- 1Drug Clinical Trial Center, Peking University Third Hospital, Beijing, China
- 2School of Pharmaceutical Sciences, Tsinghua University, Beijing, China
- 3School of Pharmaceutical Sciences, Peking University Health Science Center, Peking University, Beijing, China
- 4School of Basic Medicine and Clinical Pharmacy, China Pharmaceutical University, Nanjing, China
- 5Janssen China R&D Center, Shanghai, China
- 6Certara UK Limited, Simcyp Division, Sheffield, United Kingdom
- 7Department of Cardiology and Institute of Vascular Medicine, Peking University Third Hospital, Beijing, China
Background: Physiologically based pharmacokinetic (PBPK) modeling and simulating may be a powerful tool in predicting drug behaviors in specific populations. It is a mathematical model that relates the pharmacokinetic (PK) profile of a compound with human anatomical characteristics, physiological characteristics, and biochemical parameters. Predictions using PBPK models offer a promising way to guide drug development and can be used to optimize clinical dosing regimens. However, PK data of new drugs in the pediatric population are too limited to guide clinical therapy, which may lead to frequent adverse events or insufficient efficacy for pediatric patients, particularly in neonates and infants.
Objective: The objective of this study was to establish a virtual Chinese pediatric population based on the physiological parameters of Chinese children that could be utilized in PBPK models.
Methods: A Chinese pediatric PBPK model was developed in Simcyp Simulator by collecting published Chinese pediatric physiological and anthropometric data to use as system parameters. This pediatric population model was then evaluated in the Chinese pediatric population by predicting the pharmacokinetic characteristics of four probe drugs: theophylline (major CYP1A2 substrate), fentanyl (major CYP3A4 substrate), vancomycin, and ceftazidime (renal-eliminated).
Results: The predicted maximum concentration (Cmax), area under the curve of concentration-time (AUC), and clearance (CL) for theophylline (CYP1A2 metabolism pathway) and fentanyl (CYP3A4 metabolism pathway) were within two folds of the observed data. For drugs mainly eliminated by renal clearance (vancomycin and ceftazidime) in the Chinese pediatric population, the ratio of prediction to observation for major PK parameters was within a 2-fold error range.
Conclusion: The model is a supplement to the previous Chinese population PBPK model. We anticipate the model to be a better representative of the pediatric Chinese population for drugs PK, offering greater clinical precision for medication given to the pediatric population, ultimately advancing clinical development of pediatric drugs. We can refine this model further by collecting more physiological parameters of Chinese children.
Highlights
Pediatric rational medication and drug development have received much attention these years. However, the in vivo PK data are limited in the Chinese pediatric population, which is an obstacle in clinical trials to guide dosing regimen. In this study, we developed a Chinese pediatric PBPK model to predict in vivo drug behavior in the Chinese pediatric population. We anticipate the model to assist in pediatric drug development and rational clinical medication.
Introduction
The rate of adverse drug reactions (ADR) reported in Chinese children and newborns are two and four times higher than that of adults (NMPA, 2018). One of the key reasons for the high adverse events (AE) rate is the irrational dosing regimen found in the pediatric population. On one hand, evidence-based clinical trials are the gold-standard for the optimization of dosing regimens, which are generally missing during pediatric drug development. On the other hand, dose regimens for children are usually extrapolated from adult populations based on body weight. In some cases this extrapolation cannot be applied to pediatrics because such an approach assumes that all metabolic enzymes and transporters mature relative to body weight, which is not necessarily true. Thus in 2017, the China National Medical Products Administration (NMPA) released regulatory guidelines that required evidence-based pharmacokinetic and pharmacodynamics (PK/PD) data to support pediatric drug development (NMPA, 2017). However, it is difficult to conduct dedicated clinical trials to observe children’s PK data due to ethical reasons.
The physiologically based pharmacokinetic (PBPK) model could integrate drug and physiological information and clinical trial design to make predictions on PK in special populations, including pediatrics. For example, Abduljalil et al. developed a preterm PBPK model by collecting published information on preterm developmental physiology including demographics, hematocrit, protein binding data, tissue volume, and enzyme ontogeny data (Abduljalil et al., 2019a). The model was then evaluated in compounds metabolized by CYP1A2, CYP2C9, and CYP3A4 as well as two renal-eliminated antibiotics. The model proved reliable in predicting PK behavior in Caucasian preterm neonates. Besides, Barrett et al. have also summarized PBPK modeling and its application to assist in pediatric pharmacology studies and study design (Barrett et al., 2012). Although the PBPK approach has been frequently applied to support drug development in western countries, it is rarely utilized in China. One of the main limitations of using PBPK in the Chinese pediatric population is the lack of the physiological parameters and ontogeny data of Chinese pediatric population, which may not directly adopt the Caucasian pediatric population model due to genetic differences between distinct ethnic groups. PK differences between Caucasian and Chinese children were reported by Gao et al. and Song et al. for caffeine (Gao et al., 2020) and vancomycin (Song et al., 2017). The authors concluded that these differences could be attributed to a higher drug clearance in Chinese children than in Caucasian children. The ethnic differences may come from enzyme or transporter ontogeny, they could also come from the growth chart of body size or tissue volume. However, whether there are ethnic differences between Chinese and Caucasian children still needs to be further verified by clinical studies or mechanic method such as PBPK modeling.
Therefore, the objective of this study is to develop a Chinese pediatric population model by incorporating the physiological parameters specific to the Chinese pediatric population with reference to the Caucasian population model. The new Chinese pediatric population model will be used in Simcyp Simulator to predict PK for CYP1A2 probe substrate (theophylline), CYP3A4 probe substrate (fentanyl) and renal-eliminated drugs (vancomycin and ceftazidime) in Chinese in vivo PK data. We anticipate that this model can assist in Chinese pediatric drug development and rational clinical medication.
Methods
General Workflow
Figure 1 illustrated the overall study strategy. Briefly, physiological and demographic data of Chinese children aged from 0 to 18 years old were first collected. These data were then used to recalibrate the corresponding physiological parameters in the Caucasian pediatric population model in Simcyp® (Version 20, Simcyp Division, Certara UK Limited, United Kingdom) to generate a Chinese pediatric population model. Next, a literature search for clinical PK data in the Chinese pediatric population was carried out with specific inclusion and exclusion criteria. Based on the availability of the PK data and specificity of the substrate, four probe drugs, predominantly eliminated by three different pathways (CYP1A2, CYP3A4 and renal-filtration), were selected. Drug models were then developed for these probe drugs in Simcyp and were verified using PK data from the Caucasian adults, Caucasian children, and Chinese adults. Finally, the drug models of these probe drugs, in combination with the newly developed Chinese pediatric population model, were applied to simulate PK in the Chinese pediatric population to evaluate the population model. The prediction performance of the models was evaluated by comparing the simulated maximum concentration (Cmax), clearance (CL), and area under the concentration-time curve (AUC) with the observed value.
Development of Chinese Pediatric Population Model
Physiological and Demographic Data Collection
A systematic literature search was carried out for various physiological and demographic data of Chinese children using PubMed (https://www.ncbi.nlm.nih.gov/pubmed/). Keywords used in the search included “Chinese” in all combinations with “demographic”, “height”, “weight”, “organ weight (brain, heart, kidney, liver, lung, pancreas, spleen, thyroid gland, adrenal gland)”, “cardiac output”, “body surface area (BSA)”, “yellow marrow and blood volume”, “tissue compositions (tissue weight/volume, tissue water volume, lipid weight/volume)”, “glomerular filtration rate (GFR)”, “biologic parameters (IgG, IgE, FcRn, TNF-alfa, lymph volume, lymph flow rate)”, “ontogeny (cytochrome P450, glucuronyltransferase, transporters, flavoprotein monooxygenases, carboxylesterases)”, and “skin parameters (total skin thickness, skin surface pH, stratum corneum thickness, dermis thickness, viable epidermis thickness)”. Data were included in our study if they met the following criteria: 1) the data came from Chinese children aged 0–18 years old; 2) male and female statistics were separate (in specific parameters).
Population Model Development
The Chinese pediatric population model was developed in Simcyp based on the inbuilt Northern European Caucasian (NEC) pediatric population model. The physiological and demographic data in the Chinese pediatric population collected and included in our study were used to recalibrate the corresponding parameters in the NEC pediatric population model. All the parameters were modeled against age, height, weight, or BSA using inbuilt equations of the NEC pediatric population. By integrating the equations of physiological and anthropometric parameters into the Simcyp simulator, the equations of height and weight were set as inputs in “Population-Demographic-User-defined HT and WT relationship”. Equations of organ weight were modified in “Population-Paediatric-Tissue Volume-Define Tissue Volume”. The cardiac output function was defined in “Population-Paediatric-Blood Flows-Define Blood Flow”. For the rest of the physiological parameters, because there was very limited Chinese pediatric data available, the default value of the inbuilt NEC pediatric population model was used with the assumption that they were the same between Chinese and Caucasians.
Development of Drugs Model for Probe Drugs
Clinical Pharmacokinetic Data Collection and Probe Drug Selection
The candidate probe drugs were selected based on the following considerations: a sensitive substrate of CYP1A2 or CYP3A4, namely more than 80% of the drug was metabolized and more than 70% metabolism was contributed by CYP1A2 or CYP3A4; or more than 70% of the drug was eliminated by renal filtration. A literature search was then conducted to collect PK data of candidate probe drugs in the Chinese pediatrics population at different ages using PubMed (https://www.ncbi.nlm.nih.gov/pubmed/), Embase (https://www.embase.com), and CNKI (https://www.cnki.net/). The literature search strategy was illustrated in Figure 2.
Three keywords were used in the search: “pharmacokinetics”, keywords related to different age groupings of pediatrics (e.g., “pediatrics”, “children”, “infants”, “neonates”), and the keyword related to the targeted race and region (e.g., “Chinese”, “China”). Deadline for inclusion in the literature is 2019. The related drugs were included in the probe drug candidate list.
Among the drugs with PK data included in our study, four probe drugs (i.e., theophylline, fentanyl, vancomycin, and ceftazidime) that were mainly eliminated by three respective pathways (CYP1A2 pathway, CYP3A4 pathway and renal-eliminated pathway) were selected. Theophylline is one of the substrate drugs of CYP1A2, and CYP1A2 contributed 75% of its metabolism (Wu and Peters, 2019). Theophylline metabolism was used as a probe to investigate CYP1A2 activity in vivo (Wang et al., 2013). Fentanyl is converted to a major metabolite, namely norfentanyl, in human liver by CYP3A4-mediated pathway (Feierman and Lasker, 1996). Ceftazidime and vancomycin are mainly eliminated through renal filtration, which accounted for 70–80% of their elimination (Welage et al., 1984; DrugBank). The clearance of ceftazidime and vancomycin was used to indicate the glomerular filtration function.
There were 57 studies related to these four probe drugs. If the study met the following inclusion criteria but not exclusion criteria, the PK data such as Cmax, CL, AUC, and the concentration-time profile were included in our study. Inclusion criteria were: 1) study subjects were of the Chinese pediatric population, included neonates, infants, children, and adolescents with normal cardiac, hepatic and renal functions 2) sample size was larger than three 3) dosing regimen was reported 4) the drug was administered intravenously 5) there was no comedication in the study and 6) drug concentration-time profiles or at least one of the PK parameter was reported. Exclusion criteria were: 1) participants included preterm infants 2) the age range of subjects was not reported clearly 3) dosing regimen varied from individual to individual and the mean dosage was not reported.
Seventeen studies were included according to inclusion criteria and nine studies were excluded. Four of them were excluded for meeting criteria one, two of them were excluded for criteria two, and three of them were excluded for criteria three. Finally, eight studies met our criteria and were included in our research.
Development and Verification of Drugs Model for Probe Drugs
Theophylline compound file from Simcyp V20 library was used to carry out simulations. Fentanyl, ceftazidime and vancomycin PBPK models were self-developed compound files. The physicochemical properties, as well as the drug metabolism and pharmacokinetics (DMPK) characteristics of the probe drugs that need to be self-developed, were obtained from the Drug Bank (https://www.drugbank.ca/), SciFinder (https://scifinder.cas.org), and literature. The collected data were then integrated into a PBPK model using Simcyp Simulator.
A minimal distribution model was applied in theophylline drug model due to better performance in PK prediction, compared to full PBPK model. On the other hand, the full PBPK model was used in fentanyl, ceftazidime and vancomycin. As for absorption model, the first-order absorption model was used if the drug was administrated intravenously while the advanced dissolution, absorption and metabolism (ADAM) model was used in oral administration cases. The PBPK model parameters of probe drugs were presented in Table 1.
The drug models for all four probe drugs were verified in a stepwise manner using clinical PK data from Caucasian adults, Caucasian children, and Chinese adults. The major PK parameters (Cmax, CL, AUC) and the concentration-time profile were included in drug model verification if the study met the following inclusion criteria: 1) study subjects were of the Chinese/Caucasian adult population or the Caucasian pediatric population with normal cardiac, hepatic and renal functions; 2) sample size was larger than three; 3) dosing regimen was reported; 4) there was no comedication in the study and 5) drug concentration-time profiles or at least one of the PK parameters was reported. If the ratio of predicted and observed mean values for major PK parameters was within the range of 0.5–2.0, these drug models were deemed fit to be verified.
Evaluation of Chinese Pediatric Population Models
The verified drug models of four probe drugs in combination with the newly developed Chinese pediatric population model were applied to predict drug PK in the Chinese pediatric population. The simulation was conducted by mimicking trial designs in the literature. The predicted drug concentrations vs. the observed ones were examined. Furthermore, the predicted PK parameters (i.e., Cmax, CL, and AUC) of each probe drug were compared with the observed values. If the ratio of the predicted value was within a 2 folds difference to the observed one, the population model was considered acceptable.
Results
Chinese Pediatric Population Model Development
Body height, weight, cardiac output, albumin concentration, hematocrit and organ weight (brain, heart, kidney, liver, pancreas, spleen) in the Chinese pediatric population were collected in our study but there is still a lack of information on enzyme and transporter’s ontogeny, GFR, some of the tissue volumes (lung, blood), tissue compositions, biologic parameters (i.e., IgE, FcRn, TNF-alfa, lymph volume, lymph flow rate, etc.), and skin parameters (i.e., total skin thickness, skin surface pH, etc.).
The height and weight data were collected from two pieces of literature covering Chinese children aged 0–18 years old (Janssen et al., 2007; Zong and Li, 2013). The cardiac output data were collected from children aged from 1 to 12 years old (Cattermole et al., 2017). All the organ weight data were obtained from children aged from 0 to 15 years old (Wang et al., 1995a; Kawamura, 1998). These data were then used to develop the Chinese pediatric population model by recalibrating the equations for describing the change of these physiological characteristics with age in the NEC pediatric population model. As shown in Table 2, the regression curves fit the observation data well with regression coefficient (R2) > 0.98. Table 3 showed some anthropometry data (height, weight, BMI, and heart rate), blood biochemistry indexes (serum creatinine concentration and albumin concentration) and hematocrit in Chinese children from national physique and health database. They were used to verify the performance of Chinese pediatric model in predicting these parameters.
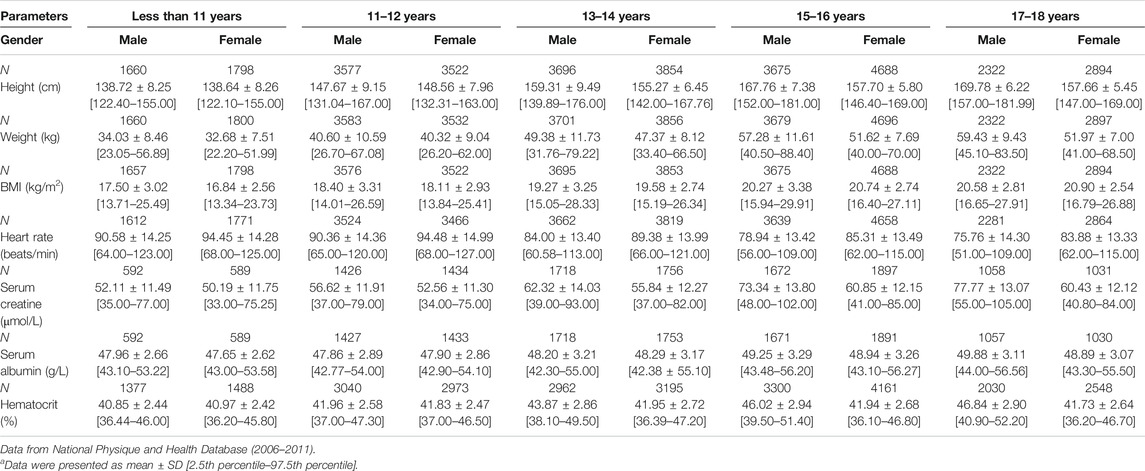
TABLE 3. Summary of anthropometry data and hematological indexes in Chinese pediatric populationa.
To evaluate the capability of the developed pediatric population model to describe the ontogeny of height, weight, cardiac output and organ size of Chinese children, a simulation of 4000 virtual Chinese pediatric population was performed using Simcyp simulator (proportion of females = 0.5; 200 subjects in each trial). As shown in Figure 3, most of the simulated height, weight, and cardiac output in Chinese children were within the 2.5th and the 97.5th percentile of observation for both male and female, and the organ weights were evenly distributed around the observed mean value, indicating that the newly developed Chinese pediatric population model can describe the ontogeny of body size, cardiac output and certain tissue volume of Chinese children.
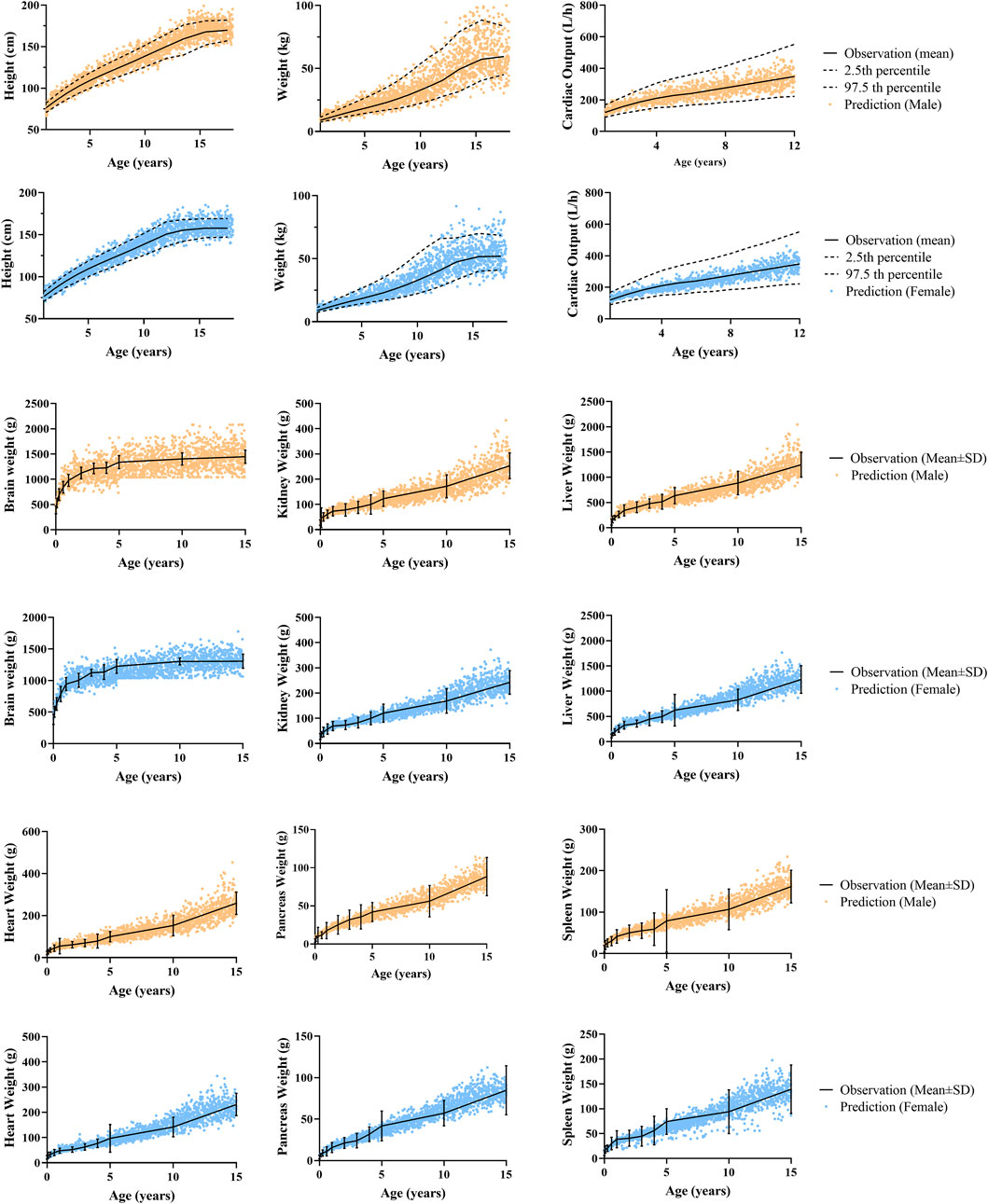
FIGURE 3. Predicted and observed physiological data in male and female. Anthropometric data (height, weight) and cardiac output data aged from 1 to 12 years old were reported by Cattermole et al. (2017), and anthropometric data aged from 13 to 18 years old were from the National Physique and Health Database (http://cnphd.bmicc.cn/chs/en/index.php). Organ weight data aged from 0 to 15 years old were reported by Wang at al. (1995a).
Probe Drugs Physiologically Based Pharmacokinetic Model Development and Verification
The drug model for all four probe drugs was verified with PK data of Caucasian adults, the Caucasian pediatric population, and the Chinese adult population from various studies. As shown in Supplementary Table S2 and Supplementary Figure S1, 90.48% of the predicted PK parameters of theophylline drug model were within the 2-fold error range, and 57.14% of them were within the 1.25 fold error range. The corresponding values of fentanyl, ceftazidime and vancomycin drug models were 84.85% and 30.30%, 92.86% and 78.57%, and 92.31% and 61.54%, respectively. In conclusion, over 89.47% of the prediction to observation (P/O) ratios were between 0.5 and 2.0 in all the established probe drug models, indicating the consistency and robustness of our probe drug model. For drug concentration-time profiles, most of the observed plasma concentrations were within the 5th to the 95th percentile of prediction (Supplementary Figure S2).
Chinese Pediatric Population Model Verification
The verified drug model of these four probe drugs in combination with the developed Chinese pediatric population model was applied to predict drug concentration in the Chinese pediatric population. As shown in Table 4 and Figure 4A, for theophylline (major CYP1A2 substrate) and fentanyl (major CYP3A4 substrate), ratios of predicted vs. observed PK parameters (Cmax, CL, AUC) from various studies were within the range of 0.5–2.0. For ceftazidime and vancomycin, which were eliminated via renal elimination pathway, the ratio of predicted vs. observed CL from the single study of ceftazidime was 0.88, and the ratio of vacomycin was 1.12 and 0.65. The ratio of predicted vs. observed AUC for vancomycin was 1.76 and 1.17, respectively. No Cmax was reported for both drugs. Figure 4B also illustrated the observed and predicted concentration-time profile for theophylline and ceftazidime. Compared to the concentration-time profile predicted in NEC pediatric model, the mean concentration decreased slightly in Chinese pediatric model and most of the observed plasma concentrations were within the 5th and 95th percentile of prediction. The concentration-time profiles of fentanyl and vancomycin were not reported in children with normal cardiac, hepatic and renal function.
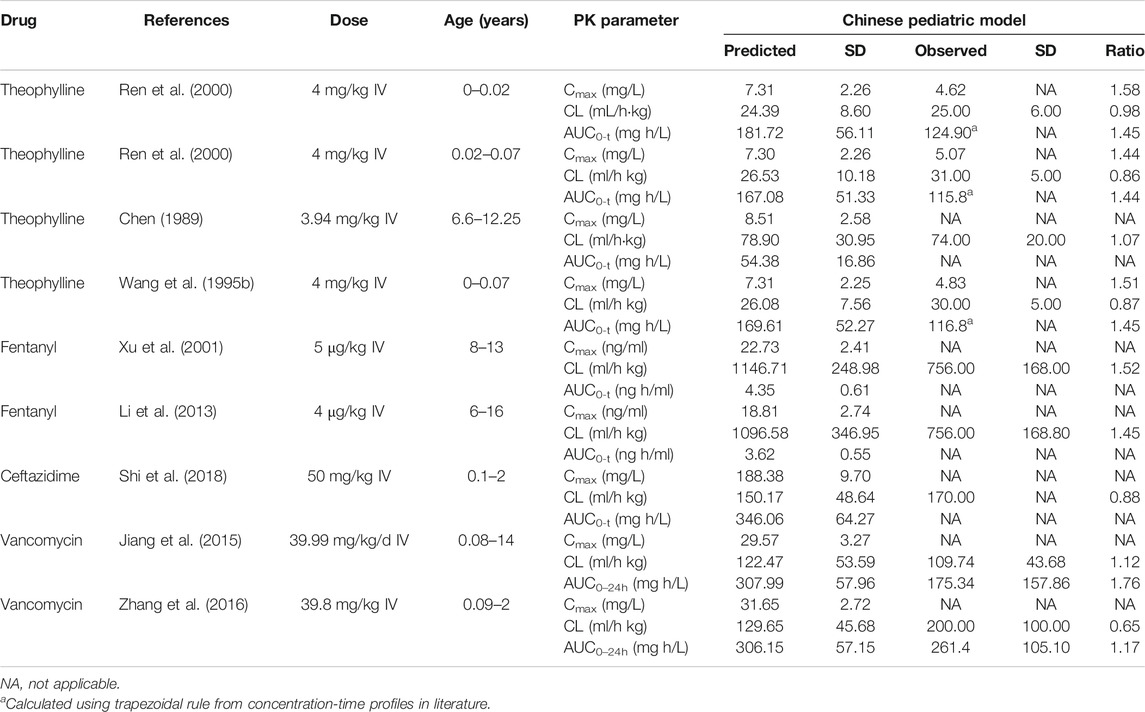
TABLE 4. PK parameters of probe drugs predicted by CN pediatric model in Chinese pediatric population.
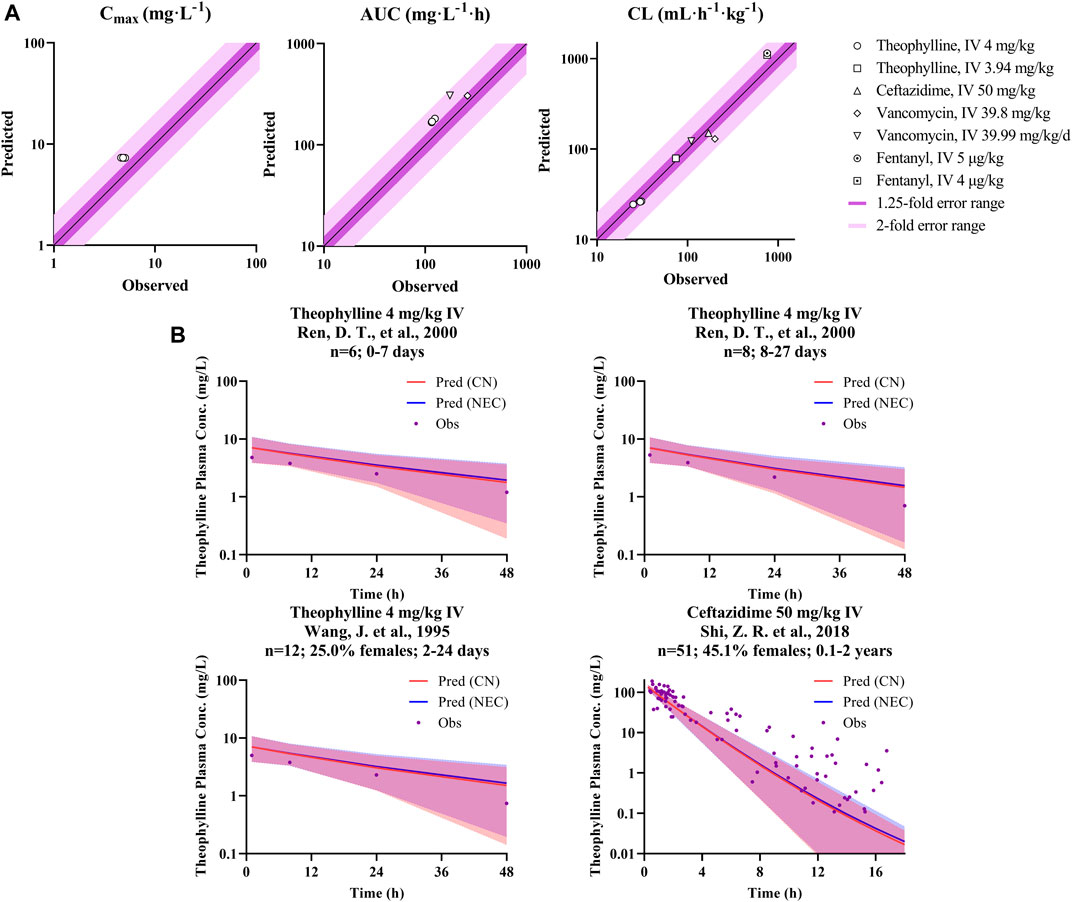
FIGURE 4. P/O ratio of PK parameters and drug concentration-time profiles. (A) The observed PK parameter (Cmax, CL, and AUC) vs. predicted values of probe drugs using developed Chinese pediatric population model. (B) The observed and predicted concentration data of probe drugs using developed Chinese pediatric population model. (Dots represents observed data; blue line represents predicted data based on Caucasian pediatric model; red line represents predicted data based on Chinese pediatric model; and blue/red shadow represents 90% prediction interval of the corresponding model, purple shadow is their overlapped parts).
Discussion
In the past decades, the PBPK model has been applied to predict pediatric drug concentrations during drug development to support dosing rationalization in the pediatric clinical trial. For example, the PBPK model was used to set a starting dose for Eribulin in children and adolescents aged 6–18 years old (Shebley et al., 2018). However, majority of its application is in Caucasian pediatrics with very limited examples to apply the PBPK model in other ethnic groups. Recently, Kim et al. developed a PBPK model for the Korean pediatric population in Simcyp. The model was verified using six substrate drugs of five major metabolic enzymes and two transporters. The model was proved useful in predicting the concentration-time profiles of these drugs (Kim et al., 2019). In our study, a similar approach was adopted to develop the Chinese pediatric population model by incorporating Chinese pediatric demographic and physiological information covering a span of children 0–15 years old. Our study, with limited examples, showed that the developed pediatric population model can achieve reasonable predictions on drug exposure in the Chinese pediatric population. This may serve as the first step in developing a more mature Chinese pediatric population model in the future.
In our study, depending on the availability of the Chinese pediatric PK data, four probe drugs that were eliminated either by CYP1A2 metabolism (theophylline), CYP3A4 metabolism (fentanyl) or by renal filtration (ceftazidime and vancomycin) were selected to evaluate the prediction performance of this pediatric population model. It should be noted that CYP1A2 and CYP3A4 are the major P450 enzyme in eliminating several drugs in clinical (Faber et al., 2005) Choonara and Sammons (2014) such as caffeine and midazolam, which are also used in pediatrics. Antibiotics are commonly used in pediatrics and many of them are eliminated by glomerular filtration. Thus, the probe drugs selected in our study can generally also represent pediatric medication eliminated by renal-filtration, CYP1A2 and CYP3A4 metabolism pathway. The probe drug models were verified in Caucasian adult population, Caucasian pediatric population, and Chinese adult population models. The accuracy of some certain simulations reduced due to the character of participants like pathological status, smoking history, or preterm birth.
Our population model was developed based on the inbuilt NEC pediatric population model in Simcyp, with height, weight, cardiac output, and tissue volumes (brain volume, heart volume, kidney volume, liver volume, pancreas volume, and spleen volume) recalibrated with Chinese pediatrics data. To confirm whether there was an ethnic difference between Chinese children and Caucasian children, we performed the same simulation using the NEC pediatric population model. However, the Chinese pediatric model showed no significant improvement in predicting the CL of CYP3A4-metabolized and renal-eliminated drugs, as shown in Figures 5A,B. This may be a system deviation resulted from the limited PK data. Figure 5C showed that the Chinese pediatric population model had a better estimation than the NEC pediatric model in CL prediction, after modification.
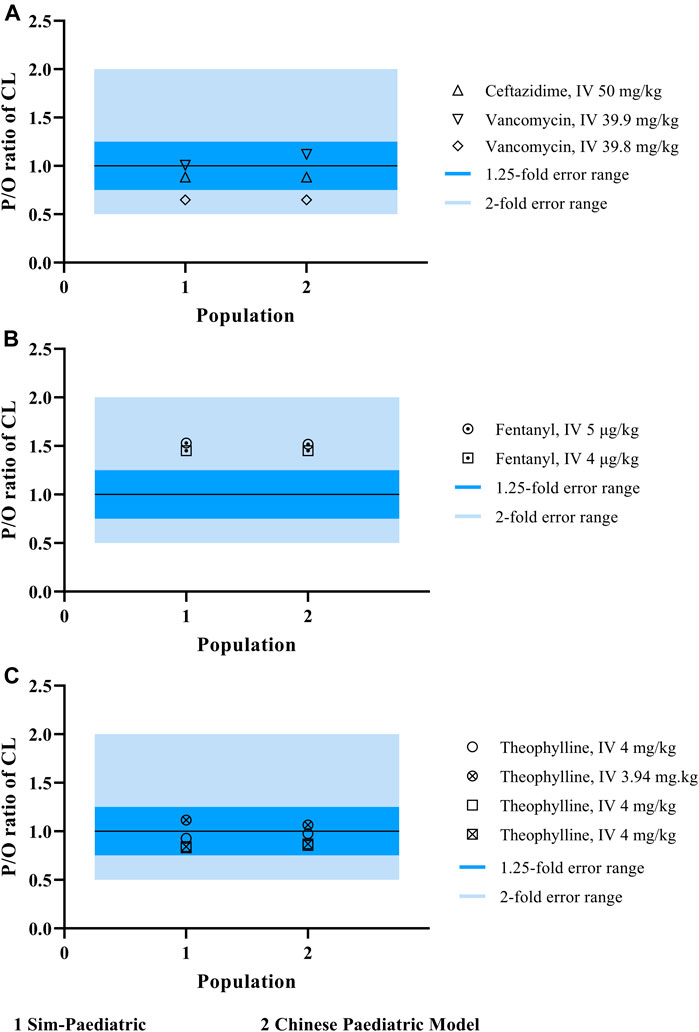
FIGURE 5. The P/O ratio of CL simulated by both NEC pediatric population model and Chinese pediatric population model.
Although great efforts have been made to search for physiological data extensively in Chinese pediatric patients, there is still a lack of information on many physiological parameters such as body surface area (BSA), glomerular filtration rate (GFR), and especially on the ontogeny of a cytochrome P450 enzyme. Microsomal protein per gram of liver (MPPGL) and CYP enzyme ontogeny is critical in order to correctly predict the exposure of drug with metabolism as the major elimination pathway. Hence in our study, we assumed the Chinese pediatric population shares the same CYP1A2 and CYP3A4 ontogeny as the Caucasian population, which may not necessarily true. More clinical trials need to be carried out in order to prove our assumption. In addition, all the PK data of the Chinese pediatric population were from patients. Pathological factors would influence the accuracy of a prediction.
Conclusion
In this study, a preliminarily physiologically based pharmacokinetic model based on Chinese pediatric physiological characteristics was developed. The model was verified in the Chinese pediatric population by predicting PK behavior of theophylline, fentanyl, ceftazidime, and vancomycin which are eliminated either through CYP1A2, CYP3A4 and renal pathway. In comparison of the observed PK data, our model successfully predicted the PK profile of these drugs within a 2-fold error range. However, it warrants further study to improve this population model.
Data Availability Statement
The original contributions presented in the study are included in the article/Supplementary Material, further inquiries can be directed to the corresponding author.
Author Contributions
DL conceived the idea and designed the study. HL supervised the study. XY developed the modeling strategies. XL, ST, and ZL collected Chinese childrens’ physiological data and drug pharmacokinetic data. XL, XL, and ZH performed the modeling and simulations. CC and ZY contributed to data analyses. XY and XL wrote and finalized the manuscript. ZD and FS addressed the comments and contributed an improvement to the manuscript.
Funding
This work was supported by the Bill and Melinda Gates Foundation (No. OPP1204780), and “13th Five-Year” National Major New Drug Projects of China, Ministry of Science and Technology of the People’s Republic of China (No. 2017ZX09101001-002-001).
Acknowledgments
We would like to sincerely thank the contributions of the interns at Peking University Third Hospital.
Conflict of Interest
Author FS was employed by the company Certara UK Limited. Author ZD was employed by company Janssen China R&D Center.
The remaining authors declare that the research was conducted in the absence of any commercial or financial relationships that could be construed as a potential conflict of interest.
Supplementary Material
The Supplementary Material for this article can be found online at: https://www.frontiersin.org/articles/10.3389/fphar.2021.648697/full#supplementary-material.
References
Abduljalil, K., Pan, X., Pansari, A., Jamei, M., and Johnson, T. N. (2019a). A preterm physiologically based pharmacokinetic model. Part I: physiological parameters and model building. Clin. Pharmacokinet. 59, 485–500. doi:10.1007/s40262-019-00825-6
Abduljalil, K., Pan, X., Pansari, A., Jamei, M., and Johnson, T. N. (2019b). Preterm physiologically based pharmacokinetic model. Part II: applications of the model to predict drug pharmacokinetics in the preterm population. Clin. Pharmacokinet. 59, 501–518. doi:10.1007/s40262-019-00827-4
Barrett, J. S., Della Casa Alberighi, O., Läer, S., and Meibohm, B. (2012). Physiologically based pharmacokinetic (PBPK) modeling in children. Clin. Pharmacol. Ther. 92 (1), 40–49. doi:10.1038/clpt.2012.64
Bjorkman, S. (2005). Prediction of drug disposition in infants and children by means of physiologically based pharmacokinetic (PBPK) modelling: theophylline and midazolam as model drugs. Br. J. Clin. Pharmacol. 59 (6), 691–704. doi:10.1111/j.1365-2125.2004.02225.x
Cattermole, G. N., Leung, P. Y., Ho, G. Y., Lau, P. W., Chan, C. P., Chan, S. S., et al. (2017). The normal ranges of cardiovascular parameters measured using the ultrasonic cardiac output monitor. Physiol. Rep. 5 (6), e13195. doi:10.14814/phy2.13195
Chen, Z. L. (1989). The pharmacokinetics of theophylline and its clinical application in asthmatic children. Chin. J. Clin. Pharmacol., 97–101. doi:10.13699/j.cnki.1001-6821.1989.02.006
Choonara, I., and Sammons, H (2014). Paediatric clinical pharmacology in the UK. Arch Dis Child 99, 1143–1146. doi:10.1136/archdischild-2014-306853 Available at: https://inis.iaea.org/search/search.aspx?orig_q=RN:29028112
Cui, C., Li, X., Liang, H., Hou, Z., Tu, S., Dong, Z., et al. (2020a). Physiologically based pharmacokinetic model of renally cleared antibacterial drugs in Chinese renal impairment patients. Biopharm. Drug Dispos. 42, 24–34. doi:10.1002/bdd.2258
Cui, C., Sia Jie En, V., Tu, S., Li, X., Dong, Z., Yu, Z., et al. (2020b). Development of a physiologically based pharmacokinetic (PBPK) population model for Chinese elderly subjects. Br. J. Clin. Pharmacol. doi:10.1111/bcp.14609
DrugBank. Vancomycin. Available at: https://www.drugbank.ca/drugs/DB00512.
Encinas, E., Calvo, R., Lukas, J. C., Vozmediano, V., Rodriguez, M., and Suarez, E. (2013). A predictive pharmacokinetic/pharmacodynamic model of fentanyl for analgesia/sedation in neonates based on a semi-physiologic approach. Paediatr. Drugs 15 (3), 247–257. doi:10.1007/s40272-013-0029-1
Faber, M. S., Jetter, A., and Fuhr, U. (2005). Assessment of CYP1A2 activity in clinical practice: why, how, and when?. Basic Clin. Pharmacol. Toxicol. 97 (3), 125–134. doi:10.1111/j.1742-7843.2005.pto_973160.x
Feierman, D. E., and Lasker, J. M. (1996). Metabolism of fentanyl, a synthetic opioid analgesic, by human liver microsomes. Role of CYP3A4. Drug Metab. Disposition 24 (9), 932–939. PMID: 8886601
Gao, X-B., Zheng, Y., Yang, F., Wang, C-H., Jiang, Z-H., Wu, Y-E., et al. (2020). Developmental population pharmacokinetics of caffeine in Chinese premature infants with apnoea of prematurity: a post-marketing study to support paediatric labelling in China. Br. J. Clin. Pharmacol. 87, 1155–1164. doi:10.1111/bcp.14483
Janssen, P. A., Thiessen, P., Klein, M. C., Whitfield, M. F., Macnab, Y. C., and Cullis-Kuhl, S. C. (2007). Standards for the measurement of birth weight, length and head circumference at term in neonates of European, Chinese and South Asian ancestry. Open Med. 1 (2), e74–e88. PMID: 20101298
Jantos, R., Schuhmacher, M., Veldstra, J. L., Bosker, W. M., Klöpping-Ketelaars, I., Touliou, K., et al. (2011). [Determination of blood/serum ratios of different forensically relevant analytes in authentic samples]. Arch. Kriminol 227 (5-6), 188–203.
Ji, B., Liu, S., Xue, Y., He, X., Man, V. H., Xie, X-Q., et al. (2019). Prediction of drug–drug interactions between opioids and overdosed benzodiazepines using physiologically based pharmacokinetic (PBPK) modeling and simulation. Drugs in R&D 19 (3), 297–305. doi:10.1007/s40268-019-00282-3
Jiang, Y. L., Chen, M. L., and Li, B. R. (2015). Serum concentration monitoring and pharmacokinetic characters of vancomycin in child patients from pediatric intensive care unit. Chin. J. New Drugs Clin. Rem 34 (02), 133–136. doi:10.14109/j.cnki.xyylc.2015.02.012
Kawamura, H. (1998). Organ mass measurements. Int. At. Energ. Agency (Iaea), 27–55. Contract No.: IAEA-TECDOC--1005(v1) Available at: https://inis.iaea.org/search/search.aspx?orig_q=RN:29028112.
Kim, Y., Hatley, O., Rhee, S-j., Yi, S., Lee, H. A., Yoon, S., et al. (2019). Development of a Korean-specific virtual population for physiologically based pharmacokinetic modelling and simulation. Biopharm. Drug Dispos. 40 (3-4), 135–150. doi:10.1002/bdd.2178
Labroo, R. B., Paine, M. F., Thummel, K. E., and Kharasch, E. D. (1997). Fentanyl metabolism by human hepatic and intestinal cytochrome P450 3A4: implications for interindividual variability in disposition, efficacy, and drug interactions. Drug Metab. Dispos. 25 (9), 1072–1080. PMID: 9311623
Li, J., Xu, d., and Gao, X. (2013). Comparative study of the pharmacokinetics of fentanyl citrate spinal anesthesia in patients with different ages. China Health Care Nutr., 548–549. doi:10.3969/j.issn.1004-7484(x).2013.02.029
NMPA. Guideline for extrapolation of adult medication data to pediatric populations. Available at: http://www.cde.org.cn/zdyz.do?method=largePage&id=9efb053e8786b209. (2017).
NMPA (2018). National annual report on adverse drug reaction monitoring (2017). Chin. J. Drug Eval. 35 (02), 154–160.
Ogilvie, R. I. (1978). Clinical pharmacokinetics of theophylline. Clin. Pharmacokinet. 3 (4), 267–293. doi:10.2165/00003088-197803040-00002
Ren, D. T., Wang, Y., Shen, J., and Wang, J. (2000). Study on pharmacokinetics of theophylline in different groups of infants. Chin. Pharm. J. 35 (6), 399–400.
Shebley, M., Sandhu, P., Emami Riedmaier, A., Jamei, M., Narayanan, R., Patel, A., et al. (2018). Physiologically based pharmacokinetic model qualification and reporting procedures for regulatory submissions: a consortium perspective. Clin. Pharmacol. Ther. 104 (1), 88–110. doi:10.1002/cpt.1013
Shi, Z. R., Chen, X. K., Tian, L. Y., Wang, Y. K., Zhang, G. Y., Dong, L., et al. (2018). Population pharmacokinetics and dosing optimization of ceftazidime in infants. Antimicrob. Agents Chemother. 62 (4), e02486-17. doi:10.1128/aac.02486-17
Song, L., He, C-Y., Yin, N-G., Liu, F., Jia, Y-T., and Liu, Y. (2017). A population pharmacokinetic model for individualised dosage regimens of vancomycin in Chinese neonates and young infants. Oncotarget 8 (62), 105211–105221. doi:10.18632/oncotarget.22114
Wang, J., Li, B., Chen, R., Zhao, Y., Xu, Y., Gao, Z., et al. (1995a). Reference values of main internal organs for Chinese. Chin. J. Radiol. Med. Prot. 15 (4), 248–254.
Wang, J., Ren, D. T., Shen, J., and Liang, W. Q. (1995b). Study on pharmacokinetics of theophylline on neonates. Chin. J. Hosp. Pharm. (12), 534–536.
Wang, L., Hu, Z., Deng, X., Wang, Y., Zhang, Z., and Cheng, Z. N. (2013). Association between common CYP1A2 polymorphisms and theophylline metabolism in non-smoking healthy volunteers. Basic Clin. Pharmacol. Toxicol. 112 (4), 257–263. doi:10.1111/bcpt.12038
Welage, L. S., Schultz, R. W., and Schentag, J. J. Pharmacokinetics of ceftazidime in patients with renal insufficiency. Antimicrob. Agents Chemother. (1984) 25(2):201–204. doi:10.1128/aac.25.2.201
Wu, Q., and Peters, S. A. (2019). A retrospective evaluation of allometry, population pharmacokinetics, and physiologically‐based pharmacokinetics for pediatric dosing using clearance as a surrogate. CPT: Pharmacometrics Syst. Pharmacol. 8 (4), 220–229. doi:10.1002/psp4.12385
Xu, K., Yue, J., Chen, S., Guo, P., and Yang, Z. (2001). Pharmacokinetics of fentanyl in patients of different ages undergoing non-cardiac surgery. Chin. J. Anes Thesiol 21 (1). 18–20.
Zhang, H., Wang, Y., Gao, P., Hu, J., Chen, Y., Zhang, L., et al. (2016). Pharmacokinetic characteristics and clinical outcomes of vancomycin in young children with various degrees of renal function. J. Clin. Pharmacol. 56 (6), 740–748. doi:10.1002/jcph.653
Keywords: PBPK, Chinese pediatric population, CYP1A2, CYP3A4, renal elimination, pharmacokinetics
Citation: Yao X, Liu X, Tu S, Li X, Lei Z, Hou Z, Yu Z, Cui C, Dong Z, Salem F, Li H and Liu D (2021) Development of a Virtual Chinese Pediatric Population Physiological Model Targeting Specific Metabolism and Kidney Elimination Pathways. Front. Pharmacol. 12:648697. doi: 10.3389/fphar.2021.648697
Received: 01 January 2021; Accepted: 23 March 2021;
Published: 11 May 2021.
Edited by:
Karel Allegaert, University Hospitals Leuven, BelgiumCopyright © 2021 Yao, Liu, Tu, Li, Lei, Hou, Yu, Cui, Dong, Salem, Li and Liu. This is an open-access article distributed under the terms of the Creative Commons Attribution License (CC BY). The use, distribution or reproduction in other forums is permitted, provided the original author(s) and the copyright owner(s) are credited and that the original publication in this journal is cited, in accordance with accepted academic practice. No use, distribution or reproduction is permitted which does not comply with these terms.
*Correspondence: Dongyang Liu, bGl1ZG9uZ3lhbmdAdmlwLnNpbmEuY29t
†These authors share first authorship