 10.1016/S0003-9993(97)90259-8
15. Charlson ME, Pompei P, Ales KL, MacKenzie CR. A new method of classifying prognostic comorbidity in longitudinal studies: development and validation. J Chronic Dis. (1987) 40:373–83. doi: 10.1016/0021-9681(87)90171-8
16. Quan H, Li B, Couris CM, Fushimi K, Graham P, Hider P, et al. Updating and validating the Charlson comorbidity index and score for risk adjustment in hospital discharge abstracts using data from 6 countries. Am J Epidemiol. (2011) 173:676–82. doi: 10.1093/aje/kwq433
17. Charlson M, Wells MT, Ullman R, King F, Shmukler C. The charlson comorbidity index can be used prospectively to identify patients who will incur high future costs. PLoS ONE (2014) 9:e112479. doi: 10.1371/journal.pone.0112479
18. Rivera R. Protocols of Seguro Popular Which are Based on Childrens Oncology Group Guidelines. México: Editores de textos Mexicanos, S.A de C.V (2010).
19. Tai D, Dick P, To T, Wright JG. Development of pediatric comorbidity prediction model. Arch Pediatr Adolesc Med. (2006) 160:293–9. doi: 10.1001/archpedi.160.3.293
20. Williams GR, Mackenzie A, Magnuson A, Olin R, Chapman A, Mohile S, et al. Comorbidity in older adults with cancer. J Geriatr Oncol. (2016) 7:249–57. doi: 10.1016/j.jgo.2015.12.002
21. Extermann M. Interaction between comorbidity and cancer. Cancer Control (2007) 14:13–22. doi: 10.1177/107327480701400103
22. Piccirillo JF, Tierney RM, Costas I, Grove L, Spitznagel EL. Prognostic importance of comorbidity in a hospital-based cancer registry. J Am Med Assoc. (2004) 291:2441–7. doi: 10.1001/jama.291.20.2441
23. Tawfik B, Pardee TS, Isom S, Sliesoraitis S, Winter A, Lawrence J, et al. Comorbidity, age, and mortality among adults treated intensively for acute myeloid leukemia (AML). J Geriatr Oncol. (2016) 7:24–31. doi: 10.1016/j.jgo.2015.10.182
24. Savic A, Kvrgic V, Rajic N, Urosevic I, Kovacevic D, Percic I, et al. The hematopoietic cell transplantation comorbidity index is a predictor of early death and survival in adult acute myeloid leukemia patients. Leuk Res. (2012) 36:479–82. doi: 10.1016/j.leukres.2011.11.021
25. Wass M, Hitz F, Schaffrath J, Muller-Tidow C, Muller LP. Value of different comorbidity indices for predicting outcome in patients with acute myeloid leukemia. PLoS ONE (2016) 11:e0164587. doi: 10.1371/journal.pone.0164587
26. van Spronsen DJ, Janssen-Heijnen ML, Breed WP, Coebergh JW. Prevalence of co-morbidity and its relationship to treatment among unselected patients with Hodgkin's disease and non-Hodgkin's lymphoma, 1993-1996. Ann Hematol. (1999) 78:315–9. doi: 10.1007/s002770050521
27. Yancik R, Wesley MN, Ries LA, Havlik RJ, Edwards BK, Yates JW. Effect of age and comorbidity in postmenopausal breast cancer patients aged 55 years and older. J Am Med Assoc. (2001) 285:885–92. doi: 10.1001/jama.285.7.885
28. Wells CK, Stoller JK, Feinstein AR, Horwitz RI. Comorbid and clinical determinants of prognosis in endometrial cancer. Arch Intern Med. (1984) 144:2004–9. doi: 10.1001/archinte.1984.04400010120020
29. Fleming ST, Rastogi A, Dmitrienko A, Johnson KD. A comprehensive prognostic index to predict survival based on multiple comorbidities: a focus on breast cancer. Med Care (1999) 37:601–14. doi: 10.1097/00005650-199906000-00009
30. Hsu CL, Chen JH, Chen KY, Shih JY, Yang JC, Yu CJ, et al. Advanced non-small cell lung cancer in the elderly: the impact of age and comorbidities on treatment modalities and patient prognosis. J Geriatr Oncol. (2015) 6:38–45. doi: 10.1016/j.jgo.2014.09.178
31. Pal SK, Hurria A. Impact of age, sex, and comorbidity on cancer therapy and disease progression. J Clin Oncol. (2010) 28:4086–93. doi: 10.1200/JCO.2009.27.0579
Keywords: charlson comorbidity index, solid tumor, pediatric, cancer, chemotherapy, mortality
Citation: Torres-Espíndola LM, Demetrio-Ríos J, Carmona-Aparicio L, Galván-Díaz C, Pérez-García M, Chávez-Pacheco JL, Granados-Montiel J, Torres-Ramírez de Arellano I, Aquino-Gálvez A and Castillejos-López MDJ (2019) Comorbidity Index as a Predictor of Mortality in Pediatric Patients With Solid Tumors. Front. Pediatr. 7:48. doi: 10.3389/fped.2019.00048
Received: 14 November 2018; Accepted: 05 February 2019;
Published: 01 March 2019.
Edited by:
Douglas Hawkins, Seattle Children's Hospital, United StatesReviewed by:
Hema Dave, Children's National Health System, United StatesAnshu Malhotra, Emory University, United States
Copyright © 2019 Torres-Espíndola, Demetrio-Ríos, Carmona-Aparicio, Galván-Díaz, Pérez-García, Chávez-Pacheco, Granados-Montiel, Torres-Ramírez de Arellano, Aquino-Gálvez and Castillejos-López. This is an open-access article distributed under the terms of the Creative Commons Attribution License (CC BY). The use, distribution or reproduction in other forums is permitted, provided the original author(s) and the copyright owner(s) are credited and that the original publication in this journal is cited, in accordance with accepted academic practice. No use, distribution or reproduction is permitted which does not comply with these terms.
*Correspondence: Luz María Torres-Espíndola, bHV6bXRvcnJlc0BnbWFpbC5jb20=
Manuel De Jesús Castillejos-López, bWNhc3RpbGxlam9zQGdtYWlsLmNvbQ==