- 1Affiliated Hospital of Nanjing University of Chinese Medicine, Nanjing, China
- 2Department of Respiratory Medicine, Jiangsu Province Hospital of Chinese Medicine, Nanjing, China
- 3Arizona Metabolomics Laboratory, College of Health Solutions, Arizona State University, Scottsdale, AZ, United States
(−)-Epigallocatechin-3-gallate (EGCG) is the main bioactive catechin in green tea. The antitumor activity of EGCG has been confirmed in various types of cancer, including lung cancer. However, the precise underlying mechanisms are still largely unclear. In the present study, we investigated the metabolite changes in A549 cells induced by EGCG in vitro utilizing liquid chromatography-mass spectrometry (LC-MS)-based metabolomics. The result revealed 33 differentially expressed metabolites between untreated and 80 μM EGCG-treated A549 cells. The altered metabolites were involved in the metabolism of glucose, amino acid, nucleotide, glutathione, and vitamin. Two markedly altered pathways, including glycine, serine and threonine metabolism and alanine, aspartate and glutamate metabolism, were identified by MetaboAnalyst 5.0 metabolic pathway analysis. These results may provide potential clues for the intramolecular mechanisms of EGCG’s effect on A549 cells. Our study may contribute to future molecular mechanistic studies of EGCG and the therapeutic application of EGCG in cancer management.
Introduction
As one of the most common cancers globally, lung cancer causes a severe social burden (Ferlay et al., 2015; Siegel et al., 2016). Worldwide, an estimated 2.2 million new lung cancer cases occurred in 2020 (Sung et al., 2021). As the second most commonly diagnosed cancer, lung cancer remained the leading cause of cancer death, with an estimated 1.8 million deaths in 2020 (Sung et al., 2021). Despite significant advances that have been made in the interventions, including surgery, radiation therapy, chemotherapy, targeted therapy, and immunotherapy on lung cancer, the 5 years survival of lung cancer only remains 21% (Miller et al., 2019; Siegel et al., 2021). It is critical to consider other preventive and therapeutic measures for lung cancer not only to decrease its incidence and mortality but also to overcome the toxicity, side effects, and cost of existing treatments (Hirsch et al., 2017).
Epigallocatechin-3-gallate (EGCG) is the most abundant and effective catechin in numerous types of white tea and green tea (Sano et al., 2001; Tang et al., 2019). It has been shown that EGCG can inhibit tumor growth and stimulate cancer cell apoptosis in various human cancers in vivo and vitro studies (Huh et al., 2004; Chen et al., 2016; Yang et al., 2016; Huang et al., 2017; Liu et al., 2017; Borutinskaite et al., 2018; Gan et al., 2018; Zhou et al., 2018; Wei et al., 2019; Wu et al., 2019; Yoshimura et al., 2019; Almatroodi et al., 2020; Panji et al., 2021; Romano and Martel, 2021). Many studies have demonstrated that EGCG may take a role in the initiation, promotion, and progression of cancer through the modulation of various mechanisms, including cellular proliferation, differentiation, apoptosis, angiogenesis, and metastasis, which leads to its anticarcinogenic activities (Chen and Dou, 2008; Ma et al., 2013; Luo et al., 2014; Luo et al., 2017; Moradzadeh et al., 2018; Pal et al., 2018; Li et al., 2019; Almatroodi et al., 2020; Yin et al., 2021). Increasing evidence has shown that EGCG possesses anti-tumorigenic property against non-small cell lung cancer (Sonoda et al., 2014; Shi et al., 2015; Gu et al., 2018; Hu et al., 2019). Even though the antitumor activity of EGCG in lung cancer has been extensively investigated, the underlying mechanism remains unclear.
Metabolomics is an exciting tool to detect small metabolic compounds and monitor small global molecule endogenous metabolite changes induced by biochemical reactions in biological systems (Dunn et al., 2011; Griffin et al., 2011; Reaves and Rabinowitz, 2011; Gu et al., 2012; Carroll et al., 2015; Zampieri et al., 2017; McCartney et al., 2018; Yan and Xu, 2018; Yeung, 2018; Shi et al., 2019; Eghlimi et al., 2020; He et al., 2020; Lim et al., 2020; Wei et al., 2021). Metabolomics is a promising approach to searching potential biomarkers and novel therapeutic strategies for lung cancer (Luengo et al., 2017; Seijo et al., 2019; Noreldeen et al., 2020; Schmidt et al., 2021). To date, the precise molecular mechanisms of antitumor activity in lung cancer induced by EGCG still keep unclear and need more investigation, especially from a metabolism point of view. Therefore, in this study, we applied liquid chromatography-mass spectrometry (LC-MS) based metabolomics and employed A549 cells as an in vitro model to further explore the effect of EGCG on lung cancer cell metabolism.
Materials and Methods
Reagents and Materials
EGCG was purchased from Sigma-Aldrich (St. Louis, MO, United States). A549 cell line (ATCC NO. CCL-185) was purchased from American Type Culture Collection (ATCC, Manassas, VA, United States). The Cell Counting Kit-8 (CK04-05) was obtained from Dojindo Molecular Technologies (Gaithersburg, MD, United States). 4′,6-diamindino-2-phenylinodole (DAPI) staining kit was purchased from FcmacsBiotechCo., Ltd. (Jiangsu, China). BCA™ Protein Assay Kit was obtained from Thermo Scientific (Waltham, MA, 84 United States). LC-MS-grade isopropanol (IPA), acetonitrile (ACN), MeOH, and CH2Cl2 were purchased from Fisher Scientific (Pittsburgh, PA). HPLC grade acetic acid, U-13C glucose, N-tert-butyldimethylsilyl-N-methyltrifluoroacetamide (MTBSTFA), methoxyamine hydrochloride, ammonia acetate, anhydrous pyridine, and dimethyl sulfoxide (DMSO) and all of the standard compounds used for metabolic identification were acquired from Sigma-Aldrich (St. Louis, MO, United States).
Cell Culture
The A549 cells were cultured in Dulbecco’s modified eagle medium (DMEM) (Corning, 10-013-CV) supplemented with 10% fetal bovine serum (FBS) (Corning, 35-010-CV). Cells were grown at 37°C and 5% CO2 in a humidified atmosphere.
Cell Viability Assay
Cells were seeded in 96-well plates (8.0 × 103 per well) and treated with different concentrations of EGCG (20, 40, 60, 80, 100, 120, 160, 200 μM) for 24 h. After treatment, the viability of A549 cells was measured via the Cell Counting Kit-8 assay. Briefly, 10 μl of CCK-8 reagents were inserted into each well before incubation in an incubator with 5% CO2 at 37°C for 3 h. Subsequently, absorbance at 450 nm was measured using a microplate reader (Molecular Devices, CA, United States). Viability is expressed as a cell activity percentage between the EGCG group and the control group.
LC-MS Metabolomics Analysis
In this study, we utilized a pathway-specific LC-MS method that can cover more than 300 metabolites from >35 metabolic pathways (Carroll et al., 2015; Gu et al., 2015; Sperber et al., 2015; Li et al., 2018; Jasbi et al., 2019; Liu et al., 2019; Shi et al., 2019). Briefly, A549 cells were seeded in 6-well plates (4.5× 105 cells/well) with 10% FBS supplemented with DMEM. Then, cells were incubated overnight in an incubator with 5% CO2 at 37°C. The cells were then treated with EGCG for 24 h. For sample preparation, the cells were first rinsed with PBS. Then 1.2 ml of 80% MeOH was added into each well for extraction of intracellular metabolites. Samples were completely lysed using an ultrasonic homogenizer in an ice bath for 20 min and centrifuged at 14,000 rpm under 4°C for 10 min. Following that, 500 μl of each supernatant was retained and dried under vacuum for 4 h. The dried samples were reconstituted using 150 μl of solvent (PBS: ACN = 4:6) and then centrifuged at 14,000 rpm under 4°C for 10 min. Sets of samples of identical volume were combined for quality-control specimens solvent (B) to assess instrument performance.
The supernatants were analyzed by liquid chromatography-mass spectrometry (LC-MS) simultaneously after centrifugation. 100 μl of the supernatant was transferred to a new vial and analyzed by an Agilent 1290 LC-6490 Triple Quadrupole mass spectrometer system equipped with an electrospray ionization (ESI) source. LC was performed on a Waters XBridge BEH Amide column (150 × 2.1 mm, 2.5 µm particle size, Waters Corporation, Milford, MA). The mobile phase for chromatographic separation was composed of solvent (A): 10 mM ammonium hydroxide, 10 mM ammonium acetate in 95% H2O/5% ACN, and solvent (B): 10 mM ammonium hydroxide, 10 mM ammonium acetate in 95% ACN/5% H2O. Following a 1 min isocratic elution of 90% solvent (B), solvent (B) was gradually reduced to 40% in 10 min (t = 11 min) and then kept at 40% for 4 min (t = 15 min). Subsequently, solvent (B) was returned to 90% to run the next sample. Each sample was injected twice, 4 μl for positive ion electrospray ionization analysis and 10 μl for negative ion analysis. Multiple reaction monitoring (MRM) mode was employed for targeted data acquisition.
The QQQ-MS system was operated with a capillary voltage of 3.5 kV. The nebulizer gas (N2) pressure was set at 30 psi with a drying gas (N2) flow rate of 15 L/min, and the temperature was 175°C. The flow rate of sheath gas (N2) was set to 11 L/min with a temperature of 225°C. A CE range of 5–50 V in increments of 5 V, and 4 CAV values (2 V, 4 V, 6 V, 8 V) were evaluated for MRM optimization; optimized CE and CAV values were determined from the highest MRM response.
The software programs used to control the LC-MS system and integrate extracted MRM peaks were Agilent MassHunter Workstation and Agilent MassHunter Quantitative Data Analysis, respectively. Protein concentrations in each sample were utilized to normalize metabolite levels.
The post-preparative stability of the sample was tested by running five prepared quality control (QC) samples kept in an autosampler (maintained at 4°C). In addition, one QC sample was inserted every 3–4 test samples during the whole process to validate system suitability and stability.
Statistical Analysis
Measurement data are the mean ± standard deviation (SD) and analyzed via the Student’s two-tailed t-test or one-way analysis of variance (ANOVA) with Tukey’s post hoc analysis, and p < 0.05 was considered as a significant difference.
Principal component analysis (PCA), partial least squares discriminant analysis (PLS-DA), pathway analysis overview, and heatmap clustering of altered metabolic profiling analysis were performed using MetaboAnalyst 5.0 (https://www.metaboanalyst.ca/). In pathway analysis, “Homo sapiens (KEGG)” library was selected, as well as Hypergeometric test for pathway enrichment analysis and relative betweenness centrality for pathway topology analysis.
Results
EGCG Suppressors Cell Viability of A549 Cells
After exposure to EGCG for 24 h, the A549 cell viability was downregulated in a dose-dependent manner within the concentration range of 60–100 μM (Figure 1). Cell viability was 92.10 ± 3.23% at 40 μM (p < 0.05) and reduced to 60.01 ± 4.02% at 80 μM (p < 0.005). As shown in Supplementary Figure S1, abnormal nucleus margin and shortening of nucleus diameter occurred at EGCG exposure groups, especially at the concentrations of 100 μM. Considering the balance between cell viability and data interpretability, a concentration of 40 μM was chosen for further experiments. The concentration of 80 μM was also selected in the subsequent metabolomics experiments to help capture more responses on cell metabolism related to the effect of EGCG.
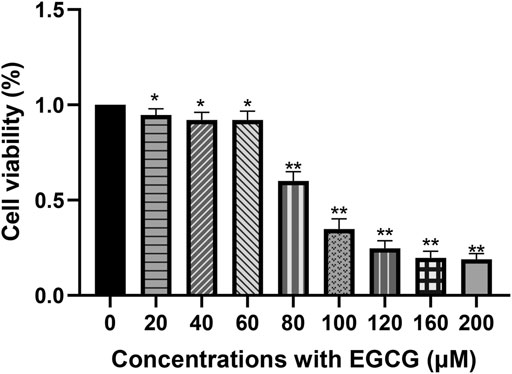
FIGURE 1. Cell viability of A549 cells after EGCG exposure. A549 cells were exposed to 20–200 μM EGCG for 24 h, and cell viability was determined utilizing a CCK-8 assay. The experimental data are expressed as the mean value with SD of three independent replicates. (*p < 0.05, **p < 0.005).
LC-MS Metabolite Profiling of A549 Cells After EGCG Treated
LC-MS of Metabolic Profiles
In total, we found that 173 metabolites were reliably detected with relative abundances >1,000 in more than 80% of all samples. After normalization by averaged values from the QC injection data, 142 metabolites had a coefficient of variation (CV) value of <30%. We analyzed the metabolic profiles of these 142 metabolites of 40 μM, 80 μM EGCG-treated and untreated A549 cells. Based on the LC-MS data, the PCA score plot of metabolites showed an obvious separation among the control group, 40 μM EGCG-treated group, and 80 μM EGCG-treated group (Figure 2A). No outlier detection was performed from the data overview. PLS-DA was further undertaken to reveal the metabolic deviations between the EGCG-treated groups and the control group. As shown in Figure 2B, the metabolite profiles of the three groups were distributed in significantly separated clusters. Although it is a supervised classification method, component 1 and component 2 in the PLS-DA model (Figure 2B) accounted for 23 and 22.9% of the total variance in the data respectively, which indicated that significant metabolic disturbances were induced in A549 cells treated by EGCG.
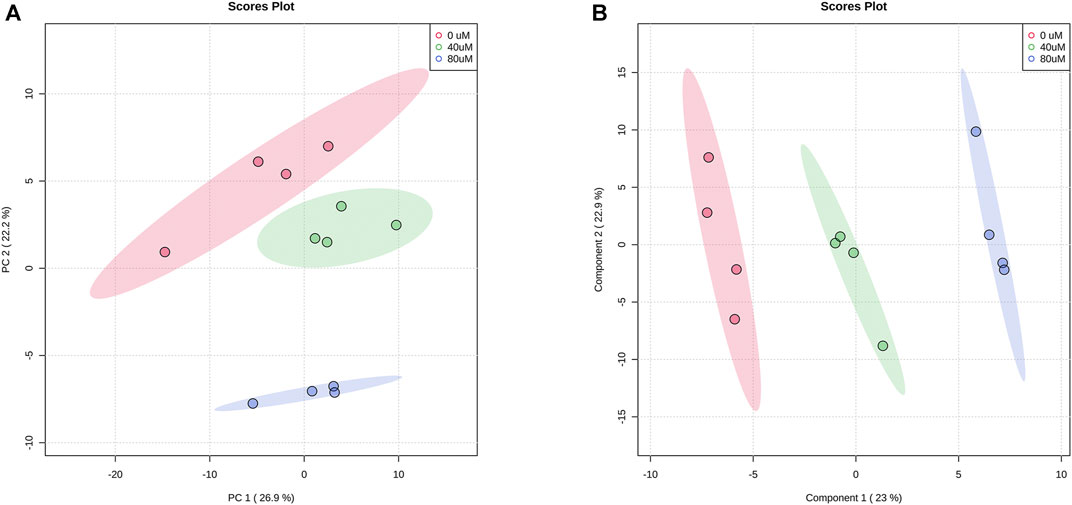
FIGURE 2. Score plots of PCA (A) and PLS-DA (B) models for the metabolome data obtained by LC-MS, showing the metabolic profile differences between control and EGCG-treated groups. Red cycle: control group; green cycle: 40 μM EGCG-treated group; blue cycle: 80 μM EGCG-treated group.
As the volcano plot shows (Figure 3, Supplementary Figure S2), the up-regulated metabolites between the control and the EGCG exposure groups were presented on the right-hand side of the valley, while the left-hand side of the valley represents those that were down-regulated. The number of significantly altered metabolic abundances in the 80 μM group was greater than that in the 40 μM group, which indicated that EGCG disturbed the A549cells in a dose-dependent manner. The ANOVA test analysis was utilized to identify potential biomarkers contributing most to the difference between control and the EGCG-treated groups. The results with metabolites (p < 0.05) are shown in Table 1 and separately in Figure 4. The top 25 significantly changed metabolites were visualized using a heat map in a red-blue scale (from higher to lower metabolite levels) (Figure 5). In pairwise comparison, metabolites with a p-value below 0.05 and fold change above 1.5 or below 0.75 were selected as potential biomarkers. As shown in Supplementary Table S1, the identified metabolites were summarized, and a total of 11 features were selected as potentially altered metabolite markers in A549 cells exposed to 80 μM EGCG compared with the control group. In addition, all of the disturbed metabolites with p < 0.05 in 80 μM EGCG-treated A549 cells compared to controls were summarized in Table 2. The corresponding results between the 40 μM EGCG-treated group and the control group are shown in Supplementary Tables S2, S3 respectively.
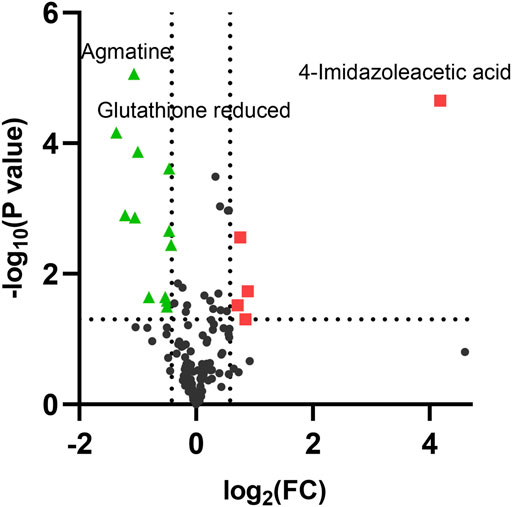
FIGURE 3. Volcano plot analysis of differential metabolites in A549 cells after exposure to 80 μM EGCG. The x-axis represents log2 (fold change), while the y-axis represents p-value in -log10 scale. The significantly up-regulated metabolites were indicated in red squares and down-regulated in green triangles. (p < 0.05 and fold change >1.5 or <0.75).
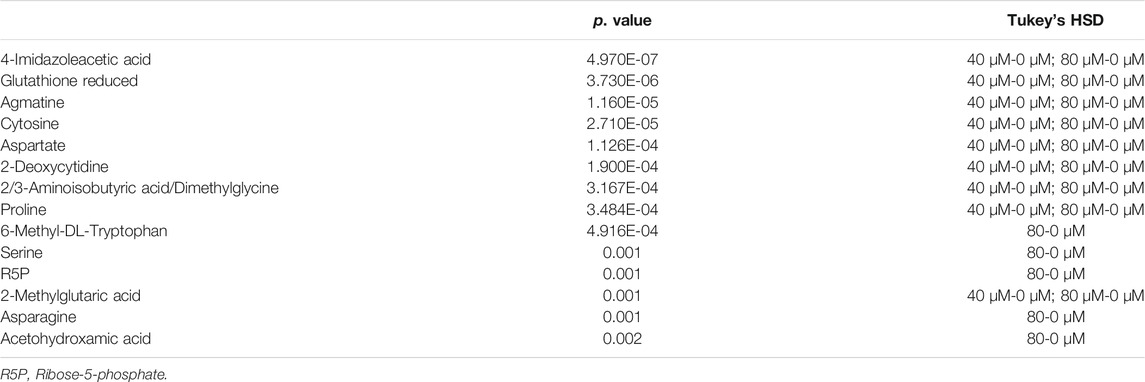
TABLE 1. Significantly altered metabolites among the control and the EGCG-treated groups by ANOVA test analysis with Tukey’s post hoc analysis.
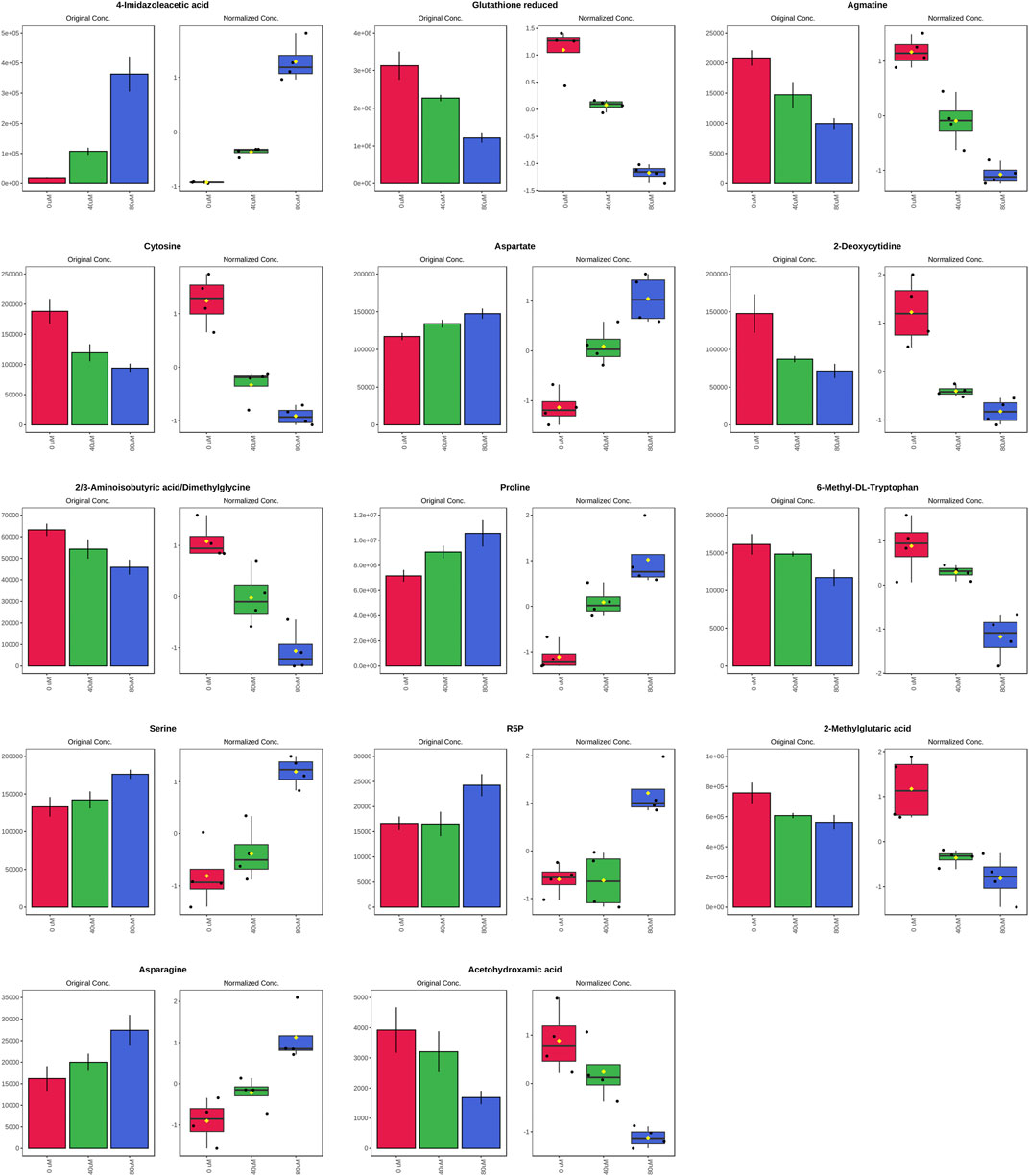
FIGURE 4. Significantly altered metabolites among the control and the EGCG-treated groups. The bar plots on the left show the original peak intensity values (mean ± SD). The box and whisker plots on the right summarize the normalized values.
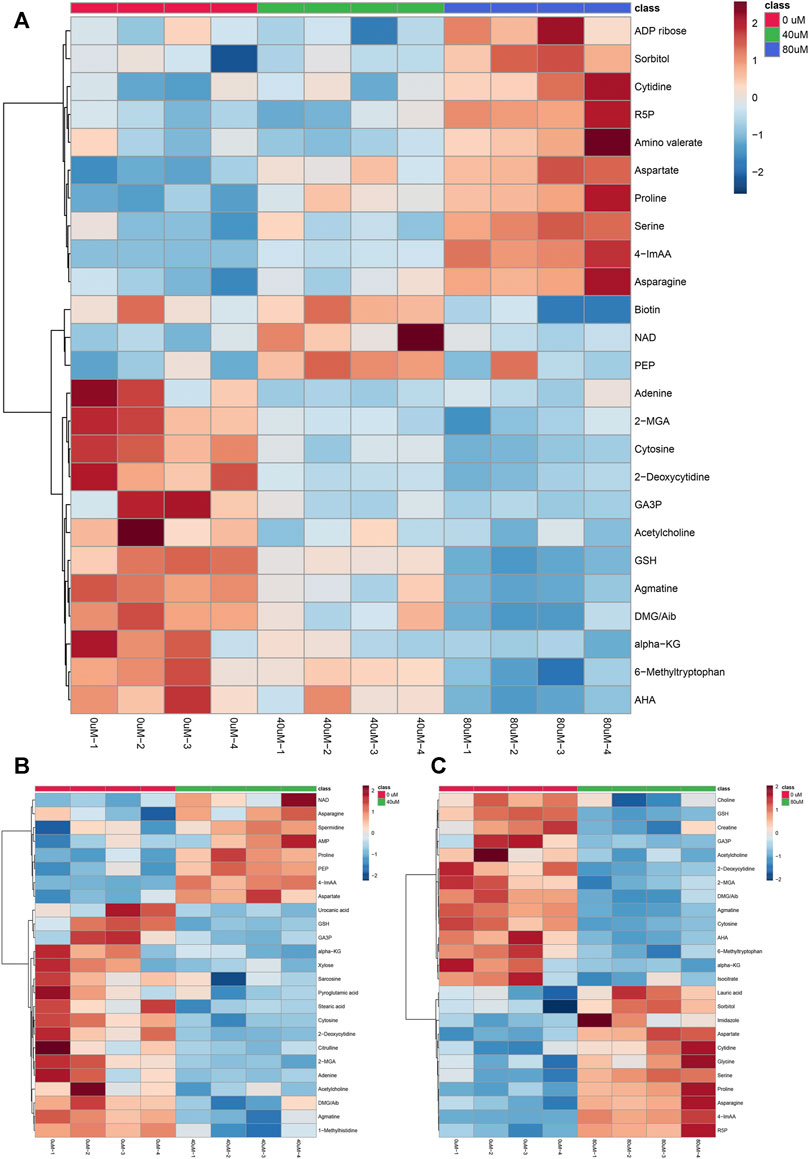
FIGURE 5. Hierarchical clustering heat map of the top 25 differential metabolites selected based on t-tests/ANOVA, with the degree of change marked with red (up-regulation) and blue (down-regulation). The distance measure was set to “Euclidean” and the clustering algorithm was set to “Ward”. (A): control group, 40 μM EGCG-treated group and 80 μM EGCG-treated group; (B): control group and 40 μM EGCG-treated group; (C): control group and 80 μM EGCG-treated group. Abbreviations: R5P, Ribose-5-phosphate; 4-ImAA, 4-Imidazoleacetic acid; NAD, nicotinamide adenine dinucleotide; PEP, phosphoenolpyruvate; 2-MGA, 2-Methylglutaric Acid; GA3P, glyceraldehyde 3-phosphate; GSH, glutathione; DMG, Dimethylglycine; Aib, 2/3-Aminoisobutyric acid; alpha-KG, alpha-Ketoglutaric acid; AHA, Acetohydroxamic acid.
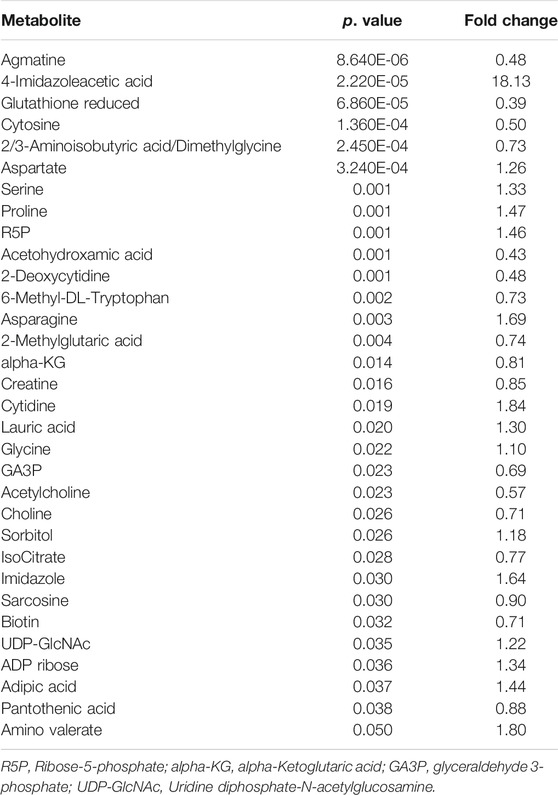
TABLE 2. The disturbed metabolites with p < 0.05 in 80 μM EGCG-treated A549 cells compared to controls.
Analysis of Metabolic Pathways
We used MetaboAnalyst 5.0 and metabolites with p < 0.05 to analyze metabolic pathways. Compared to the control group, 27 metabolic pathways were affected (Supplementary Table S4) in the 80 μM EGCG-treated group. Of these, two markedly altered pathways were filtered according to specific criteria (raw p < 0.05 and impact value >0.2): Glycine, serine and threonine metabolism (impact value = 0.628), Alanine, aspartate and glutamate metabolism (impact value = 0.272). Each metabolic pathway was represented by a colored circle within the diagram. As shown in Figure 6 and Supplementary Table S4, EGCG induced significant perturbations in Glycine, serine and threonine metabolism, Alanine, aspartate and glutamate metabolism, Aminoacyl-tRNA biosynthesis, Glyoxylate and dicarboxylate metabolism, Arginine and proline metabolism in the 80 μM EGCG group (Figure 6), as well as Histidine metabolism, arginine and proline metabolism in the 40 μM EGCG group (Supplementary Figure S3).
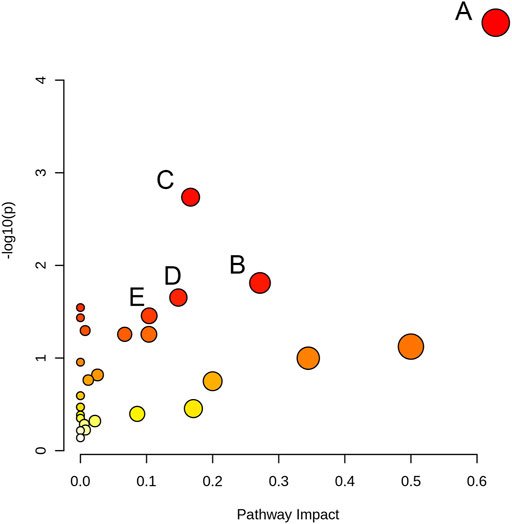
FIGURE 6. Pathway analysis overview depicting altered metabolic pathways in A549 cells from control and 80 μM EGCG-treated groups. The metabolic pathways are displayed as distinctly colored circles depending on their enrichment analysis scores (vertical axis, shade of red) and topology (pathway impact, horizontal axis, circle diameter) via MetaboAnalyst 5.0. (A): Glycine, serine and threonine metabolism, (B): Alanine, aspartate and glutamate metabolism, (C): Aminoacyl-tRNA biosynthesis, (D): Glyoxylate and dicarboxylate metabolism E: Arginine and proline metabolism.
Discussion
The anticancer ability of EGCG has been shown to be related to its antiproliferative and proapoptotic effects (Ahmad et al., 1997; Khan et al., 2006; Ma et al., 2013; Pal et al., 2018; Almatroodi et al., 2020). Similar to previous reports (Jiang et al., 2016; Li et al., 2016), our data suggest that EGCG concentrations at 40 μM or greater showed evident cell growth inhibition on A549 cells compared to the control group. Considering the balance between cell viability and data interpretability and exploring cell metabolism changes caused by different concentrations of EGCG, 40 and 80 μM were chosen for further experiments in this study, corresponding to 92 and 60% survival rate respectively (Chu et al., 2018; Daskalaki et al., 2018; Zhang et al., 2020). It has been proved that treatment with green tea-based food supplements has acceptable safety, but high doses of EGCG can induce certain toxic side effects (Peter et al., 2017). Intake up to 300 mg EGCG/person/day is a tolerable upper intake level proposed for food supplements (Dekant et al., 2017). In a previous study, the maximum plasma concentration of EGCG was 695.8 ng/ml after receiving oral EGCG in 10 day’s repeated doses of 400 mg (Ullmann et al., 2004).
Studies have demonstrated various biological and pharmacological activities of EGCG, such as antioxidant, anti-inflammatory, antiangiogenic, antiproliferative, proapoptotic, and antimetastatic properties (Gu et al., 2013; Lee et al., 2013; Zhang et al., 2013; Chen et al., 2016; Liu et al., 2016; Fujiki et al., 2017; Almatroodi et al., 2020). In vitro, EGCG has been shown to inhibit growth by increasing the percentage of cells at the G0/G1 phase of the cell cycle (Fujiki et al., 2017) and inhibit epithelial-mesenchymal transition and migration via downregulation of HIF-1α, VEGF, pAkt/ERK, COX-2 and vimentin in A549 lung cancer cell (Shi et al., 2015). Also, research has shown that EGCG stimulates apoptosis in the H1299 lung cancer cell line by inhibiting the activation of PI3K/Akt serine/threonine kinase 1 signaling pathway (Gu et al., 2018). One study demonstrated that the inhibition of A549 cell proliferation by EGCG might be achieved via suppressing the expression of the cell death-inhibiting gene, Bcl-xL (Sonoda et al., 2014). Another study showed EGCG also upregulated the expression of the apoptosis-promoting factor Bax by regulating Ku70 acetylation that blocks the interaction between Ku70 and Bax (Li et al., 2016). The amino acids alanine and glutamate were found to be significantly up-regulated in apoptotic HepG2 and HEK293 cells irrespective of the apoptosis inducer (Halama et al., 2013). Disturbed alanine, aspartate and glutamate metabolism in A549 cells under 80 μM EGCG exposure in this study may be related to the proapoptotic effect of EGCG. Long non-coding RNAs (lncRNAs) have emerged as new players in the cancer paradigm. Real-time quantitative reverse transcription-polymerase chain reaction proved a downregulation of HMMR-AS1, AL392089.1, PSMC3IP, and LINC02643 lncRNAs and upregulation of RP1-74M1.3, AC087273.2, SNAI3-AS1, LINC02532, and AC007319.1 lncRNAs in A549 cell lines treated with EGCG (Hu et al., 2019). Various lncRNAs, mRNAs, or proteins regulated by EGCG identified in these studies could affect the metabolic results of A549 cells. Synergistic inhibition of lung cancer cells by EGCG with other drugs has also been reported, such as leptomycin B (Cromie and Gao, 2015), NF-κB inhibitor BAY11-7082 (Zhang et al., 2019), gefitinib (Meng et al., 2019), and cisplatin (Jiang et al., 2016). However, the precise underlying mechanisms of the antitumor activity of EGCG in lung cancer are still largely unclear.
In this study, we used a metabolic approach to further uncover the likely mechanisms underlying the anticancer activity of EGCG in A549 cells. This approach led us to identify 32 differential metabolites (15 upregulated/17 downregulated) in the 80 μM EGCG treated group compared to the control (Table 2). Among the identified metabolites, 11 compounds were significantly changed (fold change >1.5 or <0.75) (Supplementary Table S2). Glycine, serine and threonine metabolism and alanine, aspartate and glutamate metabolism were the two most significantly disturbed pathways under 80 μM EGCG exposure. Histidine metabolism and arginine and proline metabolism were the two most significantly disturbed by exposure to 40 μM EGCG. A schematic diagram of the modulated metabolites and potential disturbed metabolic pathways is shown in Figure 7. A more specific analysis of metabolites is as follows.
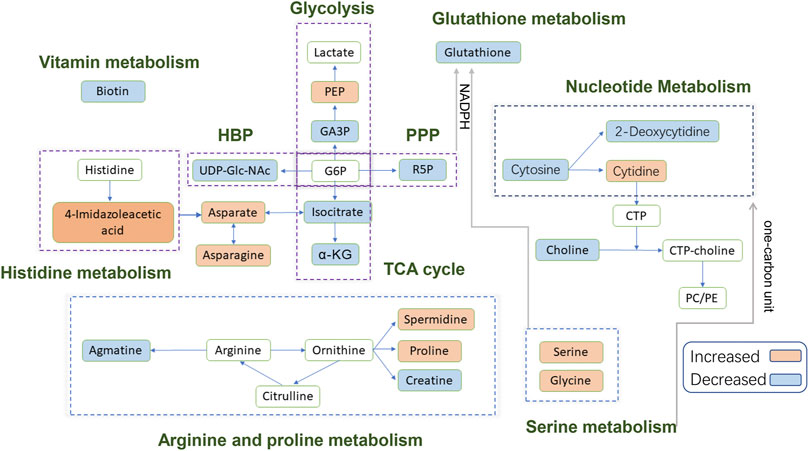
FIGURE 7. Schematic diagram of the modulated metabolites and potential disturbed metabolic pathways. Up-regulated metabolites detected are shown in the orange background; down-regulated metabolites detected are shown in the blue background; blank background means no statistically significant change or undetected. Abbreviations: PEP, phosphoenolpyruvate; GA3P, glyceraldehyde 3-phosphate; G6P, glucose-6-phosphate; α-KG, alpha-Ketoglutaric acid; R5P, Ribose-5-phosphate; CTP, cytidine triphosphate; PC, phosphatidylcholine; PE, phosphatidylethanolamine; HBP, hexosamine biosynthetic pathway; PPP, pentose phosphate pathway; TCA, tricarboxylic acid.
Energy Metabolism
In this study, the metabolomics data suggested that EGCG altered the cellular energy metabolism of A459 cells through glycolysis and the tricarboxylic acid (TCA) cycle. Consumption of glucose by tumors increased markedly compared to the nonproliferating normal tissues to meet the biosynthetic demands associated with proliferation (Warburg et al., 1927). Usually, cancer cells predominantly use glycolysis rather than the TCA cycle for energy production, a phenomenon known as the Warburg effect (Panieri and Santoro, 2016). In previous studies, EGCG significantly reduced lactate production, anaerobic glycolysis, glucose consumption and glycolytic rate in pancreatic adenocarcinoma MIA PaCa-2 cells (Lu et al., 2015). A decrease in glycolysis intermediate glyceraldehyde 3-phosphate was observed in 80 μM EGCG induced cells compared with control cells in this study. However, there were no significant differences in glycolysis intermediates such as glucose-6-phosphate/fructose-6-phosphate (G6P/F6P) and lactate between the control group and EGCG induced group, neither 40 μM nor 80 μM. Interestingly, phosphoenolpyruvate (PEP) was found to increase in the 40 μM group compared to the control. An increase in Glucose-6-phosphate isomerase (GPI), ATP-dependent 6-phosphofructokinase platelet type (PFK-P) and fructose-bisphosphate aldolase A (ALDA) were detected by Wu et al. (2017) in Dox-induced senescent cells compared with control cells, suggesting an up-regulation of the glycolytic pathway during senescence. So, we couldn’t refuse the assumption that the inhibitory effect on glycolysis may be counteracted by induced cell senescence in our research.
Ribose-5-phosphate, which can be generated by the pentose phosphate pathway (PPP), a constituent of nucleotides, was found to increase in the 80 μM group compared to the control. Wu et al. (2017) also found the activation of PPP in senescent cells. We could infer that A549 cells would produce more nucleotide precursors to fulfill the increased need for nucleosides for DNA damage repair by activating PPP when challenged with EGCG treatment.
Our data revealed an elevated level of Uridine diphosphate-N-acetylglucosamine (UDP-Glc-NAc) in the 80 μM EGCG induced group compared to the control one. UDP-Glc-NAc is the end product of a well-established pathway for nutrient sensing-the hexosamine biosynthetic pathway (HBP) and also the donor substrate for modification of nucleocytoplasmic proteins at serine and threonine residues with N-acetylglucosamine (O-GlcNAc) (Wells et al., 2003). Elevated HBP has been reported in cancers and much evidence suggests the HBP helps fuel cancer cell metabolism, growth, survival, and spread (Ferrer et al., 2016; Akella et al., 2019). Interestingly, not only in the cancer cells but also in senescent cells, up-regulation of HBP has been suggested (Wu et al., 2017). The reason for the up-regulation of the UDP-Glc-NAc induced by EGCG in A549 cells needs to be further explored.
The tricarboxylic acid (TCA) cycle is the main pathway of glucose degradation and the primary energy supplier for universal organisms. The 80 μM EGCG induced group showed a down-regulated TCA cycle activity in the A549 cell line, manifested as a decrease in two main TCA cycle intermediates: α-ketoglutarate and isocitrate. However, we didn’t find similar down-regulated TCA cycle intermediates in 40 μM EGCG induced group. This suggested that downregulated TCA cycle in A549 cells induced by EGCG may be dose-dependent and relates to the downregulation of cell viability.
Amino Acid Metabolism
TCA cycle provides metabolic precursors for the biosynthesis of non-essential amino acids, including aspartate and asparagine. In our study, the level of aspartate and asparagine was increased in 80 μM EGCG induced group, which indicated that there were other ways to supplement the synthesis of aspartic acid.
The significantly increased expression of 4-Imidazoleacetic acid (histidine’s metabolite) was observed in EGCG-treated A549 cells, which implied the disturbance of histidine metabolism. The presented evidence indicates that histamine is an important mediator in cancer development and progression (Rivera et al., 2000), and the effects of histamine’s receptor antagonists on cancer cell proliferation have been explored (Blaya et al., 2010). 4-Imidazoleacetic acid is the most apparent upregulated metabolite among the statistically different metabolites in our study, by 18.13-fold and 5.36-fold in 80 and 40 μM EGCG induced group respectively compared to the control group. 4-Imidazoleacetic acid can be generated from oxidative deamination of histamine and then transform to aspartate. So, the increased level of aspartate and asparagine is not strange in the 80 μM EGCG induced group. Asparagine has also been reported to potentiate CD8+ T-cell activation and antitumor responses (Wu et al., 2021). As tumors frequently outgrow their supply, cancer cells reside in oxygen-poor environments. Low oxygen activates a transcriptional program that induces glucose uptake and glycolysis while suppressing the electron transport chain (ETC) activity (Ackerman and Simon, 2014). Studies show that aspartate synthesis plays an essential role in the electron transport chain in cell proliferation (Birsoy et al., 2015; Sullivan et al., 2015). Therefore, aspartate may be a limiting metabolite for tumor growth, and aspartate availability may be targeted for cancer therapy (Garcia-Bermudez et al., 2018).
The metabolites of arginine are involved in multiple pathways. Creatine participates in ATP production, whereas ornithine can be converted to putrescine and spermidine for cell proliferation (Wei et al., 2001; Abraham et al., 2013). Ornithine can also be converted to proline and hydroxyproline for collagen formation and new extracellular matrix deposition (Tan et al., 1983). A meta-analysis of metabolic enzyme expression across diverse tumor types identified pyrroline-5-carboxylate reductase (PYCR1), the principal enzyme in proline biosynthesis, as one of the most commonly overexpressed genes in tumors (Nilsson et al., 2014). Compared with the normal, increased levels of spermidine (in 40 μM EGCG induced group) and proline (both in 40 and 80 μM EGCG induced group) were found in our research. However, the level of CTP itself was not changed. In addition, we found decreased levels of creatine in the 80 μM EGCG induced group, another metabolite of arginine which participates in ATP production (Abraham et al., 2013). Agmatine, which can be converted from arginine by the action of arginine decarboxylase on the cell mitochondrial membrane, was also found to decrease in the EGCG group. Agmatine can induce a decrease in cell proliferation due to decreased intracellular levels of polyamines putrescine, spermidine, and spermine (Higashi et al., 2004). Studies have indicated that agmatine administration to tumor cells in vitro results in a suppression of cell proliferation (Molderings et al., 2004; Mayeur et al., 2005). In our research, the antitumor effect of EGCG may counteract the endogenous production of agmatine.
Serine is crucial for multiple metabolic pathways required for cell growth and proliferation, including phospholipid, purine and glutathione biosynthesis, as well as being a methyl source for single carbon metabolism. Serine has been reported to be the third most consumed metabolite by cancer cells after glucose and glutamine (Jain et al., 2012; Dolfi et al., 2013). When a significant amount of serine is converted into glycine, serine releases a one-carbon unit to the one-carbon pool. Glycine could also contribute to the one-carbon pool through the glycine cleavage system. One-carbon pathway metabolites contribute to a number of cellular biosynthetic and regulatory processes. Serine was found to be elevated in our research and glycine slightly, which may indicate the decrease consumption of them for one-carbon unit generation.
Nucleotide Metabolism
The change of carbon flow in the metabolic stream will cause the abnormality of nucleotide metabolism. The increase of serine level can promote serine-mediated pyruvate kinase 2 (PKM2) activity by inducing allosteric changes of the enzyme (Mazurek, 2011; Chaneton et al., 2012). PKM2 reduces the carbon flux into the serine biosynthesis pathway and the nucleotide biosynthesis pathway, ultimately affecting nucleotide metabolism (Mazurek, 2011; Chaneton et al., 2012). In our study, the decrease of cytosine and 2-deoxycytidine in the 80 μM EGCG-treated group may be related to the up-regulation of serine. This trend is not applicable to cytidine, but it’s not strange when the trend of choline is down-regulated. It has been reported that the reduction of choline and glutathione metabolites is associated with apoptosis (Rainaldi et al., 2008; Halama et al., 2013). Cytidine is a precursor of cytidine triphosphate (CTP) needed in the phosphatidylcholine (PC) and phosphatidylethanolamine (PE) biosynthetic pathways. The down-regulation of choline may lead to a reduction in cytidine consumption.
From Supplementary Table S3, we can find that adenine was downregulated by 0.38-fold which was the most obvious reduction among all the statistically different metabolites in the 40 μM group compare to the control. Clear signaling roles for extracellular adenosine have been established in immunomodulation, vascular remodeling, and promotion of cell growth and proliferation (Chen et al., 2018; Di Virgilio et al., 2018; Morandi et al., 2018; Antonioli et al., 2019). In recent years, it has also been found that adenosine can be used as a signal molecule to affect the biological behavior of tumor cells through different signaling pathways, such as triggering cell cycle arrest, inducing tumor cell apoptosis and affecting cell proliferation (Yang et al., 2007).
Glutathione Metabolism
In our study, glutathione expression was downregulated in 80 μM EGCG-treated group by 0.39-fold compared with that in the control group. In a metabolomics study of EGCG acting on colorectal cancer cells (HT-29), glutathione expression was also downregulated in EGCG-treated cells (Zhang et al., 2020). Generation of reactive oxygen species (ROS) at high levels can damage nucleotides, proteins and lipids, so impair cell viability. In cancer cells, glutathione oxidation-reduction coupled to NADPH reduction-oxidation is a major pathway for ROS detoxification (Lv et al., 2019). NADPH for ROS turnover through this pathway can be generated from glucose via the pentose phosphate pathway or serine via one-carbon metabolism. As analyzed above, the former one was up-regulated. Taken together, disturbance of glutathione metabolism is a potential pathway involved in the antitumor mechanism of EGCG.
Vitamin Metabolism
Biotin (vitamin H) is an essential micronutrient vital for normal cellular function (Livaniou et al., 2000). To thrive and multiply rapidly, cancer cells need extra biotin compared with normal cells. Biotin overexpression is observed in wide types of cancer cells, including renal (RENCA, RD0995), leukemia (L1210FR), lung (A549, M109), ovarian (OV 2008; ID8), mastocytoma (P815), and breast (4T1, JC, MMT06056) cancer (Russell-Jones et al., 2004; Chen et al., 2010; Shi et al., 2014). The decreased biotin implied a slowdown in the proliferation of 80 μM EGCG-treated A549 cells compared to the control group.
Finally, there are several limitations in the present study. Firstly, although some previous in vitro studies employed the A549 cell line to explore the mechanism of the antitumor effects of EGCG, the A549 cell line cannot represent the true lung cancer cell environment in vivo. The concentrations of EGCG from 10 to 100 µM used in most of the studies in cell culture systems, as well as in this paper, are much higher than the concentrations monitored in human plasma (usually lower than 1 µM) after tea ingestion. Thus, it is necessary to verify the high concentration findings in cell lines utilizing lower concentrations in the human body. Secondly, in metabolomics studies, the differences in viability between the control and treated cells would affect the accuracy of the results. The dose of IC50 has been used in the metabolomics research of toxicology in recent years (Yu et al., 2019; He et al., 2020; Hou et al., 2020). To capture more responses on cell metabolism related to the anticancer effect of EGCG and explore the changes in metabolic processes with increasing EGCG concentration, the dosages of 40 and 80 μM were both used in our metabolomics experiment. Thirdly, even though we used a pathway-specific LC-MS/MS method that can cover more than 300 metabolites from over 35 metabolic pathways, there were still many important metabolites left out. This has an impact on the analysis of metabolic pathways. In addition, quantitative proteomics is needed to detect whether there was an increase or decrease in enzymes involved better to explain the upregulation or downregulation of the metabolic pathway. Further experiments are needed to investigate the specific relationship between genetic changes and metabolite changes.
Conclusion
In this study, the metabolite changes in A549 cells induced by EGCG were investigated utilizing LC-MS-based metabolomics. Our data demonstrated that altered metabolites were involved in the metabolism of glucose, amino acid, nucleotide, glutathione, vitamin and especially associated with serine and threonine metabolism, alanine, aspartate and glutamate metabolism, and histidine metabolism. These findings contribute to understanding the intramolecular metabolic processes of A549 cells caused by EGCG and may provide potential clues for the underlying mechanisms of the anti-cancer property of EGCG. Further researches are required for the therapeutic application of EGCG in cancer management.
Data Availability Statement
The original contributions presented in the study are included in the article/Supplementary Material, further inquiries can be directed to the corresponding authors.
Author Contributions
XZ and HH conceived and designed the study. HH, DH, YX, and TP carried out the experiments. TP, HH, WP, and LB drafted this manuscript and analyzed the data. HH and XZ provided final approval of the version to be published. All of the authors discussed the complete dataset to establish an integral and coherent analysis. All authors have read and approved the final manuscript.
Conflict of Interest
The authors declare that the research was conducted in the absence of any commercial or financial relationships that could be construed as a potential conflict of interest.
Publisher’s Note
All claims expressed in this article are solely those of the authors and do not necessarily represent those of their affiliated organizations, or those of the publisher, the editors and the reviewers. Any product that may be evaluated in this article, or claim that may be made by its manufacturer, is not guaranteed or endorsed by the publisher.
Supplementary Material
The Supplementary Material for this article can be found online at: https://www.frontiersin.org/articles/10.3389/fphar.2021.732716/full#supplementary-material
References
Abraham, M. R., Bottomley, P. A., Dimaano, V. L., Pinheiro, A., Steinberg, A., Traill, T. A., et al. (2013). Creatine Kinase Adenosine Triphosphate and Phosphocreatine Energy Supply in a Single kindred of Patients with Hypertrophic Cardiomyopathy. Am. J. Cardiol. 112 (6), 861–866. doi:10.1016/j.amjcard.2013.05.017
Ackerman, D., and Simon, M. C. (2014). Hypoxia, Lipids, and Cancer: Surviving the Harsh Tumor Microenvironment. Trends Cell Biol. 24 (8), 472–478. doi:10.1016/j.tcb.2014.06.001
Ahmad, N., Feyes, D. K., Nieminen, A. L., Agarwal, R., and Mukhtar, H. (1997). Green tea Constituent Epigallocatechin-3-Gallate and Induction of Apoptosis and Cell Cycle Arrest in Human Carcinoma Cells. J. Natl. Cancer Inst. 89 (24), 1881–1886. doi:10.1093/jnci/89.24.1881
Akella, N. M., Ciraku, L., and Reginato, M. J. (2019). Fueling the Fire: Emerging Role of the Hexosamine Biosynthetic Pathway in Cancer. BMC Biol. 17 (1), 52. doi:10.1186/s12915-019-0671-3
Almatroodi, S. A., Almatroudi, A., Khan, A. A., Alhumaydhi, F. A., Alsahli, M. A., and Rahmani, A. H. (2020). Potential Therapeutic Targets of Epigallocatechin Gallate (EGCG), the Most Abundant Catechin in Green Tea, and its Role in the Therapy of Various Types of Cancer. Molecules 25 (14), 3146. doi:10.3390/molecules25143146
Antonioli, L., Fornai, M., Blandizzi, C., Pacher, P., and Haskó, G. (2019). Adenosine Signaling and the Immune System: When a Lot Could Be Too Much. Immunol. Lett. 205, 9–15. doi:10.1016/j.imlet.2018.04.006
Birsoy, K., Wang, T., Chen, W. W., Freinkman, E., Abu-Remaileh, M., and Sabatini, D. M. (2015). An Essential Role of the Mitochondrial Electron Transport Chain in Cell Proliferation Is to Enable Aspartate Synthesis. Cell 162 (3), 540–551. doi:10.1016/j.cell.2015.07.016
Blaya, B., Nicolau-Galmés, F., Jangi, S. M., Ortega-Martínez, I., Alonso-Tejerina, E., Burgos-Bretones, J., et al. (2010). Histamine and Histamine Receptor Antagonists in Cancer Biology. Inflamm. Allergy Drug Targets 9 (3), 146–157. doi:10.2174/187152810792231869
Borutinskaitė, V., Virkšaitė, A., Gudelytė, G., and Navakauskienė, R. (2018). Green tea Polyphenol EGCG Causes Anti-cancerous Epigenetic Modulations in Acute Promyelocytic Leukemia Cells. Leuk. Lymphoma 59 (2), 469–478. doi:10.1080/10428194.2017.1339881
Carroll, P. A., Diolaiti, D., McFerrin, L., Gu, H., Djukovic, D., Du, J., et al. (2015). Deregulated Myc Requires MondoA/Mlx for Metabolic Reprogramming and Tumorigenesis. Cancer Cell 27 (2), 271–285. doi:10.1016/j.ccell.2014.11.024
Chaneton, B., Hillmann, P., Zheng, L., Martin, A. C. L., Maddocks, O. D. K., Chokkathukalam, A., et al. (2012). Serine Is a Natural Ligand and Allosteric Activator of Pyruvate Kinase M2. Nature 491 (7424), 458–462. doi:10.1038/nature11540
Chen, D., and Dou, Q. P. (2008). Tea Polyphenols and Their Roles in Cancer Prevention and Chemotherapy. Int. J. Mol. Sci. 9 (7), 1196–1206. doi:10.3390/ijms9071196
Chen, M., Wei, L., Law, C. T., Tsang, F. H., Shen, J., Cheng, C. L., et al. (2018). RNA N6-Methyladenosine Methyltransferase-like 3 Promotes Liver Cancer Progression through YTHDF2-dependent Posttranscriptional Silencing of SOCS2. Hepatology 67 (6), 2254–2270. doi:10.1002/hep.29683
Chen, S., Zhao, X., Chen, J., Chen, J., Kuznetsova, L., Wong, S. S., et al. (2010). Mechanism-based Tumor-Targeting Drug Delivery System. Validation of Efficient Vitamin Receptor-Mediated Endocytosis and Drug Release. Bioconjug. Chem. 21 (5), 979–987. doi:10.1021/bc9005656
Chen, S. J., Yao, X. D., Peng, B. O., Xu, Y. F., Wang, G. C., Huang, J., et al. (2016). Epigallocatechin-3-gallate Inhibits Migration and Invasion of Human Renal Carcinoma Cells by Downregulating Matrix Metalloproteinase-2 and Matrix Metalloproteinase-9. Exp. Ther. Med. 11 (4), 1243–1248. doi:10.3892/etm.2016.3050
Chu, K. O., Chan, K. P., Chan, S. O., Ng, T. K., Jhanji, V., Wang, C. C., et al. (2018). Metabolomics of Green-Tea Catechins on Vascular-Endothelial-Growth-Factor-Stimulated Human-Endothelial-Cell Survival. J. Agric. Food Chem. 66 (48), 12866–12875. doi:10.1021/acs.jafc.8b05998
Cromie, M. M., and Gao, W. (2015). Epigallocatechin-3-gallate Enhances the Therapeutic Effects of Leptomycin B on Human Lung Cancer A549 Cells. Oxid Med. Cell Longev 2015, 217304. doi:10.1155/2015/217304
Daskalaki, E., Pillon, N. J., Krook, A., Wheelock, C. E., and Checa, A. (2018). The Influence of Culture media upon Observed Cell Secretome Metabolite Profiles: The Balance between Cell Viability and Data Interpretability. Anal. Chim. Acta 1037, 338–350. doi:10.1016/j.aca.2018.04.034
Dekant, W., Fujii, K., Shibata, E., Morita, O., and Shimotoyodome, A. (2017). Safety Assessment of green tea Based Beverages and Dried green tea Extracts as Nutritional Supplements. Toxicol. Lett. 277, 104–108. doi:10.1016/j.toxlet.2017.06.008
Di Virgilio, F., Sarti, A. C., Falzoni, S., De Marchi, E., and Adinolfi, E. (2018). Extracellular ATP and P2 Purinergic Signalling in the Tumour Microenvironment. Nat. Rev. Cancer 18 (10), 601–618. doi:10.1038/s41568-018-0037-0
Dolfi, S. C., Chan, L. L., Qiu, J., Tedeschi, P. M., Bertino, J. R., Hirshfield, K. M., et al. (2013). The Metabolic Demands of Cancer Cells Are Coupled to Their Size and Protein Synthesis Rates. Cancer Metab. 1 (1), 20. doi:10.1186/2049-3002-1-20
Dunn, W. B., Broadhurst, D. I., Atherton, H. J., Goodacre, R., and Griffin, J. L. (2011). Systems Level Studies of Mammalian Metabolomes: the Roles of Mass Spectrometry and Nuclear Magnetic Resonance Spectroscopy. Chem. Soc. Rev. 40 (1), 387–426. doi:10.1039/b906712b
Eghlimi, R., Shi, X., Hrovat, J., Xi, B., and Gu, H. (2020). Triple Negative Breast Cancer Detection Using LC-MS/MS Lipidomic Profiling. J. Proteome Res. 19 (6), 2367–2378. doi:10.1021/acs.jproteome.0c00038
Ferlay, J., Soerjomataram, I., Dikshit, R., Eser, S., Mathers, C., Rebelo, M., et al. (2015). Cancer Incidence and Mortality Worldwide: Sources, Methods and Major Patterns in GLOBOCAN 2012. Int. J. Cancer 136 (5), E359–E386. doi:10.1002/ijc.29210
Ferrer, C. M., Sodi, V. L., and Reginato, M. J. (2016). O-GlcNAcylation in Cancer Biology: Linking Metabolism and Signaling. J. Mol. Biol. 428 (16), 3282–3294. doi:10.1016/j.jmb.2016.05.028
Fujiki, H., Sueoka, E., Rawangkan, A., and Suganuma, M. (2017). Human Cancer Stem Cells Are a Target for Cancer Prevention Using (-)-epigallocatechin Gallate. J. Cancer Res. Clin. Oncol. 143 (12), 2401–2412. doi:10.1007/s00432-017-2515-2
Gan, R. Y., Li, H. B., Sui, Z. Q., and Corke, H. (2018). Absorption, Metabolism, Anti-cancer Effect and Molecular Targets of Epigallocatechin Gallate (EGCG): An Updated Review. Crit. Rev. Food Sci. Nutr. 58 (6), 924–941. doi:10.1080/10408398.2016.1231168
Garcia-Bermudez, J., Baudrier, L., La, K., Zhu, X. G., Fidelin, J., Sviderskiy, V. O., et al. (2018). Aspartate Is a Limiting Metabolite for Cancer Cell Proliferation under Hypoxia and in Tumours. Nat. Cell Biol 20 (7), 775–781. doi:10.1038/s41556-018-0118-z
Griffin, J. L., Atherton, H., Shockcor, J., and Atzori, L. (2011). Metabolomics as a Tool for Cardiac Research. Nat. Rev. Cardiol. 8 (11), 630–643. doi:10.1038/nrcardio.2011.138
Gu, H., Gowda, G. A., and Raftery, D. (2012). Metabolic profiling: are we en route to better diagnostic tests for cancer. Future Oncol. 8 (10), 1207–1210. doi:10.2217/fon.12.113
Gu, H., Zhang, P., Zhu, J., and Raftery, D. (2015). Globally Optimized Targeted Mass Spectrometry: Reliable Metabolomics Analysis with Broad Coverage. Anal. Chem. 87 (24), 12355–12362. doi:10.1021/acs.analchem.5b03812
Gu, J. J., Qiao, K. S., Sun, P., Chen, P., and Li, Q. (2018). Study of EGCG Induced Apoptosis in Lung Cancer Cells by Inhibiting PI3K/Akt Signaling Pathway. Eur. Rev. Med. Pharmacol. Sci. 22 (14), 4557–4563. doi:10.26355/eurrev_201807_15511
Gu, J. W., Makey, K. L., Tucker, K. B., Chinchar, E., Mao, X., Pei, I., et al. (2013). EGCG, a Major green tea Catechin Suppresses Breast Tumor Angiogenesis and Growth via Inhibiting the Activation of HIF-1α and NFκB, and VEGF Expression. Vasc. Cell 5 (1), 9. doi:10.1186/2045-824X-5-9
Halama, A., Riesen, N., Möller, G., Hrabě de Angelis, M., and Adamski, J. (2013). Identification of Biomarkers for Apoptosis in Cancer Cell Lines Using Metabolomics: Tools for Individualized Medicine. J. Intern. Med. 274 (5), 425–439. doi:10.1111/joim.12117
He, H., Shi, X., Lawrence, A., Hrovat, J., Turner, C., Cui, J. Y., et al. (2020). 2,2',4,4'-tetrabromodiphenyl Ether (BDE-47) Induces Wide Metabolic Changes Including Attenuated Mitochondrial Function and Enhanced Glycolysis in PC12 Cells. Ecotoxicol Environ. Saf. 201, 110849. doi:10.1016/j.ecoenv.2020.110849
Higashi, K., Yoshida, K., Nishimura, K., Momiyama, E., Kashiwagi, K., Matsufuji, S., et al. (2004). Structural and Functional Relationship Among Diamines in Terms of Inhibition of Cell Growth. J. Biochem. 136 (4), 533–539. doi:10.1093/jb/mvh150
Hirsch, F. R., Scagliotti, G. V., Mulshine, J. L., Kwon, R., Curran, W. J., Wu, Y. L., et al. (2017). Lung Cancer: Current Therapies and New Targeted Treatments. Lancet 389 (10066), 299–311. doi:10.1016/S0140-6736(16)30958-8
Hou, L., Guan, S., Jin, Y., Sun, W., Wang, Q., Du, Y., et al. (2020). Cell Metabolomics to Study the Cytotoxicity of Carbon Black Nanoparticles on A549 Cells Using UHPLC-Q/TOF-MS and Multivariate Data Analysis. Sci. Total Environ. 698, 134122. doi:10.1016/j.scitotenv.2019.134122
Hu, D. L., Wang, G., Yu, J., Zhang, L. H., Huang, Y. F., Wang, D., et al. (2019). Epigallocatechin-3-gallate M-odulates L-ong N-on-coding RNA and mRNA E-xpression P-rofiles in L-ung C-ancer C-ells. Mol. Med. Rep. 19 (3), 1509–1520. doi:10.3892/mmr.2019.9816
Huang, C. Y., Han, Z., Li, X., Xie, H. H., and Zhu, S. S. (2017). Mechanism of EGCG Promoting Apoptosis of MCF-7 Cell Line in Human Breast Cancer. Oncol. Lett. 14 (3), 3623–3627. doi:10.3892/ol.2017.6641
Huh, S. W., Bae, S. M., Kim, Y. W., Lee, J. M., Namkoong, S. E., Lee, I. P., et al. (2004). Anticancer Effects of (-)-Epigallocatechin-3-Gallate on Ovarian Carcinoma Cell Lines. Gynecol. Oncol. 94 (3), 760–768. doi:10.1016/j.ygyno.2004.05.031
Jain, M., Nilsson, R., Sharma, S., Madhusudhan, N., Kitami, T., Souza, A. L., et al. (2012). Metabolite Profiling Identifies a Key Role for glycine in Rapid Cancer Cell Proliferation. Science 336 (6084), 1040–1044. doi:10.1126/science.1218595
Jasbi, P., Wang, D., Cheng, S. L., Fei, Q., Cui, J. Y., Liu, L., et al. (2019). Breast Cancer Detection Using Targeted Plasma Metabolomics. J. Chromatogr. B Analyt Technol. Biomed. Life Sci. 1105, 26–37. doi:10.1016/j.jchromb.2018.11.029
Jiang, P., Wu, X., Wang, X., Huang, W., and Feng, Q. (2016). NEAT1 Upregulates EGCG-Induced CTR1 to Enhance Cisplatin Sensitivity in Lung Cancer Cells. Oncotarget 7 (28), 43337–43351. doi:10.18632/oncotarget.9712
Khan, N., Afaq, F., Saleem, M., Ahmad, N., and Mukhtar, H. (2006). Targeting Multiple Signaling Pathways by green tea Polyphenol (-)-Epigallocatechin-3-Gallate. Cancer Res. 66 (5), 2500–2505. doi:10.1158/0008-5472.CAN-05-3636
Lee, I. T., Lin, C. C., Lee, C. Y., Hsieh, P. W., and Yang, C. M. (2013). Protective Effects of (-)-Epigallocatechin-3-Gallate against TNF-α-Induced Lung Inflammation via ROS-dependent ICAM-1 Inhibition. J. Nutr. Biochem. 24 (1), 124–136. doi:10.1016/j.jnutbio.2012.03.009
Li, C. Y., Dempsey, J. L., Wang, D., Lee, S., Weigel, K. M., Fei, Q., et al. (2018). PBDEs Altered Gut Microbiome and Bile Acid Homeostasis in Male C57BL/6 Mice. Drug Metab. Dispos 46 (8), 1226–1240. doi:10.1124/dmd.118.081547
Li, M., Li, J. J., Gu, Q. H., An, J., Cao, L. M., Yang, H. P., et al. (2016). EGCG Induces Lung Cancer A549 Cell Apoptosis by Regulating Ku70 Acetylation. Oncol. Rep. 35 (4), 2339–2347. doi:10.3892/or.2016.4587
Li, T., Zhao, N., Lu, J., Zhu, Q., Liu, X., Hao, F., et al. (2019). Epigallocatechin Gallate (EGCG) Suppresses Epithelial-Mesenchymal Transition (EMT) and Invasion in Anaplastic Thyroid Carcinoma Cells through Blocking of TGF-β1/Smad Signaling Pathways. Bioengineered 10 (1), 282–291. doi:10.1080/21655979.2019.1632669
Lim, J. J., Li, X., Lehmler, H. J., Wang, D., Gu, H., and Cui, J. Y. (2020). Gut Microbiome Critically Impacts PCB-Induced Changes in Metabolic Fingerprints and the Hepatic Transcriptome in Mice. Toxicol. Sci. 177 (1), 168–187. doi:10.1093/toxsci/kfaa090
Liu, J., Hanavan, P. D., Kras, K., Ruiz, Y. W., Castle, E. P., Lake, D. F., et al. (2019). Loss of SETD2 Induces a Metabolic Switch in Renal Cell Carcinoma Cell Lines toward Enhanced Oxidative Phosphorylation. J. Proteome Res. 18 (1), 331–340. doi:10.1021/acs.jproteome.8b00628
Liu, L., Ju, Y., Wang, J., and Zhou, R. (2017). Epigallocatechin-3-gallate Promotes Apoptosis and Reversal of Multidrug Resistance in Esophageal Cancer Cells. Pathol. Res. Pract. 213 (10), 1242–1250. doi:10.1016/j.prp.2017.09.006
Liu, S., Xu, Z. L., Sun, L., Liu, Y., Li, C. C., Li, H. M., et al. (2016). (-)-Epigallocatechin-3-gallate I-nduces A-poptosis in H-uman P-ancreatic C-ancer C-ells via PTEN. Mol. Med. Rep. 14 (1), 599–605. doi:10.3892/mmr.2016.5277
Livaniou, E., Costopoulou, D., Vassiliadou, I., Leondiadis, L., Nyalala, J. O., Ithakissios, D. S., et al. (2000). Analytical Techniques for Determining Biotin. J. Chromatogr. A. 881 (1-2), 331–343. doi:10.1016/s0021-9673(00)00118-7
Lu, Q. Y., Zhang, L., Yee, J. K., Go, V. W., and Lee, W. N. (2015). Metabolic Consequences of LDHA Inhibition by Epigallocatechin Gallate and Oxamate in MIA PaCa-2 Pancreatic Cancer Cells. Metabolomics 11 (1), 71–80. doi:10.1007/s11306-014-0672-8
Luengo, A., Gui, D. Y., and Vander Heiden, M. G. (2017). Targeting Metabolism for Cancer Therapy. Cell Chem Biol 24 (9), 1161–1180. doi:10.1016/j.chembiol.2017.08.028
Luo, H. Q., Xu, M., Zhong, W. T., Cui, Z. Y., Liu, F. M., Zhou, K. Y., et al. (2014). EGCG Decreases the Expression of HIF-1α and VEGF and Cell Growth in MCF-7 Breast Cancer Cells. J. BUON 19 (2), 435–439.
Luo, K. W., Wei Chen, C., Lung, W. Y., Wei, X. Y., Cheng, B. H., Cai, Z. M., et al. (2017). EGCG Inhibited Bladder Cancer SW780 Cell Proliferation and Migration Both In Vitro and In Vivo via Down-Regulation of NF-Κb and MMP-9. J. Nutr. Biochem. 41, 56–64. doi:10.1016/j.jnutbio.2016.12.004
Lv, H., Zhen, C., Liu, J., Yang, P., Hu, L., and Shang, P. (2019). Unraveling the Potential Role of Glutathione in Multiple Forms of Cell Death in Cancer Therapy. Oxid Med. Cell Longev 2019, 3150145. doi:10.1155/2019/3150145
Ma, J., Shi, M., Li, G., Wang, N., Wei, J., Wang, T., et al. (2013). Regulation of Id1 Expression by Epigallocatechin-3-gallate and its E-ffect on the P-roliferation and A-poptosis of P-oorly D-ifferentiated AGS G-astric C-ancer C-ells. Int. J. Oncol. 43 (4), 1052–1058. doi:10.3892/ijo.2013.2043
Mayeur, C., Veuillet, G., Michaud, M., Raul, F., Blottière, H. M., and Blachier, F. (2005). Effects of Agmatine Accumulation in Human colon Carcinoma Cells on Polyamine Metabolism, DNA Synthesis and the Cell Cycle. Biochim. Biophys. Acta 1745 (1), 111–123. doi:10.1016/j.bbamcr.2004.12.004
Mazurek, S. (2011). Pyruvate Kinase Type M2: a Key Regulator of the Metabolic Budget System in Tumor Cells. Int. J. Biochem. Cell Biol 43 (7), 969–980. doi:10.1016/j.biocel.2010.02.005
McCartney, A., Vignoli, A., Biganzoli, L., Love, R., Tenori, L., Luchinat, C., et al. (2018). Metabolomics in Breast Cancer: A Decade in Review. Cancer Treat. Rev. 67, 88–96. doi:10.1016/j.ctrv.2018.04.012
Meng, J., Chang, C., Chen, Y., Bi, F., Ji, C., and Liu, W. (2019). EGCG Overcomes Gefitinib Resistance by Inhibiting Autophagy and Augmenting Cell Death through Targeting ERK Phosphorylation in NSCLC. Onco Targets Ther. 12, 6033–6043. doi:10.2147/OTT.S209441
Miller, K. D., Nogueira, L., Mariotto, A. B., Rowland, J. H., Yabroff, K. R., Alfano, C. M., et al. (2019). Cancer Treatment and Survivorship Statistics, 2019. CA Cancer J. Clin. 69 (5), 363–385. doi:10.3322/caac.21565
Miller, K. D., Siegel, R. L., Lin, C. C., Mariotto, A. B., Kramer, J. L., Rowland, J. H., et al. (2016). Cancer Treatment and Survivorship Statistics, 2016. CA Cancer J. Clin. 66 (1), 271–289. doi:10.3322/caac.2133210.3322/caac.21349
Molderings, G. J., Kribben, B., Heinen, A., Schröder, D., Brüss, M., and Göthert, M. (2004). Intestinal Tumor and Agmatine (Decarboxylated Arginine): Low Content in colon Carcinoma Tissue Specimens and Inhibitory Effect on Tumor Cell Proliferation In Vitro. Cancer 101 (4), 858–868. doi:10.1002/cncr.20407
Moradzadeh, M., Roustazadeh, A., Tabarraei, A., Erfanian, S., and Sahebkar, A. (2018). Epigallocatechin-3-gallate Enhances Differentiation of Acute Promyelocytic Leukemia Cells via Inhibition of PML-Rarα and HDAC1. Phytother Res. 32 (3), 471–479. doi:10.1002/ptr.5990
Morandi, F., Horenstein, A. L., Rizzo, R., and Malavasi, F. (2018). The Role of Extracellular Adenosine Generation in the Development of Autoimmune Diseases. Mediators Inflamm. 2018, 7019398. doi:10.1155/2018/7019398
Nilsson, R., Jain, M., Madhusudhan, N., Sheppard, N. G., Strittmatter, L., Kampf, C., et al. (2014). Metabolic Enzyme Expression Highlights a Key Role for MTHFD2 and the Mitochondrial Folate Pathway in Cancer. Nat. Commun. 5, 3128. doi:10.1038/ncomms4128
Noreldeen, H. A. A., Liu, X., and Xu, G. (2020). Metabolomics of Lung Cancer: Analytical Platforms and Their Applications. J. Sep. Sci. 43 (1), 120–133. doi:10.1002/jssc.201900736
Pal, D., Sur, S., Roy, R., Mandal, S., and Kumar Panda, C. (2018). Epigallocatechin Gallate in Combination with Eugenol or Amarogentin Shows Synergistic Chemotherapeutic Potential in Cervical Cancer Cell Line. J. Cell Physiol 234 (1), 825–836. doi:10.1002/jcp.26900
Panieri, E., and Santoro, M. M. (2016). ROS Homeostasis and Metabolism: a Dangerous Liason in Cancer Cells. Cell Death Dis 7 (6), e2253. doi:10.1038/cddis.2016.105
Panji, M., Behmard, V., Zare, Z., Malekpour, M., Nejadbiglari, H., Yavari, S., et al. (2021). Suppressing Effects of green tea Extract and Epigallocatechin-3-Gallate (EGCG) on TGF-β- Induced Epithelial-To-Mesenchymal Transition via ROS/Smad Signaling in Human Cervical Cancer Cells. Gene 794, 145774. doi:10.1016/j.gene.2021.145774
Peter, B., Bosze, S., and Horvath, R. (2017). Biophysical Characteristics of Proteins and Living Cells Exposed to the green tea Polyphenol Epigallocatechin-3-Gallate (EGCg): Review of Recent Advances from Molecular Mechanisms to Nanomedicine and Clinical Trials. Eur. Biophys. J. 46 (1), 1–24. doi:10.1007/s00249-016-1141-2
Rainaldi, G., Romano, R., Indovina, P., Ferrante, A., Motta, A., Indovina, P. L., et al. (2008). Metabolomics Using 1H-NMR of Apoptosis and Necrosis in HL60 Leukemia Cells: Differences between the Two Types of Cell Death and independence from the Stimulus of Apoptosis Used. Radiat. Res. 169 (2), 170–180. doi:10.1667/RR0958.1
Reaves, M. L., and Rabinowitz, J. D. (2011). Metabolomics in Systems Microbiology. Curr. Opin. Biotechnol. 22 (1), 17–25. doi:10.1016/j.copbio.2010.10.001
Rivera, E. S., Cricco, G. P., Engel, N. I., Fitzsimons, C. P., Martín, G. A., and Bergoc, R. M. (2000). Histamine as an Autocrine Growth Factor: an Unusual Role for a Widespread Mediator. Semin. Cancer Biol. 10 (1), 15–23. doi:10.1006/scbi.2000.0303
Romano, A., and Martel, F. (2021). The Role of EGCG in Breast Cancer Prevention and Therapy. Mini Rev. Med. Chem. 21 (7), 883–898. doi:10.2174/1389557520999201211194445
Russell-Jones, G., McTavish, K., McEwan, J., Rice, J., and Nowotnik, D. (2004). Vitamin-mediated Targeting as a Potential Mechanism to Increase Drug Uptake by Tumours. J. Inorg. Biochem. 98 (10), 1625–1633. doi:10.1016/j.jinorgbio.2004.07.009
Sano, M., Tabata, M., Suzuki, M., Degawa, M., Miyase, T., and Maeda-Yamamoto, M. (2001). Simultaneous Determination of Twelve tea Catechins by High-Performance Liquid Chromatography with Electrochemical Detection. Analyst 126 (6), 816–820. doi:10.1039/b102541b
Schmidt, D. R., Patel, R., Kirsch, D. G., Lewis, C. A., Vander Heiden, M. G., and Locasale, J. W. (2021). Metabolomics in Cancer Research and Emerging Applications in Clinical Oncology. CA A. Cancer J. Clin. 71 (4), 333–358. doi:10.3322/caac.21670
Seijo, L. M., Peled, N., Ajona, D., Boeri, M., Field, J. K., Sozzi, G., et al. (2019). Biomarkers in Lung Cancer Screening: Achievements, Promises, and Challenges. J. Thorac. Oncol. 14 (3), 343–357. doi:10.1016/j.jtho.2018.11.023
Shi, J., Liu, F., Zhang, W., Liu, X., Lin, B., and Tang, X. (2015). Epigallocatechin-3-gallate Inhibits Nicotine-Induced Migration and Invasion by the Suppression of Angiogenesis and Epithelial-Mesenchymal Transition in Non-small Cell Lung Cancer Cells. Oncol. Rep. 33 (6), 2972–2980. doi:10.3892/or.2015.3889
Shi, J. F., Wu, P., Jiang, Z. H., and Wei, X. Y. (2014). Synthesis and Tumor Cell Growth Inhibitory Activity of Biotinylated Annonaceous Acetogenins. Eur. J. Med. Chem. 71, 219–228. doi:10.1016/j.ejmech.2013.11.012
Shi, X., Wang, S., Jasbi, P., Turner, C., Hrovat, J., Wei, Y., et al. (2019). Database-Assisted Globally Optimized Targeted Mass Spectrometry (dGOT-MS): Broad and Reliable Metabolomics Analysis with Enhanced Identification. Anal. Chem. 91 (21), 13737–13745. doi:10.1021/acs.analchem.9b03107
Siegel, R. L., Miller, K. D., Fuchs, H. E., and Jemal, A. (2021). Cancer Statistics, 2021. CA A. Cancer J. Clin. 71 (1), 7–33. doi:10.3322/caac.21654
Sonoda, J. I., Ikeda, R., Baba, Y., Narumi, K., Kawachi, A., Tomishige, E., et al. (2014). Green tea Catechin, Epigallocatechin-3-Gallate, Attenuates the Cell Viability of Human Non-small-cell Lung Cancer A549 Cells via Reducing Bcl-xL Expression. Exp. Ther. Med. 8 (1), 59–63. doi:10.3892/etm.2014.1719
Sperber, H., Mathieu, J., Wang, Y., Ferreccio, A., Hesson, J., Xu, Z., et al. (2015). The Metabolome Regulates the Epigenetic Landscape during Naive-To-Primed Human Embryonic Stem Cell Transition. Nat. Cell Biol 17 (12), 1523–1535. doi:10.1038/ncb3264
Sullivan, L. B., Gui, D. Y., Hosios, A. M., Bush, L. N., Freinkman, E., and Vander Heiden, M. G. (2015). Supporting Aspartate Biosynthesis Is an Essential Function of Respiration in Proliferating Cells. Cell 162 (3), 552–563. doi:10.1016/j.cell.2015.07.017
Sung, H., Ferlay, J., Siegel, R. L., Laversanne, M., Soerjomataram, I., Jemal, A., et al. (2021). Global Cancer Statistics 2020: GLOBOCAN Estimates of Incidence and Mortality Worldwide for 36 Cancers in 185 Countries. CA A. Cancer J. Clin. 71, 209–249. doi:10.3322/caac.21660
Tan, E. M., Ryhänen, L., and Uitto, J. (1983). Proline Analogues Inhibit Human Skin Fibroblast Growth and Collagen Production in Culture. J. Invest. Dermatol. 80 (4), 261–267. doi:10.1111/1523-1747.ep12534593
Tang, G. Y., Meng, X., Gan, R. Y., Zhao, C. N., Liu, Q., Feng, Y. B., et al. (2019). Health Functions and Related Molecular Mechanisms of Tea Components: An Update Review. Int. J. Mol. Sci. 20 (24). doi:10.3390/ijms20246196
Ullmann, U., Haller, J., Decourt, J. D., Girault, J., Spitzer, V., and Weber, P. (2004). Plasma-kinetic Characteristics of Purified and Isolated green tea Catechin Epigallocatechin Gallate (EGCG) after 10 Days Repeated Dosing in Healthy Volunteers. Int. J. Vitam Nutr. Res. 74 (4), 269–278. doi:10.1024/0300-9831.74.4.269
Warburg, O., Wind, F., and Negelein, E. (1927). The Metabolism of Tumors in the Body. J. Gen. Physiol. 8 (6), 519–530. doi:10.1085/jgp.8.6.519
Wei, L. H., Wu, G., Morris, S. M., and Ignarro, L. J. (2001). Elevated Arginase I Expression in Rat Aortic Smooth Muscle Cells Increases Cell Proliferation. Proc. Natl. Acad. Sci. U S A. 98 (16), 9260–9264. doi:10.1073/pnas.161294898
Wei, R., Penso, N. E. C., Hackman, R. M., Wang, Y., and Mackenzie, G. G. (2019). Epigallocatechin-3-Gallate (EGCG) Suppresses Pancreatic Cancer Cell Growth, Invasion, and Migration Partly through the Inhibition of Akt Pathway and Epithelial-Mesenchymal Transition: Enhanced Efficacy when Combined with Gemcitabine. Nutrients 11 (8), 1856. doi:10.3390/nu11081856
Wei, Y., Jasbi, P., Shi, X., Turner, C., Hrovat, J., Liu, L., et al. (2021). Early Breast Cancer Detection Using Untargeted and Targeted Metabolomics. J. Proteome Res. 20 (6), 3124–3133. doi:10.1021/acs.jproteome.1c00019
Wells, L., Vosseller, K., and Hart, G. W. (2003). A Role for N-Acetylglucosamine as a Nutrient Sensor and Mediator of Insulin Resistance. Cell Mol Life Sci 60 (2), 222–228. doi:10.1007/s000180300017
Wu, D., Liu, Z., Li, J., Zhang, Q., Zhong, P., Teng, T., et al. (2019). Epigallocatechin-3-gallate Inhibits the Growth and Increases the Apoptosis of Human Thyroid Carcinoma Cells through Suppression of EGFR/RAS/RAF/MEK/ERK Signaling Pathway. Cancer Cell Int 19, 43. doi:10.1186/s12935-019-0762-9
Wu, J., Li, G., Li, L., Li, D., Dong, Z., and Jiang, P. (2021). Asparagine Enhances LCK Signalling to Potentiate CD8+ T-Cell Activation and Anti-tumour Responses. Nat. Cell Biol 23 (1), 75–86. doi:10.1038/s41556-020-00615-4
Wu, M., Ye, H., Shao, C., Zheng, X., Li, Q., Wang, L., et al. (2017). Metabolomics-Proteomics Combined Approach Identifies Differential Metabolism-Associated Molecular Events between Senescence and Apoptosis. J. Proteome Res. 16 (6), 2250–2261. doi:10.1021/acs.jproteome.7b00111
Yan, M., and Xu, G. (2018). Current and Future Perspectives of Functional Metabolomics in Disease Studies-A Review. Anal. Chim. Acta 1037, 41–54. doi:10.1016/j.aca.2018.04.006
Yang, C., Du, W., and Yang, D. (2016). Inhibition of green tea Polyphenol EGCG((-)-epigallocatechin-3-gallate) on the Proliferation of Gastric Cancer Cells by Suppressing Canonical Wnt/β-Catenin Signalling Pathway. Int. J. Food Sci. Nutr. 67 (7), 818–827. doi:10.1080/09637486.2016.1198892
Yang, D., Yaguchi, T., Yamamoto, H., and Nishizaki, T. (2007). Intracellularly Transported Adenosine Induces Apoptosis in HuH-7 Human Hepatoma Cells by Downregulating C-FLIP Expression Causing Caspase-3/-8 Activation. Biochem. Pharmacol. 73 (10), 1665–1675. doi:10.1016/j.bcp.2007.01.020
Yeung, P. K. (2018). Metabolomics and Biomarkers for Drug Discovery. Metabolites 8 (1). doi:10.3390/metabo8010011
Yin, Z., Li, J., Kang, L., Liu, X., Luo, J., Zhang, L., et al. (2021). Epigallocatechin‐3‐gallate Induces Autophagy‐related Apoptosis Associated with LC3B II and Beclin Expression of Bladder Cancer Cells. J. Food Biochem. 45 (6), e13758. doi:10.1111/jfbc.13758
Yoshimura, H., Yoshida, H., Matsuda, S., Ryoke, T., Ohta, K., Ohmori, M., et al. (2019). The Therapeutic Potential of Epigallocatechin-3-gallate against H-uman O-ral S-quamous C-ell C-arcinoma through I-nhibition of C-ell P-roliferation and I-nduction of A-poptosis: In vitro and I-n vivo M-urine X-enograft S-tudy. Mol. Med. Rep. 20 (2), 1139–1148. doi:10.3892/mmr.2019.10331
Yu, L., Wu, J., Zhai, Q., Tian, F., Zhao, J., Zhang, H., et al. (2019). Metabolomic Analysis Reveals the Mechanism of Aluminum Cytotoxicity in HT-29 Cells. PeerJ 7, e7524. doi:10.7717/peerj.7524
Zampieri, M., Sekar, K., Zamboni, N., and Sauer, U. (2017). Frontiers of High-Throughput Metabolomics. Curr. Opin. Chem. Biol. 36, 15–23. doi:10.1016/j.cbpa.2016.12.006
Zhang, L., Xie, J., Gan, R., Wu, Z., Luo, H., Chen, X., et al. (2019). Synergistic Inhibition of Lung Cancer Cells by EGCG and NF-Κb Inhibitor BAY11-7082. J. Cancer 10 (26), 6543–6556. doi:10.7150/jca.34285
Zhang, Y., Owusu, L., Duan, W., Jiang, T., Zang, S., Ahmed, A., et al. (2013). Anti-metastatic and Differential Effects on Protein Expression of Epigallocatechin-3-Gallate in HCCLM6 Hepatocellular Carcinoma Cells. Int. J. Mol. Med. 32 (4), 959–964. doi:10.3892/ijmm.2013.1446
Zhang, Z., Zhang, S., Yang, J., Yi, P., Xu, P., Yi, M., et al. (2020). Integrated Transcriptomic and Metabolomic Analyses to Characterize the Anti-cancer Effects of (-)-Epigallocatechin-3-Gallate in Human colon Cancer Cells. Toxicol. Appl. Pharmacol. 401, 115100. doi:10.1016/j.taap.2020.115100
Keywords: EGCG, A549 cells, metabolomics, arginine and proline metabolism, glutamate metabolism, histidine metabolism
Citation: Pan T, Han D, Xu Y, Peng W, Bai L, Zhou X and He H (2021) LC–MS Based Metabolomics Study of the Effects of EGCG on A549 Cells. Front. Pharmacol. 12:732716. doi: 10.3389/fphar.2021.732716
Received: 29 June 2021; Accepted: 15 September 2021;
Published: 28 September 2021.
Edited by:
Shuai Ji, Xuzhou Medical University, ChinaCopyright © 2021 Pan, Han, Xu, Peng, Bai, Zhou and He. This is an open-access article distributed under the terms of the Creative Commons Attribution License (CC BY). The use, distribution or reproduction in other forums is permitted, provided the original author(s) and the copyright owner(s) are credited and that the original publication in this journal is cited, in accordance with accepted academic practice. No use, distribution or reproduction is permitted which does not comply with these terms.
*Correspondence: Xianmei Zhou, emhvdXhpYW5tZWlqc0BhbGl5dW4uY29t; Hailang He, bHlnaGVoYWlsYW5nQDE2My5jb20=