- 1Department of Pharmaceutical Sciences, School of Pharmacy and Pharmaceutical Sciences, University at Buffalo, Buffalo, NY, USA
- 2Department of Biomedical Engineering, Rutgers University, Piscataway, NJ, USA
- 3Department of Biological Sciences, University at Buffalo, Buffalo, NY, USA
- 4Department of Surgery, Rutgers Robert Wood Johnson Medical School, New Brunswick, NJ, USA
- 5BioMaPS Institute for Quantitative Biology, Rutgers University, Piscataway, NJ, USA
- 6Department of Chemical Engineering, Rutgers University, Piscataway, NJ, USA
In this paper, we discuss approaches for integrating biological information reflecting diverse physiologic levels. In particular, we explore statistical and model-based methods for integrating transcriptomic, proteomic and metabolomics data. Our case studies reflect responses to a systemic inflammatory stimulus and in response to an anti-inflammatory treatment. Our paper serves partly as a review of existing methods and partly as a means to demonstrate, using case studies related to human endotoxemia and response to methylprednisolone (MPL) treatment, how specific questions may require specific methods, thus emphasizing the non-uniqueness of the approaches. Finally, we explore novel ways for integrating -omics information with PKPD models, toward the development of more integrated pharmacology models.
Introduction
Life is complex at all scales. From a single cell to the whole body, there are myriad intricate mechanisms that control every aspect of this complexity, Figure 1. The ultimate aim of biomedical sciences is to establish a thorough understanding of how these control mechanisms function when in a healthy state and how the control is lost (or shifted to a new mode) when symptoms of a disease are displayed, in order to explain the observed phenotypic changes with the known paradigms at the molecular level. Our ability to collect information about molecular events in our bodies has tremendously increased with great advancements in technology. However, we still have a long way to go finding the best ways to fully utilize this information.
Numerous -omics tools are available, each of which makes it possible to observe the physiologic responses at their complementary level. They enable the examination of a broad array of cellular or systemic elements and functions through the use of vast amounts of quantitative or semi-quantitative data from various levels of biological organizations (Richards et al., 2010). Systems biology rises on these new technologies and its most current and significant challenge is developing methods to integrate the vast amount of information into a conceptual framework that is holistic, quantitative and predictive (Kritikou et al., 2006). The intent is to reach a thorough understanding of the biological mechanisms driving different processes in our bodies and ascertain insights how to manipulate these processes for our benefit.
Inflammation is one of those core processes through which we produce responses against various stressors, such as pathogens or trauma. It is a complex and multiscale biological phenomenon that needs to be orchestrated under tight regulation (Laroux, 2004). Factors inducing physiological stress are sensed and translated into biological cues that transmit signals throughout organs and down to the cellular level. These incoming signals are then recognized and processed to produce a response in a dynamic and highly regulated manner. Collective cell responses change the dynamic of biological metrics, manifesting change at the individual organ level and ultimately throughout the entire body. Under normal conditions, the outcome of inflammation is the mounting of required immune response for pathogen elimination or regeneration following injury; however, in any instance of dysregulation of this complex process, the uncontrolled response can induce further damage or lead to a non-sustainable systemic disease state (Bone, 1996), Figure 2. Considering its critical role in our survival, inherent complexity and intricate relationships with other essential physiologic processes; inflammation and inflammatory diseases are among the research fields that can benefit from adapting the systems approach. In this respect, the emerging -omics tools are very promising, since they offer the advantage of observing the inflammatory response at a much broader level together with the ability to analyze multiple variables simultaneously which empowers the application of systems analysis for rationalizing and modeling the course of physiologic events.
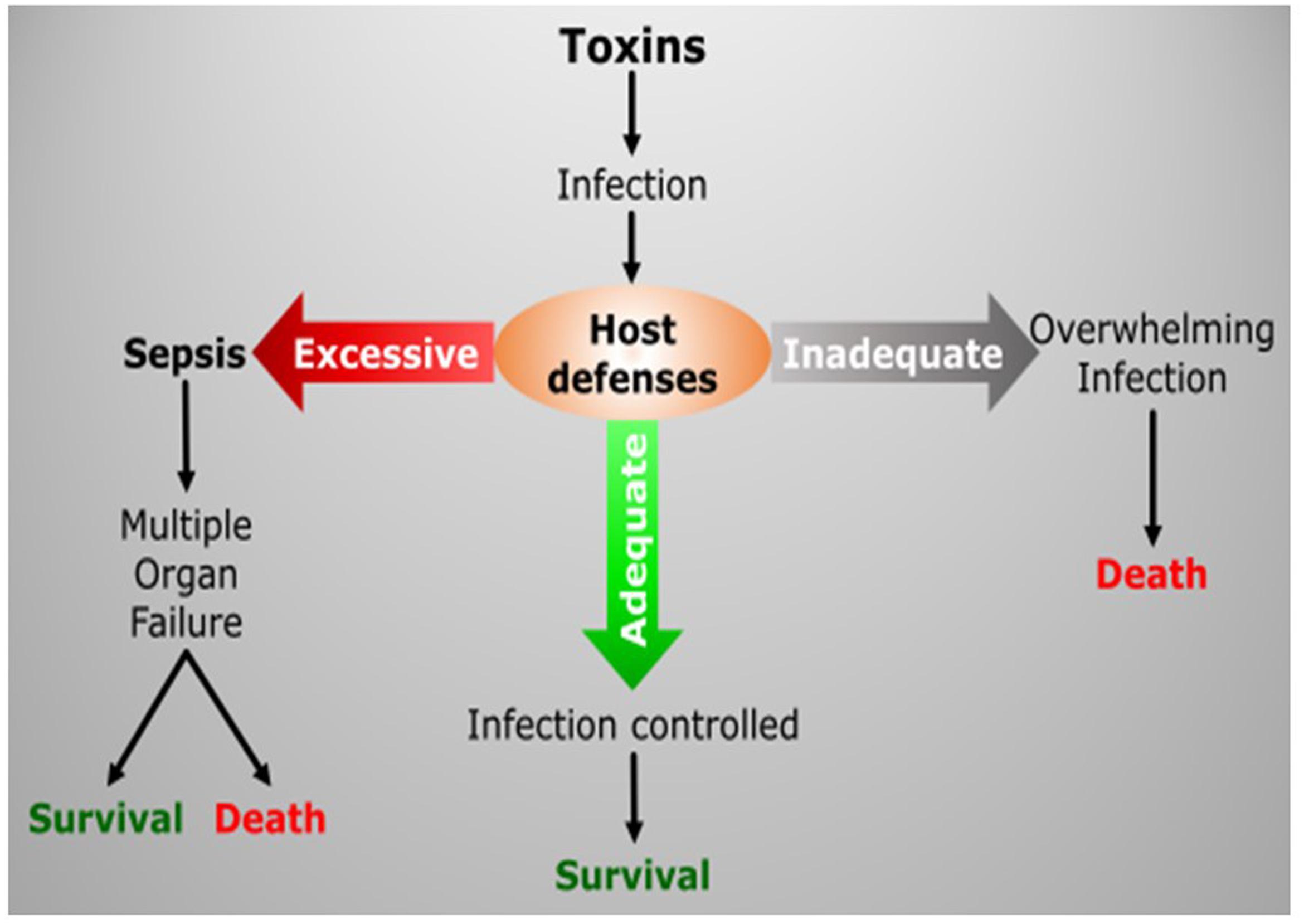
FIGURE 2. Inflammatory response is a complex process, which has to be tightly regulated in order to balance the defense mechanisms of the host with the severity of infection/tissue damage. Loss of this control in favor of either side may have fatal consequences.
Comprehending the continuum of physiologic responses to pathologic stimuli is essential for making sense of how molecular changes develop to induce observable symptoms of a particular disease. Drugs, i.e., pharmacologic stimuli, are intended to reverse this disease progression and reduce the symptoms. For most cases, physiologic effects of drugs are also complex and include re-directing physiologic responses to alleviate symptoms of a particular pathologic condition as well as inducing adverse-effects associated with off-target reactions. Analyses of the physiologic effects of drugs by monitoring a handful of markers for the targeted effects has been used for building models of drug action for many years. Extensive -omics analyses done at multiple physiologic levels, however, also impacted this research area tremendously and initiated a shift from classic pharmacokinetic/pharmacodynamic modeling (PK/PD) toward a systems approach defined as quantitative systems pharmacology (QSP) (Iyengar et al., 2012; Jusko, 2013; Androulakis, 2015, 2016). The ultimate direction for this field is the realization of personalized and precision medicine by building progressively more accurate drug action models. However, the first steps toward these goals involve devising methods to fully utilize the wealth of information produced by the extensive analyses of pharmacologic responses.
This paper is centered on integrating information from multiple physiologic levels. We focused on how critical relationships are shaped over time during the development of the response to a systemic inflammatory stimulus and in response to an anti-inflammatory treatment. The systems approach allowed us to track the continuum of physiologic responses through their evolution and in relation to multiple dynamics running in harmony. We extracted the coherent dynamic responses represented in the -omics analyses at multiple physiologic levels and integrated them using multiple approaches. The analyses described in the sections that follow include metabolic and transcriptional responses to endotoxemia, an experimental model in humans that recapitulates the dynamics of systemic inflammatory response. We then switch to an anti-inflammatory therapy and focus on the effects of a commonly used synthetic glucocorticoid in liver. This analysis represents a more direct integration approach, in which we evaluate the concordance of the hepatic response to the drug treatment at gene and protein expression levels. Finally, we discuss a network-based approach that integrates -omics information with existing pharmacokinetic/pharmacodynamic models.
Integration of -Omics Data: Why and How?
“Why” and “how” are, likely, the most critical questions that need to be addressed as we aggressively enter the -omics era. The answer to “why” is straightforward: life is a complex organization of functional entities each manifesting the actions and activities of appropriate levels through corresponding markers at a suitable level, broadly described as -omics data. Therefore, the -omics data provide a snapshot of the system across multiple levels of organization. The suffix “ome” is used to identify groups of objects sharing common characteristics, either descriptive or functional. At an elementary level we routinely think of genomic (sequence of the genome), transcriptomic (expression of the genome), proteomic (expression of the proteome), metabolomics (expression of the metabolome) and the list is continuously expanded to include the epigenome (Koch, 2016), interactome (Karagoz et al., 2016), regulome (Cheng et al., 2015), microbiome (Althani et al., 2016) to name only a few.
Clearly, one of the key challenges in the -omics era is “integration” and most precisely how to integrate. It is important to realize that although the various -omics components at some elementary level augment the number of descriptors, the augmentation is not passive, i.e., it is not simply increasing the dimensionality of the space. Rather, it introduces additional layers of knowledge which are not independent of other layers, that is the various -omics data are functionally related, most likely implicitly among themselves. Seemingly simple, yet profound, challenges emerge (Gomez-Cabrero et al., 2014) while a wide range of methods have been proposed and extensively reviewed (Rogers, 2011; Meng et al., 2014). Broadly speaking we will argue that integration can be accomplished either (a) via statistical means considering the computational challenges associated with simultaneous analysis of disparate data sources (Meng et al., 2014; Gligorijevic et al., 2016) or (b) realizing that the -omics information comes together, eventually, in the form of a yet to be determined, dynamics model expressing interactions, cross-functionality and constraints (Chen et al., 1999; Palsson, 2002; Joyce and Palsson, 2006; Hyduke et al., 2013). However, the purpose of the present discussion is not to provide a detailed account of the field and/or the approaches, but rather articulate, through the use of specific examples: (1) how the nature of the data can guide, and to some extent constrain, the type of question that can be asked; and (2) how questions can guide the approach that need to be developed and pursued. Both of these topics will be discussed in the context of specific applications related to inflammation and anti-inflammatory drugs. Each of the sections below is primarily concerned with addressing one particular questions and outlining one, of the likely many approaches that exist for addressing it.
Data-Driven Integration of Transcriptomic and Proteomic Data at the Same Level of Physiological Organization
The first case study refers to a scenario where the -omics data reflect different processes, principally within the same cell type, reflecting a sequence of events. Likely the most characteristic example of this would be transcriptomic and proteomic information. The fundamental question arising here is how to upgrade the longitudinal information quantifying gene and protein expression simultaneously, for a particular cell type in response to an external perturbation or environmental condition. In a simplistic way, one can view this problem as an extension of the so-called central dogma. We demonstrate the alternatives by focusing on the analysis of transcriptomic and proteomic data obtained from the in vivo response of a rodent model following bolus administration of synthetic glucocorticoids. Studies focusing on understanding the relationship between global mRNA transcription and protein translation have produced mixed results, many of which concluded that the transcriptomic and proteomic data is far from being easily described as complementary (Greenbaum et al., 2003; Hegde et al., 2003; Nicholson et al., 2004; Waters et al., 2006; Haider and Pal, 2013; Motta and Pappalardo, 2013; Castiglione et al., 2014). Nevertheless, both data types reflect the dynamics of the cellular response to any given perturbation, thus capture critical information reflecting different facets of the response. Without considering any type of functional relation between transcriptomic and proteomic data, each can be considered independently or in tandem. In any “data driven” query, the approach undertaken largely reflects the “biases” imposed by the type of question one wishes to address (Androulakis et al., 2007).
In the sections that follow, we will address a basic question: assuming that the response to drug elicits changes at both the transcriptional and translational level, is it reasonable to assume that one would focus on gene transcripts and corresponding protein abundance both exhibiting substantial temporal changes relative to base? (Note: in the analysis that follows, we assume that the base line expression levels, i.e., in the absence of the drug, are represented by mRNA and protein abundance levels prior to drug administration). In a number of previous publications, we have illustrated the analysis of longitudinal data with a time-varying base line (Androulakis et al., 2007; Yang et al., 2007b, 2008a, 2009b, 2012a,b; Yang E.H. et al., 2009; Almon et al., 2008a,b; Nguyen et al., 2009, 2010a,b, 2011, 2014a; Ovacik et al., 2010; Scheff et al., 2010a; Swiss et al., 2011). However, temporal relations among time-varying quantities are known to be non-trivial, extending far beyond the classic view of correlation (Qian et al., 2001).
Synthetic Glucocorticoids
Synthetic corticosteroids (CS), such as MPL, are widely used anti-inflammatory and immunosuppressive agents for the treatment of many inflammatory and auto-immune conditions including organ transplantation, rheumatoid arthritis, lupus erythematosus, asthma and allergic rhinitis (Swartz and Dluhy, 1978; Barnes, 1998). The mechanism of action of CS drugs is basically magnifying the physiological actions of the endogenous glucocorticoid hormones, which have anti-inflammatory properties depending on their secretion level and the time at which they are secreted. These hormones also have diverse effects on a variety of physiological processes including carbohydrate, lipid and protein metabolism, immune-regulation, bone homeostasis and developmental processes (Barnes, 1998; Vegiopoulos and Herzig, 2007). The well-established molecular mechanism of action for CS includes the passive diffusion of the highly lipophilic CS molecule through the cell membrane and binding to the cytosolic glucocorticoid receptor, which is held inactive through the association with heat shock proteins (Schaaf and Cidlowski, 2002). Binding of the drug to the receptor causes conformational changes, phosphorylation and activation of receptor, resulting in the formation of a homodimer of the drug receptor complex (Schaaf and Cidlowski, 2002; Oakley and Cidlowski, 2011). This activated complex translocates into the nucleus and binds to regulator sites, glucocorticoid regulatory elements (GREs) in the DNA, resulting in the regulation of transcription rate. In addition to direct binding, the activated complex can regulate gene expression by other mechanisms including tethering and composite binding to other transcription factors, activators, or repressors (Barnes, 1998; Schaaf and Cidlowski, 2002). Studies have shown that CS can regulate pathways by signaling through receptors in a transcription-independent manner, although the exact mechanisms for the non-genomic effects are still unclear (Schaaf and Cidlowski, 2002). While short-term use of CSs is beneficial for reducing the inflammation, long-term use is associated with serious side-effects – including hyperglycemia, negative nitrogen balance, and fat redistribution, leading to complications including diabetes, muscle wasting, osteoporosis, hypertension, cataracts, peptic ulcers, and reduced resistance to infection and adrenal insufficiency following withdrawal of therapy (Andrews and Walker, 1999; Morand and Leech, 1999). The undesirable metabolic effects of CS cannot usually be separated from their favorable anti-inflammatory effects since most actions manifest via the same glucocorticoid system.
The analysis of the diverse physiological effects of synthetic glucocorticoids has been the subject of many pharmacokinetic and pharmacodynamic modeling efforts. A series of models has been developed to explain the dynamics of receptor regulation and enzyme induction following MPL administration (Sun et al., 1998; Ramakrishnan et al., 2002), Figure 3 (top). The models were progressively enhanced to capture the effects of the drug under several doses and dosing regimens. However, these models were based on the data generated by traditional message quantification methods that only allow measurements of single end points. Because of the diverse effects of CS and different molecular mechanisms potentially involved in these actions, a high-throughput transcriptomic, i.e., microarray, approach was effective in gaining better understanding of the temporal and tissue-specific effects of CS on different pathways and functions (Almon et al., 2007a,b). The diversity of the available models describing gene induction by MPL have been expanded to several pharmacogenomics models that explain the response of numerous genes with various dynamic patterns (Almon et al., 2003, 2005, 2007a,b; Jin et al., 2003; Nguyen et al., 2014a). Our earlier studies in collaboration with Prof. Jusko, characterized global dynamics of the systems that are regulated by CS at the transcriptional level across multiple tissues in adrenalectomized (ADX) and intact male rats, following single and chronic dosing of MPL enabling us to: (a) develop transcription-level understanding of MPL (acute vs. chronic) effects; (b) elaborate on tissue-specific transcriptional differences; (c) assess MPL-induced dose- and tissue-specific transcriptional regulation; and (d) assess circadian dynamics and regulation of intact and MPL-dosed animals (Yang et al., 2007b, 2008a; Yang E.H. et al., 2009; Almon et al., 2008a; Nguyen et al., 2010a,b, 2014a; Ovacik et al., 2010; Scheff et al., 2010a, 2011).
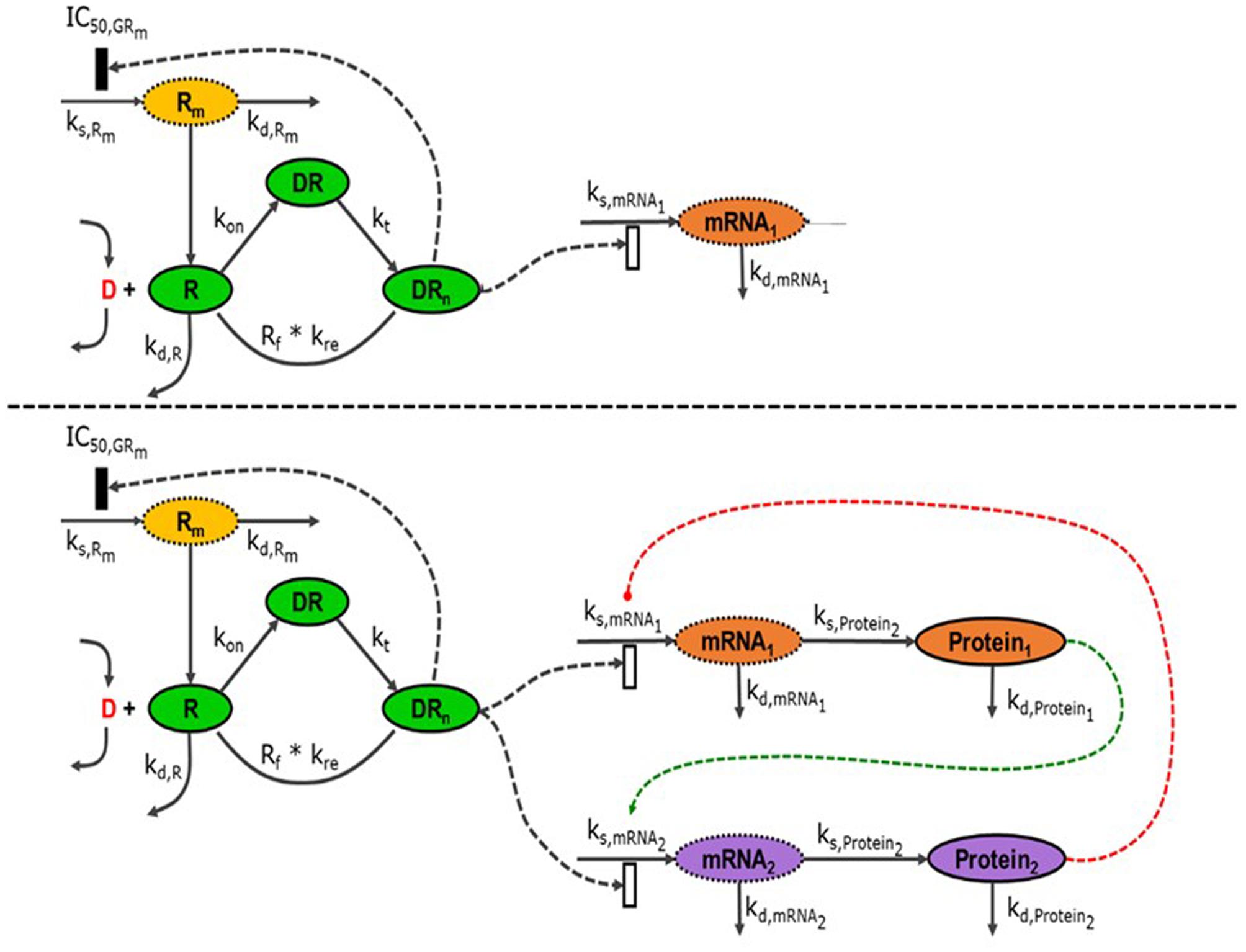
FIGURE 3. (Top) Schematic representation of the network-based indirect response model to MPL (D). Once the drug-receptor complex translocates into the nucleus (DRn), it induces its effects on the transcription of its target genes (mRNA1, mRNA2) either by stimulation (open rectangle) or by inhibiting it (closed rectangle). (Bottom) When transcribed messages are translated to the active proteins (Protein1, Protein2), they can also have effects, either stimulatory (green) or inhibitory (red), on the transcription of target genes affected by MPL. All these effects are considered as indirect as there might be additional biological processes in between.
Although transcriptional information is useful and highly relevant, direct profiling of the protein expression changes and integration of the information from proteomic data will provide deeper insights into CS actions (Nouri-Nigjeh et al., 2014). Recently, high-throughput, ion current-based liquid chromatography/mass spectrometry (LC/MS), allowed comprehensive and accurate profiling of the tissue proteome (Tu et al., 2012). Using this methodology, the temporal changes in the expression of almost a 1000 proteins in rat liver following MPL administration were characterized (Nouri-Nigjeh et al., 2014). The analysis of the combined transcriptomic and proteomic data confirmed that significant indirect regulation by MPL was evident, notably indicated by significant changes in mRNA and protein levels in the absence of glucocorticoid responsive promoter elements. With this new information from the protein expression level, it was possible to evaluate complementarities between transcription and translation of the target genes and elaborate on the interplay between gene and protein expression in liver toward a more complete understanding of the indirect mechanisms of action of MPL (Kamisoglu et al., 2014). This data confirmed that MPL effects are propagated across a network of interacting genes and proteins, Figure 3 (bottom). Without considering any type of functional relation between transcriptomic and proteomic data, each can be considered independently (Androulakis et al., 2007) and using statistical methods one can analyze temporal trends at each level. This was the approach undertaken recently in our lab and an extensive discussion of the methods and results was recently presented (Kamisoglu et al., 2014).
Animal Model, Proteomic and Transcriptomic Data
Proteomic Studies
Sixty adrenalectomized (ADX) Wistar rats were injected with 50 mg/kg methylprednisolone (MPL) intramuscularly and sacrificed at 12 different time points between 0.5 and 66 h post-dosing (five animals/time point). Five animals, injected with saline and sacrificed at random time points in the same time window, served as controls. In order to remove the high concentrations of blood protein, it was necessary to use perfused tissue for proteomic analyses, which precluded the use of the same tissues employed for transcriptomics (below). Proteins from perfused and flash frozen livers were extracted, digested and analyzed using a nano-LC/LTQ/Orbitrap instrument. The Nano Flow Ultra-high Pressure LC system (nano-UPLC) consisted of a Spark Endurance autosampler (Emmen, Holland) and an ultra-high pressure Eksigent (Dublin, CA, USA) Nano-2D Ultra capillary/nano-LC system, with a LTQ Orbitrap mass spectrometer (Thermo Fisher Scientific, San Jose, CA, USA) used for detection. Protein quantification was based on the area under the curve (AUC) of the ion-current-peaks. A more extensive description of the experimental setup and the analytical methodology can be found in the previously published study (Nouri-Nigjeh et al., 2014).
Transcriptomic Studies
Forty-three ADX Wistar rats were given a bolus dose of 50 mg/kg MPL intravenously. Animals were sacrificed at 16 different time points between 0.25 and 72 h post-dosing. Four untreated animals sacrificed at 0 h served as controls. The mRNA expression profiles of the liver were arrayed via Affymetrix GeneChips Rat Genome U34A (Affymetrix, Inc.), which contained 8800 full-length sequences and approximately 1000 expressed sequence tag clusters (Jin et al., 2003). This dataset was previously submitted to the GEO (GSE490). We have previously presented extensive analyses and studies of the transcription response to acute and chronic administration of MPL on multiple tissues (Almon et al., 2008a,b; Yang et al., 2008a; Nguyen et al., 2010a, 2014a; Ovacik et al., 2010).
All animal experiments were performed at the University of Buffalo and protocols adhered to “Principles of Laboratory Animal Care” (NIH publication 85-23, revised in 1985) and were approved by the University at Buffalo IACUC committee.
Integration and Analysis of Transcriptomic/Proteomic Data
Assessment of the problem greatly reflects the approach(es) taken (Androulakis et al., 2007) whereas in (Kamisoglu et al., 2015b) we described multiple approaches to integrate this temporal information from the proteome level with the corresponding dynamics in the transcriptomic level through data-driven approaches. In the analysis that follows we addressed the question in two different ways. First, we identified transcripts and proteins which are over-expressed post MPL administration. The intersection of the two sets indicates genes from which both transcription and translation is significantly altered. The simultaneous consideration enables us to consider the following question: what is the dynamic correlation of the subset of MPL-regulated transcripts and proteins? As mentioned earlier, and shown later, the relation is far from trivial. However, such analysis, only depicts part of the picture. It is highly likely that transcriptional events do not manifest themselves at the protein levels and also post-transcriptional alterations may not include transcriptional alterations. For such an analysis, the two -omics data need to be separated and analyses performed. Therefore, in the former case the mining of the data is concurrent but the functional interpretation separate, whereas in the latter the mining of the data is separate but the functional analysis concurrent. The case study discussed in the following section demonstrates both approaches. Once again, it is important to realize that we only discuss two of the many questions which could be posed and addressed and the presentation is by no means exhaustive or all-encompassing.
Augmenting the Feature Vector: Clustering of Concatenated Dataset
This analysis will help us identify the genes which were differentially expressed both at the transcriptional and translational levels. Data analysis for both proteomic and transcriptomic datasets started first by filtering for differential expression over time. Proteins and transcripts with differential temporal profiles were determined by using EDGE (Leek et al., 2006). We employed within-class differential expression to extract profiles that have a differential expression over time (Storey et al., 2005, 2007; Leek et al., 2006). Integration of these two datasets for any further analysis required matching the object identifiers which was achieved through running a comparison between two filtered datasets in Ingenuity Pathway Analysis (IPA, Ingenuity® Systems)1.
In order to find potential co-regulatory relationships at these two levels, hierarchical clustering was used for first-pass analysis. For this purpose, temporal transcriptomic and proteomic data for the common genes were first concatenated and then clustered using the clustergram function in the Bioinformatics toolbox of MATLAB (Mathworks, Natick, MA, USA). The two clusters obtained by using correlation as the distance metric. The workflow is illustrated in Figure 4 (top).
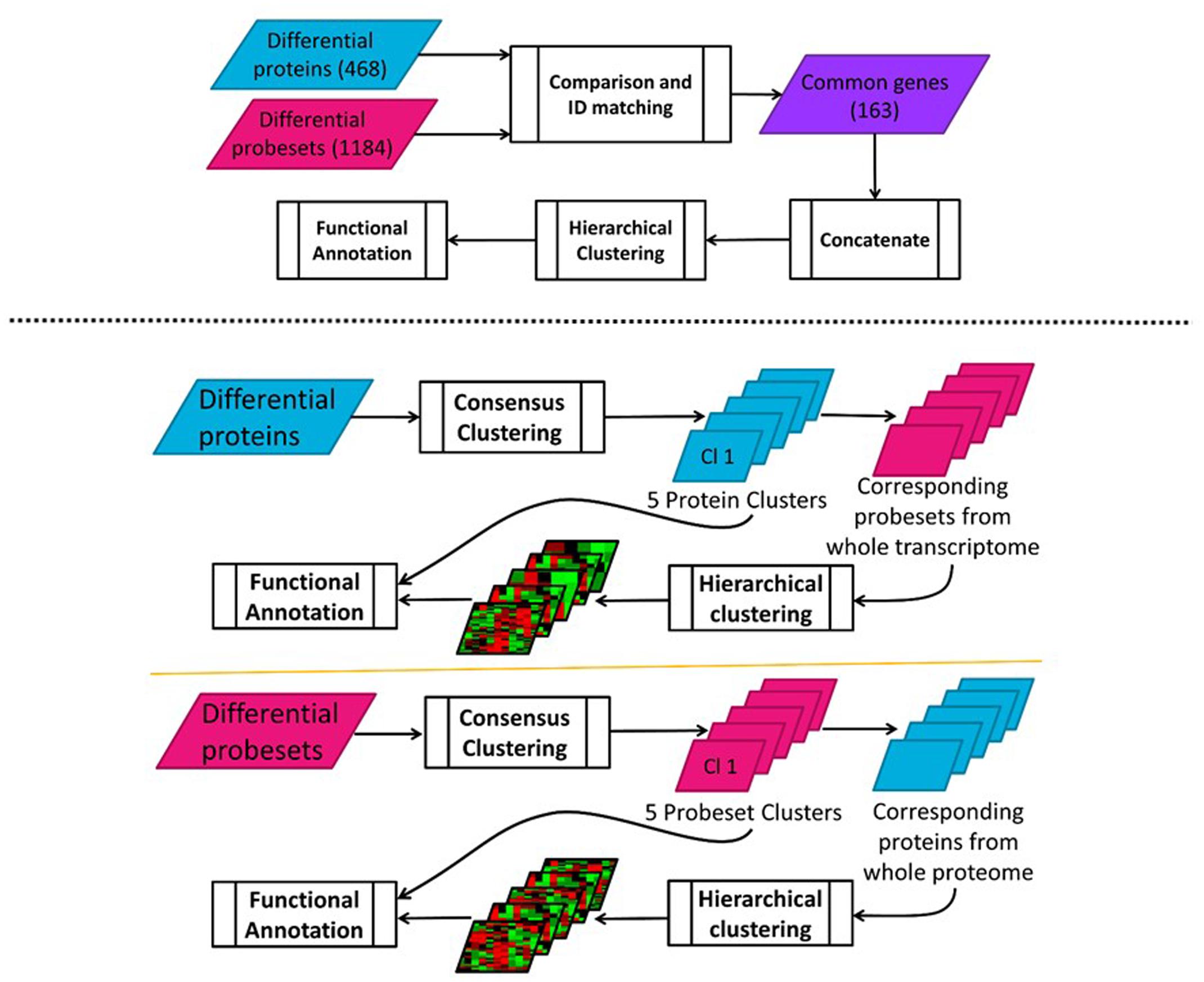
FIGURE 4. (Top) Clustering of concatenated transcriptomic-proteomic MPL data workflow; (Bottom) Sequential transcriptomic and proteomic – forward and reverse – clustering analysis of MPL data workflow.
This study aimed to compare and contrast the transcriptional and translational changes in liver induced by the exposure to a synthetic CS at a pharmacological dose. Although high-throughput -omics analyses have been obtained from samples collected from two independent studies; the strain of experimental animals, dose and type of pharmacologic agent, sampled tissue, sampling procedures, and most of the time points for sample collection were the same for these studies. These conditions allowed us to assume that the experiments are similar enough to conduct individual and integrated bioinformatics analyses.
The preprocessing before performing the first-pass analysis involved identifying the significant genes whose both transcripts and proteins existed in the individual datasets. Differential expression analysis through EDGE identified that 475 out of 959 proteins and 1624 out of around 8800 transcripts had temporal profiles that significantly varied over time (meeting p-value < 0.05 and q-value < 0.01 cut-offs). After this filtering step, both datasets were fed into IPA in order to match distinct identifiers used (Swiss-Prot IDs for proteins and Affymetrix IDs for transcripts). A comparison between two datasets indicated that 163 genes were commonly found in both transcriptomic and proteomic datasets; i.e., both mRNAs and proteins corresponding to these genes were differentially expressed over time.
The analysis is described in greater in detail (Kamisoglu et al., 2015b). However, we briefly report here that this analysis identified two dominant patterns: one with corresponding mRNA and protein expression profiles essentially parallel in direction, and another where the directionality was reversed. The early response appears to be the most critical time period during which mRNA and protein expression profiles change direction. Genes in Cluster 1 display up-regulation for both mRNA and protein expression profiles in the first 8 h, followed by down-regulation, most markedly in the transcriptional profiles. In the second cluster of response profiles, down-regulation dominates for transcriptional profiles; however, corresponding protein expression profiles are not parallel. While down-regulation is observed in these transcripts most notably in the first 8 h; expression of the same proteins seems to be up-regulated in the same time frame. After the 8th hour; both transcriptional and protein expression profiles approach basal levels, though from opposite directions; elevated mRNA levels start to be down-regulated and reduced protein levels start to be up-regulated. Functional annotation of the genes in these two clusters, indicated that the first cluster contains a number of genes coding for heat shock proteins, which take part in the negative regulation of CS signaling through direct protein-protein interaction with glucocorticoid receptor to prevent its translocation to nucleus (Chrousos and Kino, 2005). Complementary transcriptional and proteomic profiles of these genes indicated that this is a negative feedback control induced by MPL delivery, which is regulated at the transcriptional level. Proteins functioning in the regulation of protein degradation and translation machinery were also among the genes in the first cluster, implying that these processes are also controlled at the transcriptional level after CS exposure. In contrast, functions enriched by the genes in the second cluster appear to be regulated at post-transcriptional levels, likely through control of mRNA processing, initiation of protein translation or protein stability, since the transcriptional profiles are not emulated by protein expression, (Waters et al., 2006). Among these functions, most notable are the modulation of oxidative stress, lipid metabolism and bile acid biosynthesis. Concurrent analysis of promoter region identified upstream regulators that can explain the observed changes in gene/protein expression based on the prior knowledge of expected effects between the upstream regulators and target genes/proteins in the dataset.
Two-Way Sequential Clustering of Individual Proteomic and Transcriptomic Datasets
While the hierarchical clustering analysis described above identifies the potential co-regulatory schemes for the genes in the intersection of transcriptomic and proteomic datasets; it fails to capture the dynamics in the rest of the genes which may also show differences in expression over time, although they may not co-exist in both datasets. In order to evaluate the overall dynamic patterns and extract the most useful information integrating these two datasets, a consensus clustering (Nguyen et al., 2009) method was applied to these two datasets separately. First, proteins with differential temporal profiles were clustered using p-values of 0.05 for significant clusters and an agreement level of 0.70 for the genes in each cluster. Then, probe sets corresponding to the proteins in each cluster were identified through the comparison function in IPA as before. Temporal profiles of these probe sets corresponding to the proteins were compiled and separately sub-clustered through the less stringent hierarchical clustering method, again using clustergram function in MATLAB. The reverse of the same procedure was also performed – starting from transcriptional analysis and continuing with the corresponding proteomic analysis. Here, differential transcriptional profiles were first determined and then clustered using the same procedures described above. As with the previous analysis, proteins that correspond to the probe sets within each of these clusters were then identified and sub-clustered. The workflow is illustrated in Figure 4 (bottom). Functional annotations of proteins and transcripts at each level of analysis were conducted in IPA by running a core analysis for each cluster and evaluating the enriched canonical pathways (at p-value threshold of 0.05) and predicted upstream regulators obtained in IPA.
Summarizing the observations described in great detail in (Kamisoglu et al., 2015b) we note that EDGE identified 475 out of 959 while the ensuing consensus clustering revealed five coherent temporal profiles containing 217 of the 475 regulated proteins. Of the 217 clustered proteins, 158 showed regulation of at the mRNA level as well. This analysis was repeated in the reverse direction; i.e., starting from the transcriptomic dataset and progressing to the proteomic dataset. 1624 of the probe sets were differentially expressed, 1132 of those were in five clusters obtained by consensus clustering. Only 217 of these 1132 probe sets had corresponding proteins in the proteomic dataset. Compared to the first part of sequential clustering analysis, considerably fewer proteins actually correlate with the transcriptional profile of their respective clusters. Considering that protein expression is a more reliable predictor of function, the annotation analysis was based on the proteomic data in this part of the analysis. Elaboration on the results enabled a more complete characterization of the functional implications and relations among gene and proteins (Kamisoglu et al., 2015b).
Data-Driven Integration -Omics Data Across Multiple Levels of Physiological Organization
The second study addresses a question of increasing importance. Blood sampling is the most widely utilized way of probing dynamic responses, and it has tremendous translational potential as it is the most readily accessible sample in humans. Metabolomics in particular is becoming a readily available tool given the ability of the serum metabolome to capture biological responses at a higher level (Minami et al., 2009; Fitzpatrick and Young, 2013; Kosmides et al., 2013; Kaddurah-Daouk et al., 2014; Kell and Goodacre, 2014; Carroll et al., 2016). Likely one of the most celebrated examples was the Inflammation and the Host Response to Injury, so-called, “Glue Grant,” aiming at providing a blue-print of the host response to injury and trauma based on sophisticated analysis of blood samples (Nathens et al., 2005; Cuenca et al., 2011; Tompkins, 2015). However, despite the fact that the majority of studies attempting to integrate various types of -omics information, analyses based on blood measurement add an extra level of complexity. Namely, circulating metabolites originate from a wide variety of tissues and organs and eventually accumulate in systemic circulation. Therefore, although the various data structures provide actual information describing the host response, the fact that the origin of each type of information is not unique, as well as non-specific, significantly complicates the analysis. One such example, to be discussed shortly, relates to combining transcriptomic and metabolomics information from blood samples. In this direction, the question we pursued was based on the hypothesis that the drastic changes in the immediate environment of blood leukocytes might have an adaptive effect on shaping their transcriptional response in the regulation of metabolism in conjunction with the initial inflammatory stimuli. The coupling of leukocyte transcriptomic and systemic metabolomics information may enable us to provide a more complete picture of the drivers of the response of immune cells under inflammatory conditions.
Human Endotoxemia
Elective administration of bacterial endotoxin (lipopolysaccharide; LPS) to healthy human subjects has been used as a reproducible experimental procedure providing mechanistic insights into how cells, tissues and organs respond to systemic inflammation. Low doses of LPS transiently alter many physiologic and metabolic processes in a qualitatively similar manner to those observed after acute injury and systemic inflammation (Lowry, 2005; Calvano and Coyle, 2012), thus allowing the analysis of the responses to infectious stress at multiple physiologic levels. This model has been extensively employed for the development and assessment of rational clinical therapies to prevent or attenuate systemic inflammatory response syndrome (SIRS) (Calvano and Coyle, 2012).
Response to endotoxemia is closely associated with alterations in metabolism. Inflammatory processes change the direction of the substrate flow from the periphery toward splanchnic organs while also triggering the release of catabolic signals in order to meet increased energy and substrate demands (Fong et al., 1990; Khovidhunkit et al., 2004); and hence, considerably alter the levels of plasma metabolites. Individual changes in the major metabolites, such as some lipids, amino acids, and glucose, are previously documented for the case of human endotoxemia (Fong et al., 1990). However, an untargeted bioinformatics-empowered approach to elucidate the effects of endotoxemia on plasma metabolite levels is lacking.
Analysis of the complete metabolic response to systemic inflammation is of special interest since metabolic composition of a tissue is uniquely altered in response to stimuli due to collective effects of the regulations at various levels of cellular processes including transcription, translation and signal transduction. Concentrations of metabolites in a sample at a given time, i.e., the “metabolome” (Nicholson and Lindon, 2008), can be thought of as the metabolic fingerprint representative of the state of body at that time and provide information on the dominant regulatory mechanisms. The emerging field of metabonomics, combines this unique metabolic information with bioinformatics approaches to provide an integrated temporal picture of the interactions in the system (Nicholson, 2006; Holmes et al., 2008). Since the metabolic phenotype is determined by eventual production of metabolites through the complex cellular processes trickling down from transcription, translation and signal transduction, this field offers promise in advancing the knowledge in many clinical conditions. For endotoxemia, understanding the alterations in plasma metabolome is critical; since, metabolite levels impacts the regulation of anti-inflammatory defenses, in turn, through steering critical cellular processes in immune cells (Pearce and Pearce, 2013).
Global transcriptomic studies of circulating leukocytes in experimental human endotoxemia previously elucidated the intricate regulatory schemes governing the inflammatory response (Calvano et al., 2005; Nguyen et al., 2011). However, inflammatory response is also closely associated with alterations in metabolism. In Kamisoglu et al. (2013) we discussed the drastic effect of a mild inflammatory stimulus on the homeostasis of the whole-body metabolism. This single level analysis uncovered the temporal patterns in the host metabolism reflecting collective impacts of regulations at various organs and at multiple levels of cellular processes including transcription, translation and signal transduction. For endotoxemia, understanding the alterations in plasma metabolome is critical, since metabolite levels impact the regulation of anti-inflammatory defenses, in turn, by directing critical cellular processes in immune cells (Pearce and Pearce, 2013).
Building on this knowledge; we integrated the transcriptional response of leukocytes with systemic metabolic response to understand how inflammation-induced changes in the composition of plasma, in turn, affecting the transcriptional processes in the leukocytes.
Metabolomic Studies
Archived blood plasma samples, which had been flash frozen, were used in this proof-of-principle study. These samples were collected from 19 healthy subjects, ages 18–40. Fifteen of the subjects (11 males and 4 females; mean age of 22.7) were administered National Institutes of Health (NIH) Clinical Center Reference Endotoxin, at a bolus dose of 2 ng/kg body weight as previously described Alvarez et al. (2007); Jan et al. (2009) and Jan et al. (2010). Four control subjects (three males and one female; mean age of 22.2) were administered placebo (saline). During the protocol, subjects received a solution of 5% dextrose and 0.45% saline crystalloid. Blood draws were conducted sequentially at t = 1, 2, 6, and 24 h from both groups, samples were inventoried and stored at -80°C until the analysis. Metabolomic analysis was performed by Metabolon (Durham, NC, USA) according to previously published methods (Evans et al., 2009). The resulting extracts were subjected to either liquid chromatography (LC) or gas chromatography (GC) followed by mass spectroscopy (MS) analysis. Identification of known chemical entities was based on comparison to metabolomic library entries of purified standards. Complete details of the profiling of plasma metabolome are previously described (Kamisoglu et al., 2013, 2015a).
Transcriptomic Studies
For the transcriptomic study, four subjects (one female and three male) received LPS at a bolus dose of 2 ng/kg body weight and four subjects (one female and three male) received saline. Blood samples were collected before (t = 0 h) and 2, 4, 6, 9, and 24 h after LPS administration. Leukocytes were recovered by centrifugation; total cellular RNA was isolated from the leukocyte pellets and hybridized onto Hu133A and Hu133B oligonucleotide arrays (Affymetrix). Further details about the experimental design are presented in the original analysis (Calvano et al., 2005). The transcriptional analysis generated expression measurement data of over 44000 probe sets in total, which is also publicly available through the GEO Omnibus Database2 under the Accession No: GSE3284. Integration and analysis of transcriptomic/metabolomic data from different tissues. We presented extensive data analysis and modeling associated with the transcriptional response to endotoxin in humans (Foteinou et al., 2009a,b,c, 2010, 2011; Dong et al., 2010; Nguyen et al., 2011, 2013; Yang et al., 2011b; Scheff et al., 2013).
Integration and Analysis of Transcriptomic/Metabolomic Data
Data analysis for both transcriptomic and metabolomic datasets started first by filtering for differential expression over time. Transcripts and metabolites with differential temporal profiles were determined using EDGE software (Leek et al., 2006). The significance cut-off for the transcriptomic dataset were p < 0.05 at 0.10 false discovery rate. To determine the potential co-regulatory relationships, differentially expressed transcripts and metabolites with differential temporal profiles were hierarchically clustered using clustergram function in the Bioinformatics toolbox of MATLAB (Mathworks, Natick, MA, USA). The two clusters were obtained by using correlation as the distance metric.
Pathway enrichment analysis of genes in the clusters were completed in Enrichr (Chen et al., 2013) using the gene-set libraries of Kyoto Encyclopedia of Genes and Genomes (KEGG) (Kanehisa and Goto, 2000). Three types of enrichment scores are calculated by Enrichr to assess the significance of overlap between the input list and the gene sets in each gene-set library for ranking a term’s relevance to the input list. These are Fisher exact test, z-score of the deviation from the expected rank by the Fisher exact test, and a combined score that multiplies the log of the p-value computed with the Fisher exact test by the z-score. The pathways which have a combined score higher than 1.0 were called significant. The combined score was devised because Fisher exact test had a slight bias that affects the ranking of terms solely based on the length of the gene sets in each gene-set library (Chen et al., 2013).
The goal in the current analysis was to reveal transcriptional regulation of leukocyte metabolic processes, specifically, then to assess if these regulatory patterns might have been affected by concurrent fluctuations of metabolite levels in the surrounding plasma along with the initial stimuli. For this purpose, we opted to focus the transcriptional analysis to the genes that are associated with metabolic processes only. Therefore, any differential transcripts which code for genes that are not associated with any of the metabolic pathways were filtered out. Gene set libraries and pathway classifications in KEGG database were used as reference at this filtering process. Then, clustering analysis was repeated for the remaining transcripts. Clustered metabolism-associated genes were functionally annotated through Enrichr similar to the analysis of the complete transcriptome described above. A detailed account of the results is described in length in (Kamisoglu et al., 2013, 2014, 2015a).
The study aimed at defining the impact of altered plasma composition on the transcriptional response of leukocytes during an inflammatory challenge. Earlier transcriptional studies (Calvano et al., 2005) highlighted components of pro- and anti-inflammatory processes, whereas the integrative analysis focused on metabolic processes controlled at the transcriptional level and enabled the development of guiding principles driving the impact of the immediate leukocyte environment.
One of the key observations indicated that leukocytes tune the activity of lipid and protein associated processes at the transcriptional level in accordance with the fluctuations in metabolite compositions of surrounding plasma. A closer look into the transcriptional control of metabolic pathways uncovered alterations in bioenergetics and defenses against oxidative stress closely associated with mitochondrial dysfunction and shifts in energy production observed during inflammatory processes. We observed that in parallel with the peaking lipid and plunging amino acid levels in plasma, lipid associated metabolic pathways were activated while protein translation machinery slowed. We hypothesize that drastic changes in the immediate environment of the leukocytes might have an adaptive effect in this response in conjunction with the initial stimuli. Furthermore, focusing only on metabolism associated transcripts uncovered alterations in bioenergetics and defenses against oxidative stress that can shed light into the mechanisms underlying mitochondrial dysfunction and shifts in energy production observed during inflammatory processes. Besides describing the metabolic response of human body to a basic inflammatory cue at the systemic level together with affected immune mechanisms, this study can inspire future translational studies as the -omics analyses becomes more routine in clinical practice. Blood is one of the most rapid and least invasive biological samples collected from patients, yielding useful information about the state of the body. Benchmarking the metabolic state of the system and transcriptional state of the immune cells by a single biological sample may expedite clinical decision making and help reduce mortality in critical cases. The studies of (Langley et al., 2013, 2014), which formed the basis for our comparative analysis between endotoxemia and sepsis (Kamisoglu et al., 2015a), specifically aimed at benchmarking the metabolic state and identifying critical biomarkers. The analysis presented earlier, based on (Kamisoglu et al., 2015a), points to directions where control studies (human endotoxemia) with established links to clinical cases (sepsis) can be used to elaborate on likely markers and/or expected dynamics enabling easier translation of clinical data.
The integration of multiple temporal data of diverse nature, raises several issues: the temporal resolution of sampling is often assumed. The hypothesis is that if one is interested in deciphering the dynamic interactions between an input and its output, the sampling frequency and timing of the sampling need to be similar. However, data acquisition in living systems reflects a balance between what is physiologically appropriate and what is realistically feasible, accounting not only for cost, but for other practical and ethical constraints. In human studies for example, sampling frequency is limited due to ethical and other constraints. In animal studies, practicality of experiments and their cost often limit sampling, especially if/when it involves sacrificing the animal, in addition to ethical considerations evaluated by the appropriate IRB. Therefore, multiple confounding factors exist, including pre-existing conditions, multiple drugs, age, sex, ethnicity etc. It is the hope that large scale studies will provide some stratification and generate somewhat more coherent data. Furthermore, deciphering evolution of dynamics following an external perturbation needs to take into consideration that the “homeostatic” dynamics may in fact demonstrate a baseline dynamics response – most likely in the form of circadian variation (Almon et al., 2008a,b; Nguyen et al., 2010b, 2014b; Ovacik et al., 2010; Rao et al., 2016b). Therefore, deviations from homeostasis in response to a pharmacological agent need to consider the homeostatic dynamics as well. This is evident not only in terms of data, but also in the context of model development and its implications (Scheff et al., 2010b; Mavroudis et al., 2013, 2015; Pierre et al., 2016; Rao et al., 2016a).
Model-Based Integration of -Omics Data
Modeling the responses of the body to a drug is a fundamental process in the drug development and it helps us quantitatively reflect the time-course of the effects of drug on the body. Building and successful utilization of these models allow quantification of drug-system interactions and prediction of both therapeutic and adverse effects (Mager et al., 2003; Felmlee et al., 2012). Diverse physiological effects of synthetic glucocorticoids are the subject of many of these pharmacokinetic and pharmacodynamic modeling efforts. A series of models were developed to explain the dynamics of receptor regulation and enzyme induction following MPL administration (Sun et al., 1998; Ramakrishnan et al., 2002). The models progressively enhanced to capture the effects of the drug under several doses and dosing regimens. However, these models were based on the data generated by traditional message quantification methods that only allow measurements of single end points. Together with the analysis of MPL effects on various tissues via high-throughput technologies such as gene microarrays (Almon et al., 2007a,b), the diversity of the available models increased. The fifth-generation model that described the simple gene induction by MPL was expanded to several pharmacogenomics models that may explain the response of all the hepatic genes with various dynamic patterns (Jin et al., 2003). Earlier modeling analyses have primarily concentrated on the effects observed at the gene expression level (Almon et al., 2003, 2005, 2007a,b; Jin et al., 2003; Nguyen et al., 2010a, 2014a). The next quest in the development of more comprehensive models is the incorporation of information at the protein expression level. This information, made available by a novel high-throughput and reproducible method, allows the temporal profiling of tissue proteome (Nouri-Nigjeh et al., 2014). A future direction we envision is to achieve integration of this information from complementary studies with a model-driven approach. With this, the current PK/PD models of MPL response could be augmented to reflect the physiological response observed at the protein expression level.
As our results demonstrated earlier, transcriptional and proteomic expression patterns roughly correlate for some of the genes, yet for others, the dynamics are more unexpected. One way to work with the existing PK/PD models would be teasing out the protein counterparts of the transcriptional clusters that are described by the observed dynamics and examining the potential mechanisms that could explain the observed protein expression profiles corresponding to the same genes. Another approach is considering the physiologic response as systems response composed of dynamics of individual elements. However, studies focusing on understanding the relationship between global mRNA transcription and protein translation have produced mixed results, often concluding that the transcriptomic and proteomic data is far from being easily described as complementary (Greenbaum et al., 2003; Hegde et al., 2003; Nicholson et al., 2004; Waters et al., 2006; Haider and Pal, 2013). Nevertheless, both data types reflect the dynamics of the cellular response, thus capture critical information reflecting different facets of the response. The key challenge is realizing that although the various -omics (genomic, transcriptomic and proteomic in this case) components at some elementary level augment the number of descriptors, the augmentation is not passive, i.e., it is not simply increasing the dimensionality of the space.
We will present a preliminary study applying this second approach. In our study, the driver of the response is the drug-receptor complex in the nucleus; transcripts and proteins, the nodes of the network, are the individual elements with diverse dynamics. The observed phenotype reflects the systems response arising from the dynamics of these individual elements, and these elements include genes and proteins, directly and indirectly, affected by the MPL. A number of target genes to be included in the network depends on the available biological data, literature information about the interaction of the nodes, as well as the desired complexity level. As more nodes are added into the network, the direct and indirect interactions between the elements of the network, as well as the number of parameters to be estimated, increases.
Building the Response Network
A functional approach was undertaken to construct a proof-of-concept initial network. Genes included in this network are selected from the most informative transcripts that are both differentially expressed at the transcriptomic and proteomic levels, Figure 5 (left). The list was further reduced by focusing on genes functionally related to the major metabolic effects of MPL on metabolism, including hyperglycemia, dyslipidemia and muscle wasting (Schäcke et al., 2002). Genes functioning in glutathione metabolism and redox regulation, associated with oxidative stress, are situated at the major cross-roads critical for the regulation of both inflammation and metabolism (Pearce and Pearce, 2013). These nodes seem to be closely connected to one of the core genes in pyruvate metabolism, also coding for enzymes catalyzing the rate limiting step of fatty acid synthesis. Another critical response to inflammation, regulation of cytoskeleton, although mostly epithelial in origin, is also represented in this network in collaboration with other genes functioning in metabolic pathways.
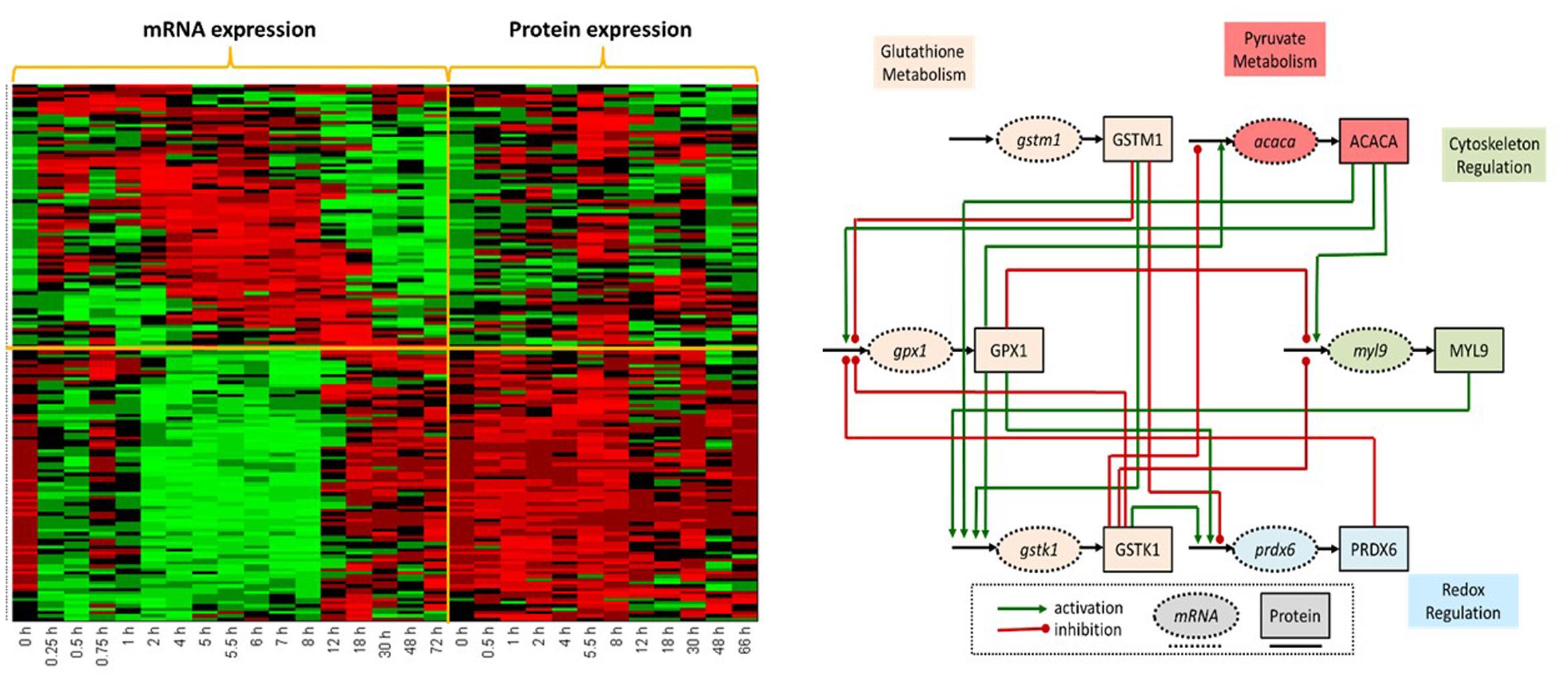
FIGURE 5. (Left) Concurrent analysis of temporal mRNA and protein liver-specific data post MPL dosing revealed two dominant patterns. The first populated with genes whose corresponding mRNA and protein expression profiles were parallel in direction; while in the second directionality was reversed. Heat shock proteins and proteins regulating protein degradation and translation were in the first cluster. The second pattern was dominated by proteins involved in the modulation of oxidative stress, lipid metabolism and bile-acid biosynthesis. Such analyses likely point to transcriptional or post-translational regulatory events. (Right) Liver-specific differentially expressed proteins and transcripts following a dose of MP were determined using EDGE and common functionally enriched pathways using Enrichr. Direct and indirect regulatory relations identified using four databases (Biocarta, KEGG, NCI, and Reactome) established a global network subsequently reduced (6 nodes, 16 edges) using a variation of Dijkstra’s algorithm. Network includes: oxidative stress genes in glutathione metabolism and redox regulation critical for the regulation of inflammation and metabolism; core genes in pyruvate metabolism and fatty acid synthesis; regulation of cytoskeleton as well as metabolic genes.
In order to establish regulatory connections between the sub-set of genes and proteins, we explored several databases, including Ingenuity Pathway Analysis (Calvano et al., 2005), KEGG (Lissauer et al., 2009), Biocarta (Amirian et al., 2011) and PSTIING (Ng et al., 2011), which provide network data. The network was constructed by combining the transcriptomic and proteomic data along with functionally annotated biological information and literature-based functional associations, with emphasis on regulatory relations. Utilizing the regulatory relations between genes/proteins from the databases, first a “global network” in which regulatory links are well known was established. The analysis produced a small, yet complex network (6 nodes, 16 connections) providing an avenue to explore both therapeutic and adverse effects of MPL, Figure 5 (right). Refinement and further extension of the network through modeling will yield an accurate representation of the complete effects of the drug. Once the sub-set of genes and proteins of interest has been identified, we then need to establish a network structure expressing putative regulatory relations. A variety of computational methodologies for further refining regulatory network structures are discussed elsewhere (Yang et al., 2007b; Foteinou et al., 2009d; Nguyen and Androulakis, 2009; Nguyen et al., 2011; Saharidis et al., 2011).
Integrating the Network With an Existing PK/PD Model for MPL
Inference (regression) methods (Fujii et al., 2017) correlate inputs and outputs without prior knowledge of the underlying network structure. Instead we pursued a “reaction-based” approach (Newman et al., 2013) which is an a priori biological knowledge enabling the quantification of the dynamics (Androulakis et al., 2007, 2013) exploring the principles of mass action (Aldridge et al., 2006) and indirect response modeling (Krzyzanski and Jusko, 1998a,b) for expressing the rates of synthesis and degradation of mRNA and protein. The general structure of the model is composed of three sub-units: (a) the PK of MPL describes a bolus injection which has already been described using a two-compartment model and is maintained throughout (Jin et al., 2003); (b) the PD module describes the transduction cascade leading to the formation of the active, nuclear, complex binding to the GRE (Jin et al., 2003); and (c) the module describing the transcription/translation dynamics. For the latter, we model the transcription/translation process as a dynamic system where the basal rate of transcription of mRNA is regulated by the activity of regulating proteins, whereas the translation is proportional to the amount of mRNA. The basic formalism = ks,m fi(P) - kd,m mRNAi;
= ks,P mRNAi - kd,PPi reflects the fundamental dynamics of transcription and translation processes through the use of ordinary differential equations (Meister et al., 2013). The function f(P) reflects the regulatory action of the various proteins on the transcription mRNA. The f(P) formally reflects the likelihood of the regulatory events and its functional form will reflect mechanistic interpretations. In its most general form, and based on thermodynamic arguments, it includes regulatory complexes as well as activation or repression of transcription (Bintu et al., 2005a,b):
The set Sij defines a regulatory complex and the coefficients λij, μij reflect activation and repression constants respectively. Discrete optimization formalisms (Yang et al., 2008b,c; Foteinou et al., 2009d; Saharidis et al., 2011), could also be used in order to augment the network connectivity. In all cases, the basic structure of the network will be as identified via the network analysis, while allowing for the possibility of minor adjustments based on the estimation results. The estimation of the model parameters take appropriate measures of identifiability and uncertainty into consideration in order to achieve robust parameter estimation and is discussed elsewhere (Yang et al., 2011a,b; Wu et al., 2015).
The network structure for Figure 5 along with the corresponding data of the same figure was used to develop a simple model (Equations 1–9).
In this model, the PK and PD of MPL, i.e., the equations for D, Rm, R, DR and DRn, and their corresponding kinetic parameters, are as established earlier in Sukumaran et al. (2011). The kinetics for mRNA follow standard mass action kinetics with a 0th order transcription and 1st order degradation. The transcription, however, is conditionally regulated by MPL and/or other proteins, depending on the network structure expressed through the matrix aij. The dynamics of protein synthesis was assumed in its simplest form (1st order translation and degradation). The normalized data associated with the mRNA and protein levels of the network in Figure 5 were used for estimating the parameters involved in the mRNA and protein dynamics only (the remaining were fixed based on prior deconvolution of the PK/PD model). MPL plasma concentration (D) exhibit a biexponential decline. Following the binding of the drug to the glucocorticoid receptor (DR), this complex translocates into the nucleus [DR(N)] and acts as the driving force for MPL-induced response patterns. Firstly, this effect is observed as inhibition of mRNA expression for the glucocorticoid receptor (Rm), and consecutively the receptor protein (R). DR(N) is also introduced as a stimulatory factor to all of the nodes in the network. Level of mRNA expression is modeled to be controlled by the presence of drug-receptor complex in the nucleus together with indirect interactions between the proteins of the network. Degradation of mRNA, protein translation from mRNA, and protein degradation are all modeled as linear processes.
The initial, motivating, results, Figure 6, are only beginning to scratch the surface and point to directions for improvement, as they should with any iterative model development process. In general, for most network elements early dynamics seem to be represented well compared to later fluctuations in the response. However, significant improvements and refinements are expected. We anticipate the need to develop complex representations, likely requiring precursor and receptor-mediated indirect responses (Sharma et al., 1998; Sharma and Jusko, 1998; Almon et al., 2002; Hazra et al., 2007). It is important to realize that model structure adjustments have the potential to lead testable hypotheses in the laboratory to further elaborate on the actual PK/PD. Given prior experience with both the modeling principles (Wang et al., 2012; Stamatelos et al., 2013; Mavroudis et al., 2014; Mavroudis et al., 2015) as well as the associated parameter estimation of such pharmacology models in terms of structure (Yang et al., 2007a, 2008b,c, 2009a; Foteinou et al., 2009d; Saharidis et al., 2011) and parameter identification (Yang et al., 2011a,b; Wang et al., 2012; Stamatelos et al., 2013; Wu et al., 2015), a reasonable estimation problem can be formulated. Some of these efforts might include introducing time delays to the elements which demonstrate more pronounced dynamics in the later phase of the response. The synthesis of the network mRNAs currently involves linear relationships. Michaelis–Menten kinetics can be introduced to allow self-limiting responses. Finally, the network structure can also be reshaped by eliminating the nodes that are not insightful as well as introducing new nodes that carry important regulatory information about the existing parts of the network. Once the model is matured to fully capture the experimental data, it can be utilized to make predictions about long-term effects, or different delivery kinetics.
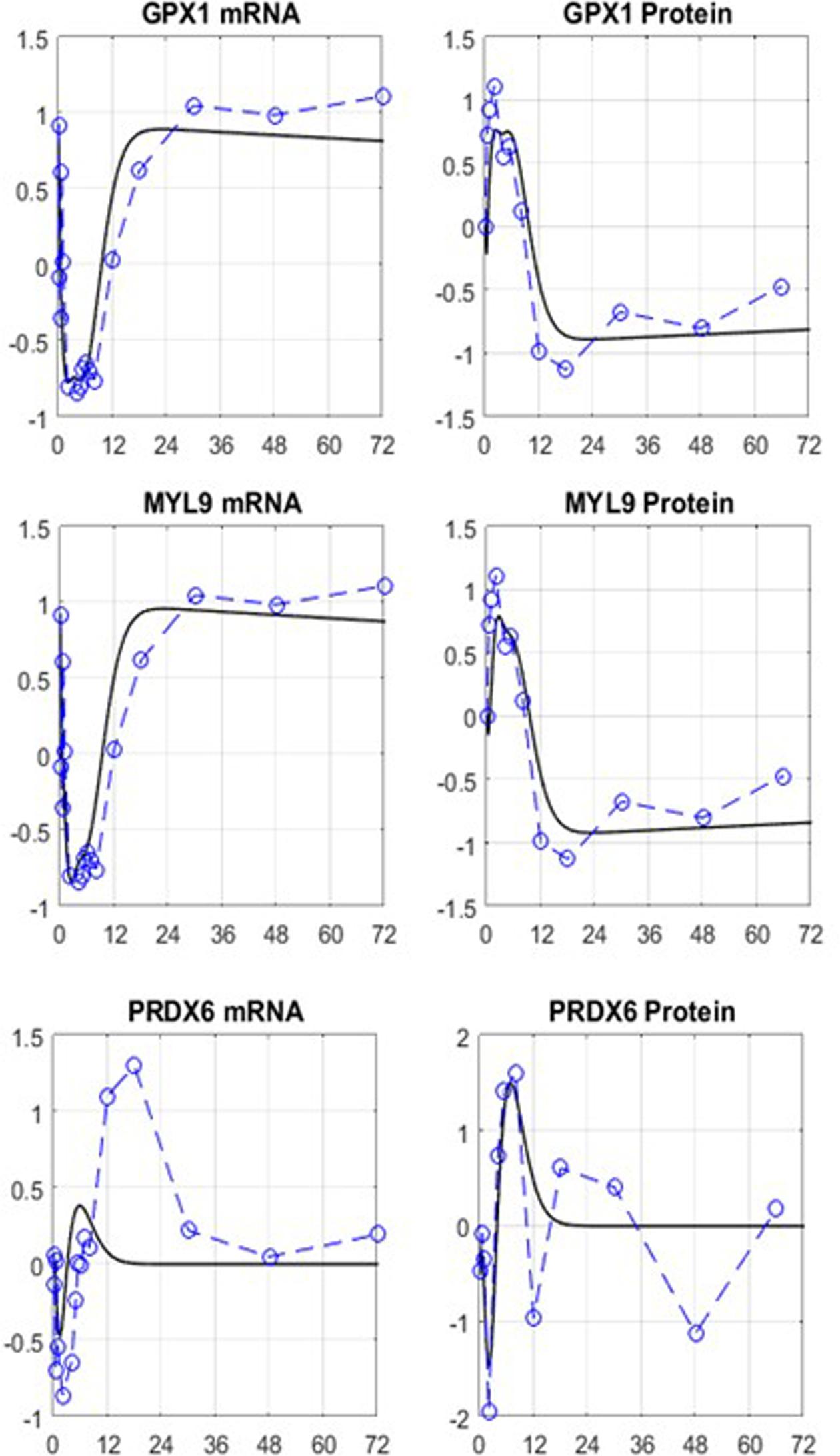
FIGURE 6. Preliminary results indicative of the potential to capture the complex dynamics of the interplay between the PK dynamics of MPL and the putative mRNA/protein cross-regulation in the form of networked interactions. Despite the simplicity of the model equations, key characteristics are captured. The simple form of the added model of regulation clearly needs to be augmented. Furthermore, the preliminary calculations did not take into account the error in measurements. Open symbol/dashed line: data, solid line: model.
Another layer of information that can be incorporated into this model in the future is the metabolome layer. As we have previously pointed out, gene expression signatures give information about the lowest level of organization, shedding light on the origin of a specific phenotype. Proteomics provide information about the abundance of proteins, elucidating the next level up from gene expression data. The integration of these fields provides a unified picture of cellular-level responses from transcription through translation. However, there are multiple other levels of processes that control the sequence of events from the translation of a protein until it becomes a fully functional piece of the organism that can shape processes affecting the metabolic phenotype. Metabolomics complements these more traditional -omics techniques by allowing the investigation of properties that cannot be directly assessed through gene and protein expression. Integration of metabolomics with transcriptomics and proteomics can help make the relationship between the levels of information produced by each technique clearer. Changes in gene expression levels and protein concentrations can be linked to physiologic changes and interpreted in the biological context.
Future metabolomic studies in the same animal model can elicit the metabolic shifts occurring in response to MPL administration that ultimately cause the development of the adverse effects. Careful assessment of the connections of these shifts with the defined alterations in hepatic gene and protein expression levels can help identify the critical nodes that control the metabolism-associated adverse effects of the drug. Importantly, the indirect effects of the drug on whole body metabolism through altering the microbiome would have to be considered here as well, since the symbiotic organisms might have tremendous influence on shaping the metabolic response to the drug. Nevertheless, integration of information from the whole-body metabolism with existing information on the hepatic response to MPL can be useful in multiple ways. Firstly, alterations in the critical nodes that are linked to long-term adverse effects can be identified and adjunct therapies that can alleviate these alterations can be devised. Secondly, patient populations which would be more susceptible to experiencing those adverse effects, or who have better drug response, can be pre-determined based on their genomic profiles. Thirdly, more realistic models of drug response can be designed integrating information from this ultimate phenotypic level and be used to evaluate different scenarios, helping in the design and development of better therapies.
Conclusion
The purpose of this review article is to provide a perspective on the opportunities and challenges associated with the integration of disparate -omics data sources. Without a doubt the next frontier in PK/PD modeling will take advantage of our increasing abilities to incorporate biological information across multiple and diverse layers of physiologic organization. Although the richness of the data is impressive, rationalizing the content in a systematic and coherent manner remains a challenge. Given the overall difficulty of the problem, upgrading the information content of the data is a major challenge.
In this review, we have attempted to discuss three topics, based on our own experience. We discussed challenges associated with integrating -omics information within and between tissues. Interestingly, the nature of the data, to some extent, drives the type of question and the corresponding methods needed for answering the question. Finally, we provided a brief summary of what we feel is a promising new frontier: integrating -omics information in a model-based manner. The expectations are twofold: (1) we may achieve a better rationalization of the information; and (2) perhaps more importantly, we may be able to further advance the frontiers of PK/PD modeling which could have significant impact. This preliminary work introduces an approach for bridging the classic PK/PD modeling efforts with the multi-level systems response. This allows us to explore the paths of utilizing the vast amount of information made available by new -omic profiling tools. These tools make it possible to evaluate the response as a whole at a certain biological level over time. The model-based integration approach discussed here ultimately aims to connect this valuable information coming from multiple layers in a useful framework which reflects the continuity of biological events in response to pharmacological stimuli. Achieving such integration will allow the development of model-based approaches for rationalizing the genomic, transcriptomic and proteomic data in the context of integrated dynamic regulatory network models, critical for the development of MPL PK/PD/pharmacogenomics models, enabling us to move beyond using -omics as a complex descriptor toward the development of pharmacologically relevant and predictive computational models.
Without a doubt, we are only scratching the surface. Numerous challenges and open questions remain, charting an exciting future. To name a few, our case studies present a modeler’s idealized scenario: relatively homogenous cohorts, whether a single animal strain in our MPL studies or a population of relatively healthy humans in our human endotoxemia studies; single dosing with a single pharmaceutical agent. Hidden within is reasonable biological variability; however the main focus of these designs was to tease the agent’s primary effects. As we consider moving integrative -omics to the next level, we must expect a number of challenges: (1) as we hinted in the comparison of metabolomics profiles between a controlled human endotoxemia study and the clinical cases, -omics data from clinical studies and/or patient population will, unavoidably, express and capture many confounding factors beyond responses elicited by the agent under study; (2) circulating, i.e., systemic, markers pose, as discussed in the manuscript, additional challenges since the tissue-specificity is lost, complicating further the interpretation of the observed responses in a cause-and-effect sense; (3) the temporal granularity of the data will remain a key challenge. The disparities in temporal resolution of the responses at different physiologic levels will further complicate any data driven-approach. In such cases, it is likely that methods aiming at features and/or models – as discussed in the paper – will prove more beneficial; (4) patient history, including medication, will constantly nuance the data obtained; (5) despite the ability to probe an ever increasing number of likely biological descriptors and mediators (genome, transcriptome, proteome, metabolome, epigenome, fluxome, etc.) leading to an increase in the dimensionality of the “input” space, the actual “output” space, that is the number of subjects, volunteers and/or patients, being sampled will always lack, especially if appropriate population stratifications are implemented. This is a classic problem in machine learning often referred to as classification/feature selection in “almost empty spaces” (Duin, 2000) Therefore, key challenges will remain, primarily focusing on the many aspects of the heterogeneous nature of the data. However, as our ability to collect, archive, annotate and query new challenges and opportunities emerges (Toga et al., 2015; Dinov et al., 2016), large cohort studies are already emerging (Hood and Price, 2015).
Finally, one of the key themes of this presentation is that integration can occur at two levels: (a) the level of the features of the data, or (b) the level of the features of the models that could describe the dynamics of the data. Each method offers distinct advantages and challenges. Considering features of the data, characteristics of the responses and likely important biomarkers are able to describe intricacies of the response. Features of the models underlying the dynamics of the data, on the other hand, enable a likely quantification of cause-and-effect relations, as well as the likelihood of expressing, and predicting, complex dynamics and emergent behaviors, not necessarily obvious while studying the features themselves. However, it is important to realize that the approaches are complimentary and are often combined to improve the overall effectiveness of the analysis. To some extent, effectiveness is also an emergent property of the concurrent and multi-prong analysis of information-rich -omics data.
Author Contributions
KK, AA, and TN: performed calculations, conducted analyses and edited the manuscript. RA, SC, SC, DD, and WJ: edited the manuscript. IA: conceived the studies, and developed the manuscript.
Funding
KK, TN, RA, SC, SC, DD, WJ, and IA acknowledge funding from GM082974, GM34695 and GM24211. AA acknowledges support from the National Institute of General Medical Sciences of the National Institutes of Health (T32 GM008339).
Conflict of Interest Statement
The authors declare that the research was conducted in the absence of any commercial or financial relationships that could be construed as a potential conflict of interest.
Footnotes
References
Aldridge, B. B., Burke, J. M., Lauffenburger, D. A., and Sorger, P. K. (2006). Physicochemical modelling of cell signalling pathways. Nat. Cell Biol. 8, 1195–1203. doi: 10.1038/ncb1497
Almon, R. R., DuBois, D. C., Brandenburg, E. H., Shi, W., Zhang, S., Straubinger, R. M., et al. (2002). Pharmacodynamics and pharmacogenomics of diverse receptor-mediated effects of methylprednisolone in rats using microarray analysis. J. Pharmacokinet. Pharmacodyn. 29, 103–129. doi: 10.1023/A:1019762323576
Almon, R. R., DuBois, D. C., and Jusko, W. J. (2007a). A microarray analysis of the temporal response of liver to methylprednisolone: a comparative analysis of two dosing regimens. Endocrinology 148, 2209–2225. doi: 10.1210/en.2006-0790
Almon, R. R., DuBois, D. C., Pearson, K. E., Stephan, D. A., and Jusko, W. J. (2003). Gene arrays and temporal patterns of drug response: corticosteroid effects on rat liver. Funct. Integr. Genomics 3, 171–179. doi: 10.1007/s10142-003-0090-x
Almon, R. R., DuBois, D. C., Yao, Z., Hoffman, E. P., Ghimbovschi, S., and Jusko, W. J. (2007b). Microarray analysis of the temporal response of skeletal muscle to methylprednisolone: comparative analysis of two dosing regimens. Physiol. Genomics 30, 282–299. doi: 10.1152/physiolgenomics.00242.2006
Almon, R. R., Lai, W., DuBois, D. C., and Jusko, W. J. (2005). Corticosteroid-regulated genes in rat kidney: mining time series array data. Am. J. Physiol. Endocrinol. Metab. 289, E870–E882. doi: 10.1152/ajpendo.00196.2005
Almon, R. R., Yang, E., Lai, W., Androulakis, I. P., DuBois, D. C., and Jusko, W. J. (2008a). Circadian variations in rat liver gene expression: relationships to drug actions. J. Pharmacol. Exp. Ther. 326, 700–716. doi: 10.1124/jpet.108.140186
Almon, R. R., Yang, E., Lai, W., Androulakis, I. P., Ghimbovschi, S., Hoffman, E. P., et al. (2008b). Relationships between circadian rhythms and modulation of gene expression by glucocorticoids in skeletal muscle. Am. J. Physiol. Regul. Integr. Comp. Physiol. 295, R1031–R1047. doi: 10.1152/ajpregu.90399.2008
Althani, A. A., Marei, H. E., Hamdi, W. S., Nasrallah, G. K., El Zowalaty, M. E., Al Khodor, S., et al. (2016). Human microbiome and its association with health and diseases. J. Cell. Physiol. 231, 1688–1694. doi: 10.1002/jcp.25284
Alvarez, S. M., Katsamanis Karavidas, M., Coyle, S. M., Lu, S. E., Macor, M., Oikawa, L. O., et al. (2007). Low-dose steroid alters in vivo endotoxin-induced systemic inflammation but does not influence autonomic dysfunction. J. Endotoxin. Res. 13, 358–368. doi: 10.1177/0968051907086465
Amirian, E. S., Scheurer, M. E., Liu, Y., D’Amelio, A. M., Houlston, R. S., Etzel, C. J., et al. (2011). A Novel Approach to Exploring Potential Interactions among Single-Nucleotide Polymorphisms of Inflammation Genes in Gliomagenesis: An Exploratory Case-Only Study. Cancer Epidemiol. Biomarkers Prev. 20, 1683–1689. doi: 10.1158/1055-9965.epi-11-0203
Andrews, R. C., and Walker, B. R. (1999). Glucocorticoids and insulin resistance: old hormones, new targets. Clin. Sci. 96, 513–523. doi: 10.1042/cs0960513
Androulakis, I. P. (2015). Systems engineering meets quantitative systems pharmacology: from low-level targets to engaging the host defenses. Wiley Interdiscip. Rev. Syst. Biol. Med. 7, 101–112. doi: 10.1002/wsbm.1294
Androulakis, I. P. (2016). Quantitative systems pharmacology: a framework for context. Curr. Pharmacol. Rep. 2, 152–160. doi: 10.1007/s40495-016-0058-x
Androulakis, I. P., Kamisoglu, K., and Mattick, J. S. (2013). Topology and dynamics of signaling networks: in search of transcriptional control of the inflammatory response. Annu. Rev. Biomed. Eng. 15, 1–28. doi: 10.1146/annurev-bioeng-071812-152425
Androulakis, I. P., Yang, E., and Almon, R. R. (2007). Analysis of time-series gene expression data: methods, challenges, and opportunities. Annu. Rev. Biomed. Eng. 9, 205–228. doi: 10.1146/annurev.bioeng.9.060906.151904
Barnes, P. J. (1998). Anti-inflammatory actions of glucocorticoids: molecular mechanisms. Clin. Sci. 94, 557–572. doi: 10.1042/cs0940557
Bintu, L., Buchler, N. E., Garcia, H. G., Gerland, U., Hwa, T., Kondev, J., et al. (2005a). Transcriptional regulation by the numbers: applications. Curr. Opin. Genet. Dev. 15, 125–135. doi: 10.1016/j.gde.2005.02.006
Bintu, L., Buchler, N. E., Garcia, H. G., Gerland, U., Hwa, T., Kondev, J., et al. (2005b). Transcriptional regulation by the numbers: models. Curr. Opin. Genet. Dev. 15, 116–124. doi: 10.1016/j.gde.2005.02.007
Bone, R. C. (1996). Immunologic dissonance: a continuing evolution in our understanding of the systemic inflammatory response syndrome (SIRS) and the multiple organ dysfunction syndrome (MODS). Ann. Intern. Med. 125, 680–687. doi: 10.7326/0003-4819-125-8-199610150-00009
Calvano, S. E., Xiao, W., Richards, D. R., Felciano, R. M., Baker, H. V., Cho, R. J., et al. (2005). A network-based analysis of systemic inflammation in humans. Nature 437, 1032–1037. doi: 10.1038/nature03985
Calvano, S. E., and Coyle, S. M. (2012). Experimental human endotoxemia: a model of the systemic inflammatory response syndrome? Surg. Infect. 13, 293–299. doi: 10.1089/sur.2012.155
Carroll, A. J., Salek, R. M., Arita, M., Kopka, J., and Walther, D. (2016). Metabolome informatics and statistics: current state and emerging trends. Front. Bioeng. Biotechnol. 4:63. doi: 10.3389/fbioe.2016.00063
Castiglione, F., Pappalardo, F., Bianca, C., Russo, G., and Motta, S. (2014). Modeling biology spanning different scales: an open challenge. Biomed Res. Int. 2014:902545. doi: 10.1155/2014/902545
Chen, E. Y., Tan, C. M., Kou, Y., Duan, Q., Wang, Z., Meirelles, G. V., et al. (2013). Enrichr: interactive and collaborative HTML5 gene list enrichment analysis tool. BMC Bioinformatics 14:128. doi: 10.1186/1471-2105-14-128
Chen, T., He, H. L., and Church, G. M. (1999). Modeling gene expression with differential equations. Pac. Symp. Biocomput. 29–40.
Cheng, C., Andrews, E., Yan, K.-K., Ung, M., Wang, D., and Gerstein, M. (2015). An approach for determining and measuring network hierarchy applied to comparing the phosphorylome and the regulome. Genome Biol. 16, 1–15. doi: 10.1186/s13059-015-0624-2
Chrousos, G. P., and Kino, T. (2005). Intracellular glucocorticoid signaling: a formerly simple system turns stochastic. Sci. STKE 2005:pe48. doi: 10.1126/stke.3042005pe48
Cuenca, A. G., Maier, R. V., Cuschieri, J., Moore, E. E., Moldawer, L. L., Tompkins, R. G., et al. (2011). The Glue Grant experience: characterizing the post injury genomic response. Eur. J. Trauma Emerg. Surg. 37, 549–558. doi: 10.1007/s00068-011-0148-8
Dinov, I. D., Heavner, B., Tang, M., Glusman, G., Chard, K., Darcy, M., et al. (2016). Predictive big data analytics: a study of Parkinson’s disease using large, complex, heterogeneous, incongruent, multi-source and incomplete observations. PLoS ONE 11:e0157077. doi: 10.1371/journal.pone.0157077
Dong, X., Foteinou, P. T., Calvano, S. E., Lowry, S. F., and Androulakis, I. P. (2010). Agent-based modeling of endotoxin-induced acute inflammatory response in human blood leukocytes. PLoS ONE 5:e9249. doi: 10.1371/journal.pone.0009249
Duin, R. P. W. (2000). “Classifiers in almost empty spaces,” in Proceedings of the 15th International Conference on Pattern Recognition, Vol. 2, Barcelona, 1–7.
Evans, A. M., DeHaven, C. D., Barrett, T., Mitchell, M., and Milgram, E. (2009). Integrated, nontargeted ultrahigh performance liquid chromatography/electrospray ionization tandem mass spectrometry platform for the identification and relative quantification of the small-molecule complement of biological systems. Anal. Chem. 81, 6656–6667. doi: 10.1021/ac901536h
Felmlee, M. A., Morris, M. E., and Mager, D. E. (2012). Mechanism-based pharmacodynamic modeling. Methods Mol. Biol. 929, 583–600. doi: 10.1007/978-1-62703-050-2_21
Fitzpatrick, M., and Young, S. P. (2013). Metabolomics–a novel window into inflammatory disease. Swiss Med. Wkly. 143:w13743. doi: 10.4414/smw.2013.13743
Fong, Y. M., Marano, M. A., Moldawer, L. L., Wei, H., Calvano, S. E., Kenney, J. S., et al. (1990). The acute splanchnic and peripheral tissue metabolic response to endotoxin in humans. J. Clin. Invest. 85, 1896–1904. doi: 10.1172/JCI114651
Foteinou, P. T., Calvano, S. E., Lowry, S. F., and Androulakis, I. P. (2009a). In silico simulation of corticosteroids effect on an NFkB- dependent physicochemical model of systemic inflammation. PLoS ONE 4:e4706. doi: 10.1371/journal.pone.0004706
Foteinou, P. T., Calvano, S. E., Lowry, S. F., and Androulakis, I. P. (2009b). Modeling endotoxin-induced systemic inflammation using an indirect response approach. Math. Biosci. 217, 27–42. doi: 10.1016/j.mbs.2008.09.003
Foteinou, P. T., Calvano, S. E., Lowry, S. F., and Androulakis, I. P. (2009c). Translational potential of systems-based models of inflammation. Clin. Transl. Sci. 2, 85–89. doi: 10.1111/j.1752-8062.2008.00051.x
Foteinou, P. T., Calvano, S. E., Lowry, S. F., and Androulakis, I. P. (2010). Multiscale model for the assessment of autonomic dysfunction in human endotoxemia. Physiol. Genomics 42, 5–19. doi: 10.1152/physiolgenomics.00184.2009
Foteinou, P. T., Calvano, S. E., Lowry, S. F., and Androulakis, I. P. (2011). A physiological model for autonomic heart rate regulation in human endotoxemia. Shock 35, 229–239. doi: 10.1097/SHK.0b013e318200032b
Foteinou, P. T., Yang, E., Saharidis, G. K., Ierapetritou, M. G., and Androulakis, I. P. (2009d). A mixed-integer optimization framework for the synthesis and analysis of regulatory networks. J. Glob. Optim. 43, 263–276. doi: 10.1007/s10898-007-9266-6
Fujii, C., Kuwahara, H., Yu, G., Guo, L., and Gao, X. (2017). Learning gene regulatory networks from gene expression data using weighted consensus. Neurocomputing 220, 23–33. doi: 10.1016/j.neucom.2016.02.087
Gligorijevic, V., Malod-Dognin, N., and Przulj, N. (2016). Integrative methods for analyzing big data in precision medicine. Proteomics 16, 741–758. doi: 10.1002/pmic.201500396
Gomez-Cabrero, D., Abugessaisa, I., Maier, D., Teschendorff, A., Merkenschlager, M., Gisel, A., et al. (2014). Data integration in the era of omics: current and future challenges. BMC Syst. Biol. 8(Suppl. 2):I1. doi: 10.1186/1752-0509-8-S2-I1
Greenbaum, D., Colangelo, C., Williams, K., and Gerstein, M. (2003). Comparing protein abundance and mRNA expression levels on a genomic scale. Genome Biol. 4, 117. doi: 10.1186/gb-2003-4-9-117
Haider, S., and Pal, R. (2013). Integrated analysis of transcriptomic and proteomic data. Curr. Genomics 14, 91–110. doi: 10.2174/1389202911314020003
Hazra, A., Pyszczynski, N., DuBois, D. C., Almon, R. R., and Jusko, W. J. (2007). Modeling receptor/gene-mediated effects of corticosteroids on hepatic tyrosine aminotransferase dynamics in rats: dual regulation by endogenous and exogenous corticosteroids. J. Pharmacokinet. Pharmacodyn. 34, 643–667. doi: 10.1007/s10928-007-9063-3
Hegde, P. S., White, I. R., and Debouck, C. (2003). Interplay of transcriptomics and proteomics. Curr. Opin. Biotechnol. 14, 647–651. doi: 10.1016/j.copbio.2003.10.006
Holmes, E., Wilson, I. D., and Nicholson, J. K. (2008). Metabolic phenotyping in health and disease. Cell 134, 714–717. doi: 10.1016/j.cell.2008.08.026
Hood, L., and Price, N. D. (2015). promoting wellness and demystifying disease: the 100k wellness project. Climincal Omics 1, 20–21. doi: 10.1089/clinomi.01.03.07
Hyduke, D. R., Lewis, N. E., and Palsson, B. O. (2013). Analysis of omics data with genome-scale models of metabolism. Mol. Biosyst. 9, 167–174. doi: 10.1039/C2MB25453K
Iyengar, R., Zhao, S., Chung, S. W., Mager, D. E., and Gallo, J. M. (2012). Merging systems biology with pharmacodynamics. Sci. Transl. Med. 4:126s127. doi: 10.1126/scitranslmed.3003563
Jan, B. U., Coyle, S. M., Macor, M. A., Reddell, M., Calvano, S. E., and Lowry, S. F. (2010). Relationship of basal heart rate variability to in vivo cytokine responses after endotoxin exposure. Shock 33, 363–368. doi: 10.1097/SHK.0b013e3181b66bf4
Jan, B. U., Coyle, S. M., Oikawa, L. O., Lu, S. E., Calvano, S. E., Lehrer, P. M., et al. (2009). Influence of acute epinephrine infusion on endotoxin-induced parameters of heart rate variability: a randomized controlled trial. Ann. Surg. 249, 750–756. doi: 10.1097/SLA.0b013e3181a40193
Jin, J. Y., Almon, R. R., DuBois, D. C., and Jusko, W. J. (2003). Modeling of corticosteroid pharmacogenomics in rat liver using gene microarrays. J. Pharmacol. Exp. Ther. 307, 93–109. doi: 10.1124/jpet.103.053256
Joyce, A. R., and Palsson, B. O. (2006). The model organism as a system: integrating ‘omics’ data sets. Nat. Rev. Mol. Cell Biol. 7, 198–210. doi: 10.1038/nrm1857
Jusko, W. J. (2013). Moving from basic toward systems pharmacodynamic models. J. Pharm. Sci. 102, 2930–2940. doi: 10.1002/jps.23590
Kaddurah-Daouk, R., Weinshilboum, R. M., and Pharmacometabolomics Research, N. (2014). Pharmacometabolomics: implications for clinical pharmacology and systems pharmacology. Clin. Pharmacol. Ther. 95, 154–167. doi: 10.1038/clpt.2013.217
Kamisoglu, K., Calvano, S. E., Coyle, S. M., Corbett, S. A., and Androulakis, I. P. (2014). Integrated transcriptional and metabolic profiling in human endotoxemia. Shock 42, 499–508. doi: 10.1097/SHK.0000000000000248
Kamisoglu, K., Haimovich, B., Calvano, S. E., Coyle, S. M., Corbett, S. A., Langley, R. J., et al. (2015a). Human metabolic response to systemic inflammation: assessment of the concordance between experimental endotoxemia and clinical cases of sepsis/SIRS. Crit. Care 19:71. doi: 10.1186/s13054-015-0783-2
Kamisoglu, K., Sukumaran, S., Nouri-Nigjeh, E., Tu, C., Li, J., Shen, X., et al. (2015b). Tandem analysis of transcriptome and proteome changes after a single dose of corticosteroid: a systems approach to liver function in pharmacogenomics. OMICS 19, 80–91. doi: 10.1089/omi.2014.0130
Kamisoglu, K., Sleight, K. E., Calvano, S. E., Coyle, S. M., Corbett, S. A., and Androulakis, I. P. (2013). Temporal metabolic profiling of plasma during endotoxemia in humans. Shock 40, 519–526. doi: 10.1097/SHK.0000000000000063
Kanehisa, M., and Goto, S. (2000). KEGG: kyoto encyclopedia of genes and genomes. Nucleic Acids Res. 28, 27–30. doi: 10.1093/nar/28.1.27
Karagoz, K., Sevimoglu, T., and Arga, K. Y. (2016). Integration of multiple biological features yields high confidence human protein interactome. J. Theor. Biol. 403, 85–96. doi: 10.1016/j.jtbi.2016.05.020
Kell, D. B., and Goodacre, R. (2014). Metabolomics and systems pharmacology: why and how to model the human metabolic network for drug discovery. Drug Discov. Today 19, 171–182. doi: 10.1016/j.drudis.2013.07.014
Khovidhunkit, W., Kim, M. S., Memon, R. A., Shigenaga, J. K., Moser, A. H., Feingold, K. R., et al. (2004). Effects of infection and inflammation on lipid and lipoprotein metabolism: mechanisms and consequences to the host. J. Lipid Res. 45, 1169–1196. doi: 10.1194/jlr.R300019-JLR200
Koch, L. (2016). Bioinformatics: taking the epigenome to another dimension. Nat. Rev. Genet. 17, 255. doi: 10.1038/nrg.2016.44
Kosmides, A. K., Kamisoglu, K., Calvano, S. E., Corbett, S. A., and Androulakis, I. P. (2013). Metabolomic fingerprinting: challenges and opportunities. Crit. Rev. Biomed. Eng. 41, 205–221. doi: 10.1615/CritRevBiomedEng.2013007736
Kritikou, E., Pulverer, B., and Heinrichs, A. (2006). All systems go! Nat. Rev. Mol. Cell Biol. 7, 801–801. doi: 10.1038/nrm2056
Krzyzanski, W., and Jusko, W. J. (1998a). Integrated functions for four basic models of indirect pharmacodynamic response. J. Pharm. Sci. 87, 67–72. doi: 10.1021/js970168r
Krzyzanski, W., and Jusko, W. J. (1998b). Mathematical formalism and characteristics of four basic models of indirect pharmacodynamic responses for drug infusions. J. Pharmacokinet. Biopharm. 26, 385–408.
Langley, R. J., Tipper, J. L., Bruse, S., Baron, R. M., Tsalik, E. L., Huntley, J., et al. (2014). Integrative “omic” analysis of experimental bacteremia identifies a metabolic signature that distinguishes human sepsis from systemic inflammatory response syndromes. Am. J. Respir. Crit. Care Med. 190, 445–455. doi: 10.1164/rccm.201404-0624OC
Langley, R. J., Tsalik, E. L., van Velkinburgh, J. C., Glickman, S. W., Rice, B. J., Wang, C., et al. (2013). An integrated clinico-metabolomic model improves prediction of death in sepsis. Sci. Transl. Med. 5, 195ra195. doi: 10.1126/scitranslmed.3005893
Laroux, F. S. (2004). Mechanisms of inflammation: the good, the bad and the ugly. Front. Biosci. 9:3156–3162. doi: 10.2741/1468
Leek, J. T., Monsen, E., Dabney, A. R., and Storey, J. D. (2006). EDGE: extraction and analysis of differential gene expression. Bioinformatics 22, 507–508. doi: 10.1093/bioinformatics/btk005
Lissauer, M. E., Johnson, S. B., Bochicchio, G. V., Feild, C. J., Cross, A. S., Hasday, J. D., et al. (2009). Differential expression of toll-like receptor genes: sepsis compared with sterile inflammation 1 day before sepsis diagnosis. Shock 31, 238–244. doi: 10.1097/SHK.0b013e3181834991
Lowry, S. F. (2005). Human endotoxemia: a model for mechanistic insight and therapeutic targeting. Shock 24(Suppl. 1), 94–100. doi: 10.1097/01.shk.0000191340.23907.a1
Mager, D. E., Wyska, E., and Jusko, W. J. (2003). Diversity of mechanism-based pharmacodynamic models. Drug Metab. Dispos. 31, 510–518. doi: 10.1124/dmd.31.5.510
Mavroudis, P. D., Corbett, S. A., Calvano, S. E., and Androulakis, I. P. (2014). Mathematical modeling of light mediated HPA axis activity and downstream implications on the entrainment of peripheral clock genes. Physiol. Genomics 46, 766–778. doi: 10.1152/physiolgenomics.00026.2014
Mavroudis, P. D., Corbett, S. A., Calvano, S. E., and Androulakis, I. P. (2015). Circadian characteristics of permissive and suppressive effects of cortisol and their role in homeostasis and the acute inflammatory response. Math. Biosci. 260, 54–64. doi: 10.1016/j.mbs.2014.10.006
Mavroudis, P. D., Scheff, J. D., Calvano, S. E., and Androulakis, I. P. (2013). Systems biology of circadian-immune interactions. J. Innate Immun. 5, 153–162. doi: 10.1159/000342427
Meister, A., Li, Y. H., Choi, B., and Wong, W. H. (2013). Learning a nonlinear dynamical system model of gene regulation: a perturbed steady-state approach. Ann. Appl. Stat. 7, 1311–1333. doi: 10.1214/13-Aoas645
Meng, C., Kuster, B., Culhane, A. C., and Gholami, A. M. (2014). A multivariate approach to the integration of multi-omics datasets. BMC Bioinformatics 15:162. doi: 10.1186/1471-2105-15-162
Minami, Y., Kasukawa, T., Kakazu, Y., Iigo, M., Sugimoto, M., Ikeda, S., et al. (2009). Measurement of internal body time by blood metabolomics. Proc. Natl. Acad. Sci. U.S.A. 106, 9890–9895. doi: 10.1073/pnas.0900617106
Morand, E. F., and Leech, M. (1999). Glucocorticoid regulation of inflammation: the plot thickens. Inflamm. Res. 48, 557–560. doi: 10.1007/s000110050503
Motta, S., and Pappalardo, F. (2013). Mathematical modeling of biological systems. Brief. Bioinform. 14, 411–422. doi: 10.1093/bib/bbs061
Nathens, A. B., Johnson, J. L., Minei, J. P., Moore, E. E., Shapiro, M., Bankey, P., et al. (2005). Inflammation and the Host Response to Injury, a large-scale collaborative project: patient-oriented research core–standard operating procedures for clinical care. I. Guidelines for mechanical ventilation of the trauma patient. J. Trauma 59, 764–769.
Newman, R. H., Hu, J., Rho, H. S., Xie, Z., Woodard, C., and Neiswinger, J. (2013). Construction of human activity-based phosphorylation networks. Mol. Syst. Biol. 9:655. doi: 10.1038/msb.2013.12
Ng, A., Bursteinas, B., Gao, Q., Mollison, E., and Zvelebil, M. (2011). pSTIING: a ‘systems’ approach towards integrating signalling pathways, interaction and transcriptional regulatory networks in inflammation and cancer. Nucleic Acids Res. 34(Suppl. 1), D527–D534. doi: 10.1093/nar/gkj044
Nguyen, T. T., and Androulakis, I. P. (2009). Recent advances in the computational discovery of transcription factor binding sites. Algorithms 2, 582–605. doi: 10.3390/a2010582
Nguyen, T. T., Almon, R. R., DuBois, D. C., Jusko, W. J., and Androulakis, I. P. (2010a). Comparative analysis of acute and chronic corticosteroid pharmacogenomic effects in rat liver: transcriptional dynamics and regulatory structures. BMC Bioinformatics 11:515. doi: 10.1186/1471-2105-11-515
Nguyen, T. T., Almon, R. R., DuBois, D. C., Jusko, W. J., and Androulakis, I. P. (2010b). Importance of replication in analyzing time-series gene expression data: corticosteroid dynamics and circadian patterns in rat liver. BMC Bioinformatics 11:279. doi: 10.1186/1471-2105-11-279
Nguyen, T. T., Almon, R. R., DuBois, D. C., Sukumaran, S., Jusko, W. J., and Androulakis, I. P. (2014a). Tissue-specific gene expression and regulation in liver and muscle following chronic corticosteroid administration. Gene Regul. Syst. Biol. 8, 75–87. doi: 10.4137/GRSB.S13134
Nguyen, T. T., Calvano, S. E., Lowry, S. F., and Androulakis, I. P. (2013). An agent-based model of cellular dynamics and circadian variability in human endotoxemia. PLoS ONE 8:e55550. doi: 10.1371/journal.pone.0055550
Nguyen, T. T., Foteinou, P. T., Calvano, S. E., Lowry, S. F., and Androulakis, I. P. (2011). Computational identification of transcriptional regulators in human endotoxemia. PLoS ONE 6:e18889. doi: 10.1371/journal.pone.0018889
Nguyen, T. T., Mattick, J. S., Yang, Q., Orman, M. A., Ierapetritou, M. G., Berthiaume, F., et al. (2014b). Bioinformatics analysis of transcriptional regulation of circadian genes in rat liver. BMC Bioinformatics 15:83. doi: 10.1186/1471-2105-15-83
Nguyen, T. T., Nowakowski, R. S., and Androulakis, I. P. (2009). Unsupervised selection of highly coexpressed and noncoexpressed genes using a consensus clustering approach. OMICS 13, 219–237. doi: 10.1089/omi.2008.0074
Nicholson, J. K. (2006). Global systems biology, personalized medicine and molecular epidemiology. Mol. Syst. Biol. 2:52. doi: 10.1038/msb4100095
Nicholson, J. K., Holmes, E., Lindon, J. C., and Wilson, I. D. (2004). The challenges of modeling mammalian biocomplexity. Nat. Biotechnol. 22, 1268–1274. doi: 10.1038/nbt1015
Nicholson, J. K., and Lindon, J. C. (2008). Systems biology: metabonomics. Nature 455, 1054–1056. doi: 10.1038/4551054a
Nouri-Nigjeh, E., Sukumaran, S., Tu, C., Li, J., Shen, X., Duan, X., et al. (2014). Highly multiplexed and reproducible ion-current-based strategy for large-scale quantitative proteomics and the application to protein expression dynamics induced by methylprednisolone in 60 rats. Anal. Chem. 86, 8149–8157. doi: 10.1021/ac501380s
Oakley, R. H., and Cidlowski, J. A. (2011). Cellular processing of the glucocorticoid receptor gene and protein: new mechanisms for generating tissue-specific actions of glucocorticoids. J. Biol. Chem. 286, 3177–3184. doi: 10.1074/jbc.R110.179325
Ovacik, M. A., Sukumaran, S., Almon, R. R., DuBois, D. C., Jusko, W. J., and Androulakis, I. P. (2010). Circadian signatures in rat liver: from gene expression to pathways. BMC Bioinformatics 11:540. doi: 10.1186/1471-2105-11-540
Palsson, B. (2002). In silico biology through “omics”. Nat. Biotechnol. 20, 649–650. doi: 10.1038/nbt0702-649
Pearce, E. L., and Pearce, E. J. (2013). Metabolic pathways in immune cell activation and quiescence. Immunity 38, 633–643. doi: 10.1016/j.immuni.2013.04.005
Pierre, K., Schlesinger, N., and Androulakis, I. P. (2016). The role of the hypothalamic-pituitary-adrenal axis in modulating seasonal changes in immunity. Physiol. Genomics 48, 719–738. doi: 10.1152/physiolgenomics.00006.2016
Qian, J., Dolled-Filhart, M., Lin, J., Yum, H., and Gerstein, M. (2001). Beyond synexpression relationships local clustering of time-shifted and inverted gene expression profiles identifies new, biologically relevant interactions. J. Mol. Biol. 314, 1053–1066. doi: 10.1006/jmbi.2000.5219
Ramakrishnan, R., DuBois, D. C., Almon, R. R., Pyszczynski, N. A., and Jusko, W. J. (2002). Fifth-generation model for corticosteroid pharmacodynamics: application to steady-state receptor down-regulation and enzyme induction patterns during seven-day continuous infusion of methylprednisolone in rats. J. Pharmacokinet. Pharmacodyn. 29, 1–24. doi: 10.1023/A:1015765201129
Rao, R., DuBois, D., Almon, R., Jusko, W. J., and Androulakis, I. P. (2016a). Mathematical modeling of the circadian dynamics of the neuroendocrine-immune network in experimentally induced arthritis. Am. J. Physiol. Endocrinol. Metab. 311, E310–E324. doi: 10.1152/ajpendo.00006.2016
Rao, R., Yang, Q., Orman, M. A., Berthiaume, F., Ierapetritou, M. G., and Androulakis, I. P. (2016b). Burn trauma disrupts circadian rhythms in rat liver. Int. J. Burns Trauma 6, 12–25.
Richards, S. E., Dumas, M.-E., Fonville, J. M., Ebbels, T. M. D., Holmes, E., and Nicholson, J. K. (2010). Intra- and inter-omic fusion of metabolic profiling data in a systems biology framework. Chemometr. Intell. Lab. Syst. 104, 121–131. doi: 10.1016/j.chemolab.2010.07.006
Rogers, S. (2011). Statistical methods and models for bridging Omics data levels. Methods Mol. Biol. 719, 133–151. doi: 10.1007/978-1-61779-027-0_6
Saharidis, G. K. D., Androulakis, I. P., and Ierapetritou, M. G. (2011). Model building using bi-level optimization. J. Glob. Optim. 49, 49–67. doi: 10.1007/s10898-010-9533-9
Schaaf, M. J., and Cidlowski, J. A. (2002). Molecular mechanisms of glucocorticoid action and resistance. J. Steroid Biochem. Mol. Biol. 83, 37–48. doi: 10.1016/S0960-0760(02)00263-7
Schäcke, H., Döcke, W.-D., and Asadullah, K. (2002). Mechanisms involved in the side effects of glucocorticoids. Pharmacol. Ther. 96, 23–43. doi: 10.1016/S0163-7258(02)00297-8
Scheff, J. D., Almon, R. R., DuBois, D. C., Jusko, W. J., and Androulakis, I. P. (2010a). A new symbolic representation for the identification of informative genes in replicated microarray experiments. OMICS 14, 239–248. doi: 10.1089/omi.2010.0005
Scheff, J. D., Almon, R. R., DuBois, D. C., Jusko, W. J., and Androulakis, I. P. (2011). Assessment of pharmacologic area under the curve when baselines are variable. Pharm. Res. 28, 1081–1089. doi: 10.1007/s11095-010-0363-8
Scheff, J. D., Calvano, S. E., Lowry, S. F., and Androulakis, I. P. (2010b). Modeling the influence of circadian rhythms on the acute inflammatory response. J. Theor. Biol. 264, 1068–1076. doi: 10.1016/j.jtbi.2010.03.026
Scheff, J. D., Mavroudis, P. D., Foteinou, P. T., An, G., Calvano, S. E., Doyle, J., et al. (2013). A multiscale modeling approach to inflammation: a case study in human endotoxemia. J. Comput. Phys. 244, 279–289. doi: 10.1016/j.jcp.2012.09.024
Sharma, A., Ebling, W. F., and Jusko, W. J. (1998). Precursor-dependent indirect pharmacodynamic response model for tolerance and rebound phenomena. J. Pharm. Sci. 87, 1577–1584. doi: 10.1021/js980171q
Sharma, A., and Jusko, W. J. (1998). Characteristics of indirect pharmacodynamic models and applications to clinical drug responses. Br. J. Clin. Pharmacol. 45, 229–239. doi: 10.1046/j.1365-2125.1998.00676.x
Stamatelos, S. K., Androulakis, I. P., Kong, A. N., and Georgopoulos, P. G. (2013). A semi-mechanistic integrated toxicokinetic-toxicodynamic (TK/TD) model for arsenic(III) in hepatocytes. J. Theor. Biol. 317, 244–256. doi: 10.1016/j.jtbi.2012.09.019
Storey, J. D., Dai, J. Y., and Leek, J. T. (2007). The optimal discovery procedure for large-scale significance testing, with applications to comparative microarray experiments. Biostatistics 8, 414–432. doi: 10.1093/biostatistics/kxl019
Storey, J. D., Xiao, W., Leek, J. T., Tompkins, R. G., and Davis, R. W. (2005). Significance analysis of time course microarray experiments. Proc. Natl. Acad. Sci. U.S.A. 102, 12837–12842. doi: 10.1073/pnas.0504609102
Sukumaran, S., Jusko, W. J., DuBois, D. C., and Almon, R. R. (2011). Mechanistic modeling of the effects of glucocorticoids and circadian rhythms on adipokine expression. J. Pharmacol. Exp. Ther. 337, 734–746. doi: 10.1124/jpet.111.179960
Sun, Y.-N., DuBois, D., Almon, R., and Jusko, W. (1998). Fourth-generation model for corticosteroid pharmacodynamics: a model for methylprednisolone effects on receptor/gene-mediated glucocorticoid receptor down-regulation and tyrosine aminotransferase induction in rat liver. J. Pharmacokinet. Biopharm. 26, 289–317. doi: 10.1023/a:1023233409550
Swartz, S. L., and Dluhy, R. G. (1978). Corticosteroids: clinical pharmacology and therapeutic use. Drugs 16, 238–255. doi: 10.2165/00003495-197816030-00006
Swiss, V. A., Nguyen, T., Dugas, J., Ibrahim, A., Barres, B., Androulakis, I. P., et al. (2011). Identification of a gene regulatory network necessary for the initiation of oligodendrocyte differentiation. PLoS ONE 6:e18088. doi: 10.1371/journal.pone.0018088
Toga, A. W., Foster, I., Kesselman, C., Madduri, R., Chard, K., Deutsch, E. W., et al. (2015). Big biomedical data as the key resource for discovery science. J. Am. Med. Inform. Assoc. 22, 1126–1131. doi: 10.1093/jamia/ocv077
Tompkins, R. G. (2015). Genomics of injury: the glue grant experience. J. Trauma Acute Care Surg. 78, 671–686. doi: 10.1097/TA.0000000000000568
Tu, C., Li, J., Bu, Y., Hangauer, D., and Qu, J. (2012). An ion-current-based, comprehensive and reproducible proteomic strategy for comparative characterization of the cellular responses to novel anti-cancer agents in a prostate cell model. J. Proteomics 77, 187–201. doi: 10.1016/j.jprot.2012.08.020
Vegiopoulos, A., and Herzig, S. (2007). Glucocorticoids, metabolism and metabolic diseases. Mol. Cell. Endocrinol. 275, 43–61. doi: 10.1016/j.mce.2007.05.015
Wang, H., Khor, T. O., Yang, Q., Huang, Y., Wu, T.-Y., Saw, C. L.-L., et al. (2012). Pharmacokinetics and pharmacodynamics of phase II drug metabolizing/antioxidant enzymes gene response by anticancer agent sulforaphane in rat lymphocytes. Mol. Pharm. 9, 2819–2827. doi: 10.1021/mp300130k
Waters, K. M., Pounds, J. G., and Thrall, B. D. (2006). Data merging for integrated microarray and proteomic analysis. Brief. Funct. Genomic Proteomic 5, 261–272. doi: 10.1093/bfgp/ell019
Wu, T. Y., Huang, Y., Zhang, C., Su, Z. Y., Boyanapalli, S., Khor, T. O., et al. (2015). Pharmacokinetics and pharmacodynamics of 3,3′-diindolylmethane (DIM) in regulating gene expression of phase II drug metabolizing enzymes. J. Pharmacokinet. Pharmacodyn. 42, 401–408. doi: 10.1007/s10928-015-9421-5
Yang, E., Almon, R. R., DuBois, D. C., Jusko, W. J., and Androulakis, I. P. (2008a). Extracting global system dynamics of corticosteroid genomic effects in rat liver. J. Pharmacol. Exp. Ther. 324, 1243–1254. doi: 10.1124/jpet.107.133074
Yang, E., Maguire, T., Yarmush, M. L., and Androulakis, I. P. (2008b). Informative gene selection and design of regulatory networks using integer optimization. Comput. Chem. Eng. 32, 633–649. doi: 10.1016/j.compchemeng.2007.01.009
Yang, E., Foteinou, P. T., King, K. R., Yarmush, M. L., and Androulakis, I. P. (2007a). A novel non-overlapping bi-clustering algorithm for network generation using living cell array data. Bioinformatics 23, 2306–2313. doi: 10.1093/bioinformatics/btm335
Yang, E., Maguire, T. J., Yarmush, M. L., Berthiaume, F., and Androulakis, I. P. (2008c). Identification of regulatory mechanisms of the hepatic response to thermal injury. Comput. Chem. Eng. 32, 356–369. doi: 10.1016/j.compchemeng.2007.02.009
Yang, E., Misra, A., Maguire, T. J., and Androulakis, I. P. (2009a). Analysis of regulatory and interaction networks from clusters of co-expressed genes. Cluster Chall. Biol. Netw. 53–82. doi: 10.1142/9789812771667_0003
Yang, E., Simcha, D., Almon, R. R., DuBois, D. C., Jusko, W. J., and Androulakis, I. P. (2007b). Context specific transcription factor prediction. Ann. Biomed. Eng. 35, 1053–1067. doi: 10.1007/s10439-007-9268-z
Yang, E., Yarmush, M. L., and Androulakis, I. P. (2009b). Transcription factor network reconstruction using the living cell array. J. Theor. Biol. 256, 393–407. doi: 10.1016/j.jtbi.2008.09.040
Yang, E. H., Almon, R. R., DuBois, D. C., Jusko, W. J., and Androulakis, I. P. (2009). Identification of global transcriptional dynamics. PLoS ONE 4:e5992. doi: 10.1371/journal.pone.0005992
Yang, Q., Berthiaume, F., and Androulakis, I. P. (2011a). A quantitative model of thermal injury-induced acute inflammation. Math. Biosci. 229, 135–148. doi: 10.1016/j.mbs.2010.08.003
Yang, Q., Calvano, S. E., Lowry, S. F., and Androulakis, I. P. (2011b). A dual negative regulation model of Toll-like receptor 4 signaling for endotoxin preconditioning in human endotoxemia. Math. Biosci. 232, 151–163. doi: 10.1016/j.mbs.2011.05.005
Yang, Q., Mattick, J. S., Orman, M. A., Nguyen, T. T., Ierapetritou, M. G., Berthiaume, F., et al. (2012a). Dynamics of hepatic gene expression profile in a rat cecal ligation and puncture model. J. Surg. Res. 176, 583–600. doi: 10.1016/j.jss.2011.11.1031
Keywords: transcriptomics, proteomics, metabolomics, pharmacogenomics, inflammation, glucocorticoids, LPS
Citation: Kamisoglu K, Acevedo A, Almon RR, Coyle S, Corbett S, Dubois DC, Nguyen TT, Jusko WJ and Androulakis IP (2017) Understanding Physiology in the Continuum: Integration of Information from Multiple -Omics Levels. Front. Pharmacol. 8:91. doi: 10.3389/fphar.2017.00091
Received: 09 September 2016; Accepted: 13 February 2017;
Published: 27 February 2017.
Edited by:
Tarek A. Leil, Bristol-Myers Squibb, USAReviewed by:
Mark Christopher Coles, University of York, UKFrancesco Pappalardo, University of Catania, Italy
Copyright © 2017 Kamisoglu, Acevedo, Almon, Coyle, Corbett, Dubois, Nguyen, Jusko and Androulakis. This is an open-access article distributed under the terms of the Creative Commons Attribution License (CC BY). The use, distribution or reproduction in other forums is permitted, provided the original author(s) or licensor are credited and that the original publication in this journal is cited, in accordance with accepted academic practice. No use, distribution or reproduction is permitted which does not comply with these terms.
*Correspondence: Ioannis P. Androulakis, eWFubmlzQHNvZS5ydXRnZXJzLmVkdQ==