- 1State Key Laboratory for Chemistry and Molecular Engineering of Medicinal Resources, Collaborative Innovation Center for Guangxi Ethnic Medicine, School of Chemistry and Pharmaceutical Science, Guangxi Normal University, Guilin, China
- 2Institute of Translational Medicine, Medical College, Jiangsu Key Laboratory of Integrated Traditional Chinese and Western Medicine for Prevention and Treatment of Senile Diseases, Yangzhou University, Yangzhou, China
Zanthoxylum nitidum (Roxb.) DC. (ZN), with strong effects of anti-inflammation and antioxidant activities is treated as a core herb in traditional Chinese medicine (TCM) preparation for treating stomachache, toothache, and rheumatoid arthritis. However, the active ingredients of ZN are not fully clarified due to its chemical complexity. In the present study, a double spectrum–effect analysis strategy was developed and applied to explore the bioactive components in herbs, and ZN was used as an example. Here, the chemical components in ZN were rapidly and comprehensively profiled based on the mass defect filtering-based structure classification (MDFSC) and diagnostic fragment-ion-based extension approaches. Furthermore, the fingerprints of 20 batches of ZN samples were analyzed by high-performance liquid chromatography, and the anti-inflammatory and antioxidant activities of the 20 batches of ZN samples were studied. Finally, the partial least squares regression (PLSR), gray relational analysis models, and Spearman’s rank correlation coefficient (SRCC) were applied to discover the bioactive compounds in ZN. As a result, a total of 48 compounds were identified or tentatively characterized in ZN, including 35 alkaloids, seven coumarins, three phenolic acids, two flavonoids, and one lignan. The results achieved by three prediction models indicated that peaks 4, 12, and 17 were the potential anti-inflammatory compounds in ZN, whereas peaks 3, 5, 7, 12, and 13 were involved in the antioxidant activity. Among them, peaks 4, 5, 7, and 12 were identified as nitidine, chelerythrine, hesperidin, and oxynitidine by comparison with the standards and other references. The data in the current study achieved by double spectrum–effect analysis strategy had great importance to improve the quality standardization of ZN, and the method might be an efficiency tool for the discovery of active components in a complex system, such as TCMs.
Introduction
Generally, traditional Chinese medicines (TCMs) achieve their therapeutic effects by initial interactions via multicomponents and multitargets. To understand how it works, it is necessary for researchers to study the relationship between TCMs’ compounds and their efficacy from a holistic perspective (Jiang et al., 2010). A pharmacological study of single-compound and holistic studies of TCMs are not birds of a feather; however, their research ideas and methods cannot be generalized (Xie and Leung, 2009). Although the above studies contributed to reveal the mechanism of pharmacological efficacy of TCMs, it is also meaningful to unravel the details of TCMs’ mechanism with important implications to quality control of TCMs and treatment of complicated diseases.
Zanthoxylum nitidum (Roxb.) DC. belongs to the genus Zanthoxylum of family Rutaceae (Seidemann, 2005; Rivera et al., 2014), and its underground roots (ZN) are used as the medicinal part recorded in the Chinese Pharmacopoeia (China Pharmacopoeia Commission, 2020). ZN has excellent curative effects, such as for the treatment of toothache, stomachache, traumatism, and rheumatoid arthritis. In daily life, ZN could be used as toothpaste and hand sanitizer. In a previous study, researchers were mainly focused on the chemical isolation and activity evaluation of ZN (Yang et al., 2008; Zhao et al., 2018; Nguyen et al., 2019). ZN extracts showed good anti-inflammatory and antioxidant activities (Xie, 2000; Liu et al., 2014). Alkaloids, the major component in ZN, have earned an increasing interest (Lu et al., 2020). Nitidine, a typical single alkaloid in ZN, has been found to have antifungal and anti-inflammatory activity (Zhang et al., 2014; Zhang et al., 2017). However, the components in ZN were very complicated, and some other active ingredients could also exist. To screen the active compounds rapidly, a comprehensive qualitative strategy and spectrum–effect relationship analysis is worth to establish.
The spectrum–effect relationship analysis is a tried-and-true method in using stoichiometric methods to figure out the connection between efficacy and components (Wang et al., 2019; Chen et al., 2021; Qiao et al., 2021). Zhang et al. developed the spectrum–effect relationship analysis strategy to discover the active compounds in Lycii Fructus; the results showed that chlorogenic acid, quercetin, kaempferol, and isorhamnetin are their potential bioactive components (Zhang et al., 2018). Moreover, a strategy-contained spectrum–effect relationship analysis was proposed to discover hepatotoxic equivalent markers from Psoraleae Fructus, and the results revealed that psoralen and isopsoralen are the hepatotoxic equivalent components (Zhang et al., 2021). The above studies have further verified the effectiveness of the spectrum–effect relationship analysis method, which were confirmed to effectively predict the active compounds in the complicated matrix.
In this study, a comprehensive filtering approach and spectrum–effect relationship were applied to discover the bioactive components in ZN (Figure 1). First, based on the mass defect filtering-based structure classification (MDFSC) and diagnostic fragment-ion-based extension (DFIBE) approaches, the chemical compounds of ZN were rapidly profiled by the ultrahigh-performance liquid chromatography-quadrupole time-of-flight mass spectrometry (UHPLC Q-TOF MS). Second, the fingerprints of 20 batches of ZN samples were established by high-performance liquid chromatography (HPLC), followed by similarity analysis (SA) and hierarchical cluster analysis (HCA). Furthermore, different activity tests including 3-(4,5-dimethylthiazol-2-yl)- 2,5-diphenyltetrazolium bromide (MTT) test, NO production assay, and the 2,2-diphenyl-1-picrylhydrazyl (DPPH) assay were determined, respectively. Finally, to discover the potential active compounds, the spectrum–effect relationship was modeled by chemometrics such as the partial least squares regression (PLSR), gray relational analysis (GRA), and Spearman’s rank correlation coefficient (SRCC).
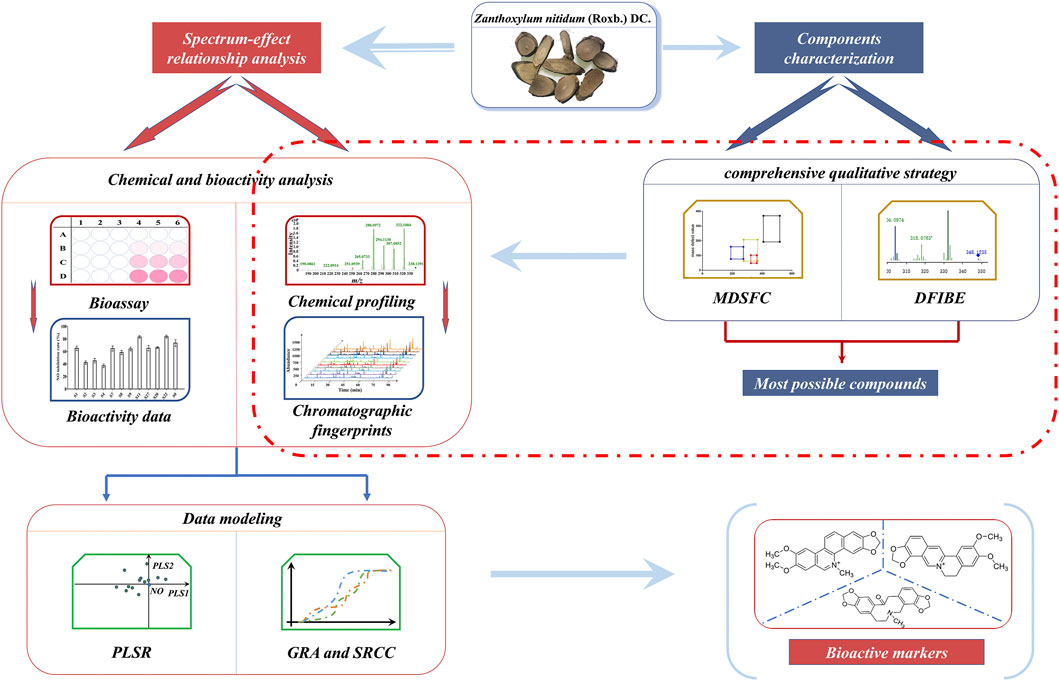
FIGURE 1. The strategy for discovering bioactive markers in Zanthoxylum nitidum (Roxb.) DC. (ZN) by ultrahigh-performance liquid chromatography-quadrupole time-of-flight mass spectrometry (UHPLC Q-TOF MS) coupled with comprehensive filtering approaches.
Materials and Methods
Materials and Reagents
Twenty batches of ZN samples were collected from different areas in China, which contain the resource areas that are shown in Supplementary Table S1). The voucher specimens were identified by Prof. Dong Liang from the Department of Chemistry and Pharmacy in Guangxi Normal University and were stored in the State Key Laboratory for the Chemistry and Molecular Engineering of Medicinal Resources, Guilin, China.
Liquid chromatography mass spectrometry (LC-MS)-grade acetonitrile and methanol were bought from MEDIA (Fairfield, CT, United States). Formic acid was HPLC grade and purchased from Aladdin (Shanghai, China). Chelerythrine, diosmin, hesperidin, nitidine chloride, sanguinarine, magnoflorine, and dihydrochelerythrine, all with purity of ≥98%, were obtained from Chengdu Push Bio-Technology (Chengdu, China). Deionized water was purified using a Milli-Q water purification system (Millipore, United States). Dulbecco’s modified Eagle’s medium (DMEM) was produced by Gibco (Grand Island, NE, United States), and DPPH was produced by Tokyo Chemical Industry (Tokyo, Japan).
Preparation of Zanthoxylum nitidum (Roxb.) DC. Extracts
After being ground into powder and air-dried (filtered with a 50-mesh sieve), each ZN sample (5 g) was dissolved with 100 ml of 80% ethanol and extracted three times at 3, 2, and 1 h on a water bath at 80°C. Then a Rotavapor OSB-2200 from EYELA Co. (Tokyo, Japan) was used to remove the solvent. The extracts were powdered by an FD5-series vacuum freeze dryer (GOLD SIM, Newark, NJ, United States) and then stored for use.
Chromatographic and Mass Spectrometric Condition
Chemical Profiling of Zanthoxylum nitidum (Roxb.) DC. Sample by UHPLC Q-TOF MS
The comprehensive characterization of ZN samples was conducted on an Agilent 6545 UHPLC Q-TOF MS (Agilent Technologies, Santa Clara, CA, United States) with the monitoring of Agilent LC-QTOF/MS Mass Hunter Workstation Acquisition Software Version B.05.01 (Agilent Technologies, Santa Clara, CA, United States). Tested samples were separated on an Agilent Zorbax clipse Plus C18 column (2.1 × 50 mm, 1.8-μm, Agilent Corp., Santa Clara, CA, United States). The mobile phase consisted of acetonitrile (Santa Clara, CA, United States). The mobile phases were 0.1% formic acid (A) and acetonitrile (B) with the following gradient elutions: 5–15% B linear 0–3 min, 15–20% B linear 3–6 min, 20–22% B linear 6–7 min, 22% B isocratic 7–9 min, 22–26% B linear 9–12 min, 26–28% B linear 12–13 min, 28% B isocratic 13–15 min, 28–34% B linear 15–16 min, 34–38% B linear 16–18 min, 38–40% B linear 18–20 min, 40–55% B linear 20–24 min, 55–65% B linear 24–25 min, 65–85% B linear 25–28 min, and 85–95% B linear 28–30 min. The injection volume and the detection wavelength were set as 0.6 μl and at 254 nm, respectively. The flow rate was 0.6 ml/min, and the column temperature was maintained at 35°C.
Both MS and MS/MS were performed in positive ion mode, and the ion source is dual AJS ESI. The MS parameters were set as follows: capillary voltage, 3,500 V; nebulizer gas (N2) pressure, 30 psig; drying gas flow rate, 8.0 L/min; drying gas (N2) temperature, 320°C; shealth gas flow rate, 12.0 L/min; shealth gas (N2) temperature, 350°C; OCT RF V, 750 V; skimmer, 65 V; fragmentor, 135 V. The scan ranges for product ions were m/z 100–3,000, and the collision energy was set at 20, 40, and 60 V. Before the analysis, mass spectrometer of the TOF was calibrated at m/z 121.0508 and 922.0098 in positive ion mode to ensure the mass accuracy. Data acquisition and analysis were obtained by Agilent LC-MS MassHunter Workstation Software (version B.08.00).
Chemical Fingerprint Analysis of Zanthoxylum nitidum (Roxb.) DC. Sample by HPLC
The SHIMADZU LC-20AT HPLC system was employed to perform the HPLC fingerprint analysis. The system consists of quad pump, online vacuum degassing machine, DAD detector, column temperature box, and automatic sampler (SHIMADZU LabTotal, Tokyo, Japan). Chromatographic separation was conducted on a column of symmetry columns (4.6 × 250 mm, 5 μm, Waters, Milford, CT, United States). The mobile phase consisted of water with 0.1% formic acid (A) and acetonitrile (B). The gradient conditions are shown in Supplementary Table S2. The temperature was set at 35°C. The purpose of the final 10 min is re-equilibrizing the column. The flow rate was at 1 ml/min, and the injection volume and detection wavelength were set at 10 μl and 254 nm, respectively.
Antioxidant and Anti-Inflammatory Bioactivity Assay
Antioxidant Activity Assay
In this study antioxidant activities were measured by the classic test DPPH assays. DPPH assay is a chemical analysis experiment, which is used in a basic vitro screening method for evaluating the radical scavenging activity (Heinrich et al., 2020). The radical scavenging activity (RSA) was calculated by the following formula:
where Acontrol is the absorbance of 100 μl of DPPH solution with 100 μl of ethanol, Ablank is the absorbance of 200 μl of ethanol, and Asample is the absorbance of 100 μl of DPPH solution with 100 μl of sample or ascorbic acid solution.
The DPPH assay was performed based on the instructions described in the literature (Kang et al., 2011; Wang et al., 2017; Zhang et al., 2018). An aliquot of 100 μl of each sample in ethanol (10, 25, 50, 100, 200 μg/ml) was mixed with 100 μl of 0.2 mM DPPH ethanolic solution. Ascorbic acid was used as a positive drug. The mixture was incubated for 30 min in the darkroom, then the absorbance was measured at 517 nm. The determination was conducted in triplicates, and the antioxidant activity was expressed by the IC50 value.
Anti-Inflammatory Activity Assay
Raw264.7 cells (100 μl) were seeded at 2.5 × 104 cells per well into 96-Transwell insert plates and incubated in a 5% CO2 atmosphere at 37°C. After 24 h, the powder of ZN extracts was dissolved in the new culture medium containing 1 μg/ml of LPS, configured as a solution of 80 μg/ml to replace the old culture medium. Cells were maintained in the incubator at 37°C for 24 h. The level of nitric (an indicator of NO synthesis) was measured using nitric oxide assay kit (Beyotime, Shanghai, China) according to the manufacturer’s instructions. The OD value was measured at 540 nm with a microplate reader (BioTek, SynergyH1, United States). The calculation formula of inhibition rate of NO production is as follows:
Cm is the NO concentration of the model group, Cs is the NO concentration of the samples, and Cn is the NO concentration of the normal control group. Finally, the original cells in 96-well plates were used to determine the cell viability by an MTT assay (Wang et al., 2019).
Spectrum–Effect Relationship Modeling
Establishing of HPLC Fingerprint and Hierarchical Cluster Analysis
Twenty batches of ZN samples were chemically profiled and matched automatically by using the “Chinese Medicine Chromatographic Fingerprint Similarity Evaluation System 2012.” Then the common peaks of 20 fingerprints were calculated by the multipoint method, and the control chromatogram was generated automatically by the average method.
The HCA of different batches of ZN samples was carried out with the SPSS statistics software (SPSS version 19.0, IBM Corp., Armonk, NY, United States). The between-group linkage method and the squared Euclidean distance were used to establish the clusters.
Partial Least Squares Regression Model
PLSR is the statistical method, which is related to principal component regression, but is not a hyperplane that looks for the maximum variance between the response variable and the independent variable (Qiao et al., 2018). It is helpful in classification and biomarker discovery. The model is a multivariate calibration model used to find the inner relationship between an n × p data matrix X and an n × q response matrix Y (Martens and Naes, 1992). In this study, PLSR was used to model the fingerprint–activity relationship based on the SIMCA-P 14.0 Software (Umetrics AB, Umea, Sweden). The X-matrix was composed of the common peak areas in chromatographic fingerprints, and the Y-matrix was constructed with the anti-inflammatory and antioxidant activities.
where
Grey Relational Analysis Model
The Grey relational analysis is often used to measure the degree of correlation among factors according to the degree of similarity or dissimilarity between the development trends of factors, which is also called “grey correlation degree” (Elena Arce et al., 2015; Jiang et al., 2018). The anti-inflammatory and antioxidant activities of 20 ZN samples were selected as the reference sequence, and 18 relative common peaks were defined as comparability sequences. Then the grey relational coefficients (GRC) between common peaks and bioactivities were conducted through the Data Processing System (DPS 9.01) software. The formula for calculation relational degree is as follows:
where
Spearman’s Rank Correlation Coefficient
Spearman’s rank correlation coefficient (ρ) is a widely used method to measure the degree of correlation between two variables. A positive correlation coefficient value of ρ (from 0 to 1) implies that the two variables are positively correlated. On the contrary (from −1 to 0), there is a negative correlation. Besides, a correlation coefficient value of 0 implies that the two variables are not related (Zar, 2005).
In this study, Spearman’s rank correlation coefficient was used to quantify the correlation between 18 common peaks and bioactivities by using SPSS version 19.0 (IBM Corp., Armonk, NY, United States). The formula for the calculation of the relational degree is as follows:
where xi is the rank of the data of the random variable X, yi is the rank of the data of the random variable Y, and
Results and Discussion
Chemical Identification of Zanthoxylum nitidum (Roxb.) DC. Sample
Establishment of the Qualitative Strategy
In TCMs, the same chemical structure compounds can be classified as a category with a unique mother skeleton. These compounds with similar structure group share identical diagnostic ions and mass defect value, which could make the structural identification more efficient (Zhang et al., 2008; Zhang et al., 2009). In this study, a structure-diagnostic ion-oriented network (Figure 2A) was established for the rapid characterization of alkaloids compounds in ZN: 1) The mass fragment behaviors of the compounds in ZN were summarized by referring to the existing reviews and database (Sci-finder, Google Scholar, and PubMed). 2) Total ions and product ion scan were used to create the comprehensive chemical profiling of ZN (Figures 2B,C). 3) Then the mass defect filtering-based structure classification (MDFSC) and diagnostic fragment-ion-based extension (DFIBE) were applied to process the MS data. 4) With the help of the classification method, most alkaloid compounds in ZN were rapidly identified by their mass fragmentation rules. A diagram for rapid classification and identification of the chemical compounds in ZN is displayed in Figure 2.
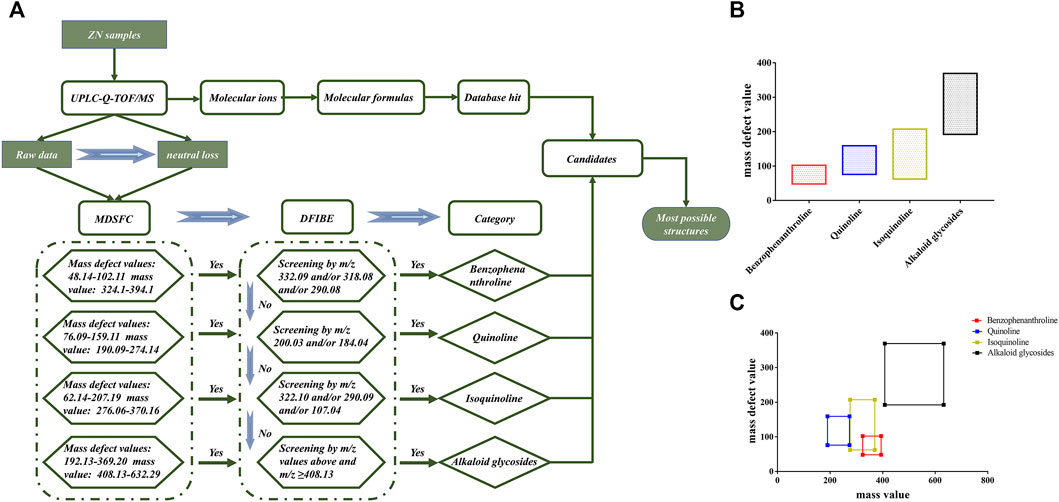
FIGURE 2. The structure-diagnostic ion-oriented network (A). The mass defect ranges of different types of alkaloids in ZN (B); the correlations between the mass ranges and mass shifts of the basic skeletons of ZN alkaloids (C). MDFSC, mass defect filtering-based structure classification; DFIBE, diagnostic fragment-ion-based extension.
MDFSC Approach for Structural Classification
With a well-preset mass defect window, the MDFSC approach can quickly find out the class of compound based on their similar mother skeleton over certain mass ranges, and can also obtain new compounds never found before (He et al., 2018; Zhou et al., 2018; Pang et al., 2019). Considering that alkaloids are just one part of compounds in ZN, the MDFSC strategy was only a preliminary structural classification for alkaloids in the whole identification of ZN.
On the basis of existing literatures (Jia et al., 2013; Yang et al., 2017; Fu et al., 2021) and authoritative database, we concluded the core substructures and corresponding substituents in ZN, and calculated their maximum and minimum mass defect values (the tolerance of mass defect is ±5 m Da). In total, the alkaloids can be divided into four categories: benzophenanthroline, quinoline, isoquinoline, and alkaloid glycosides. Respectively, mass defect values and mass values of these four categories are listed as follows: benzophenanthroline (mass defect values 48.14–102.11 m Da, mass values: 324.10–394.10 m Da), quinoline (mass defect values: 76.09–159.11 m Da, mass values: 190.09–274.14 m Da), isoquinoline (mass defect values: 62.14–207.19 m Da, mass values: 276.06–370.16 m Da), and alkaloid glycosides (mass defect values: 192.13–369.20 m Da, mass values: 408.13–632.29 m Da). Using the MDFSC approach, we could preliminarily sort part of the detected ions as the certain chemical homologs and discovered that some alkaloid glycosides have mother skeletons, which are compounds classified in the former three categories. For example, the mother skeleton of compound 39 was similar to jatrorrhizine; its MS/MS spectra had fragment peaks of 338.1390, 322.1077, and 294.1123 m/z, which correspond to jatrorrhizine.
Structure Classification and Identification of Alkaloids in Zanthoxylum nitidum (Roxb.) DC. Sample
Combined with the MDFSC approach and DFIBE, the structure-diagnostic ion-oriented strategy was employed to rapidly speculate the chemical constituents, and in total, 48 compounds were inferred from ZN. Among them, alkaloids accounted for 35 of the 48, which indicate the significance of alkaloids. All the detailed information is listed in Table 1, and the fragmentation pattern together with mass spectrogram of representative compounds are shown in Figure 3.
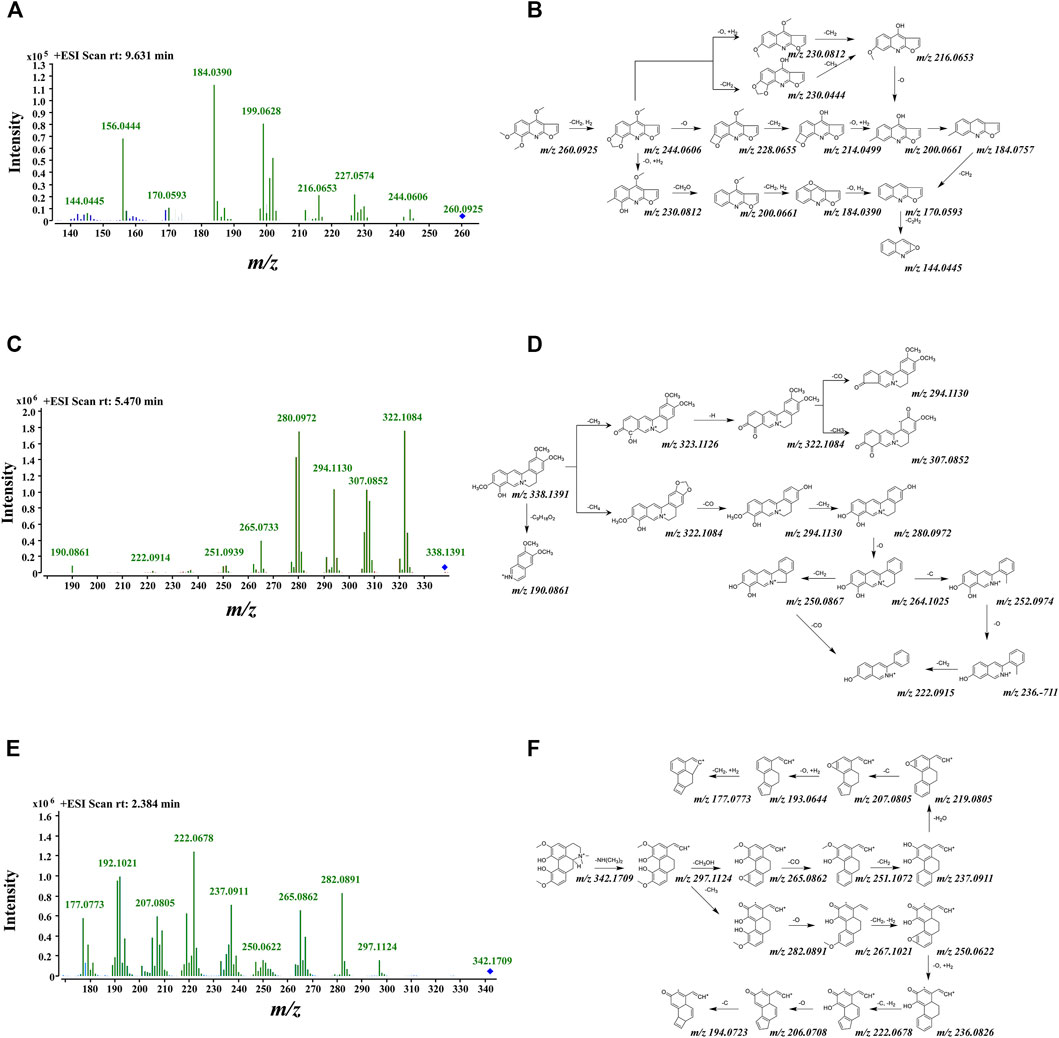
FIGURE 3. Tentative fragmentation pathway and mass spectrogram of several typical alkaloids in ZN. (A,B) Skimmianine; (C,D) jatrorrhizine; (E,F) magnoflorine.
Characterization of Typical Benzophenanthroline
For benzophenanthrolines, their basic carbon framework consists of a benzene and a phenanthridine. Benzophenanthrolines are the most studied category of compounds in ZN, such as NC and chelerythrine (Li et al., 2017; Lin et al., 2020). In the MS/MS spectra of benzophenanthrolines, some certain product ions at m/z 332.09, 318.08, and 290.08 were presented, and consecutive losses of CH2 (14 Da), CO (28 Da), and O (16 Da) could be easily found. Nitidine ([M]+ at m/z 348.1246) is the typical compound, eluted at 8.71 min, and its molecular formula was determined as C21H18NO4+. In the positive MS/MS spectra, its MS/MS spectra had fragment peaks of 332.0926 [M-O]+ and 318.0763 [M-O-CH2]+, which correspond to the core substructures of benzophenanthrolines. Its tentative fragmentation pathway is shown in Supplementary Figures S1A,B. By integrating data inquiries, MDFSC and DFIBE approaches, nitidine, oxynitidine, and dihydronitidine were confirmed (Cesar et al., 2015).
Characterization of Typical Quinoline
Quinoline consists of a benzene connecting to pyridine and other groups. The product ions of [M+H−14]+, [M+H−30]+, and [M+H−16]+ were available in the MS/MS spectra of quinoline, and certain product ions at m/z 200.03, 184.04 were the basis of DFIBE judgment. According to the patterns above, skimmianine ([M+H]+ at m/z 260.0925) was identified rapidly. It was eluted at 9.63 min, and its molecular formula was determined as C14H13NO4. It had fragment peaks of 200.0661[M+H-CH2+H2-O+H2-CH2O]+ and 184.0390 [M+H-CH2+H2-O+H2-CH2O-CH2+H2]+, which were equal to the core substructures. Then the skimmianine was identified with the support of integrating data bases (Wang, 1980), and its tentative fragmentation pathway is shown in Figures 3A,B. Besides, γ-fagarine and dictamnine are inferred as quinoline alkaloids.
Characterization of Typical Isoquinoline
For isoquinoline, the basic carbon framework consists of a phenanthridine connecting to pyridine. Its MS/MS spectra provided several certain product ions at m/z 322.10, 290.09, and 107.04, and consecutive losses of CH2 (14 Da), C (12 Da), CO (28 Da), and O (16 Da) could be easily found (Xiao et al., 2018). The typical compound is jatrorrhizine ([M+H]+ at m/z 338.1400), which was eluted at 5.47 min, and its main molecular formula was determined as C20H20NO4+. Its fragment peaks contain 322.1091 [M+H-CH4]+, 294.1130 [M+H-CH4-CO]+, and 280.0972 [M+H-CH4-CO-CH2]+. After integrating data bases, jatrorrhizine was confirmed. During the whole characterization of isoquinoline, we found that the overall structure and fragmentation pathway of magnoflorine is very interesting. Tentative fragmentation pathways of jatrorrhizine and magnoflorine are shown in Figures 3C–F.
Characterization of Typical Alkaloid Glycosides
With the assistance of MDFSC and DFIBE approaches, several alkaloid glycosides and alkaloids of larger relative molecular weights were found in ZN. It is attractive that the mother nucleuses of these compounds are similar to the known alkaloids we discovered. Take compound 40, for example; its mother nucleus is nitidine, which is described in the previous section, and its tentative fragmentation pathway is shown in Supplementary Figures S1A,B, and the tentative fragmentation pathways of oxynitidine and dihydronitidine are shown in Supplementary Figures S1C–F. Unfortunately, we could not suspect the accurate structure of these compounds and the attached groups.
Characterization of Other Types of Compounds
Several alkaloids do not belong to the above four categories or cannot be found in medium to low polarity, such as magnocurarine B ([M]+ at m/z 314.1760). It is a benzylisoquinoline alkaloid, and is eluted at 3.14 min in high polarity. Its fragment peaks contain 269.1174 [M+H-C2H8N]+, 237.0909 [M+H-C3H12NO]+, 107.0489 [M+H-C12H18NO2]+, etc. After integrating the databases and DFIBE approach, magnocurarine B was identified. Its tentative fragmentation pathway is shown in Supplementary Figures S2A,B. This result indicates that the MDFSC and DFIBE approaches cannot only be applied in the characterization of alkaloids but also can be used in other types of compounds. Finally, the tentative fragmentation pathways of all remaining identified compounds are sorted out in Supplementary Figures S4–S45.
Chromatographic Fingerprint Analysis of Zanthoxylum nitidum (Roxb.) DC. Samples
Under the optimized experimental conditions, the chromatographic fingerprints of ZN samples are neatly lined up in Figure 4. To evaluate the validation of the HPLC method, we selected and analyzed a random sample (S18) for its validation tests in terms of precision, stability, and repeatability. The RSDs of precision, stability, and repeatability were all less than 5% (shown in Supplementary Table S3), which indicated that the established method was reliable and repeatable.
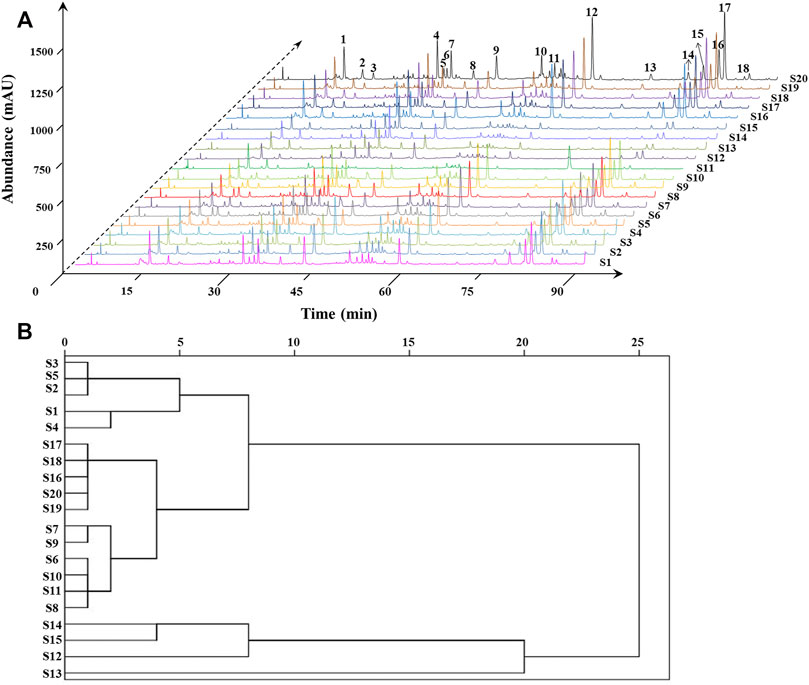
FIGURE 4. HPLC fingerprints and common peaks of 20 batches of ZN samples (A). Cluster analysis of 20 batches of ZN samples (B).
Then the fingerprint of 20 batches of ZN samples was generated automatically by the software Similarity Evaluation System for Chromatographic Fingerprint of Traditional Chinese Medicine (2012A Version, Committee of Chinese Pharmacopeia). HPLC fingerprints were provided in Figure 4A, and reference spectra are shown in Supplementary Figure S3, respectively. Eighteen common characteristic peaks were chosen as research object, which covered more than 80% of the whole peak areas in the fingerprint. Subsequently, we performed the similarity analysis to evaluate the similarity of all the chromatographic profile of the samples, which was based on vector cosine calculations. It could be noted that most of similarity values were higher than 0.90, while similarity values of several batches from Guangdong province were 0.81–0.89 (Supplementary Table S1). The results showed that the chemical fluctuation among the random samples is pretty small in major producing areas (Sun and Liu, 2007), and it proved that the established fingerprint was reliable.
Hierarchical Cluster Analysis of Zanthoxylum nitidum (Roxb.) DC. Samples
Based on the SPSS 19.0 software, we took the relative area of each common peak as the index for HCA (Liu et al., 2016). The sample similarity was measured by correlation coefficient as distance. The results are shown in Figure 4B, and 20 batches of ZN samples could be grouped into six categories: S1–S5, S6–S11 and S16–S20, S14–S15, S12, and S13 were, respectively, classified to one group. More importantly, the six groups belonged to the four resource provinces, and the group contains S16–S20 was from Guangxi with good similarity and intragroup similarity. The results indicated that ZN from different areas have good stability.
Bioactivities of Zanthoxylum nitidum (Roxb.) DC. Samples
Anti-Inflammatory Activity of Zanthoxylum nitidum (Roxb.) DC. Samples
The inhibition of NO production is positively correlated with the anti-inflammatory activity (Chen et al., 2019). Thus, the inhibition of NO production was set as the index to assess the anti-inflammatory property of ZN samples in the present study. The results showed that cell viability (Supplementary Table S4) was from 77.4 ± 2.4 to 107 ± 1.3%, which means ZN inhibits the production of NO while not producing a toxic effect in vitro. Moreover, as is shown in Supplementary Table S4, the range of NO inhibition rate was 37.1 ± 2.9 to 83.9 ± 2.2%, and S10 (83.2 ± 2.3%), S11 (81.8 ± 1.7%), S12 (83.9 ± 3.8%), and S19 (83.9 ± 2.2%) were higher than 80% (Figure 5A). Among them, the sample S19 from Guangxi province showed best inhibition of NO production (83.9 ± 2.2%). The data indicated that most batches of ZN samples from different areas showed better anti-inflammatory activity.
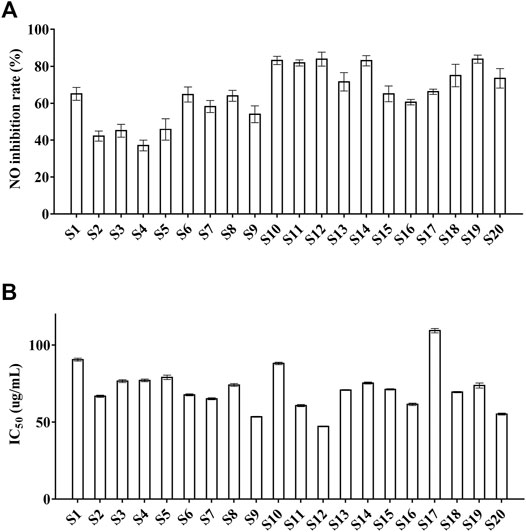
FIGURE 5. The NO inhibition and the antioxidant activities of 20 batches of ZN samples. (A) NO inhibition; (B) DPPH. Values represent means ± SD, n = 5 (A); n = 3 (B).
Antioxidant Activity of Zanthoxylum nitidum (Roxb.) DC. Samples
The IC50 value is the indicator to evaluate the antioxidant property of ZN samples, and it is negatively correlated with the antioxidant activity (Wang et al., 2017). As was shown in Supplementary Table S5, the IC50 values were from 47.21 ± 0.33 to 109.34 ± 1.43 μg/ml, and S10 (53.48 ± 0.29 μg/ml), S12 (47.21 ± 0.33 μg/ml), and S20 (55.22 ± 0.64 μg/ml) were lower than 60 μg/ml (Figure 5B). Besides, the sample S12, S20 showed strong antioxidant activity (47.21 ± 0.33 μg/ml; 55.22 ± 0.64 μg/ml), whereas the antioxidant activity of S17 (109.36 ± 1.43 μg/ml) was weak. The results revealed that most batches of ZN samples have better antioxidant activity.
Discovery of Principal Bioactive Components by Partial Least Squares Regression, Gray Relational Analysis, and Spearman’s Rank Correlation Coefficient
In the past decades, artemisinin and ephedrine were regarded as gifts that the TCMs bring to the world (Tu, 2016; Leung and Xu, 2020). They were both isolated substances from TCMs and have undergone the modernization of traditional medicines. It proved that we can clarify the effectiveness of TCMs by finding out the bioactive components, but this is desperately not enough; the efficacy of TCMs does not come from a single compound, and TCMs usually consists of hundreds of components (Ma et al., 2016; Zhang et al., 2019). Thus, the search of the biological constituents of TCMs are a complex task. To solve this problem, the spectrum-effect relationship analysis might be a suitable choice. In this study, stoichiometric methods (PLSR, GRA, and SRCC) were applied to rapidly discover bioactive compounds from ZN samples.
First, the results of GRA showed that the GRC between the relative contents of 18 common peaks and anti-inflammatory activity were in the range of 0.5691–0.8139 (Figure 6G). Peaks 1, 2, 4, 6, 7, and 10 showed significant influence to anti-inflammatory activity of ZN, and peaks 16, 11, 15, 8, 9, 17 showed influence to anti-inflammatory activity of ZN. The contribution order of chromatographic peaks to the anti-inflammatory activity was P6 > P7 > P2 > P1 > P10 > P4 > P16 > P11 > P15 > P8 > P9 > P17. Similarly, GRC between the relative contents of 18 common peaks and antioxidant activity were in the range of 0.5908–0.8520 (Figure 6G). Peaks 2, 3, 4, 7, 11, 12, 13, 14, and 17 showed an important effect to antioxidant activity of ZN, and peaks 5, 10, 15, and 16 showed influence to antioxidant activity of ZN. The contribution order of chromatographic peaks to the antioxidant activity was P2 > P4 > P13 > P11 > P3 > P17 > P14 > P12 > P7 > P16 > P15 > P10 > P5.
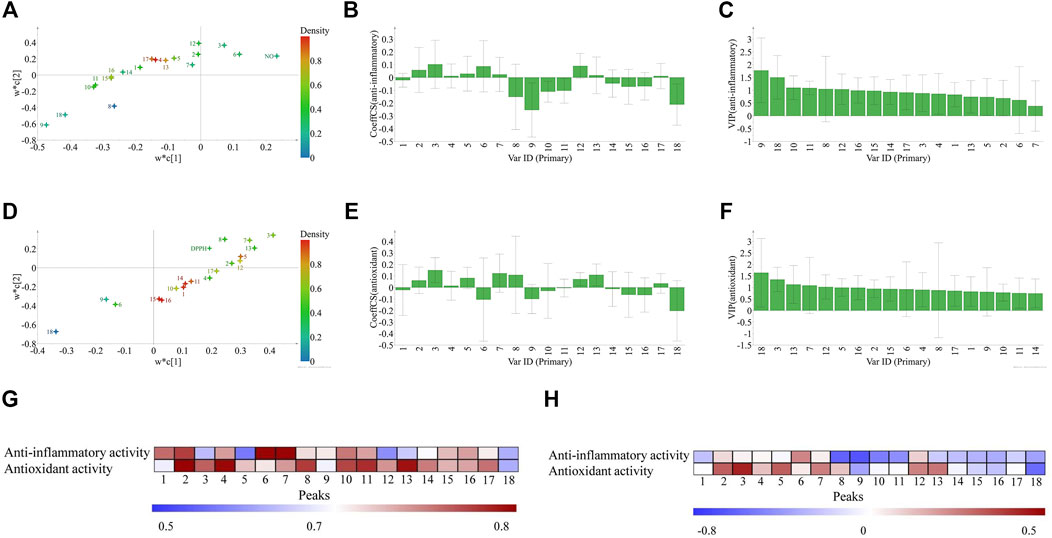
FIGURE 6. The three pharmacodynamic models to discover principal bioactive components. The results of partial least squares regression (PLSR, A–F): the loading scatters of PLSR (A: anti-inflammatory; D: antioxidant, colored according to density); regression coefficient between the 18 common peaks and the anti-inflammatory, antioxidant activities, respectively (B: anti-inflammatory; E: antioxidant), and VIP values (C: anti-inflammatory; F: antioxidant). The heat map of bioactivities of 18 compounds by GRA analysis (G) and Spearman's rank correlation coefficient (SRCC) (H) in ZN samples. Peaks 1–18 represent the common peaks.
Second, the PLSR model was used to discover the potential active compounds by correlating the fingerprint chromatographic data, antioxidant or anti-inflammatory activities. The X matrix of dimensions (20 × 18) from common peak areas and the Y matrix of DPPH and the NO inhibition activities were used in this model (Figure 5 and Supplementary Tables S4, S5. The R2 of NO inhibition is 0.764, and R2 of DPPH is 0.762, respectively). Then the PLSR loading scatters were performed (Figures 6A,D), where Y-variables are situated near the X-variables and positively correlated to them.
For the anti-inflammatory activity of ZN samples, we found that peaks 2, 3, 4, 5, 6, 7, 12, 13, and 17 were positively correlated to the anti-inflammatory activity (Figure 6B). Furthermore, we employed the parameter VIP to screen the variables responsible for the anti-inflammatory activity. Variables above the VIP value threshold of 1.0 were filtered out as candidate bioactive compounds. Among them, peaks 4 (nitidine), 12, and 17 were screened out and considered as the potential anti-inflammatory compounds of ZN (Figure 6C). For the antioxidant activity of ZN samples, similarly, peaks 3, 5 (chelerythrine), 7 (hesperidin), 12 (oxynitidine), and 13 appeared in a cluster trend. These compounds were positively correlated to the antioxidant activity, and the VIP value was above 1.0 (Figures 6E,F). According to the above results, we preliminarily inferred that the peaks 3, 4, 5, 7, 12, 13, and 17 were more relevant to the measured activities.
Finally, the results of SRCC (shown in Figure 6H) indicated that peaks 2, 3, 4, 6, 7, and 12 showed an influence to the anti-inflammatory activity of ZN, and peaks 2, 3, 5, 7, 12, and 13 have an important influence on the antioxidant activity of ZN.
By combining the results of PLSR, GRA, and SRCC, it can be concluded that peaks 4, 12, and 17 are tentatively assigned as candidate ingredients accounting for the anti-inflammatory activity, and peaks 3, 5, 7, 12, and 13 are the candidate ingredients for antioxidant activity. Among them, owning to the high content and bioactive activities in ZN, nitidine has attracted much attention. It has been found that nitidine could inhibit LPS-induced TNF alpha, IL-1beta, and IL-6 production by the inhibition of phosphorylation of MAPK in the RAW264.7 cell test (Wang et al., 2012). Taken together, nitidine might be the main anti-inflammatory component in ZN. What is more, peaks 12 and 17 might also be the potential anti-inflammatory compounds of ZN, which warrant further investigation. Among the antioxidant candidate compounds, hesperidin was identified to have an oxidative damage protective effect against γ-radiation-induced tissue damage in Sprague–Dawley rats (Pradeep et al., 2012). Additionally, peaks 3, 12, and 13 might also be the potential antioxidant compounds of ZN. As a complicated system, the potential synergistic and/or antagonism effects arising from multicomponents in ZN must be taken into consideration. Thus, it could be found that peaks 3, 4, 5, 7, 12, 13, and 17 might be the main bioactive compounds in ZN samples, and among them, peaks 4, 12, and 17 were the principal anti-inflammatory components and peaks 3, 5, 7, 12, and 13 were regarded as the principal antioxidant components, respectively.
Verification of Bioactive Compound Activities
In order to confirm the reliability of the correlation analysis, the anti-inflammatory activity of nitidine and the antioxidant activity of chelerythrine and hesperidin were determined by the antioxidant and anti-inflammatory bioactivity assays. The result of nitidine (Supplementary Figure S46A) showed that the IC50 value of nitidine is 87.241 ± 1.752 μM, which indicated that nitidine might be the main anti-inflammatory component of ZN. The results of chelerythrine and hesperidin (Supplementary Figures S46B,C) showed that chelerythrine has an antioxidant activity, and hesperidin showed a weaker antioxidant activity, which indicated that chelerythrine and hesperidin might be the main antioxidant components of ZN. Besides, according to the results of the correlation analysis, it could be noticed that diosmin has no antioxidant activity, which is in accordance with the results of these previous studies (Khlebnikov et al., 2007; Naso et al., 2016), and all these results verified the reliability of the correlation analysis.
Conclusion
In order to solve the challenge of discovering bioactive compounds in ZN, a comprehensive filtering approach and a spectrum–effect relationship were performed in our work. A total of 48 compounds were identified from ZN by the structure-diagnostic ion-oriented network, and 35 of them were alkaloids. During the identification of chemical components in ZN by integrating the MDFSC and DFIBE approaches, some new alkaloid analogs were found, such as compounds 37, 39, 40, and 48. Meanwhile, we discovered the principal bioactive components of ZN by using the GRA, PLSR, and SRCC models. Among the bioactive compounds, peaks 4 (nitidine), 12 (oxynitidine), and 17 might be the potential anti-inflammatory compounds in ZN, and peaks 3, 5 (chelerythrine), 7 (hesperidin), 12, and 13 might contribute to the antioxidant activity of ZN. Our study not only revealed the plant metabolites of anti-inflammatory and antioxidant activities of ZN, but it could also be an applicable data support for the discovery of active components of other TCMs. Our future studies will perform more comprehensive and reliable methods to verify the anti-inflammatory and antioxidant activities of ZN in vitro and in vivo.
Data Availability Statement
The original contributions presented in the study are included in the article/Supplementary Material, further inquiries can be directed to the corresponding authors.
Author Contributions
S-WR conducted the analysis of the experiment and data analysis, and contributed to the manuscript writing. Y-YD conducted the pharmacological and antioxidant experiment and manuscript revision. WS supervised the project and designed the study. S-HX, H-QP, and S-QH participated in the related auxiliary experiments. K-GC and DL guided the experiment. All authors read and approved the manuscript.
Funding
This work was supported by the BAGUI Young Scholar Program of Guangxi, the State Key Laboratory for Chemistry and Molecular Engineering of Medicinal Resources (CMEMR2018-A04), the Basic Ability Promotion Project for Young and Middle-aged Teachers of Guangxi Universities (No. 2020KY02036), and the Guangxi Natural Science Foundation of China (No. 2020GXNSFBA297085).
Conflict of Interest
The authors declare that the research was conducted in the absence of any commercial or financial relationships that could be construed as a potential conflict of interest.
Publisher’s Note
All claims expressed in this article are solely those of the authors and do not necessarily represent those of their affiliated organizations or those of the publisher, the editors, and the reviewers. Any product that may be evaluated in this article, or claim that may be made by its manufacturer, is not guaranteed or endorsed by the publisher.
Supplementary Material
The Supplementary Material for this article can be found online at: https://www.frontiersin.org/articles/10.3389/fphar.2022.794277/full#supplementary-material
References
Cesari, I., Grisoli, P., Paolillo, M., Milanese, C., Massolini, G., and Brusotti, G. (2015). Isolation and Characterization of the Alkaloid Nitidine Responsible for the Traditional Use of Phyllanthus Muellerianus (Kuntze) Excell Stem Bark against Bacterial Infections. J. Pharm. Biomed. Anal. 105, 115–120. doi:10.1016/j.jpba.2014.11.051
Chen, G. L., Fan, M. X., Wu, J. L., Li, N., and Guo, M. Q. (2019). Antioxidant and Anti-inflammatory Properties of Flavonoids from lotus Plumule. Food Chem. 277, 706–712. doi:10.1016/j.foodchem.2018.11.040
Chen, Y. H., Bi, J. H., Xie, M., Zhang, H., Shi, Z. Q., Guo, H., et al. (2021). Classification-based Strategies to Simplify Complex Traditional Chinese Medicine (TCM) Researches through Liquid Chromatography-Mass Spectrometry in the Last Decade (2011-2020): Theory, Technical Route and Difficulty. J. Chromatogr. A. 1651, 462307. doi:10.1016/j.chroma.2021.462307
China Pharmacopoeia Commission (2020). Chinese Pharmacopeia. 2020 Edition, I. Beijing: China Medical Science Press, 176–177.
Elena Arce, M., Saavedra, Á., Míguez, J. L., and Granada, E. (2015). The Use of Grey-Based Methods in Multi-Criteria Decision Analysis for the Evaluation of Sustainable Energy Systems: A Review. Renew. Sust. Energ. Rev. 47, 924–932. doi:10.1016/j.rser.2015.03.010
Fu, J. L., Yang, L. M., Fan, X. Y., Guo, Q. O., Zhou, W. M., and Zhang, J. Y. (2021). Research Progress on Chemical Constituents and Pharmacological Activities of Zanthoxylum Nitidum (Roxb.) DC. Acta Pharm. Sin. 1, 39. doi:10.16438/j.0513-4870.2020-1718
He, M., Jia, J., Li, J., Wu, B., Huang, W., Liu, M., et al. (2018). Application of Characteristic Ion Filtering with Ultra-high Performance Liquid Chromatography Quadrupole Time of Flight Tandem Mass Spectrometry for Rapid Detection and Identification of Chemical Profiling in Eucommia Ulmoides Oliv. J. Chromatogr. A. 1554, 81–91. doi:10.1016/j.chroma.2018.04.036
Heinrich, M., Appendino, G., Efferth, T., Fürst, R., Izzo, A. A., Kayser, O., et al. (2020). Best Practice in Research - Overcoming Common Challenges in Phytopharmacological Research. J. Ethnopharmacology 246, 112230. doi:10.1016/j.jep.2019.112230
Jia, C. P., Huang, X. L., Li, Y., and Feng, F. (2013). HPLC-DAD/ESI-Q-TOF-MS Analysis of Alkaloids in Zanthoxylum Nitidum (Roxb.) DC. China J. Chin. Mater. Med. 38 (8), 1198–1202. doi:10.4268/cjcmm20130816
Jiang, Y., David, B., Tu, P., and Barbin, Y. (2010). Recent Analytical Approaches in Quality Control of Traditional Chinese Medicines-Aa Review. Anal. Chim. Acta 657, 9–18. doi:10.1016/j.aca.2009.10.024
Jiang, Z., Zhao, C., Gong, X., Sun, X., Li, H., Zhao, Y., et al. (2018). Quantification and Efficient Discovery of Quality Control Markers for Emilia Prenanthoidea DC. By Fingerprint-Efficacy Relationship Modelling. J. Pharm. Biomed. Anal. 156, 36–44. doi:10.1016/j.jpba.2018.04.020
Kang, W.-Y., Song, Y.-L., and Zhang, L. (2011). α-Glucosidase Inhibitory and Antioxidant Properties and Antidiabetic Activity of Hypericum Ascyron L. Med. Chem. Res. 20, 809–816. doi:10.1007/s00044-010-9391-5
Khlebnikov, A. I., Schepetkin, I. A., Domina, N. G., Kirpotina, L. N., and Quinn, M. T. (2007). Improved Quantitative Structure-Activity Relationship Models to Predict Antioxidant Activity of Flavonoids in Chemical, Enzymatic, and Cellular Systems. Bioorg. Med. Chem. 15, 1749–1770. doi:10.1016/j.bmc.2006.11.037
Leung, E. L., and Xu, S. (2020). Traditional Chinese Medicine in Cardiovascular Drug Discovery. Pharmacol. Res. 160, 105168. doi:10.1016/j.phrs.2020.105168
Li, W., Yin, H., Bardelang, D., Xiao, J., ZhengRuibing, Y. W., and Wang, R. (2017). Supramolecular Formulation of Nitidine Chloride Can Alleviate its Hepatotoxicity and Improve its Anticancer Activity. Food Chem. Toxicol. 109, 923–929. doi:10.1016/j.fct.2017.02.022
Lin, Q., Ma, C., Guan, H., Chen, L., Xie, Q., Cheng, X., et al. (2020). Metabolites Identification and Reversible Interconversion of Chelerythrine and Dihydrochelerythrine In Vitro/In Vivo in Rats Using Ultra-performance Liquid Chromatography Combined with Electrospray Ionization Quadrupole Time-Of-Flight Tandem Mass Spectrometry. J. Pharm. Biomed. Anal. 189, 113462. doi:10.1016/j.jpba.2020.113462
Liu, H., Feng, J., Feng, K., and Lai, M. (2014). Optimization of the Extraction Conditions and Quantification by RP-LC Analysis of Three Alkaloids in Zanthoxylum Nitidum Roots. Pharm. Biol. 52 (2), 255–261. doi:10.3109/13880209.2013.826244
Liu, X., Huang, C. Y., Yang, Y. F., Xu, Y. F., and Zhao, J. G. (2016). HPLC Fingerprint of Dan'e Fukang Decocted Extract Based on Hierarchical Clus. Chin. Med. Sci. J. 47 (11), 1388–1393. doi:10.16522/j.cnki.cjph.2016.11.011
Lu, Q., Ma, R., Yang, Y., Mo, Z., Pu, X., and Li, C. (2020). Zanthoxylum Nitidum (Roxb.) DC: Traditional Uses, Phytochemistry, Pharmacological Activities and Toxicology. J. Ethnopharmacol. 260, 112946. doi:10.1016/j.jep.2020.112946
Ma, X., Lv, B., Li, P., Jiang, X., Zhou, Q., Wang, X., et al. (2016). Identification of "multiple Components-Multiple Targets-Multiple Pathways" Associated with Naoxintong Capsule in the Treatment of Heart Diseases Using UPLC/Q-TOF-MS and Network Pharmacology. Evid. Based Complement. Alternat Med. 2016, 9468087. doi:10.1155/2016/9468087
Naso, L., Martínez, V. R., Lezama, L., Salado, C., Valcarcel, M., Ferrer, E. G., et al. (2016). Antioxidant, Anticancer Activities and Mechanistic Studies of the Flavone Glycoside Diosmin and its Oxidovanadium(IV) Complex. Interactions with Bovine Serum Albumin. Bioorg. Med. Chem. 24, 4108–4119. doi:10.1016/j.bmc.2016.06.053
Nguyen, T. H. V., Tran, T. T., Cam, T. I., Pham, M. Q., Pham, Q. L., Vu, D. H., et al. (2019). Alkaloids from Zanthoxylum Nitidum and Their Cytotoxic Activity. Nat. Product. Commun. 14 (5), 1934578X1984413. doi:10.1177/1934578X19844133
Pang, H. Q., An, H. M., Yang, H., Wu, S. Q., Fan, J. L., Mi, L., et al. (2019). Comprehensive Chemical Profiling of Yindan Xinnaotong Soft Capsule and its Neuroprotective Activity Evaluation In Vitro. J. Chromatogr. A. 1601, 288–299. doi:10.1016/j.chroma.2019.05.023
Pradeep, K., Ko, K. C., Choi, M. H., Kang, J. A., Chung, Y. J., and Park, S. H. (2012). Protective Effect of Hesperidin, a Citrus Flavanoglycone, against γ-radiation-induced Tissue Damage in Sprague-Dawley Rats. J. Med. Food 15, 419–427. doi:10.1089/jmf.2011.1737
Qiao, J., Wang, G., Li, W., and Li, X. (2018). A Deep Belief Network with PLSR for Nonlinear System Modeling. Neural Netw. 104, 68–79. doi:10.1016/j.neunet.2017.10.006
Qiao, R., Zhou, L., Zhong, M., Zhang, M., Yang, L., Yang, Y., et al. (2021). Spectrum-effect Relationship between UHPLC-Q-TOF/MS Fingerprint and Promoting Gastrointestinal Motility Activity of Fructus Aurantii Based on Multivariate Statistical Analysis. J. Ethnopharmacol. 279, 114366. doi:10.1016/j.jep.2021.114366
Rivera, D., Allkin, R., Obón, C., Alcaraz, F., Verpoorte, R., and Heinrich, M. (2014). What Is in a Name? the Need for Accurate Scientific Nomenclature for Plants. J. Ethnopharmacology 152, 393–402. doi:10.1016/j.jep.2013.12.022
Seidemann, J. (2005). World Spice Plants. Berlin, Heidelberg, New York: Springer-Verlag. doi:10.1007/3-540-27908-3
Sun, G., and Liu, J. (2007). Qualitative and Quantitative Assessment of the HPLC Fingerprints of Ginkgo Biloba Extract by the Involution Similarity Method. Anal. Sci. 23, 955–958. doi:10.2116/analsci.23.955
Tu, Y. (2016). Artemisinin-a Gift from Traditional Chinese Medicine to the World (Nobel Lecture). Angew. Chem. Int. Ed. Engl. 55, 10210–10226. doi:10.1002/anie.201601967
Wang, F., Wang, B., Wang, L., Xiong, Z. Y., Gao, W., Li, P., et al. (2017). Discovery of Discriminatory Quality Control Markers for Chinese Herbal Medicines and Related Processed Products by Combination of Chromatographic Analysis and Chemometrics Methods: Radix Scutellariae as a Case Study. J. Pharm. Biomed. Anal. 138, 70–79. doi:10.1016/j.jpba.2017.02.004
Wang, M. X. (1980). Study on the Chemical Components of Zanthoxylum Nitidum. J. Sun Yat-sen Univ. (Med. Sci). 1 (4), 341–349. doi:10.13471/j.cnki.J.sun.yat-sen.univ(med.Sci).1980.0065
Wang, Y. L., Zhang, Q., Yin, S. J., Cai, L., Yang, Y. X., Liu, W. J., et al. (2019). Screening of Blood-Activating Active Components from Danshen-Honghua Herbal Pair by Spectrum-Effect Relationship Analysis. Phytomedicine 54, 149–158. doi:10.1016/j.phymed.2018.09.176
Wang, Z., Jiang, W., Zhang, Z., Qian, M., and Du, B. (2012). Nitidine Chloride Inhibits LPS-Induced Inflammatory Cytokines Production via MAPK and NF-kappaB Pathway in RAW 264.7 Cells. J. Ethnopharmacol. 144, 145–150. doi:10.1016/j.jep.2012.08.041
Xiao, J., Song, N., Lu, T., Pan, Y., Song, J., Chen, G., et al. (2018). Rapid Characterization of TCM Qianjinteng by UPLC-QTOF-MS and its Application in the Evaluation of Three Species of Stephania. J. Pharm. Biomed. Anal. 156, 284–296. doi:10.1016/j.jpba.2018.04.044
Xie, P. S., and Leung, A. Y. (2009). Understanding the Traditional Aspect of Chinese Medicine in Order to Achieve Meaningful Quality Control of Chinese Materia Medica. J. Chromatogr. A. 1216, 1933–1940. doi:10.1016/j.chroma.2008.08.045
Xie, Y. F. (2000). Antioxidation Effect of Zanthoxylum Nitidum (Roxb.) DC. Extracts. Lishizhen Med. Mater. Med. Res. 11 (1), 1–2.
Yang, C. H., Cheng, M. J., Chiang, M. Y., Kuo, Y. H., Wang, C. J., and Chen, I. S. (2008). Dihydrobenzo[c]phenanthridine Alkaloids from Stem Bark of Zanthoxylum Nitidum. J. Nat. Prod. 71 (4), 669–673. doi:10.1021/np700745f
Yang, P., Qing, Z. X., Xiang, F., Mo, C. M., and Tang, Q. (2017). HPLC-Q-TOF/MS Method for Identification of Alkaloids in Zanthoxylum Nitidum and Zanthoxylum Echinocarpum. Chin. Tradit. Pat. Med. 39 (8), 1646–1650. doi:10.3969/j.issn.1001-1528.2017.08.022
Zar, J. H. (2005). Spearman Rank Correlation. Encyclopedia of biostatistics 7. doi:10.1002/0470011815.b2a15150
Zhang, C., Qian, D. D., Yu, T., Yang, H., Li, P., and Li, H. J. (2021). Multi-parametric Cellular Imaging Coupled with Multi-Component Quantitative Profiling for Screening of Hepatotoxic Equivalent Markers from Psoraleae Fructus. Phytomedicine 93, 153518. Available online 17 February 2021. doi:10.1016/j.phymed.2021.153518
Zhang, H., Zhang, D., Ray, K., and Zhu, M. (2009). Mass Defect Filter Technique and its Applications to Drug Metabolite Identification by High-Resolution Mass Spectrometry. J. Mass. Spectrom. 44 (7), 999–1016. doi:10.1002/jms.1610
Zhang, H., Zhu, M., Ray, K. L., Ma, L., and Zhang, D. (2008). Mass Defect Profiles of Biological Matrices and the General Applicability of Mass Defect Filtering for Metabolite Detection. Rapid Commun. Mass. Spectrom. 22 (13), 2082–2088. doi:10.1002/rcm.3585
Zhang, H. L., Gan, X. Q., Fan, Q. F., Yang, J. J., Zhang, P., Hu, H. B., et al. (2017). Chemical Constituents and Anti-inflammatory Activities of Maqian (Zanthoxylum Myriacanthum Var. Pubescens) Bark Extracts. Sci. Rep. 7, 45805. doi:10.1038/srep45805
Zhang, W., Huai, Y., Miao, Z., Qian, A., and Wang, Y. (2019). Systems Pharmacology for Investigation of the Mechanisms of Action of Traditional Chinese Medicine in Drug Discovery. Front. Pharmacol. 10, 743. doi:10.3389/fphar.2019.00743
Zhang, X. F., Chen, J., Yang, J. L., and Shi, Y. P. (2018). UPLC-MS/MS Analysis for Antioxidant Components of Lycii Fructus Based on Spectrum-Effect Relationship. Talanta 180, 389–395. doi:10.1016/j.talanta.2017.12.078
Zhang, Y., Luo, Z., Wang, D., He, F., and Li, D. (2014). Phytochemical Profiles and Antioxidant and Antimicrobial Activities of the Leaves of Zanthoxylum Bungeanum. ScientificWorldJournal 2014, 181072. doi:10.1155/2014/181072
Zhao, L. N., Guo, X. X., Liu, S., Feng, L., Bi, Q. R., Wang, Z., et al. (2018). (±)-Zanthonitidine A, a Pair of Enantiomeric Furoquinoline Alkaloids from Zanthoxylum Nitidum with Antibacterial Activity. Nat. Prod. Bioprospect 8 (5), 361–367. doi:10.1007/s13659-018-0169-7
Zhou, W., Shan, J., and Meng, M. (2018). A Two-step Ultra-high-performance Liquid Chromatography-Quadrupole/time of Flight Mass Spectrometry with Mass Defect Filtering Method for Rapid Identification of Analogues from Known Components of Different Chemical Structure Types in Fructus Gardeniae-Fructus Forsythiae Herb Pair Extract and in Rat’s Blood. J. Chromatogr. A. 1563, 99–123. doi:10.1016/j.chroma.2018.05.067
Keywords: Zanthoxylum nitidum (Roxb.) DC., spectrum–effect relationship, chemical profiling, anti-inflammation, antioxidant activity
Citation: Rao S-w, Duan Y-y, Pang H-q, Xu S-h, Hu S-q, Cheng K-g, Liang D and Shi W (2022) Spectrum-Effect Relationship Analysis of Bioactive Compounds in Zanthoxylum nitidum (Roxb.) DC. by Ultra-High Performance Liquid Chromatography Mass Spectrometry Coupled With Comprehensive Filtering Approaches. Front. Pharmacol. 13:794277. doi: 10.3389/fphar.2022.794277
Received: 13 October 2021; Accepted: 31 January 2022;
Published: 09 March 2022.
Edited by:
Abdul Rohman, Gadjah Mada University, IndonesiaReviewed by:
Ying-yuan Lu, Peking University, ChinaChang-hong Wang, Shanghai University of Traditional Chinese Medicine, China
Copyright © 2022 Rao, Duan, Pang, Xu, Hu, Cheng, Liang and Shi. This is an open-access article distributed under the terms of the Creative Commons Attribution License (CC BY). The use, distribution or reproduction in other forums is permitted, provided the original author(s) and the copyright owner(s) are credited and that the original publication in this journal is cited, in accordance with accepted academic practice. No use, distribution or reproduction is permitted which does not comply with these terms.
*Correspondence: Dong Liang, bGlhbmdkb25nZ3hudUAxNjMuY29t; Wei Shi, c3d2MjAxMkAxNjMuY29t