- 1Alliance Manchester Business School, The University of Manchester, Manchester, United Kingdom
- 2Warwick Medical School, Coventry, United Kingdom
- 3Warwick Business School, Coventry, United Kingdom
- 4School of Pharmacy, University of Auckland, Auckland, New Zealand
- 5Institute for Applied Health Research, University of Birmingham, Birmingham, United Kingdom
- 6UCL School of Pharmacy, University College London, London, United Kingdom
- 7Public Health England, London, United Kingdom
- 8London School of Economics and Political Science, Public Health England, London, United Kingdom
- 9Kantar Public, London, United Kingdom
Background: The benefits of medication optimization are largely uncontroversial but difficult to achieve. Behavior change interventions aiming to optimize prescriber medication-related decisions, which do not forbid any option and that do not significantly change financial incentives, offer a promising way forward. These interventions are often referred to as nudges.
Objective: The current systematic literature review characterizes published studies describing nudge interventions to optimize medication prescribing by the behavioral determinants they intend to influence and the techniques they apply.
Methods: Four databases were searched (MEDLINE, Embase, PsychINFO, and CINAHL) to identify studies with nudge-type interventions aiming to optimize prescribing decisions. To describe the behavioral determinants that interventionists aimed to influence, data were extracted according to the Theoretical Domains Framework (TDF). To describe intervention techniques applied, data were extracted according to the Behavior Change Techniques (BCT) Taxonomy version 1 and MINDSPACE. Next, the recommended TDF-BCT mappings were used to appraise whether each intervention applied a sufficient array of techniques to influence all identified behavioral determinants.
Results: The current review located 15 studies comprised of 20 interventions. Of the 20 interventions, 16 interventions (80%) were effective. The behavior change techniques most often applied involved prompts (n = 13). The MINDSPACE contextual influencer most often applied involved defaults (n = 10). According to the recommended TDF-BCT mappings, only two interventions applied a sufficient array of behavior change techniques to address the behavioral determinants the interventionists aimed to influence.
Conclusion: The fact that so many interventions successfully changed prescriber behavior encourages the development of future behavior change interventions to optimize prescribing without mandates or financial incentives. The current review encourages interventionists to understand the behavioral determinants they are trying to affect, before the selection and application of techniques to change prescribing behaviors.
Systematic Review Registration: [https://www.crd.york.ac.uk/prospero/], identifier [CRD42020168006].
1 Introduction
To prescribe medications optimally healthcare practitioners must make decisions about whether prescribing medication is appropriate, and, if so, how much, how often, and what type of medications they use (National Institute for He, 2015). Behavior change interventions can optimize these decisions in terms of patient safety and cost-effectiveness (Michie et al., 2011; Faria et al., 2014). Behavior change interventions that do not forbid any options and do not significantly change economic incentives may be referred to as nudges (Thaler and Sunstein, 2009; Vlaev et al., 2016). The current systematic review is the first to synthesize information about behavior change interventions designed to optimize prescribing decisions, without forbidding options or changing economic incentives, according to theoretically and empirically informed frameworks that can inform future interventions.
The benefits of medication optimization are largely uncontroversial but challenging to achieve (Faria et al., 2014). This difficulty arises, in part, due to the many stakeholders (e.g., patients and clinicians) and processes involved (e.g., prescribing, dispensing, administering, monitoring, and record-keeping involved). To simplify this picture, the current review focuses on practitioner prescribing decisions. Other reviews have focused on a more specific set of intervention modes (e.g., e-prescribing) (Nuckols et al., 2014; Williams et al., 2020) or types of patients (e.g., patients with dementia) (McGrattan et al., 2017; ShafieeHanjani et al., 2019). They do not focus on interventions aiming to change prescribing behavior without forbidding options or changing economic incentives. Nudge interventions are appealing in bringing about better practice; where nudge interventions are effective, they are more likely to be socially and professionally acceptable than mandates (Nagtegaal et al., 2019).
Of course, whether an intervention succeeds depends on more than whether prescribers find it acceptable. A successful intervention must address existing reasons (also called barriers and facilitators) for whether a desirable behavior occurs (Hardeman et al., 2005). The Behavior Change Wheel (Michie et al., 2011) provides an empirically and theoretically supported guide that helps interventionists to identify these reasons and then to select techniques best suited to overcome them. For example, a prescriber may not know what medications the current guidelines recommend (a barrier related to knowledge), which could be overcome by making concise guidelines more accessible (Allen and Harkins, 2005; Elwyn et al., 2016). Alternatively, e.g., a prescriber may not feel comfortable recommending a more optimal medication because their patients seem uncomfortable considering new options (a barrier related to social influences), which could be overcome by encouraging shared decision making (Stead et al., 2017).
As more than one reason may underlie suboptimal prescribing, many interventions are complex in the sense that they involve one or more techniques to overcome each identified reason (Craig et al., 2008; Michie et al., 2009). Addressing all the reasons for suboptimal prescribing simultaneously is important, as any unaddressed reason can prevent change. For example, prescribers likely need both sufficient knowledge regarding what medications to prescribe and sufficient social support to do so.
The current review has two objectives. The main objective is to describe the techniques applied in nudge-type behavior change interventions to optimize practitioners’ prescribing and whether those interventions were effective. The second objective is to describe whether the previous interventions applied a sufficient array of techniques to address all of their identified determinants (Elwyn et al., 2016).
2 Materials and Methods
The current review is reported according to the Preferred Reporting Items for Systematic Reviews and Meta-Analyses guidelines (Moher et al., 2009). The review was registered on the February 27, 2020 on the International Prospective Register of Systematic Reviews (ID: CRD42020168006).
2.1 Eligibility
The inclusion and exclusion criteria were defined according to the PICOS framework, see Supplementary Appendix 1. We included studies reporting on evaluated behavior change interventions to optimize medication prescribing for people with a legal ability to prescribe, e.g., clinicians. Additionally, studies needed to describe potential behavioral determinants of suboptimal prescribing that interventionists intended to influence. The interventions were restricted to those that did not forbid choices and did not significantly change economic incentives. Both randomized and non-randomized studies were included, so long as there was a comparison condition, e.g., non-randomised studies could include before/after comparisons. The search was restricted to studies published in peer-reviewed journals and written in the English language, as no translation services were available to the research team. The initial publication year was bound by each database’s restrictions.
2.2 Information Sources and Search Strategy
The information sources and search strategies were designed in collaboration with a university librarian to include all PICOS characteristics combined using Boolean variables. The final search was conducted by one author (SK) on the 5th of August 2019 over the following databases: MEDLINE (1946 to June 2019), Embase (1980 to June 2019), PsycINFO (1806 to June 2019), and CINAHL (1961 to June 2019). An example of the search conducted in Medline (Ovid) is provided in Supplementary Appendix 1. SK exported the retrieved study titles and abstracts into Endnote (version X9.2 for Windows & Mac, released June 11, 2019) and deleted duplicates.
2.3 Study Selection
Two reviewers (UT and SK) assessed the eligibility of articles. First, they independently screened the titles and abstracts according to the inclusion/exclusion criteria. Their initial agreement is described using a percentage (number of agreements divided by the total number of decisions). Disagreements were resolved via consensus-based discussions with IV acting as an arbitrator. The reasons for removing titles and abstracts were not recorded. The remaining full-texts were reviewed by UT, SK, IV, and KS. Final decisions were made via consensus-based discussions. The reasons for removing full-text articles were recorded.
2.4 Data Collection Process and Data Items
Three data extraction templates were designed to meet our objectives. The first was used to compile the information about the behavioral determinants each intervention aimed to influence from each study’s introduction. As recommended in the Behaviour Change Wheel’s manual, the possible determinants are described using the Theoretical Domains Framework (TDF) (Cane et al., 2012). The TDF is a cross-disciplinary synthesis of 112 unique constructs of behavior change into 14 theoretically informed domains, also called determinants, e.g., “knowledge” and “social Influences.” All 14 determinants are listed at the left of Figure 1.
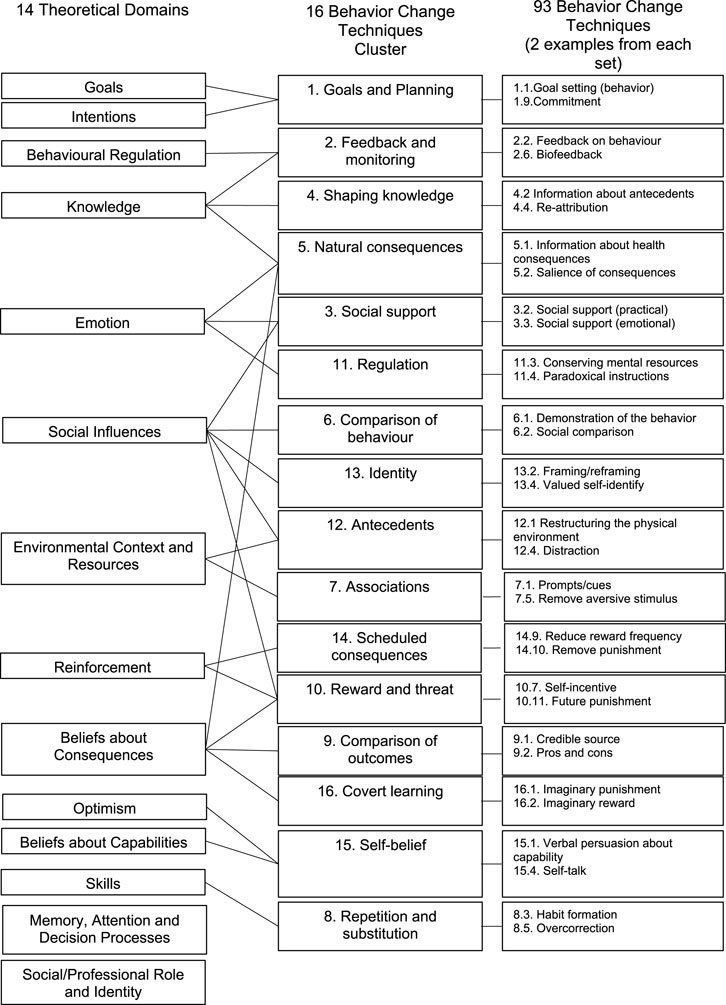
FIGURE 1. Recommended connections between the Behavior Change Techniques version 1, Technique Clusters, and Theoretical Domains Framework Domains.
The second data extraction template compiled information about the behavior change techniques applied using the Behavior Change Techniques (BCTs) Taxonomy version 1 (Michie et al., 2013). This taxonomy includes 93 empirically supported techniques that on their own have the potential to change behavior. To increase the usability of the BCTs Taxonomy, the 93 techniques have been hierarchically arranged into a more manageable set of 16 clusters (Cane et al., 2015). The techniques are typically referred to using the same verbiage, along with a number that identifies their cluster and number within that cluster. For example, the “2.3 self-monitoring of behavior” technique is the third technique listed in the second cluster (the Feedback and Monitoring cluster), and the “3.2 social support (practical)” technique is the second technique listed in the third cluster (the Social Support cluster). The 16 clusters and examples of techniques within them are provided in the middle and right of Figure 1.
The relationships between the theoretical domains and the techniques best suited to influence them are indicated in the connecting lines between the first and second columns in Figure 1. For instance, the “knowledge” determinant is linked to techniques in the Feedback and Monitoring cluster. A potential problem arises here, as no techniques are linked to the following two determinants: “memory, attention, and decision processes” and “social/professional role and identity.” Thus, there is a need for another framework to fill this gap.
To fill this gap, the third data extraction template complied information about concepts described in the behavioral economics literature. While these concepts are not explicitly called techniques, some can certainly be used to change behavior (Vlaev et al., 2016; Tagliabue et al., 2019). For instance, the MINDSPACE framework summarizes nine of the most robust and non-coercive influences on behavior (Dolan et al., 2010; Kahneman, 2011; Dolan et al., 2012). Each letter in MINDSPACE stands for a different contextual influencer including messenger, incentives, norms, defaults, salience, priming, affect, commitments, and ego, see Table 1. While some contextual influencers may already be included as BCTs in the taxonomy, others are not. For example, the commitment contextual influencer is likely captured by the “1.9 commitment” technique, but it is difficult to say which techniques capture defaults and priming. Coding the techniques and contextual influencers in this review allows us to describe those that have been applied in previous interventions, and to highlight those that are potentially underutilized and unevaluated.
In summary, there were 14 decisions made about the theoretical domains identified in each study (i.e., yes or no for each domain), 93 about the behavior change techniques applied in each intervention, and nine about the contextual influencers applied in each intervention. UT and KAS extracted these data independently. Their initial agreements are described using percentages (number of agreements divided by the total number of decisions). Disagreements were resolved via consensus-based discussions.
Data were also extracted to describe broader study characteristics (publication year, country, setting, study design, and study duration) and to describe whether each intervention optimized prescriber behavior. The effectiveness of the intervention was determined by each study’s authors in terms of their given comparison condition; for single-arm studies, the comparisons were between the pre-intervention measures and the post-intervention measures. The study designs were classified using the Scottish Intercollegiate Guidelines Network’s (SIGN’s) study design algorithm (Miller, 2002). The quality of evidence generated by each study was appraised using SIGN’s critical appraisal checklists and notes. A brief description of each intervention was compiled according to the Template for Intervention Description and Replication (TIDieR) checklist (Hoffmann et al., 2014).
2.5 Synthesis of Results
Narrative syntheses and tallies are used to summarize the behavioral determinants identified in each study, the techniques and contextual influencers applied in each intervention, and whether each intervention was effective. We also examined whether each intervention applied a sufficient array of recommended techniques to address its identified determinants. A meta-analysis was not conducted due to the heterogeneous nature of the study methods and outcomes.
3 Results
3.1 Study Selection
The study selection process is summarized in Figure 2. The initial search identified 1,596 articles with duplicates removed. Of the 1,596 articles, 1,555 were removed after reviewing the titles/abstracts. The initial agreement on the titles and abstracts was high (99%). A further 26 articles were removed after reviewing the full texts. A list of these articles and the reasons for their exclusions are provided in Supplementary Appendix 2. Ultimately, 15 articles that evaluated interventions to optimize prescribing were included (Bourdeaux et al., 2014; Harewood et al., 2011; Isenberg et al., 2018; Lemiengre et al., 2018; Mafi et al., 2018; Malhotra et al., 2016; Meeker et al., 2014; Musgrove et al., 2018; O'Connor et al., 2009; Patel et al., 2018; Patel et al., 2017; Presseau et al., 2018; Sacarny et al., 2018; Shakespeare et al., 2019; Yadav et al., 2019).
3.2 Study Characteristics
Study characteristics are described in Table 2. The studies were published between 2009 and 2018, inclusive. Most of them were conducted in North America (n = 10), followed by Europe (n = 4), and Australia (n = 1). The median duration of the studies was 12 months (range = 2–67). More studies were conducted in secondary care (n = 8) than in primary care (n = 5). While 11 studies evaluated a single intervention, four evaluated more, and the total number of evaluated interventions was 20. The interventions are described in greater depth later in the Section 3. Of the 20 interventions described, 16 (80%) were effective. Complete descriptions of each intervention according to the TIDieR checklist are provided in Supplementary Appendix 3.
3.3 Quality Assessment
Eight of the 15 studies used designs that could be evaluated with one of the SIGN’s checklists, see Table 2. Six of these studies were rated as generating high-quality evidence, one as acceptable, and one as low. The high-quality studies generally consisted of RCT methods carried out with adequate concealment. The low-quality study lacked reporting for many elements, e.g., for whether the only difference between groups is the treatment under investigation, and for the percentage of participants who dropped out before the study was completed. The remaining seven studies used an interrupted time-series design, for which SIGN does not make a checklist available and considers as entailing a higher risk of bias. The full quality assessments are provided in Supplementary Appendix 4.
3.4 Behavioral Determinants
Table 3 summarizes the data extracted to meet the review objectives. The behavioral determinants identified for each study are described in the second column. The reviewers’ initial agreements were high (84%). While all studies posited some reasons for suboptimal prescribing, none included detailed information about how they identified the determinants. Only one study, Presseau et al., included references to previous publications that did explain how the determinants of suboptimal prescribing were identified, specifically regarding prescribing medications to treat diabetes (Presseau et al., 2018).
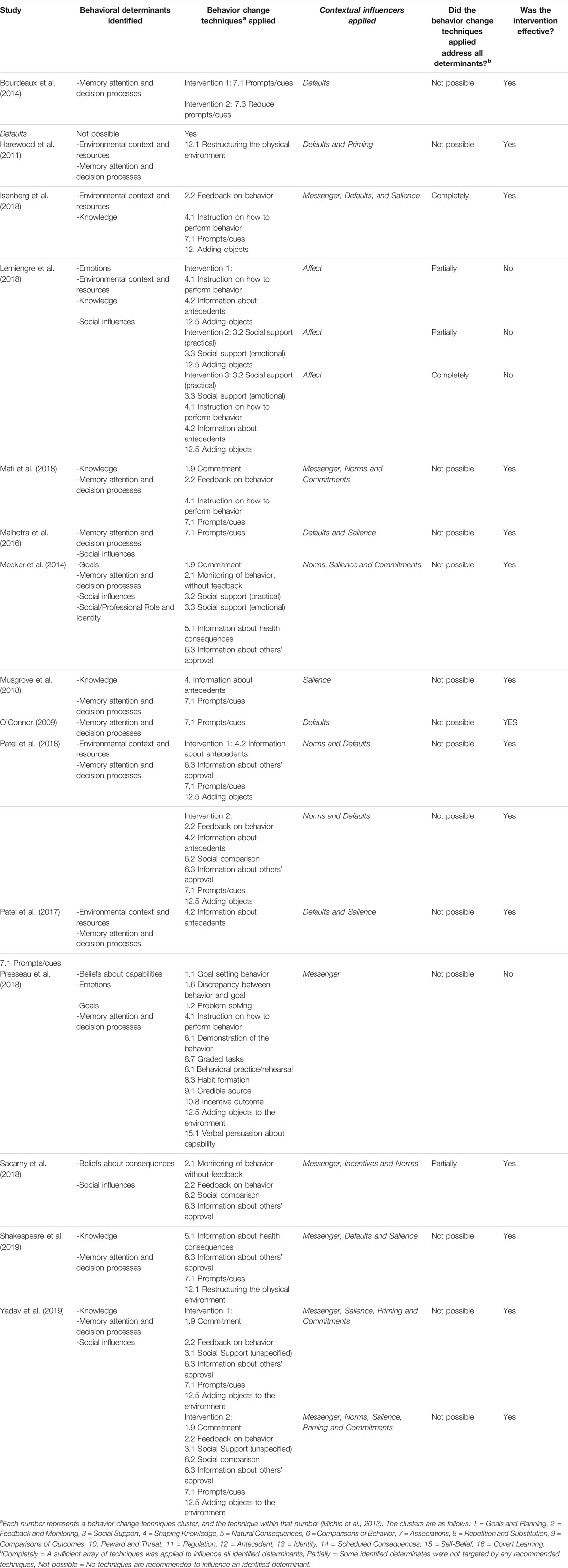
TABLE 3. Behavioral determinants identified in each study, along with the techniques recommended to influence them, the behavior change techniques/contextual influencers applied in each intervention, whether the techniques applied addressed all the determinants identified, and whether the interventions were effective.
In the 15 included studies, 9 of the 14 TDF determinants were identified. The median number of determinants identified was 2 (range 1–4). As multiple determinants were identified in most studies, we could anticipate that multiple techniques would be recommended to influence behavior effectively. The ‘memory attention and decision processes’ was identified as a determinant in 12 studies, and this poses a potential problem for interventionists because there are no recommended techniques to address this determinant. No studies identified the “behavioral regulation,” “intentions,” “optimism,” “reinforcement,” and “skills” determinants.
3.5 Behavior Change Techniques
The behavior change techniques applied in each of the 20 interventions along with their clusters are provided in the third column in Table 3. Reviewers’ initial agreements were high (96%). Twenty-six of the 93 techniques were applied, which sit in 12 of the 16 clusters. The median number of techniques applied was 4 (range 1–12). Techniques from the Associations cluster (n = 13; e.g., “7.1 prompts/cues”) and the Antecedent cluster (n = 11; e.g., “12.1 restructuring the physical environment” beyond adding prompts/cues), were applied most, followed closely by techniques from the Shaping Knowledge cluster (n = 9; e.g., “4.1 instruction on how to perform behavior”). No interventions applied techniques from the Covert Learning, Regulation, Identity, and Scheduled Consequences clusters.
3.6 MINDSPACE Contextual Influencers Applied
The MINDSPACE contextual influencers applied in each intervention are provided in the fourth column of Table 3. Reviewers’ initial agreements were high (80%). The median number of contextual influencers applied was 2 (range 1–5). Of the nine contextual influencers, eight were applied across the interventions. The most often applied contextual influencer was defaults (n = 10). No interventions applied the ego influencer.
The defaults influencer was often applied by altering the organization’s prescribing software (n = 7). For example, Patel et al. (Patel et al., 2017) inserted default reminders to vaccinate all incoming patients against influenza, and Malhotra et al. (Malhotra et al., 2016) altered the search function to return generic medication options when brand name medications were entered.
The commitments influencer was often applied by asking prescribers to sign a document in studies to optimize antibiotic prescribing (n = 4). For example, in Meeker et al.’s (Meeker et al., 2014) intervention clinicians signed a personal poster-sized commitment letter featuring their photographs and signatures along with a stated commitment to avoiding inappropriate antibiotic prescribing. These personal commitments were displayed in the practitioners’ examination rooms for 12 weeks. In Mafi et al.’s (Mafi et al., 2018) and Yadav et al.’s (Yadav et al., 2019) interventions (both of which aimed to optimize antibiotic prescribing), the similar commitment documents were displayed in more public areas, such as waiting rooms. In Yadav’s intervention, some practitioners also wore badges signaling their commitment to better antibiotic stewardship.
The norms influencer was often applied by sending prescribers letters with peer-comparison information (n = 3). Patel et al.’s (Patel et al., 2018) and Yadav et al.’s (Yadav et al., 2019) intervention letters compared practitioners’ medication use to the 90th percentile performers for statins and antibiotics, respectively, and Sacarney et al.’s (Sacarny et al., 2018) intervention letters compared practitioner medication use to the 75th percentile performers for quetiapine.
Did the interventions apply a sufficient array of recommended techniques, and were they effective?
The fifth column of Table 3 describes whether the behavior change techniques applied in each intervention addressed all its study’s identified determinants. The last column describes whether each intervention was effective; 16 of the 20 interventions (80%) were effective.
Only two of the interventions applied a sufficient array of recommended techniques to address all identified determinants, and only one of these interventions was effective. Regarding the effective intervention, Isenberg et al. (Isenberg et al., 2018) noted that emergency department practitioners may not be aware of new guidelines published around pain management for mechanically ventilated patients and that workflow inadequacies may be preventing optimal analgesic and benzodiazepine use. To build practitioners’ knowledge, they provided feedback on their current prescribing and instructions about how to follow the new guidelines. To address workflow inadequacies, they started to stock analgesics in the emergency department and removed benzodiazepines from an existing order set.
Regarding the non-effective intervention, Lemiengre et al. (Lemiengre et al., 2018) identified “environmental contexts and resources,” “knowledge,” “emotions,” and “social influences” as determinants of pediatric practitioners’ inappropriate antibiotic use. They then used a factorial design to test the effectiveness of two intervention components, in isolation and combination, against a control condition where neither intervention component was applied. The first intervention simply provided practitioners with quick finger-prick diagnostic tests to mitigate uncertainty about the diagnosis. The second intervention provided practitioners with a supportive set of questions they could ask parents and a safety net brochure they could give parents to cope with parental pressure. The third intervention applied both intervention components to address all identified determinants but was not effective.
Three of the interventions applied techniques that partially addressed their identified determinants. Two were not effective and one was effective. The two that were not effective were Lemiengre et al.’s (Lemiengre et al., 2018) single component interventions described above. The other intervention was Sacarny et al. (Sacarny et al., 2018), for which they identified “social influences” and “beliefs about consequences” as behavioral determinants of practitioners’ suboptimal quetiapine fumarate use. Their intervention involved sending practitioners letters that compared their prescribing to their peers’ prescribing (a social influence), but they did not include additional information to emphasize the natural consequences of poor prescribing practices.
The remaining 15 interventions could not address all their identified determinants, because there are no recommended techniques to address the “memory, attention, and decision processes” or “social/professional role and identity” determinants. Yet, these interventions were still effective. In these interventions, the contextual influencers may have bolstered the effectiveness of the intervention. Of these 15 interventions, the most frequently applied contextual influencers were the defaults influencer (n = 9), followed by the salience influencer (n = 7), norms and messenger (n = 5), commitments (both ns = 4), and priming (n = 3). Note that as each intervention could apply more than one contextual influencer, these numbers do not sum to 15. While space does not permit an extensive review of all the 15 interventions here (see Supplementary Appendix 3 for additional information), two of the effective interventions that creatively altered the existing choice environment are described below.
Harewood et al.’s (Harewood et al., 2011) study identified “environmental resources and contexts” and “memory, attention, and decision processes” as behavioral determinants of suboptimal midazolam use for endoscopic sedation. Their intervention involved pre-filling syringes with different amounts of midazolam. This intervention could be described as simply applying the “restructuring the physical environment” technique that is well suited to overcome the “environmental resources and context” determinant. However, we posit that the defaults and priming influencers may have bolstered the effectiveness of this intervention, as prescribers’ medication use trended towards the pre-filled amounts labeled on the syringe in a manner anticipated by the literature on default and anchoring effects (Tversky and Kahneman, 1974; Johnson and Goldstein, 2003).
As another example (Musgrove et al., 2018), identified “knowledge” and “memory attention and decision processes” as behavioral determinants of suboptimal antibiotic use for hospital in-patients. Before their intervention when there was no indication of a bacterial infection, the results of the diagnostic tests given to clinicians stated that the sample contained “commensal respiratory flora.” Their intervention modified the language in this report to emphasize that there was “commensal respiratory flora only: No S. aureus/MRSA or P. aeruginosa.” This intervention could be described as using a technique to address only the “knowledge” determinant. However, as clinicians likely already had sufficient knowledge to understand the initial reporting language, enhancing the salience of the “no” bacteria information more likely contributed to this intervention’s beneficial effect.
4 Discussion
The current systematic review included 15 studies describing interventions that aim to optimize prescribing decisions without forbidding options or changing economic incentives. Of the 20 interventions described in these 15 studies, 16 (80%) were effective. Regarding the review’s first objective, to describe the behavior change techniques and contextual influencers applied, 26 behavior change techniques and 8 contextual influencers were applied. Regarding the second objective, to describe whether the previous interventions applied a sufficient array of recommended techniques, only two did. As so few interventions applied recommended techniques, there is insufficient information to conclude whether interventions that apply recommended techniques are more effective than those that do not.
The current findings echo other reviews’ findings that behavioral interventions can optimize prescribing. For example, a systematic review of 31 randomized controlled trials finds that medication reviews can reduce the number of medication-related problems patients experience, and the number of medications ultimately prescribed (Huiskes et al., 2017). Beyond improving patients’ wellbeing, Hasan et al.’s economic analysis notes that medication reviews can also result in significant cost savings to support the financial stability of healthcare organizations (Hasan et al., 2017). Another systematic review finds that computerized clinical decision support systems improved care performance in 37 of the 59 studies examined (Hemens et al., 2011). However, the positive effects of computerized clinical decision support systems interventions on patient outcomes are less reliable, as only 6 of the 29 trials that assessed patient outcomes in their review found improvements.
To the authors’ knowledge, this is the first study to use TDF, BCTs Taxonomy version 1, and MINDSPACE framework at once to characterize behavioral change interventions. Coding the interventions according to the TDF, BCTs Taxonomy, and MINDSPACE framework allows the current review to comment not only on those intervention techniques attempted, but to highlight some techniques that have not yet been evaluated. This is of course not to say that unevaluated options will work but to emphasize that absence of evidence does not entail it is not effective (Altman and Bland, 1995). For example, several studies identified “social influences” as a behavioral determinant of suboptimal prescribing. The “social influences” determinant can be influenced by techniques in the Identity cluster, but none of these studies applied techniques from the Identity cluster (e.g., “13.1 identification of self as a role model”). In addition, several studies identified “emotions” as a behavioral determinant. The “emotions” determinant can be influenced by techniques in the Regulation cluster, but none of these studies applied techniques from the Regulation cluster (e.g., “11.2 reduce negative emotions”). Regarding the MINDSPACE contextual influencers, the ego influencer was never applied. For ego, interventions that emphasize the reputational benefits favoring certain prescribing decisions might prove effective where “social/professional role and identity” is identified as a determinant of suboptimal prescribing, e.g., around publicized low-value services (Bishop et al., 2017).
The recommended linkages provided by Cane et al. (Cane et al., 2015) were used in the current review because these linkages ensure that all 93 behavior change techniques are linked to at least one TDF domain. Without using the clusters, some behavior change techniques are missing links to the TDF domains. The Behavior Change Techniques Taxonomy version 1 is the most exhaustive list of behavioral change techniques, but the Theoretical Domains Framework is not the most exhaustive list of potential behavioral determinants. Recently the 14 domains have been joined up with 12 of the most frequently cited mechanisms of action, e.g., “self-image” and “values” to yield a more extensive list of 26 determinants. These 26 determinants have been linked to only 70 behavior change techniques via a literature review (Carey et al., 2019) and 61 techniques have been linked via an expert consensus study (Connell et al., 2019). An interactive tool, called the Theory and Techniques Tool, has been created to bring these more recent findings together. As of May 2021, this tool provides researchers with links between 74 behavior change techniques and the 26 determinants (https://theoryandtechniquetool.humanbehaviorchange.org/).
Within the Theory and Techniques Tool, the “memory attention and decisions processes” is linked with high certainty to the “7.1 prompts/cues” technique. In the current review, 12 studies identified ‘memory attention and decision processes’ as a determinant, and 9 applied the “7.1 prompts/cues technique.” Therefore, applying these new connections reveals a great deal more studies where the techniques aligned with the determinants identified. However, the “social/professional role and identity” determinant is still not conclusively linked to any of these 74 behavior change techniques. Additionally, those 19 techniques missed off the original lists (93 − 74 = 19) will not be linked up to any of the determinants in the expanded list.
Some limitations of the current review are now noted. First, the current review includes articles published before July 2019, which will be beyond the recommended 2 years when this article is published. As research around nudge interventions has likely expanded since Thaler and Sunstein published their book “Nudge,” and since Thaler’s won the Nobel prize in economics in 2017 (Earl, 2018), new articles may have been missed from this review. While the present synthesis of previous studies can inform future research and enhance methods of future literature reviews, researchers and policymakers could seek more recent evidence to inform their studies and changes in practice. Second, the search only includes articles that have been published in peer-reviewed journals in the English language, and this may exacerbate the negative effects of publication biases. A future review may increase the scope of the current findings by including articles published in non-English languages, and in the grey literature (e.g., policy documents or internal organizational reports). Third, nearly half of the studies in our review made use of non-randomized methods, which have a greater likelihood for bias than randomized methods.
A fourth limitation is that behavioral determinants are often vaguely reported in published intervention studies and the behavior change techniques are not easy to code some may have been excluded (Abraham et al., 2015). The high percentage agreements in the intervention coding are in part a function of the large number of determinants, techniques, and contextual influencers that the reviewers could easily say were not being used. To improve the reporting of interventions, we urge researchers to describe their interventions using the TIDieR checklist (Hoffmann et al., 2014). We also urge researchers to identify the techniques they apply using the BCT Taxonomy (Michie et al., 2013) and to consider what other influences are at play, particularly those developed in behavioral economics.
In conclusion, the current review included 15 studies with 20 interventions of which 16 were effective. This encouraging finding supports the development of future behavior change interventions to optimize prescribing, which do not include mandates or significantly alter financial incentives. The present review encourages interventionists to understand the behavioral determinants they are trying to affect, before selecting techniques/contextual influencers to apply. The TDF, BCTs Taxonomy version 1, and MINDSPACE framework are three theoretically informed tools that interventionists can consider when developing complex interventions to optimize prescribing and ultimately improve patient wellbeing.
Data Availability Statement
The raw data supporting the conclusions of this article will be made available by the authors, without undue reservation.
Author Contributions
All co-authors approved the study protocol. UT and SK registered the literature and conducted the initial search for articles. KS built the data extraction templates. UT and KS drafted the initial manuscript. IV provided conceptual oversight and supervision. In addition to these four authors, the following collaborating authors contributed to the enhanced the quality of the final draft: AC, AT, RH, TC, and NG. All authors have read and approved the manuscript.
Funding
This work was supported by the Health Foundation’s Behavioral Insights Research program (Award 807263) and the National Institute for Health Research (NIHR) Applied Research Center (ARC) West Midlands (NIHR200165). The views expressed are those of the author(s) and not necessarily the funders. The funders had no role in the design of the study and collection, analysis, and interpretation of data and no role in writing the manuscript.
Conflict of Interest
Author NG was employed by Kantar Public.
The remaining authors declare that the research was conducted in the absence of any commercial or financial relationships that could be construed as a potential conflict of interest.
Publisher’s Note
All claims expressed in this article are solely those of the authors and do not necessarily represent those of their affiliated organizations, or those of the publisher, the editors and the reviewers. Any product that may be evaluated in this article, or claim that may be made by its manufacturer, is not guaranteed or endorsed by the publisher.
Supplementary Material
The Supplementary Material for this article can be found online at: https://www.frontiersin.org/articles/10.3389/fphar.2022.798916/full#supplementary-material
References
Abraham, C., Wood, C. E., Johnston, M., Francis, J., Hardeman, W., Richardson, M., et al. (2015). Reliability of Identification of Behavior Change Techniques in Intervention Descriptions. Ann. Behav. Med. 49, 885–900. doi:10.1007/s12160-015-9727-y
Allen, D., and Harkins, K. J. (2005). Too Much Guidance? Lancet 365 (9473), 1768. doi:10.1016/S0140-6736(05)66578-6
Altman, D. G., and Bland, J. M. (1995). Absence of Evidence Is Not Evidence of Absence. BMJ 311, 485. doi:10.1136/bmj.311.7003.485
Bishop, T. F., Cea, M., Miranda, Y., Kim, R., Lash-Dardia, M., Lee, J. I., et al. (2017). Academic Physicians' Views on Low-Value Services and the Choosing Wisely Campaign: a Qualitative Study. Healthc (Amst) 5, 17–22. doi:10.1016/j.hjdsi.2016.04.001
Bourdeaux, C. P., Davies, K. J., Thomas, M. J., Bewley, J. S., and Gould, T. H. (2014). Using 'nudge' Principles for Order Set Design: a before and after Evaluation of an Electronic Prescribing Template in Critical Care. BMJ Qual. Saf. 23, 382–388. doi:10.1136/bmjqs-2013-002395
Cane, J., O'Connor, D., and Michie, S. (2012). Validation of the Theoretical Domains Framework for Use in Behaviour Change and Implementation Research. Implement Sci. 7, 37. doi:10.1186/1748-5908-7-37
Cane, J., Richardson, M., Johnston, M., Ladha, R., and Michie, S. (2015). From Lists of Behaviour Change Techniques (BCTs) to Structured Hierarchies: Comparison of Two Methods of Developing a Hierarchy of BCTs. Br. J. Health Psychol. 20, 130–150. doi:10.1111/bjhp.12102
Carey, R. N., Connell, L. E., Johnston, M., Rothman, A. J., de Bruin, M., Kelly, M. P., et al. (2019). Behavior Change Techniques and Their Mechanisms of Action: a Synthesis of Links Described in Published Intervention Literature. Ann. Behav. Med. 53, 693–707. doi:10.1093/abm/kay078
Connell, L. E., Carey, R. N., de Bruin, M., Rothman, A. J., Johnston, M., Kelly, M. P., et al. (2019). Links between Behavior Change Techniques and Mechanisms of Action: an Expert Consensus Study. Ann. Behav. Med. 53, 708–720. doi:10.1093/abm/kay082
Craig, P., Dieppe, P., Macintyre, S., Michie, S., Nazareth, I., and Petticrew, M. (2008). Developing and Evaluating Complex Interventions: the New Medical Research Council Guidance. BMJ 337, a1655. doi:10.1136/bmj.a1655
Dolan, P., Hallsworth, M., Halpern, D., King, D., Metcalfe, R., and Vlaev, I. (2012). Influencing Behaviour: The Mindspace Way. J. Econ. Psychol. 33, 264–277. doi:10.1016/j.joep.2011.10.009
Dolan, P., Hallsworth, M., Halpern, D., King, D., and Vlaev, I. (2010). MINDSPACE: Influencing Behavior for Public Policy. London, UK: Institute of Government.
Earl, P. E. (2018). Richard H. Thaler: A Nobel Prize for Behavioural Economics. Rev. Polit. Economy 30, 107–125. doi:10.1080/09538259.2018.1513236
Elwyn, G., Wieringa, S., and Greenhalgh, T. (2016). Clinical Encounters in the post-guidelines Era. BMJ 353, i3200. doi:10.1136/bmj.i3200
Faria, R., Barbieri, M., Light, K., Elliott, R. A., and Sculpher, M. (2014). The Economics of Medicines Optimization: Policy Developments, Remaining Challenges and Research Priorities. Br. Med. Bull. 111, 45–61. doi:10.1093/bmb/ldu021
Hardeman, W., Sutton, S., Griffin, S., Johnston, M., White, A., Wareham, N. J., et al. (2005). A Causal Modelling Approach to the Development of Theory-Based Behaviour Change Programmes for Trial Evaluation. Health Educ. Res. 20, 676–687. doi:10.1093/her/cyh022
Harewood, G. C., Clancy, K., Engela, J., Abdulrahim, M., Lohan, K., and O'Reilly, C. (2011). Randomised Clinical Trial: a 'nudge' Strategy to Modify Endoscopic Sedation Practice. Aliment. Pharmacol. Ther. 34, 229–234. doi:10.1111/j.1365-2036.2011.04703.x
Hasan, S. S., Thiruchelvam, K., Kow, C. S., Ghori, M. U., and Babar, Z. U. (2017). Economic Evaluation of Pharmacist-Led Medication Reviews in Residential Aged Care Facilities. Expert Rev. Pharmacoecon Outcomes Res. 17, 431–439. doi:10.1080/14737167.2017.1370376
Hemens, B. J., Holbrook, A., Tonkin, M., Mackay, J. A., Weise-Kelly, L., Navarro, T., et al. (2011). Computerized Clinical Decision Support Systems for Drug Prescribing and Management: a Decision-Maker-Researcher Partnership Systematic Review. Implement Sci. 6, 89. doi:10.1186/1748-5908-6-8949
Hoffmann, T. C., Glasziou, P. P., Boutron, I., Milne, R., Perera, R., Moher, D., et al. (2014). Better Reporting of Interventions: Template for Intervention Description and Replication (TIDieR) Checklist and Guide. BMJ 348, g1687–60. doi:10.1136/bmj.g1687
Huiskes, V. J., Burger, D. M., van den Ende, C. H., and van den Bemt, B. J. (2017). Effectiveness of Medication Review: a Systematic Review and Meta-Analysis of Randomized Controlled Trials. BMC Fam. Pract. 18, 5. doi:10.1186/s12875-016-0577-x
Isenberg, D. L., Kissman, K. M., Salinski, E. P., Saks, M. A., and Evans, L. B. (2018). Simple Changes to Emergency Department Workflow Improve Analgesia in Mechanically Ventilated Patients. West. J. Emerg. Med. 19, 668–674. doi:10.5811/westjem.2018.4.36879
Johnson, E. J., and Goldstein, D. (2003). Medicine. Do Defaults Save Lives? Science 302, 1338–1339. doi:10.1126/science.1091721
Lemiengre, M. B., Verbakel, J. Y., Colman, R., De Burghgraeve, T., Buntinx, F., Aertgeerts, B., et al. (2018). Reducing Inappropriate Antibiotic Prescribing for Children in Primary Care: a Cluster Randomised Controlled Trial of Two Interventions. Br. J. Gen. Pract. 68, e204–10. doi:10.3399/bjgp18X695033
Mafi, J. N., Trotzky, R., Wei, E., Carillo, C. A., Vangala, S., and Coffey, C. E. (2018). Evaluation of a Choosing wiselyTM Intervention to Reduce Low-Value Antibiotic Prescribing at a Large Safety Net Medical center. J. Gen. Intern. Med. 33, 185–186. Available at: https://link.springer.com/content/pdf/10.1007%2Fs11606-018-4413-y.pdf.
Malhotra, S., Cheriff, A. D., Gossey, J. T., Cole, C. L., Kaushal, R., and Ancker, J. S. (2016). Effects of an E-Prescribing Interface Redesign on Rates of Generic Drug Prescribing: Exploiting Default Options. J. Am. Med. Inform. Assoc. 23, 891–898. doi:10.1093/jamia/ocv192
McGrattan, M., Ryan, C., Barry, H. E., and Hughes, C. M. (2017). Interventions to Improve Medicines Management for People with Dementia: a Systematic Review. Drugs Aging 34, 907–916. doi:10.1007/s40266-017-0505-3
Meeker, D., Knight, T. K., Friedberg, M. W., Linder, J. A., Goldstein, N. J., Fox, C. R., et al. (2014). Nudging Guideline-Concordant Antibiotic Prescribing: a Randomized Clinical Trial. JAMA Intern. Med. 174, 425–431. doi:10.1001/jamainternmed.2013.14191
Michie, S., Fixsen, D., Grimshaw, J. M., and Eccles, M. P. (2009). Specifying and Reporting Complex Behaviour Change Interventions: the Need for a Scientific Method. Implement Sci. 4, 40. doi:10.1186/1748-5908-4-40
Michie, S., Richardson, M., Johnston, M., Abraham, C., Francis, J., Hardeman, W., et al. (2013). The Behavior Change Technique Taxonomy (V1) of 93 Hierarchically Clustered Techniques: Building an International Consensus for the Reporting of Behavior Change Interventions. Ann. Behav. Med. 46, 81–95. doi:10.1007/s12160-013-9486-6
Michie, S., van Stralen, M. M., and West, R. (2011). The Behaviour Change Wheel: a New Method for Characterising and Designing Behaviour Change Interventions. Implement Sci. 6, 42. doi:10.1186/1748-5908-6-42
Miller, J. (2002). The Scottish Intercollegiate Guidelines Network (SIGN). Diabetes Vasc. Dis. 2, 47–49. doi:10.1177/14746514020020010401
Moher, D., Liberati, A., Tetzlaff, J., and Altman, D. G.The PRISMA Group (2009). Preferred Reporting Items for Systematic Reviews and Meta-Analyses: The PRISMA Statement. Plos Med. 6 (7), e1000097. doi:10.1371/journal.pmed.1000097
Musgrove, M. A., Kenney, R. M., Kendall, R. E., Peters, M., Tibbetts, R., Samuel, L., et al. (2018). Microbiology Comment Nudge Improves Pneumonia Prescribing. Open Forum Infect. Dis. 5, ofy162. doi:10.1093/ofid/ofy16233
Nagtegaal, R., Tummers, L., Noordegraaf, M., and Bekkers, V. (2019). Nudging Healthcare Professionals towards Evidence-Based Medicine: a Systematic Scoping Review. Jbpa 2 (2), 1–20. doi:10.30636/jbpa.22.71
National Institute for Health and Care Excellence (2015). Medicines Optimization: The Safe and Effective Use of Medicines to Enable the Best Possible Outcomes. Rev ed. Available at: https://www.nice.org.uk/guidance/ng5/resources/medicines-optimization-the-safe-and-effective-use-of-medicines-to-enable-the-best-possible-outcomes-pdf-51041805253 (Accessed April 16, 2021).
Nuckols, T. K., Smith-Spangler, C., Morton, S. C., Asch, S. M., Patel, V. M., Anderson, L. J., et al. (2014). The Effectiveness of Computerized Order Entry at Reducing Preventable Adverse Drug Events and Medication Errors in Hospital Settings: a Systematic Review and Meta-Analysis. Syst. Rev. 3, 56. doi:10.1186/2046-4053-3-56
O'Connor, C., Adhikari, N. K., DeCaire, K., and Friedrich, J. O. (2009). Medical Admission Order Sets to Improve Deep Vein Thrombosis Prophylaxis Rates and Other Outcomes. J. Hosp. Med. 4, 81–89. doi:10.1002/jhm.399
Patel, M. S., Kurtzman, G. W., Kannan, S., Small, D. S., Morris, A., Honeywell, S., et al. (2018). Effect of an Automated Patient Dashboard Using Active Choice and Peer Comparison Performance Feedback to Physicians on Statin Prescribing: The PRESCRIBE Cluster Randomized Clinical Trial. JAMA Netw. Open 1 (1), e180818–85. doi:10.1001/jamanetworkopen.2018.0818
Patel, M. S., Volpp, K. G., Small, D. S., Wynne, C., Zhu, J., Yang, L., et al. (2017). Using Active Choice within the Electronic Health Record to Increase Influenza Vaccination Rates. J. Gen. Intern. Med. 32, 790–795. doi:10.1007/s11606-017-4046-635
Presseau, J., Mackintosh, J., Hawthorne, G., Francis, J. J., Johnston, M., Grimshaw, J. M., et al. (2018). Cluster Randomised Controlled Trial of a Theory-Based Multiple Behaviour Change Intervention Aimed at Healthcare Professionals to Improve Their Management of Type 2 Diabetes in Primary Care. Implement Sci. 13, 65. doi:10.1186/s13012-018-0754-536
Sacarny, A., Barnett, M. L., Le, J., Tetkoski, F., Yokum, D., and Agrawal, S. (2018). Effect of Peer Comparison Letters for High-Volume Primary Care Prescribers of Quetiapine in Older and Disabled Adults: a Randomized Clinical Trial. JAMA Psychiatry 75, 1003–1011. doi:10.1001/jamapsychiatry.2018.1867
Shafiee Hanjani, L., Long, D., Peel, N. M., Peeters, G., Freeman, C. R., and Hubbard, R. E. (2019). Interventions to Optimise Prescribing in Older People with Dementia: A Systematic Review. Drugs Aging 36, 247–267. doi:10.1007/s40266-018-0620-9
Shakespeare, T., Fehlberg, M., Slejko, T., Taylor, J., Srbinovska, I., and Bolsin, S. (2019). Successful Use of "Choice Architecture" and "Nudge Theory" in a Quality Improvement Initiative of Analgesia Administration after Caesarean Section. J. Eval. Clin. Pract. 25 (1), 125–129. doi:10.1111/jep.13037
Stead, U., Morant, N., and Ramon, S. (2017). Shared Decision-Making in Medication Management: Development of a Training Intervention. Bjpsych Bull. 41 (4), 221–227. doi:10.1192/pb.bp.116.053819
Tagliabue, M., Squatrito, V., and Presti, G. (2019). Models of Cognition and Their Applications in Behavioral Economics: a Conceptual Framework for Nudging Derived from Behavior Analysis and Relational Frame Theory. Front. Psychol. 10, 2418. doi:10.3389/fpsyg.2019.02418
Thaler, R. H., and Sunstein, C. R. (2009). Nudge: Improving Decisions about Health, Wealth, and Happiness. USA: Penguin.
Tversky, A., and Kahneman, D. (1974). Judgment under Uncertainty: Heuristics and Biases. Science 185 (4157), 1124–1131. doi:10.1126/science.185.4157.1124
Vlaev, I., King, D., Dolan, P., and Darzi, A. (2016). The Theory and Practice of “Nudging”: Changing Health Behaviors. Public Admin Rev. 76, 550–561. doi:10.1111/puar.12564
Williams, J., Bates, D. W., and Sheikh, A. (2020). Optimising Electronic Prescribing in Hospitals: a Scoping Review Protocol. BMJ Health Care Inform. 27, e100117. doi:10.1136/bmjhci-2019-100117
Yadav, K., Meeker, D., Mistry, R. D., Doctor, J. N., Fleming-Dutra, K. E., Fleischman, R. J., et al. (2019). A Multifaceted Intervention Improves Prescribing for Acute Respiratory Infection for Adults and Children in Emergency Department and Urgent Care Settings. Acad. Emerg. Med. 26, 719–731. doi:10.1111/acem.13690
Keywords: medical decision-making, systematic reviews, prescribing/use/costs, behavioural science, nudge
Citation: Talat U, Schmidtke KA, Khanal S, Chan A, Turner A, Horne R, Chadborn T, Gold N, Sallis A and Vlaev I (2022) A Systematic Review of Nudge Interventions to Optimize Medication Prescribing . Front. Pharmacol. 13:798916. doi: 10.3389/fphar.2022.798916
Received: 20 October 2021; Accepted: 04 January 2022;
Published: 25 January 2022.
Edited by:
Irene Lenoir-Wijnkoop, Utrecht University, NetherlandsReviewed by:
Michal Stanak, Austrian Institute for Health Technology Assessment GmbH, AustriaLuis Laranjeira, Eli Lilly, Portugal
Jaheeda Gangannagaripalli, University of Strathclyde, United Kingdom
Copyright © 2022 Talat, Schmidtke, Khanal, Chan, Turner, Horne, Chadborn, Gold, Sallis and Vlaev. This is an open-access article distributed under the terms of the Creative Commons Attribution License (CC BY). The use, distribution or reproduction in other forums is permitted, provided the original author(s) and the copyright owner(s) are credited and that the original publication in this journal is cited, in accordance with accepted academic practice. No use, distribution or reproduction is permitted which does not comply with these terms.
*Correspondence: Kelly Ann Schmidtke, S2VsbHkuQS5TY2htaWR0a2VAd2Fyd2ljay5hYy51aw==; Ivo Vlaev, SXZvLlZsYWV2QHdicy5hYy51aw==
†These authors have contributed equally to this work