- 1Fundación para el Fomento de la Investigación Sanitaria y Biomédica de la Comunidad Valenciana, Valencia, Spain
- 2Red de Investigación en Servicios de Salud en Enfermedades Crónicas, Valencia, Spain
Objective: To estimate drug exposure, Proportion of Days Covered (PDC) and percentage of patients with PDC ≥ 80% from a cohort of atrial fibrillation patients initiating oral anticoagulant (OAC) treatment. We employed three different approaches to estimate PDC, using either data from prescription and dispensing (PD cohort) or two common designs based on dispensing information only, requiring at least one (D1) or at least two (D2) refills for inclusion in the cohorts. Finally, we assessed the impact of adherence on health outcomes according to each method.
Methods: Population-based retrospective cohort of all patients with Non Valvular Atrial Fibrillation (NVAF), who were newly prescribed acenocoumarol, apixaban, dabigatran or rivaroxaban from November 2011 to December 2015 in the region of Valencia (Spain). Patients were followed for 12 months to assess adherence using three different approaches (PD, D1 and D2 cohorts). To analyze the relationship between adherence (PDC ≥ 80) defined according to each method of calculation and health outcomes (death for any cause, stroke or bleeding) Cox regression models were used. For the identification of clinical events patients were followed from the end of the adherence assessment period to the end of the available follow-up period.
Results: PD cohort included all patients with an OAC prescription (n = 38,802), D1 cohort excluded fully non-adherent patients (n = 265) and D2 cohort also excluded patients without two refills separated by 180 days (n = 2,614). PDC ≥ 80% ranged from 94% in the PD cohort to 75% in the D1 cohort. Drug exposure among adherent (PDC ≥ 80%) and non-adherent (PDC < 80%) patients was different between cohorts. In adjusted analysis, high adherence was associated with a reduced risk of death [Hazard Ratio (HR): from 0.82 to 0.86] and (except in the PD cohort) the risk for ischemic stroke (HR: from 0.61 to 0.64) without increasing the risk of bleeding.
Conclusion: Common approaches to assess adherence using measures based on days’ supply exclude groups of non-adherent patients and, also, misattribute periods of doctors’ discontinuation to patient non-adherence, misestimating adherence overall. Physician-initiated discontinuation is a major contributor to reduced OAC exposure. When using the PDC80 threshold, very different groups of patients may be classified as adherent or non-adherent depending on the method used for the calculation of days’ supply measures. High adherence and high exposure to OAC treatment in NVAF patients is associated with better health outcomes.
Introduction
While it seems obvious that patients need to take their medications to benefit from them as shown in clinical trials, several studies and systematic reviews have analyzed the association between adherence to evidence-based pharmacotherapy and outcomes in chronic diseases such as coronary ischemic disease (McDermott et al., 1997; Rasmussen et al., 2007; Bitton et al., 2013; Choudhry et al., 2014), hyperlipidemia (De Vera et al., 2014; Karlsson et al., 2018), diabetes (Hood et al., 2009; Khunti et al., 2017), hypertension (Dragomir et al., 2010; Xu et al., 2017), osteoporosis (Nikitovic et al., 2010) and other conditions (DiMatteo et al., 2002; Simpson et al., 2006). Not surprisingly, virtually all the published evidence has shown that high adherence to appropriate treatment reduces mortality, several adverse clinical events, readmissions, healthcare utilization and costs. Oral anticoagulant (OAC) treatment in atrial fibrillation (AF) patients is not an exception and several studies (Deitelzweig et al., 2013; Shore et al., 2014; Thorne et al., 2014; Spivey et al., 2015; Alberts et al., 2016; Yao et al., 2016; Borne et al., 2017; Deshpande et al., 2018) have shown the valuable impact of high levels of adherence to OAC medication on various clinical outcomes, particularly the prevention of cardioembolic stroke.
Although there are several methods for assessing adherence (metabolite or biologic marker detection, self-reporting, pill count, electronic monitoring devices and other), retrospective studies based on data from refill databases have been favored in recent years because health organizations have extensively introduced health information systems with a unique patient identifier and, also, because this methodology has some advantages regarding the alternatives: it is unobtrusive (thus patient sensitization is avoided), objective (it produces quantifiable and reproducible data for each individual), and pragmatic (it is easy to use and analyze, inexpensive and applicable to different settings and drugs) (Rudd, 1979; Steiner and Prochazka, 1997; Schneeweiss and Avorn, 2005; Suissa and Garbe, 2007; Wettermark et al., 2013).
Despite the presence of an important methodological heterogeneity, studies linking adherence and health outcomes using refill databases usually adopt a common analytical approach: patients are categorized as adherent or non-adherent according to a threshold on medication days’ supply over a definite period, and the relationship between these two groups (patients under and over the threshold) and outcomes is evaluated. Generally, in the literature on adherence to OAC (Shore et al., 2014; Alberts et al., 2016; Yao et al., 2016; Borne et al., 2017; Deshpande et al., 2018) and to other chronic treatments, patients are defined as adherent when they exceed a cut-off point of 80% in the Proportion of Days Covered (PDC) or in other analogous adherence measures based on days’ supply, as the Medication Possession Ratio (MPR).
The 80% threshold (PDC80) originated in blood pressure studies carried out in the 70s of the last century (Sackett et al., 1975), but does not have an empirical basis for dichotomizing adherence to OAC treatment in AF patients, nor in many other conditions (Watanabe et al., 2013; Lo-Ciganic et al., 2015; Gellad et al., 2017; Morrison et al., 2017; Stauffer et al., 2017) [although some studies give some support to the 80% threshold in some conditions (Hansen et al., 2009; Karve et al., 2009)], introducing an element of arbitrariness with regard to the consideration of “sufficient” adherence and also to the interpretation of the relationship between adherence and outcomes.
Moreover, adherence measures based on days’ supply such as PDC or MPR can be calculated in several ways (Steiner and Prochazka, 1997; Andrade et al., 2006; Nikitovic et al., 2010; Vrijens et al., 2012; Arnet et al., 2016; Krueger et al., 2018). This in turn generates a huge variability in the real exposure to treatment of patients categorized as “adherent” or “non-adherent” using an apparently equal cut-off point value and, therefore, in the meaning of a given threshold or in the relationships between adherence (defined as PDC ≥ 80% independently of the method used for its calculation) and outcomes.
Differences in the design of adherence studies and in the operational definitions employed in these studies can importantly skew the adherence estimates based on days’ supply. In this way, the relationship between adherence thresholds and outcomes may be more sensitive to the way in which PDC (or MPR) has been calculated than to the actual patient exposure to the medication. These differences commonly arise from: (1) the exclusion (total or partial) from adherence studies of non-adherent patients (e.g., designs where two or more prescriptions filled or a minimum of covered days are required as an inclusion criteria) (Crivera et al., 2015), (2) the inclusion of patients more likely to be adherent, e.g., studies including experienced users (in the field of OAC, those can include long term users of an index drug, or switchers to a different OAC drug) (Ray, 2003; Danaei et al., 2012; Maciejewski et al., 2013; Lund et al., 2015; Li et al., 2018), (3) the definition of the index date (the date of first prescription, filled or not, or alternatively the date of the first prescription filled), (4) the censoring of cases at the date of the last refill, avoiding the use of a fixed time-window (e.g., 12 months) for follow-up and calculation of days’ supply based estimates (Kozma et al., 2013), (5) the censoring of cases (or the classification as non-adherent) when patients switch to a different treatment of the same class (Zhu et al., 2017), (6) the consideration (or not) of stockpiling (Greevy et al., 2011), (7) the consideration (or not) of immeasurable time (Dong et al., 2017; Palmaro et al., 2017), and (8) some other variants such as the estimation of the daily dose, the duration of the look-back period (Riis et al., 2015; Roberts et al., 2015; Nakasian et al., 2017), or the censoring (or not) of deceased patients or those who lose coverage.
Finally, in most adherence studies the information about doctors’ prescription is absent from refill databases. This is especially relevant because the concept of adherence refers to the “extent to which patients take medications as prescribed by their health care providers” (Sabaté, 2003; Osterberg and Blaschke, 2005; Cramer et al., 2008). In absence of a doctors’ prescription information, studies based on refill databases do not capture patients who do not fill any prescription (therefore, they may overestimate adherence as fully non-adherent patients are not accounted for) or may misclassify some patients as non-adherent when in fact the gap in days’ supply is caused by treatment discontinuation decided by their physicians (in this case, these studies may underestimate adherence due to the erroneous classification as non-adherent of patients not on treatment anymore).
The aims of this study, using a retrospective cohort of all patients with Non Valvular Atrial Fibrillation (NVAF) newly prescribed an OAC drug in the Valencia Region during nearly 5 years, are: (1) to estimate the variability in days’ supply adherence measures (PDC and PDC80) using a doctors’ prescription-based design and two common dispensation-based designs regarding real drug exposure, and (2) to determine the impact of adherence (defined as PDC ≥ 80%) on health outcomes according to each method of calculation of PDC.
Materials and Methods
Study Design
Population-based retrospective cohort of all patients aged 40 and over with NVAF, who were newly prescribed acenocoumarol, apixaban, dabigatran or rivaroxaban from November 2011 to December 2015 in the region of Valencia (Spain). Patients were followed for 12 months to assess adherence using three different approaches, and from the end of the adherence assessment period to the end of the available follow-up period to analyze the relationship between adherence (PDC ≥ 80, defined according to each method of calculation) and health outcomes (death, stroke or bleeding).
Population and Setting
The study was conducted in the population covered by the Valencia Health System (VHS) in Spain. The VHS, part of the Spanish National Health System (sNHS), is funded and mostly provided by the Valencia Government, free at the point of care except for some co-payments for ambulatory medication, and almost universal, covering about 97% of the region’s population (approximately five million inhabitants). We constructed a main cohort comprising all patients with a diagnosis of AF or atrial flutter (ICD-9-CM: 427.31 and 427.32) who started treatment with oral anticoagulants (OACs) (acenocoumarol, apixaban, dabigatran or rivaroxaban) for the prevention of thromboembolic events between November 2011 [date of the market launch of first Non Vitamin K Antagonist Oral Anticoagulant (NOAC)] and December 2015 (Figure 1). The few users of warfarin, phenprocoumon or fluindione, mainly non-residents, were not included.
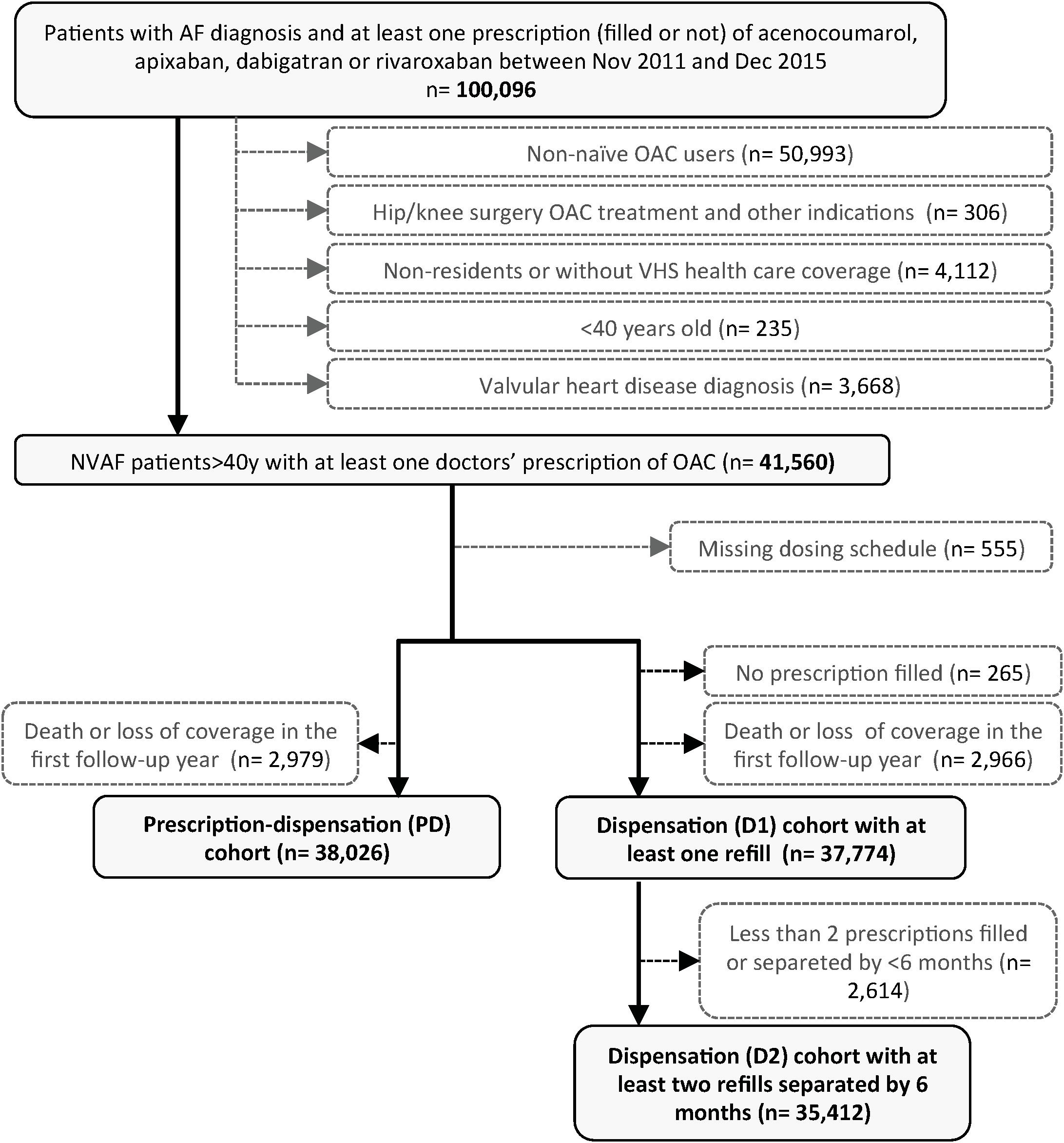
FIGURE 1. Study flow chart. AF, atrial fibrillation; NVAF, non-valvular atrial fibrillation; OAC, oral anticoagulants; VHA, valencia health agency.
One year of look-back period was used to define the baseline characteristics of the population and for excluding prevalent OAC users. We defined new users as those patients without any anticoagulant treatment in the 12 months preceding the first doctor’s prescription. We excluded patients with concomitant valvular heart disease (ICD-9-CM: 394.x–397.x, 398.9, 42.4x, V42.2, V43.3, 35.1x, 35.2x), patients with limitations of follow-up (which were people without pharmaceutical coverage by VHS, mainly certain Spanish government employees whose prescriptions are reimbursed by civil servant service insurance mutualities, and thus not included in the electronic records of the VHS), and patients not registered in the municipal census, such as non-residents or temporary residents. Finally, we excluded a small group of patients without information about the prescribed dose because limitations to calculate adherence, and patients who died or lost coverage in the 1st year of follow-up (used to calculate PDC and to classify patients as adherent or non-adherent) because for these patients there was no follow-up available to assess clinical outcomes in the year following their adherence categorization.
Data Sources
The main source of data was the VHS ambulatory electronic medical record (EMR) that includes information about diagnoses, personal medical history, laboratory test results, lifestyle factors, as well as information about both physician prescriptions and dispensations. The information on hospitalizations was based on the Minimum Basic Dataset (MBDS) at hospital discharge, a synopsis of clinical and administrative information on all hospital discharges, including diagnoses and procedures. The Population Information System (SIP) provides dynamic information on the population covered by the VHS and registers some sociodemographic characteristics, including the geographical/contextual situation of each person and dates and causes of VHS loss of coverage, including death. All these data sources can be linked at an individual level through a unique patient anonymized identifier. A detailed description of source of data is found elsewhere (Sanfélix-Gimeno et al., 2015).
Adherence Measures
We constructed three cohorts according to the different selection criteria used to calculate alternative forms of PDC (Table 1). The first cohort, called “prescription-dispensation cohort” (PD cohort) corresponds to the formal concept of adherence (“extent to which patients take medications as prescribed by their health care providers”) and estimates days’ supply (the PDC numerator) as days covered by filled medication from the first doctors’ prescription date (index date) to the end of follow-up days provided they are covered by a medical prescription. Days not covered by a medical prescription were censored. Therefore, a patient who has medical prescriptions for 1 year of treatment and does not fill any prescription will have a PDC = 0, while a patient who fills a new treatment for 1 month from the medical prescription and his/her doctor discontinues treatment at the end of that month, will have a PDC of 100% (corresponding to one prescription fully covered with the dispensed treatment).
The second cohort, called “dispensation cohort with at least one refill” (D1 cohort), represents the most common approach to calculate adherence when information on doctors’ prescription is not available. Patients were required to fill at least one prescription to be included and the index date is the date of this first refill (not the date of the first doctors’ prescription as in the PD cohort). Therefore patients who did not fill any prescription were excluded from this cohort. Days’ supply were estimated as the days covered by filled medication but doctor’s discontinuation of the treatment cannot be captured. Therefore, completely non-adherent patients (who had a PDC = 0 in the PD cohort) were not included in the D1 cohort, while patients whose doctor discontinues the prescription were considered non-compliant (non-adherent). However and despite these inaccuracies regarding the adherence to the medication as prescribed by the doctor, this approach provides a very good proxy of the total patient drug exposure in a period (regardless of whether the doctor maintains the treatment or not and although the period captured may not really correspond to the real beginning of treatment initiation according to the doctors’ first prescription).
The third cohort, called “dispensation cohort with at least two refills” (D2 cohort), corresponds to the definition of OAC adherence used by the Pharmacy Quality Alliance (PQA) (Crivera et al., 2015) that requires at least two prescription refills of OAC with at least 180-days between refills as inclusion criterion for adherence studies, and has been largely used in industry-funded OAC studies (Alberts et al., 2016; McHorney et al., 2016, 2017, 2018; Coleman et al., 2017). Patients with only one refill during the follow-up or who do not have two prescription fills separated by at least 6 months were excluded from the D2 cohort. Therefore this form of PDC estimation excludes by definition patients with short periods of exposure during the follow-up period (or low-adherent patients). As in D1 cohort, days’ supply were calculated as days covered by filled medication from the date of the first refill to the end of the fixed time-window of follow-up.
Summarizing, PD cohort included all patients with an OAC prescription. From those, D1 Cohort included patients who filled at least one prescription, therefore excluding fully non-adherent patients. D2 Cohort included only patients with at least two refills separated by at least 180 days. In the PD cohort, PDC was calculated as the PDC with filled medication out of all days covered by a medical prescription within the 12-month follow-up. In the D1 and D2 cohorts, PDC was calculated as the PDC by filled medication out of the entire duration of the 12-month follow-up regardless of medical prescription coverage.
In all three cohorts, we used a fixed time-window of 12-months from the respective index date as follow-up for adherence assessment (PDC denominator). Cases were censored in case of death or disenrollment, but no other reason for censoring (including switching) was allowed. For the PD cohort, only days under treatment (periods covered with doctors prescriptions) were considered as PDC denominator. Immeasurable time such as hospitalization days were not considered, and stockpiling was allowed for a maximum of 2 months. Days of treatment were always calculated according to doctors’ dosing instructions accompanying the respective prescription (therefore, patients prescribed a daily dose of 110 mg × 2 or 150 mg × 2 who fill a package of 60 tablets, have 30 days of treatment in both cases).
Outcomes
The pre-specified effectiveness outcomes were mortality for any cause, hospital admission for thromboembolic stroke and hospital admission for gastrointestinal bleeding or intracranial hemorrhage. Only principal discharge diagnoses based on ICD9CM (see Supplementary Table S1 for coding on clinical outcomes) were used to define endpoints. Out-of-hospital mortality was collected from the SIP system and the region’s mortality registry. All outcomes were analyzed separately and only the first event was considered for analysis. Patients were followed from the end of the period used to estimate adherence until the relevant event, health system disenrollment, death, or end of follow-up (Dec 2016 for death, and Dec 2015 for stroke or bleeding), whichever came first. All outcomes were analyzed by intention-to-treat approach, analyzing patients in the group of the first OAC they received (index OAC), regardless of whether a switch to another OAC had occurred during the follow-up.
Covariates
We considered all available factors potentially related to the risk of thromboembolic events and bleeding. These included demographic and clinical characteristics, and healthcare resource utilization in the preceding 12 months (Table 2). Comorbidity was defined as the presence of an active diagnosis of the particular condition in the EMR within a 12-month period preceding the index date (see Supplementary Table S1 for details on definitions of comorbidities). Concomitant medication (NSAID and antiplatelet) was defined as medication dispensed at least once during the 3-month pre-index period. Based on comorbidity information, concomitant medication and age, we calculated relevant patient-level risk scores of stroke (CHADS2, CHA2DS2-VASC) and bleeding (HAS-BLED). We also included as covariates some specific events (ischemic stroke, bleeding) occurred during the follow-up year used to assess adherence.
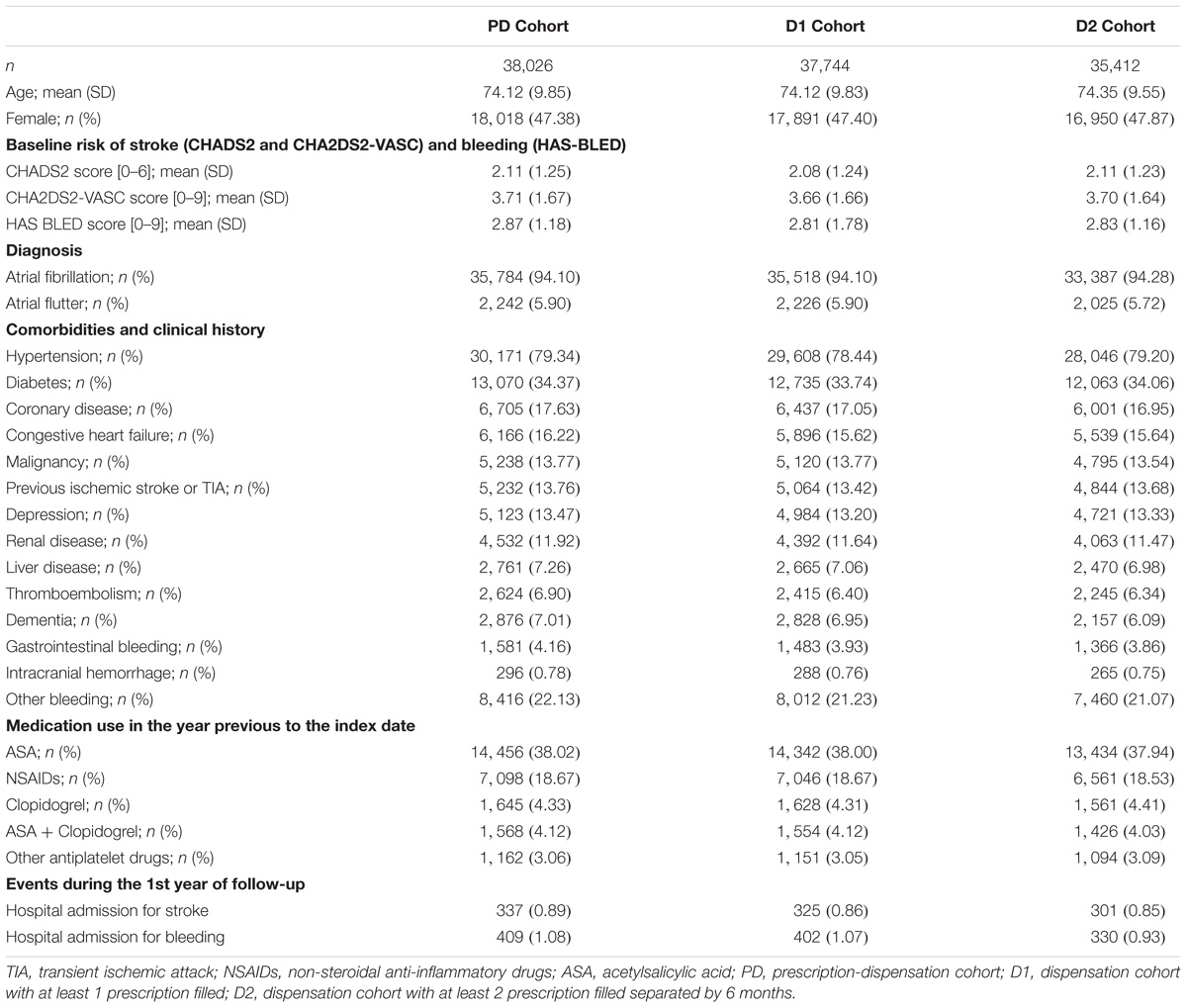
TABLE 2. Patient characteristics in the Prescription-Dispensation (PD) cohort and in the Dispensation-only cohorts with at least one (D1) or two (D2) prescriptions filled as inclusion criteria.
Ethics
The study protocol, observational in design and using retrospective anonymized non-identifiable data transferred from the Valencia Ministry of Health to the research team according to the Spanish laws and institutional requirements, was approved by the Ethics Committee for Clinical Research of the General Directorate of Public Health and the Centre for Public Health Research (CEIC DGSP-CSISP, March 5, 2014).
Statistical Analysis
First, we presented the study population baseline characteristics as means for continuous variables and frequencies for categorical variables for the three cohorts. Second, we estimated crude PDC and PDC80 for each cohort (and for each index drug within each cohort). Third, for each cohort, we described the mean number of days’ supply of medication as a measure of real drug exposure during the 1st year of follow-up (see Table 1 for definitions) for all patients, for adherent patients (PDC ≥ 80) and for non-adherent patients (PDC < 80). In the case of D1 and D2 cohorts, we also estimated the mean number of days’ supply for patients excluded from the respective cohort. Fourth, we compared the classification of patients as adherent or non-adherent among cohorts and also the proportion of patients with ≥80% of the follow-up days covered by medication.
Next, the incidence of death, stroke and hemorrhagic events was described using crude rates per 100 person-years along with 95% CIs separately for each outcome and for each cohort. Finally, we used Cox proportional hazards regression models (adjusted for baseline sociodemographic and clinical characteristics, as well as for non-fatal clinical events occurred during the follow-up period used for calculating adherence) to assess the relationship between adherence (PDC ≥ 80) and health outcomes (death, hospitalization for stroke and hospitalization for bleeding). Patients enrolled from January 2014 (for stroke and bleeding) or January 2015 (for death) were excluded from the Cox models because they did not have a 2nd year of follow-up for the identification of health outcomes.
All statistical analyses were conducted using STATA 14® (StataCorp, College Station, TX), and the 5% level of significance was considered.
Results
Baseline patient characteristics of the three cohorts were similar (Table 2), not surprisingly as the three cohorts share most of their patients. Mean age was 74 years, slightly less than a half of the patients were women, CHA2DS2-VASC score was 3.7 and HAS-BLED score 2.8. Comorbidities were those expected in this population, with a high prevalence of hypertension (79%), diabetes (34%), coronary disease (17%), heart failure (16%) and previous ischemic stroke or transient ischemic attack (14%). Almost 1% of the patients had one hospital admission for stroke and another 1% for a bleeding episode during the year employed to calculate adherence.
Mean PDC ranged from 96.2% for the PD cohort to 88.5 and 84.7% for D2 and D1 cohorts, respectively (Table 3). The percentage of patients with PDC ≥ 80 ranged from 94.2% (PD cohort), to 79.6% (D2 cohort) and 74.7% (D1 cohort). Patients in the PD cohort had a mean of 306 days covered by medication (74% of the follow-up days; 316 for adherent patients and 149 for non-adherents), compared to 309 days covered in the D1 cohort (85% of the follow-up days; adherent patients: 352; non-adherent patients: 181) and to 323 in the D2 cohort (88% of the follow-up days; adherents: 352; non-adherents: 209). The 295 patients excluded from cohort D1 with respect to the PD cohort (patients who did not refill any prescription during the year after the first doctors’ prescription) had a mean of 41 covered days (most part of patients were fully incompliant but some patients restarted after a year from the first prescription), and the 4,706 patients excluded from cohort D2 (patients with less than two refills or less than 180 days between refills) had a mean of 89 covered days.
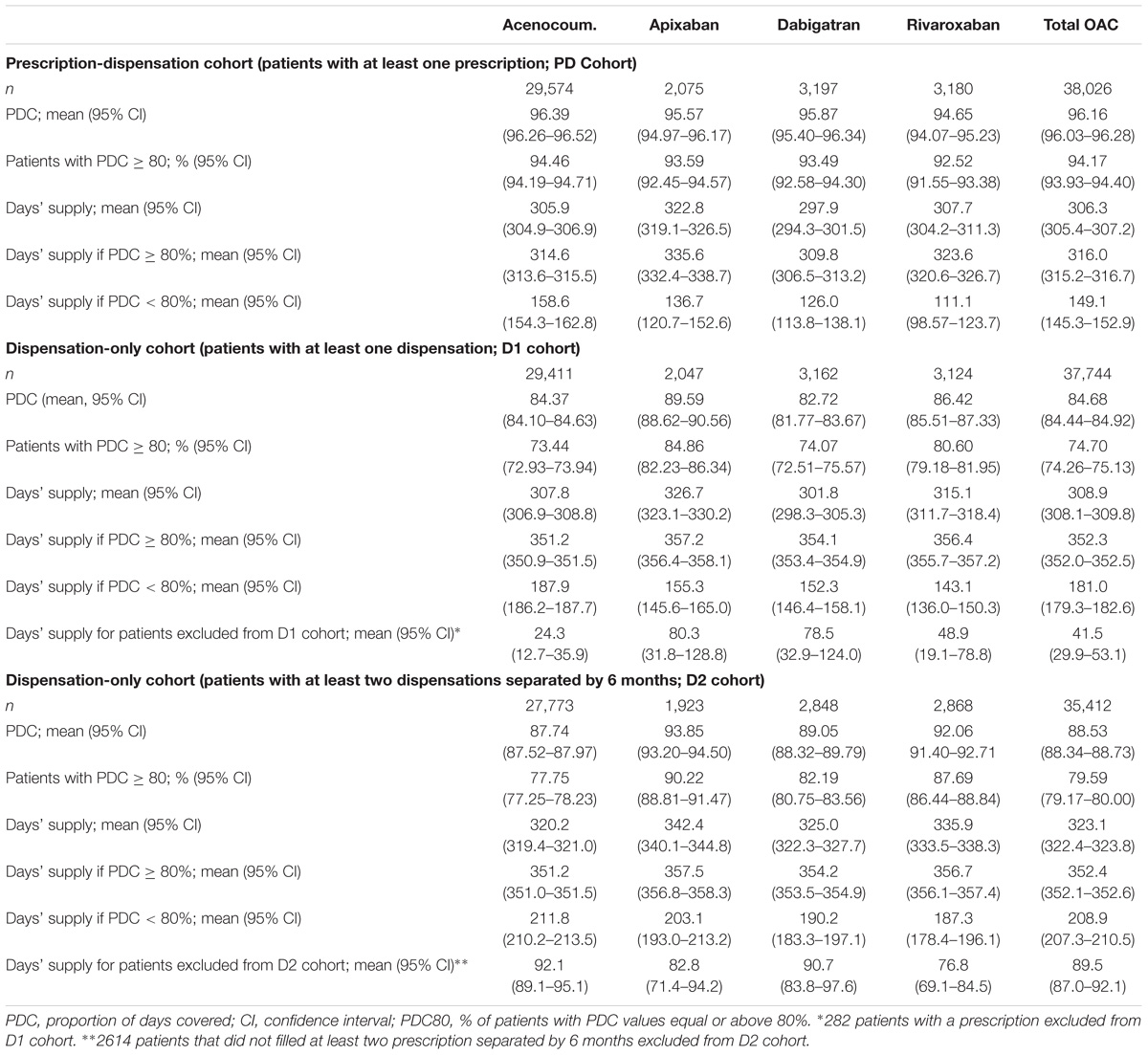
TABLE 3. Proportion of days covered and days’ supply during 1 year of follow-up, according to prescription-dispensation and dispensation-only based designs.
When observing specific drugs (Table 3), differences in mean PDC—although significant in some cases—were negligible in the PD cohort (from 96% for acenocoumarol to 94% for rivaroxaban), but widened in the dispensation-based cohorts (from 89% for apixaban to 83% for dabigatran in the D1 cohort and from 94% for apixaban to 88% for acenocoumarol in the D2 cohort). Regarding patients with PDC ≥ 80%, all drugs showed high adherence figures (93–94%) in the PD cohort. In the D1 cohort, these figures declined notably for acenocoumarol and dabigatran (73–74% of patients with PDC ≥ 80%) and to a lesser extent in the case of rivaroxaban (81%) and apixaban (84%). The D2 cohort showed even greater differences between drugs: from 90% of patients with PDC ≥ 80% when apixaban was the index drug (or 88% for rivaroxaban) to 82% for dabigatran or 78% for acenocoumarol. Therefore, while in the PD cohort acenocoumarol showed the highest number of patients with PDC equal to or greater than 80% (95%), in both D1 and D2 cohorts, rivaroxaban and especially apixaban showed the best adherence results, with acenocoumarol falling to 78% (D2 design) or 73% (D1 design).
A 20% of patients classified as adherent (PDC ≥ 80%) in the PD cohort had less than 80% days covered by medication, by virtually none in cohorts D1 and D2 whose classification of “adherence” is very close to the actual exposure to OACs (Table 4). D1 and D2 cohorts offer a very similar adherence classification, even at the expense of excluding a significant proportion of non-adherent patients in the case of the D2 cohort.
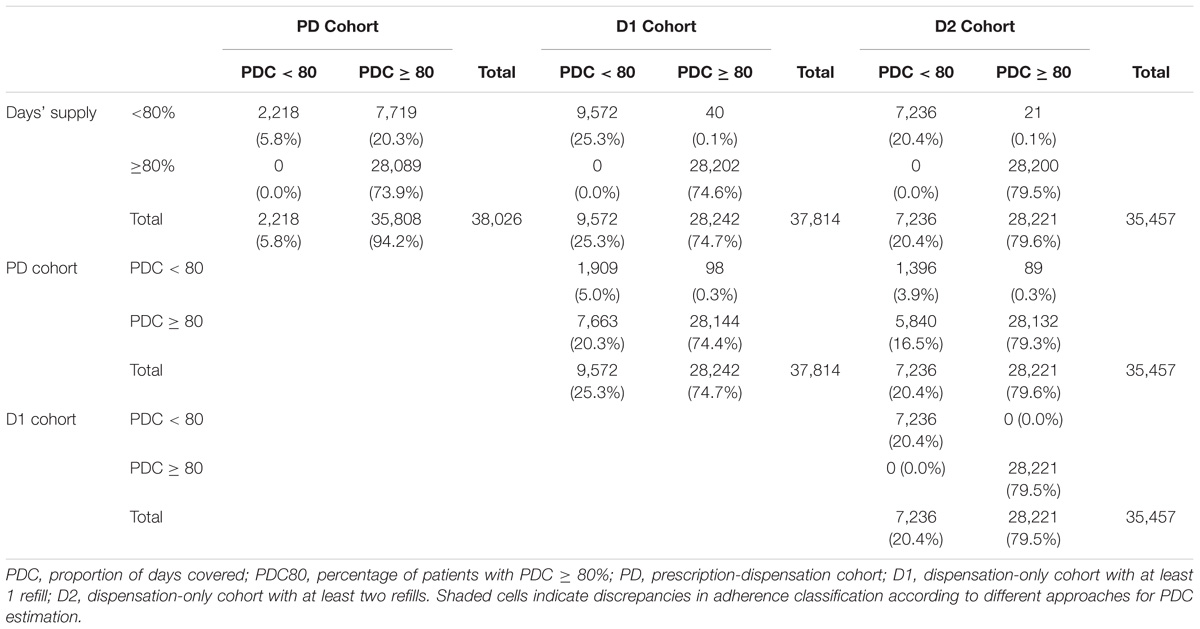
TABLE 4. Drug exposure (days’ supply) and classification of patients as adherent (PDC ≥ 80%) or non-adherent (PDC < 80%), according to different approaches to calculate PDC.
Crude events rate by 100 person-years during follow-up (1.9 years for mortality and 1.4 for stroke and bleeding) were about 6.7 for death, 0.9 for stroke and 1.4 for bleeding (Table 5). Unadjusted proportional hazard regression showed a significant protective effect of adherence on mortality (Hazard Ratio [HR] between 0.79 and 0.93 depending on the cohort) and also on stroke in the D1 and D2 cohorts (HR of 0.62 and 0.69, respectively) but not in the PD cohort, with no effect on admission for bleeding episodes. Multivariate proportional hazard regression models (Table 5 and Supplementary Tables S2–S4) showed similar results, with adherence associated with a significant reduced risk of death for the three cohorts (adjusted HR between 0.80 and 0.86). Stroke risk reduction was significant for D1 and D2 cohorts (adjusted HR between 0.61 and 0.64), and very close to statistical significance for the PD cohort (adjusted HR 0.66, 95CI: 0.43–1.02). Adherence had no effect on bleeding.
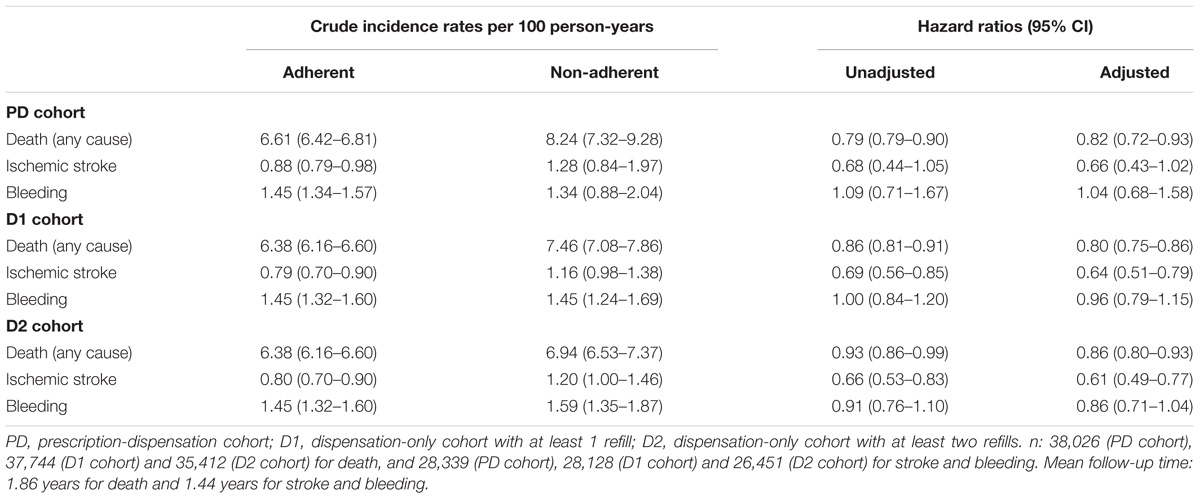
TABLE 5. Crude health outcomes rates and association between adherence (PDC ≥ 80%) and health outcomes according to different approaches for PDC estimation (crude and adjusted hazard ratios).
Discussion
Our study highlights the importance of patient adherence to OAC medications. Irrespective of the approach used for measuring PDC, adherence to OAC in NVAF patients was associated with a substantial reduction in mortality and (except in the PD cohort) in the incidence of thromboembolic stroke, without a significant increase in admissions for hemorrhagic episodes. Significance of stroke risk reduction in the PD cohort was probably not achieved because a substantial number of patients with low drug exposure due that physician-initiated treatment discontinuation (20% of patients classified as adherent, with PDC ≥ 80%, had less than 80% of days of exposure) were classified as adherent.
The association between high levels of medication adherence and better outcomes is, in general, consistent with previous research (all with D1 or D2 designs) (Shore et al., 2014; Alberts et al., 2016; Yao et al., 2016; Borne et al., 2017; Deshpande et al., 2018) evaluating the association between adherence to OACs and health outcomes using the PDC80 threshold. This consistency, despite methodological differences (some of the referenced publications include experienced users, censor switchers or censor follow-up at the time of the last refill, use variable time-windows and other process differences), also strengthens the importance of adherence to approximate the real-life OAC benefits to those obtained in clinical trials.
To the best of our knowledge our study is the first using a prescription-based design to assess OAC adherence (and of the very few who have used this methodology in any other condition). This specific design evidences that patients (at least in OAC treatment in the Valencia region public healthcare setting) are highly adherent to medical recommendations, with 94% of patients above PDC80 when adherence is assessed against doctors’ prescription. Nevertheless, and due to doctor’s discontinuation, this high “adherence” translates into a mean of only 74% of patients with at least 80% of the follow-up days covered by medication. This finding suggests that physician-initiated treatment discontinuation is a major contributor to reduced OAC exposure. When using the dispensation-based D1 design “adherence” figures represent medication exposure more accurately (PDC80: 75%; days’ supply: 74%), but at the expense of misestimating “real adherence” to the medication prescribed, attributing to patients a supposed loss of adherence when it is actually treatment discontinuation by doctors. This finding is of especial relevance because most interventions aimed to improve medication adherence are focused on patient’s behavior. In the light of our results, and especially for essential treatments and in some contexts the appropriateness of medical discontinuation should be also considered when tackling the issue of real-world drug adherence. Moreover, dispensation-only designs to assess adherence classifies some adherent patients as non-adherent, and thus, targeting improvement interventions to these patients would be inefficient.
Adherence to medical recommendations was high and similar for the most used VKA in Spain (acenocoumarol, PDC80 = 94%) and any of the marketed NOAC (PDC80 between 92 and 94%), contrasting the worst adherence to NOAC (vs. VKA) speculated by some authors based on the lack of close monitoring (INR control) (Rodriguez et al., 2013; Hendriks et al., 2017; Burn and Pirmohamed, 2018). In the same way, adherence to VKA—always taking the medical prescription as reference—does not seem inferior to NOAC adherence, contrary to what is suggested by studies assessing the comparative adherence to VKA and NOAC (McHorney et al., 2017). However, doctors discontinue treatment to a greater extent in patients initiating with acenocoumarol (PDC80 in D1 Cohort: 73.4%) or dabigatran (PDC80 in D1 cohort: 74.1%) than rivaroxaban or apixaban (PDC80: 80.6 and 84.9% in the D1 cohort). This may partially explain why in the dispensation-only cohorts used by several adherence comparative studies the latter medications appear to have better adherence results than VKA or dabigatran (Crivera et al., 2015; Hanemaaijer et al., 2015; McHorney et al., 2015, 2017; Alberts et al., 2016; Beyer-Westendorf et al., 2016; Brown et al., 2016, 2017; Coleman et al., 2016; Yao et al., 2016; Borne et al., 2017; Manzoor et al., 2017; Maura et al., 2017; Mueller et al., 2017). Note that these differences are not due to switching to another OAC (an aspect not assessed in our study, in which, by design, the days’ supply of the new OAC after switching would continue to be considered in the index drug) but to discontinuation of treatment without restart during the follow-up period.
Finally, our study showed that adherent patients (defined as patients above PDC80) and non-adherent patients have different medication exposure depending on the method employed to calculate PDC (with mean days’ supply ranging from 10 to 12 months for adherent patients and from 5 to 7 months for non-adherent patients, depending on the cohort). Also, we showed that, in D1 and D2 cohorts, the design fails to include relevant groups of low-adherent patients as non-adherent patients or patients with only one refill, and also periods of non-adherence (as primary or early non-adherence) are ignored (Rodriguez-Bernal et al., 2018). Classification of patients as adherent or non-adherent based in the PDC80 threshold is sensitive to the method used for calculating PDC, and the assessment of the relationship between adherence and health outcomes compare different groups regarding drug exposure (e.g., in some designs outcomes are compared between patients with 10 vs. 4.6 months of average treatment, while in others designs outcomes are compared between patients with 12 vs. 7 months of average treatment). These results emphasize the need to standardize study designs and methods of calculation of days’ supply-based adherence measures with refill databases.
In this regard, the PQA method to estimate adherence requiring two prescriptions filled by at least 180 separate days excludes a relevant part of non-adherent patients artificially improving adherence with respect to the D1 design and without any additional advantage over this method. In fact, some studies linking doctor’s prescription to pharmacy dispensation show that up to one in three patients either never fill their initial prescription or discontinue the drug after a single fill (Fischer et al., 2010; Shrank et al., 2010; Raebel et al., 2012).
Limitations
Our work is subject to several limitations. First, we used prescription and dispensing data to measure adherence, but patients do not necessarily consume all the drugs filled. Nevertheless, several studies have shown a high consistency between dispensation and patient consumption (Grymonpre et al., 1998; Grymonpre et al., 2006; Márquez-Contreras et al., 2018; Mehta et al., 2018).
Second, we used information on prescribing dosing schedule to construct days’ supply. This method is accurate to estimate exposure to NOAC, but may be more imprecise for acenocoumarol (dosing is frequently modified after INR control visits and may not be registered in the prescription). Since acenocoumarol is the most prevalent OAC in Spain (García-Sempere et al., 2017), this greater imprecision could affect—to some extent—some study results. Even so, we believe that this method is more accurate than using average doses or similar methods used in other studies.
Third, the high adherence to doctors’ prescription found in our study may not be generalizable to other conditions and contexts. Despite the increase of co-payments as part of the measures to reduce public spending in July 2012 (González López-Valcárcel et al., 2017), the level of co-payment for chronic disease medications and for retired people in the Spanish NHS is low. In this sense, co-payment may be a weaker barrier to adherence in Spain than in countries with higher patient cost-sharing (Choudhry et al., 2007, 2011, 2014).
Fourth, NOAC drugs have been marketed at different moments in time. It can be assumed that patterns of initiation with a specific drug when five alternative treatments are available may be different from those when less options were on the market. We carried out a sensibility analysis calculating PDC80 only for patients initiating in the period where all five alternatives were marketed (the period after apixaban release, Supplementary Table S5). No relevant changes were found with regard to our main analysis.
Fifth, regarding the relationship between adherence and outcomes, we cannot rule out the presence of a healthy adherer effect, a type of bias reflecting patient behavior, not easily identifiable and quantifiable, that could positively affect health outcomes, being the benefits incorrectly attributed to medication adherence (Chewning, 2006; Shrank et al., 2011; Ladova et al., 2014). In the same way, and despite the multivariate adjustment made, the possibility of reverse causation in our analysis between adherence and outcomes cannot be ruled out: poor expected outcomes could lead to discontinuation of treatment (by the doctor or the patient) being the outcome wrongly attributed to the discontinuation. Addressing these limitations requires the use of complex study designs and modeling techniques to control confounding by indication (patients who discontinue are different from patient who not) and information biases, both being issues that have barely been addressed to date in studies linking drug adherence and outcomes (Yu et al., 2010; LaFleur et al., 2011; Nelson et al., 2013).
Conclusion
Studies assessing adherence with data from refill databases and using a 80% threshold on days’ supply measures tend to exclude low-adherent patients and periods of non-adherence and, also, misclassify periods of treatment discontinuation by doctors as patient non-adherence. Designs based on doctors’ prescription solve these problems, but require additional information about the actual exposure to treatment since these designs classify as adherent (to the medical prescription) patients with very low drug exposure whose doctors have discontinued treatment, a major reason for low OAC exposure.
The 80% threshold to classify patients as adherent or non-adherent can include very different groups of patients according to the methods used for the construction of days’ supply measures, highlighting the need to standardize (and transparently detail) the procedures used for the construction of these measures. In any case, high adherence and high exposure to evidence-based treatments, such as OAC in NVAF patients, seem to be associated with better outcomes, strengthening the need to develop strategies (focused on the patient, doctors and/or health organizations) to bring real-world outcomes closer to those of the clinical trials.
Author Contributions
GS-G, AG-S, and SP conceived the study and wrote the first draft of the manuscript. GS-G and SP are guarantors and take full responsibility for the integrity of the data and the accuracy of the data analysis. IH-N, AG-S, CR-B, YS-A, SP, and GS-G participated in the study design and methodology. IH-N and SP performed the analysis. All authors participated in interpretation of data, and contributed to the critical revision of the manuscript for important intellectual content. All authors agree to be accountable for all aspects of the work and have read and approved the final manuscript.
Funding
This study received no specific private, non-for-profit or public funding. CR-B and YS-A were partially funded by the Instituto de Salud Carlos III, Spanish Ministry of Economy, Industry and Competitiveness (Grant No. RD16/0001/0011 cofinanced by the European Regional Development Fund).
Conflict of Interest Statement
The FISABIO foundation (a non-for-profit research institution depending on the Valencia Ministry of Health) had a Collaboration Agreement with Boehringer Ingelheim to conduct non-conditioned independent research in chronic health care, pharmacoepidemiology and medical practice variation (2013–2014), and received an unrestricted research grant from Daiichi-Sankyo (2017), both not related with the current study. GS-G participated in 2014 in an advisory meeting of Boehringer Ingelheim. None of the mentioned institutions/firms were involved in the design and conduct of the study; the collection, management, analysis, and interpretation of the data; or the preparation, review or approval of the manuscript, or in the decision to submit it for publication. The views presented here are those of the authors and not necessarily those of the FISABIO Foundation, the Valencia Ministry of Health or any other institution.
Acknowledgments
The Ministry of Health of the Valencia Government provided the data needed to carry out this study.
Supplementary Material
The Supplementary Material for this article can be found online at: https://www.frontiersin.org/articles/10.3389/fphar.2018.01353/full#supplementary-material
References
Alberts, M. J., Peacock, W. F., Fields, L. E., Bunz, T. J., Nguyen, E., Milentijevic, D., et al. (2016). Association between once- and twice-daily direct oral anticoagulant adherence in nonvalvular atrial fibrillation patients and rates of ischemic stroke. Int. J. Cardiol. 215, 11–13. doi: 10.1016/j.ijcard.2016.03.212
Andrade, S. E., Kahler, K. H., Frech, F., and Chan, K. A. (2006). Methods for evaluation of medication adherence and persistence using automated databases. Pharmacoepidemiol. Drug Saf. 15, 565–574. doi: 10.1002/pds.1230
Arnet, I., Kooij, M. J., Messerli, M., Hersberger, K. E., Heerdink, E. R., and Bouvy, M. (2016). Proposal of standardization to assess adherence with medication records: methodology matters. Ann. Pharmacother. 50, 360–368. doi: 10.1177/1060028016634106
Beyer-Westendorf, J., Ehlken, B., and Evers, T. (2016). Real-world persistence and adherence to oral anticoagulation for stroke risk reduction in patients with atrial fibrillation. Europace 18, 1150–1157. doi: 10.1093/europace/euv421
Bitton, A., Choudhry, N. K., Matlin, O. S., Swanton, K., Shrank, W. H. (2013). The impact of medication adherence on coronary artery disease costs and outcomes: a systematic review. Am. J. Med. 126, 357.e7–357.e27. doi: 10.1016/j.amjmed.2012.09.004
Borne, R. T., O’Donnell, C., Turakhia, M. P., Varosy, P. D., Jackevicius, C. A., Marzec, L. N., et al. (2017). Adherence and outcomes to direct oral anticoagulants among patients with atrial fibrillation: findings from the veterans health administration. BMC Cardiovasc Disord. 17:236. doi: 10.1186/s12872-017-0671-6
Brown, J. D., Shewale, A. R., and Talbert, J. C. (2016). Adherence to rivaroxaban, dabigatran, and apixaban for stroke prevention in incident, treatment-naïve nonvalvular atrial fibrillation. J. Manage. Care Spec Pharm. 22, 1319–1329. doi: 10.18553/jmcp.2016.22.11.1319
Brown, J. D., Shewale, A. R., and Talbert, J. C. (2017). Adherence to rivaroxaban, dabigatran, and apixaban for stroke prevention for newly diagnosed and treatment-naive atrial fibrillation patients: an update using 2013-2014 data. J. Manage. Care Spec. Pharm. 23, 958–967. doi: 10.18553/jmcp.2017.23.9.958
Burn, J., and Pirmohamed, M. (2018). Direct oral anticoagulants versus warfarin: is new always better than the old? Open Heart. 5:e000712. doi: 10.1136/openhrt-2017-000712
Chewning, B. (2006). The healthy adherer and the placebo effect. BMJ 333, 18–19. doi: 10.1136/bmj.333.7557.18
Choudhry, N. K., Avorn, J., Antman, E. M., Schneeweiss, S., and Shrank, W. H. (2007). Should patients receive secondary prevention medications for free after a myocardial infarction? An economic analysis. Health Aff. (Millwood) 26, 186–194. doi: 10.1377/hlthaff.26.1.186
Choudhry, N. K., Avorn, J., Glynn, R. J., Antman, E. M., Schneeweiss, S., Toscano, M., et al. (2011). Post-Myocardial infarction free rx event and economic evaluation (MI FREEE) trial. Full coverage for preventive medications after myocardial infarction. N. Engl. J. Med. 365, 2088–2097. doi: 10.1056/NEJMsa1107913
Choudhry, N. K., Glynn, R. J., Avorn, J., Lee, J. L., Brennan, T. A., Reisman, L., et al. (2014). Untangling the relationship between medication adherence and post-myocardial infarction outcomes: medication adherence and clinical outcomes. Am. Heart J. 167, 51.e5–58.e5. doi: 10.1016/j.ahj.2013.09.014
Coleman, C., Yuan, Z., Schein, J., Crivera, C., Ashton, V., Laliberté, F., et al. (2017). Importance of balancing follow-up time and impact of oral-anticoagulant users’ selection when evaluating medication adherence in atrial fibrillation patients treated with rivaroxaban and apixaban. Curr. Med. Res. Opin. 33, 1033–1043. doi: 10.1080/03007995.2017.1297932
Coleman, C. I., Tangirala, M., and Evers, T. (2016). Medication adherence to rivaroxaban and dabigatran for stroke prevention in patients with non-valvular atrial fibrillation in the United States. Int. J. Cardiol. 212, 171–173. doi: 10.1016/j.ijcard.2016.03.051
Cramer, J. A., Roy, A., Burrell, A., Fairchild, C. J., Fuldeore, M. J., Ollendorf, D. A., et al. (2008). Medication compliance and persistence: terminology and definitions. Value Health 11, 44–47. doi: 10.1111/j.1524-4733.2007.00213.x
Crivera, C., Nelson, W. W., Bookhart, B., Martin, S., Germain, G., Laliberté, F., et al. (2015). Pharmacy quality alliance measure: adherence to non-warfarin oral anticoagulant medications. Curr. Med. Res. Opin. 31, 1889–1895. doi: 10.1185/03007995.2015.1077213
Danaei, G., Tavakkoli, M., and Hernán, M. A. (2012). Bias in observational studies of prevalent users: lessons for comparative effectiveness research from a meta-analysis of statins. Am. J. Epidemiol. 175, 250–262. doi: 10.1093/aje/kwr301
De Vera, M. A., Bhole, V., Burns, L. C., and Lacaille, D. (2014). Impact of statin adherence on cardiovascular disease and mortality outcomes: a systematic review. Br. J. Clin. Pharmacol. 78, 684–698. doi: 10.1111/bcp.12339
Deitelzweig, S. B., Buysman, E., Pinsky, B., Lacey, M., Jing, Y., Wiederkehr, D., et al. (2013). Warfarin use and stroke risk among patients with nonvalvular atrial fibrillation in a large managed care population. Clin. Ther. 35, 1201–1210. doi: 10.1016/j.clinthera.2013.06.005
Deshpande, C. G., Kogut, S., Laforge, R., and Willey, C. (2018). Impact of medication adherence on risk of ischemic stroke, major bleeding and deep vein thrombosis in atrial fibrillation patients using novel oral anticoagulants. Curr. Med. Res. Opin. 34, 1285–1292. doi: 10.1080/03007995.2018.1428543
DiMatteo, M. R., Giordani, P. J., Lepper, H. S., and Croghan, T. W. (2002). Patient adherence and medical treatment outcomes: a meta-analysis. Med. Care 40, 794–811. doi: 10.1097/00005650-200209000-00009
Dong, Y. H., Choudhry, N. K., Krumme, A., Lee, M. P., Wu, L. C., Lai, M. S., et al. (2017). Impact of hospitalization on medication adherence estimation in claims data. J. Clin. Pharm. Ther. 42, 318–328. doi: 10.1111/jcpt.12517
Dragomir, A., Côté, R., Roy, L., Blais, L., Lalonde, L., Bérard, A., et al. (2010). Impact of adherence to antihypertensive agents on clinical outcomes and hospitalization costs. Med. Care 48, 418–425. doi: 10.1097/MLR.0b013e3181d567bd
Fischer, M. A., Stedman, M. R., Lii, J., Vogeli, C., Shrank, W. H., Brookhart, M. A., et al. (2010). Primary medication non-adherence: analysis of 195,930 electronic prescriptions. J. Gen. Int. Med. 25, 284–290. doi: 10.1007/s11606-010-1253-9
García-Sempere, A., Bejarano-Quisoboni, D., Librero, J., Rodríguez-Bernal, C. L., Peiró, S., and Sanfélix-Gimeno, G. A. (2017). Multilevel analysis of real-world variations in oral anticoagulation initiation for atrial fibrillation in valencia, a European region. Front. Pharmacol. 8:576. doi: 10.3389/fphar.2017.00576
Gellad, W. F., Thorpe, C. T., Steiner, J. F., and Voils, C. I. (2017). The myths of medication adherence. Pharmacoepidemiol. Drug Saf. 26, 1437–1441. doi: 10.1002/pds.4334
González López-Valcárcel, B., Librero, J., García-Sempere, A., Peña, L. M., Bauer, S., Puig-Junoy, J., et al. (2017). Effect of cost sharing on adherence to evidence-based medications in patients with acute coronary syndrome. Heart 103, 1082–1088. doi: 10.1136/heartjnl-2016-310610
Greevy, R. A. Jr., Huizinga, M. M., Roumie, C. L., Grijalva, C. G., Murff, H., Liu, X., et al. (2011). Comparisons of persistence and durability among three oral antidiabetic therapies using electronic prescription-fill data: the impact of adherence requirements and stockpiling. Clin. Pharmacol. Ther. 90, 813–819. doi: 10.1038/clpt.2011.228
Grymonpre, R., Cheang, M., Fraser, M., Metge, C., and Sitar, D. S. (2006). Validity of a prescription claims database to estimate medication adherence in older persons. Med. Care 44, 471–477. doi: 10.1097/01.mlr.0000207817.32496.cb
Grymonpre, R. E., Didur, C. D., Montgomery, P. R., and Sitar, D. S. (1998). Pill count, self-report, and pharmacy claims data to measure medication adherence in the elderly. Ann. Pharmacother. 32, 749–754. doi: 10.1345/aph.17423
Hanemaaijer, S., Sodihardjo, F., Horikx, A., Wensing, M., De Smet, P. A., Bouvy, M. L., et al. (2015). Trends in antithrombotic drug use and adherence to non-vitamin K oral anticoagulants in the Netherlands. Int. J. Clin. Pharm. 37, 1128–1135. doi: 10.1007/s11096-015-0174-4
Hansen, R. A., Kim, M. M., Song, L., Tu, W., Wu, J., and Murray, M. D. (2009). Comparison of methods to assess medication adherence and classify nonadherence. Ann. Pharmacother. 43, 413–422. doi: 10.1345/aph.1L496
Hendriks, J. M., Gallagher, C., and Sanders, P. (2017). Ensuring adherence to therapy with anticoagulation in patients with atrial fibrillation. Heart 103, 1308–1309. doi: 10.1136/heartjnl-2017-311305
Hood, K. K., Peterson, C. M., Rohan, J. M., and Drotar, D. (2009). Association between adherence and glycemic control in pediatric type 1 diabetes: a meta-analysis. Pediatrics 124, e1171–e1179. doi: 10.1542/peds.2009-0207
Karlsson, S. A., Hero, C., Svensson, A. M., Franzén, S., Miftaraj, M., Gudbjörnsdottir, S., et al. (2018). Association between refill adherence to lipid-lowering medications and the risk of cardiovascular disease and mortality in Swedish patients with type 2 diabetes mellitus: a nationwide cohort study. BMJ Open 8:e020309. doi: 10.1136/bmjopen-2017-020309
Karve, S., Cleves, M. A., Helm, M., Hudson, T. J., West, D. S., and Martin, B. C. (2009). Good and poor adherence: optimal cut-point for adherence measures using administrative claims data. Curr. Med. Res. Opin. 25, 2303–2310. doi: 10.1185/03007990903126833
Khunti, K., Seidu, S., Kunutsor, S., and Davies, M. (2017). Association between adherence to pharmacotherapy and outcomes in type 2 diabetes: a meta-analysis. Diabetes Care 40, 1588–1596. doi: 10.2337/dc16-1925
Kozma, C. M., Dickson, M., Phillips, A. L., and Meletiche, D. M. (2013). Medication possession ratio: implications of using fixed and variable observation periods in assessing adherence with disease-modifying drugs in patients with multiple sclerosis. Patient Pref. Adherence 7, 509–516. doi: 10.2147/PPA.S40736
Krueger, K., Griese-Mammen, N., Schubert, I., Kieble, M., Botermann, L., Laufs, U., et al. (2018). In search of a standard when analyzing medication adherence in patients with heart failure using claims data: a systematic review. Heart Fail Rev. 23, 63–71. doi: 10.1007/s10741-017-9656-x
Ladova, K., Vlcek, J., Vytrisalova, M., and Maly, J. (2014). Healthy adherer effect – The pitfall in the interpretation of the effect of medication adherence on health outcomes. J. Eval. Clin. Pract. 20, 111–116. doi: 10.1111/jep.12095
LaFleur, J., Nelson, R. E., Sauer, B. C., and Nebeker, J. R. (2011). Overestimation of the effects of adherence on outcomes: a case study in healthy user bias and hypertension. Heart 97, 1862–1869. doi: 10.1136/hrt.2011.223289
Li, X., Cole, S. R., Westreich, D., and Brookhart, M. A. (2018). Primary non-adherence and the new-user design. Pharmacoepidemiol. Drug Saf. 27, 361–364. doi: 10.1002/pds.4403
Lo-Ciganic, W. H., Donohue, J. M., Thorpe, J. M., Perera, S., Thorpe, C. T., Marcum, Z. A., et al. (2015). Using machine learning to examine medication adherence thresholds and risk of hospitalization. Med. Care 53, 720–728. doi: 10.1097/MLR.0000000000000394
Lund, J. L., Richardson, D. B., and Stürmer, T. (2015). The active comparator, new user study design in pharmacoepidemiology: historical foundations and contemporary application. Curr. Epidemiol. Rep. 2, 221–228. doi: 10.1007/s40471-015-0053-5
Maciejewski, M. L., Bryson, C. L., Wang, V., Perkins, M., and Liu, C. F. (2013). Potential bias in medication adherence studies of prevalent users. Health Serv. Res. 48, 1468–1486. doi: 10.1111/1475-6773.12043
Manzoor, B. S., Lee, T. A., Sharp, L. K., Walton, S. M., Galanter, W. L., and Nutescu, E. A. (2017). Real-world adherence and persistence with direct oral anticoagulants in adults with atrial fibrillation. Pharmacotherapy 37, 1221–1230. doi: 10.1002/phar.1989
Márquez-Contreras, E., López García-Ramos, L., Martell-Claros, N., Gil-Guillen, V. F., Márquez-Rivero, S., Pérez-López, E., et al. (2018). Validation of the electronic prescription as a method for measuring treatment adherence in hypertension. Patient Educ. Couns. 101, 1654–1660. doi: 10.1016/j.pec.2018.04.009
Maura, G., Pariente, A., Alla, F., and Billionnet, C. (2017). Adherence with direct oral anticoagulants in nonvalvular atrial fibrillation new users and associated factors: a French nationwide cohort study. Pharmacoepidemiol. Drug Saf. 26, 1367–1377. doi: 10.1002/pds.4268
McDermott, M. M., Schmitt, B., and Wallner, E. (1997). Impact of medication nonadherence on coronary heart disease outcomes. A critical review. Arch. Int. Med. 157, 1921–1929. doi: 10.1001/archinte.1997.00440380023002
McHorney, C. A., Ashton, V., Laliberté, F., Germain, G., Wynant, W., Crivera, C., et al. (2017). adherence to rivaroxaban compared with other oral anticoagulant agents among patients with nonvalvular atrial fibrillation. J. Manage. Care Spec. Pharm. 23, 980–988. doi: 10.18553/jmcp.2017.23.9.980
McHorney, C. A., Crivera, C., Laliberté, F., Germain, G., Wynant, W., and Lefebvre, P. (2018). Adherence to rivaroxaban versus apixaban among patients with non-valvular atrial fibrillation: analysis of overall population and subgroups of prior oral anticoagulant users. PLoS One 13:e0194099. doi: 10.1371/journal.pone.0194099
McHorney, C. A., Crivera, C., Laliberté, F., Nelson, W. W., Germain, G., Bookhart, B., et al. (2015). Adherence to non-vitamin-K-antagonist oral anticoagulant medications based on the pharmacy quality alliance measure. Curr. Med. Res. Opin. 31, 2167–2173. doi: 10.1185/03007995.2015.1096242
McHorney, C. A., Peterson, Laliberté, F., Germain, G., Nelson, W. W., Crivera, C., et al. (2016). Comparison of adherence to rivaroxaban versus apixaban among patients with atrial fibrillation. Clin. Ther. 38, 2477–2488. doi: 10.1016/j.clinthera.2016.09.014
Mehta, S. J., Asch, D. A., Troxel, A. B., Lim, R., Lewey, J., Wang, W., et al. (2018). Comparison of pharmacy claims and electronic pill bottles for measurement of medication adherence among myocardial infarction patients. Med Care doi: 10.1097/MLR.0000000000000950 [Epub ahead of print].
Morrison, A., Stauffer, M. E., and Kaufman, A. S. (2017). Relationship between adherence rate threshold and drug ’forgiveness’. Clin. Pharmacokinet. 56, 1435–1440. doi: 10.1007/s40262-017-0552-2
Mueller, T., Alvarez-Madrazo, S., Robertson, C., and Bennie, M. (2017). Use of direct oral anticoagulants in patients with atrial fibrillation in scotland: applying a coherent framework to drug utilisation studies. Pharmacoepidemiol. Drug Saf. 26, 1378–1386. doi: 10.1002/pds.4272
Nakasian, S. S., Rassen, J. A., and Franklin, J. M. (2017). Effects of expanding the look-back period to all available data in the assessment of covariates. Pharmacoepidemiol. Drug Saf. 26, 890–899. doi: 10.1002/pds.4210
Nelson, R. E., Nebeker, J. R., Hayden, C., Reimer, L., Kone, K., and LaFleur, J. (2013). Comparing adherence to two different HIV antiretroviral regimens: an instrumental variable analysis. AIDS Behav. 17, 160–167. doi: 10.1007/s10461-012-0266-2
Nikitovic, M., Solomon, D. H., and Cadarette, S. M. (2010). Methods to examine the impact of compliance to osteoporosis pharmacotherapy on fracture risk: systematic review and recommendations. Ther. Adv. Chronic Dis. 1, 149–162. doi: 10.1177/2040622310376137
Osterberg, L., and Blaschke, T. (2005). Adherence to medication. N. Engl. J. Med. 353, 487–497. doi: 10.1056/NEJMra050100
Palmaro, A., Boucherie, Q., Dupouy, J., Micallef, J., and Lapeyre-Mestre, M. (2017). Immeasurable time bias due to hospitalization in medico-administrative databa-ses: which impact for pharmacoepidemiological studies? Pharmacoepidemiol. Drug Saf. 26, 544–553. doi: 10.1002/pds.4193
Raebel, M. A., Ellis, J. L., Carroll, N. M., Bayliss, E. A., McGinnis, B., Schroeder, E. B., et al. (2012). Characteristics of patients with primary non-adherence to medications for hypertension, diabetes, and lipid disorders. J. Gen. Int. Med. 27, 57–64. doi: 10.1007/s11606-011-1829-z
Rasmussen, J. N., Chong, A., and Alter, D. A. (2007). Relationship between adherence to evidence-based pharmacotherapy and long-term mortality after acute myocardial infarction. JAMA 297, 177–186. doi: 10.1001/jama.297.2.177
Ray, W. A. (2003). Evaluating medication effects outside of clinical trials: new-user designs. Am. J. Epidemiol. 158, 915–920. doi: 10.1093/aje/kwg231
Riis, A. H., Johansen, M. B., Jacobsen, J. B., Brookhart, M. A., Stürmer, T., and Støvring, H. (2015). Short look-back periods in pharmacoepidemiologic studies of new users of antibiotics and asthma medications introduce severe misclassification. Pharmacoepidemiol. Drug Saf. 24, 478–485. doi: 10.1002/pds.3738
Roberts, A. W., Dusetzina, S. B., and Farley, J. F. (2015). Revisiting the washout period in the incident user study design: why 6-12 months may not be sufficient. J. Comp. Eff. Res. 4, 27–35. doi: 10.2217/cer.14.53
Rodriguez, R. A., Carrier, M., and Wells, P. S. (2013). Non-adherence to new oral anticoagulants: a reason for concern during long-term anticoagulation? J. Thromb. Haemost. 11, 390–394. doi: 10.1111/jth.12086
Rodriguez-Bernal, C. L., Peiró, S., Hurtado, I., García-Sempere, A., and Sanfélix-Gimeno, G. (2018). Primary nonadherence to oral anticoagulants in patients with atrial fibrillation: real-world data from a population-based cohort. J. Manage. Care Spec. Pharm. 24, 440–448. doi: 10.18553/jmcp.2018.24.5.440
Rudd, P. (1979). In search of the gold standard for compliance measurement. Arch. Int. Med. 139, 627–628. doi: 10.1001/archinte.1979.03630430009004
Sabaté, E. (2003). Adherence to Long-Term Therapies: Evidence for Action. Geneva: World Health Organisation. Available at: http://whqlibdoc.who.int/publications/2003/9241545992.pdf
Sackett, D. L., Haynes, R. B., Gibson, E. S., Hackett, B. C., Taylor, D. W., Roberts, R. S., et al. (1975). Randomised clinical trial of strategies for improving medication compliance in primary hypertension. Lancet 1, 1205–1207. doi: 10.1016/S0140-6736(75)92192-3
Sanfélix-Gimeno, G., Rodríguez-Bernal, C. L., Hurtado, I., Baixáuli-Pérez, C., Librero, J., and Peiró, S. (2015). Adherence to oral anticoagulants in patients with atrial fibrillation-a population-based retrospective cohort study linking health information systems in the Valencia region, Spain: a study protocol. BMJ Open 5:e007613. doi: 10.1136/bmjopen-2015-007613
Schneeweiss, S., and Avorn, J. (2005). A review of uses of health care utilization databases for epidemiologic research on therapeutics. J. Clin. Epidemiol. 58, 323–337. doi: 10.1016/j.jclinepi.2004.10.012
Shore, S., Carey, E. P., Turakhia, M. P., Jackevicius, C. A., Cunningham, F., Pilote, L., et al. (2014). Adherence to dabigatran therapy and longitudinal patient outcomes: insights from the veterans health administration. Am. Heart J. 167, 810–817. doi: 10.1016/j.ahj.2014.03.023
Shrank, W. H., Choudhry, N. K., Fischer, M. A., Avorn, J., Powell, M., Schneeweiss, S., et al. (2010). The epidemiology of prescriptions abandoned at the pharmacy. Ann. Int. Med. 153, 633–640. doi: 10.7326/0003-4819-153-10-201011160-00005
Shrank, W. H., Patrick, A. R., and Brookhart, M. A. (2011). Healthy user and related biases in observational studies of preventive interventions: a primer for physicians. J. Gen. Int. Med. 26, 546–550. doi: 10.1007/s11606-010-1609-1
Simpson, S. H., Eurich, D. T., Majumdar, S. R., Padwal, R. S., Tsuyuki, R. T., Varney, J., et al. (2006). A meta-analysis of the association between adherence to drug therapy and mortality. BMJ 333:15. doi: 10.1136/bmj.38875.675486.55
Spivey, C. A., Liu, X., Qiao, Y., Mardekian, J., Parker, R. B., Phatak, H., et al. (2015). Stroke associated with discontinuation of warfarin therapy for atrial fibrillation. Curr. Med. Res. Opin. 31, 2021–2029. doi: 10.1185/03007995.2015.1082995
Stauffer, M. E., Hutson, P., Kaufman, A. S., and Morrison, A. (2017). The adherence rate threshold is drug specific. Drugs R D 17, 645–653. doi: 10.1007/s40268-017-0216-6
Steiner, J. F., and Prochazka, A. V. (1997). The assessment of refill compliance using pharmacy records: methods, validity, and applications. J. Clin. Epidemiol. 50, 105–116. doi: 10.1016/S0895-4356(96)00268-5
Suissa, S., and Garbe, E. (2007). Primer: administrative health databases in observational studies of drug effects–advantages and disadvantages. Nat. Clin. Pract. Rheumatol. 3, 725–732. doi: 10.1038/ncprheum0652
Thorne, K., Jayathissa, S., Dee, S., Briggs, N., Taylor, J., Reid, S., et al. (2014). Adherence and outcomes of patients prescribed dabigatran (Pradaxa) in routine clinical practice. Int. Med. J. 44, 261–265. doi: 10.1111/imj.12370
Vrijens, B., De Geest, S., Hughes, D. A., Przemyslaw, K., Demonceau, J., Ruppar, T., et al. (2012). A new taxonomy for describing and defining adherence to medications. Br. J. Clin. Pharmacol. 73, 691–705. doi: 10.1111/j.1365-2125.2012.04167.x
Watanabe, J. H., Bounthavong, M., and Chen, T. (2013). Revisiting the medication possession ratio threshold for adherence in lipid management. Curr. Med. Res. Opin. 29, 175–180. doi: 10.1185/03007995.2013.766164
Wettermark, B., Zoega, H., Furu, K., Korhonen, M., Hallas, J., Norgaard, M., et al. (2013). The Nordic prescription databases as a resource for pharmacoepidemiological research – A literature review. Pharmacoepidemiol. Drug Saf. 22, 691–699. doi: 10.1002/pds.3457
Xu, T., Yu, X., Ou, S., Liu, X., Yuan, J., Tan, X., et al. (2017). Adherence to antihypertensive medications and stroke risk: a dose-response meta-analysis. J. Am. Heart Assoc. 6:e006371. doi: 10.1161/JAHA.117.006371
Yao, X., Abraham, N. S., Alexander, G. C., Crown, W., Montori, V. M., Sangaralingham, L. R., et al. (2016). Effect of adherence to oral anticoagulants on risk of stroke and major bleeding among patients with atrial fibrillation. J. Am. Heart Assoc. 5:e003074. doi: 10.1161/JAHA.115.003074
Yu, A. P., Yu, Y. F., and Nichol, M. B. (2010). Estimating the effect of medication adherence on health outcomes among patients with type 2 diabetes–an application of marginal structural models. Value Health 13, 1038–1045. doi: 10.1111/j.1524-4733.2010.00787.x
Keywords: medication adherence, proportion of days covered, threshold, oral anticoagulants, clinical outcomes
Citation: Hurtado-Navarro I, García-Sempere A, Rodríguez-Bernal C, Santa-Ana-Tellez Y, Peiró S and Sanfélix-Gimeno G (2018) Estimating Adherence Based on Prescription or Dispensation Information: Impact on Thresholds and Outcomes. A Real-World Study With Atrial Fibrillation Patients Treated With Oral Anticoagulants in Spain. Front. Pharmacol. 9:1353. doi: 10.3389/fphar.2018.01353
Received: 04 August 2018; Accepted: 05 November 2018;
Published: 03 December 2018.
Edited by:
Kurt E. Hersberger, Universität Basel, SwitzerlandReviewed by:
Alexandros A. Polymeris, University Hospital of Basel, SwitzerlandSunita Nair, Independent Researcher, Mumbai, India
Copyright © 2018 Hurtado-Navarro, García-Sempere, Rodríguez-Bernal, Santa-Ana-Tellez, Peiró and Sanfélix-Gimeno. This is an open-access article distributed under the terms of the Creative Commons Attribution License (CC BY). The use, distribution or reproduction in other forums is permitted, provided the original author(s) and the copyright owner(s) are credited and that the original publication in this journal is cited, in accordance with accepted academic practice. No use, distribution or reproduction is permitted which does not comply with these terms.
*Correspondence: Gabriel Sanfélix-Gimeno, c2FuZmVsaXhfZ2FiQGd2YS5lcw==