- 1Sydney Pharmacy School, The University of Sydney, Sydney, NSW, Australia
- 2School of Pharmacy and Medical Sciences, University of South Australia, Adelaide, SA, Australia
- 3University of South Australia Cancer Research Institute, University of South Australia, Adelaide, SA, Australia
Long-term use of imatinib is effective and well-tolerated in children with chronic myeloid leukaemia (CML) yet defining an optimal dosing regimen for imatinib in younger patients is a challenge. The potential interactions between imatinib and coadministered drugs in this “special” population also remains largely unexplored. This study implements a physiologically based pharmacokinetic (PBPK) modeling approach to investigate optimal dosing regimens and potential drug interactions with imatinib in the paediatric population. A PBPK model for imatinib was developed in the Simcyp Simulator (version 17) utilizing in silico, in vitro drug metabolism, and in vivo pharmacokinetic data and verified using an independent set of published clinical pharmacokinetic data. The model was then extrapolated to children and adolescents (aged 2–18 years) by incorporating developmental changes in organ size and maturation of drug-metabolising enzymes and plasma protein responsible for imatinib disposition. The PBPK model described imatinib pharmacokinetics in adult and paediatric populations and predicted drug interaction with carbamazepine, a cytochrome P450 (CYP)3A4 and 2C8 inducer, with a good accuracy (evaluated by visual inspections of the simulation results and predicted pharmacokinetic parameters that were within 1.25-fold of the clinically observed values). The PBPK simulation suggests that the optimal dosing regimen range for imatinib is 230–340 mg/m2/d in paediatrics, which is supported by the recommended initial dose for treatment of childhood CML. The simulations also highlighted that children and adults being treated with imatinib have similar vulnerability to CYP modulations. A PBPK model for imatinib was successfully developed with an excellent performance in predicting imatinib pharmacokinetics across age groups. This PBPK model is beneficial to guide optimal dosing regimens for imatinib and predict drug interactions with CYP modulators in the paediatric population.
Introduction
Imatinib has revolutionised the treatment for cancer and led to a subsequent discovery of a class of drugs known as small molecule kinase inhibitors (Rowland et al., 2017). It is approved as the first-line treatment for chronic myeloid leukaemia (CML) and gastrointestinal stromal tumours (GIST) in adult patients and for CML and Philadelphia chromosome-positive (Ph+) acute lymphoblastic leukaemia (ALL) in children and adolescents (Suttorp et al., 2018a). A phase III clinical trial highlighted that imatinib was well tolerated and effective for newly diagnosed paediatric CML, with a 5-year progression free survival of 94% (Suttorp et al., 2018b). A 5-year follow-up of imatinib (340 mg/m2/d) in combination with conventional chemotherapy drugs (e.g., cyclophosphamide, methotrexate, and cytarabine) showed a favorable outcome in children with Ph+ ALL, similar to that of bone marrow transplantation (Schultz et al., 2014).
The prevalence of childhood CML and Ph+ ALL, however, is very low, accounting for around 2% of all leukaemias and 3%–5% of ALL in children, respectively (Coebergh et al., 2006). Therefore, an optimal dose for imatinib in paediatric patients, let alone its potential drug-drug interactions, has been less widely explored. Imatinib is mainly metabolised by cytochrome P450 (CYP)3A4 and CYP2C8 (Barratt and Somogyi, 2017), and thus, has a potential for drug interactions with modulators of these CYP enzymes. A clinically significant interaction between imatinib and carbamazepine, a CYP3A and CYP2C8 inducer, was described in a 12-year old CML patient with epilepsy (Taguchi et al., 2014). However, little is known about imatinib interactions with other potential perpetrator drugs in paediatric patients. Conducting a dedicated clinical interaction study in paediatric population remains challenging owing to the ethical and logistical constraints (Barker et al., 2018). Clearly, a feasible and systematic approach to address this gap is warranted.
Physiologically based pharmacokinetic (PBPK) modeling can account for anatomical and physiological growth and organ maturation underlying age-related changes in the pharmacokinetics of a drug of interest (Yellepeddi et al., 2019). This facilitates an extrapolation across the age spectrum (Kuepfer et al., 2016). The PBPK approach has been increasingly embraced by regulatory authorities for the purposes of informing dose selection, providing simulation-based trial design and investigating potential drug interactions in paediatric populations (Cole et al., 2017; Bi et al., 2019). According to applications related to PBPK that were submitted to the US Food and Drug Administration (FDA) from 2008 to 2017, PBPK analyses are mainly intended for evaluating and predicting enzyme-based drug interactions (60% of all applications), followed by utilization in paediatric area (15%) (Grimstein et al., 2019). PBPK modeling and simulation has been an integral part of drug development for paediatric cancers (Rioux and Waters, 2016). PBPK models which can capture developmental changes in biological components are useful in describing in paediatrics the pharmacokinetics of anticancer drugs, including etoposide (Kersting et al., 2012), busulfan (Diestelhorst et al., 2014), docetaxel (Thai et al., 2015), actinomycin D (Walsh et al., 2016) and nilotinib (Heimbach et al., 2019).
A PBPK model for imatinib that incorporates maturational changes of key drug-metabolising enzymes and age-dependent organ development can help inform optimal dose selection in children. PBPK modeling and simulation also provides a greater understanding of potential drug interactions with imatinib in this vulnerable patient group which remains largely unexplored. The aim of this study was to develop and implement a paediatric PBPK model of imatinib for investigating optimal dosing regimens in children and the vulnerability to drug interactions relative to adults with a range of CYP3A modulators.
Methods
In this study, a PBPK model for imatinib was developed and verified in adults and subsequently extrapolated to children and adolescents (aged 2–18 years). The verified PBPK model was then implemented to explore optimal dosing regimens for imatinib in children and to evaluate potential drug interactions with CYP3A modulators. The workflow of this study is summarized in Figure 1.
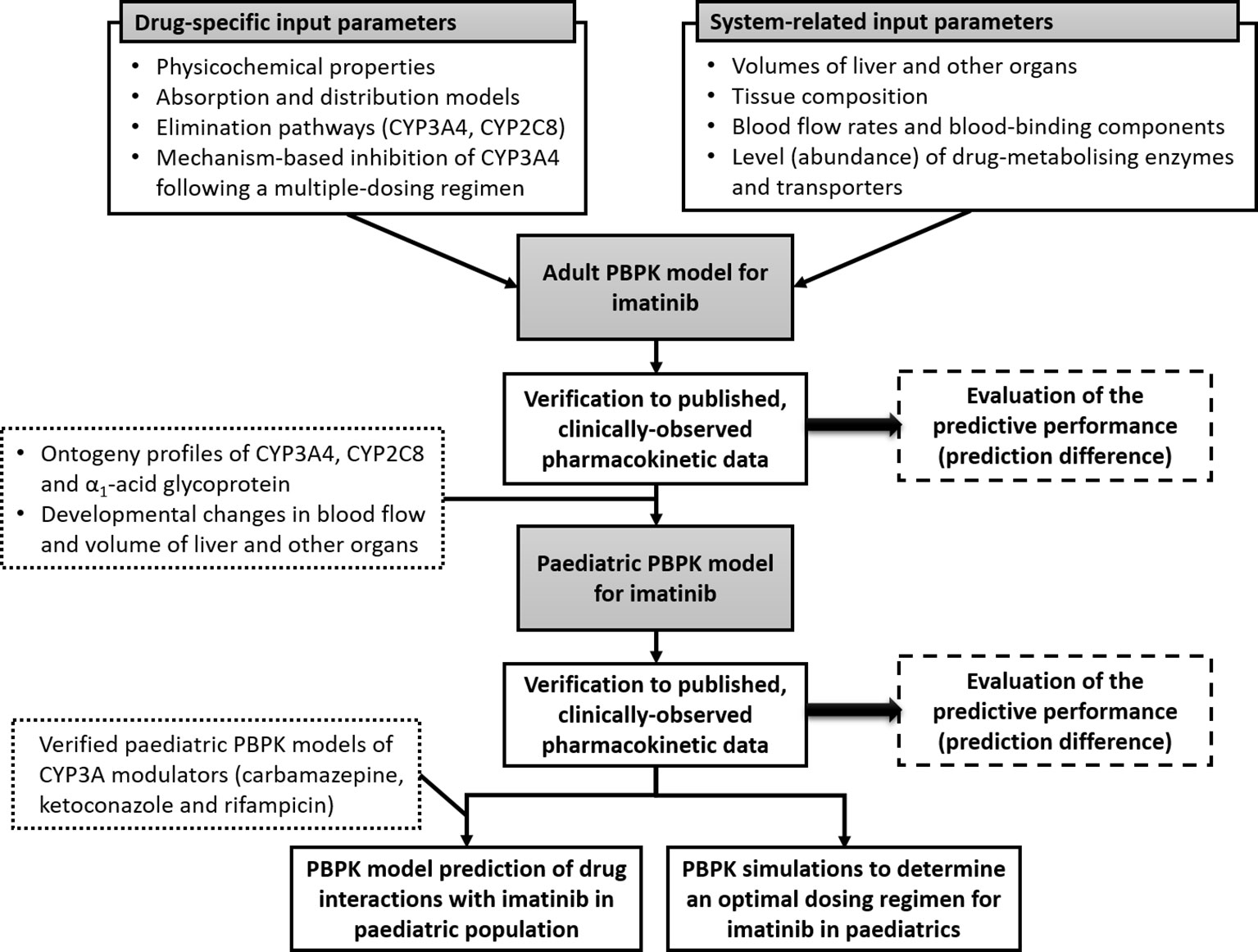
Figure 1 Schematic representation of workflow of this study. Physiologically based pharmacokinetic (PBPK) model of imatinib in adults was constructed using drug-dependent and system-related input parameters and verified using published clinical pharmacokinetic data. The verified model was subsequently extrapolated to children and adolescents by incorporating age-related changes in organ size and maturation of cytochrome P450 (CYP)3A4 and CYP2C8 and α1-acid glycoprotein and then verified to clinically observed concentrations in paediatric population. Paediatric PBPK model of imatinib was implemented to determine an optimal dosing regimen for imatinib and evaluate potential drug interactions with a range of CYP3A modulators in children older than 2 years.
Development and Verification of a PBPK Model for Imatinib in Adults
All population-based PBPK modeling and simulations were conducted using the Simcyp Simulator (version 17 release 1, Certara UK Limited, Simcyp Division, Sheffield, UK) using the “general North European Caucasian” population library data, which represents typical healthy adult people from European ancestry. The description of Simcyp Simulator workflow, basic algorithm, and ordinary differential equations have been detailed previously (Rowland-Yeo et al., 2010; Jamei et al., 2013). The drug-related input parameters for imatinib are listed in Table 1.
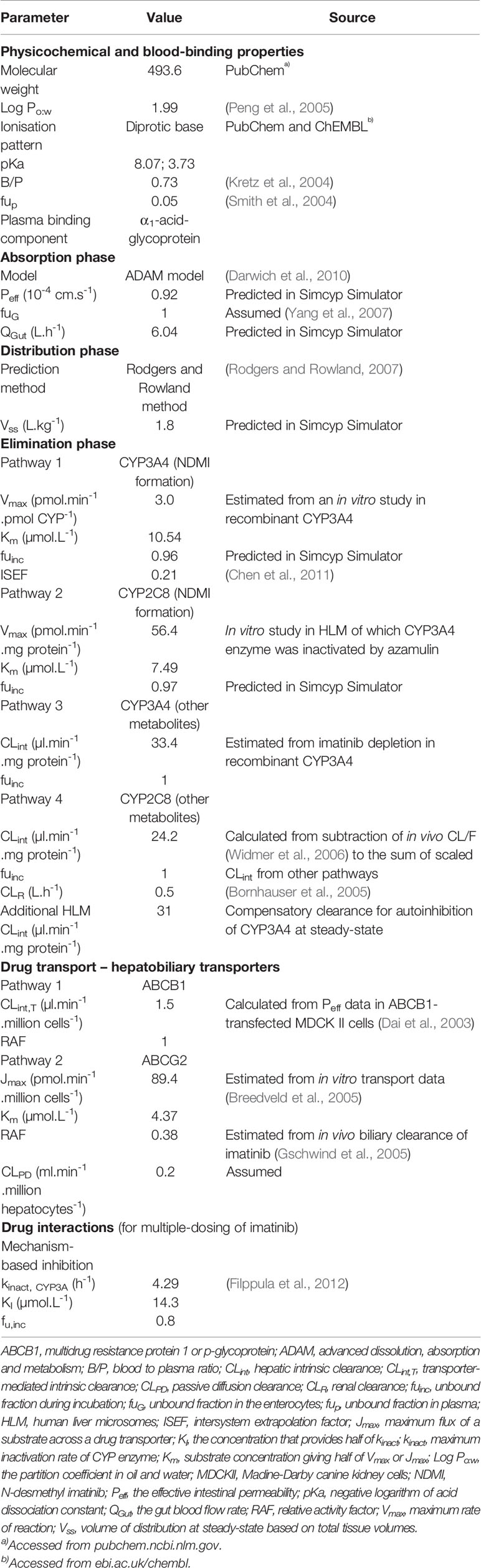
Table 1 Drug-related parameters used to build a physiologically based pharmacokinetic (PBPK) model for imatinib in Simcyp Simulator.
As a basic compound, imatinib binds extensively to α1-acid glycoprotein (AAG) (Kretz et al., 2004) with an unbound fraction (fup) of 0.05 (Smith et al., 2004). A higher level of AAG has been reported in patients with solid tumours (Thai et al., 2015). However, plasma AAG concentration is similar in healthy people when compared to patients with CML and GIST (mean value of 0.81 vs. 0.79–1.08 and 0.89 g/L, respectively) (Gambacorti-Passerini et al., 2003; Gandia et al., 2013; Haouala et al., 2013; Bins et al., 2017). This corresponded to an unbound fraction in plasma (fup) for imatinib which was not dissimilar, yet highly variable, between healthy people [0.05 (range 0.02–0.10)] and patients with CML [0.03 (range 0.01–0.10)] (Smith et al., 2004; Gandia et al., 2013). Interestingly, AAG concentrations in patients with GIST were relatively stable over a 1-year course of treatment with imatinib (Bins et al., 2017). Thus, a fixed fup of 0.05 with associated variability was assigned to adult population. There is a paucity of data on AAG concentration in paediatrics with CML. Nevertheless, clinical data in children with Ph+ ALL (n = 4, aged 6–15 years) hinted at a similar AAG concentration (mean ± standard deviation of 0.88 ± 0.39 g/L) (Marangon et al., 2009) with that of healthy adults and adult patients with CML.
The Advanced Dissolution, Absorption and Metabolism (ADAM) model (Darwich et al., 2010) was used to describe imatinib absorption. The effective intestinal permeability (Peff) of imatinib was estimated using the apparent permeability data in Caco-2 cell lines (7.9 x 10-6 cm/s). Peff was then utilized to predict the gut blood flow rate (QGut) (Yang et al., 2007). A whole-body PBPK model was used to describe the distribution of imatinib, with tissue-to-plasma partition coefficient (kp) values to each of the organs predicted in silico (Rodgers and Rowland, 2007).
The intrinsic clearances (CLint) of imatinib to N-desmethyl imatinib (NDMI) and other metabolites were estimated from in vitro kinetic data using recombinant CYP3A4 (rCYP3A4) and human liver microsomes (HLM, in the presence of azamulin) as detailed in Table 1 (unpublished). The latter represented the contribution of CYP2C8, since CYP enzymes other than CYP3A4 and CYP2C8 had a very minor contribution (3%) to imatinib metabolism (Filppula et al., 2013a). Biliary clearance (CLbile) of imatinib mediated by ABCB1 and ABCG2 transporters was parameterised by CLint,T or Jmax and Km the values of which were extracted from previous in vitro studies (Dai et al., 2003; Breedveld et al., 2005). Relative activity factor (RAF) of ABCG2 transporter was adjusted to 0.38 to give a CLbile of 28% of overall clearance of imatinib (Gschwind et al., 2005). The renal clearance value for imatinib (CLR = 0.5 L/h) was taken from a study in patients with CML and Ph+ ALL (Bornhauser et al., 2005). The CYP3A4-mediated formation clearance of metabolites other than NDMI (CLuint,others,3A4) was estimated from subtraction of depletion clearance of imatinib in rCYP3A4 enzyme (CLudep,3A4) to formation clearance of NDMI in rCYP3A4 (CLuint,NDMI,3A4) as detailed in Table 1. Intersystem extrapolation factor (ISEF) of 0.21 (Chen et al., 2011) was used to correct for differences in intrinsic activity per unit enzyme between rCYP3A4 and HLM. Clearance of imatinib to other metabolites through a CYP2C8-mediated pathway was estimated according to Eq. 1.
where CLuint,total was back-calculated from in vivo apparent clearance (CL/F = 14.4 L/h) (Widmer et al., 2006) after subtraction of CLR using the well-stirred hepatic model (a retrograde approach) (Rowland-Yeo et al., 2010).
The mechanism-based inhibition (MBI) of CYP3A4 following a chronic use of imatinib was modeled by an enzyme turnover model as follows:
where Enz0 and Enz(t) indicate the amount of CYP3A4 (Enz) at baseline as reported previously (Cubitt et al., 2011) and at time t, respectively; kdeg represents the first-order degradation (turnover) rate constant of the enzyme in hepatocytes and enterocytes (Yang et al., 2008); kinact denotes the maximum rate of inactivation, while KIu is imatinib concentration needed to reach half of kinact, both of which were obtained from a previous report (Filppula et al., 2012); [I] and fu indicate imatinib concentrations in the liver or gut at time t and the unbound fraction of imatinib at the corresponding site of enzyme, respectively. Not accounting for CYP3A4 autoinhibition by imatinib at steady-state led to an overestimation of the extent of interaction with ritonavir, a CYP3A inhibitor, as summarized in Table S1. PBPK model predictions which incorporated a CYP3A4 MBI (Eq. 2) were consistent with the clinically observed interaction, however, CL/F of imatinib was underestimated (Table S1). A nonpathway specific additional clearance was assigned to the PBPK model at steady-state (Table 1) to correct this underprediction. This was also supported by a lack of significant changes in imatinib CL/F at steady-state compared to that on day 1 (Petain et al., 2008; Gotta et al., 2013).
The importance of uptake transporter(s) has been hypothesized since imatinib is almost completely bioavailable, despite being a substrate of both ABCB1 and ABCG2 transporters (Barratt and Somogyi, 2017). The activity of this uptake transporter seems to be diminished by coadministration of gemfibrozil (Filppula et al., 2013b) and in patients who had undergone major gastrectomy (Lubberman et al., 2017). However, available clinical evidence has been conflicting as to which transporter is primarily responsible for the uptake of imatinib (Neul et al., 2016; Barratt and Somogyi, 2017). Coadministration of rifampicin, an inducer and inhibitor of CYP enzymes and SLCO1B transporters, respectively (Kalliokoski and Niemi, 2009; Asaumi et al., 2018) at 600 mg/d for 7 days decreased systemic exposure (AUC0-∞) of imatinib given as a single 400 mg oral dose by 74% in healthy adults (Bolton et al., 2004). This suggests that either the uptake process into the liver is not the rate-limiting step for hepatic metabolism of imatinib or sinusoidal uptake transporter(s) other than SLCO1B may play a role. However, the latter is unlikely given that clinical evidence of transporter-mediated drug interactions with imatinib as a victim drug is lacking. Therefore, transporter-mediated uptake processes in gut and liver were not included in the PBPK model.
PBPK simulations of imatinib in adults were performed with trial designs (number of people, age range, proportion of male/female, and dosing regimens) matched to the corresponding clinical studies (Table 2). A total of 10 virtual trials for each simulation were carried out. Clinically observed concentrations of imatinib were retrieved from the original publications using WebPlotDigitizer version 4.1 (www.automeris.io/WebPlotDigitizer) and superimposed to simulated profiles to allow visual inspection of the predictive performance. Prediction differences of imatinib pharmacokinetic parameters, expressed as the ratio of PBPK model prediction to clinically reported parameter values were also evaluated.
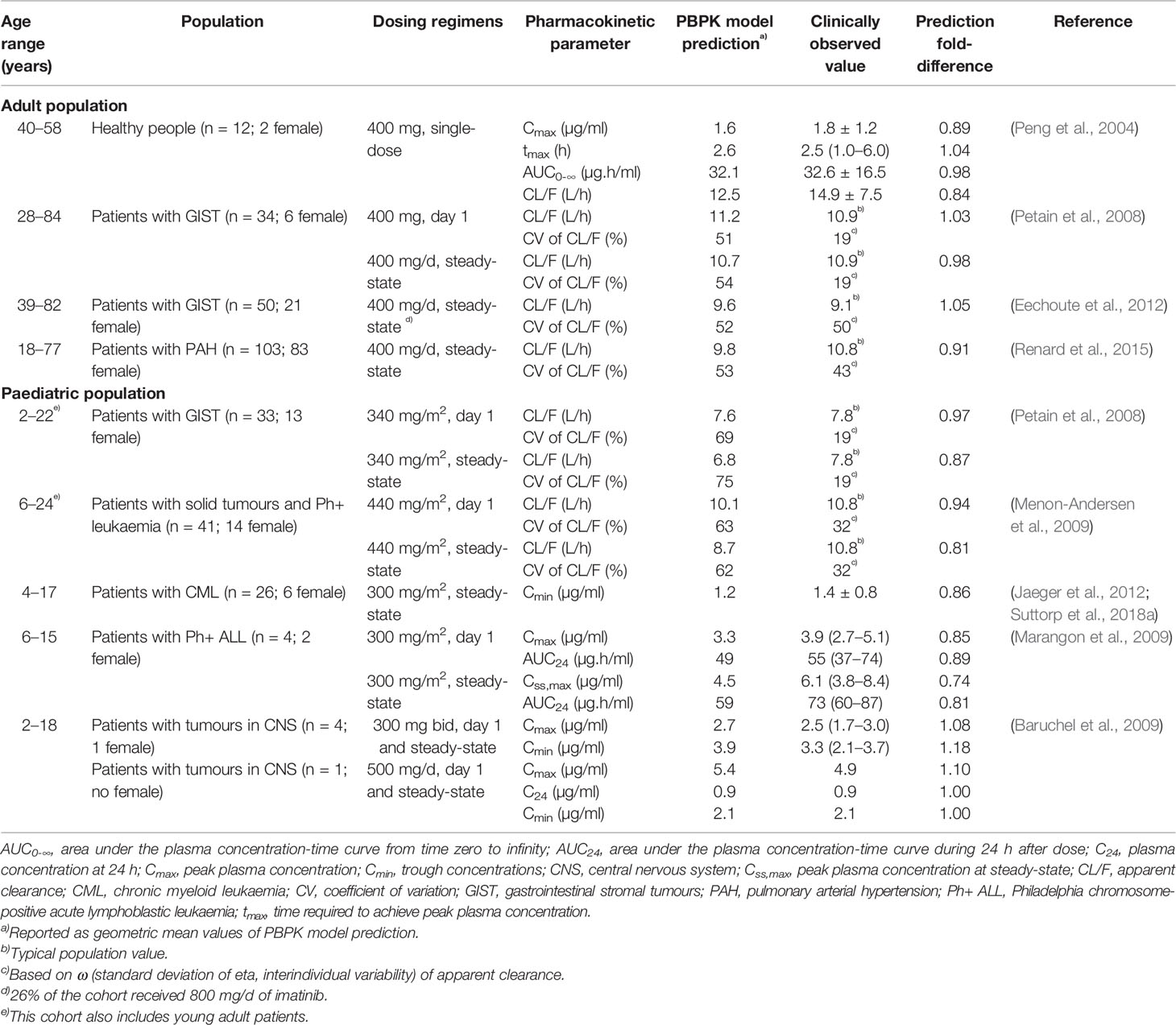
Table 2 Summary of clinical cohorts used for physiologically based pharmacokinetic (PBPK) model verification and comparison of simulated and clinically reported values for pharmacokinetic parameters of imatinib.
Extrapolation of the PBPK Model of Imatinib to Paediatric Population
The verified PBPK model of imatinib in the adult population was extrapolated to children and adolescents (2–18 years) according to the best practice in development of paediatric PBPK model (Maharaj et al., 2013; Maharaj and Edginton, 2014). Drug-specific parameters for imatinib were fixed at the same values as those defined in the adult PBPK model (Table 1). The algorithms for ontogeny profiles of CYP enzymes (Figure 2A) are incorporated into Simcyp Simulator by default (Johnson et al., 2006). A sigmoidal Emax model (Eq. 3), driven by postnatal age, adequately describes the maturation of CYP3A4 and CYP2C8. Parameters specific to each enzyme are summarized in Table 3.
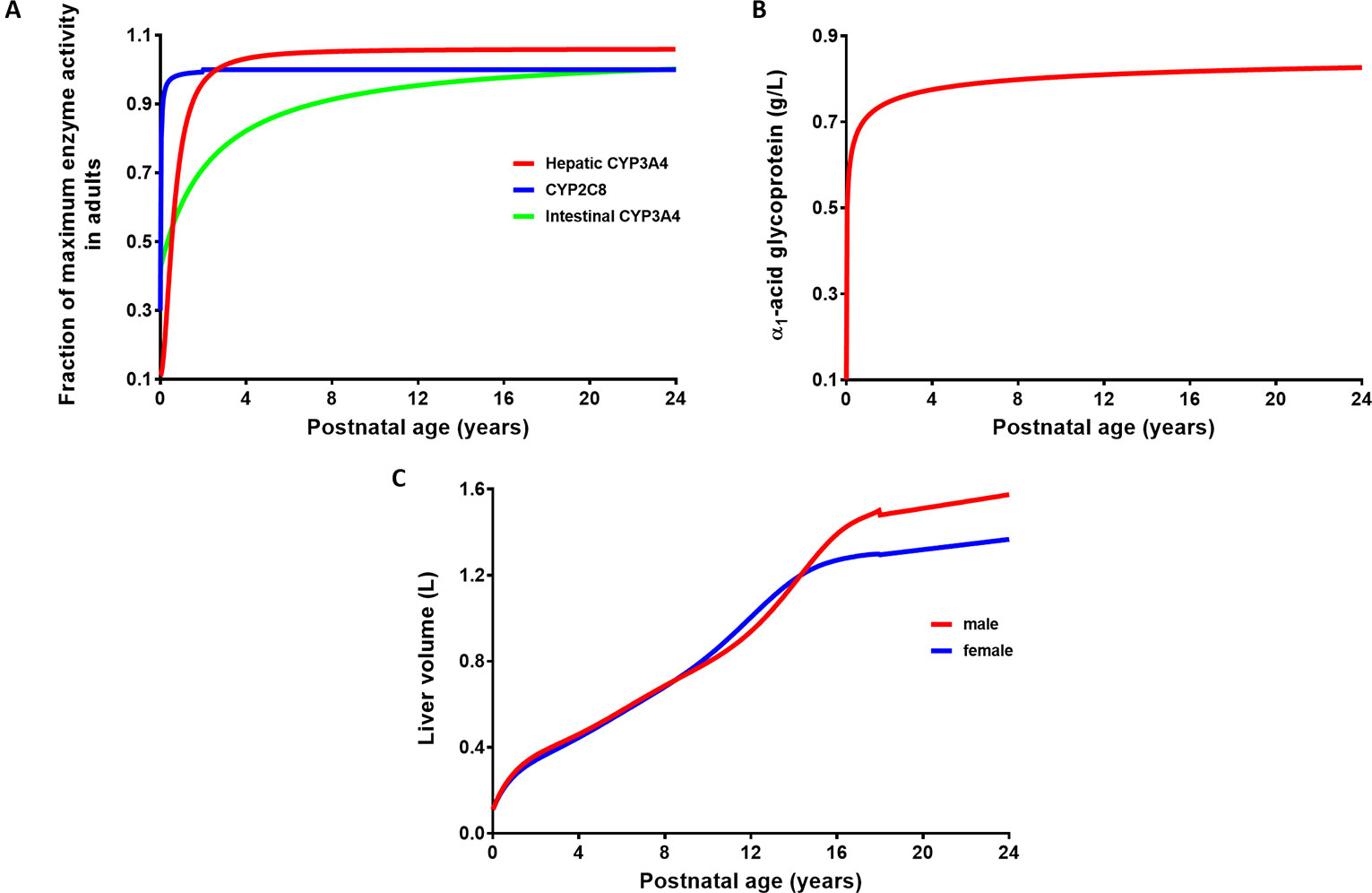
Figure 2 Ontogeny profiles of drug-metabolising enzymes responsible for imatinib metabolism (A) and age-related changes in plasma concentration of α1-acid glycoprotein (B) and liver volume (C).
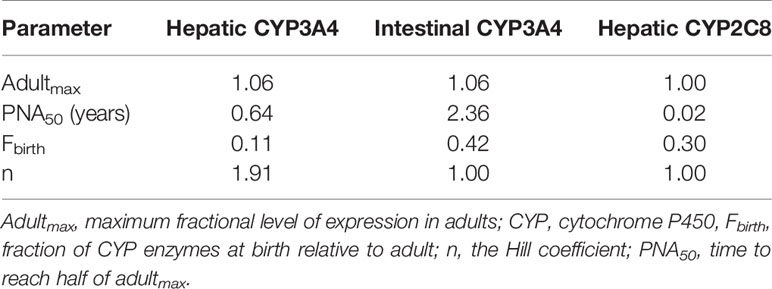
Table 3 Parameters used in sigmoidal Emax functions to describe the maturation of drug-metabolising enzymes involved in imatinib metabolism.
where adultmax represents the maximum level of expression (as a fraction) of CYP enzymes in adult population; Fbirth is the fraction of CYP enzymes at birth relative to adult; n denotes an exponent which is analogous to the Hill coefficient; PNA and PNA50 are postnatal age and the maturation half-life in years, respectively.
The ontogeny function derived for α1-acid glycoprotein (AAG) as shown in Eq. 4 and Figure 2B was based on a limited set of data compiled from previously published reports (Johnson et al., 2006) and as an update of McNamara and Alcorn’s linear equation (McNamara and Alcorn, 2002). Interestingly, this sigmoidal Emax model is very similar to the one generated recently from a larger meta-analysis in healthy people (Maharaj et al., 2018). Unbound fraction of imatinib in paediatrics (fuped) was then estimated based on the ratio of plasma concentrations of AAG to that in the adult population (Eq. 5). Developmental changes in organ blood flow (as percent cardiac output to different organs) and organ size have been detailed previously (Johnson et al., 2006). The changes in liver size with body surface area (BSA) are specified in Eq. 6 (Johnson et al., 2005), where BSA (m2) was estimated from body weight and height of each individual (DuBois and DuBois, 1916). The associated changes in liver size based on age and sex are depicted in Figure 2C.
where PNA denotes postnatal age in years; AAGadult and AAGped are plasma concentrations of AAG in adult and paediatric population, respectively; and fuadult is the unbound fraction of imatinib in adults (mean value of 0.05).
Given the importance of ABCB1 and ABCG2 transporters on biliary excretion of imatinib (Barratt and Somogyi, 2017), the maturation rates of these drug transporters need to be considered. The expression of hepatic and intestinal ABCG2 transporter was not affected by age (Prasad et al., 2016; Cheung et al., 2019), while there are conflicting data on developmental changes in protein expression of hepatobiliary ABCB1 transporter (Mooij et al., 2014; Prasad et al., 2016). However, the clinical pharmacokinetic data and PBPK simulations of digoxin, a probe drug for ABCB1, suggest a rapid maturation and attainment of adult levels of expression within first few months after birth (Johnson et al., 2016). Therefore, no age-related change was assumed for ABCB1 transporter and the adult values, which is the default setting in Simcyp Simulator, were applied.
The PBPK model in paediatrics was verified using published, clinical pharmacokinetic data following single- and multiple-dosing regimens of imatinib. Simulations were performed (10 virtual trials for each simulation) with a trial design similar to the corresponding clinical studies as presented in Table 2. It is worth mentioning that the age range of participants in a number of clinical studies overlaps with that of young adults (Petain et al., 2008; Menon-Andersen et al., 2009). However, this was acceptable since all ontogeny functions employed in the model followed a clear trajectory until adult age (Figure 2).
PBPK Simulation to Evaluate Optimal Dosing Regimens for Imatinib in Paediatrics
The paediatric population was categorised into several age groups: preschool (2–5 years) and school-age children (6–11 years), and adolescents (12–17 years) (Batchelor and Marriott, 2013). PBPK simulations of imatinib were performed using hypothetical multiple-dosing regimens given for 14 days (steady-state was assumed to be achieved within this time frame) with n = 100 (40% of female) for each age group. The male-to-female ratio was based on the value observed in paediatric patients, in which boys had an approximately 1.3-fold higher risk to be diagnosed with CML (Coebergh et al., 2006). BSA-normalized doses of imatinib of 170, 230, 340, and 460 mg in paediatrics corresponded to fixed doses of 300, 400, 600, and 800 mg in adults, respectively. The total daily doses of imatinib (in mg) for each age band were rounded to the closest 50 mg, a half-size of the smallest commercially available imatinib tablet as recommended in the clinical setting (Suttorp et al., 2018a) and were capped at the equivalent adult doses. Potential differences of imatinib Cmin across age bands were evaluated by a one-way analysis of variance (ANOVA) with Tukey post hoc test using GraphPad Prism version 7.02 (GraphPad Software, La Jolla, CA, USA).
PBPK Model Prediction of Drug Interactions With a Range of CYP3A Modulators
Verification of Paediatric PBPK Models for Carbamazepine, Ketoconazole, and Rifampicin
The default PBPK models for carbamazepine, ketoconazole, and rifampicin in Simcyp Simulator were used (Almond et al., 2016; Liu et al., 2017). The predictive performance of the PBPK models in paediatric population need to be verified prior to their further use, since the original models were developed in adults. PBPK simulations for the three CYP3A modulators were carried out across different dosing regimens and age groups as detailed in Table 4, with a total of 10 virtual trials for each of the simulations. The predicted fold-differences of pharmacokinetic parameters for each compound, expressed as PBPK model prediction over the values reported in clinical studies were determined.
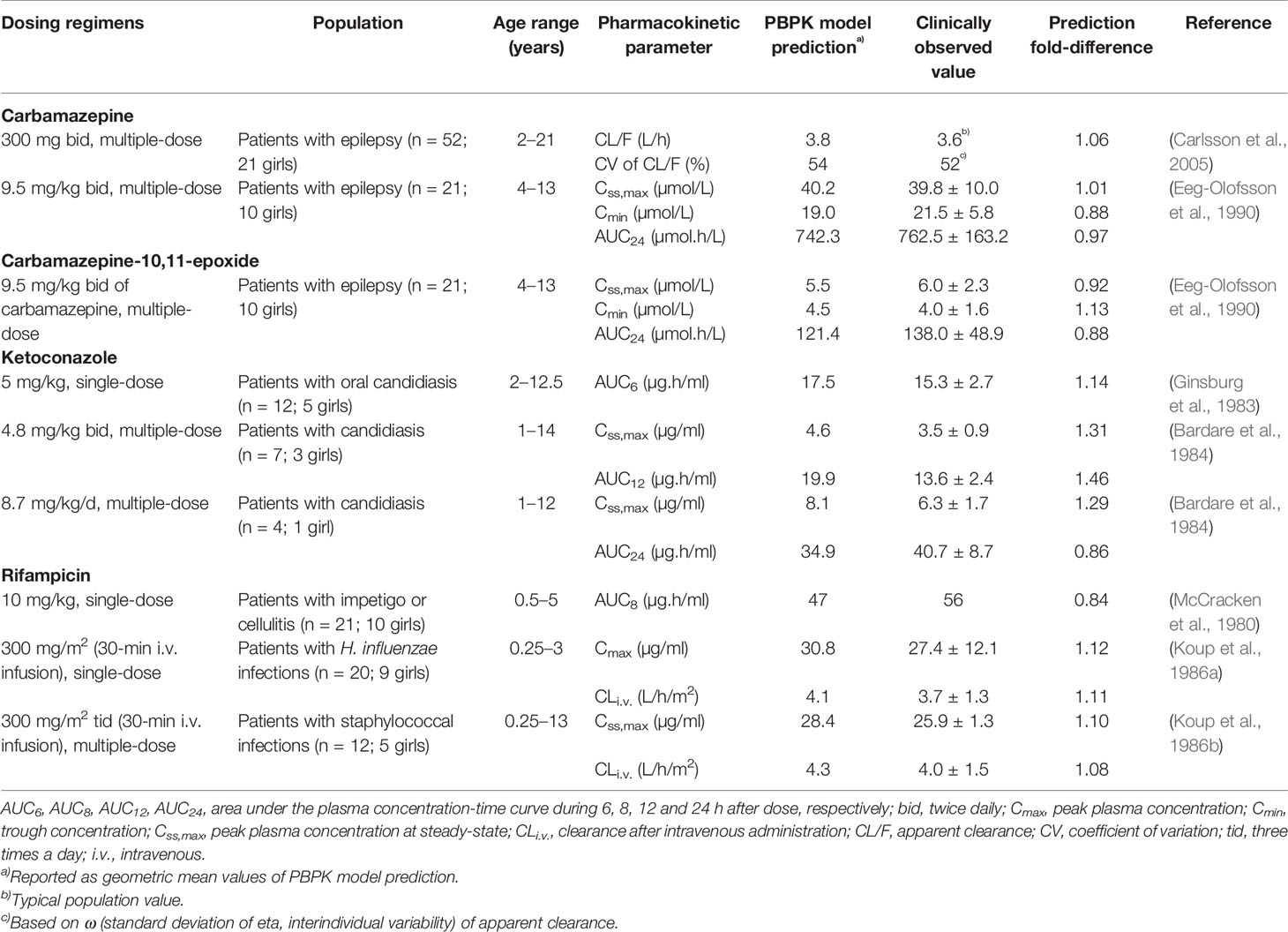
Table 4 Comparison of physiologically based pharmacokinetic (PBPK) model prediction and clinically observed values for pharmacokinetic parameters of carbamazepine, ketoconazole, and rifampicin in paediatric population.
Evaluation of PBPK Model Prediction of Interaction With Carbamazepine
PBPK simulations were performed to predict the extent of interaction between carbamazepine and imatinib in adults (n = 63, age ranging from 19 to 69 years) (Pursche et al., 2008) and paediatrics (a 12-year old male) (Taguchi et al., 2014). Designs of the clinical studies were replicated in the PBPK simulations, except for the latter which was carried out in a total of 100 subjects with age range of 11–13 years. This was necessary since the Simcyp Simulator does not allow the assignment of a single age value in a trial design. The goodness-of-fit of the PBPK predictions was evaluated via a visual inspection of the simulated pharmacokinetic profiles which were overlaid to imatinib concentrations observed in the clinical studies.
Implementation of PBPK Modeling Approach to Evaluate Drug Interactions With CYP3A Modulators Across Different Age Bands
To investigate the age-related changes in liability to CYP modulation, PBPK prediction of imatinib interactions with a set of CYP3A modulators, exemplified by carbamazepine, ketoconazole and rifampicin were conducted in adult and paediatric populations. The verified PBPK models in paediatrics were used, with the addition of CYP2C8 induction to the rifampicin model (maximum fold of induction (Indmax) = 6.27 and concentration that provides half of Indmax (IndC50) = 0.1 µmol/L) (Raucy et al., 2002). CYP2C8 induction was also incorporated to carbamazepine and its active metabolite, carbamazepine-10,11-epoxide with IndC50 and Indmax for both compounds of 22 µmol/L and 3.5, respectively (Zhang et al., 2015). The induction of CYP3A and CYP2C8 was modeled by an increase in protein synthesis (turnover) rate constant in hepatocytes and enterocytes according to an enzyme turnover model (Almond et al., 2016). Ketoconazole inhibits CYP3A4 and CYP2C8 competitively with an inhibitory constant (Kiu) of 15 nmol/L (Liu et al., 2017) and 2.2 µmol/L, respectively. PBPK simulations were carried out with n = 50 (40% of female) for each age band. Imatinib was given for 14 days with and without carbamazepine, ketoconazole or rifampicin. Imatinib was administered at a daily dose of 230 mg/m2 and 400 mg for paediatrics and adults, respectively. The typical maintenance dosing regimens were assigned for each CYP3A modulator based on age ranges. Potential changes in area under the plasma concentration-time curve (AUC) of imatinib for each age group on the last day was predicted.
Results
Development and Verification of a PBPK Model for Imatinib in Adults
The PBPK model was successfully predicted pharmacokinetic of imatinib following single- and multiple-dosing regimens in adults (Figures 3A–E). Clinically observed concentrations of imatinib fell within 5th to 95th percentiles of the PBPK model simulated pharmacokinetic profiles. Interestingly, PBPK simulation of the study by Petain et al. were in close agreement with those predicted using a population pharmacokinetic approach (Petain et al., 2008) as shown in Figures 3B, C. However, the observed interindividual variability of imatinib concentrations on day 1 appears to be underestimated (Figure 3B). All the key pharmacokinetic parameters of imatinib were predicted within a 1.25-fold difference (range: 0.84–1.05).
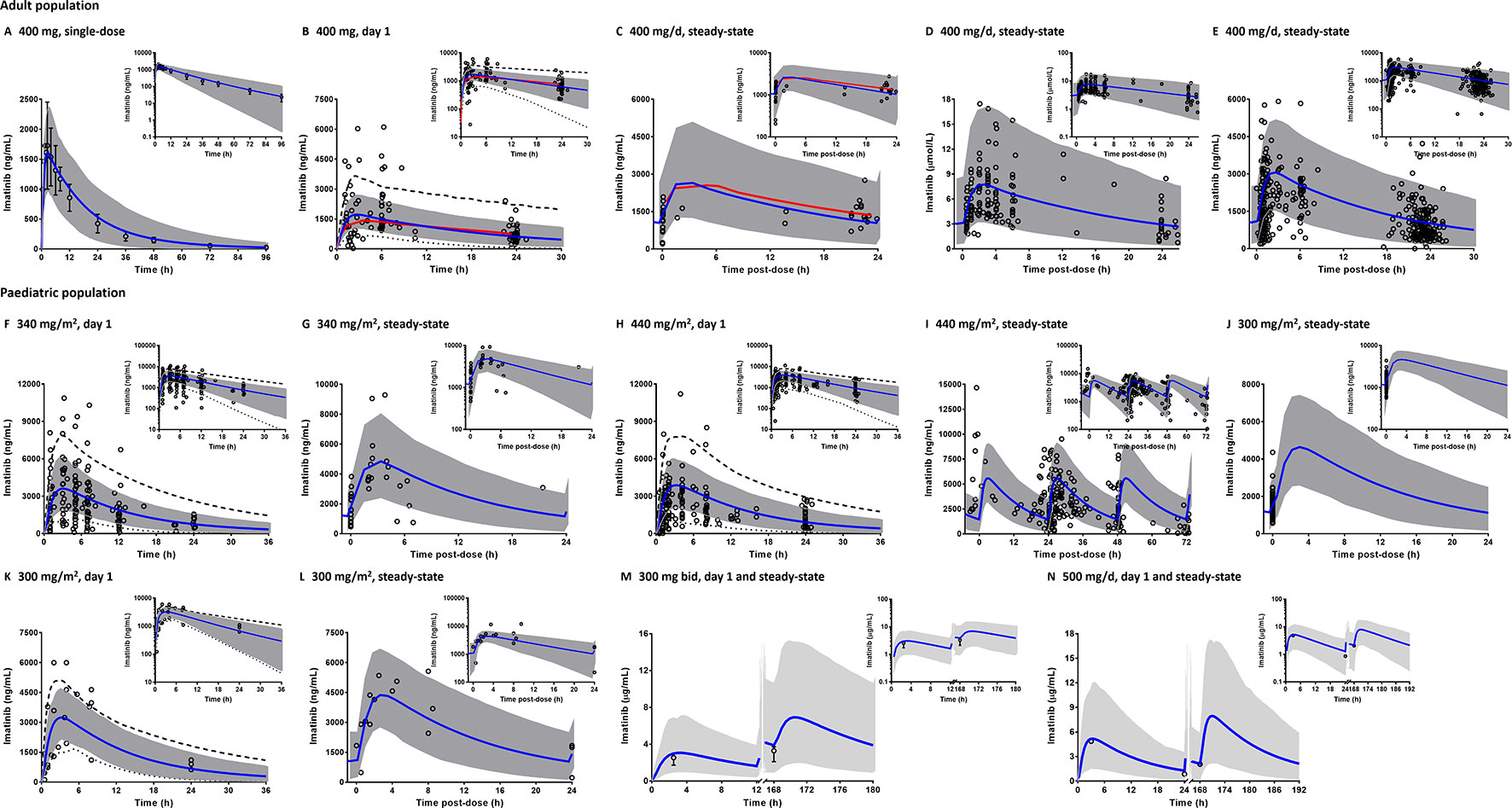
Figure 3 Comparison of physiologically based pharmacokinetic (PBPK) model prediction and clinically observed concentrations of imatinib in adult (A–E) and paediatric populations (F–N). PBPK simulations are presented as mean simulated concentrations (blue line) with their 5th to 95th percentiles (grey area) in linear scale with the corresponding semi-logarithmic plots as insets. Dashed and dotted black lines represent maximum and minimum simulated concentrations, respectively. Clinical pharmacokinetic data (circles) are depicted as either individual data (B–L, N) or mean concentrations with whiskers as corresponding standard deviations (A, M). Population pharmacokinetic predictions of imatinib concentration are shown by red line (B, C). Bid, twice a day.
Extrapolation of the PBPK Model of Imatinib to Paediatric Population
PBPK model predictions in paediatrics (2–18 years) were consistent with clinically observed pharmacokinetic data (Figures 3F–N), although the interindividual variability of imatinib concentrations following single-doses of 300 (Marangon et al., 2009), 340 (Petain et al., 2008) and 440 mg/m2 (Menon-Andersen et al., 2009) appeared to be underpredicted. A number of the clinical pharmacokinetic data came from studies with sparse sampling points, e.g. restricted to imatinib Cmin (Suttorp et al., 2018a) or only 1–2 samples from few children (Baruchel et al., 2009). However, PBPK simulations were able to capture the overall trend observed in the corresponding clinical studies (Figures 3J, M, N). All simulated pharmacokinetic parameters fell within 1.25-fold of those reported in clinical pharmacokinetic studies (Table 2), except for peak concentrations of imatinib at steady-state (Css,max) in the study by Marangon et al (2009).
PBPK Simulation to Evaluate Optimal Dosing Regimens for Imatinib in Paediatrics
The Cmin targets of at least 1,000 ng/ml (Larson et al., 2008; Verheijen et al., 2017) and more strictly, between 1,000 and 3,200 ng/ml (Lankheet et al., 2017) were used for the simulations. PBPK simulations indicated that the variability of the attained Cmin of imatinib was higher in the paediatric population at age 2 to 5 years and middle-aged adults compared to other age groups (Figure 4). The mean Cmin after a daily dose of 340 mg/m2 were predicted to be above the target concentration of 1,000 ng/ml irrespective of the age group. At a lower dose (230 mg/m2), imatinib Cmin values were predicted to be lower than the predefined target concentration in a large subset of children above 5 years of age (Figure 4). Statistical analysis of Cmin of imatinib given at a daily dose of 230 and 340 mg/m2 in paediatrics (corresponded to 400 and 600 mg in adults, respectively) indicated that there was no significant difference among different age bands (p > 0.01).
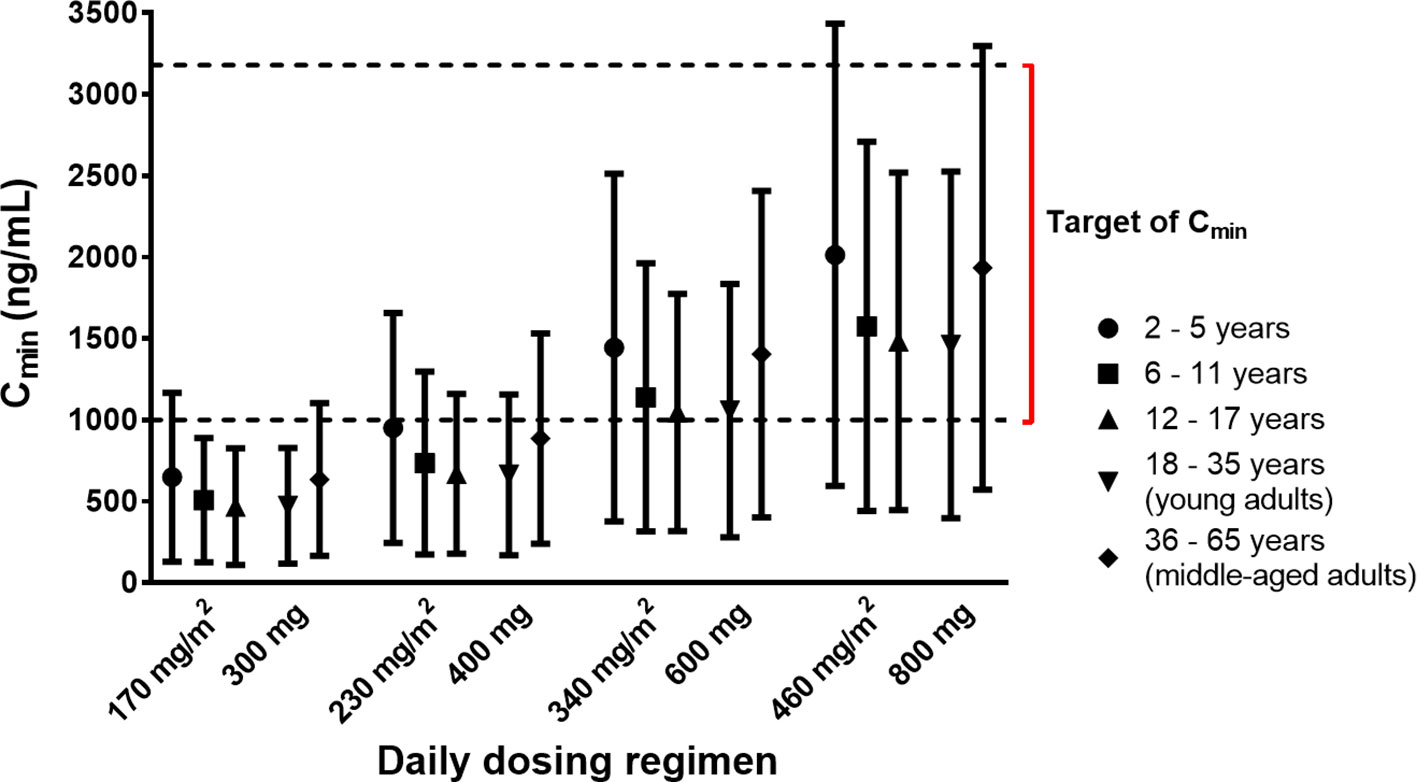
Figure 4 Simulated trough concentrations (Cmin) of imatinib stratified by age bands following various dosing regimens. Simulated data are shown as mean (symbols) with whiskers correspond to standard deviations. The lower and upper limits of target Cmin (1,000–3,200 ng/ml) are indicated by dashed black lines.
PBPK Model Prediction of Drug Interactions With a Range of CYP3A Modulators
Comparisons of the prediction interval (mean concentrations and 5th to 95th percentiles) with the clinically observed pharmacokinetic data for carbamazepine, rifampicin, and ketoconazole at various dosing regimens in paediatrics are presented in Figure 5. Carbamazepine is primarily metabolised by CYP3A and CYP2C8 enzymes and thus, induces its own metabolism (Thorn et al., 2011). Interestingly, accounting for CYP2C8 induction in the PBPK model of carbamazepine and its active metabolite (carbamazepine-10,11-epoxide) in paediatrics improved the predictions (Figures 5A–C; PBPK simulations without CYP2C8 induction are not shown). Prediction differences for pharmacokinetic parameters of carbamazepine and its metabolite in the presence and absence of CYP2C8 autoinduction were within 1.25-fold (range: 0.88–1.13) and 1.5-fold (range: 0.89–1.45), respectively (Table S2). In line with that, the decrease of imatinib Cmin when coadministered with carbamazepine (Figure 6A) was better predicted by the PBPK model that incorporates CYP2C8 induction [Cmin ratio of 0.38 vs. 0.47, compared to the clinically reported value of 0.34 (Pursche et al., 2008)]. Clinical pharmacokinetic data for the corresponding interaction in paediatrics are sparse, limited to imatinib concentrations from a child on day 1 and at steady-state in the presence of multiple-doses of carbamazepine (Taguchi et al., 2014). Despite that, the verified PBPK model of imatinib in paediatric population described the clinical interaction data with a good accuracy, as shown in Figures 6B, C.
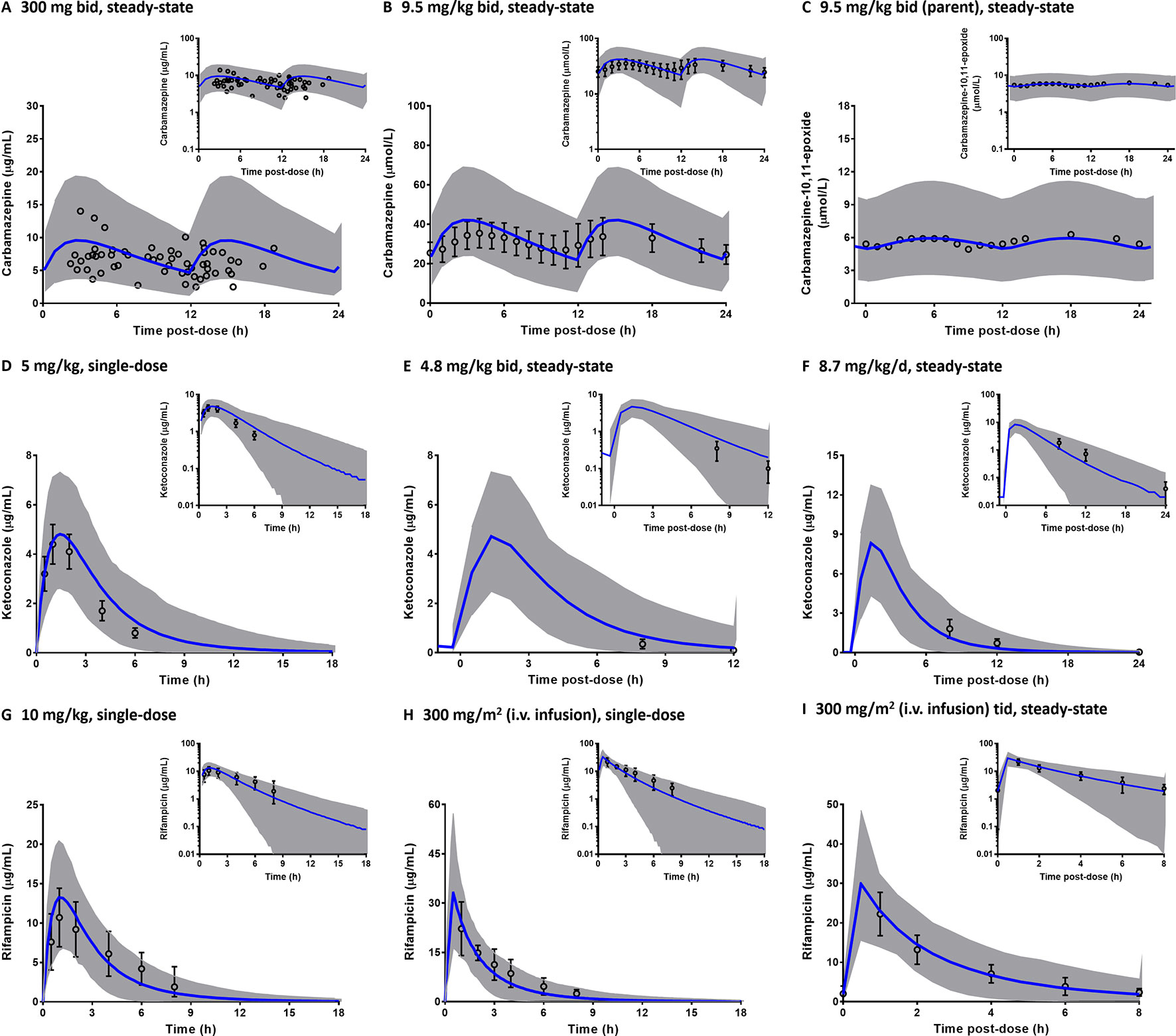
Figure 5 Predicted pharmacokinetic profiles of carbamazepine (A–B) and its metabolite, carbamazepine-10,11-epoxide (C), ketoconazole (D–F); and rifampicin (G–I) in paediatrics. The predictions are depicted in linear scale with the corresponding semi-logarithmic plots as insets (blue line: mean, grey area: 5th to 95th percentiles). Clinically observed concentrations (circles) are presented either as individual data (A), mean (C) or mean with the associated standard deviations (B, D–I). Tid, three times a day.
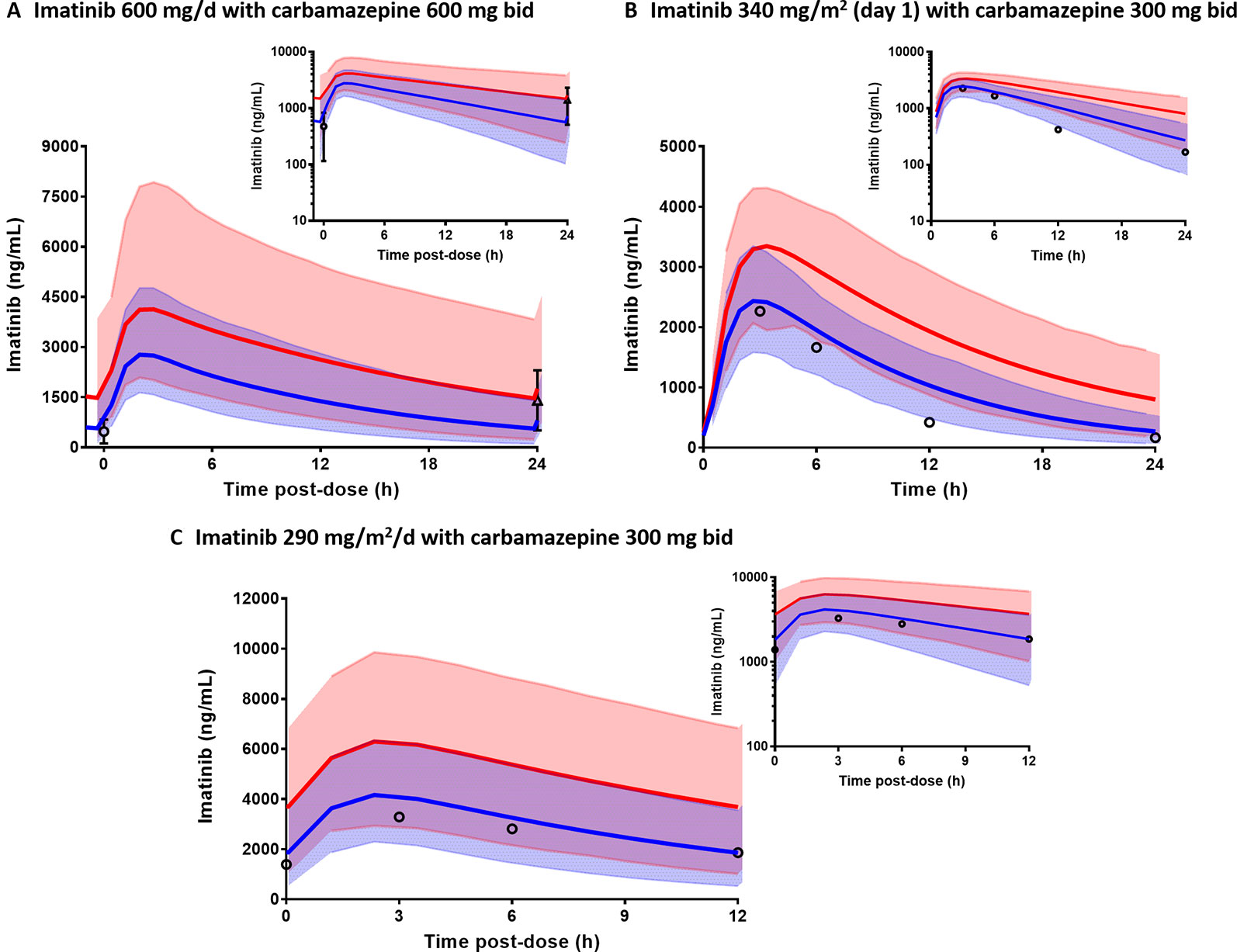
Figure 6 Physiologically based pharmacokinetic (PBPK) model prediction of imatinib concentrations in the presence (blue line) and absence of carbamazepine (red line) in adults (A) and paediatric (B, C). Prediction intervals (5th to 95th percentiles) for imatinib concentrations with and without carbamazepine are represented by light blue and pink area, respectively. Clinically observed data are represented by mean concentrations of imatinib alone (triangle) or with carbamazepine (circles) with whiskers as corresponding standard deviations.
In addition to carbamazepine, the PBPK model was also implemented for prediction of interactions with ketoconazole and rifampicin across different age groups (2–65 years). Predicted AUC ratios of imatinib in the presence and absence of each of the modulators are summarized in Figure 7. It is noteworthy that the administration CYP3A modulators at their typical maintenance dosing regimens according to age bands yielded Css,max that were comparable across all groups, except for rifampicin, where Css,max was around 30% lower in middle-aged adults compared to children less than 18 years (Figure 7). This was important to evaluate the extent of interactions among different age groups without being confounded by steady-state concentrations of the modulators. Further statistical analysis suggested that there were no significant differences in the extent of interactions between different age bands (one-way ANOVA followed by a Tukey post-hoc analysis, p > 0.01).
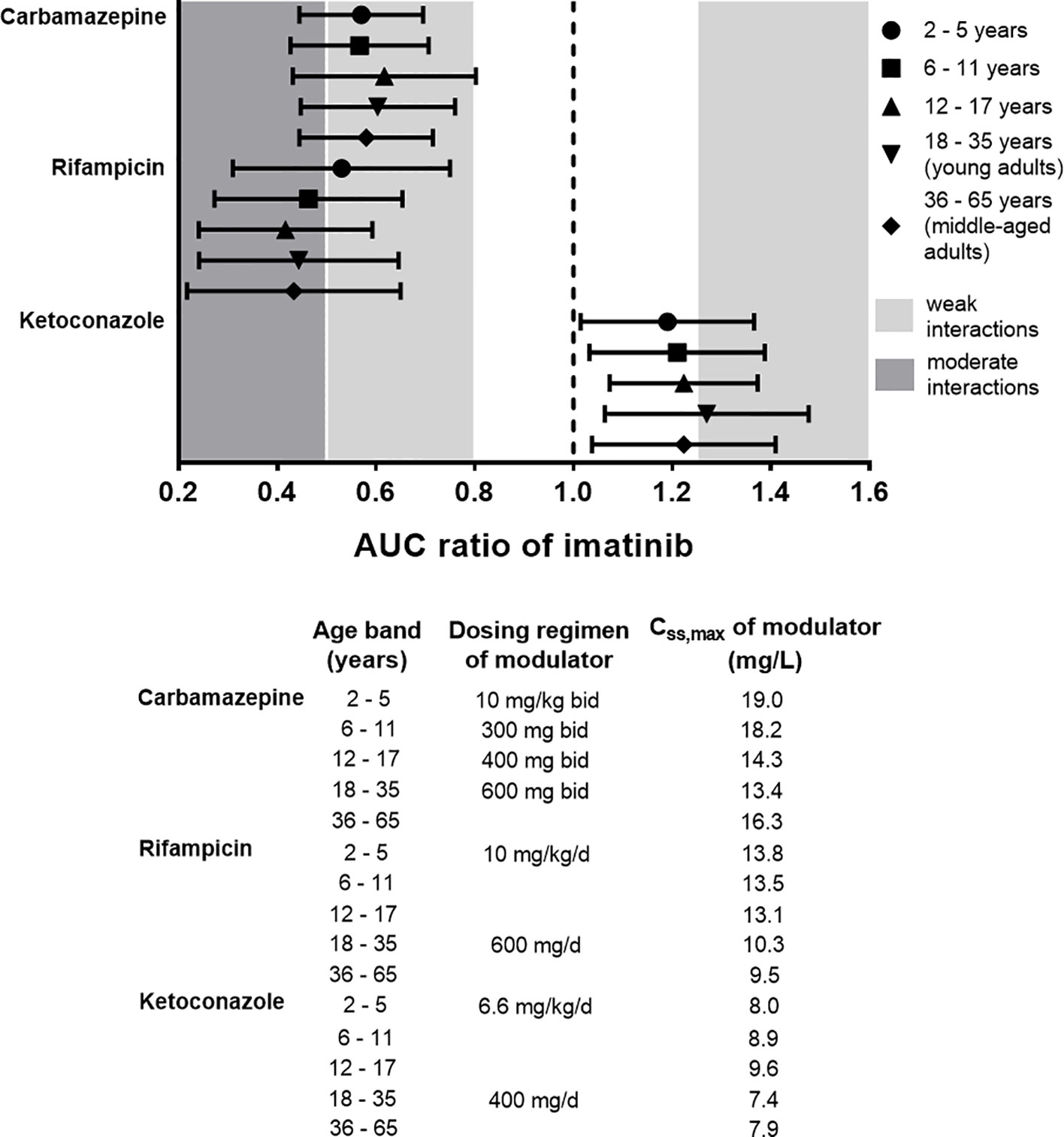
Figure 7 Physiologically based pharmacokinetic (PBPK) prediction of imatinib interactions with a set of CYP3A modulators (carbamazepine, ketoconazole, and rifampicin) at steady-state across different age bands. Imatinib at daily doses of 400 mg and 230 mg/m2 was administered to adult and paediatric populations, respectively along with CYP3A modulators for 14 days. The extent of interactions was evaluated based on AUC ratio metric (ratio of area under the plasma concentration-time curve of imatinib in the presence and absence of CYP3A modulators). Symbols represent median simulated AUC ratio with whiskers crossing from 5th to 95th percentiles. Css,max, peak concentration at steady-state. AUC ratio of 1 (dotted black line) indicates absence of drug interactions with imatinib. Typical dosing regimens and the attained Css,max of the modulators for each age band in the PBPK simulations are also detailed.
Discussion
We developed a PBPK model for imatinib in adult populations and extrapolated its use to paediatrics. The PBPK model was able to describe imatinib pharmacokinetics in both populations and had a capability to predict drug interactions with a range of CYP3A modulators.
A paediatric PBPK model for imatinib has been reported previously in a regulatory document submitted to European Medicines Agency (2013). Unfortunately, a lack of details regarding this PBPK model’s structure and parameters limits its further use and interpretation. The PBPK model in the current study was verified to a larger set of clinically published pharmacokinetic data and its implementation was extended to predict drug interactions in paediatrics.
Scaling drug doses from adults to children is far from a straightforward process (Johnson, 2008). Both population pharmacokinetic and PBPK approaches have been used independently or in combination to guide drug dosing in paediatric patients (Johnson, 2005). A population pharmacokinetic model incorporating body weight as a primary covariate with an allometric exponent, e.g. ¾ for clearance, often does not perform well in infants and young children due to maturation of drug eliminating processes (Germovsek et al., 2017). In most cases, the predictions are improved by employing a sigmoidal ontogeny function driven by postmenstrual age (Anderson and Holford, 2011). However, the maturation half-life and Hill coefficient which parameterise the function vary across different drugs (Holford et al., 2013; Germovsek et al., 2017) and thus, sufficient number of individuals with age around the maturation half-life is necessary for precise parameter estimation. PBPK modeling and simulation offers an alternative approach to evaluate an optimal dosing regimen in the paediatric population. It integrates drug-specific inputs and system-related parameters, the latter of which encompass developmental changes in physiology and maturational rates of drug-metabolising enzymes and proteins involved in drug disposition (Maharaj and Edginton, 2014). This approach enables extrapolation from adults or between age groups within paediatric populations and increases the mechanistic understanding of potential sources of interindividual variability in systemic exposure to a drug.
The ontogeny profiles of key CYP enzymes responsible for imatinib metabolism (Figure 2) are based on a meta-analysis of in vitro CYP activity in post-mortem livers of donors from different ages (Johnson et al., 2006). The maturation functions tend to underestimate the apparent clearance of CYP3A substrates in neonates and infants (Salem et al., 2014). Two in vivo-derived algorithms have been proposed to improve the prediction (Salem et al., 2014; Upreti and Wahlstrom, 2016). The Upreti and Wahlstrom model for CYP3A4 maturation has been shown to perform better with less underprediction of clearance (Johnson et al., 2019). However, as expected, the PBPK simulations that implemented this ontogeny for children older than 2 years of age yielded a similar result to that of in vitro maturation function (results not shown). Therefore, the latter, which is incorporated in the Simcyp Simulator (version 17) by default, was utilized throughout the simulations. Developmental changes in organ size, particularly liver volume were also incorporated in the PBPK model. Liver volume was most parsimoniously described by a nonlinear regression against BSA as shown in Eq. 6 (Johnson et al., 2005). Interestingly, this equation was in concordance with an allometric weight model with an exponent of ¾ in estimating liver volume from infants to adolescents (Fanta et al., 2007). The correlation between liver volume and BSA alone was superior than that with other covariates (Johnson et al., 2005), in agreement with the findings of a nonlinear mixed effect modeling approach (Small et al., 2017). All the ontogeny equations used in the current study were driven by postnatal age. Postmenstrual age is more useful if preterm neonates are included in PBPK simulations (Abduljalil et al., 2019; Germovsek et al., 2019).
It is noteworthy that the PBPK models may overestimate clinically observed peak plasma concentrations (Cmax) since they report predicted concentrations at the central venous compartment rather than the peripheral vein from which blood (plasma) samples were collected. This is particularly important for intravenous (i.v.) administration routes where a substantial amount of drug is delivered to central venous compartment directly and equilibration to the peripheral venous sites may not be instantaneous (Musther et al., 2015). A PBPK model prediction of drug concentrations at a peripheral sampling site based on contributions from surrounding tissues (e.g., adipose, muscle, and skin) as proposed by Musther et al (2015) has proven to be useful to correct the PBPK predictions at initial time following i.v. administrations. As depicted in Figure S1, implementation of this strategy within the Simcyp Simulator improved the PBPK model predictions of Cmax following a 1-h infusion of imatinib (100 mg) (Peng et al., 2004) compared to that of central venous compartment (prediction differences of imatinib Cmax of 0.99 vs. 1.42). Prediction differences for other pharmacokinetic parameters of imatinib were similar between the two strategies (results not shown). Conversely, the peripheral sampling site model has little to no effect on PBPK predictions of Cmax of imatinib given orally (results not shown). Unlike i.v. administration over a short period of time, oral administrations of drugs are likely to give sufficient time for central venous compartment (pooled venous return) and peripheral vein in the arm to equilibrate (Musther et al., 2015).
The observed interindividual variability of imatinib concentrations in children on day 1 appeared to be higher than that at steady-state from the corresponding patient cohort (Figures 3F–I). The reason for this trend was not clear, but may be related to a lower between individual variability in CYP3A4 activity due to the autoinhibition by imatinib following chronic exposure (Filppula et al., 2012; Filppula et al., 2013a). PBPK simulations also highlighted a higher interindividual variability of imatinib concentrations at a fixed daily dose compared to a BSA-normalized dosing regimen (Figures 3M, N vs. 3F–L). A daily dose administered on a mg/m2 basis in paediatric populations is usually preferred to body weight-based and flat-fixed dosing regimens owing to more favorable pharmacokinetic variability, particularly over a wide age range (Bartelink et al., 2006; Hempel and Boos, 2007).
A clear exposure-response relationship for imatinib has not been established in younger patients with CML. Thus, the proposed targets in children and adolescents were based on the concentration known to be safe and efficacious in adults (Cmin ranging from 1,000 to 3,200 ng/ml (Lankheet et al., 2017)). This was further supported by the similar biological and clinical features of CML observed in adult and younger patients (Barr, 2010), with only a slight difference, particularly a higher leukocyte count presented in the latter (Millot et al., 2005). Paediatric and adult patients also had comparable response and safety profiles (e.g., occurrence of grade 3/4 haematological toxicities and musculoskeletal adverse events) to an equivalent dose of imatinib (Millot et al., 2011). This was not the case for solid tumours harboring mutations in the gene that encodes tyrosine kinase KIT (e.g. GIST). Imatinib exerted minor anticancer activity in children with GIST compared to the adult cohort, despite similar systemic concentrations (Geoerger et al., 2009).
The observed trend of a higher interindividual variability of simulated Cmin in children aged between 2 and 5 years and middle-aged adults compared to other age groups (Figure 4) was likely attributed to a higher variability within these age bands due to maturational changes of CYP enzymes that have not attained adult levels of expression (Johnson et al., 2006) and a reduction of total hepatic clearance related to a decrease of liver weight and scaling factor (e.g., microsomal protein per gram of liver/MPPGL) (Barter et al., 2007; Chetty et al., 2018), respectively.
PBPK simulations suggested a similar Cmin following imatinib doses of 230 and 340 mg/m2/d in paediatrics and 400 and 600 mg/d in adult population, respectively (p > 0.01). This was in agreement with the finding in clinical studies in children with Ph+ leukaemias or GIST which indicated a similar systemic exposure of imatinib at daily doses of 230 and 340 mg/m2 compared to those of adult patients treated with 400 and 600 mg/d of imatinib, respectively (Champagne et al., 2004; Geoerger et al., 2009). The Cmin target of 1,000 ng/ml was predicted to be attainable by a 230 mg/m2/d dose in paediatric age groups (similar to an adult dose of 400 mg/d), albeit with a large subset of the population below the target. Due to this variability, a higher dose of 340 mg/m2/d (corresponds to an adult dose of 600 mg/d) might be needed. This was in line with the recommendation for the treatment of CML in children with the recommended initial doses of 260–300 mg/m2/d and 400 mg/m2/d for chronic and accelerated phases, respectively (de la Fuente et al., 2014).
There was a good agreement between PBPK model prediction and clinically observed changes in imatinib concentrations due to the coadministration of carbamazepine in adult and paediatric populations (Figure 6). It should be noted that clinical pharmacokinetic data in the latter came from one Japanese paediatric patient (a case study) (Taguchi et al., 2014). PBPK simulations in paediatrics refer to European ancestry, from which the ontogeny functions for drug-metabolising enzymes and AAG were derived (Johnson et al., 2006). However, our previous simulation study suggested little to no difference in imatinib pharmacokinetic between people from Japanese and European ancestry (unpublished).
Clinical drug-drug interaction (DDI) data in adults may not be suitable for extrapolation across all paediatric age bands (Salem et al., 2013b; Salerno et al., 2019). The magnitudes of enzyme-based DDI are dictated by the level of contribution (fm) and maturational rates of corresponding CYP enzymes (Salem et al., 2013a). In this study, a PBPK modeling approach was utilized to evaluate drug interactions with imatinib in paediatrics. The trend and extent of interactions between imatinib and CYP3A modulators (carbamazepine, rifampicin and ketoconazole) were predicted to be similar between paediatric and adult populations, despite a slight difference in the simulated means and interindividual variabilities (Figure 7). Imatinib inhibits its own CYP3A4-mediated metabolism following multiple-dosing regimen (Filppula et al., 2012). Thus, the effect of CYP3A modulators on imatinib metabolism was likely to be diminished following a long-term use of imatinib, as observed in a clinical interaction study between imatinib and ritonavir (van Erp et al., 2007). The extent of modulation by CYP3A inhibitors, either direct (reversible) or mechanism-based inhibitors, e.g., ketoconazole and ritonavir, respectively was predicted to be more affected following repeated-dose administration of imatinib, compared to that observed with CYP3A inducers (e.g., rifampicin and carbamazepine). This was due to limited residual CYP3A activity which can further be inhibited in the former. Since imatinib undergoes little to no metabolism in the enterocytes (Barratt and Somogyi, 2017), inducers of CYP3A confined to intestinal enzymes (e.g., hyperforin in St John’s wort) are unlikely to affect steady-state CL/F of imatinib (Adiwidjaja et al., 2019).
The limitation of this study is a lack of specific maturation functions for children with cancer implemented in the PBPK model. The trend of developmental changes in organ size, CYP enzymes and plasma proteins observed in healthy children may not hold true for the paediatric cancer population (Thai et al., 2015). A further limitation to this study is the exclusion of children less than 2 years of age from the simulations (Figures 4 and 7) due to a paucity of clinical pharmacokinetic data for this age group (CML is exceptionally rare in very young children (de la Fuente et al., 2014)). Moreover, there is a high uncertainty in the maturation pattern of CYP3A4 in this challenging age group (Johnson et al., 2019), which is further complicated by the potential presence of CYP3A7 enzyme. The latter is absent in adults, but expressed at a high level during foetal life and decreases progressively throughout the first 2 years after birth (Allegaert and van den Anker, 2019). A further study to elucidate CYP3A7 contribution to imatinib metabolism is necessary in order to perform a PBPK prediction with confidence in children less than 2 years.
In conclusion, a PBPK model for imatinib was successfully developed in adults and extrapolated to the paediatric population. The PBPK model was able to describe clinical pharmacokinetic data from published studies observed in adults, children and adolescents. PBPK simulation suggested an optimal dosing regimen range for imatinib of 230–340 mg/m2/d in paediatrics, in concordance with the recommended initial dose for treatment of childhood CML. The simulations also highlighted that children and adults being treated with imatinib have similar vulnerability to drug interactions that modulate drug metabolising enzyme activity. These findings suggest that at steady-state, imatinib is more susceptible to hepatic induction compared to inhibition of CYP3A enzymes.
Data Availability Statement
All datasets generated for this study are included in the article/Supplementary Material.
Author Contributions
JA, AB, and AM wrote the manuscript, designed the research, and contributed to the interpretation. JA performed the simulations and analyzed the data.
Conflict of Interest
The authors declare that the research was conducted in the absence of any commercial or financial relationships that could be construed as a potential conflict of interest.
Acknowledgments
JA is receiving a postgraduate scholarship from Indonesia Endowment Fund for Education (LPDP), Ministry of Finance of the Republic of Indonesia. Certara UK Limited (Simcyp Division) is gratefully acknowledged for providing the access to Simcyp Simulator.
Supplementary Material
The Supplementary Material for this article can be found online at: https://www.frontiersin.org/articles/10.3389/fphar.2019.01672/full#supplementary-material
References
Abduljalil, K., Pan, X., Pansari, A., Jamei, M., Johnson, T. N. (2019). A preterm physiologically based pharmacokinetic model. part I: physiological parameters and model building. Clin. Pharmacokinet. doi: 10.1007/s40262-019-00825-6
Adiwidjaja, J., Boddy, A. V., McLachlan, A. J. (2019). Physiologically based pharmacokinetic modelling of hyperforin to predict drug interactions with St John’s wort. Clin. Pharmacokinet. 58 (7), 911–926. doi: 10.1007/s40262-019-00736-6
Allegaert, K., van den Anker, J. (2019). Ontogeny of phase I metabolism of drugs. J. Clin. Pharmacol. 59 Suppl 1, S33–S41. doi: 10.1002/jcph.1483
Almond, L. M., Mukadam, S., Gardner, I., Okialda, K., Wong, S., Hatley, O., et al. (2016). Prediction of drug-drug interactions arising from CYP3A induction using a physiologically based dynamic model. Drug Metab. Dispos. 44 (6), 821–832. doi: 10.1124/dmd.115.066845
Anderson, B. J., Holford, N. H. (2011). Tips and traps analyzing pediatric PK data. Paediatr. Anaesth. 21 (3), 222–237. doi: 10.1111/j.1460-9592.2011.03536.x
Asaumi, R., Toshimoto, K., Tobe, Y., Hashizume, K., Nunoya, K. I., Imawaka, H., et al. (2018). Comprehensive PBPK Model of Rifampicin for quantitative prediction of complex drug-drug interactions: CYP3A/2C9 Induction and OATP inhibition effects. CPT Pharmacometrics Syst. Pharmacol. 7 (3), 186–196. doi: 10.1002/psp4.12275
Bardare, M., Tortorano, A. M., Pietrogrande, M. C., Viviani, M. A. (1984). Pharmacokinetics of ketoconazole and treatment evaluation in candidal infections. Arch. Dis. Child 59 (11), 1068–1071. doi: 10.1136/adc.59.11.1068
Barker, C. I. S., Standing, J. F., Kelly, L. E., Hanly Faught, L., Needham, A. C., Rieder, M. J., et al. (2018). Pharmacokinetic studies in children: recommendations for practice and research. Arch. Dis. Child 103 (7), 695–702. doi: 10.1136/archdischild-2017-314506
Barr, R. D. (2010). Imatinib mesylate in children and adolescents with cancer. Pediatr. Blood Cancer 55 (1), 18–25. doi: 10.1002/pbc.22484
Barratt, D. T., Somogyi, A. A. (2017). Role of pharmacogenetics in personalised imatinib dosing. Trans. Cancer Res. 6 (Suppl 10), S1541–S1557. doi: 10.21037/tcr.2017.09.08
Bartelink, I. H., Rademaker, C. M., Schobben, A. F., van den Anker, J. N. (2006). Guidelines on paediatric dosing on the basis of developmental physiology and pharmacokinetic considerations. Clin. Pharmacokinet. 45 (11), 1077–1097. doi: 10.2165/00003088-200645110-00003
Barter, Z. E., Bayliss, M. K., Beaune, P. H., Boobis, A. R., Carlile, D. J., Edwards, R. J., et al. (2007). Scaling factors for the extrapolation of in vivo metabolic drug clearance from in vitro data: reaching a consensus on values of human microsomal protein and hepatocellularity per gram of liver. Curr. Drug Metab. 8 (1), 33–45. doi: 10.2174/138920007779315053
Baruchel, S., Sharp, J. R., Bartels, U., Hukin, J., Odame, I., Portwine, C., et al. (2009). A Canadian paediatric brain tumour consortium (CPBTC) phase II molecularly targeted study of imatinib in recurrent and refractory paediatric central nervous system tumours. Eur. J. Cancer 45 (13), 2352–2359. doi: 10.1016/j.ejca.2009.05.008
Batchelor, H. K., Marriott, J. F. (2013). Paediatric pharmacokinetics: key considerations. Br. J. Clin. Pharmacol. 79 (3), 395–404. doi: 10.1111/bcp.12267
Bi, Y., Liu, J., Li, L., Yu, J., Bhattaram, A., Bewernitz, M., et al. (2019). Role of model-informed drug development in pediatric drug development, regulatory evaluation, and labeling. J. Clin. Pharmacol. 59 Suppl 1, S104–S111. doi: 10.1002/jcph.1478
Bins, S., Eechoute, K., Kloth, J. S., de Man, F. M., Oosten, A. W., de Bruijn, P., et al. (2017). Prospective analysis in GIST patients on the role of alpha-1 acid glycoprotein in imatinib exposure. Clin. Pharmacokinet. 56 (3), 305–310. doi: 10.1007/s40262-016-0441-0
Bolton, A. E., Peng, B., Hubert, M., Krebs-Brown, A., Capdeville, R., Keller, U., et al. (2004). Effect of rifampicin on the pharmacokinetics of imatinib mesylate (Gleevec, STI571) in healthy subjects. Cancer Chemother Pharmacol. 53 (2), 102–106. doi: 10.1007/s00280-003-0722-9
Bornhauser, M., Pursche, S., Bonin, M., Freiberg-Richter, J., Jenke, A., Illmer, T., et al. (2005). Elimination of imatinib mesylate and its metabolite N-desmethyl-imatinib. J. Clin. Oncol. 23 (16), 3855–3856. author reply 3857-3858. doi: 10.1200/JCO.2005.05.246
Breedveld, P., Pluim, D., Cipriani, G., Wielinga, P., van Tellingen, O., Schinkel, A. H., et al. (2005). The effect of Bcrp1 (Abcg2) on the in vivo pharmacokinetics and brain penetration of imatinib mesylate (Gleevec): implications for the use of breast cancer resistance protein and P-glycoprotein inhibitors to enable the brain penetration of imatinib in patients. Cancer Res. 65 (7), 2577–2582. doi: 10.1158/0008-5472.CAN-04-2416
Carlsson, K. C., Hoem, N. O., Glauser, T., Vinks, A. A. (2005). Development of a population pharmacokinetic model for carbamazepine based on sparse therapeutic monitoring data from pediatric patients with epilepsy. Clin. Ther. 27 (5), 618–626. doi: 10.1016/j.clinthera.2005.05.001
Champagne, M. A., Capdeville, R., Krailo, M., Qu, W., Peng, B., Rosamilia, M., et al. (2004). Imatinib mesylate (STI571) for treatment of children with Philadelphia chromosome-positive leukemia: results from a Children’s Oncology Group phase 1 study. Blood 104 (9), 2655–2660. doi: 10.1182/blood-2003-09-3032
Chen, Y., Liu, L., Nguyen, K., Fretland, A. J. (2011). Utility of intersystem extrapolation factors in early reaction phenotyping and the quantitative extrapolation of human liver microsomal intrinsic clearance using recombinant cytochromes P450. Drug Metab. Dispos. 39 (3), 373–382. doi: 10.1124/dmd.110.035147
Chetty, M., Johnson, T. N., Polak, S., Salem, F., Doki, K., Rostami-Hodjegan, A. (2018). Physiologically based pharmacokinetic modelling to guide drug delivery in older people. Adv. Drug Deliv. Rev. 135, 85–96. doi: 10.1016/j.addr.2018.08.013
Cheung, K. W. K., van Groen, B. D., Burckart, G. J., Zhang, L., de Wildt, S. N., Huang, S. M. (2019). Incorporating ontogeny in physiologically based pharmacokinetic modeling to improve pediatric drug development: what we know about developmental changes in membrane transporters. J. Clin. Pharmacol. 59 Suppl 1, S56–S69. doi: 10.1002/jcph.1489
Coebergh, J. W., Reedijk, A. M., de Vries, E., Martos, C., Jakab, Z., Steliarova-Foucher, E., et al. (2006). Leukaemia incidence and survival in children and adolescents in Europe during 1978-1997. report from the automated childhood cancer information system project. Eur. J. Cancer 42 (13), 2019–2036. doi: 10.1016/j.ejca.2006.06.005
Cole, S., Hay, J. L., Luzon, E., Nordmark, A., Rusten, I. S. (2017). European regulatory perspective on pediatric physiologically based pharmacokinetic models. Int. J. Pharmacokinet. 2 (2), 113–124. doi: 10.4155/ipk-2016-0025
Cubitt, H. E., Yeo, K. R., Howgate, E. M., Rostami-Hodjegan, A., Barter, Z. E. (2011). Sources of interindividual variability in IVIVE of clearance: an investigation into the prediction of benzodiazepine clearance using a mechanistic population-based pharmacokinetic model. Xenobiotica 41 (8), 623–638. doi: 10.3109/00498254.2011.560294
Dai, H., Marbach, P., Lemaire, M., Hayes, M., Elmquist, W. F. (2003). Distribution of STI-571 to the brain is limited by P-glycoprotein-mediated efflux. J. Pharmacol. Exp. Ther. 304 (3), 1085–1092. doi: 10.1124/jpet.102.045260
Darwich, A. S., Neuhoff, S., Jamei, M., Rostami-Hodjegan, A. (2010). Interplay of metabolism and transport in determining oral drug absorption and gut wall metabolism: a simulation assessment using the "Advanced Dissolution, Absorption, Metabolism (ADAM)" model. Curr. Drug Metab. 11 (9), 716–729. doi: 10.2174/138920010794328913
de la Fuente, J., Baruchel, A., Biondi, A., de Bont, E., Dresse, M. F., Suttorp, M., et al. (2014). Managing children with chronic myeloid leukaemia (CML): recommendations for the management of CML in children and young people up to the age of 18 years. Br. J. Haematol. 167 (1), 33–47. doi: 10.1111/bjh.12977
Diestelhorst, C., Boos, J., McCune, J. S., Russell, J., Kangarloo, S. B., Hempel, G. (2014). Predictive performance of a physiologically based pharmacokinetic model of busulfan in children. Pediatr. Hematol. Oncol. 31 (8), 731–742. doi: 10.3109/08880018.2014.927945
DuBois, D., DuBois, E. F. (1916). A formula to estimate the approximate surface area if height and weight be known. Arch. Intern Med. 17, 863–871. doi: 10.1001/archinte.1916.00080130010002
Eechoute, K., Fransson, M. N., Reyners, A. K., de Jong, F. A., Sparreboom, A., van der Graaf, W. T., et al. (2012). A long-term prospective population pharmacokinetic study on imatinib plasma concentrations in GIST patients. Clin. Cancer Res. 18 (20), 5780–5787. doi: 10.1158/1078-0432.CCR-12-0490
Eeg-Olofsson, O., Nilsson, H. L., Tonnby, B., Arvidsson, J., Grahn, P. A., Gylje, H., et al. (1990). Diurnal variation of carbamazepine and carbamazepine-10,11-epoxide in plasma and saliva in children with epilepsy: a comparison between conventional and slow-release formulations. J. Child Neurol. 5 (2), 159–165. doi: 10.1177/088307389000500219
European Medicines Agency. (2013). EMA/CHMP/161314/2013: Assessment report for Glivec (imatinib). www.ema.europa.eu/en/documents/variation-report/glivec-h-c-406-ii-0080-epar-assessment-report-variation_en.pdf.
Fanta, S., Jonsson, S., Backman, J. T., Karlsson, M. O., Hoppu, K. (2007). Developmental pharmacokinetics of ciclosporin–a population pharmacokinetic study in paediatric renal transplant candidates. Br. J. Clin. Pharmacol. 64 (6), 772–784. doi: 10.1111/j.1365-2125.2007.03003.x
Filppula, A. M., Laitila, J., Neuvonen, P. J., Backman, J. T. (2012). Potent mechanism-based inhibition of CYP3A4 by imatinib explains its liability to interact with CYP3A4 substrates. Br. J. Pharmacol. 165 (8), 2787–2798. doi: 10.1111/j.1476-5381.2011.01732.x
Filppula, A. M., Neuvonen, M., Laitila, J., Neuvonen, P. J., Backman, J. T. (2013a). Autoinhibition of CYP3A4 leads to important role of CYP2C8 in imatinib metabolism: variability in CYP2C8 activity may alter plasma concentrations and response. Drug Metab. Dispos. 41 (1), 50–59. doi: 10.1124/dmd.112.048017
Filppula, A. M., Tornio, A., Niemi, M., Neuvonen, P. J., Backman, J. T. (2013b). Gemfibrozil impairs imatinib absorption and inhibits the CYP2C8-mediated formation of its main metabolite. Clin. Pharmacol. Ther. 94 (3), 383–393. doi: 10.1038/clpt.2013.92
Gambacorti-Passerini, C., Zucchetti, M., Russo, D., Frapolli, R., Verga, M., Bungaro, S., et al. (2003). Alpha1 acid glycoprotein binds to imatinib (STI571) and substantially alters its pharmacokinetics in chronic myeloid leukemia patients. Clin. Cancer Res. 9 (2), 625–632.
Gandia, P., Arellano, C., Lafont, T., Huguet, F., Malard, L., Chatelut, E. (2013). Should therapeutic drug monitoring of the unbound fraction of imatinib and its main active metabolite N-desmethyl-imatinib be developed? Cancer Chemother Pharmacol. 71 (2), 531–536. doi: 10.1007/s00280-012-2035-3
Geoerger, B., Morland, B., Ndiaye, A., Doz, F., Kalifa, G., Geoffray, A., et al. (2009). Target-driven exploratory study of imatinib mesylate in children with solid malignancies by the Innovative Therapies for Children with Cancer (ITCC) European Consortium. Eur. J. Cancer 45 (13), 2342–2351. doi: 10.1016/j.ejca.2009.03.007
Germovsek, E., Barker, C. I., Sharland, M., Standing, J. F. (2017). Scaling clearance in paediatric pharmacokinetics: all models are wrong, which are useful? Br. J. Clin. Pharmacol. 83 (4), 777–790. doi: 10.1111/bcp.13160
Germovsek, E., Barker, C. I. S., Sharland, M., Standing, J. F. (2019). Pharmacokinetic-pharmacodynamic modeling in pediatric drug development, and the importance of standardized scaling of clearance. Clin. Pharmacokinet. 58 (1), 39–52. doi: 10.1007/s40262-018-0659-0
Ginsburg, C. M., McCracken, G. H., Jr., Olsen, K. (1983). Pharmacology of ketoconazole suspension in infants and children. Antimicrob. Agents Chemother 23 (5), 787–789. doi: 10.1128/AAC.23.5.787
Gotta, V., Buclin, T., Csajka, C., Widmer, N. (2013). Systematic review of population pharmacokinetic analyses of imatinib and relationships with treatment outcomes. Ther. Drug Monit. 35 (2), 150–167. doi: 10.1097/FTD.0b013e318284ef11
Grimstein, M., Yang, Y., Zhang, X., Grillo, J., Huang, S. M., Zineh, I., et al. (2019). Physiologically based pharmacokinetic modeling in regulatory science: an update from the u.s. food and drug administration’s office of clinical pharmacology. J. Pharm. Sci. 108 (1), 21–25. doi: 10.1016/j.xphs.2018.10.033
Gschwind, H. P., Pfaar, U., Waldmeier, F., Zollinger, M., Sayer, C., Zbinden, P., et al. (2005). Metabolism and disposition of imatinib mesylate in healthy volunteers. Drug Metab. Dispos. 33 (10), 1503–1512. doi: 10.1124/dmd.105.004283
Haouala, A., Widmer, N., Guidi, M., Montemurro, M., Leyvraz, S., Buclin, T., et al. (2013). Prediction of free imatinib concentrations based on total plasma concentrations in patients with gastrointestinal stromal tumours. Br. J. Clin. Pharmacol. 75 (4), 1007–1018. doi: 10.1111/j.1365-2125.2012.04422.x
Heimbach, T., Lin, W., Hourcade-Potelleret, F., Tian, X., Combes, F. P., Horvath, N., et al. (2019). Physiologically based pharmacokinetic modeling to supplement nilotinib pharmacokinetics and confirm dose selection in pediatric patients. J. Pharm. Sci. 108 (6), 2191–2198. doi: 10.1016/j.xphs.2019.01.028
Hempel, G., Boos, J. (2007). Flat-fixed dosing versus body surface area based dosing of anticancer drugs: there is a difference. Oncologist 12 (8), 924–926. doi: 10.1634/theoncologist.12-8-924
Holford, N., Heo, Y. A., Anderson, B. (2013). A pharmacokinetic standard for babies and adults. J. Pharm. Sci. 102 (9), 2941–2952. doi: 10.1002/jps.23574
Jaeger, B. A., Tauer, J. T., Ulmer, A., Kuhlisch, E., Roth, H. J., Suttorp, M. (2012). Changes in bone metabolic parameters in children with chronic myeloid leukemia on imatinib treatment. Med. Sci. Monit. 18 (12), CR721–CR728. doi: 10.12659/MSM.883599
Jamei, M., Marciniak, S., Edwards, D., Wragg, K., Feng, K., Barnett, A., et al. (2013). The simcyp population based simulator: architecture, implementation, and quality assurance. In Silico Pharmacol. 1, 9. doi: 10.1186/2193-9616-1-9
Johnson, T. N., Tucker, G. T., Tanner, M. S., Rostami-Hodjegan, A. (2005). Changes in liver volume from birth to adulthood: a meta-analysis. Liver Transpl. 11 (12), 1481–1493. doi: 10.1002/lt.20519
Johnson, T. N., Rostami-Hodjegan, A., Tucker, G. T. (2006). Prediction of the clearance of eleven drugs and associated variability in neonates, infants and children. Clin. Pharmacokinet. 45 (9), 931–956. doi: 10.2165/00003088-200645090-00005
Johnson, T. N., Jamei, M., Rowland-Yeo, K. (2016). How does in vivo biliary elimination of drugs change with age? evidence from in vitro and clinical data using a systems pharmacology approach. Drug Metab. Dispos. 44 (7), 1090–1098. doi: 10.1124/dmd.115.068643
Johnson, T. N., Cleary, Y., Parrott, N., Reigner, B., Smith, J. R., Toovey, S. (2019). Development of a physiologically based pharmacokinetic model for mefloquine and its application alongside a clinical effectiveness model to select an optimal dose for prevention of malaria in young caucasian children. Br. J. Clin. Pharmacol. 85 (1), 100–113. doi: 10.1111/bcp.13764
Johnson, T. N. (2005). Modelling approaches to dose estimation in children. Br. J. Clin. Pharmacol. 59 (6), 663–669. doi: 10.1111/j.1365-2125.2005.02429.x
Johnson, T. N. (2008). The problems in scaling adult drug doses to children. Arch. Dis. Child 93 (3), 207–211. doi: 10.1136/adc.2006.114835
Kalliokoski, A., Niemi, M. (2009). Impact of OATP transporters on pharmacokinetics. Br. J. Pharmacol. 158 (3), 693–705. doi: 10.1111/j.1476-5381.2009.00430.x
Kersting, G., Willmann, S., Wurthwein, G., Lippert, J., Boos, J., Hempel, G. (2012). Physiologically based pharmacokinetic modelling of high- and low-dose etoposide: from adults to children. Cancer Chemother Pharmacol. 69 (2), 397–405. doi: 10.1007/s00280-011-1706-9
Koup, J. R., Williams-Warren, J., Viswanathan, C. T., Weber, A., Smith, A. L. (1986a). Pharmacokinetics of rifampin in children. II. Oral bioavailability. Ther. Drug Monit. 8 (1), 17–22. doi: 10.1097/00007691-198603000-00004
Koup, J. R., Williams-Warren, J., Weber, A., Smith, A. L. (1986b). Pharmacokinetics of rifampin in children. I. Multiple dose intravenous infusion. Ther. Drug Monit. 8 (1), 11–16. doi: 10.1097/00007691-198603000-00003
Kretz, O., Weiss, H. M., Schumacher, M. M., Gross, G. (2004). In vitro blood distribution and plasma protein binding of the tyrosine kinase inhibitor imatinib and its active metabolite, CGP74588, in rat, mouse, dog, monkey, healthy humans and patients with acute lymphatic leukaemia. Br. J. Clin. Pharmacol. 58 (2), 212–216. doi: 10.1111/j.1365-2125.2004.02117.x
Kuepfer, L., Niederalt, C., Wendl, T., Schlender, J. F., Willmann, S., Lippert, J., et al. (2016). Applied Concepts in PBPK Modeling: How to Build a PBPK/PD Model. CPT Pharmacometrics Syst. Pharmacol. 5 (10), 516–531. doi: 10.1002/psp4.12134
Lankheet, N. A. G., Desar, I. M. E., Mulder, S. F., Burger, D. M., Kweekel, D. M., van Herpen, C. M. L., et al. (2017). Optimizing the dose in cancer patients treated with imatinib, sunitinib and pazopanib. Br. J. Clin. Pharmacol. 83 (10), 2195–2204. doi: 10.1111/bcp.13327
Larson, R. A., Druker, B. J., Guilhot, F., O’Brien, S. G., Riviere, G. J., Krahnke, T., et al. (2008). Imatinib pharmacokinetics and its correlation with response and safety in chronic-phase chronic myeloid leukemia: a subanalysis of the IRIS study. Blood 111 (8), 4022–4028. doi: 10.1182/blood-2007-10-116475
Liu, B., Crewe, H. K., Ozdemir, M., Rowland Yeo, K., Tucker, G., Rostami-Hodjegan, A. (2017). The absorption kinetics of ketoconazole plays a major role in explaining the reported variability in the level of interaction with midazolam: Interplay between formulation and inhibition of gut wall and liver metabolism. Biopharm. Drug Dispos. 38 (3), 260–270. doi: 10.1002/bdd.2058
Lubberman, F. J. E., Gelderblom, H., Wilmer, C. M., Kweekel, D. M., Desar, I. M. E., Colbers, A., et al. (2017). Does a glass of Coke boost the exposure to imatinib in gastrointestinal stromal tumour patients after gastrectomy? Br. J. Clin. Pharmacol. 83 (10), 2312–2314. doi: 10.1111/bcp.13333
Maharaj, A. R., Edginton, A. N. (2014). Physiologically based pharmacokinetic modeling and simulation in pediatric drug development. CPT Pharmacometrics Syst. Pharmacol. 3, e150. doi: 10.1038/psp.2014.45
Maharaj, A. R., Barrett, J. S., Edginton, A. N. (2013). A workflow example of PBPK modeling to support pediatric research and development: case study with lorazepam. AAPS J. 15 (2), 455–464. doi: 10.1208/s12248-013-9451-0
Maharaj, A. R., Gonzalez, D., Cohen-Wolkowiez, M., Hornik, C. P., Edginton, A. N. (2018). Improving pediatric protein binding estimates: an evaluation of alpha1-acid glycoprotein maturation in healthy and infected subjects. Clin. Pharmacokinet. 57 (5), 577–589. doi: 10.1007/s40262-017-0576-7
Marangon, E., Citterio, M., Sala, F., Barisone, E., Lippi, A. A., Rizzari, C., et al. (2009). Pharmacokinetic profile of imatinib mesylate and N-desmethyl-imatinib (CGP 74588) in children with newly diagnosed Ph+ acute leukemias. Cancer Chemother Pharmacol. 63 (3), 563–566. doi: 10.1007/s00280-008-0764-0
McCracken, G. H., Jr., Ginsburg, C. M., Zweighaft, T. C., Clahsen, J. (1980). Pharmacokinetics of rifampin in infants and children: relevance to prophylaxis against Haemophilus influenzae type b disease. Pediatrics 66 (1), 17–21.
McNamara, P. J., Alcorn, J. (2002). Protein binding predictions in infants. AAPS PharmSci. 4 (1), E4. doi: 10.1208/ps040104
Menon-Andersen, D., Mondick, J. T., Jayaraman, B., Thompson, P. A., Blaney, S. M., Bernstein, M., et al. (2009). Population pharmacokinetics of imatinib mesylate and its metabolite in children and young adults. Cancer Chemother Pharmacol. 63 (2), 229–238. doi: 10.1007/s00280-008-0730-x
Millot, F., Traore, P., Guilhot, J., Nelken, B., Leblanc, T., Leverger, G., et al. (2005). Clinical and biological features at diagnosis in 40 children with chronic myeloid leukemia. Pediatrics 116 (1), 140–143. doi: 10.1542/peds.2004-2473
Millot, F., Baruchel, A., Guilhot, J., Petit, A., Leblanc, T., Bertrand, Y., et al. (2011). Imatinib is effective in children with previously untreated chronic myelogenous leukemia in early chronic phase: results of the French national phase IV trial. J. Clin. Oncol. 29 (20), 2827–2832. doi: 10.1200/JCO.2010.32.7114
Mooij, M. G., Schwarz, U. I., de Koning, B. A., Leeder, J. S., Gaedigk, R., Samsom, J. N., et al. (2014). Ontogeny of human hepatic and intestinal transporter gene expression during childhood: age matters. Drug Metab. Dispos. 42 (8), 1268–1274. doi: 10.1124/dmd.114.056929
Musther, H., Gill, K. L., Chetty, M., Rostami-Hodjegan, A., Rowland, M., Jamei, M. (2015). Are physiologically based pharmacokinetic models reporting the right c (max)? central venous versus peripheral sampling site. AAPS J. 17 (5), 1268–1279. doi: 10.1208/s12248-015-9796-7
Neul, C., Schaeffeler, E., Sparreboom, A., Laufer, S., Schwab, M., Nies, A. T. (2016). Impact of membrane drug transporters on resistance to small-molecule tyrosine kinase inhibitors. Trends Pharmacol. Sci. 37 (11), 904–932. doi: 10.1016/j.tips.2016.08.003
Peng, B., Dutreix, C., Mehring, G., Hayes, M. J., Ben-Am, M., Seiberling, M., et al. (2004). Absolute bioavailability of imatinib (Glivec) orally versus intravenous infusion. J. Clin. Pharmacol. 44 (2), 158–162. doi: 10.1177/0091270003262101
Peng, B., Lloyd, P., Schran, H. (2005). Clinical pharmacokinetics of imatinib. Clin. Pharmacokinet. 44 (9), 879–894. doi: 10.2165/00003088-200544090-00001
Petain, A., Kattygnarath, D., Azard, J., Chatelut, E., Delbaldo, C., Geoerger, B., et al. (2008). Population pharmacokinetics and pharmacogenetics of imatinib in children and adults. Clin. Cancer Res. 14 (21), 7102–7109. doi: 10.1158/1078-0432.CCR-08-0950
Prasad, B., Gaedigk, A., Vrana, M., Gaedigk, R., Leeder, J. S., Salphati, L., et al. (2016). Ontogeny of Hepatic Drug Transporters as Quantified by LC-MS/MS Proteomics. Clin. Pharmacol. Ther. 100 (4), 362–370. doi: 10.1002/cpt.409
Pursche, S., Schleyer, E., von Bonin, M., Ehninger, G., Said, S. M., Prondzinsky, R., et al. (2008). Influence of enzyme-inducing antiepileptic drugs on trough level of imatinib in glioblastoma patients. Curr. Clin. Pharmacol. 3 (3), 198–203. doi: 10.2174/157488408785747656
Raucy, J. L., Mueller, L., Duan, K., Allen, S. W., Strom, S., Lasker, J. M. (2002). Expression and induction of CYP2C P450 enzymes in primary cultures of human hepatocytes. J. Pharmacol. Exp. Ther. 302 (2), 475–482. doi: 10.1124/jpet.102.033837
Renard, D., Bouillon, T., Zhou, P., Flesch, G., Quinn, D. (2015). Pharmacokinetic interactions among imatinib, bosentan and sildenafil, and their clinical implications in severe pulmonary arterial hypertension. Br. J. Clin. Pharmacol. 80 (1), 75–85. doi: 10.1111/bcp.12584
Rioux, N., Waters, N. J. (2016). Physiologically based pharmacokinetic modeling in pediatric oncology drug development. Drug Metab. Dispos. 44 (7), 934–943. doi: 10.1124/dmd.115.068031
Rodgers, T., Rowland, M. (2007). Mechanistic approaches to volume of distribution predictions: understanding the processes. Pharm. Res. 24 (5), 918–933. doi: 10.1007/s11095-006-9210-3
Rowland, A., van Dyk, M., Mangoni, A. A., Miners, J. O., McKinnon, R. A., Wiese, M. D., et al. (2017). Kinase inhibitor pharmacokinetics: comprehensive summary and roadmap for addressing inter-individual variability in exposure. Expert Opin. Drug Metab. Toxicol. 13 (1), 31–49. doi: 10.1080/17425255.2016.1229303
Rowland-Yeo, K., Jamei, M., Yang, J., Tucker, G. T., Rostami-Hodjegan, A. (2010). Physiologically based mechanistic modelling to predict complex drug-drug interactions involving simultaneous competitive and time-dependent enzyme inhibition by parent compound and its metabolite in both liver and gut - the effect of diltiazem on the time-course of exposure to triazolam. Eur. J. Pharm. Sci. 39 (5), 298–309. doi: 10.1016/j.ejps.2009.12.002
Salem, F., Johnson, T. N., Barter, Z. E., Leeder, J. S., Rostami-Hodjegan, A. (2013a). Age related changes in fractional elimination pathways for drugs: assessing the impact of variable ontogeny on metabolic drug-drug interactions. J. Clin. Pharmacol. 53 (8), 857–865. doi: 10.1002/jcph.100
Salem, F., Rostami-Hodjegan, A., Johnson, T. N. (2013b). Do children have the same vulnerability to metabolic drug-drug interactions as adults? A critical analysis of the literature. J. Clin. Pharmacol. 53 (5), 559–566. doi: 10.1002/jcph.13
Salem, F., Johnson, T. N., Abduljalil, K., Tucker, G. T., Rostami-Hodjegan, A. (2014). A re-evaluation and validation of ontogeny functions for cytochrome P450 1A2 and 3A4 based on in vivo data. Clin. Pharmacokinet. 53 (7), 625–636. doi: 10.1007/s40262-014-0140-7
Salerno, S. N., Burckart, G. J., Huang, S. M., Gonzalez, D. (2019). Pediatric Drug-Drug Interaction Studies: Barriers and Opportunities. Clin. Pharmacol. Ther. 105 (5), 1067–1070. doi: 10.1002/cpt.1234
Schultz, K. R., Carroll, A., Heerema, N. A., Bowman, W. P., Aledo, A., Slayton, W. B., et al. (2014). Long-term follow-up of imatinib in pediatric philadelphia chromosome-positive acute lymphoblastic leukemia: children’s oncology group study AALL0031. Leukemia 28 (7), 1467–1471. doi: 10.1038/leu.2014.30
Small, B. G., Wendt, B., Jamei, M., Johnson, T. N. (2017). Prediction of liver volume - a population-based approach to meta-analysis of paediatric, adult and geriatric populations - an update. Biopharm. Drug Dispos. 38 (4), 290–300. doi: 10.1002/bdd.2063
Smith, P., Bullock, J. M., Booker, B. M., Haas, C. E., Berenson, C. S., Jusko, W. J. (2004). The influence of St. John’s wort on the pharmacokinetics and protein binding of imatinib mesylate. Pharmacotherapy 24 (11), 1508–1514. doi: 10.1592/phco.24.16.1508.50958
Suttorp, M., Bornhauser, M., Metzler, M., Millot, F., Schleyer, E. (2018a). Pharmacology and pharmacokinetics of imatinib in pediatric patients. Expert Rev. Clin. Pharmacol. 11 (3), 219–231. doi: 10.1080/17512433.2018.1398644
Suttorp, M., Schulze, P., Glauche, I., Gohring, G., von Neuhoff, N., Metzler, M., et al. (2018b). Front-line imatinib treatment in children and adolescents with chronic myeloid leukemia: results from a phase III trial. Leukemia 32 (7), 1657–1669. doi: 10.1038/s41375-018-0179-9
Taguchi, K., Kouroki, M., Ohmura, T., Jono, H., Endo, F., Saito, H. (2014). Carbamazepine-imatinib interaction in a child with chronic myeloid leukemia. Pediatr. Int. 56 (4), e33–e36. doi: 10.1111/ped.12382
Thai, H. T., Mazuir, F., Cartot-Cotton, S., Veyrat-Follet, C. (2015). Optimizing pharmacokinetic bridging studies in paediatric oncology using physiologically-based pharmacokinetic modelling: application to docetaxel. Br. J. Clin. Pharmacol. 80 (3), 534–547. doi: 10.1111/bcp.12702
Thorn, C. F., Leckband, S. G., Kelsoe, J., Leeder, J. S., Muller, D. J., Klein, T. E., et al. (2011). PharmGKB summary: carbamazepine pathway. Pharmacogenet. Genomics 21 (12), 906–910. doi: 10.1097/FPC.0b013e328348c6f2
Upreti, V. V., Wahlstrom, J. L. (2016). Meta-analysis of hepatic cytochrome P450 ontogeny to underwrite the prediction of pediatric pharmacokinetics using physiologically based pharmacokinetic modeling. J. Clin. Pharmacol. 56 (3), 266–283. doi: 10.1002/jcph.585
van Erp, N. P., Gelderblom, H., Karlsson, M. O., Li, J., Zhao, M., Ouwerkerk, J., et al. (2007). Influence of CYP3A4 inhibition on the steady-state pharmacokinetics of imatinib. Clin. Cancer Res. 13 (24), 7394–7400. doi: 10.1158/1078-0432.CCR-07-0346
Verheijen, R. B., Yu, H., Schellens, J. H. M., Beijnen, J. H., Steeghs, N., Huitema, A. D. R. (2017). Practical recommendations for therapeutic drug monitoring of kinase inhibitors in oncology. Clin. Pharmacol. Ther. 102 (5), 765–776. doi: 10.1002/cpt.787
Walsh, C., Bonner, J. J., Johnson, T. N., Neuhoff, S., Ghazaly, E. A., Gribben, J. G., et al. (2016). Development of a physiologically based pharmacokinetic model of actinomycin D in children with cancer. Br. J. Clin. Pharmacol. 81 (5), 989–998. doi: 10.1111/bcp.12878
Widmer, N., Decosterd, L. A., Csajka, C., Leyvraz, S., Duchosal, M. A., Rosselet, A., et al. (2006). Population pharmacokinetics of imatinib and the role of alpha-acid glycoprotein. Br. J. Clin. Pharmacol. 62 (1), 97–112. doi: 10.1111/j.1365-2125.2006.02719.x
Yang, J., Jamei, M., Rowland-Yeo, K., Tucker, G. T., Rostami-Hodjegan, A. (2007). Prediction of intestinal first-pass drug metabolism. Curr. Drug Metab. 8 (7), 676–684. doi: 10.2174/138920007782109733
Yang, J., Liao, M., Shou, M., Jamei, M., Rowland-Yeo, K., Tucker, G. T., et al. (2008). Cytochrome p450 turnover: regulation of synthesis and degradation, methods for determining rates, and implications for the prediction of drug interactions. Curr. Drug Metab. 9 (5), 384–394. doi: 10.2174/138920008784746382
Yellepeddi, V., Rower, J., Liu, X., Kumar, S., Rashid, J., Sherwin, C. M. T. (2019). State-of-the-Art review on physiologically based pharmacokinetic modeling in pediatric drug development. Clin. Pharmacokinet. 58 (1), 1–13. doi: 10.1007/s40262-018-0677-y
Zhang, G. J., Patel, R., Clark, R. J., Ho, T., Trisdale, S. K., Fang, Y., et al. (2015). Effect of fifteen CYP3A4 in vitro inducers on the induction of CYP2B6, CYP2C8, CYP2C9, CYP2C19 and CYP3A5 in plated human hepatocytes: a trend analysis. 20th North American ISSX Meeting. www.corning.com/catalog/cls/documents/posters/poster_2015_ISSX_P119_Effect_of_Fifteen_Inducer.pdf.
Keywords: imatinib, physiologically based pharmacokinetic (PBPK), simulation, paediatrics, drug interactions
Citation: Adiwidjaja J, Boddy AV and McLachlan AJ (2020) Implementation of a Physiologically Based Pharmacokinetic Modeling Approach to Guide Optimal Dosing Regimens for Imatinib and Potential Drug Interactions in Paediatrics. Front. Pharmacol. 10:1672. doi: 10.3389/fphar.2019.01672
Received: 17 October 2019; Accepted: 23 December 2019;
Published: 30 January 2020.
Edited by:
Rob ter Heine, Radboud University Nijmegen Medical Centre, NetherlandsReviewed by:
Geoffrey Thomas Tucker, University of Sheffield, United KingdomMuhammad Usman, University of Veterinary and Animal Sciences, Pakistan
Copyright © 2020 Adiwidjaja, Boddy and McLachlan. This is an open-access article distributed under the terms of the Creative Commons Attribution License (CC BY). The use, distribution or reproduction in other forums is permitted, provided the original author(s) and the copyright owner(s) are credited and that the original publication in this journal is cited, in accordance with accepted academic practice. No use, distribution or reproduction is permitted which does not comply with these terms.
*Correspondence: Andrew J. McLachlan, YW5kcmV3Lm1jbGFjaGxhbkBzeWRuZXkuZWR1LmF1