- 1Department of Pediatrics and Child Health, Faculty of Medicine and Health Sciences, Stellenbosch University, Cape Town, South Africa
- 2Foundation for Innovative New Diagnostics, Geneva, Switzerland
- 3Division IC Neonatology (NICU), Department of Pediatrics, VU University Medical Center, Amsterdam, Netherlands
Background and objectives: Infection prediction scores are useful ancillary tests in determining the likelihood of neonatal hospital-acquired infection (HAI), particularly in very low birth weight (VLBW; <1,500 g) infants who are most vulnerable to HAI and have high antibiotic utilization rates. None of the existing infection prediction scores were developed for or evaluated in South African VLBW neonates.
Methods: We identified existing infection prediction scores through literature searches and assessed each score for suitability and feasibility of use in resource-limited settings. Performance of suitable scores were compared using a retrospective dataset of VLBW infants (2016–2017) from a tertiary hospital neonatal unit in Cape Town, South Africa. Sensitivity, specificity, predictive values, and likelihood ratios were calculated for each score.
Results: Eleven infection prediction scores were identified, but only five were suitable for use in resource-limited settings (NOSEP1, Singh, Rosenberg, and Bekhof scores). The five selected scores were evaluated using data from 841 episodes of HAI in 659 VLBW infants. The sensitivity for the scores ranged between 3% (NOSEP1 ≥14; proven and presumed infection), to a maximum of 74% (Singh score ≥1; proven infection). The specificity of these scores ranged from 31% (Singh score ≥1; proven and presumed infection) to 100% (NOSEP1 ≥11 and ≥14, NOSEP-NEW-1 ≥11; proven and presumed infection).
Conclusion: Existing infection prediction scores did not achieve comparable predictive performance in South African VLBW infants and should therefore only be used as an adjunct to clinical judgment in antimicrobial decision making. Future studies should develop infection prediction scores that have high diagnostic accuracy and are feasible to implement in resource-limited neonatal units.
Introduction
In 2015, neonatal infections contributed to over 500,000 deaths worldwide, the majority of which occurred in low- and middle-income countries (LMICs) (1, 2). Hospital-acquired infection (HAI; >72 h following birth) occurs 3–20 times more frequently in LMICs than in high-income countries and is a major contributor to neonatal morbidity and mortality (3). HAI among preterm (<37 weeks gestational age) and very low birth weight (VLBW; <1,500 g) infants is one of the most frequent complications encountered in neonatal units worldwide, affecting up to 20% of VLBW infants (blood culture positive episodes; proven HAI) with 28% experiencing multiple infection episodes (3). Reported mortality from proven HAI in Africa ranges from 27 to 72% (4–6), with up to 14–42% of surviving infants suffering significant cognitive impairment and 9–21% suffering from cerebral palsy secondary to central nervous system involvement, septic shock, and hypoxemia (7–9).
Despite HAI being common in neonates, the diagnosis thereof is challenging. Blood culture is considered the gold standard for the diagnosis of neonatal blood stream infections; however, the blood culture positivity rate is low and is affected by factors such as the volume of blood inoculated, the level of bacteremia and laboratory capability (10). Newer tests, such as interleukin-6, interleukin-8, procalcitonin, and real-time polymerase chain reaction (PCR) assays for bacterial detection (11, 12) have been investigated for applicability in neonates with HAI, but are not yet available in most resource-limited settings. For this reason, clinicians make use of additional tools such as infection prediction scores, i.e., combinations of clinical evaluations and readily available laboratory screening tests such as complete blood counts and C-reactive protein (CRP) tests, to guide decisions regarding empiric antimicrobial treatment initiation in neonates suspected to have HAI (13–15).
Although clinically useful, infection prediction scores have variable performance in different practice settings and should be validated locally before incorporation into routine use (16). When using infection prediction scores, clinicians should consider its diagnostic accuracy to avoid inappropriate or unnecessary antimicrobial therapy, which may promote development of antimicrobial resistance, compromise gastro-intestinal immunity, and contribute to adverse clinical outcomes (17, 18). There are several infection prediction scores available as screening tests for HAI in neonates. The first infection prediction score was developed by Tollner in Germany almost 40 years ago, using a combination of clinical evaluations and laboratory investigations (19). Subsequently, scores were developed in a variety of settings, including Belgium, Australia, India, Bangladesh, and Thailand, but none has been developed and validated in Africa. Diagnostic accuracy of these infection prediction scores varies widely across these existing models. In a meta-analysis of twelve infection prediction models (13), a NOSEP1 score of 8 or more by Mahieu et al. (20, 21) which made use of clinical and laboratory evaluations, showed the most potential for use, but authors cautioned that these models should be considered as guidance rather than an absolute indicator to commence or withhold antimicrobial treatment (13). Based on the vast differences in resources available in high-income countries compared to LMICs, scores which rely on indicators such as continuous heart rate monitoring, blood pressure monitoring, and laboratory testing may lack generalizability to LMIC settings.
The overall objective of this study was to evaluate the performance of neonatal infection prediction scores at a large, resource-limited South African neonatal unit with a high proportion of VLBW infant deliveries.
Materials and Methods
Study Design
Stage 1 of the study included a literature search strategy to identify English language publications pertaining to neonatal infection prediction scores, searching PubMed, Medline, and EBSCO Host databases between 1970 and 2020 or since inception, with inclusion of publications identified in the gray literature. Existing infection prediction scores were evaluated for their suitability and feasibility of application in LMIC neonatal units by assessing the availability of each score variable within neonatal units in a low-resource setting.
In Stage 2, performance comparison of the selected scores deemed appropriate and feasible for use in LMIC settings was performed against an existing dataset of HAI episodes in VLBW infants from Tygerberg Hospital, Cape Town, South Africa. The dataset was a retrospectively collected REDCap dataset of VLBW infants >72 h of age admitted between 1 January 2016–31 December 2017 (22, 23) which had been collected for an epidemiological study (publication under review). Since VLBW infants frequently have a hospital stay in excess of the 28-day neonatal period, any VLBW infants investigated for HAI during their neonatal unit stay were included. Medical records including demographic data, risk factors for HAI, relevant laboratory data, and clinical signs and symptoms on the day of investigation for suspected HAI were reviewed.
Study Definitions and Population
Hospital-acquired infection episodes occurring after 72 h of admission to the neonatal unit were classified into three categories (24):
1. Proven HAI: Positive blood culture. Organisms were classified using the United States Centers for Disease Control (US CDC) list of pathogens and contaminants (25). Repeat blood cultures isolating the same pathogen within 10 days of the original specimen were considered to represent a single episode of infection. Patients who isolated coagulase-negative staphylococci (CoNS) from two separate blood cultures taken 24–48 h apart, or from a single positive blood culture combined with a serum CRP ≥10 mg/L and clinical features suggestive of infection, were included in the analysis. Contaminants were excluded from further analysis.
2. Presumed HAI: Clinical signs and symptoms of infection, such as respiratory distress, apnea, tachycardia, abdominal distention, temperature instability, lethargy, and vomiting; in the presence of a CRP ≥10 mg/L and a negative blood culture, where antibiotic treatment was continued for ≥5 days.
3. Excluded HAI: This included patients with short-term symptoms but no objective findings of infection, with negative blood culture, CRP <10 mg/L, where antibiotics were discontinued within 48–72 h based on local treatment guidelines.
Positive cultures from other sterile sites, e.g., cerebrospinal fluid and urine, and viral infections were not included in the analysis as the relevant data was not available.
The diagnosis of bronchopulmonary dysplasia was based on the Vermont Oxford Network algorithm of supplemental oxygen requirement at 36 weeks postmenstrual age (26). Patent ductus arteriosus was diagnosed according to the Vermont Oxford Network definition which incorporates a combination of Doppler echocardiogram and clinical criteria (26). Severe intraventricular hemorrhage was defined as grades III and IV hemorrhage according to the grading method described by Papile et al. (27).
Tygerberg Hospital is a 1,384-bed public teaching hospital in the Western Cape, South Africa. The obstetric-neonatal service manages approximately 8,000 high-risk deliveries (37% low birth weight; <2,500 g) and 3,000 neonatal admissions annually (28). The 132-bed neonatal unit includes a 12-bed neonatal intensive care unit (NICU), three high-dependency wards, and one kangaroo mother care ward.
Statistical Analysis and Evaluation of Existing Scores
Statistical analysis was performed using IBM SPSS Statistics for Macintosh, Version 27.0 using an α level of 0.05. For normally distributed continuous variables means and standard deviations were calculated. Medians and interquartile ranges (IQR) were used for non-normally distributed continuous data.
Diagnostic test evaluation was performed using MedCalc Software, version 20.0.5. For each score the sensitivity, specificity, positive predictive value (PPV), negative predictive value (NPV), and likelihood ratios were calculated for proven HAI, and proven and presumed HAI, using the local dataset. Where specific variables in our VLBW infants were not available, it was replaced with a suitable related variable, e.g., pre-feed aspirates are not routinely performed in our setting; thus, this variable was replaced with vomiting in calculating the Singh score (29). Episodes where any of the variables were missing were not included in the analysis. Score cut-off values were based on those used in the original studies. A good screening test should have a low false-negative rate, and thus high sensitivity (30). A test with a positive likelihood ratio (PLR) of >10 or conversely a negative likelihood ratio (NLR) of <0.1, is considered a good screening test (30, 31). The discriminative performance of the scores were evaluated by assessing their receiver operating characteristic (ROC) curves and area under ROC curves (AUC).
All findings were reported in accordance with the STROBE-NI criteria (32). The Stellenbosch University Health Research Ethics Committee and the Tygerberg Hospital management reviewed and approved the study protocol (S20/11/325).
Results
Identification and Selection of Existing Neonatal Infection Prediction Scores for the Purpose of Performance Comparison
Eleven infection prediction scores were identified from the literature search (Table 1). Five of these scores [Tollner (19), Okascharoen et al. (33), Rubarth (34), Walker et al. (35), and Husada et al. (36)] were evaluated as unsuitable for use in resource-limited setting such as our South African unit as such as blood gas analysis, blood pressure monitoring, and continuous heart rate monitoring were not routinely performed on all patients. The Rodwell et al. hematological score (37) was also excluded as it uses seven hematological parameters, of which four require differential white blood cell counts. Although the authors stated that one or more parameters may be omitted without affecting the outcome of the score, the unreliability of using the differential count to diagnose infections was demonstrated by Schelonka et al. (38).
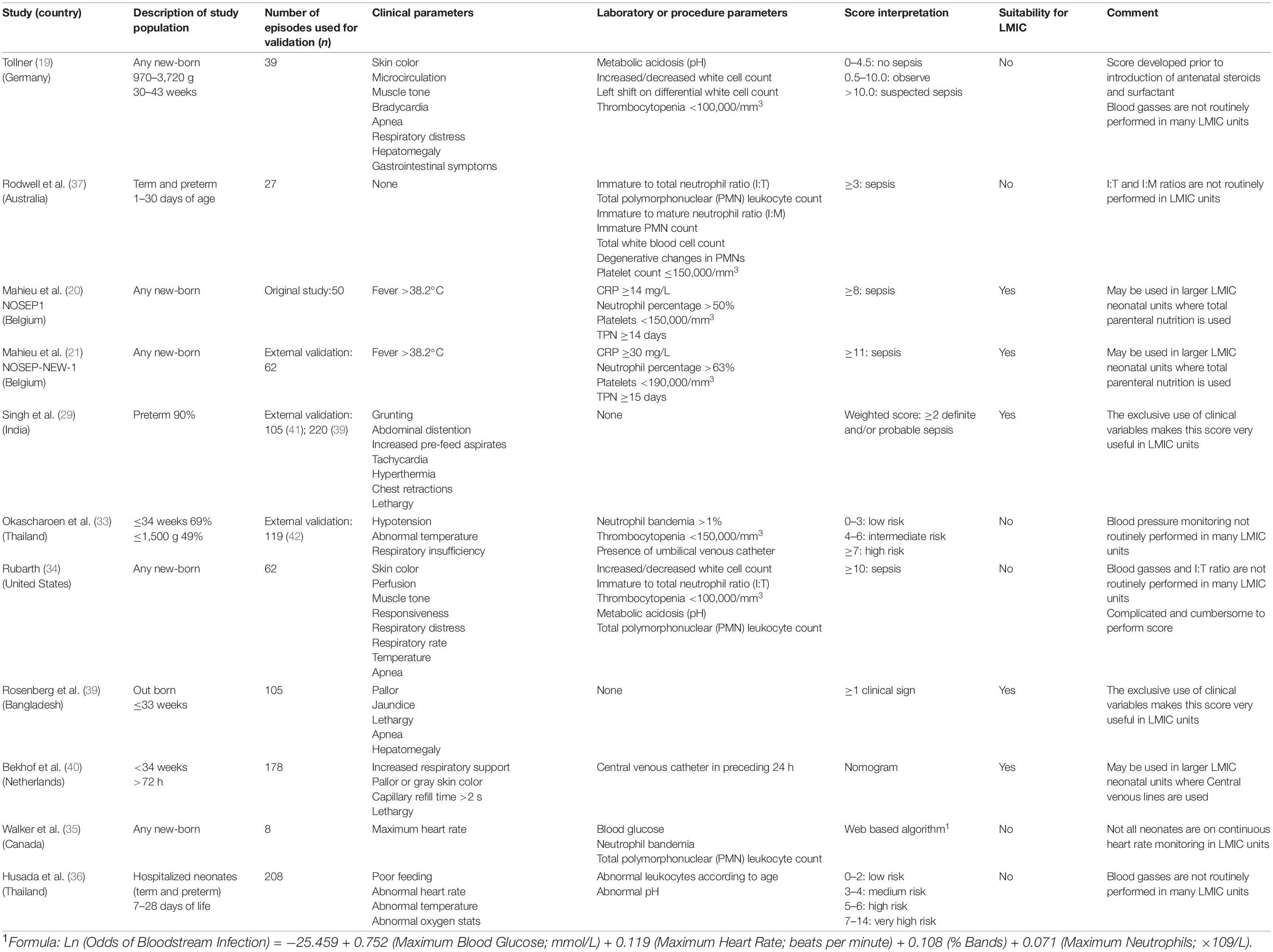
Table 1. Description of existing infection prediction scores for hospital-acquired infection in neonates.
Only five of the scores listed in Table 1 were considered feasible and appropriate for resource-limited settings (NOSEP1, NOSEP-NEW1, Singh, Rosenberg, and Bekhof scores) (20, 21, 29, 39, 40) and were therefore included in the current analysis. The NOSEP1 score included three laboratory features (CRP, platelet count, and neutrophil percentage), one clinical feature (fever) and one intervention (total parenteral nutrition), all of which are available in our setting (20). A variation of the NOSEP1 score, the NOSEP-NEW1, included the same parameters as the NOSEP1 score but used different cut-off values for each individual parameter (21). The Singh and Rosenberg scores used only clinical features, making them particularly useful in a resource-limited setting (29, 39). The Bekhof score consisted of three clinical features and one intervention (presence of a central venous catheter in the last 24 h) (40).
Description of the Study Population
Over a 2-year period, 1,510 VLBW neonates >72 h of age were admitted to the Tygerberg Hospital neonatal unit, of which 731 were investigated for 1,694 episodes of infection. Of these, 658 neonates with 841 episodes of HAI were eligible to be included in this study (Figure 1). Of the 841 suspected episodes of HAI investigated, 224 (26.6%) were proven HAI, 227 (27.0%) were presumed HAI and in 390 (46.4%) HAI was excluded.
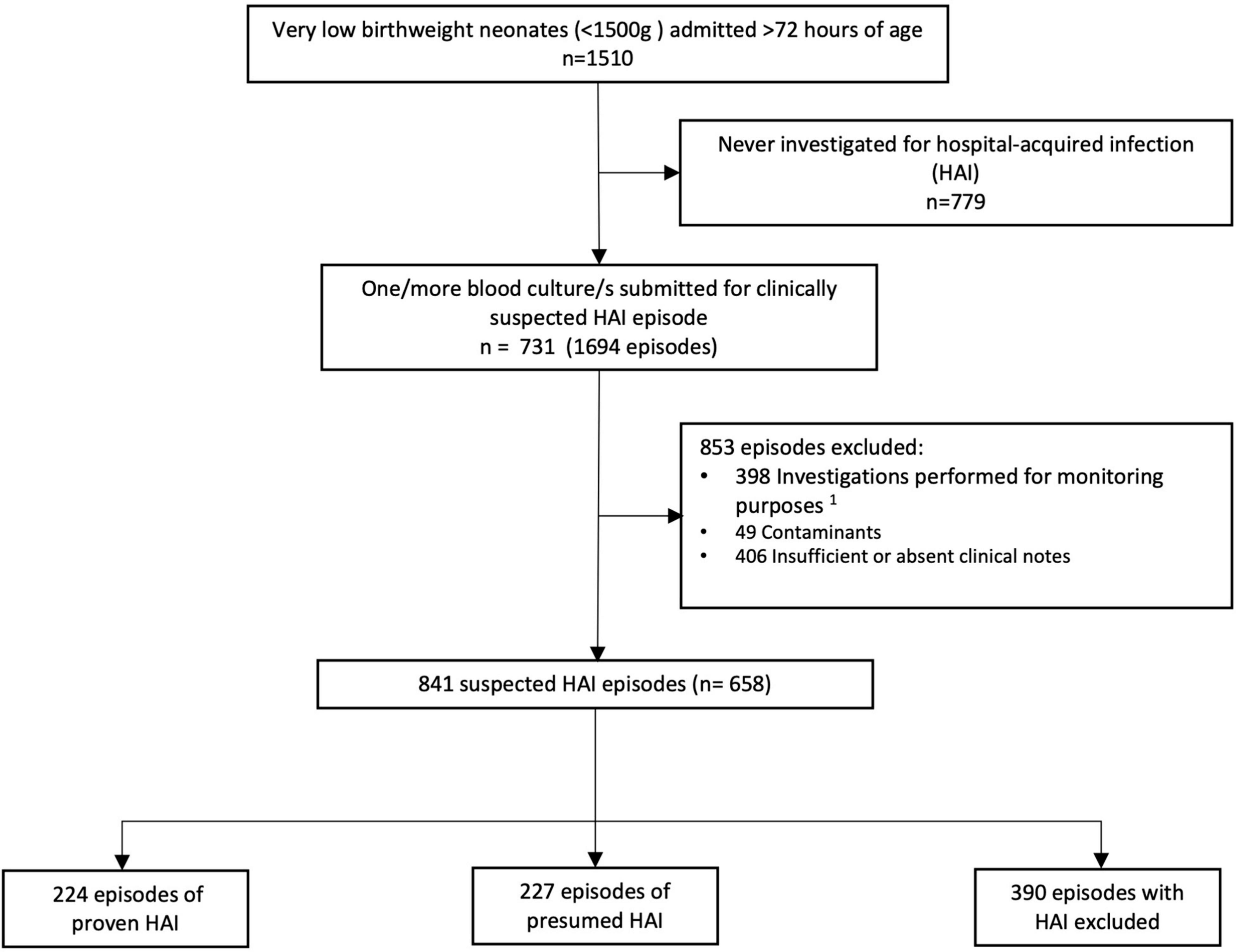
Figure 1. Flow diagram of neonatal hospital-acquired infection episodes in very low birth weight infants included in the analysis. 1Monitoring purposes: this refers to blood culture/s performed after 72 h of life in response to a positive blood culture or raised CRP obtained <72 h of life to monitor response to antimicrobial therapy.
The study population had a median birth weight and gestational age of 1,060 g and 28 weeks, respectively (Table 2). The majority of these infants were delivered via cesarean section and 10.3% were born outside of the tertiary center. Bronchopulmonary dysplasia, patent ductus arteriosus, and severe intraventricular hemorrhage were diagnosed in 18 (2.7%), 90 (13.7%), and 28 (4.2%), respectively. The overall mortality was 15.9%.
There was a Gram-negative predominance among the episodes of proven HAI (Table 3). At the time of investigation 83 (12.6%) had a central venous catheter in situ, and 24 (3.6%) were receiving total parenteral nutrition.
Performance Comparison of Infection Prediction Scores (Table 4)
Proven Hospital-Acquired Infection
The highest sensitivity of 74% was achieved by the Singh score ≥1, paired with low specificity and PPV of 33 and 28%, respectively (Table 4). A NOSEP1 score ≥11 achieved a low sensitivity of 25%, specificity of 95%, NPV of 80%, and PLR of 5.37 and a NOSEP ≥14 achieved a sensitivity of 4%, specificity 99%, PPV 70%, and the highest PLR of 7.21. On the ROC curve analysis for the scores to compare the discriminative performance, the NOSEP1 and NOSEP-NEW1 achieved the highest AUC of 0.753 and 0.737, respectively (Figure 2).
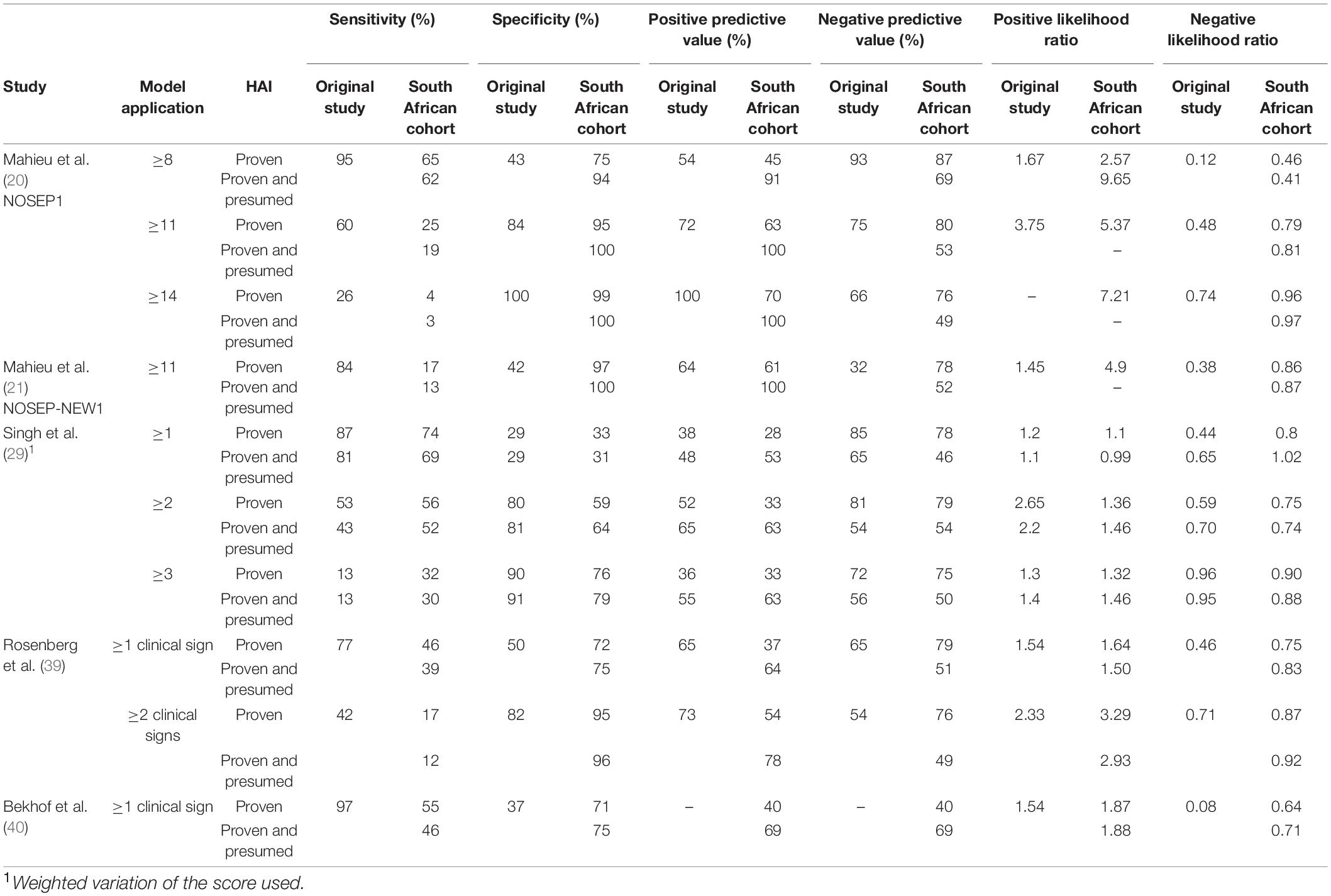
Table 4. Performance comparison of previously reported infection prediction scores in very low birth weight infants at a South African neonatal unit, compared to the original studies.
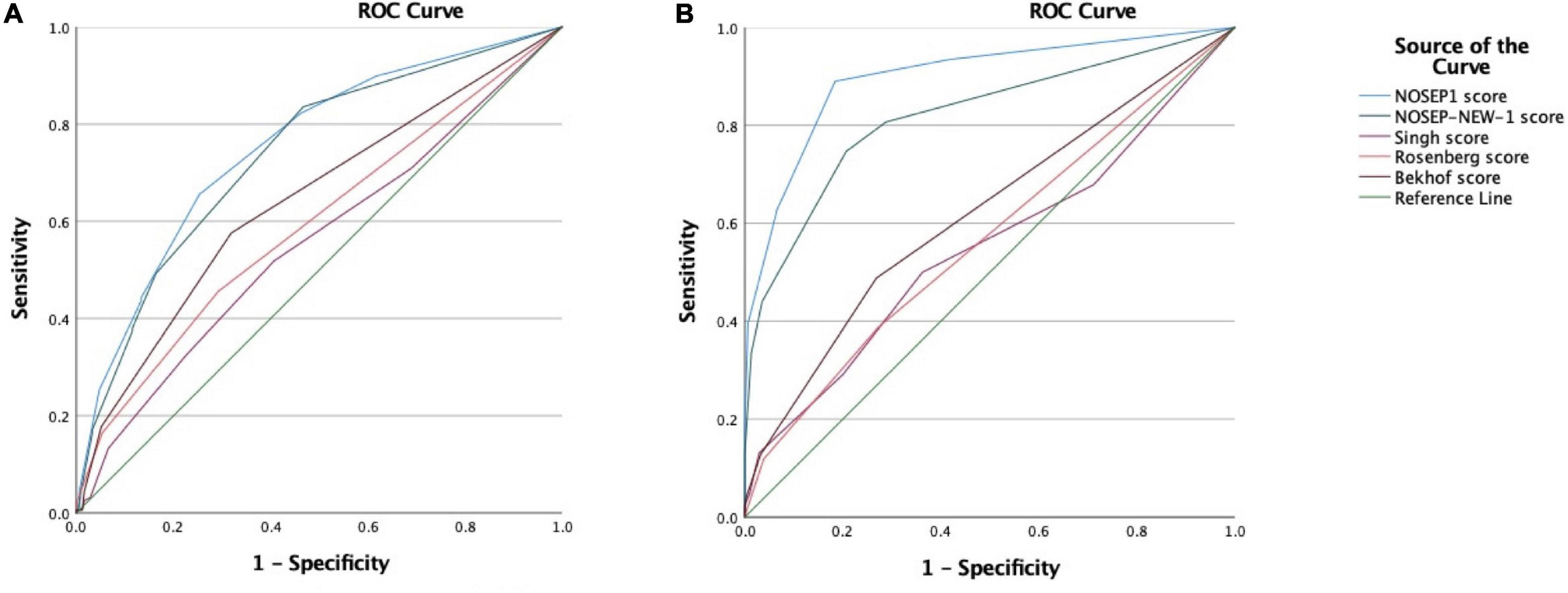
Figure 2. (A) Graph showing the receiver operating characteristic (ROC) curves for the analysis of the diagnostic accuracy of the NOSEP1 score (area under curve 0.753), NOSEP-NEW-1 score (area under curve 0.737), Singh score (area under curve 0.555), Rosenberg score (area under curve 0.594), and Bekhof score (area under curve 0.641), for the prediction of proven hospital-acquired infection. (B) Graph showing the ROC curves for the analysis of the diagnostic accuracy of the NOSEP1 score (area under curve 0.898), NOSEP-NEW-1 score (area under curve 0.820), Singh score (area under curve 0.550), Rosenberg score (area under curve 0.566), and Bekhof score (area under curve 0.620), for the prediction of proven hospital-acquired infection and/or presumed hospital-acquired infection. The reference line on both graphs represents a curve with no predictive value (area under curve = 0.50).
Proven and Presumed Hospital-Acquired Infection
A NOSEP1 score of ≥8 achieved a sensitivity of 62%, specificity of 94%, PPV of 91%, PLR of 9.65 and NLR of 0.41 (Table 4). The Singh score ≥1 achieved a higher sensitivity of 69%, and the NOSEP1 ≥11 and ≥14, as well as the NOSEP-NEW1 ≥11 all achieved specificities and PPVs of 100%. The highest AUC on the ROC curve analysis was achieved by the NOSEP1 and NOSEP-NEW1 of 0.898 and 0.820, respectively (Figure 2).
Discussion
None of the five infection prediction scores we retrospectively evaluated, achieved sufficient diagnostic accuracy to recommend their routine use in our setting. To rule-out HAI, a score would have to achieve a high sensitivity (≥95% for a potentially lethal condition (16) and a NLR <0.1 (30, 31). None of the scores we evaluated can be used to rule-out HAI in our setting. To rule-in disease, a high specificity and PLR >10 is needed (30, 31). The NOSEP1 ≥8 achieved a specificity of 94% and PLR of 9.65 for proven and presumed infection and may be a useful adjunct to rule-in disease in our setting.
The strength of our study lies in the number of patients used to evaluate the infection prediction scores, far exceeding the sample size used in the original studies and including a previously unrepresented South African study population. The discrepancy found in the performance of these scores may be attributed to the fact that in this cohort we included only VLBW infants, admitted to a tertiary unit in a resource-limited setting. As VLBW infants have an increased risk of contracting a HAI during their hospital stay, we felt that they were the appropriate target group to use in this study. VLBW infants have a higher prevalence of respiratory symptoms due to prematurity, limiting the validity of any score that utilizes respiratory parameters.
A major limitation of our study was the inability to assess all the available infection prediction scores. This can be ascribed to the retrospective nature of the study, as well as our resource-limited setting. In our unit, the majority of the VLBW infants are cared for outside of the NICU in high- or intermediate care wards. Consequently, blood pressure and continuous heart rate monitoring data are not routinely available. Blood gas analysis is usually performed during a resuscitation and for ventilated patients but is seldom performed in patients investigated for HAI (Table 1).
The use of proven HAI, as well as proven and presumed HAI may be viewed as both a strength, and a limitation. Except for the Singh score, all the studies used a positive blood culture (proven HAI) as the reference standard, thus our comparison of proven HAI to combined proven and presumed HAI may provide valuable data to neonatal units where access to microbiological services may be limited. Four of the five studies reported a Gram-positive predominance amongst their positive cultures, compared to our Gram-negative predominance, and this may also have contributed to the different findings in our cohort.
Mahieu et al. developed and validated the NOSEP1 prediction score (20, 21) with one clinical, three laboratory, and one treatment variable, making its practical application in a resource-limited setting, where central venous catheters and total parenteral nutrition is often not available, difficult. The score performed well in ruling-in HAI in this South African cohort, with high specificity and high PLR. For the NOSEP1 score, Mahieu et al. (20) reported a sensitivity of 95% for a score of ≥8 for proven HAI, compared to our 65% for proven HAI, and 62% for proven and presumed infection. The Mahieu study included all neonates, with only 52% VLBW infants (54/80) in the study and had a Gram-positive pathogen predominance (82%) compared to the relative predominance of Gram-negative pathogens in our unit (48%). In a meta-analysis of prediction scores by Verstraete et al. (13), they concluded that the NOSEP1 score of greater than 8 had the greatest potential for use in the clinical setting. In our setting, it is likely most useful to rule-in HAI due to its high specificity and PLR. The NOSEP-NEW1 was developed as a variation on the NOSEP1 score and was found to improved performance in the external validation group in their study (21), however in our cohort it performed similar to the NOSEP1 score.
Singh et al. (29) developed a clinical score, specifically for resource-limited settings. Their score assessed for the presence of 7 clinical signs, regardless of gestational age. Kudawla et al. (41) performed an external validation in similar settings and achieved a sensitivity of 90% for a score ≥1, which compared well to the sensitivity of 87% that was achieved in the original study. In our cohort, the Singh score did achieve the highest sensitivity of all the scores assessed, but this was paired with the lowest specificity and PLR, which limits its usefulness in our setting. This difference is difficult to explain, as the Singh study cohort was very similar to ours, consisting of 91% preterm infants, and was performed in a tertiary level neonatal department in a resource-limited setting. The Singh score was also the only one that included proven and presumed infection in their analysis. However, in the Singh study the most common organism was Staphylococcus aureus (30%), at an incidence rate almost double that seen in our study (16%), which may partially explain the difference.
Rosenberg et al. (39) also failed to validate the Singh score in a cohort of premature infants and found that the inclusion of respiratory symptoms in a score that is used to assess premature infants who have a high incidence of underlying respiratory conditions unrelated to infection, was not appropriate. The Rosenberg score, with 5 clinical signs which did not include any respiratory parameters, achieved high specificity (95 and 96%) and moderately high PLR of 3.29 and 2.92 in the presence of ≥2 clinical signs in our cohort. In the original study they achieved higher sensitivity (42 vs 17% and 12% in our study), and a lower specificity. The cohort they used was similar (≤33-week gestational age infants) with a predominance of Gram-negative organisms of 87%, however they only used infants born outside of the referral facility compared to our cohort that had only 10.3% born outside of the referral facility. A recent review on impact of place of birth on outcomes of babies born between 1,000 and 1,500 g failed to demonstrate any difference, however, the review did not include any LMIC units, and further research is needed on this topic (43). The Rosenberg score has the potential to be used to rule-in disease in our setting.
The score developed by Bekhof et al. (40) incorporated only clinical signs, with one management parameter (central venous catheter for >24 h). Despite the patient cohort being similar in gestation (this study only included <34 weeks’ gestation premature babies), they achieved sensitivity and specificity of 97 and 37% in the original study, but we failed to replicate those results with a sensitivity of 55 and 46% and specificity of 71 and 75% in our cohort. As the Bekhof study was performed in a high-income setting with a Gram-positive HAI pathogen predominance, this may contribute to the difference in diagnostic accuracy when compared to our resource-limited setting. Neonates with Gram-negative HAI have a higher risk of adverse outcomes and death compared to Gram-positive HAI or no sepsis (44, 45).
The generalizability and feasibility of existing infection prediction scores in similar resource-limited neonatal units is an important consideration when diagnosing HAI in neonates and considering initiation of antibiotic treatment. An optimal infection prediction score should be user friendly, statistically sound, and generalizable to clinical settings outside of those in which the score was developed. External validation should be performed at another center or by different individuals (46).
Conclusion
None of the five infection prediction scores evaluated in our study can be recommended for routine use in our setting. Future studies should develop bedside infection prediction scores using easily available clinical information and should assess the impact of infection scores on antibiotic prescribing behavior, until new low-cost and easy to use technologies can support HAI diagnosis.
Data Availability Statement
The raw data supporting the conclusions of this article will be made available by the authors, without undue reservation.
Ethics Statement
The studies involving human participants were reviewed and approved by the Stellenbosch University Health Research Ethics Committee. Written informed consent from the participants’ legal guardian/next of kin was not required to participate in this study in accordance with the national legislation and the institutional requirements.
Author Contributions
LL, AD, AB, and MV conceptualized the study. LL collected and analyzed the data and prepared the first draft. All authors read, edited, and approved the final manuscript.
Funding
LL received funding for this study from FIND, Diagnosis for all. AD was supported by an NIH Fogarty Emerging Global Leader Award (K43 TW010682).
Conflict of Interest
The authors declare that the research was conducted in the absence of any commercial or financial relationships that could be construed as a potential conflict of interest.
Publisher’s Note
All claims expressed in this article are solely those of the authors and do not necessarily represent those of their affiliated organizations, or those of the publisher, the editors and the reviewers. Any product that may be evaluated in this article, or claim that may be made by its manufacturer, is not guaranteed or endorsed by the publisher.
Acknowledgments
We thank the patients and staff of the Tygerberg Hospital Neonatal Unit. LL thanks her sponsors FIND for their contribution to the study. Study data was collected and managed using REDCap electronic data capture tools hosted at Stellenbosch University.
Abbreviations
CRP, C-reactive protein; HAI, hospital-acquired infection; LMIC, lower-middle income country; PLR, positive likelihood ratio; PPV, positive predictive value; NICU, neonatal intensive care unit; NLR, negative likelihood ratio; NPV, negative predictive value; VLBW, very low birth weight.
References
1. Liu L, Oza S, Hogan D, Chu Y, Perin J, Zhu J, et al. Global, regional, and national causes of under-5 mortality in 2000–15: an updated systematic analysis with implications for the sustainable development goals. Lancet. (2016) 388:3027–35. doi: 10.1016/S0140-6736(16)31593-8
2. Lawn JE, Cousens S, Zupan J. Neonatal survival 1 4 million neonatal deaths: when? where? why? Lancet. (2005) 365:891–900. doi: 10.1016/S0140-6736(05)71048-5
3. Zaidi AKM, Huskins WC, Thaver D, Bhutta ZA, Abbas Z, Goldmann DA. Hospital-acquired neonatal infections in developing countries. Lancet. (2005) 365:1175–88. doi: 10.1016/S0140-6736(05)71881-X
4. English M, Ngama M, Musumba C, Wamola B, Bwika J, Mohammed S, et al. Causes and outcome of young infant admissions to a Kenyan district hospital. Arch Dis Child. (2003) 88:438–43. doi: 10.1136/adc.88.5.438
5. Kabwe M, Tembo J, Chilukutu L, Chilufya M, Ngulube F, Lukwesa C, et al. Etiology, antibiotic resistance and risk factors for neonatal sepsis in a large referral center in Zambia. Pediatr Infect Dis J. (2016) 35:e191–8. doi: 10.1097/INF.0000000000001154
6. Campagne G, Schuchat A, Djibo S, Ousséini A, Cissé L, Chippaux J. Epidemiology of bacterial meningitis in Niamey, Niger, 1981-96. Bull World Health Organ. (1999) 77:499–508.
7. Haller S, Deindl P, Cassini A, Suetens C, Zingg W, Abu Sin M, et al. Neurological sequelae of healthcare-associated sepsis in very-low-birthweight infants: umbrella review and evidence-based outcome tree. Euro Surveill. (2016) 21:1–10. doi: 10.2807/1560-7917.ES.2016.21.8.30143
8. Woldu MA, Guta MB, Lenjisa JL, Tegegne GT, Tesafye G, Dinsa H, et al. Assessment of the incidence of neonatal sepsis, its risk factors, antimicrobials use and clinical outcomes in Bishoftu general hospital, neonatal intensive care unit, Debrezeit-Ethiopia. Int J Contemp Pediatr. (2017) 1:135–41.
9. Mitha A, Foix-L’Hélias L, Arnaud C, Marret S, Vieux R, Aujard Y, et al. Neonatal infection and 5-year neurodevelopmental outcome of very preterm infants. Pediatrics. (2013) 132:e372–80. doi: 10.1542/peds.2012-3979
10. Zea-Vera A, Ochoa TJ. Challenges in the diagnosis and management of neonatal sepsis. J Trop Pediatr. (2015) 61:1–13. doi: 10.1093/tropej/fmu079
11. Dillenseger L, Langlet C, Iacobelli S, Lavaux T, Ratomponirina C, Labenne M, et al. Early inflammatory markers for the diagnosis of late-onset sepsis in neonates: the nosodiag study. Front Pediatr. (2018) 6:346. doi: 10.3389/fped.2018.00346
12. Van den Brand M, Peters RPH, Catsburg A, Rubenjan A, Broeke FJ, Van den Dungen FAM, et al. Development of a multiplex real-time PCR assay for the rapid diagnosis of neonatal late onset sepsis. J Microbiol Methods. (2014) 106:8–15. doi: 10.1016/j.mimet.2014.07.034
13. Verstraete EH, Blot K, Mahieu L, Vogelaers D, Blot S. Prediction models for neonatal health care-associated sepsis: a meta-analysis. Pediatrics. (2015) 135:e1002–14. doi: 10.1542/peds.2014-3226
14. Dong Y, Glaser K, Speer CP. Late-onset sepsis caused by Gram-negative bacteria in very low birth weight infants: a systematic review. Expert Rev Anti Infect Ther. (2019) 17:177–88. doi: 10.1080/14787210.2019.1568871
15. Paolucci M, Landini MP, Sambri V. How can the microbiologist help in diagnosing neonatal sepsis? Int J Pediatr. (2012) 2012:1–14. doi: 10.1155/2012/120139
16. Trevethan R. Sensitivity, specificity, and predictive values: foundations, pliabilities, and pitfalls in research and practice. Front Public Health. (2017) 5:307. doi: 10.3389/fpubh.2017.00307
17. Gordon A, Jeffery HE, Gordon A, Jeffery HE. Antibiotic regimens for suspected late onset sepsis in newborn infants. Cochrane Database Syst Rev. (2005) 2005:CD004501. doi: 10.1002/14651858.CD004501.pub2
18. Apisarnthanarak A, Holzmann-Pazgal G, Hamvas A, Olsen MA, Fraser VJ. Antimicrobial use and the influence of inadequate empiric antimicrobial therapy on the outcomes of nosocomial bloodstream infections in a neonatal intensive care unit. Infect Control Hosp Epidemiol. (2004) 25:735–41. doi: 10.1086/502469
19. Tollner U. Early diagnosis of septicemia in the newborn: clinical studies and sepsis score. Eur J Pediatr. (1982) 138:331–7. doi: 10.1007/BF00442511
20. Mahieu LM, De Muynck AO, De Dooy JJ, Laroche SM, Van Acker KJ. Prediction of nosocomial sepsis in neonates by means of a computer– weighted bedside scoring system (NOSEP score). Crit Care Med. (2000) 28:2026–33. doi: 10.1097/00003246-200006000-00058
21. Mahieu LM, de Dooy JJ, Cossey VR, Goossens LL, Vrancken SL, Jespers AY, et al. Internal and external validation of the NOSEP prediction score for nosocomial sepsis in neonates. Crit Care Med. (2002) 30:1459–66. doi: 10.1097/00003246-200207000-00010
22. Harris PA, Taylor R, Thielke R, Payne J, Gonzalez N, Conde JG. Research electronic data capture (REDCap)–a metadata-driven methodology and workflow process for providing translational research informatics support. J Biomed Inform. (2009) 42:377–81. doi: 10.1016/j.jbi.2008.08.010
23. Harris PA, Taylor R, Minor BL, Elliott V, Fernandez M, O’Neal L, et al. The REDCap consortium: building an international community of software platform partners. J Biomed Inform. (2019) 95:103208. doi: 10.1016/j.jbi.2019.103208
24. Wirtschafter DD, Padilla G, Suh O, Wan K, Trupp D, Simon Fayard EE. Antibiotic use for presumed neonatally acquired infections far exceeds that for central line-associated blood stream infections: an exploratory critique. J Perinatol. (2011) 31:514–8. doi: 10.1038/jp.2011.39
25. Centers for Disease Control and Prevention and the NHS Network. CDC/NHSH Bloodstream Infection Event (Central Line-Associated Bloodstream Infection and Non-Central Lineassociated Bloodstream Infection). (2015). Available online at: http://www.cdc.gov/nhsn/XLS/Common-SkinContaminant-List-June-2011.xlsx (accessed October 28, 2020).
26. VO Network. Vermont Oxford Netowork 2018 Manual of Operations: Part 2. Data Definitions and Infant Data Forms. Vermont: VON (2017).
27. Papile LA, Burstein J, Burstein R, Koffler H. Incidence and evolution of subependymal and intraventricular hemorrhage: a study of infants with birth weights less than 1,500 gm. J Pediatr. (1978) 92:529–34. doi: 10.1016/s0022-3476(78)80282-0
28. Milambo JPM, Cho K, Okwundu C, Olowoyeye A, Ndayisaba L, Chand S, et al. Newborn follow-up after discharge from a tertiary care hospital in the Western Cape region of South Africa: a prospective observational cohort study. Glob Health Res Policy. (2018) 3:2. doi: 10.1186/s41256-017-0057-4
29. Singh SA, Dutta S, Narang A. Predictive clinical scores for diagnosis of late onset neonatal septicemia. J Trop Pediatr. (2003) 49:235–9. doi: 10.1093/tropej/49.4.235
30. Karakaya J. Evaluation of binary diagnostic tests accuracy for medical researches. Turk J Biochem. (2020) 46:103–13. doi: 10.1515/tjb-2020-0337
32. Fitchett EJA, Seale AC, Vergnano S, Sharland M, Heath PT, Saha SK, et al. Strengthening the reporting of observational studies in epidemiology for newborn infection (STROBE-NI): an extension of the STROBE statement for neonatal infection research. Lancet Infect Dis. (2016) 16:e202–13. doi: 10.1016/S1473-3099(16)30082-2
33. Okascharoen C, Sirinavin S, Thakkinstian A, Kitayaporn D, Supapanachart SA. Bedside prediction-scoring model for late-onset neonatal sepsis. J Perinatol. (2005) 25:778–83. doi: 10.1038/sj.jp.7211404
34. Rubarth L. Nursing patterns of knowing in assessment of newborn sepsis. Clin Nurse Spec. (2007) 21:15–156.
35. Walker SAN, Cormier M, Elligsen M, Choudhury J, Rolnitsky A, Findlater C, et al. Development, evaluation and validation of a screening tool for late onset bacteremia in neonates – a pilot study. BMC Pediatr. (2019) 19:253. doi: 10.1186/s12887-019-1633-1
36. Husada D, Chanthavanich P, Chotigeat U, Sunttarattiwong P, Sirivichayakul C, Pengsaa K, et al. Predictive model for bacterial late-onset neonatal sepsis in a tertiary care hospital in Thailand. BMC Infect Dis. (2020) 20:151. doi: 10.1186/s12879-020-4875-5
37. Rodwell RL, Leslie AL, Tudehope DI. Early diagnosis of neonatal sepsis using a hematologic scoring system. J Pediatr. (1988) 112:761–7. doi: 10.1016/s0022-3476(88)80699-1
38. Schelonka RL, Yoder BA, Hall RB, Trippett TM, Louder DS, Hickman JR, et al. Differentiation of segmented and band neutrophils during the early newborn period. J Pediatr. (1995) 127:298–300. doi: 10.1016/s0022-3476(95)70314-4
39. Rosenberg RE, Ahmed ASMNU, Saha SK, Chowdhury MAKA, Ahmed S, Law PA, et al. Nosocomial sepsis risk score for preterm infants in low-resource settings. J Trop Pediatr. (2009) 56:82–9. doi: 10.1093/tropej/fmp061
40. Bekhof J, Reitsma JB, Kok JH, van Straaten IHLM. Clinical signs to identify late-onset sepsis in preterm infants. Eur J Pediatr. (2013) 172:501–8. doi: 10.1007/s00431-012-1910-6
41. Kudawla M, Dutta S, Narang A. Validation of a clinical score for the diagnosis of late onset neonatal septicemia in babies weighing 1000-2500g. J Trop Pediatr. (2008) 54:66–9. doi: 10.1093/tropej/fmm065
42. Okascharoen C, Hui C, Cairnie J, Morris AM, Kirpalani H. External validation of bedside prediction score for diagnosis of late-onset neonatal sepsis. J Perinatol. (2007) 27:496–501. doi: 10.1038/sj.jp.7211767
43. Ismail AQT, Boyle EM, Pillay T. The impact of level of neonatal care provision on outcomes for preterm babies born between 27 and 31 weeks of gestation, or with a birth weight between 1000 and 1500 g: a review of the literature. BMJ Paediatr Open. (2020) 4:e000583. doi: 10.1136/bmjpo-2019-000583
44. Shah J, Jefferies AL, Yoon EW, Lee SK, Shah PS. Risk factors and outcomes of late-onset bacterial sepsis in preterm neonates born at < 32 weeks’ gestation. Am J Perinatol. (2015) 32:675–82. doi: 10.1055/s-0034-1393936
45. Wen SCH, Ezure Y, Rolley L, Spurling G, Lau CL, Riaz S, et al. Gram-negative neonatal sepsis in low- and lower-middle-income countries and WHO empirical antibiotic recommendations: a systematic review and meta-analysis. PLoS Med. (2021) 18:e1003787. doi: 10.1371/journal.pmed.1003787
Keywords: neonate, low birth weight, bloodstream infection, sepsis, infection prediction scores
Citation: Lloyd LG, Dramowski A, Bekker A, Malou N, Ferreyra C and Van Weissenbruch MM (2022) Performance Comparison of Infection Prediction Scores in a South African Neonatal Unit: A Retrospective Case-Control Study. Front. Pediatr. 10:830510. doi: 10.3389/fped.2022.830510
Received: 07 December 2021; Accepted: 18 February 2022;
Published: 11 March 2022.
Edited by:
Suksham Jain, Government Medical College and Hospital, IndiaReviewed by:
Philipp Agyeman, University of Bern, SwitzerlandSaadet Arsan, Ankara University, Turkey
Copyright © 2022 Lloyd, Dramowski, Bekker, Malou, Ferreyra and Van Weissenbruch. This is an open-access article distributed under the terms of the Creative Commons Attribution License (CC BY). The use, distribution or reproduction in other forums is permitted, provided the original author(s) and the copyright owner(s) are credited and that the original publication in this journal is cited, in accordance with accepted academic practice. No use, distribution or reproduction is permitted which does not comply with these terms.
*Correspondence: Lizel Georgi Lloyd, bGdsQHN1bi5hYy56YQ==