- 1Beijing Shijitan Hospital, Capital Medical University, Beijing, China
- 2Key Laboratory of Marine Drugs (Ministry of Education of China), School of Medicine and Pharmacy, Ocean University of China, Qingdao, China
- 3Laboratory for Marine Drugs and Bioproducts, Qingdao National Laboratory for Marine Science and Technology, Qingdao, China
- 4College of Pharmacy, Chengdu University of Traditional Chinese Medicine, Chengdu, China
- 5Information Center, Beijing University of Chinese Medicine, Beijing, China
The rapidly increasing diabetes mellitus (DM) is becoming a major global public health issue. Traditional Chinese medicine (TCM) has a long history of the treatment of DM with good efficacy. Huangqi and Huanglian are one of the most frequently prescribed herbs for DM, and the combination of them occurs frequently in antidiabetic formulae. However, the synergistic mechanism of Huangqi (Radix Astragali) and Huanglian (Rhizoma Coptidis) has not been clearly elucidated. To address this problem, a feasible system pharmacology model based on chemical, pharmacokinetic and pharmacological data was developed via network construction approach to clarify the synergistic mechanisms of these two herbs. Forty-three active ingredients of Huangqi (mainly astragalosides and isoflavonoids) and Huanglian (primarily isoquinoline alkaloids) possessing favorable pharmacokinetic profiles and biological activities were selected, interacting with 50 DM-related targets to provide potential synergistic therapeutic actions. Systematic analysis of the constructed networks revealed that these targets such as GLUT2, NOS2, PTP1B, and IGF1R were mainly involved in PI3K-Akt signaling pathway, insulin resistance, insulin signaling pathway, and HIF-1 signaling pathway, and were mainly located in retina, pancreatic islet, smooth muscle, immunity-related organ tissues, and whole blood. The contribution index of every active ingredient also indicated five compounds, including berberine (BBR), astragaloside IV (AIV), quercetin, palmatine, and astragalus polysaccharides, as the principal components of this herb combination. These results successfully explained the polypharmcological and synergistic mechanisms underlying the efficiency of Huangqi and Huanglian for the treatment of DM and its complications.
Introduction
Diabetes mellitus (DM) is a chronic metabolic disorder influenced by interactions between genetic and environmental factors. The global prevalence of DM among adults aged 20–79 years was 8.8% in 2015, and its incidence is increasing rapidly (International Diabetes Federation, 2015). As complementary and alternative medicine, traditional Chinese medicine (TCM) has been proven to possess satisfactory effectiveness toward DM and its complications clinically, such as Gegen Qinlian Decoction (Tong et al., 2011) and Huangqi San (Xu et al., 2015), wherein Huangqi (Radix Astragali, the dried roots of Astragalus membranaceus (Fisch.) Bunge. var. mongholicus (Bunge.) Hsiao or A. membranaceus (Fisch.) Bunge, Fabaceae) and Huanglian (Rhizoma Coptidis, the rhizomes of Coptis chinensis Franch, Ranunculaceae) are one of the most frequently prescribed herbs (Xie et al., 2011). Specifically, Huangqi is widely used in East Asia to reinforce Qi (Ma et al., 2002) and its use to treat DM, classified as Xiao Ke syndrome in TCM, has been firstly documented in Shen Nong Ben Cao Jing (206 BC–24 AD, Western Han Dynasty). Huangqi has been developed into the intravenous injection (mainly astragalosides) in China to treat DM with good clinical effects (Nie et al., 2014). As a holy herb to treat Xiao Ke syndrome, Huanglian is frequently used in diabetic care partially due to its antihyperglycemic, antihyperlipidemic, antihypertensive, anti-inflammatory, and antioxidant activities (Tong et al., 2011; Pang et al., 2015). Based on a previous statistics, Huanglian has been used as a major ingredient in many antidiabetic Chinese patent medicines (CPMs) approved by the China Food and Drug Administration and the majority of them are combined with Huangqi, such as Jinqi Jiangtang tablets, Xiaokeping tablets, Tangmaikang capsules, and Shenjing Zhike Wan (Xie et al., 2011). However, although the combination of Huangqi and Huanglian has been frequently used in antidiabetic formulae and CPMs (Supplementary Figure S1), we still know little about how the active ingredients in Huangqi and Huanglian modulate the synergistic network for combating DM.
System pharmacology is emerging as a holistic and efficient tool to study the role of TCM due to its capable of describing complex interactions between drugs and biological systems including the human body, organs, and diseases from a network perspective (Kloft et al., 2016; Zhang et al., 2016). Combined with pharmacology and pharmacodynamics, it has been successfully applied to interpret the synergistic mechanisms of herb combinations at molecular network level (Zhou et al., 2016; Yu et al., 2017; Yue et al., 2017). In the present study, we tried to establish the compound-target (C-T), target-pathway (T-P), and target-organ (T-O) networks by the system pharmacology model based on chemical, pharmacokinetic and pharmacological data at the system, organ, and molecular levels (Supplementary Figure S2), so as to uncover the underlying synergistic mechanisms of Huangqi and Huanglian for treating DM.
Materials and Methods
Chemical Ingredients Database Building
All of the constituent data of Huangqi and Huanglian were retrieved from TCM Systems Pharmacology Database and Analysis Platform (TcmSP™, http://ibts.hkbu.edu.hk/LSP/tcmsp.php) (Ru et al., 2014), and then manually supplemented through a wide-scale text-mining method. Meanwhile, four important pharmacology-related properties were also obtained from TcmSP™, including MW, CLogP, nHDon, and nHAcc. The principal component analysis (PCA) of the chemical distribution of Huangqi and Huanglian was built with the above four properties using the SIMCAP+ software package (version 11.0, Umetrics). The variances of PC1, PC2, and PC3 in Figure 1 account for 0.71, 0.23, and 0.04, respectively. The PCA of 34 known drug/drug-like compounds retrieved from DrugBank (http://www.drugbank.ca/) was performed in the same process as above (Supplementary Table S1).
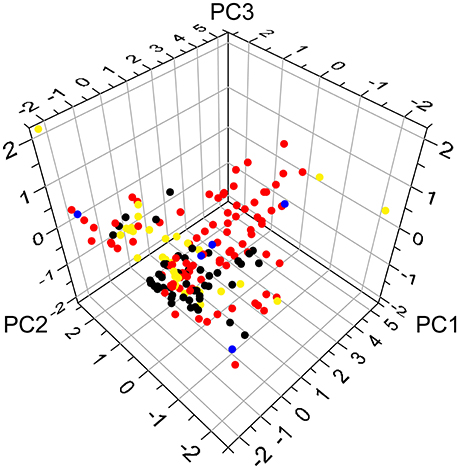
Figure 1. The chemical distribution according to principal component analysis. The red and black circles represent ingredients of Huangqi and Huanglian, respectively, while the blue circles delineate common ingredients of Huangqi and Huanglian. The yellow circles stand for antidiabetic drugs from DrugBank.
Active Ingredients Screening
The active ingredients from Huangqi and Huanglian were mainly filtered by integrating oral bioavailability (OB) and drug-likeness (DL). A robust in silico model OBioavail 1.1 that integrated the metabolism (P450 3A4) and transport (P-glycoprotein) information was employed to calculate the OB values of all herbal ingredients (Xu et al., 2012). Those ingredients with OB ≥ 30% were selected. Database-dependent DL evaluation approach based on Tanimoto coefficient (Ma et al., 2011) was applied and shown as T(A, B) = (A×B)/(|A|2+|B|2−A×B). In this equation, A represents the molecular descriptors of herbal compounds, and B displays the average molecular properties of all compounds in DrugBank. Those ingredients with DL ≥ 0.18 were preserved. In this study, the ingredients were adopted as the candidate compounds for further analysis when they met both of these criteria. Besides, owing to the profound pharmacological effects and high contents, those compounds with low OB or DL values were also selected for further research.
Targets Prediction
To identify the corresponding targets of the active ingredients of Huangqi and Huanglian, several approaches combined with chemometric method, information integration, and data-mining were implemented. First of all, the active ingredients were submitted to various servers viz. DRAR-CPI (Luo et al., 2011), Similarity Ensemble Approach (SEA, http://sea.bkslab.org/) (Keiser et al., 2007), STITCH (http://stitch.embl.de/) (Kuhn et al., 2012), and PharmMapper server (Wang et al., 2017). All active compounds were also sent to Herbal Ingredients' Targets database (HIT) (Ye et al., 2011), Therapeutic Targets Database (TTD, http://bidd.nus.edu.sg/group/ttd/) (Zhu et al., 2012), BindingDB database (http://www.bindingdb.org/bind/index.jsp) (Gilson et al., 2016), DrugBank and Google Scholar to mine C-T, interactions supported by literature. Then, to better dissect the role of Huangqi and Huanglian in DM treatment, all targets obtained from the previous two steps were sent to TTD, Comparative Toxicogenomics Database (CTD, http://ctdbase.org/), Online Mendelian Inheritance in Man (OMIM) database (http://www.omim.org/) and PharmGKB (http://www.pharmgkb.org) (Whirl-Carrillo et al., 2012) to mine whether these targets are related to DM. Noteworthy, only the targets of Homo sapiens were kept for further analysis.
Gene Ontology (GO) and Target Organ Location Analysis
The Molecule Annotation System 3.0 (MAS 3.0, http://bioinfo.capitalbio.com/mas3/) and DAVID (The Database for Annotation, Visualization and Integrated Discovery, http://david.abcc.ncifcrf.gov) webservers were employed to perform GO enrichment analysis for the 50 genes targeted by Huangqi and Huanglian (Huang da et al., 2009). The target organ distribution was determined based on the microarray analyses data of different tissue types lodged in the BioGPS bank (http://biogps.org) (Wu et al., 2009).
Network Construction
Three visualized networks were constructed: (1) Compound-Target network (C-T network). Active ingredients of Huangqi and Huanglian and their corresponding targets were employed to generate the C-T network; (2) Target-Pathway network (T-P network). The pathway information of targets were extracted from KEGG (Kyoto Encyclopedia of Genes and Genomes, http://www.kegg.jp), and then a bipartite T-P network composed of targets and their corresponding putative pathways was built; (3) T-O network. The potential targets and their tissue types were applied to T-O network. All visualized networks were constructed by Cytoscape 3.5.1 (http://www.cytoscape.org/), an open software package project for visualizing, integrating, modeling and analyzing the interaction networks (Smoot et al., 2011).
Contribution Indexes Calculation
In order to estimate the contribution of each active ingredient to the antidiabetic effects of Huangqi and Huanglian, a contribution index (CI) based on network based efficacy (NE) weighted by literature was calculated by Equation (1) and equation (2) (Yue et al., 2017):
Where n is the number of targets associated with ingredient j in the C-T network; di is the degree of target i associated with ingredient j in the T-P network; ci is the number of DM-related literature of ingredient i; m is the number of ingredients. For DM-related literature-mining approach, the following keywords were used for DM terms: diabetes, hyperglycemia, and insulin resistance and the common names of active ingredients were also used as search keywords. The numbers of papers having keywords in the title or abstract published in 1990–2017 were obtained from the PubMed database. If the sum of CIs for the top N ingredients was more than 85%, these relevant N ingredients were considered to contribute the most to the antidiabetic effects.
Results
Chemical Distribution of Huangqi and Huanglian
The ingredients in Huangqi and Huanglian were retrieved from TcmSP™ and published literatures. Since those glycosides in Huangqi and Huanglian might be deglycosylated in the intestinal tract associated with gut microbiota, 13 aglycones were also incorporated into the compound library labeled by _qt. Thus, a total of 171 ingredients were retrieved for Huangqi (112) and Huanglian (64), including five common ingredients. The detailed information about these molecules was provided in Supplementary Table S2.
The PCA was further conducted to give visual illustration in chemical distribution. The constituents of Huangqi and Huanglian were diverse and both of them possessed a broad diversity in chemical space (Figure 1), but the majority of them satisfied the Lipinski's rule of five. Moreover, the large overlap between the ingredients in Huangqi and Huanglian and 34 known drug/drug-like compounds for DM demonstrated that many compounds contained in these two herbs had drug potential on DM. Noticeably, since PC2 was highly associated with the value of nHDon, those ingredients of Huangqi (red circle) including astragalosides with PC2 in the range 3~5 possessed larger nHDon values than the antidiabetic drugs from DrugBank (yellow circle), whereas the ingredients of Huanglian (black circle) overlap better with the antidiabetic drugs in Figure 1. Thus, it is meaningful to figure out these active ingredients in Huangqi and Huanglian for DM treatment.
Active Ingredients in Huangqi and Huanglian
Although a single herb or TCM formula usually contains large numbers of compounds, virtual screening approaches are always of great help to distinguish those active ingredients. In the present work, two ADME (absorption, distribution, metabolism, and excretion)-related models, including OB and DL were employed to screen most of the active ingredients from Huangqi and Huanglian. A few of active compounds that do not meet either of these two criteria were also selected in the cases of high bioactivities and huge amounts. Consequently, a total of 43 active compounds were selected from the 171 compounds of these two herbs (Table 1).
Active Ingredients from Huangqi
In Huangqi, only 26 ingredients passed through the strict filtering criteria, and most of them exhibited potent pharmacological activities. For examples, calycosin (M120, OB = 47.75%, and DL = 0.24) displayed therapeutic effects on diabetic complication (Xu et al., 2011); formononetin (M125, OB = 69.67%, and DL = 0.21) showed significant antihyperglycemic activity (Qiu et al., 2017). Other characteristic isoflavonoids in Huangqi accounted for large contents and were also preserved. Specifically, calycosin-7-O-β-D-glucoside (M121, OB = 10.05%, and DL = 0.81), formononetin-7-O-β-D-glucoside (M126, OB = 11.52%, and DL = 0.78), (6αR, 11αR) 3-hydroxy-9,10-dimethoxypterocarpan-3-O-β-D-glucoside (M123, OB = 36.74%, and DL = 0.92), together with calycosin and formononetin in Huangqi were measured up to 0.44–1.76 mg/g (Wu et al., 2005). Surprisingly, astragalosides, the main active and characteristic compounds in Huangqi, exhibited low OB values (Gu et al., 2004; Ma et al., 2017). Among them, astragaloside IV (AIV, M98, OB = 2.20%, and DL = 0.15) has been determined as the quality marker of Huangqi in Chinese Pharmacopoeia (The State Pharmacopoeia Commission of China, 2015) and exhibited significant hypoglycemic effect (Lv et al., 2010). Astragaloside II (M94, OB = 0.79%, and DL = 0.13) and isoastragaloside I (M104, OB = 37.80%, and DL = 0.14) could alleviate insulin resistance and glucose intolerance by enhancing the expression of an insulin-sensitizing adiponectin (Xu et al., 2009). Besides, the contents of astragaloside I (M92, OB = 46.79%, and DL = 0.11), astragaloside II, astragaloside III (M96, OB = 31.83%, and DL = 0.10) and AIV in Huangqi were 0.78, 0.35, 0.20, and 0.26 mg/g, respectively (Zu et al., 2009). Importantly, astragalus injection (the major components are astragalosides) has been used in China to treat DM with good clinical effects (Nie et al., 2014). Thus, astragalosides were also selected for targeting. Astragalus polysaccharides (M171) as a polysaccharide mixture from Huangqi (accounted for 90.00–288.75 mg/g) was also preserved because it could ameliorate insulin resistance and restore glucose homeostasis, in part, via gut microbiota (Zou et al., 2009; Zhang et al., 2013; Liu et al., 2014).
Active Ingredients from Huanglian
By ADME screening, 21 out of 64 ingredients with excellent pharmacological effects were extracted from Huanglian, and half of them possessed satisfactory pharmacokinetic profiles. It should be pointed out that most of the representative isoquinoline alkaloids in Huanglian showed extremely low OB values (Li et al., 2006; Chen et al., 2011; Bao et al., 2015), but they exhibited potent antidiabetic, anti-inflammatory, and antioxidant activities (Patel and Mishra, 2012; Zhang et al., 2012). For instances, berberine (BBR, M1, OB = 0.68%, and DL = 0.78) had lipid- and glucose-lowing effects in treatment of DM and obesity, which may be associated with gut microbiota (Zhang et al., 2012); magnoflorine (M10, OB = 22.60%, and DL = 0.55) could exert antioxidant and antiglycemic effects in vivo (Patel and Mishra, 2012). Besides, BBR and coptisine (M23, OB = 7.21%, and DL = 0.86) together with epiberberine (M12, OB = 43.09%, and DL = 0.78) and palmatine (M21, OB = 64.60%, and DL = 0.65) have been chosen as the marker components for quality control of Huanglian in Chinese Pharmacopoeia (The State Pharmacopoeia Commission of China, 2015). Meanwhile, the isoquinoline alkaloids accounted for large amounts in Huanglian. For example, the contents of BBR, coptisine, jatrorrhizine (M20, OB = 19.65%, and DL = 0.59), palmatine, and epiberberine ranged from 58.47 to 71.35, 19.38 to 22.56, 4.96 to 5.33, 15.68 to 20.57, and 11.05 to 12.44 mg/g, respectively, and the total content of these five alkaloids was 111.33–129.42 mg/g (Ding et al., 2012). In view of the facts mentioned above, isoquinoline alkaloids were deemed as the active ingredients for further analysis. Strikingly, ferulic acid (M32, OB = 39.56%, and DL = 0.06) as a minor constituent in Huanglian exhibited synergistic effect on antihyperglycemic activity in combination with BBR (Chen et al., 2012). Vanillic acid (M33, OB = 35.47%, and DL = 0.04) has the potential to prevent the progression of DM via ameliorating insulin resistance (Chang et al., 2015). It was reasonable to believe that the above compounds could be listed as potential active ingredients for Huanglian (Table 1).
Noteworthy, besides choline, other four shared compounds were selected and have beneficial effects on DM. Specifically, β-sitosterol (M60, OB = 36.23%, and DL = 0.78) exhibited potent antidiabetic and antioxidant activities (Gupta et al., 2011); rutin (M141, OB = 11.70%, and DL = 0.68) had hypoglycemic effect and may confer protective effects against diabetic nephropathy (Han et al., 2017); quercetin (M148, OB = 46.43%, and DL = 0.28) presented anti-obesity and antidiabetic activities (Aguirre et al., 2011); additionally, kaempferol (M154, OB = 67.43%, and DL = 0.24) could exert antidiabetic benefits through protecting beta-cells against glucotoxicity (Zhang and Liu, 2011).
Target Proteins of Huangqi and Huanglian
Searching for the targets of candidate drugs solely by the experimental approaches is overspending, labor-intensive, and time-consuming. In the present work, an integrated in silico approach was introduced to identify the target proteins for the active ingredients of Huangqi and Huanglian. Predictive models were used including DRAR-CPI, SEA, STITCH and PharmMapper server, and databases were mined including HIT, TTD, BindingDB database, DrugBank and Google Scholar. Finally, 50 DM-related targets were determined, interacting with the selected 43 active ingredients of this herb combination (Table 2). Of note, we have implemented molecular docking and surface plasmon resonance (SPR) assay to explore the reliability of the interactions between the active ingredients and their putative targets. As shown in Supplementary Table S3, Supplementary Figures S3, S4, it could be concluded that the predicted targets were convincible to explore the network interactions of Huangqi and Huanglian.
Target Proteins of Huangqi
For Huangqi, by target fishing, 26 active ingredients were validated to bind with 42 DM-related target proteins. For example, AIV may have the potential to act on 21 targets including NR3C1, VEGFA, NOS2, CASP3, PYGL, PPARG, and AKR1B1. Actually, AIV has been identified as an inhibitor of NR3C1, which might contribute to its therapeutic application in DM (Liu et al., 2016). Beyond that, it also has a strong antagonistic effect on PYGL, suggestive of its contribution to the control of blood glucose homeostasis (Lv et al., 2010). Analogously, formononetin and calycosin exhibited strong activation on PPARA and PPARG to correct dyslipidemia and to restore glycemic balance (Shen et al., 2006). Formononetin could also alleviate the retinal neovascularization of diabetic retinopathy by inhibiting VEGFA (Wu et al., 2016). Cycloastragenol, as the aglycone of astragalosides could improve hepatic steatosis through activating FXR, thereby alleviating DM-related hyperglycemia and hyperlipidemia (Gu et al., 2017). Hederagenin and lupeol interacted with GSTM1 to decrease the risk of diabetic retinopathy and nephropathy (Datta et al., 2010; Sun et al., 2015). Notably, although it is hardly to predict the targets of astragalus polysaccharides in silico, some DM-related targets, such as GAA, PTP1B, and AMPK, have been reported to be associated with its antidiabetic effect (Zou et al., 2009; Zhao et al., 2012; Zhu et al., 2014).
Target Proteins of Huanglian
Forty-three targets were identified for 21 active ingredients of Huanglian with 318 interactions, such as PIK3CG, PTP1B, AMPK, PIK3R1, IL1B, HNF4A, and MAPK1. For instance, BBR, epiberberine, magnoflorine, and coptisine inhibited PTP1B to increase insulin and leptin activities, thereby possibly exerting antidiabetic activity (Choi et al., 2015). Vanillic acid may interact with four potential targets including GLUT2, IDE, GLP1R, and GAA to display antidiabetic activity (Chang et al., 2015). It was worthy to mention that some major targets, such as AKR1B1, ADRB2, HMOX1, and NOS3, were also closely concerned with the various symptoms of diabetic complications. BBR, epiberberine, coptisine, and groenlandicine could inhibit AKR1B1 to alleviate the diabetic complications (Jung et al., 2008; Liu et al., 2008). BBR, palmatine and (R)-canadine may interact with ADRB2 to prevent the progression of obesity and hypertriglyceridaemia (Ishiyama-Shigemoto et al., 1999).
GO Enrichment Analysis for Targets
Analysis of interaction network regulation of 50 targets was performed using MAS 3.0. As shown in Figure 2, biological process (BP, GO:0008150), molecular function (MF, GO:0003674), and cellular component (CC, GO:0005575) accounted for 64.82, 24.10, and 11.08%, respectively. Further, BP, MF, and CC enrichment analysis were performed by DAVID bioinformatics resources. The top 10 significantly enriched terms in BP, MF, and CC categories (P < 0.05, P-values were corrected using the Benjamini-Hochberg procedure) were listed in Figure 2, indicating that Huangqi and Huanglian may regulate glucose homeostasis, insulin secretion, and nitric oxide biosynthetic process via enzyme binding, insulin binding, and kinase binding in the cytosol, caveola, and plasma membrane so as to exert antidiabetic potential. It is interesting to note that a large number of targets were associated with a variety of BP terms such as regulation of insulin secretion, glucose homeostasis, cellular response to insulin stimulus, lipopolysaccharide-mediated signaling pathway, and positive regulation of nitric oxide biosynthetic process, which are closely related to the pathogenesis of DM. KEGG pathway enrichment analysis was also performed by DAVID bioinformatics resources and displayed in Supplementary Figure S5. Furthermore, network and functional association of 50 targets of Huangqi and Huanglian was mapped in Supplementary Figure S6 using GeneMANIA (http://www.genemania.org/).
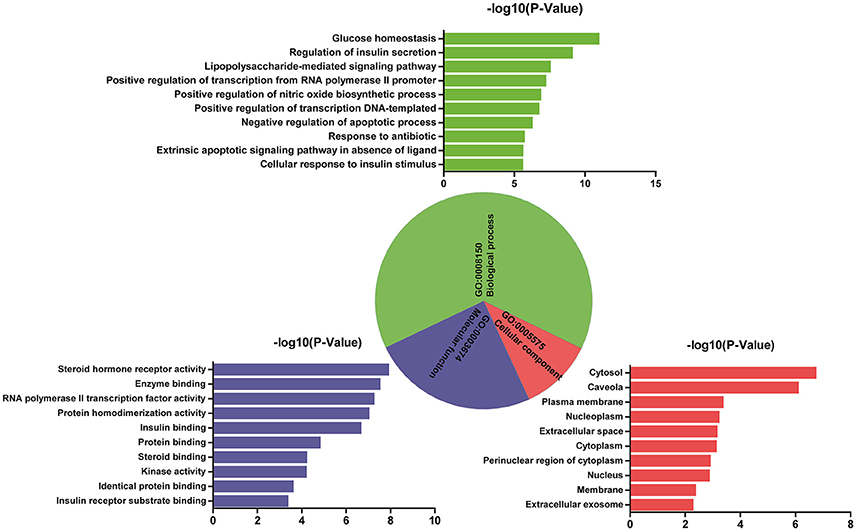
Figure 2. GO enrichment analysis of the targets of Huangqi and Huanglian. Biological process (green), molecular function (blue), and cellular component (red) accounted for 64.82, 24.10, and 11.08%, respectively.
Network Analysis to Decipher the Synergistic Mechanisms of Huangqi and Huanglian
Compound-Target Network Analysis
To facilitate the visualization and interpretation of the complex relationships between all active ingredients of Huangqi and Huanglian and their targets, a bipartite graph of C-T network was constructed (Figure 3A). All active ingredients in these two herbs were potential multiple-kinase inhibitors or activators. Amongst them, those ones with high interconnection degrees were responsible for the high interconnectedness of the C-T network, especially BBR (degree = 33), lupeol (degree = 22), quercetin (degree = 22), AIV (degree = 21), epiberberine (degree = 21), calycosin (degree = 21), and (R)-canadine (degree = 21). Importantly, as shown in the C-T network (Figure 3A), the efficacy of this herb combination not only concentrated on modulating the crucial targets involving in the glucose and insulin homeostasis (IGF1R, GAA, IDE, HNF1A, GCK, and DPP4), but also, more essentially, focused on the regulation of the other proteins mediating diabetic complications including inflammation, retinopathy, neuropathy, nephropathy, and abdominal pain (NOS2, AKR1B1, VEGFA, PTGS2, ESR2, and AChE) to relieve the pathological changes and prolong the efficient curing process. Additionally, as expected, the majority of the targets (32) such as GLUT2, NOS2, PTP1B, and IGF1R were synergistically regulated by different components of Huanglian and Huangqi.
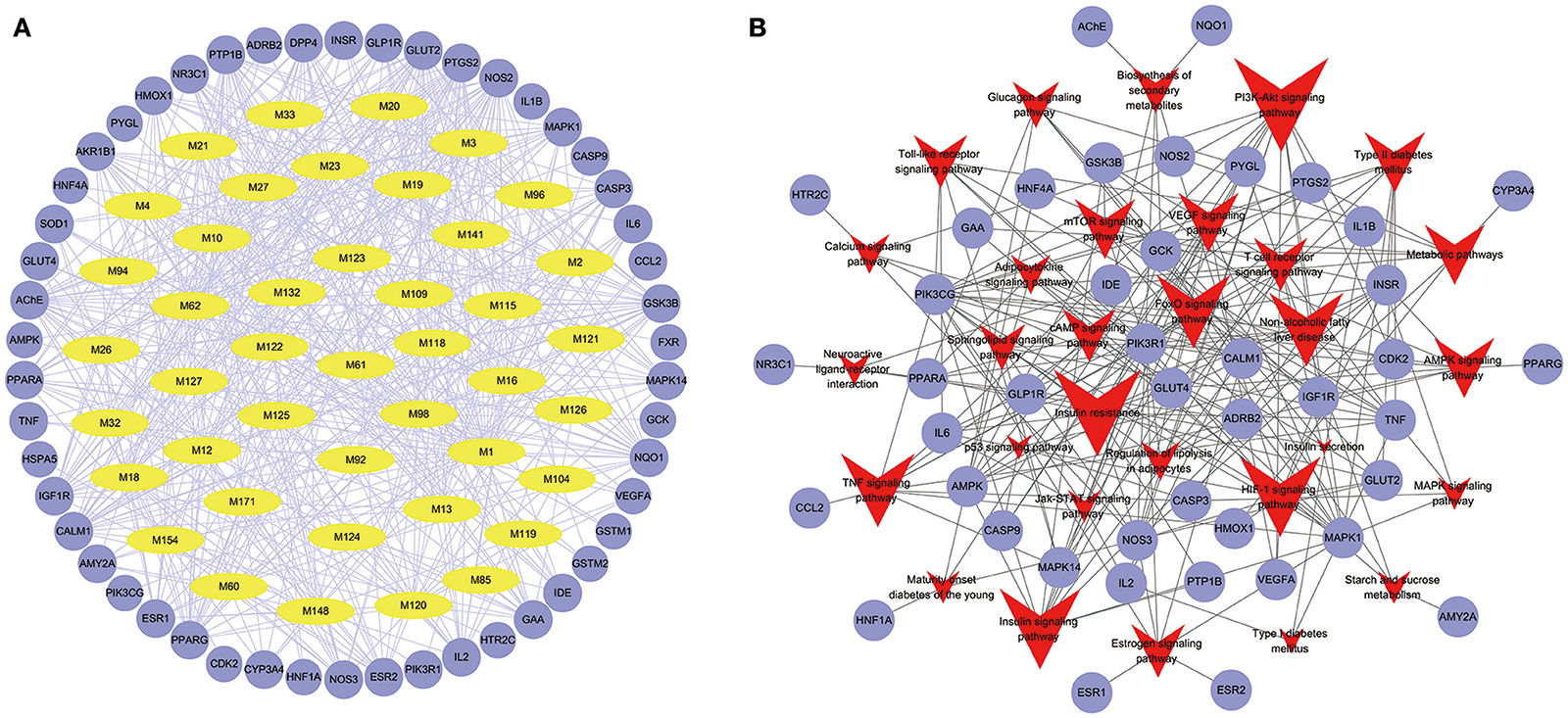
Figure 3. Compound-Target (A) and Target-Pathway (B) networks of Huangqi and Huanglian. The yellow and light blue nodes are active ingredients and their potential targets of Huangqi and Huanglian, while the red nodes represent the pathways.
Target-Pathway Network Analysis
Signaling pathways, as an important component of the system pharmacology, link receptor-ligand interactions to pharmacodynamics outputs. All of the targets interacting with the active ingredients were mapped onto the 30 KEGG pathways and the T-P network was generated (Figure 3B). The PI3K-Akt signaling pathway exhibited the highest number of target connections (degree = 13), followed by insulin resistance with 12 targets, insulin signaling pathway and HIF-1 signaling pathway with 11 ones, respectively. These high-degree pathways have well-established roles in the insulin secretion and glucose homeostasis (Taniguchi et al., 2006). Besides, the VEGF signaling pathway played an important role in the diabetic retinopathy involved in multiple targets including PIK3CG, PTGS2, and VEGFA (Antonetti et al., 2012). The activation of mTOR signaling pathway was an underlying cause of renal hypertrophy at the early stage of DM (Sakaguchi et al., 2006). Interestingly, we also found that the combination of Huangqi and Huanglian may exert its therapeutic effects on DM by regulating the pathways related to the adipogenesis and/or lipolysis in adipocytes, liver, and vascular tissues. As shown in the compressed pathway (Figure 4), Huangqi and Huanglian synergistically acted on the multiple targets in these high-degree pathways.
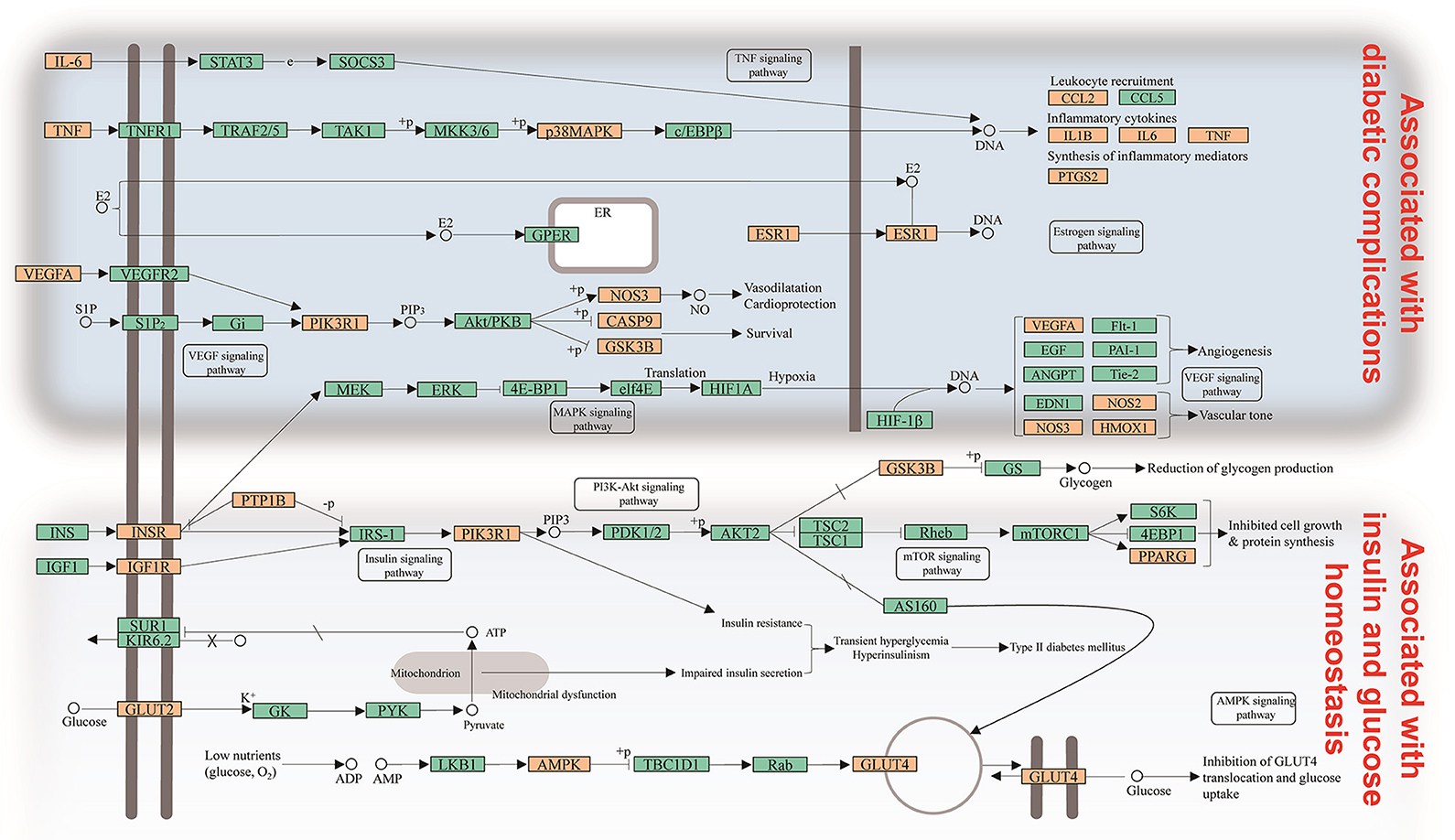
Figure 4. Distribution of partial targets of Huangqi and Huanglian on the compressed pathway. The orange nodes are potential targets of Huangqi and Huanglian, while the light blue nodes are relevant targets in the pathway.
Integrating with the above two networks, a CI of every active ingredient was proposed based on NE weighted by literature (Figure 5, Supplementary Table S4). Five compounds emerged from the active ingredients, including BBR, AIV, quercetin, palmatine, and astragalus polysaccharides. They displayed the most contribution to the antidiabetic effects of Huangqi and Huanglian with a sum of CIs of 85.01%. Therefore, the above discussion may fully clarify why Huangqi and Huanglian could produce synergistic and complementary effects.
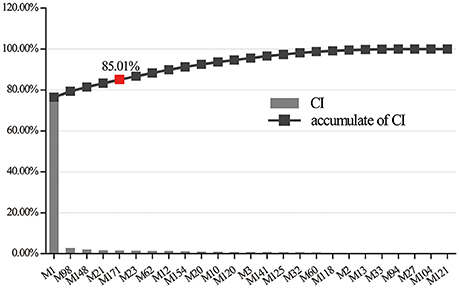
Figure 5. The CI and accumulative CI of active ingredients in Huangqi and Huanglian. The sum of CIs for the top five ingredients including M1 (BBR), M98 (AIV), M148 (quercetin), M21 (palmatine), and M171 (astragalus polysaccharides) was more than 85%.
Target-Organ Network Analysis
It may facilitate the development of enhanced detection and treatment modalities for DM by understanding how the multi-organs respond to indications on a system level. We compared the expression patterns of 50 targets across different tissues at different levels according to the BioGPS database. The tissue distribution network of the 50 targets were mapped and shown in Figure 6. Most targets acted on two or more tissues, suggesting that these tissues are closely correlated. Specifically, 30 targets contained high mRNA expression in retina, accounting for 60% of all the targets. There were 29 targets (accounting for 58% of all the targets) located in the CD33 + myeloid, suggesting they were potential effective targets for the treatment of autoimmune DM. In addition, 20 targets were overexpressed in small intestine and 15 targets in colon. It is evident that patients with DM have a high incidence of gastrointestinal dysfunction (Abrahamsson, 1995) and gut microbiota disturbance (Kootte et al., 2012). Similarly, 27 targets acted in cardiac myocytes and 22 targets in heart, consistent with the fact that DM increases coronary heart disease (CHD) morbidity and mortality and is considered a CHD risk equivalent (Newman et al., 2017). Noticeably, the targets in whole blood were linked with tissues in almost all the forms, indicating that whole blood acted as the bridge and glycemic excursion played a vital role in the pathological processes of these tissues.
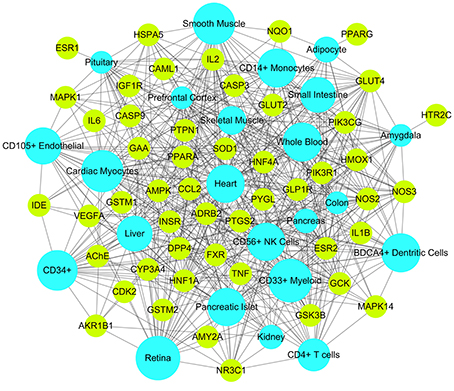
Figure 6. Target-Organ network of Huangqi and Huanglian where kelly nodes represent the targets and light blue nodes represent the organs.
Discussion
According to the TCM practice for thousands of years, DM can be classified as Xiao Ke (wasting thirst syndrome), which is usually associated with the deficiency of both Qi (vital energy) and Yin (body fluids) resulting in the Heat of tissues and blood or urine stasis. The “Gan” (sweetish taste) and “Ku” (bitter taste) are recognized as the popular therapeutic flavors to Xiao Ke syndrome (Xia et al., 2016). Among the commonly used herbs beneficial for DM, Huangqi (“Gan” flavor) and Huanglian (“Ku” flavor) are given high priority for selection and their combination has been frequently used in TCM prescriptions (Zhang et al., 2010; Xie et al., 2011). In our work, an integrated system pharmacology approach was successfully applied to illuminate the molecular synergy of Huangqi and Huanglian on DM. Forty-three active ingredients and 50 corresponding DM-related targets were selected and predicted, which were mainly involved in 30 KEGG signaling pathways associated with DM treatment and prophylaxis. By systematic analysis of the C-T network, the astragalosides and isoflavonoids of Huangqi may mainly stimulate insulin secretion, improve insulin resistance and promote glucose utilization, but the isoquinoline alkaloids of Huanglian could regulate inflammatory cytokines, promote the utilization of glucose, and improve endocrine and metabolism so as to achieve the synergistic and complementary curative effects of Huangqi and Huanglian.
DM is inevitably accompanied with the development of serious complications, including retinopathy, neuropathy, and nephropathy. Therefore, in clinical practice, hypoglycemic drugs are always prescribed with other drugs for complications, such as antibiotics and antiulcer agents, which may increase the risk of adverse drug events (Held et al., 2017). In our study, this herb combination could not only regulate the insulin secretion and glucose homeostasis but also inflammation and immunity. And the targets were mainly located in retina, pancreatic islet, smooth muscle, immunity-related organ tissues, and whole blood, which were highly associated with Xiao Ke, especially San Xiao syndromes. These results are expected to take full clinical advantage of Huangqi and Huanglian for diabetic complications.
Disruptions in gut microbiota composition and function are increasingly implicated in the pathogenesis of obesity, insulin resistance, and DM (Tremaroli and Bäckhed, 2012). On the other hand, gut microbiota plays a crucial role in TCM therapy by complicated interplay with herb-derived compounds, such as alkaloids, saponins, and polysaccharides (Xu et al., 2017). Therefore, the availability of these active constituents of Huangqi and Huanglian by gut microbiota especially under the DM state may be a critical step toward the emergence of their bioactivities in vivo.
Besides, through SPR assay, we demonstrated that calycosin and coptisine could bind with GAA, which were consistent with the previous reports (Zhou et al., 2012; Zhao et al., 2015). Astragaloside I and AIV were found to exhibit significant interaction with TNF and PTP1B, respectively, suggestive of their potential for diabetic complications. However, instead of AIV, there are very few reports that focus on the pharmacological actions of other astragalosides. Jatrorrhizine and palmatine showed a strong interaction with GLP1R; calycosin and calycosin 7-O-β-D-glucopyranoside were observed to bind with PPARG and INSR, respectively. Therefore, more experiments are anticipated to support our intriguing findings.
Author Contributions
CW and DY conceived of and proposed the idea. SY and JL designed the study. SY, JL, and FZ performed the experiments. SY, JL, WF, JC, and DY participated in data analysis. CW, DY, CP, and HG contributed to writing, revising and proof-reading the manuscript. All authors read and approved the final manuscript.
Conflict of Interest Statement
The authors declare that the research was conducted in the absence of any commercial or financial relationships that could be construed as a potential conflict of interest.
Acknowledgments
We thank Prof. Li P. (Shanxi Agricultural University, China) for OB and OL calculation as well as constructive comments. This work was supported by the National Natural Science Foundation of China (grant No. 81773891, 81322052, and 81274058), the National Great New Drugs Development Project of China (grant No. 2017ZX09301-040), the Beijing Natural Science Foundation (grant No. 7162092). This work was also supported by the National High Technology Research and Development Program of China (863 Program) (grant No. 2013AA093001), and the Taishan Scholars Program, China.
Supplementary Material
The Supplementary Material for this article can be found online at: https://www.frontiersin.org/articles/10.3389/fphar.2017.00694/full#supplementary-material
References
Abrahamsson, H. (1995). Gastrointestinal motility disorders in patients with diabetes mellitus. J. Intern. Med. 237, 403–409. doi: 10.1111/j.1365-2796.1995.tb01194.x
Aguirre, L., Arias, N., Macarulla, M. T., Gracia, A., and Portillo, M. P. (2011). Beneficial effects of quercetin on obesity and diabetes. Open Nutraceuticals J. 4, 189–198. doi: 10.2174/1876396001104010189
Antonetti, D. A., Klein, R., and Gardner, T. W. (2012). Diabetic retinopathy. N. Engl. J. Med. 366, 1227–1239. doi: 10.1056/NEJMra1005073
Bao, S. H., Geng, P. W., Wang, S. H., Zhou, Y. F., Hu, L. F., and Yang, X. Z. (2015). Pharmacokinetics in rats and tissue distribution in mouse of magnoflorine by ultra performance liquid chromatography-tandem mass spectrometry. Int. J. Clin. Exp. Med. 8, 20168–20177.
Chang, W. C., Wu, J. S. B., Chen, C. W., Kuo, P. L., Chien, H. M., Wang, Y. T., et al. (2015). Protective effect of vanillic acid against hyperinsulinemia, hyperglycemia and hyperlipidemia via alleviating hepatic insulin resistance and inflammation in high-fat diet (HFD)-fed rats. Nutrients 7, 9946–9959. doi: 10.3390/nu7125514
Chen, H. Y., Ye, X. L., Cui, X. L., He, K., Jin, Y. N., Chen, Z., et al. (2012). Cytotoxity and antihyperglycemic effect of minor constitunts from Rhizoma Coptis in HepG2 cells. Fitoterapia 83, 67–73. doi: 10.1016/j.fitote.2011.09.014
Chen, W., Miao, Y. Q., Fan, D. J., Yang, S. S., Lin, X., Meng, L. K., et al. (2011). Bioavailabitity study of berberine and the enhancing effects of TPGS on intestinal absorption in rats. AAPS PharmSciTech. 12, 705–711. doi: 10.1208/s12249-011-9632-z
Choi, J. S., Ali, M. Y., Jung, H. A., Oh, S. H., Choi, R. J., and Kim, E. J. (2015). Protein tyrosine phosphatase 1B inhibitory activity of alkaloids from Rhizoma Coptidis and their molecular docking studies. J. Ethnopharmacol. 171, 28–36. doi: 10.1016/j.jep.2015.05.020
Datta, S. K., Kumar, V., Pathak, R., Tripathi, A. K., Ahmed, R. S., Kalra, O. P., et al. (2010). Association of glutathione S-transferase M1 and T1 gene polymorphism with oxidative stress in diabetic and nondiabetic chronic kidney disease. Ren. Fail. 32, 1189–1195. doi: 10.3109/0886022X.2010.517348
Ding, P. L., Chen, L. Q., Lu, Y., and Li, Y. G. (2012). Determination of protoberberine alkaloids in Rhizoma Coptidis by ERETIC 1H NMR method. J. Pharm. Biomed. Anal. 60, 44–50. doi: 10.1016/j.jpba.2011.10.030
Gilson, M. K., Liu, T. Q., Baitaluk, M., Nicola, G., and Hwang, L. (2016). BindingDB in 2015: a public database for medicinal chemistry, computational chemistry and systems pharmacology. Nucleic Acids Res. 44, D1045–D1053. doi: 10.1093/nar/gkv1072
Gu, M., Zhang, S. Y., Zhao, Y. Y., Huang, J. W., Wang, Y. H., Li, Y., et al. (2017). Cycloastragenol improves hepatic steatosis by activating farnesoid X receptor signalling. Pharmacol. Res. 121, 22–32. doi: 10.1016/j.phrs.2017.04.021
Gu, Y. C., Wang, G. J., Pan, G. Y., Fawcett, J. P., A, J. Y., and Sun, J. G. (2004). Transport and bioavailability studies of astragaloside IV, an active ingredient in Radix Astragali. Basic Clin. Pharmacol. Toxicol. 95, 295–298. doi: 10.1111/j.1742-7843.2004.t01-1-pto950508.x
Gupta, R., Sharma, A. K., Dobhal, M. P., Sharma, M. C., and Gupta, R. S. (2011). Antidiabetic and antioxidant potential of β-sitosterol in streptozotocin-induced experimental hyperglycemia. J. Diabetes 3, 29–37. doi: 10.1111/j.1753-0407.2010.00107.x
Han, C. S., Liu, K., Zhang, N., Li, S. W., and Gao, H. C. (2017). Rutin suppresses high glucose-induced ACTA2 and p38 protein expression in diabetic nephropathy. Exp. Ther. Med. 14, 181–186. doi: 10.3892/etm.2017.4509
Held, F., Couteur, D. G. L., Blyth, F. M., Hirani, V., Naganathan, V., Waite, L. M., et al. (2017). Polypharmacy in older adults: association rule and frequent-set analysis to evaluate concomitant medication use. Pharmacol. Res. 116, 39–44. doi: 10.1016/j.phrs.2016.12.018
Huang da, W., Sherman, B. T., and Lempicki, R. A. (2009). Systematic and integrative analysis of large gene lists using DAVID bioinformatics resources. Nat. Protoc. 4, 44–57. doi: 10.1038/nprot.2008.211
Ishiyama-Shigemoto, S., Yamada, K., Yuan, X., Ichikawa, F., and Nonaka, K. (1999). Association of polymorphisms in the β2-adrenergic receptor gene with obesity, hypertriglyceridaemia, and diabetes mellitus. Diabetologia 42, 98–101. doi: 10.1007/s001250051120
International Diabetes Federation (2015). Data from: IFD Diabetes Altas Globally, 7th Edn. Available online at: http://www.diabetesatlas.org
Jung, H. A., Yoon, N. Y., Bae, H. J., Min, B. S., and Choi, J. S. (2008). Inhibitory activities of the alkaloids from Coptidis Rhizoma against aldose reductase. Arch. Pharm. Res. 31, 1405–1412. doi: 10.1007/s12272-001-2124-z
Keiser, M. J., Roth, B. L., Armbruster, B. N., Ernsberger, P., Irwin, J. J., and Shoichet, B. K. (2007). Relating protein pharmacology by ligand chemistry. Nat. Biotechnol. 25, 197–206. doi: 10.1038/nbt1284
Kloft, C., Trame, M. N., and Ritter, C. A. (2016). Systems pharmacology in drug development and therapeutic use – a forthcoming paradigm shift. Eur. J. Pharm. Sci. 93, 1–3. doi: 10.1016/j.ejps.2016.07.014
Kootte, R. S., Vrieze, A., Holleman, F., Dallinga-Thie, G. M., Zoetendal, E. G., de Vos, W. M., et al. (2012). The therapeutic potential of manipulating gut microbiota in obesity and type 2 diabetes mellitus. Diabetes Obes. Metab. 14, 112–120. doi: 10.1111/j.1463-1326.2011.01483.x
Kuhn, M., Szklarczyk, D., Franceschini, A., von Mering, C., Jensen, L. J., and Bork, P. (2012). STITCH 3: zooming in on protein-chemical interactions. Nucleic Acids Res. 40, 876–880. doi: 10.1093/nar/gkr1011
Li, H. L., Zhang, W. D., Zhang, C., Liu, R. H., Wang, X. W., Wang, X. L., et al. (2006). Bioavailability and pharmacokinetics of four active alkaloids of traditional Chinese medicine Yanhuanglian in rats following intravenous and oral administration. J. Pharm. Biomed. Anal. 41, 1342–1346. doi: 10.1016/j.jpba.2006.03.029
Liu, H. S., Shi, H. L., Huang, F., Peterson, K. E., Wu, H., Lan, Y. Y., et al. (2016). Astragaloside IV inhibits microglia activation via glucocorticoid receptor mediated signaling pathway. Sci. Rep. 6:19137. doi: 10.1038/srep19137
Liu, H. Z., Bai, J. M., Weng, X. G., Wang, T., and Li, M. J. (2014). Amelioration of insulin resistance in rat cells by Astragalus polysaccharides and associated mechanisms. Exp. Ther. Med. 7, 1599–1604. doi: 10.3892/etm.2014.1626
Liu, W. H., Liu, P. Q., Tao, S., Deng, Y. H., Li, X. J., Lan, T., et al. (2008). Berberine inhibits aldose reductase and oxidative stress in rat mesangial cells cultured under high glucose. Arch. Biochem. Biophys. 475, 128–134. doi: 10.1016/j.abb.2008.04.022
Luo, H., Chen, J., Shi, L. M., Mikailov, M., Zhu, H., Wang, K. J., et al. (2011). DRAR-CPI: a server for identifying drug repositioning potential and adverse drug reactions via the chemical-protein interactome. Nucleic Acids Res. 39, W492–W498. doi: 10.1093/nar/gkr299
Lv, L., Wu, S. Y., Wang, G. F., Zhang, J. J., Pang, J. X., Liu, Z. Q., et al. (2010). Effect of astragaloside IV on hepatic glucose-regulating enzymes in diabetic mice induced by a high-fat diet and streptozotocin. Phytother. Res. 24, 219–224. doi: 10.1002/ptr.2915
Ma, C., Wang, L., and Xie, X. Q. (2011). GPU accelerated chemical similarity calculation for compound library comparison. J. Chem. Inf. Model. 51, 1521–1527. doi: 10.1021/ci1004948
Ma, P. K., Wei, B. H., Cao, Y. L., Miao, Q., Chen, N., Guo, C. E., et al. (2017). Pharmacokinetics, metabolism, and excretion of cycloastragenol, a potent telomerase activator in rats. Xenobiotica 47, 526–537. doi: 10.1080/00498254.2016.1204568
Ma, X. Q., Shi, Q., Duan, J. A., Dong, T. T. X., and Tsim, K. W. K. (2002). Chemical analysis of Radix Astragali (Huangqi) in China: a comparison with its adulterants and seasonal variations. J. Agric. Food Chem. 50, 4861–4866. doi: 10.1021/jf0202279
Newman, J. D., Rockman, C. B., Kosiborod, M., Guo, Y., Zhong, H., Weintraub, H. S., et al. (2017). Diabetes mellitus is a coronary heart disease risk equivalent for peripheral vascular disease. Am. Heart J. 184, 114–120. doi: 10.1016/j.ahj.2016.09.002
Nie, Y. N., Li, S. Y., Yi, Y. E., Su, W. L., Chai, X. L., Jia, D. X., et al. (2014). Effects of astragalus injection on the TGFβ/Smad pathway in the kidney in type 2 diabetic mice. BMC Complement. Altern. Med. 14:148. doi: 10.1186/1472-6882-14-148
Pang, B., Yu, X. T., Zhou, Q., Zhao, T. Y., Wang, H., Gu, C. J., et al. (2015). Effect of Rhizoma Coptidis (Huang Lian) on treating diabetes mellitus. Evid. Based Complement. Alternat. Med. 2015:921416. doi: 10.1155/2015/921416
Patel, M. B., and Mishra, S. M. (2012). Magnoflorine from Tinospora cordifolia stem inhibits α-glucosidase and is antiglycemic in rats. J. Funct. Foods 4, 79–86. doi: 10.1016/j.jff.2011.08.002
Qiu, G. Z., Tian, W., Huan, M., Chen, J. L., and Fu, H. T. (2017). Formononetin exhibites anti-hyperglycemic activity in alloxan-induced type 1 diabetic mice. Exp. Biol. Med. 242, 223–230. doi: 10.1177/1535370216657445
Ru, J. L., Li, P., Wang, J. N., Zhou, W., Li, B. H., Huang, C., et al. (2014). TCMSP: a database of systems pharmacology for drug discovery from herbal medicines. J. Cheminform. 6, 1498–1504. doi: 10.1186/1758-2946-6-13
Sakaguchi, M., Isono, M., Isshiki, K., Sugimoto, T., Koya, D., and Kashiwagi, A. (2006). Inhibition of mTOR signaling with rapamycin attenuates renal hypertrophy in the early diabetic mice. Biochem. Biophys. Res. Commun. 340, 296–301. doi: 10.1016/j.bbrc.2005.12.012
Shen, P., Liu, M. H., Ng, T. Y., Chan, Y. H., and Yong, E. L. (2006). Differential effects of isoflavones, from Astragalus membranaceus and Pueraria Thomsonii, on the activation of PPARalpha, PPARgamma, and adipocyte differentiation in vitro. J. Nutr. 136, 899–905.
Smoot, M. E., Ono, K., Ruscheinski, J., Wang, P. L., and Ideker, T. (2011). Cytoscape 2.8: new features for data integration and network visualization. Bioinformatics 27, 431–432. doi: 10.1093/bioinformatics/btq675
Sun, Y., Zhang, Y., and Xiong, Y. T. (2015). GSTM1 and GSTT1 null genotype and diabetic retinopathy: a meta-analysis. Int. J. Clin. Exp. Med. 8, 1677–1683.
Taniguchi, C. M., Emanuelli, B., and Kahn, C. R. (2006). Critical nodes in signaling pathways: insights into insulin action. Nat. Rev. Mol. Cell Biol. 7, 85–96. doi: 10.1038/nrm1837
The State Pharmacopoeia Commission of China (2015). Pharmacopoeia of the People's Republic of China, Part I, Beijing: Chemical Industry Press, 302–303.
Tong, X. L., Zhao, L. H., Lian, F. M., Zhou, Q., Xia, L., Zhang, J. C., et al. (2011). Clinical observations on the dose-effect relationship of Gegen Qin Lian Decoction on 54 out-patients with type 2 diabetes. J. Tradit. Chin. Med. 31, 56–59. doi: 10.1016/S0254-6272(11)60013-7
Tremaroli, V., and Bäckhed, F. (2012). Functional interactions between the gut microbiota and host metabolism. Nature 489, 242–249. doi: 10.1038/nature.11552
Wang, X., Shen, Y. H., Wang, S. W., Li, S. L., Zhang, W. L., Liu, X. F., et al. (2017). PharmMapper 2017 update: a web server for protential drug target identification with a comprehensive target pharmacophore database. Nucleic Acids Res. 45, W356–W360. doi: 10.1093/nar/gkx374
Whirl-Carrillo, M., McDonagh, E. M., Hebert, J. M., Gong, L., Sangkuhl, K., Thorn, C. F., et al. (2012). Pharmacogenomics knowledge for personalized medicine. Clin. Pharmacol. Ther. 92, 414–417. doi: 10.1038/clpt.2012.96
Wu, C. L., Orozco, C., Boyer, J., Leglise, M., Goodale, J., Batalov, S., et al. (2009). BioGPS: an extensible and customizable portal for querying and organizing gene annotation resources. Genome Biol. 10:R130. doi: 10.1186/gb-2009-10-11-r130
Wu, J. M., Ke, X., Ma, N., Wang, W., Fu, W., Zhang, H. C., et al. (2016). Formononetin, an active compound of Astragalus membranaceus (Fisch) Bunge, inhibits hypoxia-induced retinal neovascularization via the HIF-1α/VEGF signaling pathway. Drug Des. Devel. Ther. 10, 3071–3081. doi: 10.2147/DDDT.S114022
Wu, T., Annie Bligh, S. W., Gu, L. H., Wang, Z. T., Liu, H. P., Cheng, X. M., et al. (2005). Simultaneous determination of six isoflavonoids in commercial Radix Astragali by HPLC-UV. Fitoterapia 76, 157–165. doi: 10.1016/j.fitote.2004.11.006
Xia, Z. S., Du, Z. C., Deng, J. G., Li, Y., Wei, L. Y., and Xie, L. H. (2016). Chinese medicine treatment of diabetes drugs based on analysis of large data patterns. World Chin. Med. 11, 2223–2230. doi: 10.3969/j.issn.1673-7202.2016.11.005
Xie, W. D., Zhao, Y. N., and Zhang, Y. O. (2011). Traditional Chinese medicines in treatment of patients with type 2 diabetes mellitus. Evid. Based Complement. Alternat. Med. 2011:726723. doi: 10.1155/2011/726723
Xu, A. M., Wang, H. B., Hoo, R. L. C., Sweeney, G., Vanhoutte, P. M., Wang, Y., et al. (2009). Selective elevation of adiponectin production by the natural compounds derived from a medicinal herb alleviates insulin resistance and glucose intolerance in obese mice. Endocrinology 150, 625–633. doi: 10.1210/en.2008-0999
Xu, J., Chen, H. B., and Li, S. L. (2017). Understanding the molecular mechanisms of the interplay between herbal medicines and gut microbiota. Med. Res. Rev. 2016, 1–46. doi: 10.1002/med.21431
Xu, M. J., Wang, L., Chang, X., Zhang, Z., and Huang, R. L. (2015). Clinical observations on the treatment of Huangqi San on diabetic cardiomyopathy patients. China Prac. Med. 10, 169–170. doi: 10.14163/j.cnki.11-5547/r.2015.18.109
Xu, X., Zhang, W. X., Huang, C., Li, Y., Yu, H., Wang, Y. H., et al. (2012). A novel chemometric method for prediction of human oral bioavailability. Int. J. Mol. Sci. 13, 6964–6982. doi: 10.3390/ijms13066964
Xu, Y. H., Feng, L., Wang, S. S., Zhu, Q., Zheng, Z. G., Xiang, P., et al. (2011). Calycosin protects HUVECs from advanced glycation end products-induced macrophage infiltration. J. Ethnopharmacol. 137, 359–370. doi: 10.1016/j.jep.2011.05.041
Ye, H., Ye, L., Kang, H., Zhang, D. F., Tao, L., Tang, K. L., et al. (2011). HIT: linking herbal active ingredients to targets. Nucleic Acids Res. 39, 1055–1059. doi: 10.1093/nar/gkq1165
Yu, J. G., Guo, J. M., Zhu, K. Y., Tao, W. W., Chen, Y. Y., Liu, P., et al. (2017). How impaired efficacy happened between Gancao and Yuanhua: compounds, targets and pathways. Sci. Rep. 7:3828. doi: 10.1038/s41598-017-03201-1
Yue, S. J., Xin, L. T., Fan, Y. C., Li, S. J., Tang, Y. P., Duan, J. A., et al. (2017). Herb pair Danggui-Honghua: mechanisms underlying blood stasis syndrome by system pharmacology approach. Sci. Rep. 7:40318. doi: 10.1038/srep40318
Zhang, H., Tan, C. E., Wang, H. Z., Xue, S. B., and Wang, M. Q. (2010). Study on the history of traditional Chinese medicine to treat diabetes. Eur. J. Integr. Med. 2, 41–46. doi: 10.1016/j.eujim.2010.02.004
Zhang, X., Zhao, Y. F., Zhang, M. H., Pang, X. Y., Xu, J., Kang, C. Y., et al. (2012). Structural changes of gut microbiota during berberine-mediated prevention of obesity and insulin resistance in high-fat diet-fed rats. PLoS ONE 7:e42529. doi: 10.1371/journal.pone.0042529
Zhang, Y. H., Zhang, L. M., Liu, X. B., Gong, H., Ren, W. C., and Ma, W. (2013). Content determination of Astragalus polysaccaride from Astragalus in different regions. Zhongyiyao Xinxi 30, 69–71.
Zhang, Y. L., and Liu, D. M. (2011). Flavonol kaempferol improves chronic hyperglycemia-impaired pancreatic beta-cell viability and insulin secretory function. Eur. J. Pharmacol. 670, 325–332. doi: 10.1016/j.ejphar.2011.08.011
Zhang, Y. Q., Mao, X., Guo, Q. Y., Lin, N., and Li, S. (2016). Network pharmacology-based approaches capture essence of Chinese herbal medicines. Chin. Herb. Med. 8, 107–116. doi: 10.1016/S1674-6384(16)60018-7
Zhao, H. D., Zhang, Y. P., Guo, Y., and Shi, S. Y. (2015). Identification of major α-glucosidase inhibitors in Radix Astragali and its human microsomal metabolites using ultrafiltration HPLC-DAD-MSn. J. Pharm. Biomed. Anal. 104, 31–37. doi: 10.1016/j.jpba.2014.09.029
Zhao, M., Zhang, Z. F., Ding, Y., Wang, J. B., and Li, Y. (2012). Astragalus polysaccharide improves palmitate-induced insulin resistance by inhibiting PTP1B and NF-κB in C2C12 myotubes. Molecules 17, 7083–7092. doi: 10.3390/molecules17067083
Zhou, H., Li, H. L., Zheng, Z., Song, F. R., Xing, J. P., Liu, Z. Q., et al. (2012). Screening for α-glucosidase inhibitors from coptidis-rehmanniae herb couple by using ultrafiltration liquid chromatography/mass spectrometry. J. Liq. Chrom. Rel. Technol. 35, 1–14. doi: 10.1080/10826076.2011.593225
Zhou, W., Wang, J. N., Wu, Z. Y., Huang, C., Lu, A. P., and Wang, Y. H. (2016). Systems pharmacology exploration of botanic drug pairs reveals the mechanism for treating different disease. Sci. Rep. 6:36985. doi: 10.1038/srep36985
Zhu, F., Shi, Z., Qin, C., Tao, L., Liu, X., Xu, F., et al. (2012). Therapeutic target database updata 2012: a resource for facilitating target-oriented drug discovery. Nucleic Acids Res. 40, 1128–1136. doi: 10.1093/nar/gkr797
Zhu, Z. Y., Zhang, J. Y., Chen, L. J., Liu, X. C., Liu, Y., Wang, W. X., et al. (2014). Comparative evaluation of polysaccharides isolated from Astragalus, oyster mushroom, and yacon as inhibitors of α-glucosidase. Chin. J. Nat. Med. 12, 290–293. doi: 10.1016/S1875-5364(14)60056-X
Zou, F., Mao, X. Q., Wang, N., Liu, J., and Ou-Yang, J. P. (2009). Astragalus polysaccharides alleviates glucose toxicity and restores glucose homeostasis in diabetic states via activation of AMPK. Acta Pharmacol. Sin. 30, 1607–1615. doi: 10.1038/aps.2009.168
Keywords: Huangqi, Huanglian, synergistic mechanism, diabetes, system pharmacology
Citation: Yue S-J, Liu J, Feng W-W, Zhang F-L, Chen J-X, Xin L-T, Peng C, Guan H-S, Wang C-Y and Yan D (2017) System Pharmacology-Based Dissection of the Synergistic Mechanism of Huangqi and Huanglian for Diabetes Mellitus. Front. Pharmacol. 8:694. doi: 10.3389/fphar.2017.00694
Received: 07 August 2017; Accepted: 19 September 2017;
Published: 05 October 2017.
Edited by:
Honglin Li, East China University of Science and Technology, ChinaReviewed by:
E-Hu Liu, China Pharmaceutical University, ChinaYu-Ping Tang, Nanjing University of Chinese Medicine, China
Shuo Gu, Massachusetts Institute of Technology, United States
Copyright © 2017 Yue, Liu, Feng, Zhang, Chen, Xin, Peng, Guan, Wang and Yan. This is an open-access article distributed under the terms of the Creative Commons Attribution License (CC BY). The use, distribution or reproduction in other forums is permitted, provided the original author(s) or licensor are credited and that the original publication in this journal is cited, in accordance with accepted academic practice. No use, distribution or reproduction is permitted which does not comply with these terms.
*Correspondence: Chang-Yun Wang, Y2hhbmd5dW5Ab3VjLmVkdS5jbg==
Dan Yan, eWQyNzdAMTI2LmNvbQ==