- 1Qatif Central Hospital, Al-Qatif, Saudi Arabia
- 2King Fahad Medical City, Riyadh, Saudi Arabia
Objective: To assess the performance of the Pediatric Risk of Mortality III (PRISM III) and Pediatric Index of Mortality III (PIM III) indices in a tertiary pediatric intensive care unit (PICU) in Saudi Arabia and to identify the factors affecting the observed performance.
Design: Retrospective, single-center study using data collected from the Virtual Pediatric Systems web-based database.
Setting: King Fahad Medical City PICU, Saudi Arabia.
Patients: All pediatric patients <14 years of age admitted between 1 January 2015, and 31 December 2019.
Interventions: Comparison of PRISM III and PIM III performances in predicting mortality across different age groups, disease categories, and resuscitation decision statuses.
Measurements: Normality of distribution was assessed using the Kolmogorov–Smirnov and Shapiro–Wilk tests. Patient characteristics were compared between survivors and non-survivors. The medians and ranges were calculated for continuous data, whereas frequencies and percentages were used for nominal data. The Mann–Whitney U test, Kruskal–Wallis test, and Chi-square test were used to compare the characteristics of survivors and non-survivors.
Main Results: There was a significant difference between the predicted mortality and observed mortality in both the PRISM III and PIM III. Better discrimination was found after excluding do-not-resuscitate (DNR) patients. The worst calibration and discrimination were recorded for infants <12 months of age. The PRISM III performed significantly better in patients with metabolic/genetic and central nervous system illnesses. Non-DNR patients had a lower standardized mortality rate using the PRISM III and PIM III. The PRISM III and PIM III indices performed better in patients who died within the first week of admission.
Conclusion: These models had sufficient discrimination ability and poor calibration. Since they were designed for particular patient characteristics and PICUs, further testing in different environments is necessary before utilization for planning and assessing performance. Alternatively, new models could be developed which are suitable for local PICUs.
Introduction
Critically ill pediatric patients display significant variability in illness severity, disease course, and outcomes (1). This variability is usually multifactorial and can be related to the patient’s intrinsic factors, nature or stage of illness, available resources, therapeutic approach, and other factors (2). Hence, multiple pediatric intensive care unit (PICU) outcome indices were developed and used for prognostication, resource allocation, and performance comparison with other PICUs for quality improvement purposes (3–5). These tools are designed to predict an individual patient’s outcome based on a constellation of factors available at admission, including patient characteristics, severity of illness parameters, and laboratory workup (6, 7).
The Pediatric Risk of Mortality (PRISM) is a common physiologically based scoring system used to predict mortality in critically ill children. It was first developed in North American PICUs in 1988 by Pollack et al. and then updated to the Pediatric Risk of Mortality III (PRISM III) (7, 8). Additionally, the Pediatric Index of Mortality (PIM) is another widely used scoring system initially created by Shann et al. and modified to the Pediatric Index of Mortality II then III (PIM III) (6, 9). Both scoring tools are used for mortality prediction and quality control in several PICUs worldwide (6, 7). With the improvement of the delivery of advanced care and outcomes in PICUs, these scoring tools have shown variable mortality predictions over the years, and have been modified mainly to improve their prediction capacity (6, 10, 11).
Because the PRISM III and PIM III are widely used worldwide, they can be used as quality indicators to benchmark and compare outcomes and to guide PICUs in monitoring and improving their performance (4, 12–14). However, indices may overestimate or underestimate patient mortality if applied to different patient groups or populations. To the best of our knowledge, there have been no similar comparative studies in our population. This study aimed to assess the performance of the PRISM III and PIM III tools in the King Fahad Medical City (KFMC) PICU and to identify the factors that may have led to the observed performance differences.
Materials and Methods
Study Design and Settings
This retrospective study used the Virtual Pediatric Systems (VPS) web-based database (Los Angeles, CA, United States1) in which the KFMC PICU has participated in since 2014. The data were entered into the VPS by a trained and licensed medical record officer and retrieved and reviewed by the researchers (pediatric intensive care physicians). The data collected included baseline characteristics, patient origin, diagnosis at admission to the PICU, comorbidities (including hospital-acquired, device-related infections, advanced respiratory support and procedures), all variables needed to calculate the PRISM and PIM, and mortality status. Ethical approval to anonymously analyze the data was obtained from the KFMC Institutional Review Board (approval number: FWA00018774).
The PICU at the KFMC is a multidisciplinary unit in a tertiary hospital. It provides specialized care to patients with complex medical and surgical diseases, not including acute trauma or solid organ transplantation, and serves as a referral center for other hospitals in the region.
All pediatric patients <14 years of age admitted to the KFMC PICU between 1 January 2015 and 31 December 2019, were included in this study. Neonates and preterm patients <44 weeks of gestational age with neonatal diseases of age admitted to other units not included in the VPS database were excluded from our study. Children older than 14 years of age were admitted to the adult critical care unit per the Saudi ministry of health regulations and thus not included in this study.
Patients transferred to another hospital or unit and those still admitted to the PICU at the end of the study period were excluded because mortality status could not be determined. In addition, patients with incomplete data to calculate the PIM III and PRISM III indices were also excluded.
Statistical Analyses
After data collection, IBM SPSS Statistics for Windows (version 25.0; IBM Corp., Armonk, NY, United States) was used for the statistical analyses.
Kolmogorov–Smirnov and Shapiro–Wilk tests were used to assess the normality of the distribution. Patient characteristics were compared between survivors and non-survivors. The medians and ranges were calculated for continuous numerical data, whereas frequencies and percentages were used for nominal data. The Mann–Whitney U test, Kruskal–Wallis test, and Chi-square test were used to compare the characteristics of survivors and non-survivors, and a P-value < 0.05 was considered statistically significant.
The performance of the PRISM III and PIM III scores was assessed by their discriminatory power and calibration in all patients, and subgroups were categorized by age, diagnosed disease at admission, and do-not-resuscitate (DNR) status. Discriminatory power (i.e., the ability to predict survival and death at admission for each patient) was assessed by calculating the area under the receiver operating characteristic curve (AUC-ROC). Simultaneously, the standardized mortality ratio (SMR) and 95% confidence interval (CI) were analyzed. The SMR was calculated using the mid-P test. The AUC-ROCs and SMRs with corresponding 95% CIs were calculated for each age subgroup and diagnostic category.
The Hosmer–Lemeshow goodness-of-fit test was used to evaluate the calibration of the scoring system, which refers to the level of agreement between individual probabilities, including predicted and observed mortality. A value of P > 0.05 was accepted as good calibration.
Results
A total of 4,019 admissions, corresponding to 2,620 different patients, were recorded during the study period. In 50 (1.2%) admissions, the patients were transferred to another PICU and were excluded, while 573 (14.3%) admissions, of which 95.5% were survivors, were excluded for missing data needed to calculate the indices. Thus, 3,396 admissions records were analyzed. Baseline patient characteristics are summarized in Table 1. The most frequent primary cause of admission was respiratory disease (30.3%), followed by infection-related illnesses (16.2%). Approximately 14.8% of the admitted patients had previous PICU admissions. More than a quarter (34.5%) of the included patients were admitted from the emergency department, 32.5% from the inpatient ward, and 28.8% postoperatively. When comparing survivors with non-survivors, non-survivors had significantly more comorbidities, hospital-acquired infections, and device-related infections than survivors. All patients with DNR status were in the non-survivor group, representing approximately 50% of all PICU mortalities. In addition, non-survivors required more procedures and advanced respiratory support during their PICU admission, as summarized in Table 2 and Figures 1, 2.
Pediatric Risk of Mortality III and Pediatric Index of Mortality III Performances
The predicted mortality rates for the PRISM III and PIM III were 2.5 and 2.38%, respectively, for all patients included in the study. The observed mortality (6.54%, 222 deaths) was more than double that predicted by the indices, with a significant difference of P < 0.001. By further subcategorizing the patients according to the predicted risk of mortality, both the PRISM III and PIM III showed a statistically significant difference between observed and predicted mortalities in most of the deciles apart from lower-risk cases, as demonstrated in Tables 3, 4 (PRISM III χ2, 435.44 and PIM III χ2, 534.15; 5 degrees of freedom; P < 0.001). The AUC-ROC for both the PRISM III and PIM III was 0.81 (95% CI, 0.79–0.84; P < 0.001) and 0.80 (95% CI, 0.77–0.82; P < 0.001), respectively, when all patients were included. After excluding the patients with DNR status, the discrimination improved to 0.87 (95% CI, 0.84–0.90; P < 0.001) and 0.82 (95% CI, 0.79–0.86; P < 0.001), respectively as seen in Figure 3.
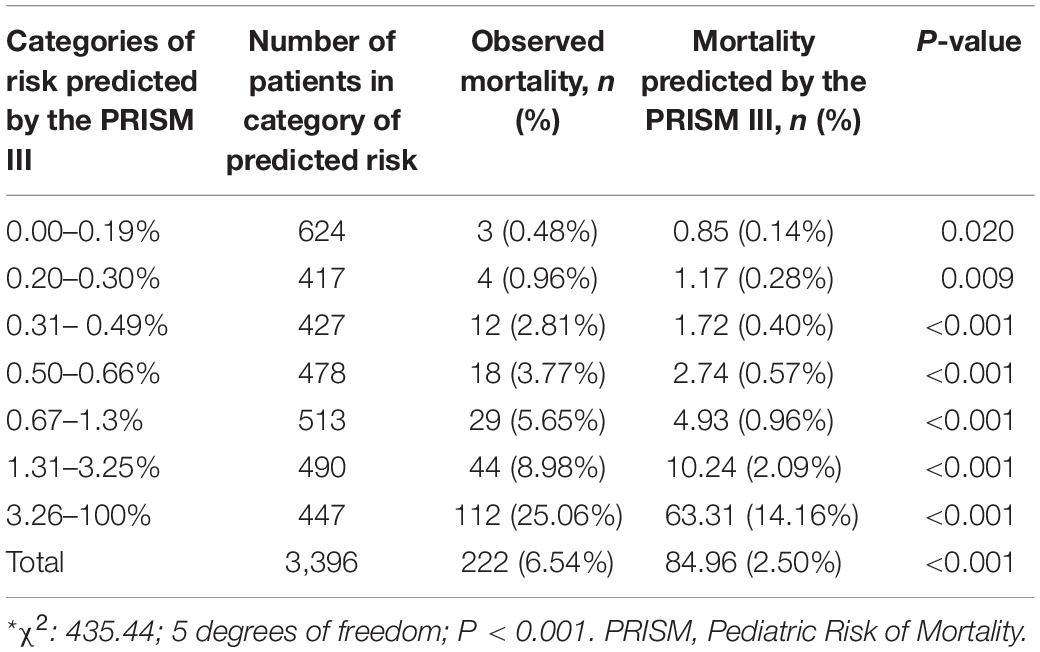
Table 3. Mortality observed and predicted per category of predicted risk for the Pediatric Risk of Mortality III*.
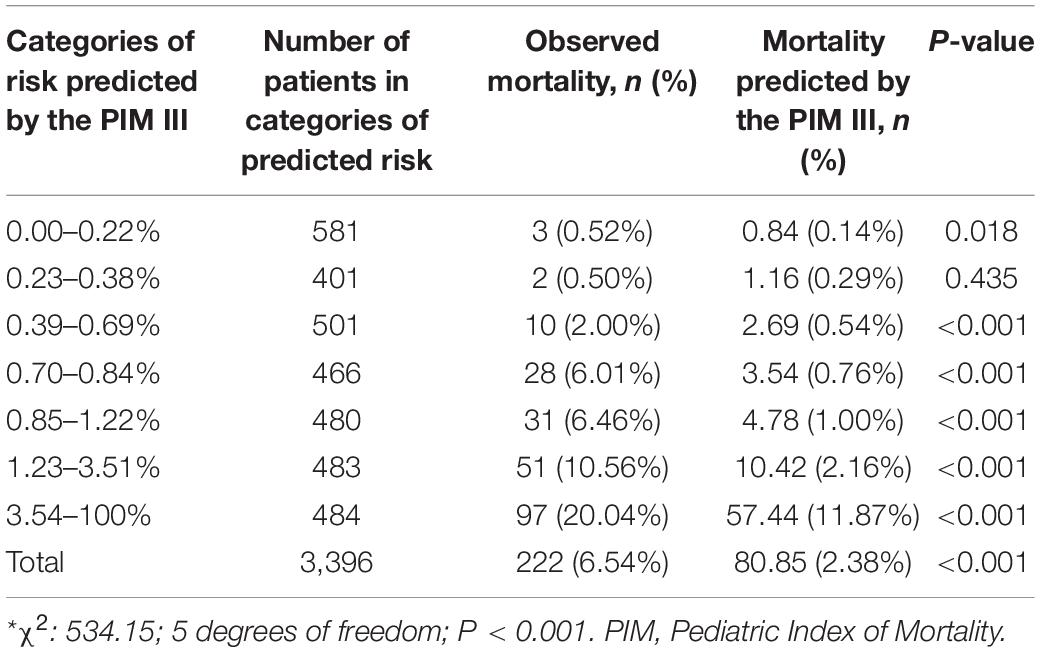
Table 4. Mortality observed and predicted per category of predicted risk for the Pediatric Index of Mortality III*.
Indices Performance by Age Subgroups
Discrimination assessments for both indices showed variable accuracies across age groups. There was a significant difference in the mortality predictions across all age groups for the PRISM III and PIM III indices, indicating poor calibration. PRISM III’s best performance was found in children between 60 and 120-months old, with an AUC-ROC of 0.87 (95% CI, 0.82–0.93; P < 0.001). On the other hand, the PIM III was better in older patients but worse than the PRISM III in most age groups (Table 5).
The worst calibration and discrimination were recorded for infants <12 months of age in which the calculated SMR for the PRISM III and PIM III was 3.96 (95% CI, 3.16–4.76) and 3.42 (95% CI, 2.73–4.12), respectively. This age group had more patients admitted with infectious (254 patients; P < 0.001) and metabolic/genetic (69; P < 0.001), and immunological (18; P < 0.001) diseases than other age groups.
Indices Performance by Disease Subgroups
The largest group (30.1%) of patients included in this study were admitted with respiratory-related illnesses, followed by those admitted with infections (16.2%) and neurological illnesses (15.8%). Mortality was the highest among patients admitted with immunological illnesses (40.9% mortality, 9/22), followed by cardiovascular (13%, 7/54) and infection-related illnesses (11.25%, 62/551). The PRISM III showed better overall discrimination in predicting mortality than the PIM III across different disease subcategories. The PRISM III performed significantly better in patients admitted with metabolic/genetic and central nervous system illnesses, with an AUC-ROC of 0.93 (95% CI, 0.87–0.99) and 0.90 (95% CI, 0.85–0.94), respectively. The PIM III performance was less discriminative across most disease subcategories. Both indices showed good agreement in patients with gastrointestinal, endocrine, and hematological illnesses.
The highest SMR for the PRISM III was recorded in patients admitted with immunological diseases (4.27; 95% CI, 1.48–7.05) and malignancies (4.74; 95% CI, 2.71–6.77), while for the PIM III, the highest was recorded in patients admitted with infectious diseases (4.01; 95% CI, 3.01–5.01). A detailed analysis of both indices is provided in Table 6. Patients with immunological and infectious diseases were found to have more comorbidities (median: 3; P < 0.001), while the lowest albumin (median: 2.31 g/dL, range: 1.5–3.6; P < 0.001) and hemoglobin levels (median: 7.90 g/dL, range: 6–11.1; P < 0.001) were recorded in patients admitted with immunological diseases.
Non-survivors’ Analysis and Do-Not-Resuscitate Status
Non-survivors were further subcategorized according to DNR status and length of stay, as summarized in Table 7. Of the non-survivors, 50% signed DNR after their admission to the PICU. Almost half of the non-DNR mortalities occurred after 1 week of admission, while the majority (73%) of DNR mortalities occurred after 7 days. Patients who died within the first week of admission had significantly higher PRISM III (median, 11.9; range, 0.1–99.5; P = 0.01) and PIM III (median: 4.6, range: 0.4–93; P = 0.019) scores. The PRISM III and PIM III performed better in patients who died within the first week of admission (AUC-ROC of 0.90 and 0.86, respectively) than in patients who died after 7 days of admission (AUC-ROC of 0.63 and 0.64, respectively). Patients who died 1 week after admission had significantly more hospital-acquired infections. DNR patients who died after 1 week had more respiratory and neurological-related illnesses (40.7 and 22.2%, respectively; P = 0.005) than those in the other groups. There were no significant differences in the demographic data between the groups.
Discussion
This study was conducted to validate the performance of the PRISM III and PIM III indices in patients admitted to a tertiary health care facility in Riyadh, Saudi Arabia. The PRISM III generally showed better discrimination between survivors and non-survivors than the PIM III across all age groups. The lowest performance of both indices was observed in those ≤12 months of age. The PRISMS III performed less well in patients with respiratory diseases, whereas the PIM III showed less discrimination in patients with cardiovascular, immunological, and metabolic/genetic diseases. In contrast, PRISM III had the worst calibration in patients with immunological and malignancy-related diseases, whereas PIM III’s worst calibration was demonstrated in patients with infectious diseases. Similar observations were reported by Malhotra et al. in their study of comparable population groups (15). A number of studies conducted in different countries, including India and Austria, compared the performance of different indices and showed variable results between the indices in the populations studied (16–18). In 2021, Shen and Jiang reported a good overall but variable performance for mortality prediction in the PICU across studies included in their meta-analysis (19). The variations in calibration and discrimination between the PRISM III and PIM III across disease groups could be due to the difference in the parameters used to calculate each score.
The performance of the PRISM III in this study was weaker compared with that in another study performed in a tertiary medical center in Saudi Arabia, by Albuali et al. (20). Their study showed a PRISM III AUC-ROC of 0.955 (95% CI, 0.924–0.986) and a significantly higher PRISM III score in non-survivors. In their study, the sample size was much smaller and the proportions of metabolic, genetic, and immune-deficient patients were not mentioned, making real comparison inaccurate. To the best of our knowledge, no other studies have assessed the performance of the PRISM III or PIM III for similar populations and medical infrastructures. Other studies on PIM III performance conducted in different geographical areas and populations have shown results comparable to ours. One study conducted in another Middle Eastern country showed an AUC-ROC of 0.78 (95% CI, 0.69–0.87), while a multicenter study by Arias López et al. showed an AUC-ROC of 0.84 (95% CI, 0.82–0.87) and 0.82 (95% CI, 0.80–0.85) in smaller and larger units, respectively (12, 15). These variable performances are expected when the model is developed using a different population, disease categories, and healthcare systems, including differences in PICU advancement and quality of care provided.
The varying accuracies of predictive models can result from different patient characteristics; the PIM III was initially developed on data gathered from the United Kingdom, Ireland, Australia, and New Zealand Pediatric Intensive Care registries. Of the patients included in their study, 39.7% were admitted for postprocedural recovery compared with 28.8% in our study (6). Moreover, the proportion of the variety of diseases between our study and the original study can influence the accuracy of such models. In particular, metabolic/genetic and immunological diseases can vary widely in type and prevalence between countries, especially the particularities of the Arabian population (21). In the study by Straney et al., 5% of patients were admitted with metabolic or genetic diseases (6). In comparison, 22.6% of our study population had metabolic/genetic comorbidities as a reason for PICU admission. Both models showed different calibration and predictive abilities in the analysis performed on subgroups stratified by disease at admission.
In both models, predicted mortality was lower than observed mortality, as reflected by an SMR of 2.61 and 2.75 for the PRISM III and PIM III predictions, respectively. However, after excluding patients with DNR status from the analysis, the calculated SMR improved to 1.52 and 1.64, respectively. The difference demonstrated in the SMR before and after excluding DNR patients from the calculation is probably related to the difference in the practice of life-sustaining treatments and timing and rationale for withholding specific aggressive support modalities and labeling patients as DNR among different intensivists and institutions. Few studies have examined and demonstrated, without doubt, the impact of culture, religion, and sometimes lack of knowledge on delaying DNR decisions and active withdrawal of support in our community (22–24).
The practice of DNR can differ significantly from one center and culture to another. Determining the DNR status early in the course of an extremely critical patient with a high probability of death is completely different compared with deciding on life support based on quality of life and expected comorbidities after the acute life-threatening presentation is over. Our DNR patients had a significantly longer stay in the PICU, possibly secondary to the slow weaning of support and lack of active withdrawal of life-sustaining management practices in our PICU. It was interesting that 81 of the 222 total mortalities were for cases labeled as DNR and expired inside the PICU after the first week of admission. We are extremely skeptical about the capability of the two-scoring system to predict mortality accurately in this large group.
A sub-analysis of non-survivor patients showed significantly higher PRISM III and PIM III probabilities of mortality in patients who died within the first week of admission. Similar findings were demonstrated by Jacob et al., who demonstrated that these scores performed better at predicting early rather than late mortality (14). DNR patients who died after 1 week had more respiratory and neurological illnesses.
Generally, indices that predict the risk of mortality differ based on patients’ characteristics, medical resources where they were developed, and other factors. The difference in the performance of the PIM III and PRISM III indices in this tertiary PICU compared with that of other health care facilities can be, as demonstrated before, denoted by multiple factors, including the difference in population, disease group, and DNR practice in this institute. DNR patients in this study population had a prolonged length of stay, making the PIM III and PRISM III mortality predictions less accurate. These models should be validated locally before they are implemented as tools for predicting outcomes and measuring performance.
Our study had limitations; it was conducted retrospectively in a single tertiary care center dealing with high-risk patients with multiple comorbidities and fewer trauma patients. In addition, a large number of cases were excluded because of missing data. Additionally, including different ICUs with patients with different disease characteristics may demonstrate different performances for the models tested in this study.
Conclusion
Both models showed adequate discrimination ability, but poor calibration. We suggest calibrating these models before they are used as quality measures. These models were designed to fit specific patient characteristics and PICUs. Testing these models further in different regional institutions or developing new models to better suit patients admitted to local PICUs is warranted before utilizing them for planning and assessing performance.
Data Availability Statement
The raw data supporting the conclusions of this article will be made available by the authors, without undue reservation.
Author Contributions
ASA organized the database, performed the statistical analysis, and wrote the first draft of the manuscript. All authors contributed to the conception and design of the study, wrote sections of the manuscript, and contributed to manuscript revision, read, and approved the submitted version.
Author Disclaimer
VPS data was provided by Virtual Pediatric Systems, LLC. No endorsement or editorial restriction of the interpretation of these data or opinions of the authors has been implied or stated.
Conflict of Interest
The authors declare that the research was conducted in the absence of any commercial or financial relationships that could be construed as a potential conflict of interest.
Publisher’s Note
All claims expressed in this article are solely those of the authors and do not necessarily represent those of their affiliated organizations, or those of the publisher, the editors and the reviewers. Any product that may be evaluated in this article, or claim that may be made by its manufacturer, is not guaranteed or endorsed by the publisher.
Acknowledgments
We thank Ahmad M. Saleh, the chairperson of the Biostatistics Department, and Mohammed Abu Gazal, a VPS qualified staff nurse, King Fahad Medical City, for their continuous support. We also thank the research center at KFMC and all PICU team for their help in accomplishing this work.
Abbreviations
AUC-ROC, area under the receiver operating characteristic curve; CI, confidence interval; DNR, do-not-resuscitate; KFMC, King Fahad Medical City; PIM III, Pediatric Index of Mortality III; PICU, pediatric intensive care unit; PRISM III, Pediatric Risk of Mortality III; SMR, standardized mortality ratio.
Footnotes
References
1. Rosenberg DI, Moss MM American Academy of Pediatrics Section on Critical Care, American Academy of Pediatrics Committee on Hospital Care. Guidelines and levels of care for pediatric intensive care units. Pediatrics. (2004) 114:1114–25. doi: 10.1542/peds.2004-1599
2. Thukral A, Lodha R, Irshad M, Arora NK. Performance of pediatric risk of mortality (PRISM), pediatric index of mortality (PIM), and PIM2 in a pediatric intensive care unit in a developing country. Pediatr Crit Care Med. (2006) 7:356–61. doi: 10.1097/01.PCC.0000227105.20897.89
3. Brady AR, Harrison D, Black S, Jones S, Rowan K, Pearson G, et al. Assessment and optimization of mortality prediction tools for admissions to pediatric intensive care in the United Kingdom. Pediatrics. (2006) 117:e733–42. doi: 10.1542/peds.2005-1853
4. Abdelatif RG, Mohammed MM, Mahmoud RA, Bakheet MAM, Gima M, Nakagawa S. Characterization and outcome of two pediatric intensive care units with different resources. Crit Care Res Pract. (2020) 2020:5171790. doi: 10.1155/2020/5171790
5. Slater A, Shann F ANZICS Paediatric Study Group. The suitability of the pediatric index of mortality (PIM), PIM2, the pediatric risk of mortality (PRISM), and PRISM III for monitoring the quality of pediatric intensive care in Australia and New Zealand. Pediatr Crit Care Med. (2004) 5:447–54. doi: 10.1097/01.PCC.0000138557.31831.65
6. Straney L, Clements A, Parslow RC, Pearson G, Shann F, Alexander J, et al. Paediatric index of mortality 3: an updated model for predicting mortality in pediatric intensive care. Pediatr Crit Care Med. (2013) 14:673–81. doi: 10.1097/PCC.0b013e31829760cf
7. Pollack MM, Patel KM, Ruttimann UE. PRISM III: an updated pediatric risk of mortality score. Crit Care Med. (1996) 24:743–52. doi: 10.1097/00003246-199605000-00004
8. Pollack MM, Ruttimann UE, Getson PR. Pediatric risk of mortality (PRISM) score. Crit Care Med. (1988) 16:1110–6. doi: 10.1097/00003246-198811000-00006
9. Shann F, Pearson G, Slater A, Wilkinson K. Paediatric index of mortality (PIM): a mortality prediction model for children in intensive care. Intensive Care Med. (1997) 23:201–7. doi: 10.1007/s001340050317
10. Pollack MM, Holubkov R, Funai T, Dean JM, Berger JT, Wessel DL, et al. The pediatric risk of mortality score: update 2015. Pediatr Crit Care Med. (2016) 17:2–9. doi: 10.1097/PCC.0000000000000558
11. Jung JH, Sol IS, Kim MJ, Kim YH, Kim KW, Sohn MH. Validation of pediatric index of mortality 3 for predicting mortality among patients admitted to a pediatric intensive care unit. Acute Crit Care. (2018) 33:170–7. doi: 10.4266/acc.2018.00150
12. Arias López MDP, Boada N, Fernández A, Fernández AL, Ratto ME, Siaba Serrate A, et al. Performance of the pediatric index of mortality 3 score in PICUs in Argentina: a prospective, national multicenter study. Pediatr Crit Care Med. (2018) 19:e653–61. doi: 10.1097/PCC.0000000000001741
13. Russell RA, Rettiganti M, Brundage N, Jeffries HE, Gupta P. Performance of pediatric risk of mortality score among critically ill children with heart disease. World J Pediatr Congenit Heart Surg. (2017) 8:427–34. doi: 10.1177/2150135117704656
14. Jacobs A, Flechet M, Vanhorebeek I, Verstraete S, Ingels C, Casaer MP, et al. Performance of pediatric mortality prediction scores for PICU mortality and 90-day mortality. Pediatr Crit Care Med. (2019) 20:113–9. doi: 10.1097/PCC.0000000000001764
15. Malhotra D, Nour N, El Halik M, Zidan M. Performance and analysis of pediatric index of mortality 3 score in a pediatric ICU in Latifa Hospital, Dubai, UAE. Dubai Med J. (2019) 3:19–25. doi: 10.1159/000505205
16. Tyagi P, Tullu MS, Agrawal M. Comparison of pediatric risk of mortality III, pediatric index of mortality 2, and pediatric index of mortality 3 in predicting mortality in a pediatric intensive care unit. J Pediatr Intensive Care. (2018) 7:201–6. doi: 10.1055/s-0038-1673671
17. Niederwanger C, Varga T, Hell T, Stuerzel D, Prem J, Gassner M, et al. Comparison of pediatric scoring systems for mortality in septic patients and the impact of missing information on their predictive power: a retrospective analysis. Peer J. (2020) 8:e9993. doi: 10.7717/peerj.9993
18. Solomon LJ, Naidoo KD, Appel I, Doedens LG, Green RJ, Long MA, et al. Pediatric index of mortality 3-an evaluation of function among ICUs in South Africa. Pediatr Crit Care Med. (2021) 22:813–21. doi: 10.1097/PCC.0000000000002693
19. Shen Y, Jiang J. Meta-analysis for the prediction of mortality rates in a pediatric intensive care unit using different scores: PRISM-III/IV, PIM-3, and PELOD-2. Front Pediatr. (2021) 9:712276. doi: 10.3389/fped.2021.712276
20. Albuali WH, Algamdi AA, Hasan EA, Al-Qahtani MH, Yousef AA, Al Ghamdi MA, et al. Use of a mortality prediction model in children on mechanical ventilation: a 5-year experience in a tertiary university hospital. J Multidiscip Healthc. (2020) 13:1507–16. doi: 10.2147/JMDH.S282108
21. Al-Gazali L, Hamamy H, Al-Arrayad S. Genetic disorders in the Arab world. BMJ. (2006) 333:831–4. doi: 10.1136/bmj.38982.704931.AE
22. Gouda A, Alrasheed N, Ali A, Allaf A, Almudaiheem N, Ali Y, et al. Knowledge and attitude of ER and intensive care unit physicians toward do-not-resuscitate in a tertiary care center in Saudi Arabia: a survey study. Indian J Crit Care Med. (2018) 22:214–22. doi: 10.4103/ijccm.IJCCM_523_17
23. Hafez SA, Snethen JA, Ngui E, Ellis J, Taani M. Pediatric end of life care: impact of Islamic faith. West J Nurs Res. (2022) 44:773–9. doi: 10.1177/01939459211016503
Keywords: Pediatric Risk of Mortality score, Pediatric Index of Mortality, mortality, pediatric intensive care unit, discrimination, calibration
Citation: Alkhalifah AS, AlSoqati A and Zahraa J (2022) Performance of Pediatric Risk of Mortality III and Pediatric Index of Mortality III Scores in Tertiary Pediatric Intensive Unit in Saudi Arabia. Front. Pediatr. 10:926686. doi: 10.3389/fped.2022.926686
Received: 22 April 2022; Accepted: 20 June 2022;
Published: 07 July 2022.
Edited by:
Kenneth E. Remy, Case Western Reserve University, United StatesReviewed by:
Deepika Gandhi, SRM University, IndiaPhuc Huu Phan, Vietnam National Children’s Hospital, Vietnam
Copyright © 2022 Alkhalifah, AlSoqati and Zahraa. This is an open-access article distributed under the terms of the Creative Commons Attribution License (CC BY). The use, distribution or reproduction in other forums is permitted, provided the original author(s) and the copyright owner(s) are credited and that the original publication in this journal is cited, in accordance with accepted academic practice. No use, distribution or reproduction is permitted which does not comply with these terms.
*Correspondence: Ahmed S. Alkhalifah, QWhtZWRzazJAZ21haWwuY29t