- 1College of Chemistry, Sichuan University, Chengdu, China
- 2College of Management, Southwest University for Nationalities, Chengdu, China
- 3College of Computer Science, Sichuan University, Chengdu, China
Modulating protein–protein interactions (PPIs) with small drug-like molecules targeting it exhibits great promise in modern drug discovery. G protein-coupled receptors (GPCRs) are the largest family of targeted proteins and could form dimers in living biological cells through PPIs. However, compared to drug development of the orthosteric site, there has been lack of investigations on the druggability of the PPI interface for GPCRs and its functional implication on experiments. Thus, in order to address these issues, we constructed a novel computational strategy, which involved in molecular dynamics simulation, virtual screening and protein structure network (PSN), to study one representative GPCR homodimer (CXCR4). One druggable pocket was identified in the PPI interface and one small molecule targeting it was screened, which could strengthen PPI mainly through hydrophobic interaction between the benzene rings of the PPI molecule and TM4 of the receptor. The PSN results further reveals that the PPI molecule could increase the number of the allosteric regulation pathways between the druggable pocket of the dimer interface to the orthostatic site for the subunit A but only play minor role for the other subunit B, leading to the asymmetric change in the volume of the binding pockets for the two subunits (increase for the subunit A and minor change for the subunit B). Consequently, the screening performance of the subunit A to the antagonists is enhanced while the subunit B is unchanged nearly, implying that the PPI molecule may be beneficial to enhance the drug efficacies of the antagonists. In addition, one main regulation pathway with the highest frequency was identified for the subunit A, which consists of Trp1955.34–Tyr190ECL2–Val1965.35–Gln2005.39–Asp2626.58–Cys28N-term, revealing their importance in the allosteric regulation from the PPI molecule. The observations from the work could provide valuable information for the development of the PPI drug-like molecule for GPCRs.
Introduction
In living cells, only a few proteins perform their biological functions independently, and the vast majority (more than 80%) of proteins function through interacting with other molecules (Keskin et al., 2016; Wang et al., 2018b). It is estimated that there are approximately 130,000 to 650,000 protein–protein interactions (PPIs) in the human interactome (Venkatesan et al., 2009; Sheng et al., 2015; Tortorella et al., 2016), and targeting protein–protein interactions (PPIs) with small drug-like molecules (Sheng et al., 2015; Shin et al., 2017; Han et al., 2018) become one of the most promising methods in modern drug discovery (Li et al., 2017; Tang et al., 2019a; Tang et al., 2019b). If drugs could strengthen the PPI interaction or damage it, the function of PPI will be inevitably influenced. With increasing understanding of PPIs, significant progress has been made for investigations on PPI small drug-like molecules (Wells and McClendon, 2007; Jubb et al., 2012; Song et al., 2016; Shin et al., 2017). It was observed that the PPI molecules commonly have larger molecular weight, higher hydrophobicity, and lower solubility than most of traditional drug molecules (Villoutreix et al., 2012; Wang et al., 2018b). In previous studies, most of the drugs bind a targeted protein and inhibit it to form functional complexes with its binding partners, in turn influencing the downstream signals. For example, small molecule LEDGINs could block the interaction between HIV-1 integrase and human LEDGF/p75 so that it could inhibit HIV replication(Reddy et al., 2014). The peptidemimics MAIT was found to inhibit the migration of colorectal cells by disrupting APC–Asef interaction (Jiang et al., 2017). Although researches on strengthening PPI interaction are very limited with respect to inhibiting one, it is also highly valuable for some specific proteins. For example, ISD could strengthen the interaction between Neph1 and ZO-1 so that it could prevent podocyte injury and preserve glomerular filtration function (Sagar et al., 2017).
G protein-coupled receptors (GPCRs) are the largest membrane protein families with more than 800 members, which play key roles in various signal transductions. Approximate 50 percent of drugs target them (Rosenbaum et al., 2009; Venkatakrishnan et al., 2013; Lao et al., 2017). Monomers have long been recognized as functional units of GPCR signaling (Whorton et al., 2007; Maurice et al., 2011). However, recently increasing biochemical and biophysical evidences have indicated that the GPCR dimers and oligomers also exist in living biological cells (Ferré et al., 2014; Navarro et al., 2018; Pediani et al., 2018), which could significantly affect the signal transduction process of GPCRs like receptor activation, internalization, ligand binding and coupling with G protein (Huang et al., 2013; Xue et al., 2015; Damian et al., 2018). Some experimental works already found that positive or negative cooperativity exists between the two subunits of the GPCR dimer (Maurice et al., 2011). For example, when the ligand binds to one of the subunits, it will increase or decrease the binding affinity of another subunit to the ligand (Gherbi et al., 2015; Liu et al., 2017). Therefore, the GPCR dimers possess unique pharmacological profiles, being potential drug targets for the discovery of novel drugs.
Chemokine receptors are members of family A GPCRs, which regulate cell migration in development, immune system function and inflammatory diseases, thus being important therapeutic targets (Kufareva et al., 2014; Van Hout et al., 2018). CXCR4 is one of 23 known human chemokine receptors, which plays a key role in leukocyte trafficking, hematopoiesis, organ development and cancer metastases (Zweemer et al., 2014). It was revealed that CXCR4 is associated with more than 23 types of cancers (Wu et al., 2010; Nguyen et al., 2018). CXCR4 and related CC chemokine receptor 5 (CCR5) are not only the key regulators of signal transduction, but also involve in the entry of HIV-1 virus as coreceptors of HIV-1 into leukocytes (Shaik et al., 2019). Several observations suggested that the dimer may be the minimal functional units of the chemokine receptors and CXCR4 was demonstrated to form homo- or hetero-dimers (Percherancier et al., 2005; Muñoz et al., 2012). In 2010, the crystal structure of the CXCR4 homo-dimer (PDBID: 3ODU) was resolved (Wu et al., 2010). Chemotaxis assay shows that the migration index of T-REx-293 cells stably transfected with CXCR4 gene changes with the oligomeric status of CXCR4, indicating a correlation between the functions and the oligomeric status of CXCR4 (Lao et al., 2017). These findings clearly indicate that the polymerization of GPCRs could affect the structure and the function of the receptors. Therefore, it is also valuable to design small drug-like molecules targeting PPIs of GPCRs, which are beneficial to their therapeutic effects, to enhance their polymerization. However, the investigations on drug-like small molecules targeting the interface to enhance PPIs have been lacked so far. Thus, many questions have been remained to be unclear. For example, is the PPI interface druggable for GPCRs like CXCR4? What drugs could target the interface? How does the drug regulate the dimerization and the structure of the receptor, in turn influence its drug efficacy? In fact, these questions mainly involve in microscopic structure changes of the receptor upon the ligand bound the PPI interface. Therefore, it is highly desired to introduce computational techniques to assist the experiments to probe these issues.
Molecular dynamics (MD) simulation could acquire the structural evolution of proteins at the atomic level. Therefore, it become a powerful tool to study the structural and functional mechanisms for biological systems (Xue et al., 2018), including GPCRs. However, previous MD researches on GPCRs were mainly focused on the GPCR monomers, including their structures (Liang et al., 2017; Zhang et al., 2018a), interactions with ligands (Bai et al., 2014; Sader et al., 2018), activation mechanisms (Miao et al., 2015; Stanley et al., 2016), water channels (Yuan et al., 2013; Yuan et al., 2015) and so on. In contrast, the studies on GPCR oligomers by MD are very limited, mainly concerning the self-assembly behavior (Provasi et al., 2015), activation mechanisms (Kim et al., 2017), interaction of dimers (Petersen et al., 2017). Recently, our group probed the effect of the dimerization on the activation and ligand-binding for some GPCRs (Wang et al., 2018a; Zhang et al., 2018b; Zhang et al., 2019). Based on our previous studies on the mechanism of GPCR dimers, we hope to further probe the druggability of their PPI interfaces and its regulation mechanism on the drug function of the receptor, using molecular dynamics simulation, virtual screening and protein structure network. Herein, we selected the CXCR4 dimer (PDBID:3ODU) as a representative of the GPCR dimers, which is sole crystal-structure of the GPCR dimers resolved for the chemokine receptors so far. Ultimately, we screened one ligand, which could significantly enhance the dimer interaction, and revealed its regulation mechanism on the drug binding for the orthosteric site. The observations could provide valuable information for the development of the GPCR PPI drugs.
Materials and Methods
Workflow
Figure 1 shows the entire workflow. Considering the protein flexibility, 300 ns MD is first carried out for the crystal structure of CXCR4 homo-dimer. Then, according to the root-mean-square-deviation (RMSD) of residues of the dimer interface, eight representative conformations are obtained through clustering. The druggable pocket of the dimer interface is identified by FTmap. Based on the pocket, ligands targeting the PPI interface are screened, and then 1us MD simulations are performed for the four representative complexes of the dimer bound by the PPI ligand. Finally, we discussed the effect of the ligand on the dimerization and the screening performance of the orthostatic site to antagonists, and revealed its regulation mechanism.
System Preparation
X-ray crystal structure of CXCR4 dimer with a resolution of 2.5 Å was obtained from PDB bank (PDBID: 3 ODU) (Wu et al., 2010). We removed ligands and other non-essential components used for crystallizing and purification, including T4 lysozyme (T4L) inserted between transmembrane (TM) helices V and VI at the cytoplasmic side of the receptor, small isothiourea derivative (IT1t). In addition, crystal water molecules outside the receptor were also deleted. But the crystal water molecules inside the receptor were retained. All protein residues were set to the standard CHARMM protonation state under physiological pH. The receptor was inserted into a palmitoyl-oleoyl-phosphatidyl-choline (POPC) (Filizola et al., 2006) bilayer. Then, water molecules were added to the system, which was described by the TIP3P model. The whole system was neutralized with 0.15M NaCl by CHARMM-GUI (Lee et al., 2015). According to the tertiary structure information of the protein system, the two subunits were manually added with two disulfide bonds between Cys28N-term and Cys2747.25, Cys1093.25, and Cys1865.50.
MD Simulation
All molecular dynamics simulations were performed by the sander module of AMBER 16 (Case et al., 2016). The MD trajectories were analyzed using the correlation analysis module of AMBER 16 and VMD, as well as some other specific trajectory analysis softwares. Ff14SB force field (Maier et al., 2015) was used for the receptor and the lipid14 force field (Dickson et al., 2014) was utilized for the POPC lipids. Twenty thousand step energy minimization was performed to eliminate bad contacts in the initial structures. After the minimization, the entire system was heated from 0 K to 310 K within 250 ps, then 5 ns NVT pre-equalization was performed at 310 K temperature. Finally, 300 ns and 1 us simulations were carried out using the NPT ensemble at 300 K and 1 bar for the apo dimer system and the dimer bound the PPI ligand, respectively. The cutoff distance of 10 Å was set for nonbonded interactions and the electrostatic interaction was computed by the particle mesh Ewald (PME) algorithm (Essmann et al., 1995). The SHAKE algorithm (Berendsen et al., 1984) was used to constrain all hydrogen-containing bonds. The time step was 2-fs and trajectories were saved at interval of 10 ps for further analysis.
Clustering Analysis
For the last 200 ns trajectory of the apo dimer system, clustering was carried out using the k-means algorithm (Han and Zhang, 2009; Li et al., 2014) embedded in the ptraj module of the AmberTools package in terms of RMSD of the backbone atoms of 136 residues of TM5-TM6/TM5-TM6 interface (Wu et al., 2010). Consequently, eight classes were obtained (vide in Supplement 1) and the center of each class was selected as a representative conformation for subsequent analysis.
FTMap Analysis
FTmap analysis (Kozakov et al., 2015) was performed in order to identify the druggable pocket in the dimer interface, using FTMap computational map server. The server probes small molecule binding sites using CSM method (Dennis et al., 2002), which places molecular probes on a protein surface to identify the most favorable binding positions. The eight representative structures from the clustering above were individually computed using this server (www.ftmap.bu.edu). Pymol (Janson et al., 2016) was utilized to inspect visually the results.
Virtual Screening
A ligand set was constructed by a focused chemical compound collection (iPPI-lib) with a total of 51,232 ligand molecules, which was tuned to target PPIs. The PPI-specific database was provided by MTiOpenScreen (Labbé et al., 2015). First, the initial drug-like compounds containing 384,372 PubChem molecules was selected and collected. Then PPI-HitProfiler (Reynès et al., 2010) was used to select PPI-friendly compounds. Finally, these molecules were aggregated by Cluster Molecule Protocol (Accelrys Pipeline Pilot v8.5), resulting in 51,232 drug-like molecules in the final iPPI-lib. Approximately 4,000 molecules (including isomers) were obtained through preliminary screening of MTiOpenScreen, and further docking evaluations were performed using Autodock 4.2 (Morris et al., 2009). All docking input files were prepared by AutoDockTools 1.5.6 (Sanner, 1999) package, and Lattice files for active sites were generated by the AutoGrid 4.2. In order to cover the ligand-binding site, the box site was set to 75 Å × 75 Å × 75 Å with 0.375 Å spacing. The dockings with the flexible ligand and the rigid receptor were performed by AutoDock 4.2. To ensure the accuracy of the result, each ligand was done by 100 docking calculations separately, and 1,000,000 energy evaluations were carried out using Lamarck genetic algorithm for each docking calculation. We selected the docking pose with the lowest binding energy as the best binding mode for further analysis. The ROC (Metz, 1978) plot was used to assess virtual screening performance, which is a curve of true-positive rates versus false-positive rates. They could be calculated in terms of the following equations.
Where TP (true positive) and FN (false negative) refer to the number of active substances in positive and negative classes, respectively. FP (false positive) and TN (true negative) refer to the number of decoys in positive and negative classes, respectively. The AUC is the area under the receiver operating characteristic curve (Hanley and McNeil, 1982). The larger AUC value, the better the performance of the receptor in screening the active molecules from the decoys. For example, when the AUC value is 0.5, it represents random screening. When the AUC value is 1, the receptor has the strongest ability to screen the active molecules. The AUC value could reflect the affinity of the receptor to a class of active molecules in the ligand set. Therefore, it has been widely used to characterize the performance of virtual screening.
MMPBSA
As accepted, molecular Mechanics Poisson-Boltzmann surface area (MM/PBSA) (Sun et al., 2014; Sun et al., 2018; Wang et al., 2019; Weng et al., 2019) is a versatile method to calculate the binding free energy ΔGbinding between two molecules in terms of equation (3).
Herein, Gcomplex, Greceptor and Gligand denote the free energies of the complex, receptor, and ligand, respectively, which could be calculated by MMPBSA.py.MPI algorithm (Miller III et al., 2012) of the SANDER module [vide equations (4)–(6)].
The gas phase energy (Egas) is calculated by the internal energy (Eint), the electrostatic interaction energy (Eele) and van der Waals interaction energy (Evdw) in equation (5). Gsol denotes the solvation energy, which consists of polar solvation energy (Gpsolv) and the nonpolar solvation (Gnpsolv) [vide equation (6)]. Gpsolv could be obtained by solving the Poisson-Boltzmann equation while Gnpsolv could be estimated by γ×SASA. Herein, γ uses 0.0072 kcal Å-2 value and SASA denotes the solvent-accessible area of the molecular. The dielectric constants are set to be 1 for the receptor interior and 80 for the external water. T represents absolute temperature and S is the total conformational entropy. Similar to many computational studies (Niu et al., 2017; Tu et al., 2018), the contribution of entropy is not considered in the calculation of free energy since we mainly concern with the relative change of the binding energy, rather than its absolute value.
Protein Structure Network
Protein structure network (PSN) (Kannan and Vishveshwara, 1999) could exhibit the structure of proteins as an interaction network. In PSN, residues are served as nodes. If the percentage of interaction [vide equation (7)] between the two nodes is greater than or equal to a given cutoff, the two nodes are connected to one edge.
In equation (7), Iij represents the percentage of interaction between nodes i and j, and nij represents the pair number of side chain atoms within a given distance cut-off range (the default cutoff is 4.5 Å). Ni and Nj are the normalization factors of residue i and j, respectively. Based on the network, we could gain insight into inter-residue communication, which play a vital role for proteins to execute their biological functions. Consequently, PSN has been successfully applied to study unfolding, stability and allosteric interaction (Brinda and Vishveshwara, 2005; Vishveshwara et al., 2009; Gao et al., 2016).
In addition, the shortest paths between pairs of nodes could be obtained through searching PSN by Dijkstra’s algorithm (Dijkstra, 1959), which considers the PSN node inter-connectivities and residue correlated motions. The dynamic cross-correlation (DCC) (McCammon and Harvey, 1988) could be evaluated along an MD trajectory, in which DCC values (Cij) are computed in terms of equation (8):
i and j denotes atoms or residues, and ri(t) and rj(t) are the corresponding position vectors at time t. means the ensemble average over a period time. DCC could characterize the extent of atom or residue movement correlations within a range from 1.0 to -1.0, where 1.0 indicates completely correlated displacements and -1.0 denotes completely anti-correlated displacements. Cross correlation analysis and PSN were performed using Wordom software (Seeber et al., 2011).
Results and Discussion
Prediction of the Druggable Pocket in the Interface of the CXCR4 Dimer Based on Representative Conformations
The crystal structure is not completely equal to the functional conformation due to the flexibility of protein, which play a crucial role in the protein function. Thus, we first performed 300 ns MD simulation to obtain representative conformations for the apo dimer. Figure 2 shows the root-mean-square-deviation (RMSD) of the backbone atoms with respect to its crystal structure for the dimer. It can be seen that the RMSD values present minor fluctuations after 100 ns. Thus, we used the k-means algorithm to cluster the last 200 ns trajectories, based on RMSD of the backbone atoms of 136 residues of the dimer interface (Wu et al., 2010). Consequently, eight classes of the conformations were obtained, as shown in Supplement 1. Figure 3 shows the proportion of conformations for each class and populations of the a and b classes are significantly higher than the other classes. The center of each class was selected as representative conformation to probe its druggability with the aid of FTmap method. Only one druggable pocket was identified in the dimer interface for the classes a, b, d, and g, which account for 72% conformations of the last 200 ns trajectories, thus being highly representative. Furthermore, the druggable pockets in the dimer interfaces are highly similar for the four classes, which are mainly involved in Trp1955.34, Val1985.37, Phe1995.38 of the subunit A, Val1975.36, Gln2005.39, Phe2015.40, Ile2596.55, Ser2606.56, Ser2636.59, and Leu2676.63 of subunit B. It was revealed from the CXCR4 crystal structure that the residue Trp1955.34, Val1975.36, Val1985.37, Phe2015.40, and Leu2676.63 play an important role in the dimerization of dimer (Wu et al., 2010). Thus, it can be assumed that a ligand targeting the pocket could significantly influence the dimerization of CXCR4.
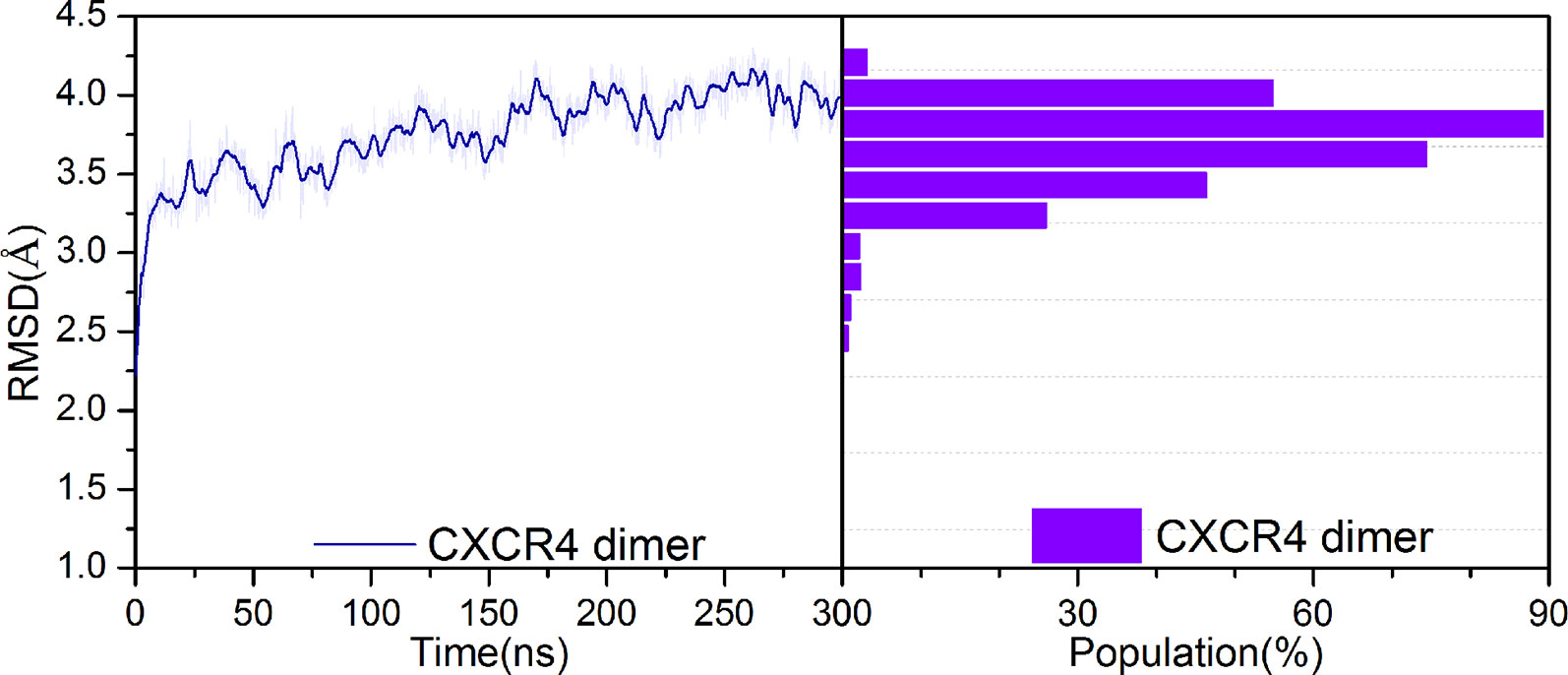
Figure 2 Changes in RMSD values of backbone atoms for the apo CXCR4 dimer along with simulation time (left) and its distribution (right).
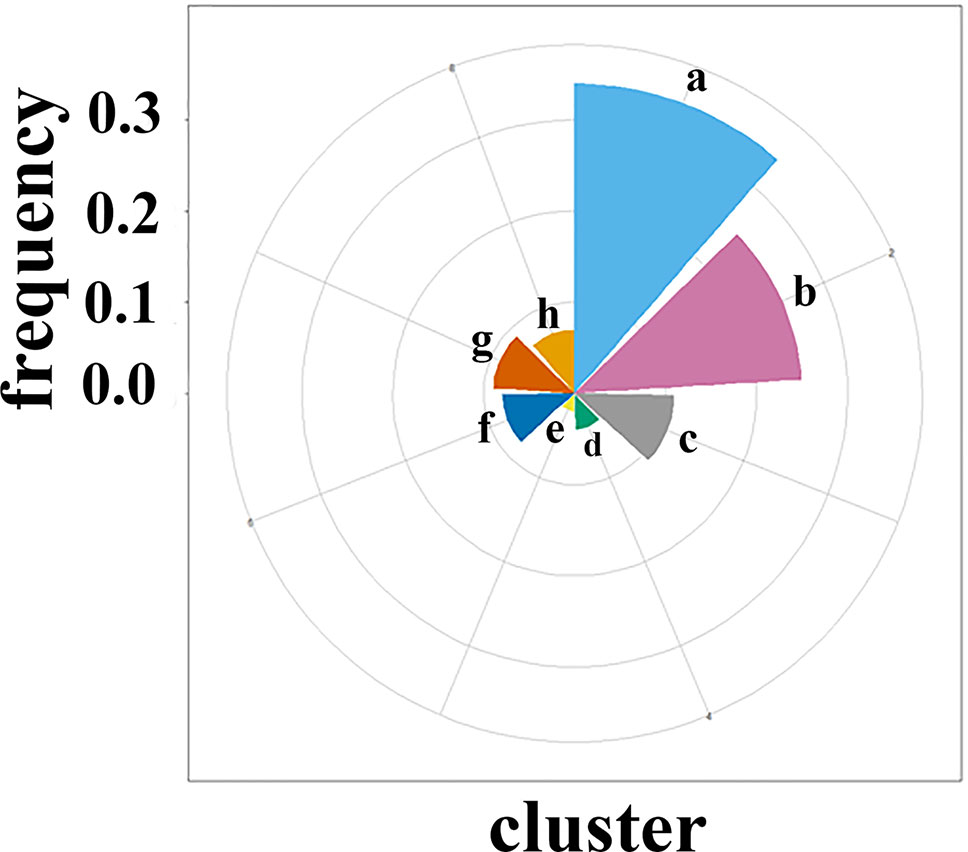
Figure 3 The proportion of the eight representative conformations obtained by clustering in the last 200-ns trajectory for the apo CXCR4 dimer. One druggable pocket was identified by FTmap only for four types of conformations a, b, d, and g.
Screening Potential Ligands to the Druggable Pocket in the Dimer Interface
The ligand set was constructed by a focused chemical compound collection (iPPI-lib) with a total of 51,232 ligand molecules, which were docked to the four representative conformations of the a, b, d, and g classes. The complex with the lowest binding energy was selected as the best binding mode for each of the four classes. Consequently, four small molecules (vide in Figure 4) were screened for the four representative conformations, which present best binding. The ligands 1, 2, 3, and 4 correspond to the conformations a, b, d, and g, respectively. Table 1 lists some important physicochemical properties calculated by the SwissADME (Daina et al., 2017) for the four small molecules. It can be seen that their molecular weights are between 340 and 500. LogP values are between 3 and 5. LogS values are between -5 and -6. These properties are in line with those of the PPI drugs reported. Furthermore, the four molecules satisfy “Rule-of-Five” proposed by Lipinski (Lipinski et al., 1997; Lipinski, 2004), which indicates MW≤500, Log P≤5, N or O ≤10, NH or OH≤5, maybe potential drugs.
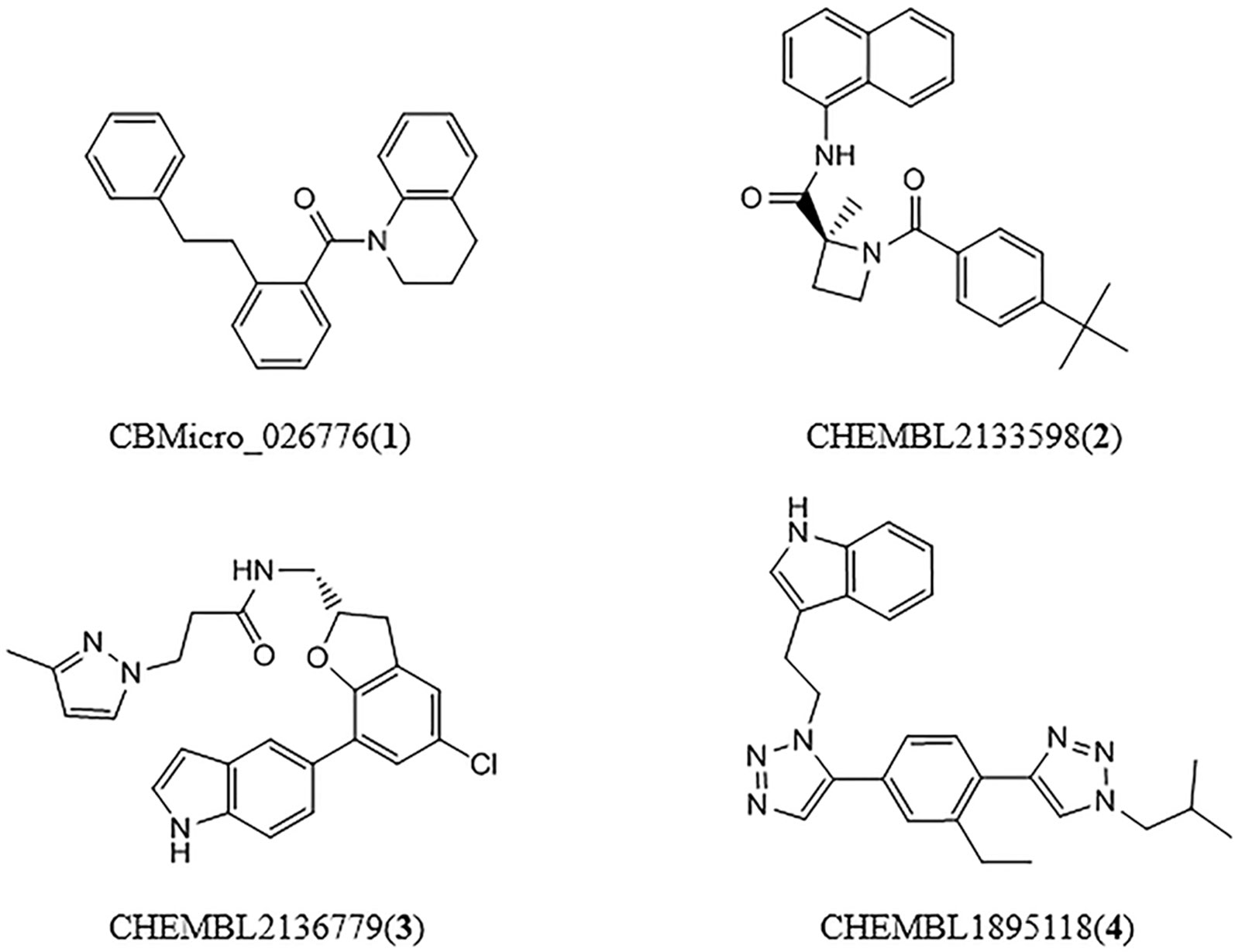
Figure 4 Chemical structural formulas of four ligands (ligand 1 binds to conformational a, ligand 2 binds to conformational b, ligand 3 binds to conformational d, ligand 4 binds to conformational g).
Effect of the Four Ligands on the CXCR4 Dimerization
In order to probe the impact of the four ligands on the dimerization of CXCR4, we further performed 1us MD simulation for the four dimer conformations, the interfaces of which were docked by the individual ligand. The centroid distance and the contact area between the two subunits of the CXCR4 dimer were calculated based on the 1 us trajectory, as shown in Figure 5. It can be seen from Figure 5 that only the ligand 1 targeting the conformation a reduces the centroid distance between the two subunits and increases their contact area, suggesting enhanced dimerization. However, an opposite trend is presented for the conformations b and d. For the conformation g, the two parameters change little. The observations indicate that the ligand 1 could enhance the dimerization of CXCR4 while the ligands 2 and 3 disfavor the dimerization. The ligand 4 only plays a negligible role in the dimerization. Since our objective is to search the PPI ligand enhancing the dimerization, we only focused on the ligand 1 in the following analysis.
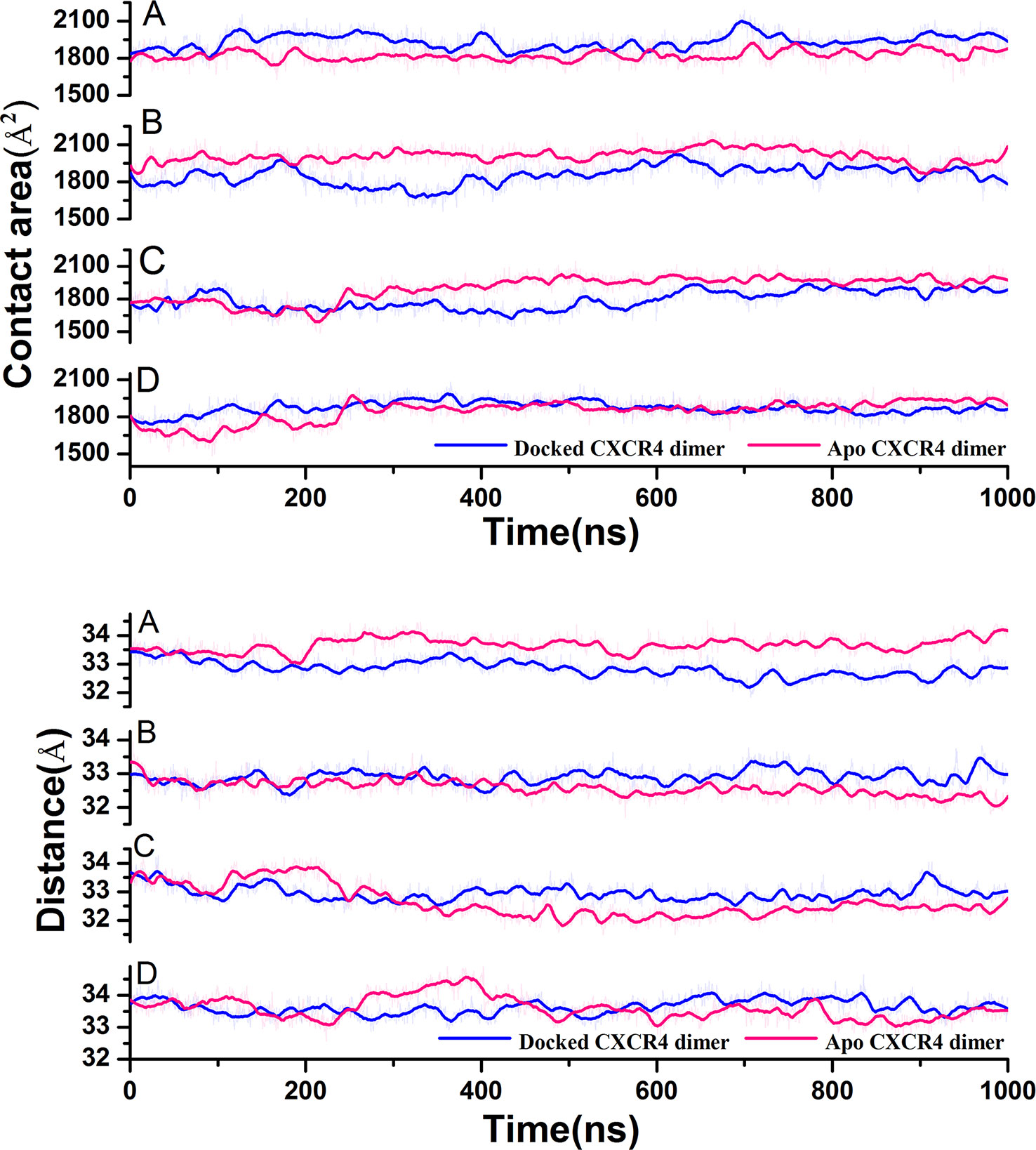
Figure 5 Variations of the contact area and the centroid distance between the two protomers along with simulation time for the CXCR4 dimer with and without the ligand targeting the PPI interface (A) Corresponds to conformation a, (B) Corresponds to conformation b, (C) Corresponds to conformation d, (D) corresponds to conformation g).
Interaction Energy Between the Ligand 1 and the CXCR4 Dimer
In order to estimate the interaction strength between the dimer and the ligand, the binding free energy between them was calculated using the MM-PBSA method, based on the last 100 ns trajectory, as shown in Table 2. The ΔGbinding value is -46.77 kcal/mol and van der Waals interaction is main driving force, as judged from -46.18 kcal/mol of ΔEvdw. In the other words, van der Waals interaction devotes main contributions for the interaction between the CXCR4 dimer and the ligand 1.
To identify important residues contributed to the ligand binding, we decomposed the binding free energy into the corresponding residue. Figure 6 shows residues with binding energy less than -1 kcal mol-1, including residues Phe2015.40, Ile2045.43, and Phe2646.60of the subunit A, residues Ile1694.58, Pro1704.59, Ile1734.62, Val1985.37, and Phe1995.38of the subunit B. To identify important groups of the ligand contributed to the binding, we also calculated the interaction between the CXCR4 dimer and the ligand using protein–ligand interaction analysis software (PLIP) (Salentin et al., 2015). Figure 7 shows the interaction mode between the CXCR4 dimer and the ligand 1 before the simulation and after that. Herein, the snapshot of the lowest energy in the last 100 ns MD trajectory was selected as representative conformation for calculating the binding mode after the simulation. It can be seen from Figure 7 that the benzene ring of the small molecule devotes main contribution to the hydrophobic interaction between the ligand and the dimer, indicating the importance of the benzene group of the ligand in enhancing PPI. A comparison of the interaction modes in Figure 7 indicates that TM5 mainly contributes to the binding before the simulation while TM4 also devotes to the binding besides TM5 after 1us simulation. Thus, it should be the interaction between TM4 and the ligand that drives the two subunits closer.
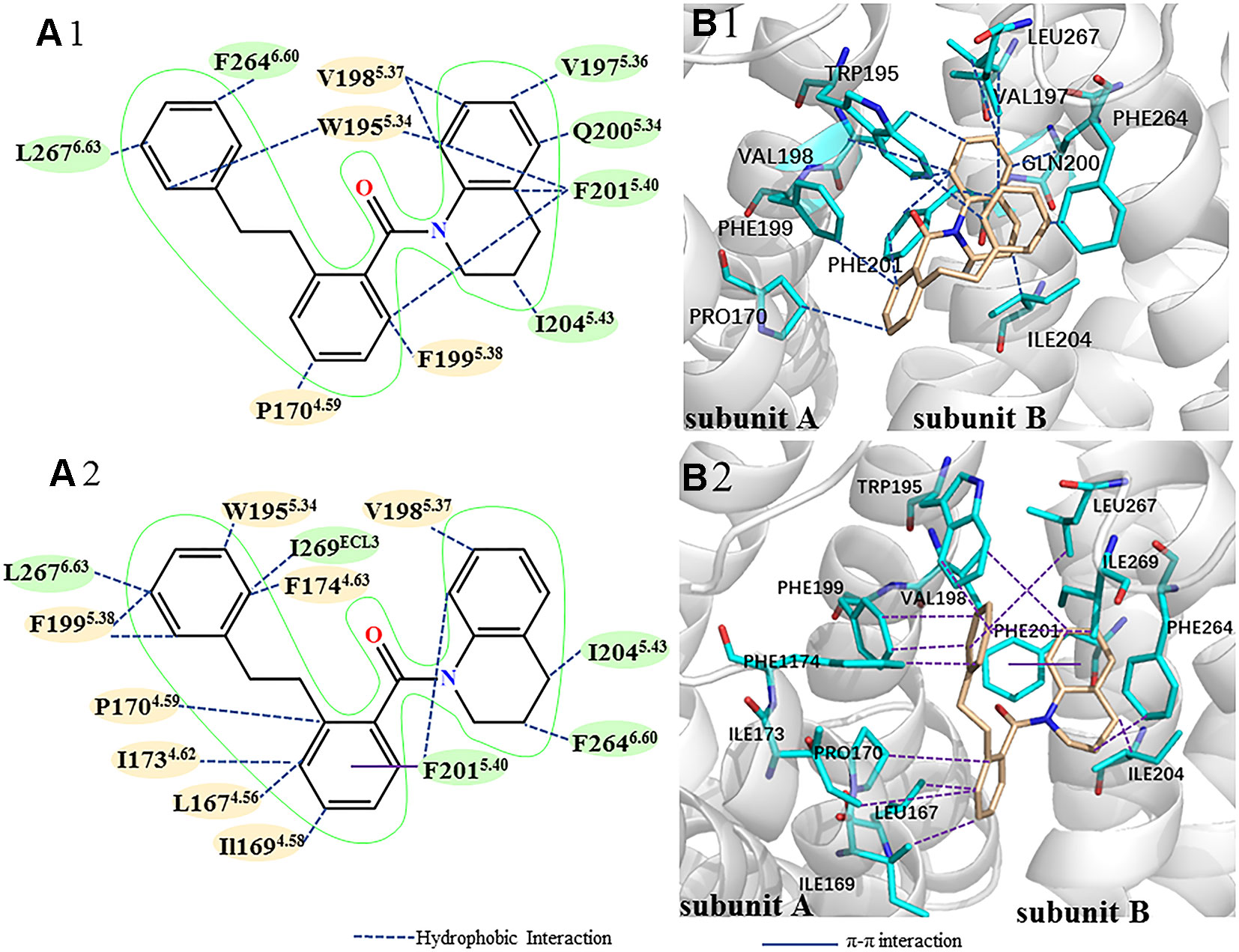
Figure 7 The comparison of binding modes between the CXCR4 dimer and the PPI ligand before (Top) and after (Bottom) 1us simulation. (A) 2D diagram of binding mode between the CXCR4 dimer and the ligand (yellow represents residues from the subunit A, green represents residues from the subunit B). (B) 3D diagram of binding mode between the CXCR4 dimer and the ligand, blue for residues, orange for the ligand. Hydrophobic and π-π interactions are represented by different lines.
Effect of Ligand 1 on Drug Screening of Orthosteric Site
Since the existing drugs targeting CXCR4 are mainly antagonists, we, herein, focused the impact of the PPI ligand on the selectivity of the orthosteric site to the antagonists. One ligand set was constructed. The active molecules are extracted from the ZINC database (Irwin et al., 2012), GPCR-ligand database (Okuno et al., 2007) and PubChem database (Kim et al., 2015). The decoys stem from the DUD-E database (Mysinger et al., 2012). The ratio of decoys to the active molecules (N activity/N decoy) is 1:36. Consequently, the ligand set contains 1,480 small molecules (40 antagonists and 1,440 decoys). The ligand set was docked to the orthosteric site of the receptor (Wu et al., 2010; Venkatakrishnan et al., 2013; Qin et al., 2015), which consists of Lys251.19, Cys281.22, Arg301.24, Asp972.64, His1133.29, Asp1714.60, Cys1865.25, Asp1875.26, Asp2626.58, Glu2777.28, His2817.32, and Glu2887.32. Figure 8 shows the receiver operating characteristic (ROC) curve and the area (AUC) under the ROC.
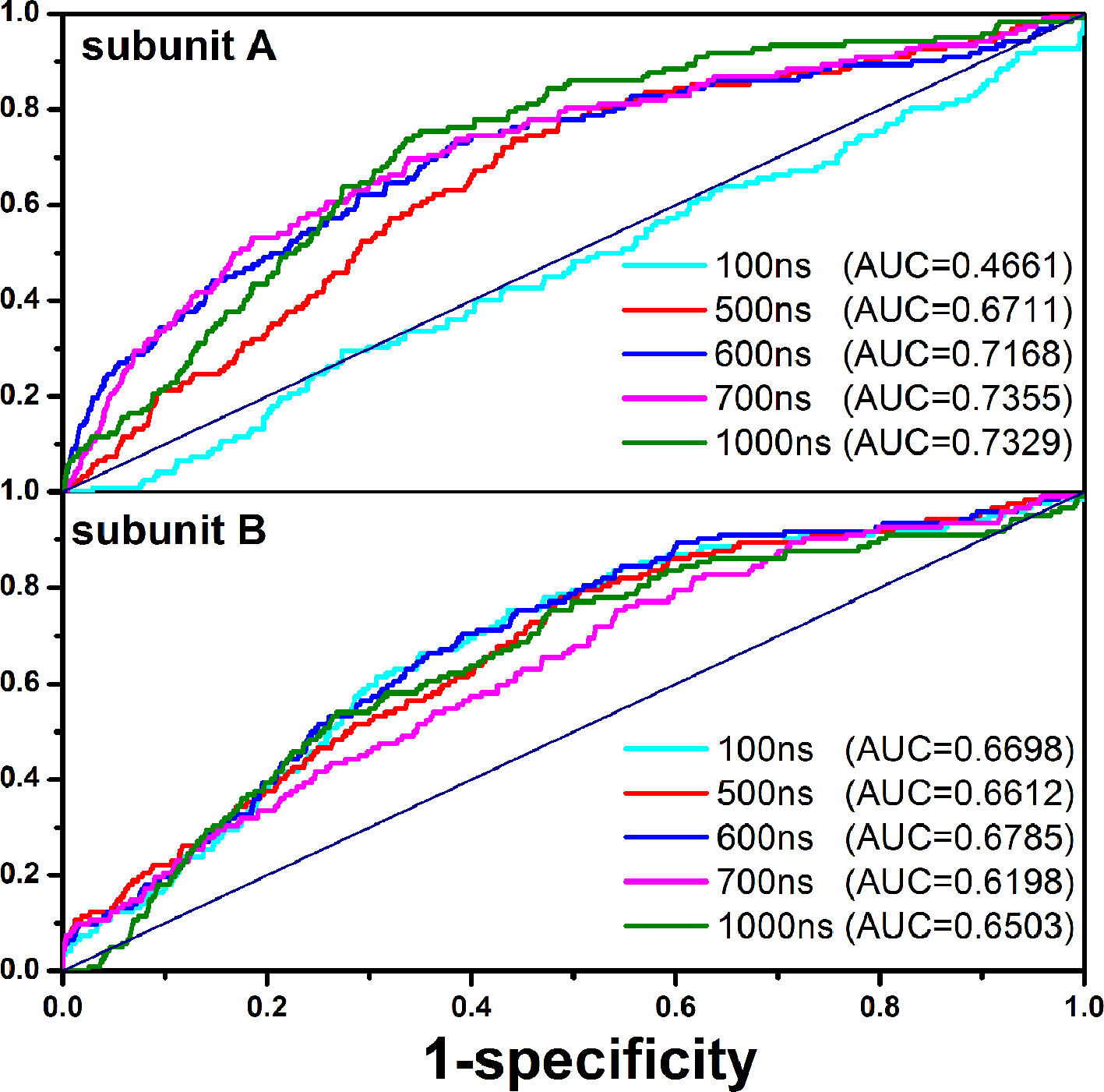
Figure 8 Effects of the PPI ligand on the screening performance of the orthosteric site to the antagonists for the subunit A (top) and the subunit B (bottom).
It can be seen that the screening performance of the subunit A increases with the simulation time (AUC=0.4661 at 100 ns, AUC=0.6711 at 500 ns, and AUC=0.7329 at 1,000 ns). However, there is little change for the subunit B (AUC=0.6698 at 100 ns, AUC=0.6612 at 500 ns, and AUC=0.6503 at 1 us). In addition, we also compared the drug screening performance of the orthosteric site between the CXCR4 dimer bound the PPI ligand and one without the ligand, as shown in Figure 9. Similarly, the PPI ligand improves the screening performance of the subunit A but nearly has no effect on the subunit B, exhibiting asymmetric regulation. The asymmetric effect was also observed for the activation and the ligand binding for some GPCR dimers (Han et al., 2009; Liu et al., 2017).
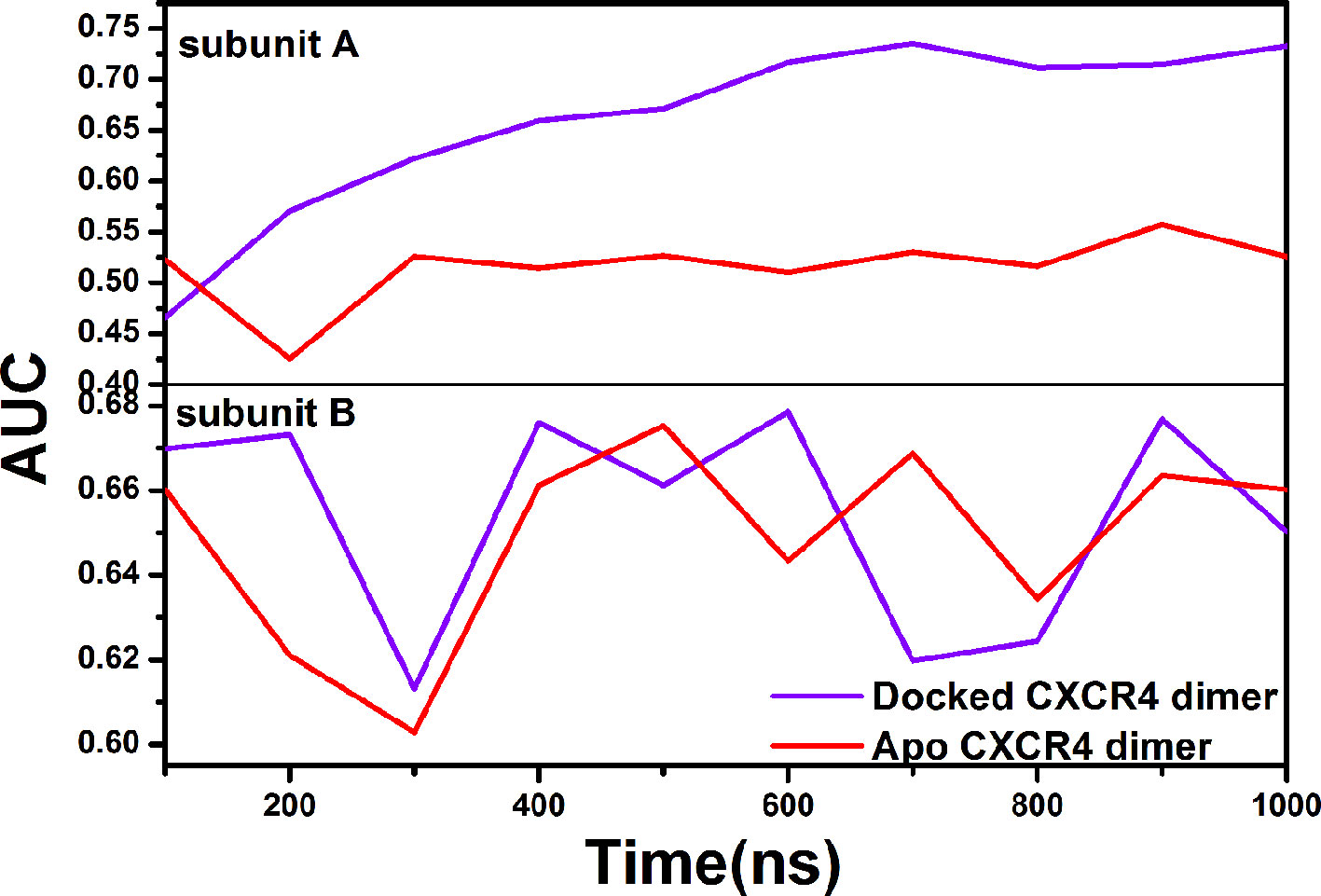
Figure 9 Comparison of the screening performance for the orthosteric sites of the subunit A and the subunit B between the apo CXCR4 dimer (red lines) and the CXCR4 dimer bound by the PPI ligand (purple lines).
In order to probe the origin of the asymmetric impact of the PPI molecule on the ligand binding of the orthosteric site for the two subunits, we calculated the pocket volumes of the orthosteric sites of CXCR4, as shown in Figure 10. It is clear that the PPI ligand significantly increases the volume of the orthosteric pocket for the subunit A but plays a minor role in the subunit B, which should contribute to the asymmetric screening.
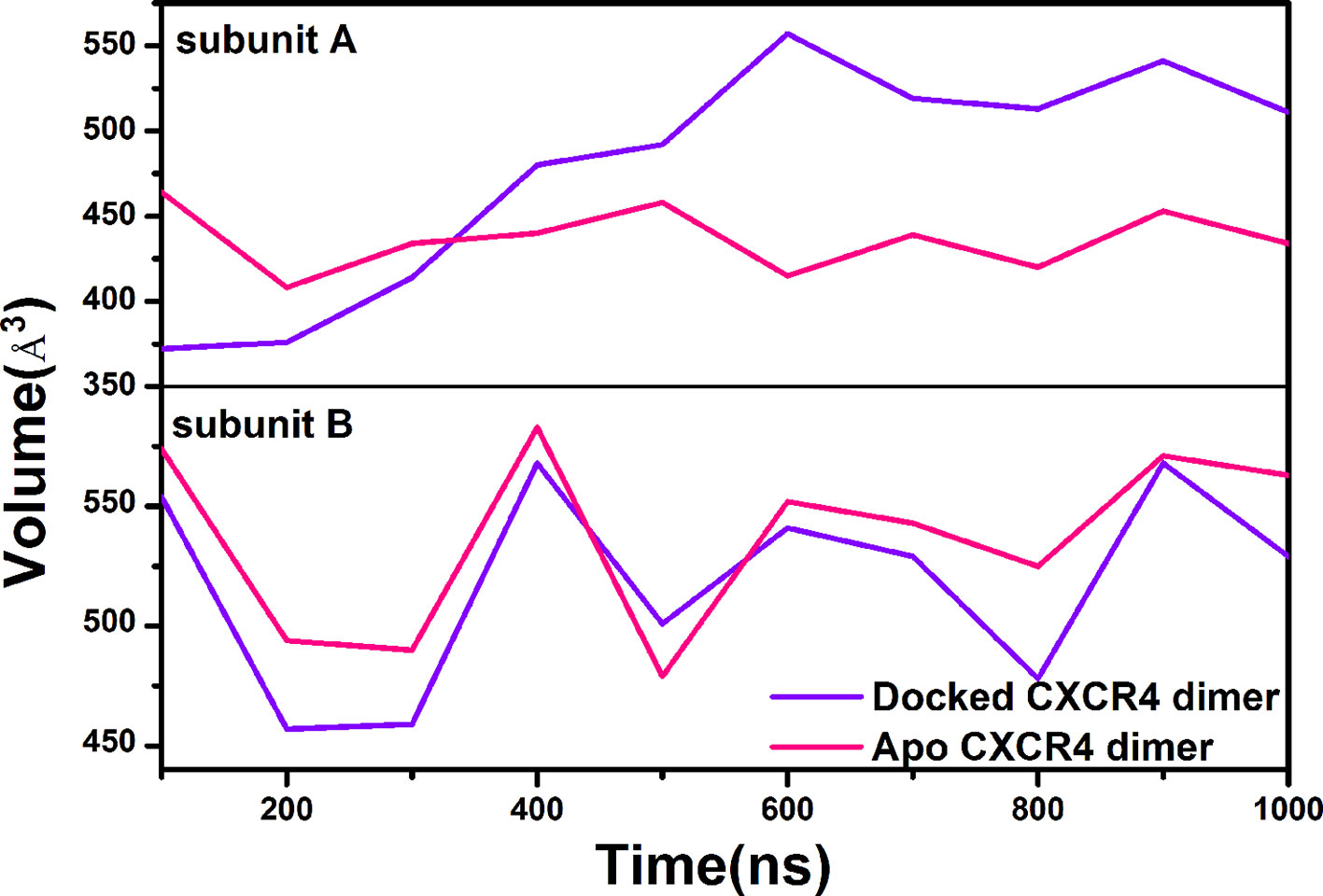
Figure 10 Comparison of volumes for the orthosteric pockets of the subunit A and the subunit B between the apo CXCR4 dimer (red lines) and the CXCR4 dimer bound by the PPI ligand (purple lines).
The Allosteric Pathway for the Regulation Impact of the PPI Molecule on the Ligand Binding of the Orthosteric Site
In order to probe how the PPI molecule regulates the ligand binding of the orthosteric site of the receptor, we used the protein structure network to identify the allosteric pathway between the druggable pocket in the dimer interface and the orthosteric site of the two subunits. The residues consisted of the two types of the binding pockets are served as the starting and ending nodes in the PSN calculation, respectively, based on the last 100 ns equilibrium trajectory. Table 3 lists the number of main pathways with frequency higher than 30%. Compared to the dimer without the PPI small molecule, the binding of the PPI molecule significantly increases the number of the pathway for the subunit A while there is little change for the subunit B. The observation suggests that the PPI ligand enhances the role of the interface in regulating the orthosteric site of the subunit A but only plays minor role for the subunit B. As a result, the volume of the orthosteric pocket is increased for the subunit A while the slight change is observed for that of the subunit B. In order to identify important residues in the allosteric regulation pathway, we searched the shortest pathway with the highest frequency between the PPI pocket and the orthosteric pocket for the subunit A. It can be seen from Figure 11 that the pathway is composed of Trp1955.34–Tyr190ECL2–Val1965.35–Gln2005.39–Asp2626.58–Cys28N-term. As revealed above, Trp1955.34 is an important residue contributed to the binding of the PPI ligand. Residue Tyr190ECL2 locates in ECL2, which was revealed to be switch for the ligand binding in the orthosteric site (Scarselli et al., 2007; Arkin et al., 2014). Residue Gln2005.39 plays a specific role in the dimerization of CXCR4 dimer (Altwaijry et al., 2017). The residues Cys2747.25 and Cys28N-term bind closely through disulfide bonds, which play an important role in the formation of entrance to the ligand binding pocket at orthosteric site (Wu et al., 2010; Pawig et al., 2015). Residue Asp2626.58 is an important residue for the binding of orthosteric site ligands (Wu et al., 2010; Qin et al., 2015). It can be seen that most of the residues composed of the allosteric pathway are associated with the ligand binding, which should be the reason why the PPI ligand significantly affect the ligand binding pockets, in turn influence its screening performance to the ligands. Although there is no report on the importance of the residue Val1965.35 of this pathway, our observations suggest that the residue Val1965.35 is also important for the ligand binding of the dimer and should be concerned by experiments. In addition, we also searched the shortest pathway with the highest frequency between the PPI pocket and the orthosteric pocket of the subunit B, as shown in Supplement 2. The pathway is composed of Ile269ECL3–Phe2646.60–Ile270ECL3–Ile2656.61–Glu2777.28, only Glu2777.28 of which was reported to be the pocket residue of the orthosteric site (Wu et al., 2010; Venkatakrishnan et al., 2013; Qin et al., 2015). Compared to the pathway of the subunit A, there are fewer residues involved in the ligand binding for that of the subunit B, which should contribute to the observation above that the PPI ligand plays a minor role in influencing the screening ability of the subunit B to the antagonists.
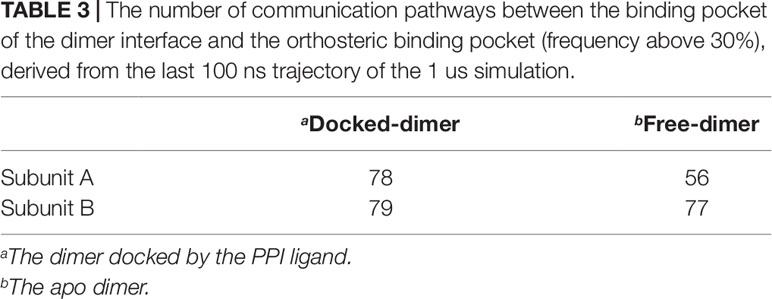
Table 3 The number of communication pathways between the binding pocket of the dimer interface and the orthosteric binding pocket (frequency above 30%), derived from the last 100 ns trajectory of the 1 us simulation.
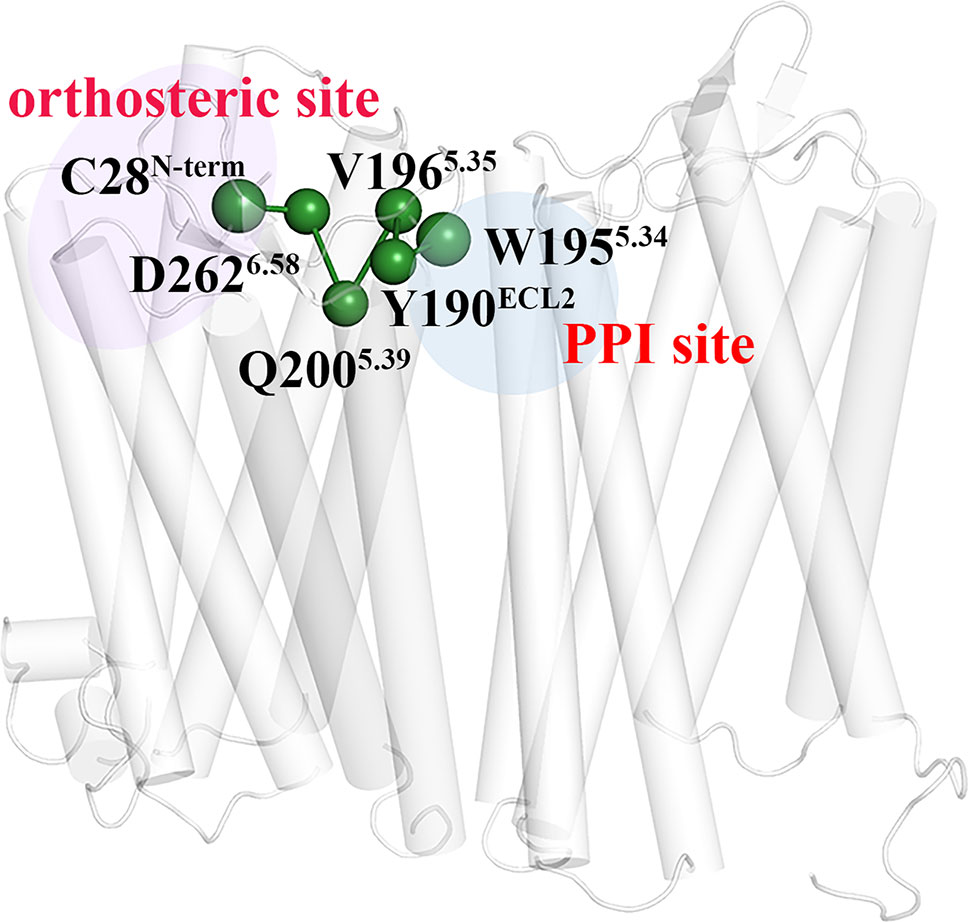
Figure 11 The shortest pathway with the highest frequency between the druggable pocket on the dimer interface and the orthosteric site of subunit A. The blue region represents the PPI pocket. The purple region represents the orthosteric site of the subunit A. The green balls represent the residues composed of the pathway.
Conclusions
PPIs offer a rich source of novel drug targets. As the largest family of drug-targeted proteins, it was evidenced that GPCRs could form the dimers through the protein–protein interaction. Unfortunately, the drugs targeting the PPI interface of the GPCR dimers have not been explored so far. In the work, we utilized molecular dynamics simulation coupled with the virtual screening and the protein structure network to probe the druggability in the PPI interface of CXCR4 homodimer and its regulation mechanism on the receptor structure and the drug screening ability of the orthosteric site.
One druggable pocket is identified in the PPI interface. One small molecule is screened from the PPI drug-like small molecule dataset which could enhance the dimerization mainly through hydrophobic interactions between the benzene rings of the PPI molecule and TM4 of the receptor. The enhancement of PPI by the small molecule changes the screening performance of the two subunits to the antagonists targeting the orthosteric pocket. One subunit exhibits an enhanced screening performance to the antagonists while the minor change is observed for the other subunit, exhibiting an asymmetric cooperativity. The structural analysis indicates that the negative cooperativity should be attributed to the asymmetric change in the orthosteric pocket volumes induced by the binding of the PPI molecule, which leads to the significant increase in the pocket volume of the subunit A but only plays a minor role for the subunit B.
The results of PSN reveal that the number of the regulatory pathways from the PPI pocket to the orthosteric pocket is significantly increased for the subunit A while a minor change is observed for the subunit B, which should contribute to the asymmetric change of the binding pockets between the two subunits. In addition, one main regulatory pathway from the PPI binding site to the pocket of the subunit A is identified, revealing that the PPI ligand molecule allosterically regulates the structural change of the orthosteric pocket of the subunit A mainly through the pathway consisted of Trp1955.34–Tyr190ECL2–Val1965.35–Gln2005.39–Asp2626.58–Cys28N-term. These residues were revealed to significantly contribute to the dimerization and the ligand binding to the PPI interface and the orthosteric site. Consequently, the PPI small molecule could significantly regulate the dimerization and the screening ability of the orthosteric site to the ligands.
It is first time revealed the druggability of the GPCR dimer interface and its role in influencing the drug recognition ability of the orthosteric site. Since the antagonists of CXCR4 are used to treat CXCR4-related diseases like AIDS and some cancers, it is reasonable to assume that the PPI molecule identified from the work should enhance their drug efficacies. In addition, the strategy proposed by the work could be applied to probe the other GPCR PPI drugs.
Data Availability Statement
All datasets generated for this study are included in the article/Supplementary Material.
Author Contributions
XP designed the experiments. YY and YG finished all the molecular dynamic simulations and analyzed the trajectories. LS organized the main manuscript text and plotted all the figures. XP, CL, and ML revised the manuscript. All authors contributed to the work.
Funding
This project is supported by the National Science Foundation of China (Grant No. 21573151), NSAF (Grand No. U1730127), and National Natural Science Youth Foundation of China (Grand No. 2170511).
Conflict of Interest
The authors declare that the research was conducted in the absence of any commercial or financial relationships that could be construed as a potential conflict of interest.
Supplementary Material
The Supplementary Material for this article can be found online at: https://www.frontiersin.org/articles/10.3389/fphar.2019.01310/full#supplementary-material
References
Altwaijry, N. A., Baron, M., Wright, D. W., Coveney, P. V., Townsend-Nicholson, A. (2017). An ensemble-based protocol for the computational prediction of helix–helix interactions in G Protein-coupled receptors using coarse-grained molecular dynamics. J. Chem. Theroy. Comput. 13 (5), 2254–2270. doi: 10.1021/acs.jctc.6b01246
Arkin, M. R., Tang, Y., Wells, J. A. (2014). Small-molecule inhibitors of protein-protein interactions: progressing toward the reality. Chem. Biol. 21 (9), 1102–1114. doi: 10.1016/j.chembiol.2014.09.001
Bai, Q., Zhang, Y., Li, X., Chen, W., Liu, H., Yao, X. (2014). Computational study on the interaction between CCR5 and HIV-1 entry inhibitor maraviroc: insight from accelerated molecular dynamics simulation and free energy calculation. Phys. Chem. Chem. Phys. 16 (44), 24332–24338. doi: 10.1039/C4CP03331K
Berendsen, H. J., Postma, J.v., van Gunsteren, W. F., DiNola, A., Haak, J. (1984). Molecular dynamics with coupling to an external bath. J. Chem. Phys. 81 (8), 3684–3690. doi: 10.1063/1.448118
Brinda, K., Vishveshwara, S. (2005). A network representation of protein structures: implications for protein stability. Biophys. J. 89 (6), 4159–4170. doi: 10.1529/biophysj.105.064485
Case, D. A., Betz, R., Cerutti, D., Cheatham, T., Darden, T., Duke, R., et al. (2016). AMBER 2016 Reference Manual. CA, USA: University of California: San Francisco, 1–923.
Daina, A., Michielin, O., Zoete, V. (2017). SwissADME: a free web tool to evaluate pharmacokinetics, drug-likeness and medicinal chemistry friendliness of small molecules. Sci. Rep. 7, 42717. doi: 10.1038/srep42717
Damian, M., Pons, V., Renault, P., M’Kadmi, C., Delort, B., Hartmann, L., et al. (2018). GHSR-D2R heteromerization modulates dopamine signaling through an effect on G protein conformation. Proc. Natl. Acad. Sci. U. S. A. 115 (17), 4501–4506. doi: 10.1073/pnas.1712725115
Dennis, S., Kortvelyesi, T., Vajda, S. (2002). Computational mapping identifies the binding sites of organic solvents on proteins. Proc. Natl. Acad. Sci. U. S. A. 99 (7), 4290–4295. doi: 10.1073/pnas.062398499
Dickson, C. J., Madej, B. D., Skjevik, Å.A., Betz, R. M., Teigen, K., Gould, I. R., et al. (2014). Lipid14: the amber lipid force field. J. Chem. Theroy. Comput. 10 (2), 865–879. doi: 10.1021/ct4010307
Dijkstra, E. W. (1959). A note on two problems in connexion with graphs. Numer. Math. 1 (1), 269–271. doi: 10.1007/BF01386390
Essmann, U., Perera, L., Berkowitz, M. L., Darden, T., Lee, H., Pedersen, L. G. (1995). A smooth particle mesh Ewald method. J. Chem. Phys. 103 (19), 8577–8593. doi: 10.1063/1.470117
Ferré, S., Casadó, V., Devi, L. A., Filizola, M., Jockers, R., Lohse, M. J., et al. (2014). G protein–coupled receptor oligomerization revisited: functional and pharmacological perspectives. Pharmacol. Rev. 66 (2), 413–434. doi: 10.1124/pr.113.008052
Filizola, M., Wang, S. X., Weinstein, H. (2006). Dynamic models of G-protein coupled receptor dimers: indications of asymmetry in the rhodopsin dimer from molecular dynamics simulations in a POPC bilayer. J. Comput. Aid. Mol. Des. 20 (7-8), 405–416. doi: 10.1007/s10822-006-9053-3
Gao, N., Liang, T., Yuan, Y., Xiao, X., Zhao, Y., Guo, Y., et al. (2016). Exploring the mechanism of F282L mutation-caused constitutive activity of GPCR by a computational study. Phys. Chem. Chem. Phys. 18 (42), 29412–29422. doi: 10.1039/c6cp03710k
Gherbi, K., May, L. T., Baker, J. G., Briddon, S. J., Hill, S. J. (2015). Negative cooperativity across β1-adrenoceptor homodimers provides insights into the nature of the secondary low-affinity CGP 12177 β1-adrenoceptor binding conformation. FASEB J. 29 (7), 2859–2871. doi: 10.1096/fj.14-265199
Han, L., Yang, Q., Liu, Z., Li, Y., Wang, R. (2018). Development of a new benchmark for assessing the scoring functions applicable to protein–protein interactions. Future Med. Chem. 10 (13), 1555–1574. doi: 10.4155/fmc-2017-0261
Han, M., Zhang, J. Z. (2009). Class I phospho-inositide-3-kinases (PI3Ks) isoform-specific inhibition study by the combination of docking and molecular dynamics simulation. J. Chem. Inf. Model. 50 (1), 136–145. doi: 10.1021/ci900175n
Han, Y., Moreira, I. S., Urizar, E., Weinstein, H., Javitch, J. A. (2009). Allosteric communication between protomers of dopamine class A GPCR dimers modulates activation. Nat. Chem. Biol. 5 (9), 688–695. doi: 10.1038/nchembio.199
Hanley, J. A., McNeil, B. J. (1982). The meaning and use of the area under a receiver operating characteristic (ROC) curve. Radiology 143 (1), 29–36. doi: 10.1148/radiology.143.1.7063747
Huang, J., Chen, S., Zhang, J. J., Huang, X.-Y. (2013). Crystal structure of oligomeric β 1-adrenergic G protein–coupled receptors in ligand-free basal state. Nat. Struct. Mol. Biol. 20 (4), 419–425. doi: 10.1038/nsmb.2504
Irwin, J. J., Sterling, T., Mysinger, M. M., Bolstad, E. S., Coleman, R. G. (2012). ZINC: a free tool to discover chemistry for biology. J. Chem. Inf. Model. 52 (7), 1757–1768. doi: 10.1021/ci3001277
Janson, G., Zhang, C., Prado, M. G., Paiardini, A. (2016). PyMod 2.0: improvements in protein sequence-structure analysis and homology modeling within PyMOL. Bioinformatics 33 (3), 444–446. doi: 10.1093/bioinformatics/btw638
Jiang, H., Deng, R., Yang, X., Shang, J., Lu, S., Zhao, Y., et al. (2017). Peptidomimetic inhibitors of APC–Asef interaction block colorectal cancer migration. Nat. Chem. Biol. 13 (9), 994–1001. doi: 10.1038/nchembio.2442
Jubb, H., Higueruelo, A. P., Winter, A., Blundell, T. L. (2012). Structural biology and drug discovery for protein–protein interactions. Trends Pharmacol. Sci. 33 (5), 241–248. doi: 10.1016/j.tips.2012.03.006
Kannan, N., Vishveshwara, S. (1999). Identification of side-chain clusters in protein structures by a graph spectral method. J. Mol. Biol. 292 (2), 441–464. doi: 10.1006/jmbi.1999.3058
Keskin, O., Tuncbag, N., Gursoy, A. (2016). Predicting protein–protein interactions from the molecular to the proteome level. Chem. Rev. 116 (8), 4884–4909. doi: 10.1021/acs.chemrev.5b00683
Kim, S.-K., Chen, Y., Abrol, R., Goddard, W. A., Guthrie, B. (2017). Activation mechanism of the G protein-coupled sweet receptor heterodimer with sweeteners and allosteric agonists. Proc. Natl. Acad. Sci. U. S. A. 114 (10), 2568–2573. doi: 10.1073/pnas.1700001114
Kim, S., Thiessen, P. A., Bolton, E. E., Chen, J., Fu, G., Gindulyte, A., et al. (2015). PubChem substance and compound databases. Nucleic Acids Res. 44 (D1), D1202–D1213. doi: 10.1093/nar/gkv951
Kozakov, D., Grove, L. E., Hall, D. R., Bohnuud, T., Mottarella, S. E., Luo, L., et al. (2015). The FTMap family of web servers for determining and characterizing ligand-binding hot spots of proteins. Nat. Protoc. 10 (5), 733–755. doi: 10.1038/nprot.2015.043
Kufareva, I., Stephens, B. S., Holden, L. G., Qin, L., Zhao, C., Kawamura, T., et al. (2014). Stoichiometry and geometry of the CXC chemokine receptor 4 complex with CXC ligand 12: Molecular modeling and experimental validation. Proc. Natl. Acad. Sci. U. S. A. 111 (50), E5363–E5372. doi: 10.1073/pnas.1417037111
Labbé, C. M., Rey, J., Lagorce, D., Vavruša, M., Becot, J., Sperandio, O., et al. (2015). MTiOpenScreen: a web server for structure-based virtual screening. Nucleic Acids Res. 43 (W1), W448–W454. doi: 10.1093/nar/gkv306
Lao, J., He, H., Wang, X., Wang, Z., Song, Y., Yang, B., et al. (2017). Single-Molecule imaging demonstrates ligand regulation of the oligomeric status of CXCR4 in living cells. J. Phys. Chem. B. 121 (7), 1466–1474. doi: 10.1021/acs.jpcb.6b10969
Lee, J., Cheng, X., Swails, J. M., Yeom, M. S., Eastman, P. K., Lemkul, J. A., et al. (2015). CHARMM-GUI input generator for NAMD, GROMACS, AMBER, OpenMM, and CHARMM/OpenMM simulations using the CHARMM36 additive force field. J. Chem. Theroy. Comput. 12 (1), 405–413. doi: 10.1021/acs.jctc.5b00935
Li, B., Tang, J., Yang, Q., Li, S., Cui, X., Li, Y., et al. (2017). NOREVA: normalization and evaluation of MS-based metabolomics data. Nucleic Acids Res. 45 (W1), W162–W170. doi: 10.1093/nar/gkx449
Li, Y., Li, X., Ma, W., Dong, Z. (2014). Conformational transition pathways of epidermal growth factor receptor kinase domain from multiple molecular dynamics simulations and Bayesian clustering. J. Chem. Theroy. Comput. 10 (8), 3503–3511. doi: 10.1021/ct500162b
Liang, T., Yuan, Y., Wang, R., Guo, Y., Li, M., Pu, X., et al. (2017). Structural features and ligand selectivity for 10 intermediates in the activation process of β2-Adrenergic receptor. ACS Omega. 2 (12), 8557–8567. doi: 10.1021/acsomega.7b01031
Lipinski, C. A. (2004). Lead-and drug-like compounds: the rule-of-five revolution. Drug Discovery Today: Technol. 1 (4), 337–341. doi: 10.1016/j.ddtec.2004.11.007
Lipinski, C. A., Lombardo, F., Dominy, B. W., Feeney, P. J. (1997). Experimental and computational approaches to estimate solubility and permeability in drug discovery and development settings. Adv. Drug Deliver. Rev. 23 (1-3), 3–25. doi: 10.1016/S0169-409X(96)00423-1
Liu, J., Zhang, Z., Moreno-Delgado, D., Dalton, J. A., Rovira, X., Trapero, A., et al. (2017). Allosteric control of an asymmetric transduction in a G protein-coupled receptor heterodimer. Elife 6, e26985. doi: 10.7554/eLife.26985
Maier, J. A., Martinez, C., Kasavajhala, K., Wickstrom, L., Hauser, K. E., Simmerling, C. (2015). ff14SB: improving the accuracy of protein side chain and backbone parameters from ff99SB. J. Chem. Theroy. Comput. 11 (8), 3696–3713. doi: 10.1021/acs.jctc.5b00255
Maurice, P., Kamal, M., Jockers, R. (2011). Asymmetry of GPCR oligomers supports their functional relevance. Trends Pharmacol. Sci. 32 (9), 514–520. doi: 10.1016/j.tips.2011.05.006
McCammon, J. A., Harvey, S. C. (1988). Dynamics of proteins and nucleic acids. New York: Cambridge University Press. doi: 10.1063/1.2811564.
Metz, C. E. (1978). Basic principles of ROC analysis. Semin. Nucl. Med. 8 (4), 283–298. doi: 10.1016/S0001-2998(78)80014-2
Miao, Y., Caliman, A. D., McCammon, J. A. (2015). Allosteric effects of sodium ion binding on activation of the m3 muscarinic g-protein-coupled receptor. Biophys. J. 108 (7), 1796–1806. doi: 10.1016/j.bpj.2015.03.003
Miller, B. R., McGee, T. D., Swails, J. M., Homeyer, N., Gohlke, H., Roitberg, A. E. (2012). MMPBSA. py: an efficient program for end-state free energy calculations. J. Chem. Theroy. Comput. 8 (9), 3314–3321. doi: 10.1021/ct300418h
Morris, G. M., Huey, R., Lindstrom, W., Sanner, M. F., Belew, R. K., Goodsell, D. S., et al. (2009). AutoDock4 and AutoDockTools4: automated docking with selective receptor flexibility. J. Comput. Chem. 30 (16), 2785–2791. doi: 10.1002/jcc.21256
Muñoz, L. M., Holgado, B. L., Martínez-A, C., Rodríguez-Frade, J. M., Mellado, M. (2012). Chemokine receptor oligomerization: a further step toward chemokine function. Immunol. Lett. 145 (1-2), 23–29. doi: 10.1016/j.imlet.2012.04.012
Mysinger, M. M., Carchia, M., Irwin, J. J., Shoichet, B. K. (2012). Directory of useful decoys, enhanced (DUD-E): better ligands and decoys for better benchmarking. J. Med. Chem. 55 (14), 6582–6594. doi: 10.1021/jm300687e
Navarro, G., Cordomí, A., Casadó-Anguera, V., Moreno, E., Cai, N.-S., Cortés, A., et al. (2018). Evidence for functional pre-coupled complexes of receptor heteromers and adenylyl cyclase. Nat. Commun. 9 (1), 1242. doi: 10.1038/s41467-018-03522-3
Nguyen, H. H., Kim, M. B., Wilson, R. J., Butch, C. J., Kuo, K. M., Miller, E. J., et al. (2018). Design, synthesis, and pharmacological evaluation of second-generation tetrahydroisoquinoline-based CXCR4 antagonists with favorable ADME properties. J. Med. Chem. 61 (16), 7168–7188. doi: 10.1021/acs.jmedchem.8b00450
Niu, Y., Shi, D., Li, L., Guo, J., Liu, H., Yao, X. (2017). Revealing inhibition difference between PFI-2 enantiomers against SETD7 by molecular dynamics simulations, binding free energy calculations and unbinding pathway analysis. Sci. Rep. 7, 46547. doi: 10.1038/srep46547
Villoutreix, O., B., M., Labbe, C., Lagorce, D., Laconde, G., Sperandio, O. (2012). A leap into the chemical space of protein-protein interaction inhibitors. Curr. Pharm. Design. 18 (30), 4648–4667. doi: 10.2174/138161212802651571
Okuno, Y., Tamon, A., Yabuuchi, H., Niijima, S., Minowa, Y., Tonomura, K., et al. (2007). GLIDA: GPCR—ligand database for chemical genomics drug discovery—database and tools update. Nucleic Acids Res. 36 (suppl_1), D907–D912. doi: 10.1093/nar/gkm948
Pawig, L., Klasen, C., Weber, C., Bernhagen, J., Noels, H. (2015). Diversity and inter-connections in the CXCR4 chemokine receptor/ligand family: molecular perspectives. Front. Immunol. 6, 429. doi: 10.3389/fimmu.2015.00429
Pediani, J. D., Ward, R. J., Marsango, S., Milligan, G. (2018). Spatial intensity distribution analysis: studies of G protein-coupled receptor oligomerisation. Trends Pharmacol. Sci. 39 (2), 175–186. doi: 10.1016/j.tips.2017.09.001
Percherancier, Y., Berchiche, Y. A., Slight, I., Volkmer-Engert, R., Tamamura, H., Fujii, N., et al. (2005). Bioluminescence resonance energy transfer reveals ligand-induced conformational changes in CXCR4 homo-and heterodimers. J. Biol. Chem. 280 (11), 9895–9903. doi: 10.1074/jbc.M411151200
Petersen, J., Wright, S. C., Rodríguez, D., Matricon, P., Lahav, N., Vromen, A., et al. (2017). Agonist-induced dimer dissociation as a macromolecular step in G protein-coupled receptor signaling. Nat. Commun. 8 (1), 226. doi: 10.1038/s41467-017-00253-9
Provasi, D., Boz, M. B., Johnston, J. M., Filizola, M. (2015). Preferred supramolecular organization and dimer interfaces of opioid receptors from simulated self-association. PloS Comput. Biol. 11 (3), e1004148. doi: 10.1371/journal.pcbi.1004148
Qin, L., Kufareva, I., Holden, L. G., Wang, C., Zheng, Y., Zhao, C., et al. (2015). Crystal structure of the chemokine receptor CXCR4 in complex with a viral chemokine. Science 347 (6226), 1117–1122. doi: 10.1126/science.1261064
Reddy, K. K., Singh, P., Singh, S. K. (2014). Blocking the interaction between HIV-1 integrase and human LEDGF/p75: mutational studies, virtual screening and molecular dynamics simulations. Mol. Biosyst. 10 (3), 526–536. doi: 10.1039/C3MB70418A
Reynès, C., Host, H., Camproux, A.-C., Laconde, G., Leroux, F., Mazars, A., et al. (2010). Designing focused chemical libraries enriched in protein-protein interaction inhibitors using machine-learning methods. PloS Comput. Biol. 6 (3), e1000695. doi: 10.1371/journal.pcbi.1000695
Rosenbaum, D. M., Rasmussen, S. G., Kobilka, B. K. (2009). The structure and function of G-protein-coupled receptors. Nature 459 (7245), 356–363. doi: 10.1038/nature08144
Sader, S., Anant, K., Wu, C. (2018). To probe interaction of morphine and IBNtxA with 7TM and 6TM variants of the human µ-opioid receptor using all-atom molecular dynamics simulations with an explicit membrane. Phys. Chem. Chem. Phys. 20 (3), 1724–1741. doi: 10.1039/c7cp06745c
Sagar, A., Arif, E., Solanki, A. K., Srivastava, P., Janech, M. G., Kim, S.-H., et al. (2017). Targeting Neph1 and ZO-1 protein-protein interaction in podocytes prevents podocyte injury and preserves glomerular filtration function. Sci. Rep. 7 (1), 12047. doi: 10.1038/s41598-017-12134-8
Salentin, S., Schreiber, S., Haupt, V. J., Adasme, M. F., Schroeder, M. (2015). PLIP: fully automated protein–ligand interaction profiler. Nucleic Acids Res. 43 (W1), W443–W447. doi: 10.1093/nar/gkv315
Sanner, M. F. (1999). Python: a programming language for software integration and development. J. Mol. Graph. Model. 17 (1), 57–61. doi: 10.1016/S1093-3263(99)00019-4
Scarselli, M., Li, B., Kim, S.-K., Wess, J. (2007). Multiple residues in the second extracellular loop are critical for M3 muscarinic acetylcholine receptor activation. J. Biol. Chem. 282 (10), 7385–7396. doi: 10.1074/jbc.M610394200
Seeber, M., Felline, A., Raimondi, F., Muff, S., Friedman, R., Rao, F., et al. (2011). Wordom: a user-friendly program for the analysis of molecular structures, trajectories, and free energy surfaces. J. Comput. Chem. 32 (6), 1183–1194. doi: 10.1002/jcc.21688
Shaik, M. M., Peng, H., Lu, J., Rits-Volloch, S., Xu, C., Liao, M., et al. (2019). Structural basis of coreceptor recognition by HIV-1 envelope spike. Nature 565 (7739), 318–323. doi: 10.1038/s41586-018-0804-9
Sheng, C., Dong, G., Miao, Z., Zhang, W., Wang, W. (2015). State-of-the-art strategies for targeting protein–protein interactions by small-molecule inhibitors. Chem. Soc Rev. 44 (22), 8238–8259. doi: 10.1039/C5CS00252D
Shin, W.-H., Christoffer, C. W., Kihara, D. (2017). In silico structure-based approaches to discover protein-protein interaction-targeting drugs. Methods 131, 22–32. doi: 10.1016/j.ymeth.2017.08.006
Song, X., Chen, J., Zhao, M., Zhang, C., Yu, Y., Lonard, D. M., et al. (2016). Development of potent small-molecule inhibitors to drug the undruggable steroid receptor coactivator-3. Proc. Natl. Acad. Sci. U. S. A. 113 (18), 4970–4975. doi: 10.1073/pnas.1604274113
Stanley, N., Pardo, L., De Fabritiis, G. (2016). The pathway of ligand entry from the membrane bilayer to a lipid G protein-coupled receptor. Sci. Rep. 6, 22639. doi: 10.1038/srep22639
Sun, H., Duan, L., Chen, F., Liu, H., Wang, Z., Pan, P., et al. (2018). Assessing the performance of MM/PBSA and MM/GBSA methods. 7. Entropy effects on the performance of end-point binding free energy calculation approaches. Phys. Chem. Chem. Phys. 20 (21), 14450–14460. doi: 10.1039/c7cp07623a
Sun, H., Li, Y., Shen, M., Tian, S., Xu, L., Pan, P., et al. (2014). Assessing the performance of MM/PBSA and MM/GBSA methods. 5. improved docking performance using high solute dielectric constant MM/GBSA and MM/PBSA rescoring. Phys. Chem. Chem. Phys. 16 (40), 22035–22045. doi: 10.1039/c4cp03179b
Tang, J., Fu, J., Wang, Y., Li, B., Li, Y., Yang, Q., et al. (2019a). ANPELA: analysis and performance assessment of the label-free quantification workflow for metaproteomic studies. Brief. Bioinform. 00 (00), 1–16. doi: 10.1093/bib/bby127
Tang, J., Fu, J., Wang, Y., Luo, Y., Yang, Q., Li, B., et al. (2019b). Simultaneous improvement in the precision, accuracy and robustness of label-free proteome quantification by optimizing Data manipulation chains. Mol. Cell. Proteomics 18 (8), 1683–1699. doi: 10.1074/mcp.RA118.001169
Tortorella, P., Laghezza, A., Durante, M., Gomez-Monterrey, I., Bertamino, A., Campiglia, P., et al. (2016). An effective virtual screening protocol to identify promising p53–MDM2 inhibitors. J. Chem. Inf. Model. 56 (6), 1216–1227. doi: 10.1021/acs.jcim.5b00747
Tu, J., Li, J. J., Song, L. T., Zhai, H. L., Wang, J., Zhang, X. Y. (2018). Molecular modeling study on resistance of WT/D473H SMO to antagonists LDE-225 and LEQ-506. Pharmacol. Res. 129, 491–499. doi: 10.1016/j.phrs.2017.11.025
Van Hout, A., Klarenbeek, A., Bobkov, V., Doijen, J., Arimont, M., Zhao, C., et al. (2018). CXCR4-targeting nanobodies differentially inhibit CXCR4 function and HIV entry. Biochem. Pharmacol. 158, 402–412. doi: 10.1016/j.bcp.2018.10.015
Venkatakrishnan, A., Deupi, X., Lebon, G., Tate, C. G., Schertler, G. F., Babu, M. M. (2013). Molecular signatures of G-protein-coupled receptors. Nature 494 (7436), 185–194. doi: 10.1038/nature11896
Venkatesan, K., Rual, J.-F., Vazquez, A., Stelzl, U., Lemmens, I., Hirozane-Kishikawa, T., et al. (2009). An empirical framework for binary interactome mapping. Nat. Methods 6 (1), 83–90. doi: 10.1038/nmeth.1280
Vishveshwara, S., Ghosh, A., Hansia, P. (2009). Intra and inter-molecular communications through protein structure network. Curr. Protein Pept. Sci. 10 (2), 146–160. doi: 10.2174/138920309787847590
Wang, E., Sun, H., Wang, J., Wang, Z., Liu, H., Zhang, J. Z., et al. (2019). End-point binding free energy calculation with MM/PBSA and MM/GBSA: strategies and applications in drug design. Chem. Rev. 119 (16), 9478–9508. doi: 10.1021/acs.chemrev.9b00055
Wang, L., Yuan, Y., Chen, X., Chen, J., Guo, Y., Li, M., et al. (2018a). Probing the cooperative mechanism of the µ–δ opioid receptor heterodimer by multiscale simulation. Phys. Chem. Chem. Phys. 20 (47), 29969–29982. doi: 10.1039/C8CP06652C
Wang, Z., Kang, Y., Li, D., Sun, H., Dong, X., Yao, X., et al. (2018b). Benchmark study based on 2P2IDB to gain insights into the discovery of small-molecule PPI inhibitors. J. Phys. Chem. B. 122 (9), 2544–2555. doi: 10.1021/acs.jpcb.7b12658
Wells, J. A., McClendon, C. L. (2007). Reaching for high-hanging fruit in drug discovery at protein–protein interfaces. Nature 450 (7172), 1001–1009. doi: 10.1038/nature06526
Weng, G., Wang, E., Chen, F., Sun, H., Wang, Z., Hou, T. (2019). Assessing the performance of MM/PBSA and MM/GBSA methods. 9. Prediction reliability of binding affinities and binding poses for protein–peptide complexes. Phys. Chem. Chem. Phys. 21 (19), 10135–10145. doi: 10.1039/c9cp01674k
Whorton, M. R., Bokoch, M. P., Rasmussen, S. G., Huang, B., Zare, R. N., Kobilka, B., et al. (2007). A monomeric G protein-coupled receptor isolated in a high-density lipoprotein particle efficiently activates its G protein. Proc. Natl. Acad. Sci. U. S. A. 104 (18), 7682–7687. doi: 10.1073/pnas.0611448104
Wu, B., Chien, E. Y., Mol, C. D., Fenalti, G., Liu, W., Katritch, V., et al. (2010). Structures of the CXCR4 chemokine GPCR with small-molecule and cyclic peptide antagonists. Science 330 (6007), 1066–1071. doi: 10.1126/science.1194396
Xue, L., Rovira, X., Scholler, P., Zhao, H., Liu, J., Pin, J.-P., et al. (2015). Major ligand-induced rearrangement of the heptahelical domain interface in a GPCR dimer. Nat. Chem. Biol. 11 (2), 134–140. doi: 10.1038/nchembio.1711
Xue, W., Yang, F., Wang, P., Zheng, G., Chen, Y., Yao, X., et al. (2018). What contributes to serotonin–norepinephrine reuptake inhibitors’ dual-targeting mechanism? the key role of transmembrane domain 6 in human serotonin and norepinephrine transporters revealed by molecular dynamics simulation. ACS Chem. Neurosci. 9 (5), 1128–1140. doi: 10.1021/acschemneuro.7b00490
Yuan, S., Hu, Z., Filipek, S., Vogel, H. (2015). W2466. 48 opens a gate for a continuous intrinsic water pathway during activation of the adenosine A2A receptor. Angew. Chem. Int. Edit. 54 (2), 556–559. doi: 10.1002/anie.201409679
Yuan, S., Vogel, H., Filipek, S. (2013). The role of water and sodium ions in the activation of the µ-Opioid receptor. Angew. Chem. Int. Edit. 52 (38), 10112–10115. doi: 10.1002/anie.201302244
Zhang, F., Yuan, Y., Xiang, M., Guo, Y.-Z., Li, M.-L., Liu, Y., et al. (2019). Molecular mechanism regarding allosteric modulation of ligand binding and the impact of mutations on dimerization for CCR5 homodimer. J. Chem. Inf. Model. 59 (5), 1965–1976. doi: 10.1021/acs.jcim.8b00850
Zhang, L., Yuan, Y., Ren, T., Guo, Y., Li, C., Pu, X. (2018a). Shining light on molecular mechanism for odor-selectivity of CNT-immobilized olfactory receptor. Sci. Rep. 8 (1), 7824. doi: 10.1038/s41598-018-26105-0
Zhang, X., Yuan, Y., Wang, L., Guo, Y., Li, M., Li, C., et al. (2018b). Use multiscale simulation to explore the effects of the homodimerizations between different conformation states on the activation and allosteric pathway for the µ-opioid receptor. Phys. Chem. Chem. Phys. 20 (19), 13485–13496. doi: 10.1039/C8CP02016G
Keywords: target, GPCR dimer interface, druggability, regulation mechanism, computation
Citation: Shen L, Yuan Y, Guo Y, Li M, Li C and Pu X (2019) Probing the Druggablility on the Interface of the Protein–Protein Interaction and Its Allosteric Regulation Mechanism on the Drug Screening for the CXCR4 Homodimer. Front. Pharmacol. 10:1310. doi: 10.3389/fphar.2019.01310
Received: 23 August 2019; Accepted: 15 October 2019;
Published: 07 November 2019.
Edited by:
Feng Zhu, Zhejiang University, ChinaReviewed by:
Hai-Bin Luo, Sun Yat-sen University, ChinaTingjun Hou, Zhejiang University, China
Jin Yu, University of California, Irvine, United States
Copyright © 2019 Shen, Yuan, Guo, Li, Li and Pu. This is an open-access article distributed under the terms of the Creative Commons Attribution License (CC BY). The use, distribution or reproduction in other forums is permitted, provided the original author(s) and the copyright owner(s) are credited and that the original publication in this journal is cited, in accordance with accepted academic practice. No use, distribution or reproduction is permitted which does not comply with these terms.
*Correspondence: Xuemei Pu, eG1wdXNjdUBzY3UuZWR1LmNu