Current Status and Future Directions of Neuromonitoring With Emerging Technologies in Neonatal Care
- 1Division of Neonatology, Department of Pediatrics, Irmandade de Misericordia da Santa Casa de São Paulo, São Paulo, Brazil
- 2Clinical Research Department, Protecting Brains and Saving Futures Organization, São Paulo, Brazil
- 3Division of Neonatology, Grupo Santa Joana, São Paulo, Brazil
- 4Data Science Department, OPD Team, São Paulo, Brazil
- 5Pediatric Nursing Department, Escola Paulista de Enfermagem, Universidade Federal de São Paulo, São Paulo, Brazil
- 6Department of Pediatrics, Faculdade de Ciências Médicas da Santa Casa de São Paulo, São Paulo, Brazil
- 7Department of Pathology, Faculdade de Ciências Médicas da Santa Casa de São Paulo, São Paulo, Brazil
- 8Department of Pediatrics, Irmandade da Santa Casa de Misericórdia de São Paulo, São Paulo, Brazil
Neonatology has experienced a significant reduction in mortality rates of the preterm population and critically ill infants over the last few decades. Now, the emphasis is directed toward improving long-term neurodevelopmental outcomes and quality of life. Brain-focused care has emerged as a necessity. The creation of neonatal neurocritical care units, or Neuro-NICUs, provides strategies to reduce brain injury using standardized clinical protocols, methodologies, and provider education and training. Bedside neuromonitoring has dramatically improved our ability to provide assessment of newborns at high risk. Non-invasive tools, such as continuous electroencephalography (cEEG), amplitude-integrated electroencephalography (aEEG), and near-infrared spectroscopy (NIRS), allow screening for seizures and continuous evaluation of brain function and cerebral oxygenation at the bedside. Extended and combined uses of these techniques, also described as multimodal monitoring, may allow practitioners to better understand the physiology of critically ill neonates. Furthermore, the rapid growth of technology in the Neuro-NICU, along with the increasing use of telemedicine and artificial intelligence with improved data mining techniques and machine learning (ML), has the potential to vastly improve decision-making processes and positively impact outcomes. This article will cover the current applications of neuromonitoring in the Neuro-NICU, recent advances, potential pitfalls, and future perspectives in this field.
Introduction
Despite recent breakthroughs in perinatal care, impaired outcomes in newborns at high risk for brain injury remain common, posing a challenge in neonatal care and public health (1, 2).
Neonatal encephalopathy (NE) and perinatal stroke in term infants, as well as the repercussions of germinal matrix-intraventricular hemorrhage (IVH) and white matter injury (WMI) in extremely low birth weight preterm infants, are the most frequent illnesses. Encephalopathy and seizures may also occur due to metabolic and uncommon genetic disorders. All these infants are at high risk of developing neurological impairment, including cerebral palsy, cognitive delay, epilepsy, and other neurological disabilities (3).
No single intervention can reduce newborn brain injury because of the complex interactions between pathological processes, such as inflammation, oxidative stress, developmental trajectory, genetic susceptibility, and environmental influence. However, new approaches focusing on early diagnosis of brain injury together with neuroprotective strategies may improve outcomes. This philosophy has motivated the creation of the neonatal neurocritical intensive care unit (Neuro-NICU) model (4). One of the critical approaches of a Neuro-NICU is continuous brain monitoring, which has been increasingly used to assess brain health in neonates. Continuous EEG (cEEG), amplitude-integrated electroencephalography (aEEG), and near-infrared spectroscopy (NIRS) are non-invasive techniques used at the bedside to evaluate brain function, screen for seizures, and measure regional tissue oxygenation.
The rapid advancement of technology in the NICU may combine the increasing use of telemedicine and artificial intelligence to potentially improve decision-making processes and impact patient outcomes (5, 6). Using these techniques in an integrated manner, also known as multimodal monitoring, may allow practitioners to better understand the physiological processes occurring in critically ill neonates (7). This article will discuss the current applications of neuromonitoring and recent advancements, potential pitfalls, and future perspectives in this field.
Neonatal Brain Injury
Gestational age continues to be the most critical factor in determining the long-term effects of brain injury, which is complex and multifactorial (8). WMI is the most common type of brain injury in preterm newborns, and it can be found in up to 50% of infants born with very low birth weight (9, 10). However, the spectrum of periventricular WMI has shifted from the severe and commonly cystic lesions toward a milder pattern of WMI as a result of improvements in neonatal care (11). In extremely preterm population, the incidence of all grades of IVH ranges from 31 to 36%, while severe IVH has an incidence between 10 and 17% (12–14). Although brain injury is not always the primary event in the life of a preterm infant, the consequences of preterm birth and associated comorbidities have a significant impact on brain development.
In term infants, perinatal asphyxia and hypoxic-ischemic encephalopathy (HIE) represent the most common cause of brain injury and disability, with an incidence ranging from 1 to 2 per 1,000 live births in high-income countries and significantly higher in low-resource settings (15, 16). Despite being commonly under-diagnosed in the NICU, perinatal stroke has an incidence of approximately 2.5 per 1,000 live births. It is the second most common cause of neonatal seizures and the most common cause of childhood hemiplegia (17).
Strategies for Neuroprotection and the Neuro-NICU Concept
Following decades of concentrated efforts to improve neonatal intensive care and newborn survival, the focus has shifted to examining the long-term outcomes in this population.
As the standard treatment for infants with HIE, cooling is a significant advance in the field and has effectively reduced the combined risk of death or disability (18, 19). However, there are also several different neurological diseases present throughout the neonatal period, in which early recognition and diagnosis may have a significant impact on disease progression.
The interdisciplinary approach to newborn brain injury care is not new, with numerous units collaborating closely with pediatric neurologists, neurosurgeons, and other professionals. However, the introduction of therapeutic hypothermia represented a paradigm shift that required a concerted approach to identifying and managing patients with NE. The University of California, San Francisco (UCSF) was the first unit in the United States to establish a neonatal neurointensive care nursery (4). This strategy has acquired significant popularity globally during the last decade, with several teams describing their experience establishing similar models of care (20–22).
The objective is to combine advances in neuromonitoring and imaging with novel treatments to enhance neurodevelopmental outcomes. Numerous patient subgroups were identified as possibly benefiting from this therapy approach: term infants with HIE and seizures, extremely low birth-weight preterm infants, and newborns with congenital or uncommon neurological disorders. Additionally, four strategies were developed during the creation of Neuro-NICU programs: (i) infant co-management; (ii) standardized protocols and care bundles; (iii) wider neuromonitoring and neuroimaging utilization; and (iv) establishment of training programs (23–25).
The Existing Neuromonitoring Tools in the NICU
aEEG and cEEG
The use of aEEG represents a non-invasive, bedside, and simplified method of continuous brain monitoring, mainly accessed by the neonatologist, which has been increasingly used to assess brain function. Similar to cEEG, it records differences in electrical potentials and changes in electrical activity displayed over time. Then, after being filtered for frequency, the activity is time-compressed, rectified, smoothed, and displayed in a 6 cm/h chart semi-logarithmically.
Compared with the cEEG, the aEEG has the advantage of being more straightforward and quicker to apply. It can be used to evaluate the background activity, sleep-wake cycling, and seizure screening in critically ill neonates as a continuous bedside monitor (26–31).
Persistent pathological background activity and absence of sleep-wake cycling in the first days/weeks of life were associated with higher risk of neurological impairment at 2 years of age in infants with HIE and the preterm population (28, 32–34). On the other hand, the presence of continuous background activity and sleep-wake cycling within the first postnatal week has been associated with a good neurodevelopmental outcome (33).
Klebermass et al. study evaluated 143 preterm infants with gestational age below 30 weeks during the first 2 weeks of life. The aEEG was classified into a graded score according to background activity, appearance of sleep-wake cycling, seizure activity and correlated with neurological outcomes at 3 years of age. Specificity and sensitivity were 95 and 83% respectively in aEEG findings in the second week of life and were superior compared to cranial ultrasound findings (34).
The neonatal period, particularly the first week after birth, is the most susceptible time of human life for seizure development. Seizures are commonly related to acute brain injury, are associated with increased mortality and impairment, and may constitute a neurological emergency, making monitoring and accurate seizure identification critical components of newborn intensive care management (35–37). Higher seizure burden in the neonatal period is commonly associated with abnormal neurological outcomes (38). A clinical trial conducted by Srinivasakumar et al. compared newborns with HIE treated for electrographic seizures with newborns treated only for clinical seizures. The authors found that the treatment of electrographic seizures reduced the seizure burden, severity of MRI findings and improved neurodevelopmental outcomes (39).
Several studies have shown that using two-channel aEEG associated with raw EEG interpretation improves seizure detection accuracy (26, 35–37, 40–44). Seizure activity is characterized by a sudden change on aEEG background activity as an abrupt rise in minimum and maximum amplitudes correlated with an evolving, stereotypical, and rhythmic wave pattern, with repeating forms such as spikes or sharp waves seen in raw EEG, with a total duration of at least ten seconds (45). The aEEG seizures are classified into (a) single seizures (SS): one electrographic seizure per each epoch; (b) repetitive seizures (RS): more than one electrographic seizure per epoch but less than one electrographic seizure over a 10 min period; (c) status epilepticus (SE) in the neonate. SE is defined by: (a) continuous seizure activity for at least 30 min or (b) recurrent seizures for over 50% of the recording time ranging from 1 to 3 h (42).
Figure 1 displays an example of seizures shown on two-channel aEEG and raw EEG.
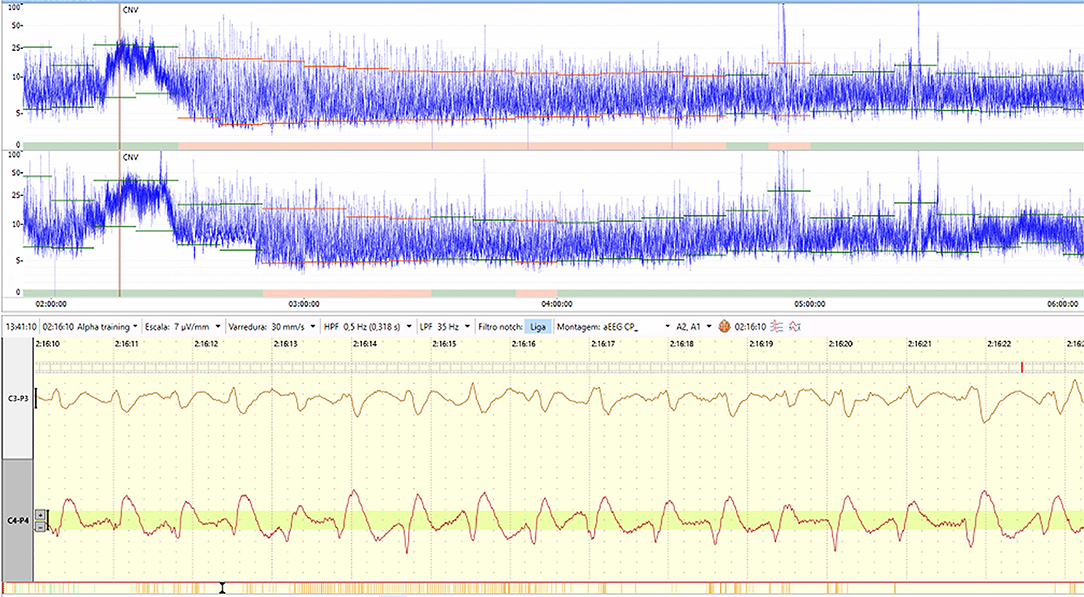
Figure 1. An example of seizures displayed on two-channel aEEG and raw EEG. Figure from the authors' personal file.
Other high-risk populations, such as infants born with congenital heart diseases (CHD), stroke, metabolic disorders, inborn errors of metabolism (IEM), cerebral malformations, and congenital infections, may benefit from this screening tool (46–51). Gunn et al. evaluated a large cohort of 150 newborn infants undergoing cardiac surgery who underwent aEEG monitoring in the perioperative period (prior to and during surgery, and for 72 h postoperatively). Perioperative electrical seizures were found in 30%, of whom 1/4 had any clinical correlation. Failure to recover to a continuous background aEEG by 48 postoperative hours was associated with impairment in all outcome domains and abnormal aEEG at seven postoperative days was highly associated with mortality (46).
The cEEG is a noninvasive procedure performed using electrodes attached to the scalp surface in accordance with the international 10–20 system (47) and is the gold standard tool for seizure diagnosis in the NICU (48). The American Clinical Neurophysiological Society (ACNS) has published recommendations on the use of full-montage electroencephalography (52). Although the idea of the Neuro-NICU has been around for over a decade, the use of extended cEEG is not widespread, with only between 35 and 67% of NICUs reporting its use, indicating an implementation problem (18).
NIRS
NIRS is a non-invasive technique for monitoring regional tissue oxygenation continuously at the bedside. It can be used as a trend monitor in critically sick newborns to assess the balance of tissue oxygen delivery and consumption, providing cerebral and somatic oximetry readings and enabling early detection of hemodynamic alterations (53). Near-infrared light emitted from a light source on a sensor penetrates the infants' skin and tissue. It is partially absorbed by oxygenated and deoxygenated hemoglobin before being reflected to a detector on the same sensor. After that, a tissue saturation level (rSO2) is determined based on arterial to venous blood (25:75) ratio and the balance of oxygen delivery and consumption in the underlying tissue.
Regional cerebral oxygen saturation (rScO2) may be easily measured with a sensor placed on the forehead, and readings are verified against jugular venous saturations in newborns (54). The interpretation of rScO2 readings requires consideration of other factors that may impact cerebral blood flow and oxygenation, including systemic oxygenation (SpO2), cardiac output, anemia, carbon dioxide (CO2) tension, glucose levels, and metabolic demand. In an extensive multi-center investigation of the preterm population, normal rScO2 levels were shown to range between 55 and 85% (55). Cerebral fractional tissue oxygen extraction (cFTOE) represents the balance between oxygen supply and consumption and is computed using the formula cFTOE = (SpO2 – rScO2) / (SpO2) (56).
Sustaining rScO2 levels below 40–50% has been shown to cause cellular, physiological, biochemical, and neuroradiographic brain damage in animal models and infant studies. Hippocampal histology revealed that hypoxic piglets exposed to rScO2 40% for at least 30 mins showed mitochondrial damage and signs of fragmented cellular structure (57). In a study of newborns with hypoplastic left heart syndrome who underwent a Norwood surgical procedure, those with rScO2 <45% for over 180 cumulative minutes had a higher risk of developing new or progressive ischemic abnormalities on brain magnetic resonance imaging (58).
In order to implement a NIRS neuromonitoring program, a minimum rScO2 threshold and management protocol must be established, so the healthcare team can provide interventions to avoid long-term injury. Causes of abnormal cerebral oxygen supply or demand need to be investigated, including hypocarbia, hypotension, anemia, and low arterial saturation. High tissue oxygen demand in infants with seizures, pain, fever, or infections may also decrease rScO2 values.
Several studies highlight the value of two-site NIRS monitoring with brain and somatic measures. Common sites for somatic monitoring include renal or mesenteric/splanchnic regions. Renal regional oxygen saturation (rSrO2) values are measured by sensor placement on the posterior flank below the costal margin and above the iliac crest. rSrO2 values are sensitive to compromise of systemic blood flow and usually are 10–15% higher than cerebral saturation (59–61). Due to preservation of the cerebral autoregulatory mechanism, hemodynamic compromise typically results in an earlier reduction of somatic oxygenation prior to changes in rScO2 (60). Mesenteric regional saturations (mScO2) are significantly lower in preterm infants and may fluctuate considerably from 32 to 82 percent (62). Due to its higher variability and more challenging interpretation, mScO2 is most commonly applied in research settings.
NIRS monitoring has been shown to be an effective biomarker for early organ dysfunction in many conditions occurring in neonates admitted to the NICU. Additionally, early investigations associated NIRS results with worse short- and long-term outcomes in severely ill infants (62, 63). NIRS has been reported as a valuable technique for monitoring patients following stage 1 palliation for hypoplastic left heart syndrome (HLHS) and demonstrated to improve prediction of neurodevelopmental outcomes at two years of age in newborns with CHD (63, 64). Preoperatively, babies with HLHS who were monitored with NIRS required less mechanical ventilation and inspired nitrogen gas than a historical cohort who were not followed with NIRS (65).
Similarly, in the preterm population, the SafeBoosC consortium recently completed a phase II randomized controlled trial. It examined extremely preterm infants during their first 72 h of life and established the feasibility and efficacy of implementing NIRS continuous monitoring in conjunction with a dedicated treatment guideline (66). Compared to the control group, infants in the NIRS-monitored group exhibited a reduced prevalence of cerebral hypoxia or hyperoxia. Although the study was not powered to detect differences in clinical outcomes, the NIRS-monitored group also tended toward reduced mortality and severe intraventricular hemorrhage.
Also, NIRS may be useful to verify the presence of a hemodynamic significant patent ductus arteriosus (hsPDA). A hsPDA contributes to low cerebral blood flow (CBF) during left to right shunting early in transition when the left ventricle cannot compensate (67). The renal oxygen saturation is decreased and a higher variability is seen. Chock et al. (68) found that renal saturation (rSrO2 < 66%) was associated with the presence of an hsPDA and studies were able to detect an increase in cerebral and renal saturation after successful treatment for PDA closure (67). A systematic review conducted by Prescott et al. concluded that NIRS could help clinicians identify hsPDAs and provide information on organ perfusion that may guide treatment decisions (67).
Previously, studies compared rScO2 and cFTOE findings before and after drainage, in newborns with post hemorrhagic ventricular dilatation (PHVD). The authors concluded that PHVD is associated with a significant decrease in rScO2 and increase in cFTOE due to a decrease in cerebral perfusion. Therefore, NIRS could potentially be a useful biomarker to determine the optimal time point for ventricular decompression (69, 70).
NIRS monitoring has been shown to be useful to evaluate tissue oxygenation in a set of clinical scenarios in the NICU. However, more extensive studies are needed to establish that NIRS monitoring effectively improves outcomes.
Multimodal Brain Monitoring
According to research in adults and children, multimodal monitoring facilitates the diagnosis and prevention of neurological repercussions in the setting of hemodynamic compromise (71). Simultaneous use of NIRS and aEEG may aid in comprehending the physiology of hemodynamic changes and the risk of cerebral injury.
Several studies described their correlations, especially in HIE. For cooled infants, an abnormal aEEG at 48–72 h and higher tissue oxygenation measured by NIRS at 12–24 h of life is associated with adverse outcomes (62, 72–74). In a cohort of 39 term infants with HIE, Lemmers et al. investigated the predictive value of dual-use of aEEG and NIRS. Combining NIRS and aEEG data enhanced the positive predictive value (PPV) (NIRS 67 % and aEEG 62% vs. combined 91%) and negative predictive value (NPV) (73 and 100% vs. 100%) at 12–18 h of age when compared to either modality alone (62).
Other conditions may also benefit from this combined approach (74). Our group recently published a case review study for this integrated approach in four clinical scenarios, including HIE, hemodynamic instability, patent ductus arteriosus, and seizures (7). Several studies also showed that brain monitoring with aEEG and NIRS is feasible in the delivery room and may provide information on newborns' condition during immediate transition and resuscitation (75, 76). In the Neu-Prem Trial, Katheria et al. evaluated 127 newborns under 32 weeks of gestational age to determine whether NIRS and aEEG can predict infants at risk for IVH and death in the first 72 h of life. The aEEG was not predictive of IVH or death, but NIRS may be used to predict severe IVH and early death (77). Those 127 evaluated newborns were followed out between 22 and 26 months of corrected age in the follow up clinic to assess for neurodevelopmental impairment or death. Authors found that increased duration of hypoxia in infants born under 32 weeks was associated with neurodevelopmental impairment or death (78).
A published recommended neuromonitoring approach for newborns in the NICU is displayed in Table 1. The duration of each brain monitoring approach should be related to periods of higher risk for seizures and infants' clinical instability.
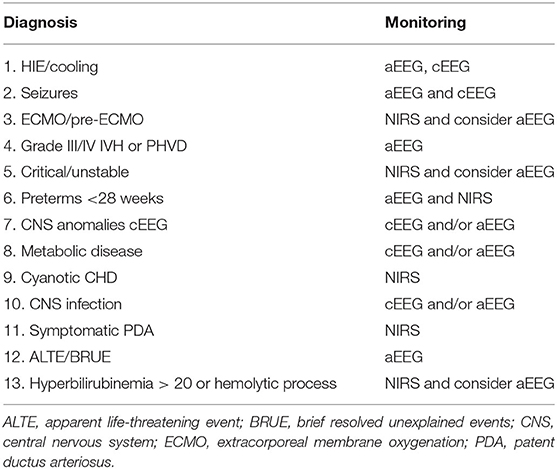
Table 1. Neuro-NICU eligibility and recommended neuromonitoring [adapted from Van Meurs et al. (20)].
A multimodal approach can combine these dual brain monitoring techniques with other vital signs and clinical information to study systemic and cerebral hemodynamics and electrographic findings early after birth, allowing a better understanding of the critically ill infant physiology.
A high degree of illness severity, along with limited handling to prevent oscillations in cerebral blood flow, may make multimodal monitoring challenging to apply. Additionally, the small head size, delicate skin, ventilatory support, and high ambient incubator humidity may complicate sensor placement. However, Deshpande et al. presented an early study using a multimodal strategy to examine systemic and cerebral hemodynamics and electrical changes shortly after birth using echocardiogram (ECHO), NIRS, and aEEG to identify infants at risk of IVH. This study established that this is a safe and well-tolerated technique associated with a low risk of adverse events (79).
Physiologic information collected by multimodal monitoring may enable the development of neuroprotective strategies. Algorithms for treatment may be based on measures for preventing cerebral hypo- and hyperperfusion. Such an approach may include oxygen delivery modification, ventilatory changes to avoid hypo- or hypercarbia, increasing cardiac output by volume expansion or inotropes, keeping hemoglobin concentrations within a particular range, and PDA closure. Figures 2A,B represent examples of a multimodal approach combining different parameters.
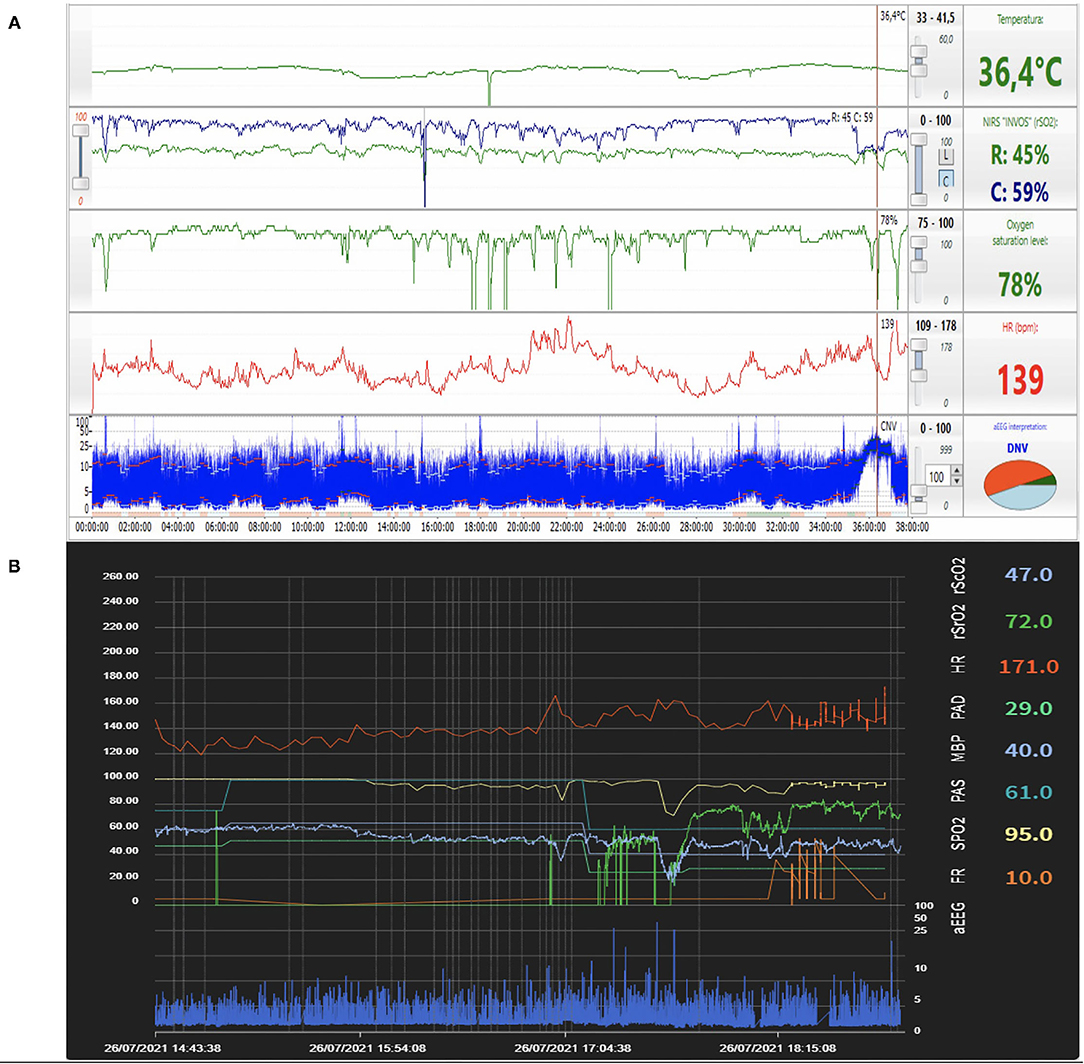
Figure 2. (A) Example of a multimodal approach combining aEEG, NIRS, pulse oximetry, heart rate, and temperature findings in real-time. During the seizure episode noted on aEEG (arrow), a decrease in rScO2 was observed together with significant fluctuations in pulse oximetry and heart rate. Figure from the authors' personal file. (B) Example of a multimodal approach combining aEEG, NIRS, pulse oximetry, heart rate findings in real-time in proprietary software developed using a specific programming language (Python). Correlation between aEEG (continuous low voltage pattern) and low rScO2 is observed. Figure from the authors' personal file.
Existing technology for brain monitoring in the NICU, mainly multimodal approach, provides a substantial quantity of data. Data must be stored in a suitable and safe system, with efficient processing and can be integrated with another system or equipment. The improvement of technological capacity generates a number of research possibilities and new perspectives to potentially improve the quality of care.
Data Integration and Processing
Commercially distributed aEEG/EEG and NIRS monitors are designed primarily for clinical application rather than long-term storage and analysis. Data collection is usually a secondary issue that requires extra planning, infrastructure, and financial resources.
The basics of data capture systems include the following: (a) synchronized gathering of vital signs, (b) precise time/date information, and (c) data loss prevention. Whatever technique is chosen, data is essential and should be collected at the greatest sampling rate feasible (80, 81).
Direct Download From a Local Device
The easiest method of obtaining data from brain monitoring equipment is by direct download. While this is the simplest method, it does have several disadvantages. Data is only accessible following the conclusion of a monitoring session. Data loss can occur as a consequence of power failure or unintentional deletion from the device. The limited memory capacity of each device may result in data files being overwritten if not retrieved on a regular basis. Second, this technique allows collecting a single monitoring data, and other vital signs are commonly omitted from the file. As a result, the data collected in this method must be externally reconciled with other vital signs data. Finally, the timestamp for this monitor data comes from the monitor's clock, which is frequently not updated for daylight savings time or is reset in a power failure.
Data Collection Through a Central Hub and Central Server
A central computer is required to coordinate the synchronized acquisition of physiological signals from numerous sources. Typically, a central-hub method involves placing a laptop computer at the patient's bedside directly linked to the patient monitors (including aEEG/EEG, NIRS, and vital signs monitor), the ventilator, or other equipment. This technique is helpful for time syncing because the computer supplies the timing data, and the program writes the data straight to disk, ensuring that previously recorded data is not lost. Additionally, some software packages offer integrated analytic tools and can serve as an accessible open path for inexperienced investigators. The primary downside of the central-hub model is that it typically needs extra hardware (laptops, computer, cabling) or software purchases and may impose limits due to device compatibility limitations.
The most commonly used protocols for data export and integration are Lab Stream Layer (LSL)—for EEG equipment—and Health Level Seven (HL7)—widely used in medical devices (e.g., vital signs monitor, electronic records, ventilators) (82, 83). Many other protocols are used by different devices, some unique according to the model and manufacturer. For this reason, when gathering data from multiple types of equipment, a tool to convert each data input into a structured format is needed. Programing languages like Python and Matlab are commonly used for the development of these tools (84–86).
A variation on this data collection approach is the central-server concept, in which separate monitoring devices are linked to a centrally placed server through a network. The technique retains the benefit of synchronized timing and adds the use of primarily automatic capture, requiring minimum human input to start and stop the recording and eliminating the requirement for a laptop at each patient's bedside. The main drawback is that it is the most complicated infrastructure option, frequently necessitating substantial capital expenditure.
Figure 3 represents an overview of data collection and integration for multimodal monitoring in the NICU.
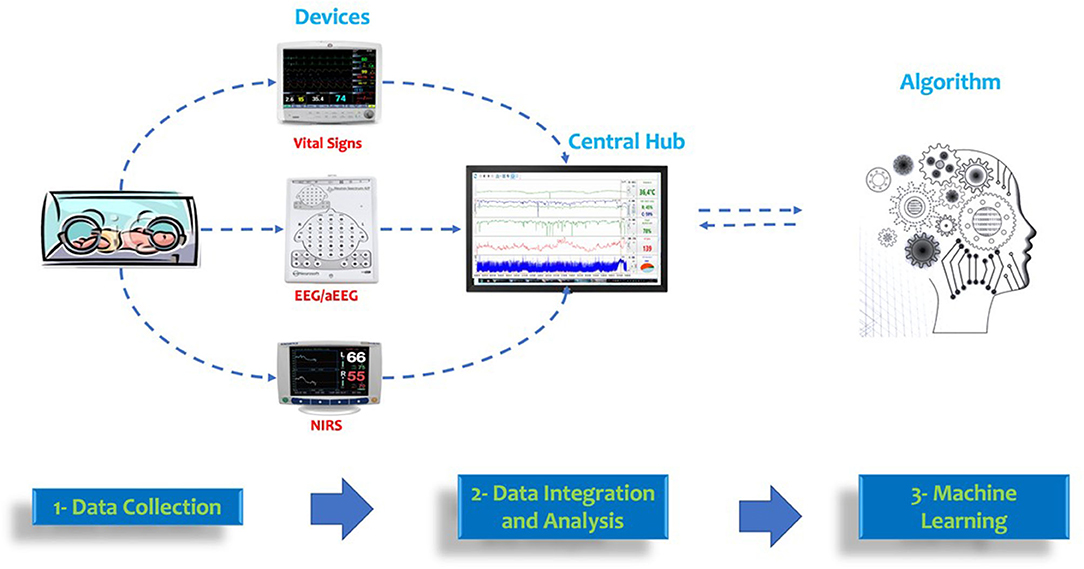
Figure 3. Overview of data collection and integration for multimodal monitoring in the NICU. Data from vital signs, EEG/aEEG, and NIRS devices can be integrated into a central hub. This data can be used for machine learning algorithms using continuous monitoring data.
Artificial Intelligence and Machine Learning
With the advancement in computing power, data storage, and processing capabilities, artificial intelligence (AI) enables computer systems to perform activities that would typically require human intelligence and that people deem “clever.” AI is a term that refers to a system that is endowed with human-like intellectual processes, such as the capacity to reason, discover meaning, generalize, and learn from prior experience (87).
AI has been used for drug development, individualized diagnostics and treatments, molecular biology, bioinformatics, and medical imaging in healthcare. Additionally, AI systems can decipher illness patterns by scrutinizing and analyzing enormous volumes of data in electronic medical records.
Machine learning (ML) is a subfield of artificial intelligence in which computer algorithms learn to build predictive models from a given dataset. The main difference between ML and classical statistics is that ML aims to create the best possible predictive model from a training dataset, leveraging a test dataset to validate its results. Statistics aims to infer and validate relationships between variables on the whole dataset and are not optimized for its predictive capabilities. There are two broad categories of ML frameworks: supervised and unsupervised.
Supervised Machine Learning
Supervised ML aims to create an algorithm capable of predicting an individual output given a specific input. In other words, the machine is shown instances of both input (x) and output (y), such that y = f(x). ML is dependent on large data sets containing several examples of how one or more input variables are related to a particular output. The expectation is that the resulting algorithm will make accurate predictions when confronted with new and previously unseen data. When big datasets are used to train and evaluate the system, supervised learning needs a significant amount of human labor (83).
Supervised learning can be used to predict both continuous and discrete values. For example, the expected heart rate of a patient in the next hour of monitoring is a continuous numerical value, and regression models should be used for prediction. In contrast, when building an automated seizure detection tool, the algorithm must learn from a binary input, either if a seizure is detected or not. For that kind of issue, classification models can be applied.
The choice of the model to apply to the desired task is a crucial step in developing predictive algorithms. For supervised learning, dozens of different models can be applied, including decision trees and deep learning, both widely used in the ICU for seizure detection, mortality prediction, phenotypic discovery, and disease prediction (88, 89).
Unsupervised Machine Learning
No definitions are provided to the algorithm on how to process the data in this method of ML. Thus, the computer is expected to extract information from a vast set of unclassified data using either a set of rules or an unknown output. Given the absence of label information, a significant problem for the investigator when evaluating an unsupervised algorithm is determining the usefulness of the findings or determining whether the desired output was obtained. In addition, explainability may be challenging. However, unsupervised algorithms may be sufficiently efficient in exploratory attempts to comprehend vast sets of data. Clustering, anomaly detection, and dimensionality reduction are the most often utilized approaches (88).
Clustering algorithms are assigned to identify or partition huge data sets into subgroups and patterns with common characteristics. The algorithm is tasked with detecting unusual patterns in the dataset, such as outliers. When analyzing data with a large number of features, or dimensions, dimensionality reduction is advantageous. These algorithms may simplify the data by summarizing its main features and making it more understandable to humans and other ML algorithms. A machine learning pipeline is shown in Figure 4.
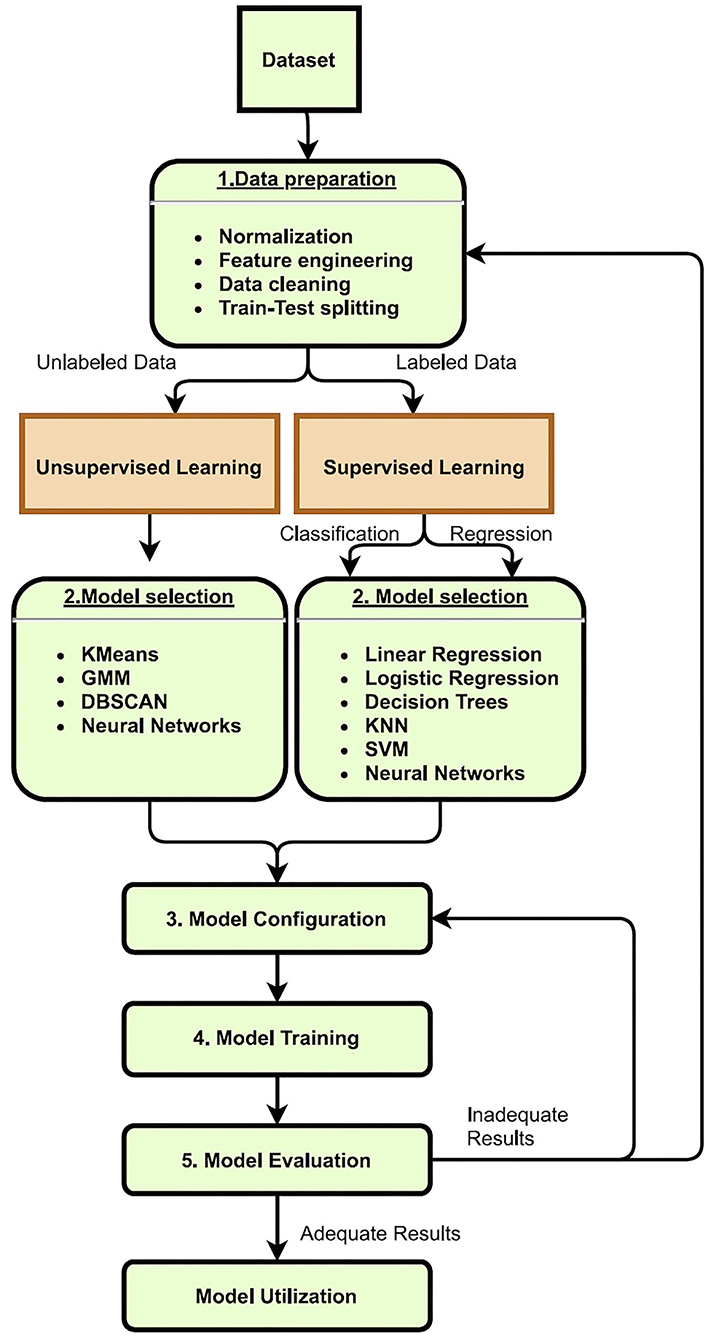
Figure 4. Machine learning pipeline: (1) Data preparation is the step where modifications to the dataset are made to improve the final results. That includes transforming, cleaning, and creating new features (feature engineering). In this step, the train-test data splitting can also be defined. (2) Model selection is the step where the appropriate model is chosen. That can significantly vary according to the dataset characteristics (like size and dimensionality) and the target variable. (3) Model configuration is the step in which all the parameters of the model must be set. (4) Model training is the step in which the machine finds the best-fitting predictive model according to the train dataset and model parameters. (5) Model evaluation is the step in which the user will analyze the results, mainly the train and test error of the generated predictive model.
AI Applications in Critical Care
There are multiple opportunities to implement AI in the medical context. Massive quantities of data contained in electronic medical records have been analyzed using unsupervised ML algorithms. Models have been created to extract critical information from medical files and identify individuals with a high cost of care (89). Supervised ML methods have demonstrated their value in radiography and histopathology due to their ability for automatic pattern detection of pictures (90). ML has been extensively utilized in the disciplines of surgery, particularly as it relates to robotics, cardiology, and cancer research to categorize tumor types and growth rates (91, 92).
Although ML is still in its inception in the ICU, multiple papers have already been published demonstrating its application in the care of critically ill patients. Several researchers have utilized massive population datasets to forecast duration of stay, ICU readmission, and death rates, as well as the likelihood of acquiring diseases such as sepsis or respiratory distress syndrome (93–95). Several other studies used smaller clinical and physiological datasets to help monitor patients receiving ventilatory support (96).
AI in the Neonatal ICU
The NICU is a place where life-altering decisions are constantly made. Neonatologists collect data from various sources to create a picture of a newborn's status to guarantee they receive the best medical treatment available. Highly trained professionals utilize their judgment in conjunction with a continuous stream of patient data to ensure that as many infants have the best possible outcome. The use of AI could enhance the decision-making process and lead to better outcomes. Below we describe some applications of AI in the NICU.
Diagnosis of HIE
Clinical diagnosis of asphyxia involves analysis of arterial blood gas and a standard neurological exam. Infants diagnosed with moderate to severe HIE meet the entry criteria for cooling, but new evidence show that neonates with mild HIE may also be at risk for disability (97). Precise identification of populations at high risk poses a clinical challenge in the field. A few approaches using AI have been studied to address this issue.
O'Boyle et al. published a study validating potential metabolites and evaluating their capacity to predict HIE independently and combined with clinical data. Term neonates with symptoms of perinatal asphyxia, with and without HIE, and matched controls were recruited prospectively at birth from two large centers. The study enrolled 511 infants, and by using logistic regression modeling and ML approaches, the optimal set of clinical and metabolite characteristics capable of predicting the development of HIE was found (98). Fifteen of twenty-seven potential metabolites revealed substantial changes in babies with perinatal asphyxia or HIE. Lactic acid and alanine were the significant metabolite predictors of HIE development, with an area under the curve (AUC) of 0.96 when combined with clinical data (95% CI, 0.92–0.95) (97).
EEG signal monitoring is known to be a valuable technique for identifying possible biomarkers of HIE (94). Over the last two decades, researchers throughout the world have developed a range of signal processing techniques for automated analysis and diagnosis of disease by examining aberrant activity using various forms of EEG recordings (99–101).
Temko et al. investigated an automated grading system (AGS) based on a multi-class linear classifier that graded short-term EEG epochs and converted them to a long-term EEG grade using a majority vote procedure (100). The AGS employed summary measurements of two sub-signals derived from a quadratic time-frequency distribution: amplitude modulation and instantaneous frequency. When compared to human assessment of the EEG, the four-grade AGS exhibited an accuracy of 83%. The features calculated from the generated sub-signals were shown to be more accurate in grading the EEG than measures based purely on the EEG, and the addition of new sub-grades based on EEG states to the AGS enhanced performance as well.
Utilizing the infant cry to diagnose asphyxia may present a novel approach for developing an accessible diagnostic tool. Previous studies have hypothesized that breathing difficulty resulting from asphyxia alters the patterns in the cry waves of affected infants, primarily attributed to the fact that speech and breathing are controlled by the same underlying physiologic process (102, 103).
Ubenwa is an application that uses voice recognition algorithms to identify early indications of birth asphyxia in a newborn's cry. A machine-based learning model capable of accurately classifying recordings of known asphyxiated children reported an accuracy of 89% in a laboratory environment. In 2017, a prototype mobile application was developed based on this concept with the potential to become a widely accessible solution even in low-resource settings (104).
Automated Seizure Detection
Despite the availability of cEEG, reliable newborn seizure diagnosis remains challenging in clinical practice. Since 1992, techniques for automatically detecting newborn seizures in the EEG have been published (105). Significant advancements in data acquisition techniques, along with advances in computer technology, have substantially increased the accuracy of automated seizure detection approaches, and numerous research groups have developed and validated seizure detection algorithms for the newborn population (37, 106). The development of user-friendly ML-based software may facilitate the integration of automated EEG technology into the NICU, potentially increasing diagnostic accuracy and speed. A few of these algorithms have been included into commercially accessible EEG or aEEG systems (37, 106, 107).
The Efficacy of Intravenous Levetiracetam in Neonatal Seizures trial (NEOLEV2) used Persyst, a commercially available software, to evaluate participants' cEEGs (108). The software provided real-time seizure detection, which resulted in a higher detection rate, but failed to alleviate neurologists' burden, requiring human assessment due to low accuracy. RiskSLIM is a sparse linear integer machine with a high prediction accuracy (AUC = 0.83; similar to other widely used ML algorithms) (108).
Lawrence et al. studied a cohort of 40 encephalopathic infants for 72 h using a limited-channel aEEG and a software-based seizure event detector. Offline evaluation of EEG data was conducted independently. During an average of 68 h of monitoring, twenty-five babies developed EEG seizures on a limited channel aEEG, and 1,116 EEG seizures were detected retrospectively. 615 (55%) of the seizures were recognized in real-time by the seizure detection algorithm. Seizure detection software accuracy increased with seizure duration, up to 73% for seizures lasting more than 30 s and 87% for seizures lasting more than 60 s. Seizure detection had a false-positive rate of 1 event every 11 h of monitoring, resulting in a positive predictive value (PPV) of 73% and a negative predictive value (NPV) of 99% (37).
In a large randomized controlled trial conducted in eight centers in Europe, the diagnostic accuracy of an automated seizure detection system named Algorithm for Neonatal Seizure Recognition (ANSeR) was assessed. This study included 264 babies between the age of 36 and 44 weeks with or at high risk of seizures requiring EEG monitoring. One arm of the trial used cEEG plus ANSeR, connected to the EEG monitor and displayed a real-time seizure likelihood trend. In contrast, the other arm received only cEEG monitoring. Electrographic seizures occurred in 25% of newborns in the algorithm group compared to 29.2% in the non-algorithm group. Despite not enhancing the identification of individual neonates with seizures, the algorithm group correctly identified a greater proportion of seizure hours when compared to the non-algorithm group (109, 110).
There is a growing number of papers evaluating automated seizure detection algorithms in the NICU. Studies from the last five years are displayed in Table 2.
Prediction of Mortality
Numerous ML models have been reported in adult research, including those that predict death in a variety of disease processes (87, 88). However, there are much fewer AI models reported in pediatrics and neonatology to date. A recent systematic review included eleven studies examining the prediction accuracy of AI models for newborn mortality. This research included a total of 1.26 million babies born between 22 weeks and term age. The mean AUC ranged from 58.3 to 97.0 percent in this review, indicating their capacity to predict neonatal death. The most common features used in the predicting models are birth weight, gestational age and APGAR scores. Other standard features include pH, blood pressure, use of prenatal steroids, and multiple births (113).
Early Recognition of Sepsis
Sepsis is a leading cause of newborn mortality. The commercially available “HeRO” monitor analyzes ECG data from current bedside monitors for reduced heart rate variability and brief decelerations associated with sepsis and transforms them to a score (the HRC index or HeRO score). This score represents the chance of a patient having clinical deterioration due to sepsis within 24 h. Displaying the HeRO score reduced mortality by more than 20% in a randomized study of 3003 very low birth-weight babies. Current research attempts to integrate respiratory and heart rate data to enhance ICU care (122).
Predicting Extubation Failure
Given the independent association between mechanical ventilation and significant unfavorable outcomes, efforts should be taken to restrict its duration. However, existing methods for identifying extubation readiness are ineffective, and a large proportion of newborns fail extubation and require reintubation, a procedure that may result in increased morbidity. Numerous objective metrics have been proposed to improve the definition of the ideal time for extubation, but none have been demonstrated to be clinically helpful.
A recent multi-center diagnostic study investigated data from 259 newborns at five neonatal intensive care units over 5 years as part of the prospective Automated Prediction of Extubation Readiness (APEX) project (123). The study included 274 intubated neonates less than 1,250 g who were considered ready for extubation and had ET-CPAP before extubation. Cardiorespiratory signals were recorded electronically for 5 mins before extubation, and signs of clinical instability were monitored (124).
The data imply that extremely preterm newborns frequently exhibit clinical instability during ET-CPAP and that clinical event combinations were insufficient to define Spontaneous Breathing Trials (SBT's) accurately. As a result, the authors concluded SBTs might offer minimal value to the evaluation of extubation readiness.
Predicting Gestational Age
Particularly in low-middle income countries, the last menstrual period is frequently considered an inaccurate estimate of gestational age at birth (125). Using AI for prediction might be an interesting approach to minimize this effect. Models to evaluate pregnancy status were built to predict gestational age when the last menstrual period and fetal anatomical evaluation were not accessible (126).
A recent study involving 1318 newborns from Africa and Asia found that applying ML to birth weight and new-born metabolomic screening data can improve postnatal prediction of gestational age (GA) at birth. 85.21 % (95% CI 72.31–94.65) of GAs were properly predicted to within one week. The model also showed high sensitivity (100%) and specificity (92.6%) for differentiation of preterm and term birth (127). Meenakshi et al. (128) showed good results of a model to automatically estimate the GA at third trimester by using biparietal diameter and kidney length.
Additional ML approaches were also studied in the NICU, including automatic detection of sleep-wake-cycling for prediction of neurodevelopmental outcomes (129–132).
Telemedicine
Telemedicine is defined by the World Health Organization (WHO) as “the delivery of health care services by all health care professionals using information and communication technologies for the exchange of valid information for the diagnosis, treatment, and prevention of disease and injury, research and evaluation, and continuing education of health care professionals” (133). Additionally, four critical components of telemedicine were identified: (i) provision of clinical support; (ii) purpose to overcome geographic barriers; (iii) the use of a variety of information and communication technology; and (iv) the objective of improving population health (134). This model represents the natural growth of healthcare in the digital era, reducing distances between distant places, increasing access and reach of specific techniques, and decreasing structural costs. In education, it can be used to facilitate learning through live interactive audiovisual connections, live video broadcast, or viewing online-stored educational materials (133–136).
Telemedicine has the potential to play an essential role in providing specialized care to remote populations with limited resources. Centralized systems may communicate in real-time with a large number of centers, leveraging educational activities, consulting, and monitoring to improve service quality. Protecting Brains and Saving Futures (PBSF) is a Brazilian program that has already connected 35 hospitals in the country. Neonates undergoing EEG or aEEG and NIRS monitoring are assisted by a remote team in a monitoring center, using encrypted data to ensure the privacy of sent information. This approach may also be used in research contexts, where studies done in low-middle income countries (LMIC) might benefit from teams' assistance in developed countries, potentially improving the quality of data obtained substantially. The Prevention of Epilepsy by Reducing Neonatal Encephalopathy (PREVENT) study (NCT04054453) used a similar method to monitor and collect data from newborns in three sites in India. All EEG data is securely sent to a cloud-based server. Cost-effectiveness analysis and legal and regulatory concerns are significant challenges to this approach in LMICs.
A model of architecture for multimodal monitoring accessed by telemedicine approach is shown in Figure 5.
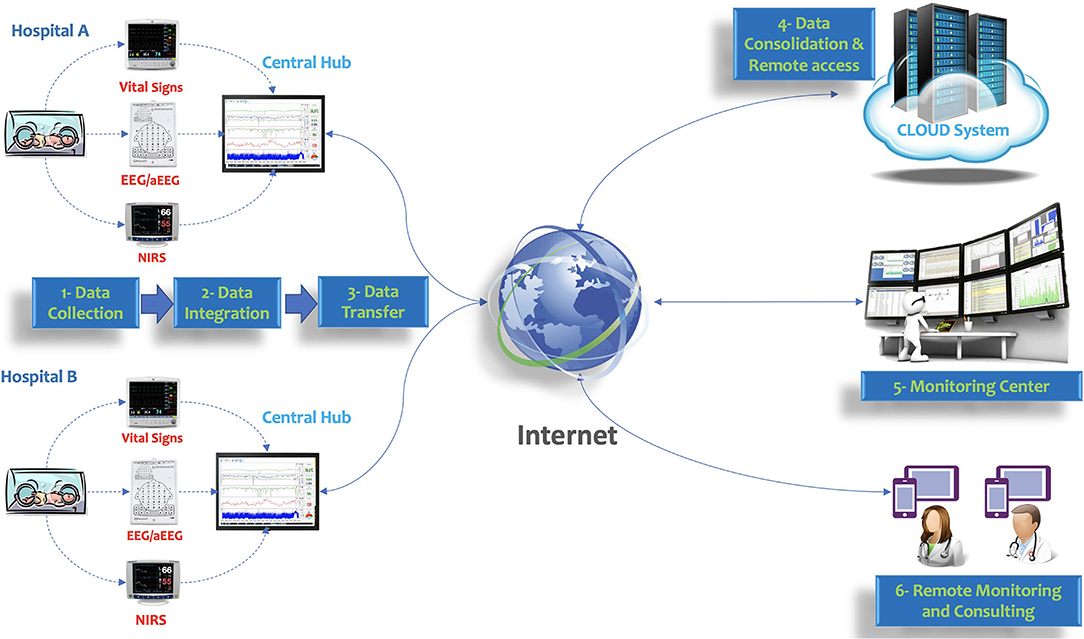
Figure 5. Model of architecture for multimodal monitoring accessed by telemedicine approach. Vital signs, EEG/aEEG, and NIRS are integrated using a central hub. The encrypted data is transferred to the cloud system and accessed by a monitoring center or remote assistance.
Futures Directions and Conclusions
The creation of the Neuro-NICU demonstrates a genuine potential for closing the gap between survival and improved neurodevelopmental outcome. Given the increased emphasis on neurocritical care, both aEEG/EEG and NIRS have demonstrated value as bedside monitors in the NICU. Multimodal brain and hemodynamic monitoring in newborns are possible, safe, and well-tolerated. Further research should be conducted to determine the impact of this strategy in a more extensive range of clinical scenarios, emphasizing evidence to improve clinical outcomes.
Tele-health is increasingly used to assist remote centers with education, monitoring, and consulting (137). The combination of multimodal monitoring and telemedicine may improve understanding of physiology and provide a vast repertoire of data.
With the advancement of processing power and the growth of data availability, ML algorithms are proving to be an interesting tool in ICU research. However, one of the primary challenges to adopting ML in clinical practice is the ethical considerations of depending on ML for clinical decision-making. While ML has been proven to surpass traditional methods for clinical decision-making, there is significant concern over who is responsible if an ML makes a mistake.
One of the key challenges in clinical research is gathering and evaluating big datasets for prospective studies. Only a small percentage of previously published studies verified their model using an independent cohort.
In conclusion, neonatology is one of the medical specialties that have scientifically advanced the most in recent decades and today is focused on preserving the quality of life in high-risk infants. The broader use of brain monitoring, associated with intelligent analysis of large amounts of information collected on-site or remotely, has the potential to significantly change clinical management and neonatal outcomes within the next 10 years.
Author Contributions
GV conceived the idea and wrote the manuscript. DR, MM, and MJM reviewed, edited, and provided contributions to the manuscript. JC provided critical feedback and support on data integration and artificial intelligence topics. All authors contributed to the article and approved the submitted version.
Conflict of Interest
The authors declare that the research was conducted in the absence of any commercial or financial relationships that could be construed as a potential conflict of interest.
Publisher's Note
All claims expressed in this article are solely those of the authors and do not necessarily represent those of their affiliated organizations, or those of the publisher, the editors and the reviewers. Any product that may be evaluated in this article, or claim that may be made by its manufacturer, is not guaranteed or endorsed by the publisher.
References
1. Younge N, Goldstein RF, Bann CM, Hintz SR, Patel RM, Smith PB, et al. Survival and neurodevelopmental outcomes among periviable infants. N Engl J Med. (2017) 376:617–28. doi: 10.1056/NEJMoa1605566
2. Rysavy MA Li L, Bell EF, Das A, Hintz SR, Stoll BJ, et al. Between-hospital variation in treatment and outcomes in extremely preterm infants. N Engl J Med. (2015) 372:1801–11. doi: 10.1056/NEJMoa1410689
3. Volpe J, Inder T, Darras B, de Vries L, du Plessis A, Neil J, et al. Volpe's Neurology of the Newborn 6th ed., Philadelphia: Saunders, Elsevier (2018).
4. Glass HC, Bonifacio SL, Peloquin S, Shimotake T, Sehring S, Sun Y, et al. Neurocritical care for neonates. Neurocrit Care. (2010) 12:421–9. doi: 10.1007/s12028-009-9324-7
5. Peiffer-Smadja N, Rawson TM, Ahmad R, Buchard A, Georgiou P, Lescure FX, et al. Machine learning for clinical decision support in infectious diseases: a narrative review of current applications. Clin Microbiol Infect. (2020) 26:584–95. doi: 10.1016/j.cmi.2019.09.009
6. Rush B, Celi LA, Stone DJ. Applying machine learning to continuously monitored physiological data. J Clin Monit Comput. (2019) 33:887–93. doi: 10.1007/s10877-018-0219-z
7. Variane GFT, Chock VY, Netto A, Pietrobom RFR, Van Meurs KP. Simultaneous near-infrared spectroscopy (NIRS) and amplitude-integrated electroencephalography (aEEG): dual use of brain monitoring techniques improves our understanding of physiology. Front Pediatr. (2020) 7:560. doi: 10.3389/fped.2019.00560
8. Larroque B, Ancel PY, Marret S, Marchand L, André M, Arnaud C, et al. Neurodevelopmental disabilities and special care of 5-year-old children born before 33 weeks of gestation (the EPIPAGE study): a longitudinal cohort study. Lancet. (2008) 371:813–20. doi: 10.1016/S0140-6736(08)60380-3
9. Dyet LE, Kenna N, Counsell SJ, Maalouf EF, Ajayi-Obe M, Duggan PJ, et al. Natural history of brain leasions in extremely preterm infants studied with serial magnetic resonance imaging from birth and neurodevelopmental assessment. Pediatrics. (2006) 118:536–48. doi: 10.1542/peds.2005-1866
10. Inder TE, Wells SJ, Mogridge NB, Spencer C, Volpe JJ. Defining the nature of the cerebral abnormalities in the premature infant: a qualitative magnetic resonance imaging study. J Pediatrics. (2003)143:171–9. doi: 10.1067/S0022-3476(03)00357-3
11. Back SA. White matter injury in the preterm infant: pathology and mechanisms. Acta Neuropathol. (2017) 134:331–49. doi: 10.1007/s00401-017-1718-6
12. Bolisetty S, Dhawan A, Abdel-Latif M, Bajuk B, Stack J, Lui K, et al. Intraventricular hemorrhage and neurodevelopmental outcomes in extreme preterm infants. Pediatrics. (2014) 133:155–62. doi: 10.1542/peds.2013-0372
13. Stoll BJ, Hansen NI, Bell EF, Shankaran S, Laptook AR, Walsh MC, et al. Neonatal outcomes of extremely preterm infants from the NICHD Neonatal Research Network. Pediatrics. (2010) 126:443–56. doi: 10.1542/peds.2009-2959
14. Shah PS, Lui K, Sjors G, Mirea L, Reichman B, Adams M, et al. Neonatal outcomes of very low birth weight and very preterm neonates: an international comparison. J Pediatr. (2016) 177:144–52.e6. doi: 10.1016/j.jpeds.2016.04.083
15. Kurinczuk JJ, White-Koning M, Badawi N. Epidemiology of neonatal encephalopathy and hypoxic-ischaemic encephalopathy. Early Hum Dev. (2010) 86:329–38. doi: 10.1016/j.earlhumdev.2010.05.010
16. Lawn J, Shibuya K, Stein C. No cry at birth: global estimates of intrapartum stillbirths and intrapartum-related neonatal deaths. Bull World Health Organ. (2005) 83:409–17. doi: 10.1590/S0042-96862005000600008
17. Fluss J, Dinomais M, Chabrier S. Perinatal stroke syndromes: Similarities and diversities in aetiology, outcome and management. Eur Paediatr Neurol. (2019) 23:368–83. doi: 10.1016/j.ejpn.2019.02.013
18. Tagin MA, Woolcott CG, Vincer MJ, Whyte RK, Stinson DA. Hypothermia for neonatal hypoxic ischemic encephalopathy: an updated systematic review and meta-analysis. Arch Pediatr Adolesc Med. (2012) 166:558–66. doi: 10.1001/archpediatrics.2011.1772
19. Jacobs SE, Berg M, Hunt R, Tarnow-Mordi WO, Inder TE, Davis PG. Cooling for newborns with hypoxic ischaemic encephalopathy. Cochrane Database Syst Rev. (2013) 2013:CD003311. doi: 10.1002/14651858.CD003311.pub3
20. Van Meurs KP, Yan ES, Randall KS, Chock VY, Davis AS, Glennon CS, et al. Development of a NeuroNICU with a broader focus on all newborns at risk of brain injury: the First 2 Years. Am J Perinatol. (2018) 35:1197–205. doi: 10.1055/s-0038-1646954
21. Bashir RA, Espinoza L, Vayalthrikkovil S, Buchhalter J, Irvine L, Bello-Espinosa L, et al. Implemenation of a neurocritical care program: improved seizure detection and decreased antiseizure medication at discharge in neonates with hypoxic–ischemic encephalopathy. Pediatr Neurol. (2016) 64:38–43. doi: 10.1016/j.pediatrneurol.2016.07.007
22. Harbert MJ, Sey R, Arnell K, Salinda L, Brown MK, Lazarus D, et al. Impact of a neuro-intensive care service for newborns. J Neonatal Perinat Med. (2018) 11:173–8. doi: 10.3233/NPM-181751
23. Glass HC, Ferriero DM, Rowitch DH, Shimotake TK. The neurointensive nursery: concept, development and insights gained. Curr Opin Pediatr. (2019) 31:202–9. doi: 10.1097/mop.0000000000000733
24. Leotsakosi A, Zheng H, Croteau R, Loeb JM, Sherman H, Hoffman C, et al. Standardization in patient safety: the WHO high 5s project. Int J Qual Health Care. (2014) 26:109–16. doi: 10.1093/intqhc/mzu010
25. Austin T. The development of neonatal neurointensive care. Pediatr Res. (2019) 18:1–7. doi: 10.1038/s41390-019-0729-5
26. Shellhaas RA, Soaita AI, Clancy RR. Sensitivity of amplitude-integrated electro- encephalography for neonatal detection of neonatal seizures. Pediatrics. (2007) 120:770–7. doi: 10.1542/peds.2007-0514
27. Murray DM, O'Connor CM, Ryan CA, Korotchikova I, Boylan GB. Early EEG grade and outcome at 5 Years after mild neonatal hypoxic ischemic encephalopathy. Pediatrics. (2016) 138:e20160659. doi: 10.1542/peds.2016-0659
28. Variane GFT, Magalhães M, Gasperine R, Alves HCBR, Scoppetta TLPD, Figueredo RJG, et al. Early amplitude-integrated electroencephalography for monitoring neonates at high risk for brain injury. J Pediatr. (2017) 93:460–6. doi: 10.1016/j.jpes.2016.12.003
29. Shellhaas RA, Clancy RR. Characterization of neonatal seizures by conventional EEG and single-channel EEG. Clin Neurophysiol. (2007) 118:2156–61. doi: 10.1016/j.clinph.2007.06.061
30. Patrizi S, Holmes GL, Orzalesi M, Allemand F. Neonatal seizures: characteristics of EEG ictal activity in preterm and fullterm infants. Brain Dev. (2003) 25:427–37. doi: 10.1016/s0387-7604(03)00031-7
31. Kidokoro H, Inder T, Okumura A, Watanabe K. What does cyclicity on amplitude-integrated EEG mean? J Perinatol. (2012) 32:565–9. doi: 10.1038/jp.2012.25
32. Wikström S, Pupp IH, Rosén I, et al. Early single-channel aEEG/EEG predicts outcome in very preterm infants. Acta Paediatr. (2012) 101:719–26. doi: 10.1111/j.1651-2227.2012.02677.x
33. Soubasi V, Mitsakis K, Sarafidis K, Griva M, Nakas CT, Drossou V, et al. Early abnormal amplitude-integrated electroencephalography (aEEG) is associated with adverse short-term outcome in premature infants. Eur J Paediatric Neurol. (2012) 16:625–30. doi: 10.1016/j.ejpn.2012.02.008
34. Klebermass K, Olischar M, Waldhoer T, et al. Amplitude-integrated EEG pattern predicts further outcome in preterm infants. Pediatr Res. (2011) 70:102–8. doi: 10.1203/PDR.0b013e31821ba200
35. Shah DK, Mackay MT, Lavery S, Watson S, Harvey AS, Zempel J, et al. Accuracy of bedside electroencephalographic monitoring in comparison with simultaneous continuous conventional electroencephalography for detection of neonatal seizures in term infants. Pediatrics. (2008) 121:1146–54. doi: 10.1542/peds.2007-1839
36. Toet MC, van der Meij W, de Vries LS, Uiterwaal CSPM, van Huffelen KC. Comparison between simultaneously recorded amplitude integrated electroencephalogram (cerebral function monitor) and standard electroencephalogram in neonates. Pediatrics. (2002) 109:772–9. doi: 10.1542/peds.109.5.772
37. Lawrence R Mathur A Nguyen Nguyen The Tich S Zempel J Inder T A. pilot study of continuous limited-channel aEEG in term infants with encephalopathy. J Pediatr. (2009) 154:835–41. doi: 10.1016/j.jpeds.2009.01.002
38. Kharoshankaya L, Stevenson NJ, Livingstone V, Murray DM, Murphy BP, Ahearne CA, et al. Effect of treatment of subclinical neonatal seizzures detected with aEEG: randomized, controlled trial. Pediatric. (2010) 125:e358–66. doi: 10.1542/peds.2009-0136
39. Srinivasakumar P, Zempel J, Trivedi S, Wallendorf M, Rao R, Smith B, et al. Treating EEG seizures in hypoxic ischemic encephalopaty: a randomized controlled trial. Pediatrics. (2015) 136:e1302–9. doi: 10.1542/peds.2014-3777
40. Frenkel N, Friger M, Meledin I, Berger I, Marks K, Bassan H, et al. Neonatal seizure recognition—comparative study of continuous-amplitude integrated EEG versus short conventional EEG recordings. Clin Neurophysiol. (2011) 122:1091–7
41. Glass HC, Wusthoff CJ, Shellhaas RA. Amplitude Integrated EEG: the Child Neurologist's Perspective. J Child Neurol. (2013) 28:1342–50
42. Mastrangelo M, Fiocchi I, Fontana P, Gorgone G, Lista G, Belcastro V. Acute neonatal encephalopathy and seizures recurrence: a combined aEEG/EEG study. Seizure. (2013) 22:703–7. doi: 10.1016/j.seizure.2013.05.006
43. Rakshasbhuvankar A, Paul S, Nagarajan L, Ghosh S, Rao S. Amplitude-integrated EEG for detection of neonatal seizures: a systematic review. Seizure. (2015) 33:90–8. doi: 10.1016/j.seizure.2015.09.014
44. Rennie JM, Chorley G, Boylan GB, Pressler R, Nguyen Y, Hooper R. Non-expert use of the cerebral function monitor for neonatal seizure detection. Arch Dis Child Fetal Neonatal Ed. (2004) 89:F37–40. doi: 10.1136/fn.89.1.f37
46. Gunn JK, Beca J, Hunt RW, Olischar M, Shekerdemian LS. Perioperative amplitude-integrated EEG and neurodevelopment in infants with congenital heart disease. Intensive Care Med. (2012) 38:1539–47. doi: 10.1007/s00134-012-2608-y
47. Kubota Y, Nakamoto H, Egawa S, Kawamata T. Continuous EEG monitoring in ICU. J Intensive Care. (2018) 6:39. doi: 10.1186/s40560-018-0310-z
48. Neubauer D, Osredkar D, Paro-Panjan D, Skofjanec A, Derganc M. Recording conventional and amplitude-integrated EEG in neonatal intensive care. Eur J Paediatr Neurol. (2011) 15:405–16. doi: 10.1016/j.ejpn.2011.03.001
49. Latal B, Wohlrab G, Brotschi B, Beck I, Knirsch W, Bernet V. Postoperative amplitude-integrated electroencephalography predicts four-year neurodevelopmental outcome in children with complex congenital heart disease. J Pediatr. (2016) 178:55–60. doi: 10.1016/j.jpeds.2016.06.050
50. Olischar M, Shany E, Aygün C, Azzopardi D, Hunt RW, Toet MC, et al. Amplitude-integrated electroencephalography in newborns with inborn errors of metabolism. Neonatology. (2012) 102:203–11. doi: 10.1159/000339567
51. Helderman JB, Welch CD, Leng X, O'Shea TM. Sepsis-associated electroencephalographic changes in extremely low gestational age neonates. Early Hum Dev. (2010) 86:509–13. doi: 10.1016/j.earlhumdev.2010.06.006
52. Shellhaas RA, Chang T, Tsuchida T, Scher MS, Riviello JJ, Abend NS, et al. The American Clinical Neurophysiology Society's guideline on continuous electroencephalography monitoring in neonates. J Clin Neurophysiol. (2011) 28:611–7. doi: 10.1097/WNP.0b013e31823e96d7
53. Hunter CL, Oei JL, Suzuki K, Lui K, Schindler T. Patterns of use of near-infrared spectroscopy in neonatal intensive care units: international usage survey. Acta Paediatr. (2018) 107:1198–204. doi: 10.1111/apa.14271
54. Abdul-Khaliq H, Troitzsch D, Berger F, Lange PE. Regional transcranial oximetry with near infrared spectroscopy (NIRS) in comparison with measuring oxygen saturation in the jugular bulb in infants and children for monitoring cerebral oxygenation. Biomed Tech. (2000) 45:328–32. doi: 10.1515/bmte.2000.45.11.328
55. Alderliesten T, Dix L, Baerts W, Caicedo A, van Huffel S, Naulaers G, et al. Reference values of regional cerebral oxygen saturation during the first 3 days of life in preterm neonates. Pediatr Res. (2016) 79:55–64. doi: 10.1038/pr.2015.186
56. Ter Horst HJ, Verhagen EA, Keating P, Bos AF. The relationship between electrocerebral activity and cerebral fractional tissue oxygen extraction in preterm infants. Pediatr Res. (2011) 70:384–8. doi: 10.1203/PDR.0b013e3182294735
57. Kurth CD, Levy WJ, McCann J. Near-infrared spectroscopy cerebral oxygen saturation thresholds for hypoxia- ischemia in piglets. J Cereb Blood Flow Metab. (2002) 22:335–41. doi: 10.1097/00004647-200203000-00011
58. Dent CL, Spaeth JP, Jones BV, Schwartz SM, Glauser TA, Hallinan B, et al. Brain magnetic resonance imaging abnormalities after the Norwood procedure using regional cerebral perfusion. J Thorac Cardiovasc Surg. (2006) 131:190–7. doi: 10.1016/j.jtcvs.2005.10.003
59. Chock VY, Variane GFT, Netto A, Krisa P, Van Meurs KP. NIRS improves hemodynamic monitoring and detection of risk for cerebral injury: cases in the neonatal intensive care nursery. J Maternal-Fetal Neonat Med. (2018) 33:1802–10. doi: 10.1080/14767058.2018.1528223
60. Hoffman GM, Stuth EA, Jaquiss RD, Vanderwal PL, Staudt SR, Troshynski TJ, et al. Changes in cerebral and somatic oxygenation during stage 1 palliation of hypoplastic left heart syndrome using continuous regional cerebral perfusion. J Thorac Cardiovasc Surg. (2004) 127:223–33. doi: 10.1016/j.jtcvs.2003.08.021
61. McNeill S, Gatenby JC, McElroy S, Engelhardt B. Normal cerebral, renal and abdominal regional oxygen saturations using near-infrared spectroscopy in preterm infants. J Perinatol. (2011) 31:51–7. doi: 10.1038/jp.2010.71
62. Lemmers PM, Zwanenburg RJ, Benders MJ, de Vries LS, Groenendaal F, van Bel F, et al. Cerebral oxygenation and brain activity after perinatal asphyxia: does hypothermia change their prognostic value? Pediatr Res. (2013) 74:180–5. doi: 10.1038/pr.2013.84
63. Phelps HM, Mahle WT, Kim D, Simsic JM, Kirshbom PM, Kanter KR, et al. Postoperative cerebral oxygenation in hypoplastic left heart syndrome after the Norwood procedure. Ann Thorac Surg. (2009) 87:1490–4. doi: 10.1016/j.athoracsur.2009.01.071
64. Sood ED, Benzaquen JS, Davies RR, Woodford E, Pizarro C. Predictive value of perioperative near-infrared spectroscopy for neurodevelopmental outcomes after cardiac surgery in infancy. J Thorac Cardiovasc Surg. (2013) 145:438–45. doi: 10.1016/j.jtcvs.2012.10.033
65. Johnson BA, Hoffman GM, Tweddell JS, Cava JR, Basir M, Mitchell ME, et al. Near-infrared spectroscopy in neonates before palliation of hypoplastic left heart syndrome. Ann Thorac Surg. (2009) 87:571–7. doi: 10.1016/j.athoracsur.2008.10.043
66. Hyttel-Sorensen S, Pellicer A, Alderliesten T, Austin T, van Bel F, Benders M, et al. Cerebral near infrared spectroscopy oximetry in extremely preterm infants: phase II randomised clinical trial. BMJ. (2015) 350:g7635. doi: 10.1136/bmj.g7635
67. Prescott S. Near infrared spectroscopy and patent ductus arteriosus in the preterm neonate: a systematic review. J Neonatal Nurs. (2016) 23:9–27. doi: 10.1016/j.jnn.2016.07.002
68. Chock VY, Rose LA, Mante JV, Punn R. Near-infrared spectroscopy for detection of a significant patent ductus arteriosus. Pediatric Res. (2016) 80:675–80. doi: 10.1038/pr.2016.148
69. Norooz F, Urlesberger B, Giordano V, Klebermasz-Schrehof K, Weninger M, Berger A, et al. Descompressing posthaemorragic ventricular dilatation significantly improves regional cerebral oxygen saturation in preterm infants. Acta Paediatr. (2015) 104:663–9. doi: 10.1111/apa.12942
70. Kochan M, McPadden J, Bass WT, Shah T, Brown WT, Tye GW, et al. Changes in cerebral oxygenation in preterm infants with progressive posthemorragic ventricular dilatation. Pediatr Neurol. (2017) 73:57–63. doi: 10.1016/j.pediatrneurol.2017.05.012
71. Zanatta P, Benvenuti SM, Bosco E, Baldanzi F, Palomba D, Valfrè C. Multimodal brain monitoring reduces major neurologic complications in cardiac surgery. J Cardiothorac Vasc Anesth. (2011) 25:1076–85. doi: 10.1053/j.jvca.2011.05.015
72. Ancora G, Maranella E, Grandi S, Sbravati F, Coccolini E, Savini S, et al. Early predictors of short term neurodevelopmental outcome in asphyxiated cooled infants. A combined brain amplitude integrated electroencephalography and near infrared spectroscopy study. Brain Dev. (2013) 35:26–31. doi: 10.1016/j.braindev.2011.09.008
73. Niezen CK, Bos AF, Sival DA, Meiners LC, ter Horst HJ. Amplitude-integrated EEG and cerebral near-infrared spectroscopy in cooled, asphyxiated infants. Am J Perinatol. (2017) 35:904–10. doi: 10.1055/s-0038-1626712
74. Goeral K, Urlesberger B, Giordano V, Kasprian G, Wagner M, Schmidt L, et al. prediction of outcome in neonates with hypoxic-ischemic encephalopathy II: role of amplitude-integrated electroencephalography and cerebral oxygen saturation measured by near-infrared spectroscopy. Neonatology. (2017) 112:193–202. doi: 10.1159/000468976
75. Tamussino A, Urlesberger B, Baik N, Schwaberger B, Binder-Heschl C, Schmölzer GM, et al. Low cerebral activity and cerebral oxygenation during immediate transition in term neonates-a prospective observational study. Resuscitation. (2016) 103:49–53. doi: 10.1016/j.resuscitation.2016.03.011
76. Pichler G, Avian A, Binder C, Zotter H, Schmölzer GM, Morris N, et al. aEEG and NIRS during transition and resuscitation after birth: promising additional tools; an observational study. Resuscitation. (2013) 84:974–8. doi: 10.1016/j.resuscitation.2012.12.0233
77. Katheria AC, Harbert MJ, Nagaraj SB, Arnell K, Poeltler DM, Brown MK, et al. The Neu-Prem trial: neuromonitoring of brains of infants born preterm during resuscitation-a prospective observational cohort study. J Pediatr. (2018) 198:209–13. doi: 10.1016/j.jpeds.2018.02.065
78. Katheria AC, Stout J, Morales AL, Poeltler D, Rich WD, Steen J, et al. Association between early cerebral oxygenation and neurodevelopmental impairment of death in premature infants. J Perinatol. (2021) 41:743–8. doi: 10.1038/s41372-021-00942-w
79. Deshpande P, Jain A, Ríos DI, Bhattacharya S, Dirks J, Baczynski M, et al. Combined multimodal cerebral monitoring and focused hemodynamic assessment in the first 72 h in extremely low gestational age infants. Neonatology. (2020) 117:504–12. doi: 10.1159/000508961
80. Citerio G, Park S, Schmidt JM, Moberg R, Suarez JI, Le Roux PD, et al. Data collection and interpretation. Neurocrit Care. (2015) 22:360–8. doi: 10.1007/s12028-015-0139-4
81. Vesoulis ZA, Mintzer JP, Chock VY. Neonatal NIRS monitoring: recommendations for data capture and review of analytics. J Perinatol. (2021) 41:675–88. doi: 10.1038/s41372-021-00946-6
82. Saripalle R, Runyan C, Russell M. Using HL7 FHIR to achieve interoperability in patient health record. J Biomed Inform. (2019) 94:103188. doi: 10.1016/j.jbi.2019.103188
83. Reis PMR, Hebenstreit F, Gabsteiger F, von Tscharner V, Lochmann M. Methodological aspects of EEG and body dynamics measurements during motion. Front Hum Neurosci. (2014) 8:156. doi: 10.3389/fnhum.2014_00156
84. Virtanen P, Gommers R, Oliphant TE, Haberland M, Reddy T, Cournapeau D, et al. SciPy 10: fundamental algorithms for scientific computing in Python. Nat Methods. (2020) 17:261–72. doi: 10.1038/s41592-019-0686-2
85. Bahrami M, Laurienti PJ, Simpson SL. A MATLAB toolbox for multivariate analysis of brain networks. Hum Brain Mapp. (2019) 40:175–86. doi: 10.1002/hbm.24363
86. Vesoulis ZA, Gamble PG, Jain S, El Ters NM, Liao SM, Mathur AM. WU-NEAT: a clinically validated, open-source MATLAB toolbox for limited-channel neonatal EEG analysis. Comput Methods Programs Biomed. (2020) 196:105716. doi: 10.1016/j.cmpb.2020.105716
87. Copeland BJ. Artificial intelligence. Encyclopedia Britannica (2020). Available online at: https://www.britannica.com/technology/artificial-intelligence (accessed 25 July 2021).
88. Raschka S. Model evaluation, model selection, and algorithm selection in machine learning. arXiv preprint arXiv (2018):1811.12808
89. Gutierrez G. Artificial Intelligence in the Intensive Care Unit. Crit Care. (2020) 24:101. doi: 10.1186/s13054-020-2785-y
90. Beam AL, Kohane IS. Big data and machine learning in health care. JAMA. (2018) 319:1317–8. doi: 10.1001/jama.2017.18391
91. Hosny A, Parmar C, Quackenbush J, Schwartz LH, Aerts HJWL. Artificial intelligence in radiology. Nat Rev Cancer. (2018) 18:500–10. doi: 10.1038/s41568-018-0016-5
92. Kassahun Y, Yu B, Tibebu AT, Stoyanov D, Giannarou S, Metzen JH, et al. Surgical robotics beyond enhanced dexterity instrumentation: a survey of machine learning techniques and their role in intelligent and autonomous surgical actions. Int J Comput Assist Radiol Surg. (2016) 11:553–68. doi: 10.1007/s11548-015-1305-z
93. Johnson KW, Soto JT, Glicksberg BS, Shameer K, Miotto R, Ali M, et al. Artificial intelligence in Cardiology. J Am Coll Cardiol. (2018) 71:2668–79. doi: 10.1016/j.jacc.2018.03.521
94. Brajer N, Cozzi B, Gao M, Nichols M, Revoir M, Balu S, et al. Prospective and external evaluation of a machine learning model to predict in-hospital mortality of adults at time of admission. JAMA Netw Open. (2020) 3:e1920733. doi: 10.1001/jamanetworkopen.2019.20733
95. Houthooft R, Ruyssinck J, van der Herten J, Stijven S, Couckuyt I, Gadeyne B, et al. Predictive modelling of survival and length of stay in critically ill patients using sequential organ failure scores. Artif Intell Med. (2015) 63:191–207. doi: 10.1016/j.artmed.2014.12.009
96. Zeiberg D, Prahlad T, Nallamothu BK, Iwashyna TJ, Wiens J, Sjoding MW. Machine learning for patient risk stratification for acute respiratory distress syndrome. PLoS ONE. (2019) 14:e0214465. doi: 10.1371/journal.pone.0214465
97. Chalak LF, Nguyen KA, Prempunpong C, Heyne R, Thayyil S, Shankaran S, et al. Prospective research in infants with Mild Encephalopathy (PRIME) identified in the first six hours of life: neurodevelopmental outcomes at 18-22 months. Pediatr Res. (2018) 84:861–8. doi: 10.1038/s41390-018-0174-x
98. O'Boyle DS, Dunn WB, O'Neill D, Kirwan JA, Broadhurst DI, Hallberg B, et al. improvement in the prediction of neonatal Hypoxic ischemic Encephalopathy with the integration of umbilical cord metabolites and current clinical makers. J Pediatr. (2021) 229:175–81.e1. doi: 10.1016/j.jpeds.2020.09.065
99. Merchant N, Azzopardi A. Early predictors of outcome in infants treated with hypothermia for hypoxic–ischaemic encephalopathy. Dev Med Child Neurol. (2015) 57 Suppl 3:8–16. doi: 10.1111/dmcn.12726
100. Temko A, Stevenson N, Marnane W, Boylan G, Lightbody G. Inclusion of temporal priors for automated neonatal EEG classification. J Neural Eng. (2012) 9:046002. doi: 10.1088/1741.2560/9/4/046002
101. Stevenson NJ, Korotchikova I, Temko A, Lightbody G, Marnane WP, Boylan GB. An automated system for grading EEG abnormality in term neonates with hypoxic-ischaemic encephalopathy. Ann Biomed Eng. (2013) 41:775–85. doi: 10.1007/s10439-012-0710-5
102. Abbasi H, Bennet L, Gunn AJ, Unsworth CP. Robust wavelet stabilized ‘Footprints of uncertainty' for fuzzy system classifiers to automatically detect sharp waves in the EEG after hypoxia ischemia. Int J Neural Syst. (2017) 27:1650051. doi: 10.1142/S0129065716500519
103. Michelsson K, Sirviö P, Wasz-Höckert O. Pain cry in full-term asphyxiated newborn infants correlated with late findings. Acta Paediatr Scand. (1977) 66:611–6. doi: 10.1111/j.1651-2227.1977.tb07956.x
104. Onu CC, Udeogu I, Ndiomu E, Kengni U, Precup D, et al. Ubenwa: Cry-based diagnosis of birth asphyxia. arXiv preprint arXiv (2017).
105. Liu A, Hahn JS, Heldt GP, Coen RW. Detection of neonatal seizures through computerized EEG analysis. Electroencephalogr Clin Neurophysiol. (1992) 82:30–7. doi: 10.1016/0013-4694(92)90179-l
106. Gotman J, Flanagan D, Rosenblatt B, Bye A, Mizrahi EM. Evaluation of an automatic seizure detection method for the newborn EEG. Electroencephalogr Clin Neurophysiol. (1997) 103:363–9. doi: 10.1016/s0013-4694(97)00005-2
107. Navakatikyan MA, Colditz PB, Burke CJ, Inder TE, Richmond J, Williams CE. Seizure detection algorithm for neonates based on wave-sequence analysis. Clin Neurophysiol. (2006) 117:1190–203. doi: 10.1016/j.clinph.2006.02.016
108. Sharpe C, Davis SL, Reiner GE, Lee LI, Gold JJ, Nespeca M, et al. Assessing the feasibility of providing a real-time response to seizures detected with continuous long-term neonatal electroencephalography monitoring. J Clin Neurophysiol. (2019) 36:9–13. doi: 10.1097/WNP.0000000000000525
109. Struck AF, Rodriguez-Ruiz AA, Osman G, Gilmore EJ, Haider HA, Dhakar MB, et al. Comparison of machine learning models for seizure prediction in hospitalized patients. Ann Clin Transl Neurol. (2019) 6:1239–47. doi: 10.1002/acn3.50817
110. Pavel AM, Rennie JM, de Vries LS, Blennow M, Foran A, Shah DK, et al. A machine-learning algorithm for neonatal seizure recognition: a multicentre, randomised, controlled trial. Lancet Child Adolesc Health. (2020) 4:740–9. doi: 10.1016/S2352-4642(20)30239-X
111. Ansari AH, Cherian PJ, Dereymaeker A, Matic V, Jansen K, De Wispelaere L, et al. Improved multi-stage neonatal seizure detection using a heuristic classifier and data-driven-post-processor. Clin Neurophysiol. (2016) 127:3014–24. doi: 10.1016/j.clinph.2016.06.018
112. Ansari AH, Cherian PJ, Caicedo A, De Vos M, Naulaers G, Van Huffel S. Improved neonatal seizure detection using adaptative learning. In: Conference proceedings: Annual International Conference of the IEEE Engineering in Medicine and Biology Society. (2017), p. 2810–13
113. Ansari AH, Cherian PJ, Caicedo A, Naulaers G, De Vos M, Van Huffel S. Neonatal seizure detection using deep convolutional neural networks. Int J Neural Syst. (2019) 29:1850011. doi: 10.1142/S0129065718500119
114. Bogaarts JG, Gommer ED, Hilkman DMW, van Kranen-Mastenbroek VHJM, Reulen JPH. Optimal training dataset composition for SVM-based, age-independent, automated epileptic seizure detection. Med Biol Eng Comput. (2016) 54:1285–93. doi: 10.1007/s11517-016-1468-y
115. Mathieson S, Rennie J, Livingstone V, Temko A, Low E, Pressler RM, et al. In-depth performance analysis of an EEG based neonatal seizure detection algorithm. Clin Neurophysiol. (2016) 127:2246–56. doi: 10.1016/j.clinph.2016.01.026
116. Mathieson SR, Stevenson NJ, Low E, Marnane WP, Rennie JM, Temko A, et al. Validation of an automated seizure detection algorithm for term neonates. Clin Neurophysiol. (2016) 127:156–68. doi: 10.1016/j.clinph.2015.04.075
117. Mathieson SR, Livingstone V, Low E, Pressler R, Rennie JM, Boylan GB. Phenobarbital reduces EEG amplitude and propagation of neonatal seizures but does not alter performance of automated seizure detection. Clin Neurophysiol. (2016) 127:3343–50. doi: 10.1016/j.clinph.2016.07.007
118. Temko A, Sarkar AK, Boylan GB, Mathieson S, Marnane WP, Lightbody G. Toward a personalized real-time diagnosis in neonatal seizure detection. IEEE J Transl Eng Health Med. (2017) 5:2800414. doi: 10.1109/JTEHM.2017.2737992
119. Tapani KT, Vanhatalo S, Stevenson NJ. Incorporating spike correlations into an SVM-based neonatal seizure detector. In: Conference: European Medical and Biological Engineering Conference Nordic-Baltic Conference on Biomedical Engineering and Medical Physics. (2018) 65:322–5. doi: 10.1007/978-981-10-5122-7_81
120. Tapani KT, Vanhatalo S, Stevenson NJ. Time-varying EEG correlations improve automated neonatal seizure detection. Int J Neural Syst. (2019) 29:1850030. doi: 10.1142/S0129065718500302
121. Stevenson N, Tapani K, Vanhalato S. Hybrid-neonatal EEG seizure detection algorithm achieve the benchmark of visual interpretation of the human expert. In: Conference Proceedings: Annual International Conference of the IEEE Engineering in Medicine and Biology Society. (2019), p. 5991–4. doi: 10.1109/EMBC.2019.8857367
122. Mangold C, Zoretic S, Thallapureddy K, Moreira A, Chorath K, Moreira A. Machine learning models for predicting neonatal mortality: a systematic review. Neonatology. (2021):1-12. doi: 10.1159/000516891
123. Shalish W, Kanbar L, Kovacs L, Chawla S, Keszler M, Rao S, et al. Assessment of extubation readiness using spontaneous breathing trials in extremely preterm neonates. JAMA Pediatr. (2020) 174:178–85. doi: 10.1001/jamapediatrics.2019.4868
124. Fairchild KD. Predictive monitoring for early detection of sepsis in neonatal ICU patients. Curr Opin Pediatr. (2013) 25:172–9. doi: 10.1097/MOP.0b013e32835e8fe6
125. Pereira APE, Leal MC, Gama SGN, Domingues RMSM, Schilithz AOC, Bastos MH. Determinação da idade gestacional com base em informações no estudo Nascer no Brasil. Cad Saúde Pública. (2014) 30:S59–70. doi: 10.1590/0102-311X00160313
126. Yamauchi T, Ochi D, Matsakawa N, Saigusa D, Ishikuro M, Obara T, et al. Machine learning approaches to predict gestational age in normal and complicated pregnancies via urinary metabolomics analysis. Sci Rep. (2021) 11:17777. doi: 10.1038/s41598-021-97342-z
127. Sazawal S, Ryckman KK, Das S, Khanam R, Nisar I, Jasper E, et al. Machine learning guided postnatal gestational age assessment using new-born screening metabolomic data in South Asia and sub-Saharan Africa. BMC Pregnancy Childbirth. (2021) 21:609. doi: 10.1186/s12884-021-04067-y
128. Meenakshi S, Suganthi M, Kumar PS. An approach for automatic detection of fetal gestational age at the third trimester using kidney length and biparietal diameter. Soft Comput. (2019) 23:2839–48. doi: 10.1007/s00500-019-03913-8
129. Koolen L, Oberdorfer L, Rona Z, Giordano V, Werther T, Klebermass-Schrehof K, et al. Automated classification of neonatal sleep states using EEG. Clin Neurophysiol (2017) 128:1100–8. doi: 10.1016/j.clinph.2017.02.025
130. Dereymaeker A, Pillay K, Vervisch J, van Huffel S, Naulaers G, Jansen K, de Vos M. An automated quiet sleep detection approach in preterm infants as a gateway to assess brain maturation. Int J Neural Syst. (2017) 27:1750023. doi: 10.1142/S012906571750023X
131. Paul R, Cho K, Mellins C, Malee K, Robbins R, Keer S, et al. Predicting neurodevelopmental outcomes in children with perinatal HIV using a novel machine learning algorithm. bioRxiv. (2019). doi: 10.1101/632273
132. Le H, Li H, Wang J, Chen M, Gozdas E, Dillman JR, Parikh NA. A multi-task, multi-stage deep transfer learning model for early prediction of neurodevelopment in very preterm infants. Sci Rep. (2020) 10:1–13. doi: 10.1038/s41598-020-71914-x
133. World Health Organization. Telemedicine: Opportunities and Development in Member States: Report on the second global survey on eHealth. Geneva (Switzerland): WHO (2010).
134. World Health Organization. A Health Telematics Policy in Support of WHO's Health-for-All Strategy for Global Health Development. Geneva (Switzerland): WHO (1998).
135. Hall RW, Hall-Barrow J, Garcia-Rill E. Neonatal regionalization through telemedicine using a community based research and education core facility. Ethn Dis. (2010) 20:S1-136-40. Available online at: http://europepmc.org/abstract/MED/20521402
136. Burke BL Jr, Hall RW. Section on telehealth care. Telemed Pediatric Appl Pediatr. (2015) 136:e293–308. doi: 10.1542/peds.2015-1517
Keywords: amplitude-integrated electroencephalography, near-infrared spectroscopy, multimodal monitoring, telemedicine, artificial intelligence, machine learning
Citation: Variane GFT, Camargo JPV, Rodrigues DP, Magalhães M and Mimica MJ (2022) Current Status and Future Directions of Neuromonitoring With Emerging Technologies in Neonatal Care. Front. Pediatr. 9:755144. doi: 10.3389/fped.2021.755144
Received: 08 August 2021; Accepted: 18 November 2021;
Published: 23 March 2022.
Edited by:
Gunnar Naulaers, KU Leuven, BelgiumReviewed by:
Deepak Chawla, Government Medical College and Hospital, IndiaFrank Van Bel, University Medical Center Utrecht, Netherlands
Vito Giordano, Medical University of Vienna, Austria
Copyright © 2022 Variane, Camargo, Rodrigues, Magalhães and Mimica. This is an open-access article distributed under the terms of the Creative Commons Attribution License (CC BY). The use, distribution or reproduction in other forums is permitted, provided the original author(s) and the copyright owner(s) are credited and that the original publication in this journal is cited, in accordance with accepted academic practice. No use, distribution or reproduction is permitted which does not comply with these terms.
*Correspondence: Gabriel Fernando Todeschi Variane, gabriel.variane@pbsf.com.br