- Department of Human Genetics, Hannover Medical School, Hannover, Germany
Introduction: Rare genetic diseases are a major cause for severe illness in children. Whole exome sequencing (WES) is a powerful tool for identifying genetic causes of rare diseases. For a better and faster assessment of the vast number of variants that are identified in the index patient in WES, parental sequencing can be applied (“trio WES”).
Methods: We assessed the diagnostic rate of routine trio WES including analysis of copy number variants in 224 pediatric patients during an evaluation period of three years.
Results: Trio WES provided a diagnosis in 67 (30%) of all 224 analysed children. The turnaround time of trio WES analysis has been reduced significantly from 41 days in 2019 to 23 days in 2021. Copy number variants could be identified to be causative in 10 cases (4.5%), underlying the importance of copy number variant analysis. Variants in three genes which were previously not associated with a clinical condition (GAD1, TMEM222 and ZNFX1) were identified using the matching tool GeneMatcher and were part of the first description of a new syndrome.
Discussion: Trio WES has proven to have a high diagnostic yield and to shorten the process of identifying the correct diagnosis in paediatric patients. Re-evaluation of all 224 trio WES 1–3 years after initial analysis did not establish new diagnoses. Initiating (trio) WES as a first-tier diagnostics including copy number variant detection should be considered as early as possible, especially for children treated in ICU, if a monogenetic disease is suspected.
1. Introduction
Rare genetic disorders often manifest as severe multisystemic disorders and represent a significant cause of pediatric hospitalisation (1–3). Studies show that 21%–57% of children admitted to an intensive care unit (ICU) suffer from a genetic disorder (4–6). In a recent study, monogenetic diseases were identified as causative in 41% of infant deaths. Treatment options, that have the potential to improve the clinical outcome, would have been available for 30% of these diseases (7). Consequently, several studies have shown that children with suspected monogenetic disorders benefit from a fast and precise diagnosis to ensure rapid and efficient personalized treatment (8, 9). Recently, next generation sequencing (NGS) technologies such as whole exome sequencing (WES) or whole genome sequencing (WGS) have become more accessible in terms of cost and turnaround time (TAT) and are increasingly used to identify the underlying genetic causes of rare diseases. Simultaneous analysis of the patient and their parents [trio WES (tWES)] has the potential to speed up the process of identifying the correct genetic diagnosis even more (10). In particular, severely ill infants who are admitted to neonatal or paediatric intensive care units (NICU/PICU) benefit from access to rapid, extensive genetic diagnostics leading to precision treatments (9). This in turn leads not only to reduced costs of care but also shortens the often very long time (“diagnostic odyssey”) from the clinical description of an unidentified rare disease to a definitive diagnosis (11). The large majority of parents and clinicians agreed that genomic results were beneficial and had a very high perceived utility even when testing did not yield a diagnosis (12, 13).
However, in many countries, the implementation of broad genetic testing has not been established in routine diagnostics yet and coverage by health insurances still remains a challenge for WES or WGS and especially for trio analysis (6, 14–16).
The aim of this study is to demonstrate how a holistic, multimodal approach that includes patient´s accurate clinical information, the use of parental information, a comprehensive analytic and clinical variant assessment, and the use of GeneMatcher (17) can maximize the chance of establishing a genetic diagnosis in routine diagnostics.
2. Methods and materials
2.1. Patient cohort
The study was conducted in accordance with the general principles outlined in the Declaration of Helsinki (18) and approved by the local Institutional Review Board of Hanover Medical School (ID 8657_BO_K_2019). The analyzed cohort included 224 index patients and their unaffected parents of different ethnicity with a suspected genetic condition who were referred to our laboratory for genetic testing between the years 2019 and 2021.
2.2. Sample preparation for whole exome sequencing
DNA was extracted from blood using NucleoMag Blood kit (Macherey-Nagel, Germany). Exome sequencing was performed using the IDT Exome (xGen Exome V02, IDT, Leuven, Belgium). The captured DNA was sequenced using Illumina NextSeq 500, Illumina NextSeq550, NovaSeq 6000 or MGI DNB SEQ G400RS Sequencer to generate 150-bp paired-end reads. Reads were aligned to the human reference genome (UCSC Genome Browser build GRCh37/hg19). Sequence data were processed using the megSAP analysis pipeline (https://github.com/imgag/megSAP), regarding quality control, read alignment and variant annotation/detection. To verify the DNA sample, 14 single nucleotide polymorphisms (SNP) were amplified by competitive allele-specific PCR using fluorescence-labeled primers and analysed by StepOnePlus software for genotyping experiments (StepOnePlus System, Thermo Fischer Scientific, Inc., Waltham, MA, USA). SNP results were compared with the data from the NGS analysis (megSAP). Reads were aligned to the human reference genome (UCSC Genome Browser build GRCh37).
2.3. Sequence variant filter strategy
Sequence data were analyzed using GSvar (https://github.com/imgag/ngs-bits) and IGV (http://software.broadinstitute.org/software/igv/). All quality metrics were examined prior to analyzation of data. Default cut-offs were used for target region read depth (20x coverage), target region 20x percentage (above 95%), known variants percentage (above 98%) and SNV allele frequency deviation (indicative of DNA contamination, must be below 3%). The aforementioned QC thresholds were established using aggregated sample QC data (derived from >150 exome datasets). Variant filtering was applied to identify candidate disease-causing single nucleotide variants (SNVs) in the index patient using the following parameters: rare variants with allele frequency <1% in the overall population of the 1,000 Genomes Project and the genome Aggregation Database (gnomAD); variants that occur in our internal database less than 20 times; variants annotated as pathogenic (p) or likely pathogenic (lp) in ClinVar or the Human Gene Mutation Database (HGMD); homozygous or compound heterozygous variants, de novo variants or inherited X-chromosomally variants in male patients. Variants that were localised in the intron region beyond ±1–20 bases from the intron/exon border as well as variants with low mapping quality were excluded. Intronic variants at ±>20 bases from the intron/exon border were evaluated for pathogenicity if they were classified in ClinVar as p/lp.
2.4. Clinical interpretation and confirmation of variants
Variants which passed the filtering criteria, were evaluated using the ACMG/AMP (American College of Medical Genetics and Genomics/Association for Molecular Pathology) guidelines on variant interpretation and classification (19, 20) using the following terms: class 5 (pathogenic, p), class 4 (likely pathogenic, lp), class 3 (variants of unknown significance, VUS), class 2 (likely benign) and class 1 (benign). Variant nomenclature is based on standardized Human Genome Variation Society (HGVS) conventions as referenced in den Dunnen et al. (21). In addition to variant allele frequency data, we used prediction tools, including phyloP, SIFT, PolyPhen2, FATHMM, REVEL and CADD, and searched databases, including LOVD, ClinVar, and gnomAD. Furthermore, for each gene carrying a rare de novo variant or rare biallelic variants, we performed an extensive literature search and, if necessary, contacted the authors of publications. Additionally, all potentially p/lp variants in genes that are not associated with a specific phenotype and met the filter criteria, were submitted to GeneMatcher (17).
2.5. Secondary findings
The analysis of clinically actionable genetic variants that are unrelated to the primary indication for testing (secondary findings) was offered to each individual tested as part of trio-based analysis our in-house recommendations for the return of secondary findings (22). We performed a targeted search for (p/lp) variants in patients who consented to the receipt of secondary findings: In children, we reported p/lp variants in APC, APOB, LDLR, MEN1, MLH1*, MSH2*, MSH6*, PCSK9, PMS2*, RET, TP53 and VHL. In adults, we reported p/lp variants in APC, APOB, BRCA1, BRCA2, CDH1, LDLR, MEN1, MLH1, MSH2, MSH6, MUTYH*, PALB2, PCSK9, PMS2*, RET, TP53 and VHL [* = only homozygous or compound heterozygous p/lp variants (potentially biallelic)].
2.6. Copy number variants
Copy number variant (CNV) calling was performed using ClinCNV (23) based on the depth of coverage, i.e., the number of reads, in similar probes. In WES, exon target regions alternate with non-target intron regions. For each region, the expected depth of coverage is compared with the observed depth of coverage and a log-likelihood of a copy-number alteration is given for each region. The following sample-specific information of the CNV caller were used to assess variants: genomic position, size (kb), number of regions (exons), affected genes, copy-number change, log-likelihood (logarithm of the ratio between likelihoods of the no CN change model vs. the CN equal to the reported state model), potential allele frequency (AF, frequency of the copy-number change in the analysed cohort, i.e., the 100–200 most similar samples), q-value (p-value corrected for the number of CNVs detected). Rare copy number variants affecting OMIM genes were followed up similarly as SNV and small insertions and deletions (indels) (19) described above.
2.7. Submission to GeneMatcher
For non-synonymous, frameshift and nonsense variants or variants that affect donor/acceptor splice sites in genes of unknown significance (GUS) which were in homozygous or compound heterozygous state or represented as a de novo germline event in the patient, an intensive literature search was carried out. If an association between the GUS and the patient's disease was suspected, potential p/lp variants in GUS were shared through the international collaborative platform GeneMatcher to identify additional patients and to improve genotype–phenotype correlations (24). If a match was obtained, stakeholders were contacted by mail and the phenotypes were compared.
2.8. Re-evaluation of tWES data
Re-evaluation analysis of all tWES was carried out in November 2022 after a period of at least 1 year (range 1–3 years) after the last diagnostic test using GSVar and VarSeq® 2.2.4 software (Golden Helix). For this effort, annotations were renewed, variant filtration was repeated and databases LOVD, ClinVar, and gnomAD were re-searched. If new clinical data of the index patient was available, prioritised variants underwent further clinical-genetic assessment.
3. Results
3.1. Diagnostic testing results
224 children and adolescents (134 males and 90 females), ranging in age from 2 days to 18 years with a mean age of 3.7 years, suspected of having a monogenetic disease were tested in parallel with their parents (tWES) in a diagnostic setting over a period of 3 years (2019–2021). Disease phenotypes were highly diverse and categorized in 12 groups according to the clinical data provided by the referring physician (Table 1). Most patients had a suspected genetic immunodeficiency (n = 68; 30%), followed by patients with a complex disorder belonging to the spectrum of syndromic (n = 55; 25%) and neurologic disease (n = 42; 19%). Overall, a molecular diagnosis was provided for 67 patients [causative p/lp variant(s), 30%]. In 32 patients (14%), identification of a VUS resulted in an inconclusive report. In 125 patients (56%), no variants that were (obviously) related to the disease phenotype could be identified. The highest diagnostic rate was achieved in patients affected by syndromic disorders (n = 23/55, 42%) (Table 1). No relevant difference was observed when comparing the age of patients with or without molecular diagnosis (data not shown).
We also analyzed the diagnostic yield in different sub-cohorts (NICU/PICU, inpatient, outpatient): tWES identified causative variants indicating a monogenetic disorder in 36% (n = 18) of patients hospitalized on a NICU or PICU (n = 50), while 64% of these patients carried VUS (inconclusive, n = 7) or had a negative result (no diagnosis, n = 25). In comparison, we achieved a diagnostic yield of 21% (n = 10) in non-critically ill inpatients and 27% (n = 39) in outpatients, respectively. 79% of inpatients and 73% of outpatient children had an inconclusive or negative result (Figure 1).
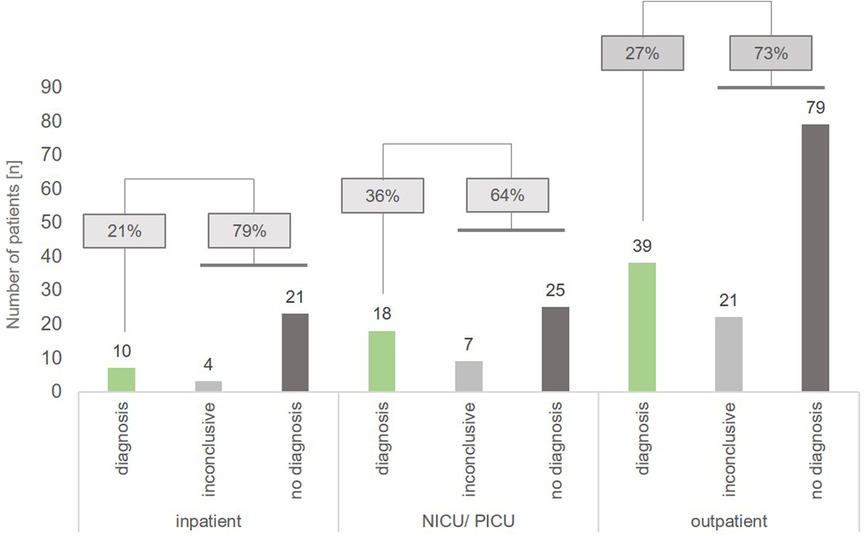
Figure 1. Diagnostic yield in the different sub-cohorts inpatient, NICU/PICU and outpatient. For inpatients in normal care units, a genetic diagnosis (class 4 and 5 variants) was achieved in 21% of patients (n = 10), while ICU patients had a diagnosic rate of 36% (n = 18). In outpatients, a genetic diagnosis was obtained in 27% of cases (n = 39).
The overall mean time to report the results of the molecular analysis was 30 days but the average duration of evaluation was reduced from 41 days in 2019 (FAM01-FAM14), and 34 days in 2020 (FAM15-FAM126) to 23 days in 2021 (FAM127-FAM224), reflecting a TAT reduction of almost 44% (Figure 2). This is mainly explained by expertise acquired over time in the analysis of the data and a better understanding of the clinical interpretation of the results. Furthermore, due to an increasing demand of diagnostic exome sequencing and thus a higher sample volume per time, the TAT could be reduced because the required minimum batch size for economic sequencing runs could be utilized faster. The overall median time to report the results of the molecular analysis especially in children treated at ICU was 39 days in 2019, 33 days in 2020 and 19 days in 2021. This even corresponds to a reduction of more than 52%. No relevant difference in TAT was obtained when comparing reports with and without genetic diagnosis or with in inconclusive result (data not shown).
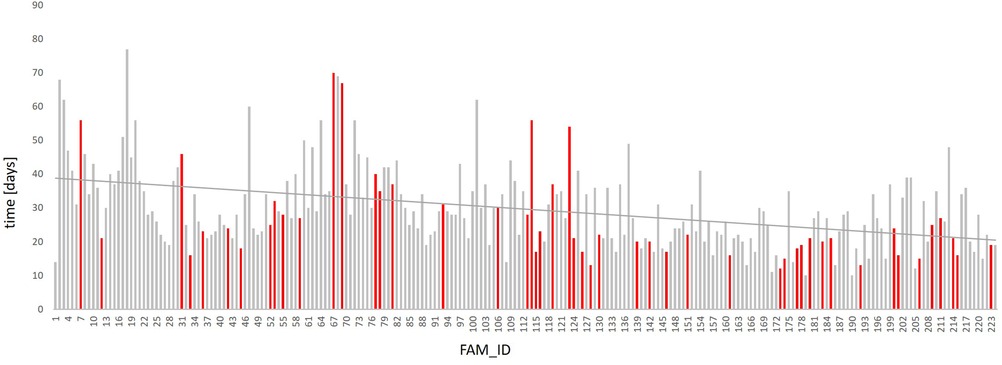
Figure 2. Time required for genetic analysis. The reported time required for DNA preparation, NGS and preparation of scientific report of all 224 analyzed patients is given. The reporting time for the 224 trio analyses have been continuously dropping since 2019. While the turnover time still averaged at 41 days in 2019 (FAM1-FAM14), it was reduced to 34 days in 2020 (FAM15-FAM126) and to 23 days in 2021 (FAM127-FAM224). The family IDs of the individual patients are listed (FAM_ID) (X-axis). Each bar represents one patient; red bars refer to patients admitted to NICU/PICU; the time required for genetic analysis is given in days (Y-axis, time). The linear trend line is shown in red.
3.2. Genetic spectrum of diagnostic variants
We identified causative variants in 54 genes as classified according to ACMG guidelines. Table 2 shows the diagnoses per disease group. Three molecular diagnoses were made by submitting novel candidate genes with highly suspicious variants to GeneMatcher (17). Matching to multiple other cases reported in GeneMatcher enabled establishment of novel causative gene–disease associations, namely TMEM222 (FAM_60), ZNFX1 (FAM_75) and GAD1 (FAM_116). Biallelic p/lp variants in TMEM222 cause an autosomal recessive neurodevelopmental disorder (25), biallelic p/lp variants in ZNFX1 lead to multisystem inflammation and susceptibility to viral infections (26) and biallelic p/lp variants in GAD1 are causal for an early-infantile onset epilepsy and developmental delay (27, 28). GeneMatcher matches were obtained for five additional genes; these genes are currently subject of further investigation (data not shown). Variants in other five genes were initially reported as VUS but were reclassified as p/lp variants following segregation analysis of further family members [FTDC (FAM149); WWOX, (FAM31); SLC52A2 (FAM126)], allowing to apply the ACMG criteria PP1 (cosegregation with disease in multiple affected family members in a gene definitively known to cause the disease) or PS3 (well established in vitro or in vivo functional studies supportive of a damaging effect on the gene or gene product). For two genes, functional studies that were published in the meantime allowed the use of PS3 [XIAP (FAM44) (29); STAT3 (FAM72)] (30), leading to a reclassification. Detailed information about identified variants are given in Supplementary Tables S1, S2. Ten patients (15% of all diagnosed patients) with a monogenetic diagnosis [immunodeficiency (n = 2), hepatopathy (n = 1), neurology (n = 1) and syndromic disorder (n = 6)] had large CNVs that were detected via WES (Table 3).
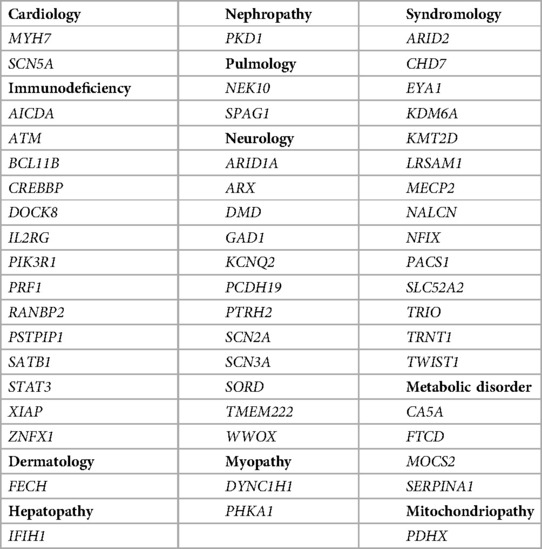
Table 2. Genes, in which pathogenic and likely pathogenic variants were identified by tWES in our cohort, categorized in 12 disease groups.
Among the 67 patients with confirmed molecular diagnosis, the mode of inheritance of the identified hereditary diseases was autosomal dominant in 52% (n = 35), autosomal recessive in 34% (n = 23) and X-linked in 14% (n = 9). De novo variants accounted for 46% (n = 31) of all p/lp variants, whereas inherited variants accounted for 54% (n = 36). 6 out of 9 X-linked variants were inherited from the unaffected mother (Supplementary Tables S1, S2).
3.3. Re-evaluation of exome data
To test the validity and usability of trio analyses, we performed new variant annotation and re-evaluated all 224 tWES data at least 1 year after the last reporting. In none of the trio analyses, a new diagnosis was established by re-evaluation. Also, no additional variants could be identified, that were suitable for a GeneMatcher upload.
3.4. Secondary findings
In three parents of the analyzed 224 paediatric patients (1.3% of all tested families), we identified a p/lp variant which was not associated with the medical indication for testing and were disclosed to the families as secondary findings. This involves variants in the genes BRCA1 (n = 1; FAM215) and BRCA2 (n = 2; FAM91; FAM209), which are associated with an increased risk of hereditary breast and ovarian cancer (HBOC).
4. Conclusion
Positive as well as negative genetic test results in patients with suspected rare genetic diseases can lead to adjustment of treatment and facilitate accurate and evidence-based decisions in affected families (9). In this diagnostic setting, analysing 224 paediatric patients with suspected rare mendelian disorders, we provided a diagnosis in 67 (30%) of all analysed children, and in 18 (36%) children treated in ICUs using tWES. P/lp variants could be identified in 54 different genes, including three genes that were previously not associated with a clinical condition. 10 children (15%) with a genetic diagnosis had large copy number variations that were detected via tWES. To the best of our knowledge, this is the largest study published to date on paediatric patients with a broad spectrum of suspected genetic disorders analysed by tWES.
The American College of Medical Genomics (ACMG) recommends re-evaluation of sequencing data every 2 years (31). Some studies report that periodic data re-evaluation can be a valuable approach to identify additional variants and increases the diagnostic yield up to 10%–12% (32–34). Interestingly, in none of our trio analyses a new diagnosis was established by re-evaluation the data at least 1 year after the last reporting. This underlines the particular effectiveness and reliability of a trio-analysis at the time of diagnostic reporting. The combination of a thorough analysis of the sequencing data (and CNV data), including literature search of candidate genes and the use of GeneMatcher seems to be very effective. In this study, a diagnosis was established in three cases using GeneMatcher (25, 26, 28) and another five cases are currently subject of further analysis after identification of further patients via GeneMatcher (data not shown). This corresponds to a rate of 1.9% (3/157) of definitely and an additional 3.2% (5/157) of potentially solved cases using GeneMatcher in all analysed 157 tWES families with an initially negative or inconclusive diagnostic report.
A few studies are available that evaluate the effectiveness of different NGS methods in pediatric patients. Some studies describe the diagnostic impact of WES in children with neurodevelopmental disorder (35). All of these studies differ slightly in patient's population, size and diagnostic yield. Since 2015, reports have also been published about the diagnostic value of WGS and the comparison to WES in different disease groups (14, 36–40). A few published data are available describing the diagnostic yield of tWES or tWGS (41–46), but to the best of our knowledge there is only one detailed description of such a large cohort analysed via tWES in a diagnostic setting (47).
In our cohort, p/lp de novo variants of 15 patients would have been classified as VUS in singleton WES analysis according to the current ACMG guidelines. This corresponds to a rate of 22% (15/67) of all diagnosed patients. Particularly, in patients with early-onset and severe complex phenotypes, a fast genetic diagnosis could be essential for clinical decisions, which is facilitated by parental genetic information. Five out of 15 infants with de novo variants were critically ill and treated at NICU/PICU at the time of the genetic analysis, therefore potentially benefitting from a fast diagnosis.
However, all de novo variants classified in this cohort as p/lp would also have been highly suspicious candidates in singleton analysis. This raises the question of whether a singleton analysis is sufficient in patients who do not need to be diagnosed particularly quickly. tWES avoids the delay of confirmatory testing for phasing variants in recessive disorders and the determination of inheritance which can upgrade or downgrade their pathogenicity classification according to ACMG guidelines, thereby increasing diagnostic yield. Finding the right approach for each patient is therefore not self-evident.
Unfortunately, only a few studies have been published so far that provide a comparative analysis of the diagnostic efficacy and the costs between singleton and trio analyses (48, 49). In 2019, Tan et al. analysed 30 cases as tWES and singleton WES in parallel (48). The authors concluded that tWES does not significantly increase diagnostic yield compared to single WES, but that tWES approximately halves the time required for prioritization and curation of variants compared to singleton analysis. In addition, they pointed out that analysis of tWES data reduces the cost by selecting fewer variants for curation, avoiding reporting of variants with unclear significance, and eliminates the need for time consuming sequential parental sequencing. Thus, at least in critically ill patients, where a timely genetic diagnosis has the potential to crucial changes in medical management, tWES should be considered as first-line diagnostics.
In our study, the inclusion of exome-based CNV analysis made tWES a powerful tool for diagnosing genetic diseases in additional ten patients just as other studies have reported an increase in detection rate after inclusion of CNV analysis (50). The rate of “secondary findings” in our cohort was 1.3% for the above mentioned 12 genes (in minors) and 17 genes (in adults). The commonly known rate for the detection of secondary findings in clinical exome sequencing is 1%–3%, mainly depending on how many genes were included in secondary analysis. The ACMG initially defined a list of 59 genes associated with secondary findings that should be reported in patients undergoing clinical exome sequencing (51). This gene list was increased to 73 genes (v3.0) (52) and currently includes 78 genes (v3.1) (53).
In summary, our study shows that trio-based exome sequencing in routine diagnostics is effective to obtain a fast genetic diagnosis in paediatric patients. A holistic approach including comprehensive variant assessment of suspected variants, evaluation of CNV data and the use of GeneMatcher maximises the diagnostic output. It is desirable to offer trio sequencing to all patients with suspected monogenetic disease, but especially to critically ill patients, even outside studies within regular healthcare service to minimize the “diagnostic odyssey”.
Data availability statement
The datasets for this article are not publicly available due to concerns regarding participant/patient anonymity. Requests to access the datasets should be directed to the corresponding author.
Ethics statement
The studies involving human participants were reviewed and approved by local Institutional Review Board of Hanover Medical School (ID 8657_BO_K_2019). Written informed consent to participate in this study was provided by the participants’ legal guardian/next of kin.
Author contributions
SH analysed genetic data; SH, GS and BA supported data interpretation; HW, CD and BA provided samples and clinical data; SH, CD, HW wrote the manuscript; GS addressed the bioinformatics issues, SH, BA, GS designed and supervised the study. All authors contributed to the article and approved the submitted version.
Acknowledgments
We are grateful to Manuela Losch and Bernd Haermeyer for their excellent technical assistance. The authors thank all patients, their families, and referring physicians who participated in this study.
Conflict of interest
The authors declare that the research was conducted in the absence of any commercial or financial relationships that could be construed as a potential conflict of interest.
Publisher's note
All claims expressed in this article are solely those of the authors and do not necessarily represent those of their affiliated organizations, or those of the publisher, the editors and the reviewers. Any product that may be evaluated in this article, or claim that may be made by its manufacturer, is not guaranteed or endorsed by the publisher.
Supplementary material
The Supplementary Material for this article can be found online at: https://www.frontiersin.org/articles/10.3389/fped.2023.1183891/full#supplementary-material.
Abbreviations
ACMG, American College of Medical Genetics and Genomics; ICU, intensive care unit; GUS, gene of unknown significance; HBOC, hereditary breast and ovarian cancer; HGVS, Human Genome Variation Society; NGS, next generation sequencing; NICU, neonatal intensive care unit; OMIM, Online Mendelian Inheritance in Man; PICU, pediatric intensive care unit; p, pathogenic; lp, likely pathogenic; rWES, rapid whole exome sequencing; rWGS, rapid whole genome sequencing; SNP, single nucleotide polymorphisms; SNVs, single nucleotide variants; TAT, turn-around time; tWES, trio whole exome sequencing; urWES, urgent whole exome sequencing; urWGS, urgent whole genome sequencing; VUS, variant of unknown significance; WES, whole exome sequencing; WGS, whole genome sequencing.
References
1. Dodge JA, Chigladze T, Donadieu J, Grossman Z, Ramos F, Serlicorni A, et al. The importance of rare diseases: from the gene to society. Arch Dis Child. (2011) 96(9):791–2. doi: 10.1136/adc.2010.193664
2. Stevenson DA, Carey JC. Contribution of malformations and genetic disorders to mortality in a children's hospital. Am J Med Genet A. (2004) 126a(4):393–7. doi: 10.1002/ajmg.a.20409
3. Yoon PW, Olney RS, Khoury MJ, Sappenfield WM, Chavez GF, Taylor D. Contribution of birth defects and genetic diseases to pediatric hospitalizations. A population-based study. Arch Pediatr Adolesc Med. (1997) 151(11):1096–103. doi: 10.1001/archpedi.1997.02170480026004
4. Swaggart KA, Swarr DT, Tolusso LK, He H, Dawson DB, Suhrie KR. Making a genetic diagnosis in a level IV neonatal intensive care unit population: who, when, how, and at what cost? J Pediatr. (2019) 213:211–217.e214. doi: 10.1016/j.jpeds.2019.05.054
5. Daoud H, Luco SM, Li R, Bareke E, Beaulieu C, Jarinova O, et al. Next-generation sequencing for diagnosis of rare diseases in the neonatal intensive care unit. Can Med Assoc J. (2016) 188(11):E254–60. doi: 10.1503/cmaj.150823
6. Sanford EF, Clark MM, Farnaes L, Williams MR, Perry JC, Ingulli EG, et al. Rapid whole genome sequencing has clinical utility in children in the PICU. Pediatr Crit Care Med. (2019) 20(11):1007–20. doi: 10.1097/PCC.0000000000002056
7. Owen MJ, Wright MS, Batalov S, Kwon Y, Ding Y, Chau KK, et al. Reclassification of the etiology of infant mortality with whole-genome sequencing. JAMA Netw Open. (2023) 6(2):e2254069. doi: 10.1001/jamanetworkopen.2022.54069
8. Dimmock DP, Clark MM, Gaughran M, Cakici JA, Caylor SA, Clarke C, et al. An RCT of rapid genomic sequencing among seriously ill infants results in high clinical utility, changes in management, and low perceived harm. Am J Hum Genet. (2020) 107(5):942–52. doi: 10.1016/j.ajhg.2020.10.003
9. Dimmock D, Caylor S, Waldman B, Benson W, Ashburner C, Carmichael JL, et al. Project baby bear: rapid precision care incorporating rWGS in 5 California children's hospitals demonstrates improved clinical outcomes and reduced costs of care. Am J Hum Genet. (2021) 108(7):1231–8. doi: 10.1016/j.ajhg.2021.05.008
10. French CE, Delon I, Dolling H, Sanchis-Juan A, Shamardina O, Megy K, et al. Whole genome sequencing reveals that genetic conditions are frequent in intensively ill children. Intensive Care Med. (2019) 45(5):627–36. doi: 10.1007/s00134-019-05552-x
11. Group TNS. Effect of whole-genome sequencing on the clinical management of acutely ill infants with suspected genetic disease: a randomized clinical trial. JAMA Pediatr. (2021) 175(12):1218–26. doi: 10.1001/jamapediatrics.2021.3496
12. Cakici JA, Dimmock DP, Caylor SA, Gaughran M, Clarke C, Triplett C, et al. A prospective study of parental perceptions of rapid whole-genome and -exome sequencing among seriously ill infants. Am J Hum Genet. (2020) 107(5):953–62. doi: 10.1016/j.ajhg.2020.10.004
13. Krabbenborg L, Vissers LE, Schieving J, Kleefstra T, Kamsteeg EJ, Veltman JA, et al. Understanding the psychosocial effects of WES test results on parents of children with rare diseases. J Genet Couns. (2016) 25(6):1207–14. doi: 10.1007/s10897-016-9958-5
14. Farnaes L, Hildreth A, Sweeney NM, Clark MM, Chowdhury S, Nahas S, et al. Rapid whole-genome sequencing decreases infant morbidity and cost of hospitalization. NPJ Genom Med. (2018) 3:10. doi: 10.1038/s41525-018-0049-4
15. Gubbels CS, VanNoy GE, Madden JA, Copenheaver D, Yang S, Wojcik MH, et al. Prospective, phenotype-driven selection of critically ill neonates for rapid exome sequencing is associated with high diagnostic yield. Genet Med. (2020) 22(4):736–44. doi: 10.1038/s41436-019-0708-6
16. Kingsmore SF, Cakici JA, Clark MM, Gaughran M, Feddock M, Batalov S, et al. A randomized, controlled trial of the analytic and diagnostic performance of singleton and trio, rapid genome and exome sequencing in ill infants. Am J Hum Genet. (2019) 105(4):719–33. doi: 10.1016/j.ajhg.2019.08.009
17. Sobreira N, Schiettecatte F, Valle D, Hamosh A. Genematcher: a matching tool for connecting investigators with an interest in the same gene. Hum Mutat. (2015) 36(10):928–30. doi: 10.1002/humu.22844
18. World Medical Association. World medical association declaration of Helsinki: ethical principles for medical research involving human subjects. J Am Med Assoc. (2000) 284(23):3043–5. doi: 10.1001/jama.284.23.3043
19. Richards S, Aziz N, Bale S, Bick D, Das S, Gastier-Foster J, et al. Standards and guidelines for the interpretation of sequence variants: a joint consensus recommendation of the American college of medical genetics and genomics and the association for molecular pathology. Genet Med. (2015) 17(5):405–24. doi: 10.1038/gim.2015.30
20. Riggs ER, Andersen EF, Cherry AM, Kantarci S, Kearney H, Patel A, et al. Correction: technical standards for the interpretation and reporting of constitutional copy-number variants: a joint consensus recommendation of the American college of medical genetics and genomics (ACMG) and the clinical genome resource (ClinGen). Genet Med. (2021) 23(11):2230. doi: 10.1038/s41436-021-01150-9
21. den Dunnen JT, Dalgleish R, Maglott DR, Hart RK, Greenblatt MS, McGowan-Jordan J, et al. HGVS recommendations for the description of sequence variants: 2016 update. Hum Mutat. (2016) 37(6):564–9. doi: 10.1002/humu.22981
22. Kalia SS, Adelman K, Bale SJ, Chung WK, Eng C, Evans JP, et al. Recommendations for reporting of secondary findings in clinical exome and genome sequencing, 2016 update (ACMG SF v2.0): a policy statement of the American college of medical genetics and genomics. Genet Med. (2017) 19(2):249–55. doi: 10.1038/gim.2016.190
23. Demidov G. ClinCNV: novel method for allele-specific somatic copy-number alterations detection (2019). doi: 10.1101/837971. https://www.biorxiv.org/content/10.1101/837971v1.
24. Sobreira N, Schiettecatte F, Boehm C, Valle D, Hamosh A. New tools for Mendelian disease gene identification: phenoDB variant analysis module; and GeneMatcher, a web-based tool for linking investigators with an interest in the same gene. Hum Mutat. (2015) 36(4):425–31. doi: 10.1002/humu.22769
25. Polla DL, Farazi Fard MA, Tabatabaei Z, Habibzadeh P, Levchenko OA, Nikuei P, et al. Biallelic variants in TMEM222 cause a new autosomal recessive neurodevelopmental disorder. Genet Med. (2021) 23(7):1246–54. doi: 10.1038/s41436-021-01133-w
26. Vavassori S, Chou J, Faletti LE, Haunerdinger V, Opitz L, Joset P, et al. Multisystem inflammation and susceptibility to viral infections in human ZNFX1 deficiency. J Allergy Clin Immunol. (2021) 148(2):381–93. doi: 10.1016/j.jaci.2021.03.045
27. Neuray C, Maroofian R, Scala M, Sultan T, Pai GS, Mojarrad M, et al. Early-infantile onset epilepsy and developmental delay caused by bi-allelic GAD1 variants. Brain. (2020) 143(8):2388–97. doi: 10.1093/brain/awaa178
28. von Hardenberg S, Richter MF, Hethey S, Yaspo ML, Auber B, Schlegelberger B, et al. Rational therapy with vigabatrin and a ketogenic diet in a patient with GAD1 deficiency. Brain. (2020) 143(11):e91. doi: 10.1093/brain/awaa289
29. Marsh RA, Bleesing JJ, Filipovich AH. Using flow cytometry to screen patients for X-linked lymphoproliferative disease due to SAP deficiency and XIAP deficiency. J Immunol Methods. (2010) 362(1-2):1–9. doi: 10.1016/j.jim.2010.08.010
30. Asano T, Khourieh J, Zhang P, Rapaport F, Spaan AN, Li J, et al. Human STAT3 variants underlie autosomal dominant hyper-IgE syndrome by negative dominance. J Exp Med. (2021) 218(8):e20202592.. doi: 10.1084/jem.20202592
31. Deignan JL, Chung WK, Kearney HM, Monaghan KG, Rehder CW, Chao EC. Points to consider in the reevaluation and reanalysis of genomic test results: a statement of the American college of medical genetics and genomics (ACMG). Genet Med. (2019) 21(6):1267–70. doi: 10.1038/s41436-019-0478-1
32. Salfati EL, Spencer EG, Topol SE, Muse ED, Rueda M, Lucas JR, et al. Re-analysis of whole-exome sequencing data uncovers novel diagnostic variants and improves molecular diagnostic yields for sudden death and idiopathic diseases. Genome Med. (2019) 11(1):83. doi: 10.1186/s13073-019-0702-2
33. Liu P, Meng L, Normand EA, Xia F, Song X, Ghazi A, et al. Reanalysis of clinical exome sequencing data. N Engl J Med. (2019) 380(25):2478–80. doi: 10.1056/NEJMc1812033
34. Baker SW, Murrell JR, Nesbitt AI, Pechter KB, Balciuniene J, Zhao X, et al. Automated clinical exome reanalysis reveals novel diagnoses. J Mol Diagn. (2019) 21(1):38–48. doi: 10.1016/j.jmoldx.2018.07.008
35. Savatt JM, Myers SM. Genetic testing in neurodevelopmental disorders. Front Pediatr. (2021) 9:526779. doi: 10.3389/fped.2021.526779
36. Stavropoulos DJ, Merico D, Jobling R, Bowdin S, Monfared N, Thiruvahindrapuram B, et al. Whole genome sequencing expands diagnostic utility and improves clinical management in pediatric medicine. NPJ Genom Med. (2016) 1:15012. doi: 10.1038/npjgenmed.2015.12
37. Group NIS, Krantz ID, Medne L, Weatherly JM, Wild KT, Biswas S, et al. Effect of whole-genome sequencing on the clinical management of acutely ill infants with suspected genetic disease: a randomized clinical trial. JAMA Pediatr. (2021) 175(12):1218–26. doi: 10.1001/jamapediatrics.2021.3496
38. Hauser NS, Solomon BD, Vilboux T, Khromykh A, Baveja R, Bodian DL. Experience with genomic sequencing in pediatric patients with congenital cardiac defects in a large community hospital. Mol Genet Genomic Med. (2018) 6(2):200–12. doi: 10.1002/mgg3.357
39. van Diemen CC, Kerstjens-Frederikse WS, Bergman KA, de Koning TJ, Sikkema-Raddatz B, van der Velde JK, et al. Rapid targeted genomics in critically ill newborns. Pediatrics. (2017) 140(4):e20162854. doi: 10.1542/peds.2016-2854
40. Willig LK, Petrikin JE, Smith LD, Saunders CJ, Thiffault I, Miller NA, et al. Whole-genome sequencing for identification of Mendelian disorders in critically ill infants: a retrospective analysis of diagnostic and clinical findings. Lancet Respir Med. (2015) 3(5):377–87. doi: 10.1016/S2213-2600(15)00139-3
41. Hauer NN, Popp B, Schoeller E, Schuhmann S, Heath KE, Hisado-Oliva A, et al. Clinical relevance of systematic phenotyping and exome sequencing in patients with short stature. Genet Med. (2018) 20(6):630–8. doi: 10.1038/gim.2017.159
42. Lionel AC, Costain G, Monfared N, Walker S, Reuter MS, Hosseini SM, et al. Improved diagnostic yield compared with targeted gene sequencing panels suggests a role for whole-genome sequencing as a first-tier genetic test. Genet Med. (2018) 20(4):435–43. doi: 10.1038/gim.2017.119
43. Hiatt SM, Amaral MD, Bowling KM, Finnila CR, Thompson ML, Gray DE, et al. Systematic reanalysis of genomic data improves quality of variant interpretation. Clin Genet. (2018) 94(1):174–8. doi: 10.1111/cge.13259
44. Thiffault I, Farrow E, Zellmer L, Berrios C, Miller N, Gibson M, et al. Clinical genome sequencing in an unbiased pediatric cohort. Genet Med. (2019) 21(2):303–10. doi: 10.1038/s41436-018-0075-8
45. Taylor JC, Martin HC, Lise S, Broxholme J, Cazier JB, Rimmer A, et al. Factors influencing success of clinical genome sequencing across a broad spectrum of disorders. Nat Genet. (2015) 47(7):717–26. doi: 10.1038/ng.3304
46. Gilissen C, Hehir-Kwa JY, Thung DT, van de Vorst M, van Bon BW, Willemsen MH, et al. Genome sequencing identifies major causes of severe intellectual disability. Nature. (2014) 511(7509):344–7. doi: 10.1038/nature13394
47. Farwell KD, Shahmirzadi L, El-Khechen D, Powis Z, Chao EC, Tippin Davis B, et al. Enhanced utility of family-centered diagnostic exome sequencing with inheritance model-based analysis: results from 500 unselected families with undiagnosed genetic conditions. Genet Med. (2015) 17(7):578–86. doi: 10.1038/gim.2014.154
48. Tan TY, Lunke S, Chong B, Phelan D, Fanjul-Fernandez M, Marum JE, et al. A head-to-head evaluation of the diagnostic efficacy and costs of trio versus singleton exome sequencing analysis. Eur J Hum Genet. (2019) 27(12):1791–9. doi: 10.1038/s41431-019-0471-9
49. Alfares A, Alsubaie L, Aloraini T, Alaskar A, Althagafi A, Alahmad A, et al. What is the right sequencing approach? Solo VS extended family analysis in consanguineous populations. BMC Med Genomics. (2020) 13(1):103. doi: 10.1186/s12920-020-00743-8
50. Testard Q, Vanhoye X, Yauy K, Naud ME, Vieville G, Rousseau F, et al. Exome sequencing as a first-tier test for copy number variant detection: retrospective evaluation and prospective screening in 2418 cases. J Med Genet. (2022) 59(12):1234–40. doi: 10.1136/jmg-2022-108439
51. Green RC, Berg JS, Grody WW, Kalia SS, Korf BR, Martin CL, et al. ACMG recommendations for reporting of incidental findings in clinical exome and genome sequencing. Genet Med. (2013) 15(7):565–74. doi: 10.1038/gim.2013.73
52. Miller DT, Lee K, Chung WK, Gordon AS, Herman GE, Klein TE, et al. Correction to: ACMG SF v3.0 list for reporting of secondary findings in clinical exome and genome sequencing: a policy statement of the American college of medical genetics and genomics (ACMG). Genet Med. (2021) 23(8):1582–4. doi: 10.1038/s41436-021-01278-8
53. Miller DT, Lee K, Abul-Husn NS, Amendola LM, Brothers K, Chung WK, et al. ACMG SF v3.1 list for reporting of secondary findings in clinical exome and genome sequencing: a policy statement of the American college of medical genetics and genomics (ACMG). Genet Med. (2022) 24(7):1407–14. doi: 10.1016/j.gim.2022.04.006
Keywords: trioWES, CNV, GeneMatcher, re-evaluation, diagnostic odyssey
Citation: von Hardenberg S, Wallaschek H, Du C, Schmidt G and Auber B (2023) A holistic approach to maximise diagnostic output in trio exome sequencing. Front. Pediatr. 11:1183891. doi: 10.3389/fped.2023.1183891
Received: 10 March 2023; Accepted: 2 May 2023;
Published: 19 May 2023.
Edited by:
Pilar Giraldo, University of Zaragoza, SpainReviewed by:
Laurel Willig, Children's Mercy Kansas City, United StatesAmmar Husami, Cincinnati Children's Hospital Medical Center, United States
© 2023 von Hardenberg, Wallaschek, Du, Schmidt and Auber. This is an open-access article distributed under the terms of the Creative Commons Attribution License (CC BY). The use, distribution or reproduction in other forums is permitted, provided the original author(s) and the copyright owner(s) are credited and that the original publication in this journal is cited, in accordance with accepted academic practice. No use, distribution or reproduction is permitted which does not comply with these terms.
*Correspondence: Sandra von Hardenberg dm9uaGFyZGVuYmVyZy5zYW5kcmFAbWgtaGFubm92ZXIuZGU= Bernd Auber YXViZXIuYmVybmRAbWgtaGFubm92ZXIuZGU=
†These authors have contributed equally to this work and share last authorship
‡ORCID Sandra von Hardenberg orcid.org/0000-0002-3680-7450 Bernd Auber orcid.org/0000-0003-1880-291X