- 1Department of Nephrology, The Third Affiliated Hospital of Chongqing Medical University, Chongqing, China
- 2Core Research Laboratory, The Second Affiliated Hospital, Xi’an Jiaotong University, Xi’an, China
- 3Beijing Key Laboratory of Bioprocess, College of Life Science and Technology, Beijing University of Chemical Technology, Beijing, China
COVID-19-associated acute kidney injury (COVID-19 AKI) is an independent risk factor for in-hospital mortality and has the potential to progress to chronic kidney disease. Prunella vulgaris L., a traditional Chinese herb that has been used for the treatment of a variety of kidney diseases for centuries, could have the potential to treat this complication. In this study, we studied the potential protective role of Prunella vulgaris in COVID-19 AKI and explored its specific mechanisms applied by network pharmacology and bioinformatics methods. The combination of the protein-protein interaction network and Gene Ontology and Kyoto Encyclopedia of Genes and Genomes enrichment -target gene network revealed eight key target genes (VEGFA, ICAM1, IL6, CXCL8, IL1B, CCL2, IL10 and RELA). Molecular docking showed that all these eight gene-encoded proteins could be effectively bound to three major active compounds (quercetin, luteolin and kaempferol), thus becoming potential therapeutic targets. Molecular dynamics simulation also supports the binding stability of RELA-encoded protein with quercetin and luteolin. Together, our data suggest that IL6, VEGFA, and RELA could be the potential drug targets by inhibiting the NF-κB signaling pathway. Our in silico studies shed new insights into P. vulgaris and its ingredients, e.g., quercetin, as potential botanical drugs against COVID-19 AKI, and warrant further studies on efficacy and mechanisms.
1 Introduction
Coronavirus disease 2019 (COVID-19) is an extremely contagious disease caused by the severe acute respiratory syndrome coronavirus 2 (SARS-CoV-2) that emerged suddenly in 2019 (Hu et al., 2021a). The World Health Organization (WHO) has reported 632 million confirmed cases and 6.5 million deaths as of 13 November 2022 (Dong et al., 2020). The continuous outbreak of COVID-19 poses a great threat not only to human health but also to the global economy, society and human life (Daniels and Morton, 2023). Although a large number of studies have been conducted focusing on the prevention and treatment of the disease, the mechanism behind treatment efficacy is not fully understood.
The main symptoms of COVID-19 are fever, cough and dyspnoea, and some patients in the acute phase may experience acute respiratory distress syndrome, sepsis, acute cardiac injury and secondary infection. Moreover, patients may develop multiple organ failure and shock in severe cases (Huang et al., 2020a). For instance, studies have reported a relatively high incidence rate of acute kidney injury (AKI, up to 77%) in patients with COVID-19, especially among those in the ICU (Huang et al., 2020b; Chen et al., 2020; Cheng et al., 2020; Guan et al., 2020; Yang et al., 2020; Matsumoto and Prowle, 2022). The chief pathologic change of AKI is renal tubular damage, with major physical signs of hematuria and proteinuria (Kudose et al., 2020; Han and Ye, 2021; Lin et al., 2022). Studies have reported that COVID-19-associated AKI (COVID-19 AKI) (Nadim et al., 2020) is not only linked to high in-hospital mortality rates but also accompanied by long-term complications, such as persistent AKI, end-stage renal disease and chronic renal insufficiency (Lin et al., 2020; Matsumoto and Prowle, 2022). Therefore, attention must be paid to the occurrence of AKI in patients with COVID-19, and early prevention, timely diagnosis and monitoring are important.
There is limited knowledge about the pathogenesis of AKI related to SARS-CoV-2; however, the current mainstream views are as follows. First, SARS-CoV-2 binds to ACE2 receptors on the surface of resident renal cells, including podocytes and tubular cells, to enter the cells and thus exert a direct damaging effect. Second, SARS-CoV-2 N protein can interact with Smad3 to form a complex to promote Smad3 signaling in response to TGF-β1and activate Smad3 to induce kidney cell death and cause acute kidney injury (AKI) (Wang et al., 2022). Third, the virus-induced cytokine storm and inflammation cause damage to the kidney (Ahmadian et al., 2021). Fourth, vascular endothelial dysfunction, coagulation dysfunction and complement activation may also be important mechanisms for the development of AKI in a subset of patients with COVID-19 (Nadim et al., 2020; Ahmadian et al., 2021; Han and Ye, 2021).
The efficacy of traditional Chinese medicine (TCM) in prevention and control of COVID-19, with medications having antiviral, anti-inflammatory and immune regulatory properties, can significantly reduce the hospitalisation rate and shorten the symptom recovery time (Runfeng et al., 2020; Hu et al., 2021b; Gajewski et al., 2021). Prunella vulgaris L. is a Chinese herbal medicine effective against a variety of diseases and has significant antiviral, anti-inflammatory, anti-oxidative and immunomodulatory effects (Bai et al., 2016). Early studies have reported inhibition of Prunella vulgaris on HIV, HSV and Ebola infection (Tabba et al., 1989; Yao et al., 1992; Xu et al., 1999; Zhang et al., 2016). In addition, APV can exert a significant antiviral effect by blocking the entry of SARS-CoV-2 into cells (Ao et al., 2021). What is more, P. vulgaris is the principal ingredient of Xia Sang Ju formula, a 3-herb traditional herbal remedy recommended for COVID-19 treatment by the Chinese government, and it alone can also be used as an herbal tea. APV can also protect the kidney through its anti-inflammatory and anti-oxidative effects, which are accomplished by effectively inhibiting the activation, translocation of nuclear factor kappa-B (NF-kB), and generation of ROS, thereby inhibiting the expression of inflammatory factors, such as intracellular cell adhesion molecule-1 (ICAM-1) and monocyte chemoattractant protein-1 (MCP-1) (Namgung et al., 2017). These findings suggest a potential therapeutic role for P. vulgaris in COVID-19 AKI; however, the exact mechanism has not been fully investigated.
Network pharmacology is a method for the systematic construction of the ingredients-target-disease network. It reveals the potential pathogenetic molecular mechanisms in a high-throughput manner and provides a new scientific paradigm for the study of Chinese medicine (Nogales et al., 2022). In this study, we aim to gain novel mechanistic insights into P. vulgaris and its ingredients, as potential botanical drugs against COVID-19 AKI and to guide further translational research and development. Our research provides new therapeutic ideas and targets for COVID-19 AKI.
2 Materials and methods
2.1 Obtaining active pharmaceutical ingredients and protein targets
The Traditional Chinese Medicine Systems Pharmacology Database (TCMSP, http://tcmspw.com) was used to search for the main ingredients of P. vulgaris, following which the active molecules were filtered by setting a pharmacokinetic index, which considers oral bioavailability (OB) greater than 30% and drug-like (DL) index >0.18 (Ru et al., 2014; Zheng and Wang, 2014). The targets of all active ingredients were downloaded, and protein IDs, gene symbols, as well as annotations were obtained using the Uniprot protein database (https://www.uniprot.org) and STRING database (https://string-db.org) (Consortium, 2015).
2.2 Prediction of disease targets
The COVID-19-related target genes were downloaded from the GeneCards database (http://www.genecards.org), Treatment Target Database (TTD, https://db.idrblab.org/ttd) and Human Online Mendelian Inheritance database (OMIM, https://OMIM.org) (Rebhan et al., 1997; Chen et al., 2002; Amberger et al., 2015). The AKI-related target genes were then downloaded from the DisGeNET database (http://www.disgenet.org) and the GeneCards database (Piñero et al., 2017). The intersection target of COVID-19 and AKI was obtained with the Wayne analysis tool, and these target genes were considered the relevant genes for COVID-19-induced AKI. The disease target genes were then intersected with the drug target genes to obtain the drug-disease intersection genes and were considered the possible target genes for P. vulgaris against COVID-19 AKI. The Venn diagram was drawn using the online Venny platform (https://bioinfogp.cnb.csic.es/tools/Venny/index.html).
2.3 Construction of the compound-target gene network
We used Cytoscape 3.9.1 (http://www.Cytoscape.org) to construct a network of compound-target interactions between the aforementioned drug-disease intersection targets and active compounds of the drug (Shannon et al., 2003). In the network, nodes represent the selected active compounds and targets, while the edges between the nodes represent the interactions between these molecules and genes. The connectivity between molecules and targets in the core structure of the network was determined by means of betweenness (implemented by the plug-in CytoNCA), and the molecule with a larger value was more likely to become a critical compound of COVID-19 AKI (Xia et al., 2020).
2.4 Construction of the protein-protein interaction network
The aforementioned disease-drug intersection gene targets were imported into the STRING database (https://string-db.org) to construct the protein-protein interaction (PPI) network (Szklarczyk et al., 2019). Species were selected as Homo sapiens, the meaning of network interaction was set as evidence, active sources involved Textmining, Experiments, Databases, Co-expression, Neighborhood, Gene Fusion and Co-occurrence and the minimum interaction score was set as medium confidence (0.4). Following this, the PPI network results of STRING were imported into Cytoscape 3.9.1, and the CytoNCA plugin was used to analyse network topology characteristics, with betweenness as an index to select key target genes by which P. vulgaris treat COVID-19 AKI.
2.5 GO functional enrichment and KEGG pathway analysis
Gene ontology (GO) functional annotation and Kyoto Encyclopedia of Genes and Genomes (KEGG) pathway enrichment analyses were performed for the aforementioned disease-drug intersection gene targets to reveal potential mechanisms based on biological processes (BP), cellular compounds (CC), molecular functions (MF), and critical signaling pathways. These were implemented using the ClusterProfiler package in R (version 4.1.2) (Li et al., 2021). p-values <0.05 and q-value (an adjusted p-value, taking in to account the false discovery rate) < 0.05 were considered statistically significant. In addition, visual analysis was performed using the ggplot package and pathview package in R. Cytoscape was used to network all the top 15 enriched BP, CC, MF and the top 20 KEGG pathways that intersected with the aforementioned disease-drug gene targets to identify the genes that appeared most in these enriched results.
2.6 Molecular docking technology
First, we downloaded and processed the protein structures for molecular docking. The three-dimensional structures of the proteins were downloaded from the RCSB Protein Data Bank (http://www.rcsb.org) (Berman et al., 2000). Crystal structures of proteins of human origin with a resolution greater than 2 Å were selected, and pdb files with high resolution and single proteins were downloaded. The proteins were processed using AutoDock Tools (version 1.5.6) (Morris et al., 2009), including water removal, hydrogenation, charge calculation and addition of atom type. Finally, the processed proteins were saved as PDBQT files. We then downloaded and processed the ligands for molecular docking. The 3D structure of the molecule was downloaded from PubChem (https://pubchem.ncbi.nlm.nih.gov/) and processed using AutoDock Tools (version 1.5.6) (Wang et al., 2017), involving adjusting charge, detecting and selecting rotatable keys of ligands. Molecular docking was performed using AutoDock Vina 1.12 with the setting of exhaustiveness = 10 and num_modes = 10. Each docking calculation generates ten structures that construct the least energetic ligand-protein complexes. Finally, 3D structure visualisation and 2D interaction mapping of proteins and ligands were performed using Discovery Studio Visualizer 2019, and the docking results were visualised using PyMol 2.5.2.
2.7 Molecular dynamics (MD)simulation
MD simulation were performed using Amber 22 (San Francisco, CA, United States of America) (Case et al., 2022). The ff19SB force field (Tian et al., 2019) was used to calculate the system force field parameters. Solvation was performed using the TIP3P water model, and Na+ and Cl− were added to neutralize the system. Once the system energy was minimised, the system was heated from 0 K to 300 K within 500 ps. System confinement was performed in a canonical ensemble system synthesis, followed by system pre-equilibration at 300 K. Finally, 200 ns MD simulations were carried out in an isothermal isobaric system synthesis, maintaining periodic boundary conditions. All covalent bonds involving hydrogen were constrained by the SHAKE method. The root mean square deviation (RMSD), the root mean square value of atomic fluctuations (RMSF), Solvent accessible surface area (SASA) (Weiser et al., 1999), the free energy of the binding reaction and hydrogen binding were analysed. RMSD and RMSF were defined as 1) and 2), respectively.
3 Results
3.1 Acquisition of the P. vulgaris-target and COVID-19 AKI-associated genes
We obtained 11 active compounds of P. vulgaris from the TCMSP database (Table 1), and a total of 197 drug target genes were obtained after de-duplication. In addition, we obtained 372, 52 and three genes related to COVID-19 from the GeneCards, OMIM and TTD databases, respectively, and finally acquired 356 targets after de-duplication. Following this, 163 and 1,315 AKI-related target genes were obtained from the DisGeNET and GeneCards databases, respectively, and finally 1,370 genes were obtained after de-duplication. The intersection of the target genes of AKI and COVID-19 yielded 183 genes that can be considered potential target genes for COVID-19 AKI (Figure 1A).
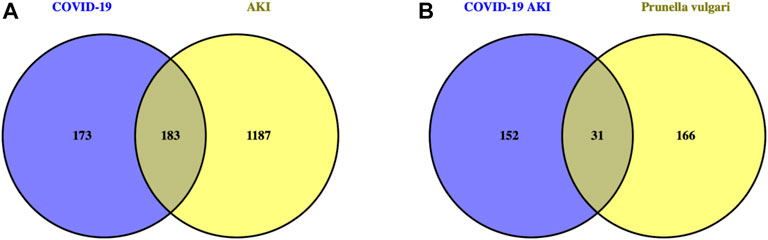
FIGURE 1. Venn diagram identifies intersection targets of drug and disease. (A). The intersection genes of AKI with COVID-19 are potential targets for COVID-19 induced AKI. (B). The intersection targets of Prunella vulgaris with COVID-19 AKI are possible target genes for Prunella vulgaris against COVID-19 AKI.
3.2 Construction of the active compound-target network
By intersecting drug target genes and disease-related genes, we finally obtained a relevant gene set, comprising 31 genes, for P. vulgaris treating COVID-19 AKI (Figure 1B). Based on the targets of the active ingredient, we found that this intersection gene set was associated with only seven active ingredients (quercetin, luteolin, morin, kaempferol, delphinidin, vulgaxanthin-I and beta-sitosterol). A composite active compounds-target interaction network with 38 nodes (31 genes and seven compounds) and 59 edges (Figure 2A) was visualised using Cytoscape 3.8.0, and node size indicated the importance of the node. We plotted the histogram of the seven chemical compounds based on the results of betweenness (indicates the proportion of the shortest paths through node to all the shortest paths) (Figure 2B) and found that five compounds, including quercetin, luteolin, morin, kaempferol and delphinidin, were more important.
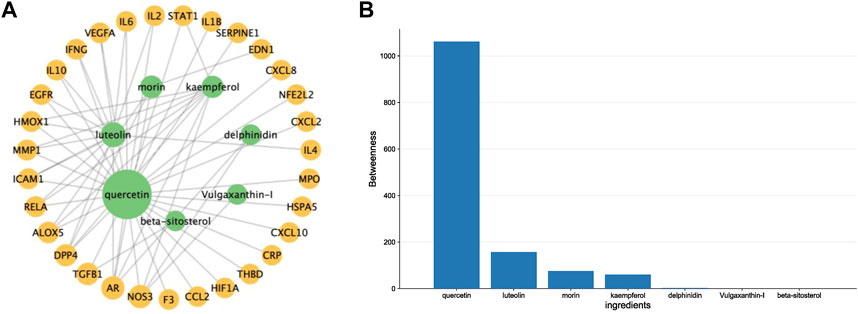
FIGURE 2. Constructing a drug-ingredients-target network to identify the crucial compounds. (A). A network diagram of Prunella vulgaris compound-target interactions was constructed by Cytoscape 3.9.1, and node size indicated the value of betweenness. (B). Histograms show betweenness for the ingredients, and the molecule with a larger value was more likely to become a critical compound of COVID-19 AKI.
3.3 Construction of the PPI network
The PPI (Figure 3A) network of the 31 drug-disease intersection genes was exported from the STRING database, and the result showed that the proteins encoded by these target genes had complex interactions. We imported the PPI network into Cytoscape for further analysis. The CytoNca plugin was used to rank the strength of action of these proteins according to the betweenness, and each node showed different circle sizes, with larger circles having higher values (Figure 3B). The top 20 ranked genes were visualised on a histogram (Figure 3C). The top 10 genes with the strongest protein interactions were IL6, VEGFA, CXCL8, IL1B, ICAM1, CCL2, IL10, HMOX1, EGFR and HIF1A.
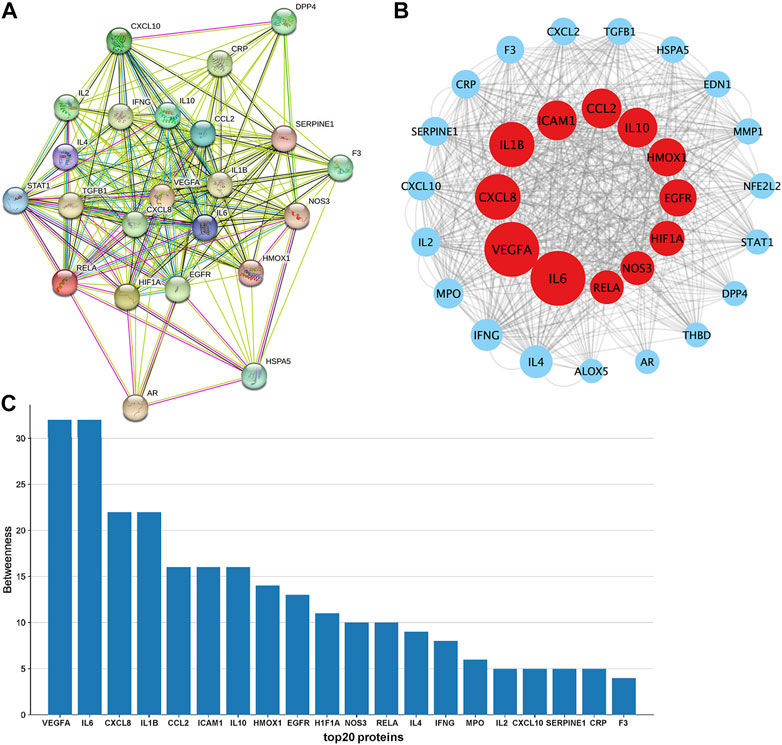
FIGURE 3. Constructing a PPI network to find essential target genes. (A). PPI network map of targets was downloaded from the STRING database. (B). PPI network map was further visualized with Cytoscape software. (C). Target genes representing the top 20 of betweenness were visualised with histograms.
3.4 GO enrichment analysis
We performed an enrichment analysis of GO functional annotation to understand the function of intersecting genes. A total of 1,560 GO terms, including 1,510, 12 and 38 biological processes (BP), cellular compounds (CC), molecular functions (MF), respectively, and the top 15 terms were identified (Figure 4A). BP are mainly enriched in cells and the body in response to external stimuli and bacteria, inflammatory responses (leukocyte migration and chemotaxis), regulation of reactive oxygen species (ROS) and apoptosis, in addition to the regulation of angiogenesis and vascular development. CC are mainly concentrated in cell membranes, vesicles, secretory granules and the extracellular matrix. MF is mainly manifested in cytokines, receptor ligands, chemokines, growth factor activity and viral receptor activity. We have presented the results of all 10 GO terms associated with viral invasion after analysis (Table 2), which showed mechanisms that could inhibit SARS-CoV-2 mainly as follows: response to virus, defense response to virus, viral life cycle, cellular response to virus, viral entry into host cell.
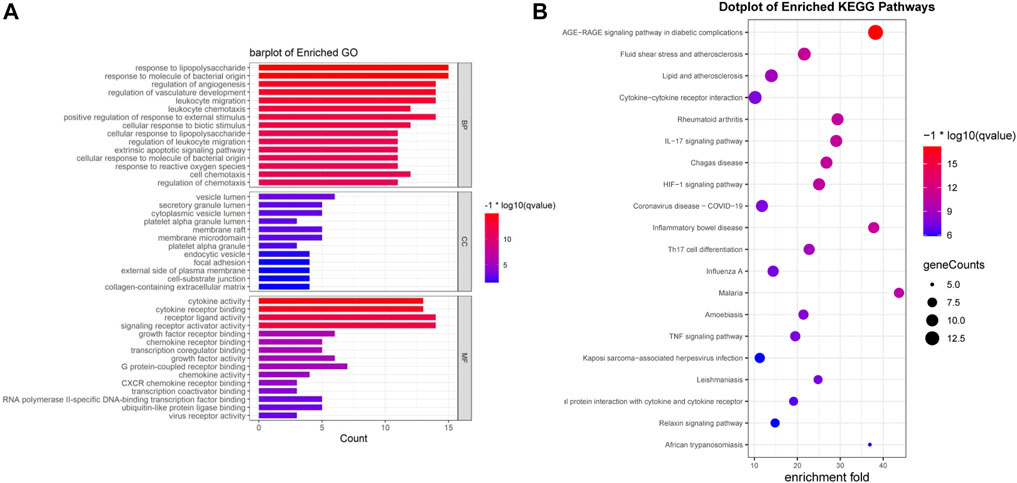
FIGURE 4. Enrichment analysis was performed for intersecting genes. (A). A total of top 15 BP, CC and MF terms are visualised with bar chat. (B). Top 20 enriched KEGG terms are visualised with bubble chart. (*p-value<0.05, *q-value<0.05).
3.5 KEGG pathway analysis
Seventy-six terms were enriched through the KEGG pathway enrichment analysis, ranked by q-value, and the top 20 KEGG terms were identified (Figure 4B). The KEGG suggests that these target genes are associated with a series of important pathological processes, such as the route of infection by external microorganisms (bacteria, viruses and protozoa), the differentiation of immune cells, and inflammation-related pathways (signal transduction pathways). We have presented the top enriched pathway map, the advanced glycation end products (AGEs)- regulation of the receptor for AGEs (RAGE) signaling pathway in diabetic complications, which shows that P. vulgaris may regulate VEGFA, IL6, IL-8 and ICAM1 expression through RELA (Figure 5A), thereby alleviating angiogenesis, thrombosis and the inflammatory response (Figures 5B,C). All top 20 KEGG pathway maps have been provided (Supplementary File S1). In addition, we have presented 13 terms associated with viral invasion based on the results of the KEGG analysis (Table 3), which suggests that these target genes play an essential role in viral infection. The top 15 BP, 12 CC, 15 MF, and the top 20 KEGG pathways and their associated genes were visualised using Cytoscape (Figure 6). The top nine genes enriched were RELA, ICAM1, IL6, TGFB1, CXCL8, VEGFA, CXCL10, IL1B and SERPINE1. Furthermore, in combination with the results of the PPI network analysis, we identified eight key target genes of P. vulgaris associated with COVID-19 AKI, including IL6, VEGFA, CXCL8, IL1B, ICAM1, CCL2, IL10 and RELA.
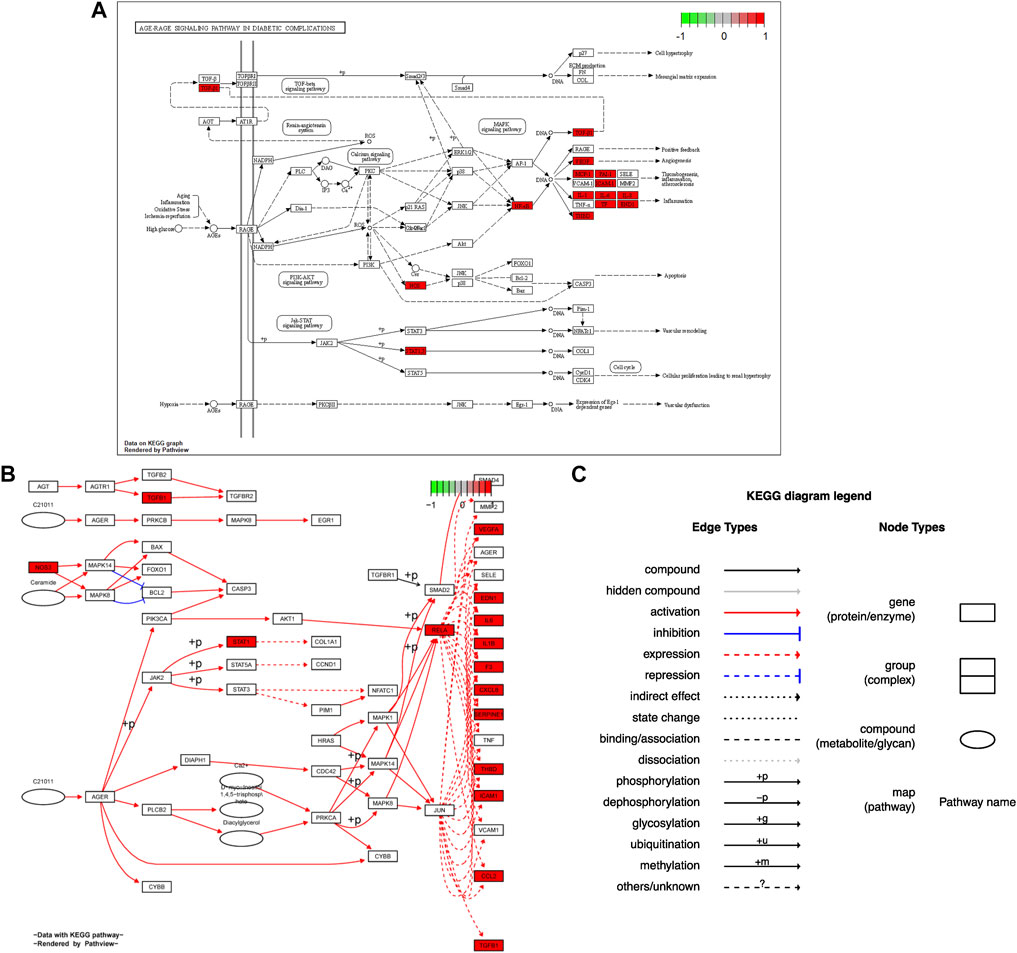
FIGURE 5. Hsa 04933 KEGG pathway map were acquired from pathview package in R. (A). Protein KEGG pathway map was present. (B). Gene KEGG pathway map was shown. (C). Annotation of gene KEGG pathway map was obtained.
3.6 Molecular docking of active compounds and eight key proteins
Based on the previous results, we identified eight key proteins that play critical roles in the effects of P. vulgaris on COVID-19 AKI and obtained their 3D structures from the RCSB Protein Data Bank (Table 4). Based on the identified drug compound targets, we found that only three drug molecules (quercetin, luteolin and kaempferol) acted on the aforementioned eight proteins. Table 5 lists these three compounds and their corresponding predicted targets. Quercetin, luteolin and kaempferol were selected for molecular docking, and their 3D structures were downloaded from PubChem (Table 6). As previously described, binding affinity less than −5.0 and −7.0 kcal/mol suggests good and strong binding activities, respectively (Liu et al., 2022). The molecular docking results (Figure 7) revealed that the three predicted ingredients demonstrated a good docking effect with their corresponding targets, and the interactions were relatively strong. Quercetin had a good affinity for all eight key proteins, luteolin had a good binding ability for RELA, ICAM1, VEGFA, IL6 and CXCL8, and kaempferol could effectively bind to ICAM1. Thus, the results suggested that these three active compounds, especially quercetin, exerted protective effects against COVID-19 AKI by regulating the aforementioned key target genes.
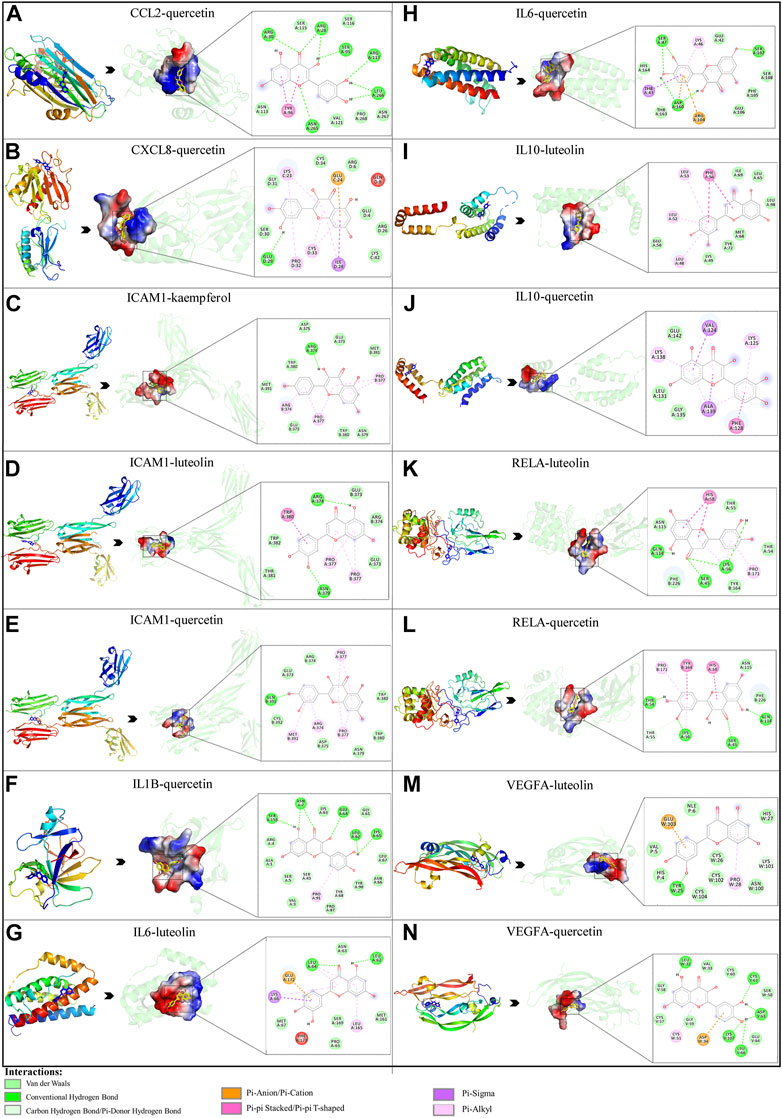
FIGURE 7. Molecular docking of three active compounds and eight proteins were performed. (A–N) A total of 14 docking results were obtained. On the left of each docking result, 3D structures of ligands and receptors are shown, with active pockets of proteins highlighted in the middle and 2D interaction maps on the right.
3.7 MD simulation
Considering that RELA-encoded protein (NF-κB) presents the highest docking scores with molecules, it was chosen for MD simulations. After 200 ns of long-scale MD simulations, the RMSD trend of protein α-carbon atoms (protein backbone) and ligands in the simulated trajectories of the two systems over time showed that the RMSD was small for both RELA-luteolin and RELA-quercetin systems, with the RELA-luteolin system undergoing conformational transitions in a small conformational space and RELA-quercetin system fluctuating in a relatively constant conformational space (Figures 8A,B). The RMSF represents the flexibility changes of the protein during the simulation. The protein in RELA-luteolin and RELA-quercetin shows similar flexibility changes, with larger fluctuations in the loop conformation around residues 150 and 280, consistent with the high flexibility of the loops (Figures 8C,D). The Solvent accessible surface area (SASA) reflects the relative surface area contribution of the ligand exposed to the solvent and the SASA value fluctuated within a relatively constant range for both RELA-luteolin and RELA-quercetin systems (Figure 8E), which is consistent with the stability of the RMSD analysis. Free energy calculations using the Molecular Mechanics-Poisson Bolzmann Surface Area (MM/PBSA) method showed that the free energies of both RELA-luteolin and RELA-quercetin systems were relatively stable (Table 7; Table 8). Additionally, the hydrogen bond analysis indicates strong hydrogen bond interactions in both systems (Figure 8F; Figure 8G; Table 9; Table 10).
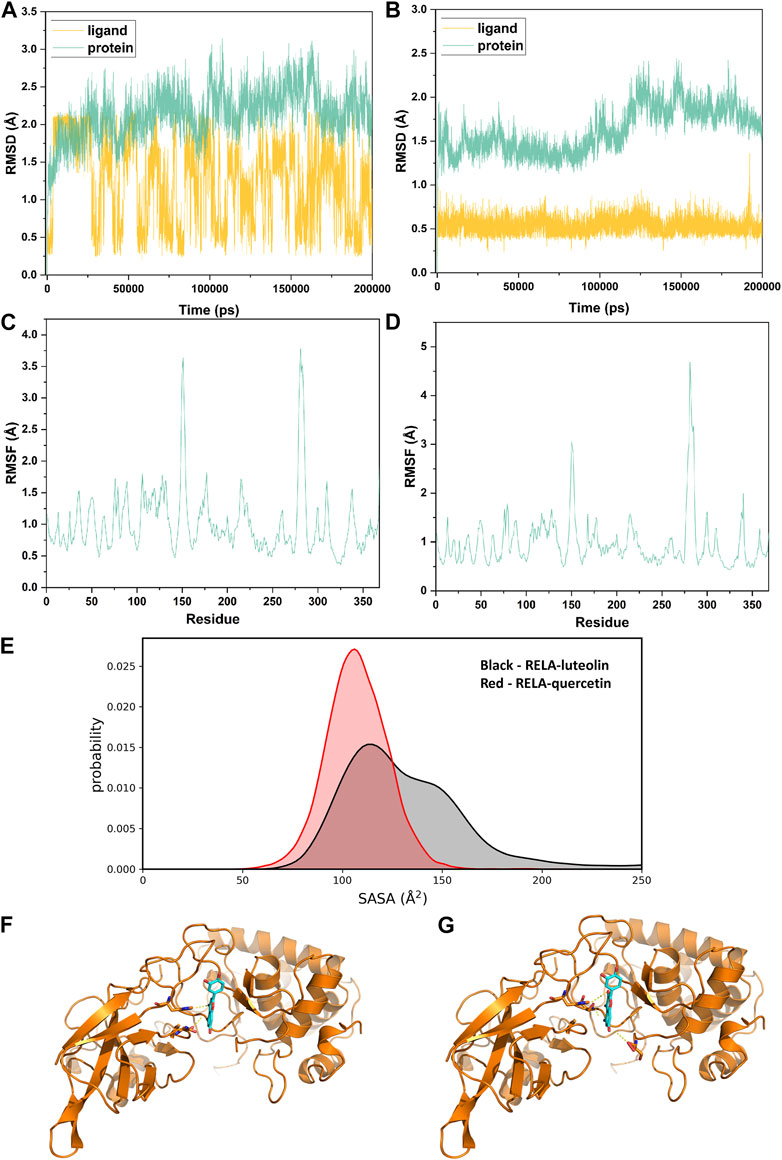
FIGURE 8. MD simulation analysis. (A) and (B). The RMSD value of RELA-luteolin and RELA-quercetin, respectively. (C) and (D). Changes in protein flexibility during simulation of luteolin and quercetin, respectively. (E). Relative surface area contribution of the ligand exposed to the solvent of luteolin and quercetin. (F) and (G). Kinetic conformation of the RELA-luteolin and RELA-quercetin systems, respectively.
Overall, both RELA-luteolin and RELA-quercetin show good kinetic properties, indicating the correct docking pose. Both luteolin and quercetin fall in small conformational space domains with stable kinetic characteristics of the systems. In addition, they both have strong binding energies and stable hydrogen bond interactions. The results showed that both components luteolin and quercetin of P. vulgaris could stably bind NF-κB, which suggested that P. vulgaris could ameliorate COVID-19 AKI through NF-κB.
Discussion
The incidence of COVID-19-associated acute kidney injury (AKI) has received increasing attention, particularly in severe cases. Traditional Chinese Medicine (TCM) has made significant contributions to the medical field, with reported preventative and therapeutic effects against COVID-19 and AKI (Li et al., 2019; Huang Y. F. et al., 2020; Gajewski et al., 2021). In this study, we developed a P. vulgaris target COVID-19–related gene set comprising of 31 genes. Analysis using GO and KEGG revealed that P. vulgaris can regulate the inflammatory response process and virus defence mechanism. Furthermore, we identified eight key target genes from the total of 31 genes via PPI network and GO and KEGG network analyses. Amongst all the molecules, RELA-encoded protein (NF-κB) showed the highest docking scores; therefore, it was chosen for MD simulations. These results can potentially promote basic research on SARS-CoV-2 infection and help with target drug design.
P. vulgaris is a traditional Chinese herb used in the treatment of various kidney diseases, including diabetic nephropathy and AKI (Bai et al., 2016; Peng et al., 2016; Namgung et al., 2017; Wu et al., 2020). Studies have shown that P. vulgaris can inhibit SARS-CoV-2, preventing it from entering cells by blocking the binding of the virus to the ACE2 receptor using its aqueous extract (APV) (Ao et al., 2021). Therefore, we hypothesize that P. vulgaris may be pharmacologically effective in treating COVID-19 AKI due to its inhibitory effects on SARS-CoV-2 and protective effects on the kidney. According to the principles of network pharmacology, we first identified effective active compounds of P. vulgaris that may ameliorate COVID-19 AKI. Initially, 11 active molecules of P. vulgaris were selected from the TCM database based on OB and DL, followed by the construction of an ingredients-targets network map and molecular docking, three ingredients including quercetin, luteolin and kaempferol were found most significant, as they showed revealed strong interactions with the previously mentioned eight key target proteins. The three identified molecules belong to the group of flavonoid steroids (Kang et al., 2011; Yu et al., 2020; Derosa et al., 2021) and are potential compounds for the treatment of COVID-19 (Luo et al., 2020; Yu et al., 2020; Derosa et al., 2021). Quercetin, luteolin and kaempferol play important roles in the activity of several TCMs against COVID-19. A clinical trial found that quercetin is safe and effective in lowering the serum levels of ALP, q-CRP, and LDH as critical markers involved in COVID-19 severity (Shohan et al., 2022). In addition, their renal protective effects have also been verified in several studies, wherein quercetin has been shown to ameliorate renal injury by inhibiting iron death as well as downregulating the phosphorylation of NF-κB (Tan et al., 2020; Wang et al., 2021), luteolin by regulating P53-dependent tubular apoptosis (Kang et al., 2011), and kaempferol by improving cell injury, oxidative stress and inflammation (Yuan et al., 2021).
Combined with the PPI network and the GO and KEGG-target network, eight key target genes of P. vulgaris against COVID-19 AKI were identified, including IL6, VEGFA, CXCL8, IL1B, ICAM1, CCL2, IL10 and RELA. The expression level of these eight genes was strongly associated with COVID-19 AKI. The secretion of cytokines and chemokines, including IL6, CXCL8, IL1B, IL10 and CCL2, is significantly increased in COVID-19 AKI. These inflammatory factors participate in the cytokine storm and act as the important agents causing renal injury (Ahmadian et al., 2021). Studies have shown that IL6 is the most critical mediator in COVID-19 and is closely associated with poor prognosis and mortality (Coomes and Haghbayan, 2020). Excessive IL6 signaling causes several biological effects, such as induction of vascular endothelial growth factor (VEGF) expression, thereby increasing vascular permeability, hyperactivation of T-helper 17 (Th17) cells, and induction of effector T-cell death, resulting in kidney injury (Nechemia-Arbely et al., 2008; Liu et al., 2020). The expression levels of CXCL8, IL1B, CCL-2 and IL10 are also significantly upregulated after SARS-CoV-2 infection and are related to the severity of the disease. CXCL8 control the chemotactic activity of neutrophils and monocytes, IL1B increase the production of IL6 and participate in the differentiation of Th17 cells, CCL-2/MCP-1 can chemoattract monocytes, and the expression of IL10, an anti-inflammatory factor, are feedback increased. These cytokines can result in AKI by causing excessive inflammatory responses (Coperchini et al., 2020; Ahmadian et al., 2021). ICAM1 promotes leukocyte migration through the endothelium, which leads to the infiltration of inflammatory cells and aggravates tissue damage (Ahmadian et al., 2021). VEGFA, a marker of vascular endothelial cell damage, is regulated by hypoxia-inducible factor (HIF). VEGFA is primarily involved in angiogenesis and vascular permeability and is significantly increased in patients with COVID-19, wherein it correlates with the disease severity (Rovas et al., 2021). In addition, TGFB1 is also a related target in the 31genes obtained by intersecting drug target genes and disease-related genes, which may regulate the smad3 activation. As SARS-CoV-2 N protein can enhance TGF-β/Smad3 signaling to cause tubular epithelial cell death and AKI via the G1 cell cycle arrest mechanism, the regulation of TGFB1 might be significant in COVID-19 AKI (Wang et al., 2022).
Molecular docking results revealed that quercetin could bind to all eight key target proteins; luteolin could bind to RELA, ICAM1, VEGFA, IL6 and CXCL8, and kaempferol could bind to ICAM1. Quercetin, luteolin and kaempferol have been reported to have anti-inflammatory and anti-oxidative properties. They can downregulate the production of ICAM1 and MCP-1 to reduce the infiltration of mononuclear cells and inhibit the expression of inflammatory cytokines IL6, TNF-α, IL8 and IL1B through the NF-κB signaling pathway (Li et al., 2016; Hu et al., 2019). In addition, these compounds can reduce ROS production and VEGF protein concentrations through the Nrf2/HO-1 signaling pathway to alleviate oxidative stress and improve vascular function (Jia et al., 2015; Boeing et al., 2020; Yao et al., 2020; Ozyel et al., 2021). Therefore, it is reasonable to say that P. vulgaris can modulate the cytokine storm and improve inflammation, oxidative stress and vascular endothelial function in patients with COVID-19 by targeting the eight core proteins, thereby reducing renal injury.
GO and KEGG pathway analysis revealed that drug-disease intersection genes were substantially enriched in virus-related functions and pathways, which verified the ability of P. vulgaris against viruses. GO enrichment mainly focused on inflammation, cytokines, oxidative stress and angiogenesis, among other factors, which coincided with the biological effects of the eight key target genes described earlier. Analysis of the top 20 KEGG signaling pathways revealed that these target genes were mainly enriched in HIF-1, VEGF, NF-κB, JAK/STAT, Th17 cell differentiation, TNF-α and Nrf2 signaling pathways. NF-κB signaling pathway was enriched to the most number of key target genes, and based on its significant effect on COVID-19 (Hariharan et al., 2021), it can be inferred that it is the most important signaling pathway of P. vulgaris in antagonising COVID-19 AKI. The effects are accomplished by anti-oxidative and anti-inflammatory actions, improvement in the function of blood vessels, and by reducing inflammatory factors. Th17 cells also play an important role in the pathogenesis of COVID-19. These cells can promote neutrophil migration and reduce Treg responses by releasing cytokines, such as IL-17 and GM-CSF and thus exacerbate inflammation. This is also a key mechanism causing kidney injury (Martonik et al., 2021).
Because of the highest docking score of RELA-encoded protein (NF-κB) with luteolin and quercetin, NF-κB was selected to perform the MD simulation to further examine the binding effect of NF-κB with luteolin and quercetin, respectively, which verified the result of molecular docking. And the MD simulation results suggested good binding stability. In most cells, NF-κB complexes remain inactive and are mainly located in the cytoplasm where they interact with inhibitory IκB proteins. However, when signaling pathways become activated, the IκB protein is degraded, allowing NF-κB dimers to translocate into the nucleus and regulate the expression of target genes (Hayden and Ghosh, 2011). RELA encodes NF-κB, which can be activated by SARS-CoV-2 Viral DNA and RNA, ROS and cytokines, such as TNF-α, IL1B and IL6, can cause nuclear translocation of NF-κB. (Hariharan et al., 2021). NF-κB can participate in host immunity, inflammation, and regulation of proliferation, differentiation, and apoptosis of B cell and T lymphocytes (Hayden and Ghosh, 2011). Activated NF-κB promotes the expression of multiple cytokines (IL1, IL2, IL6, IL12, TNF-α, LT-α, LT-1β and GM-CSF), chemokines (IL8, MIP-1 and MCP-1), adhesion molecules (ICAM, VCAM and E-selectin), and induces effector enzymes (inducible nitric oxide synthase [iNOS]) (Hariharan et al., 2021), which can aggravate the cytokine storm and oxidative stress on the kidney. As mentioned in the manuscript, NF-κB pathway is a major pathway in this study, which can regulate the expression of other core protein, such as IL6. And the inhibition of NF-κB pathway has the potential to reduce the effect of cytokine storm and improve oxidative stress and vascular function. In summary, NF-κB may be the most potential target for the therapy of P. vulgaris on COVID-19 AKI.
In the present study, we investigated the potential therapeutic mechanism of the Chinese medicine P. vulgaris in COVID-19 AKI based on network pharmacology and molecular docking approaches. Our findings indicated that NF-κB might be the potent pharmacological target of P. vulgaris against COVID-19 induced AKI. In addition, we provided several potential targets for COVID-19 AKI treatment, mainly IL6, VEGFA and RELA, which may help to develop new therapeutic strategies. However, as an in silico study, our results have not been experimentally verified. But it is still valuable which can and can provide guidance for drug design and further investigations warranted by the study, such as biological studies on binding affinity and activities of target proteins; preclinical and clinical efficacy and toxicity, etc.
Data availability statement
The original contributions presented in the study are included in the article/Supplementary Materials, further inquiries can be directed to the corresponding authors.
Author contributions
J-RY and KL designed the study; X-LY, C-XW, J-XW, S-MW, QY performed the experiments and collected and analyzed the data; J-RY, KL and X-LY wrote and revised the manuscript. All authors listed have made a substantial, direct, and intellectual contribution to the work and approved it for publication.
Funding
This work was financially supported by the National Natural Science Foundation of China, Grant/Award Numbers: 82270723, 81770682 and the Chongqing Talent Program Project, Grant/Award Number: cstc2021ycjh-bgzxm0090.
Conflict of interest
The authors declare that the research was conducted in the absence of any commercial or financial relationships that could be construed as a potential conflict of interest.
Publisher’s note
All claims expressed in this article are solely those of the authors and do not necessarily represent those of their affiliated organizations, or those of the publisher, the editors and the reviewers. Any product that may be evaluated in this article, or claim that may be made by its manufacturer, is not guaranteed or endorsed by the publisher.
Supplementary material
The Supplementary Material for this article can be found online at: https://www.frontiersin.org/articles/10.3389/fphar.2023.1188086/full#supplementary-material
References
Ahmadian, E., Hosseiniyan Khatibi, S. M., Razi Soofiyani, S., Abediazar, S., Shoja, M. M., Ardalan, M., et al. (2021). Covid-19 and kidney injury: Pathophysiology and molecular mechanisms. Rev. Med. Virol. 31 (3), e2176. doi:10.1002/rmv.2176
Amberger, J. S., Bocchini, C. A., Schiettecatte, F., Scott, A. F., and Hamosh, A. (2015). OMIM.org: Online Mendelian Inheritance in Man (OMIM®), an online catalog of human genes and genetic disorders. Nucleic Acids Res. 43, D789–D798. Database issue). doi:10.1093/nar/gku1205
Ao, Z., Chan, M., Ouyang, M. J., Olukitibi, T. A., Mahmoudi, M., Kobasa, D., et al. (2021). Identification and evaluation of the inhibitory effect of Prunella vulgaris extract on SARS-coronavirus 2 virus entry. PLoS One 16 (6), e0251649. doi:10.1371/journal.pone.0251649
Bai, Y., Xia, B., Xie, W., Zhou, Y., Xie, J., Li, H., et al. (2016). Phytochemistry and pharmacological activities of the genus Prunella. Food Chem. 204, 483–496. doi:10.1016/j.foodchem.2016.02.047
Berman, H. M., Westbrook, J., Feng, Z., Gilliland, G., Bhat, T. N., Weissig, H., et al. (2000). The protein data bank. Nucleic Acids Res. 28 (1), 235–242. doi:10.1093/nar/28.1.235
Boeing, T., De Souza, P., Speca, S., Somensi, L. B., Mariano, L. N. B., Cury, B. J., et al. (2020). Luteolin prevents irinotecan-induced intestinal mucositis in mice through antioxidant and anti-inflammatory properties. Br. J. Pharmacol. 177 (10), 2393–2408. doi:10.1111/bph.14987
Case, D. A., Duke, R. E., Walker, R. C., Skrynnikov, N. R., Cheatham Iii, T. E., Mikhailovskii, O., et al. (2022). AMBER 22 reference manual. San Francisco: University of California.
Chen, N., Zhou, M., Dong, X., Qu, J., Gong, F., Han, Y., et al. (2020). Epidemiological and clinical characteristics of 99 cases of 2019 novel coronavirus pneumonia in wuhan, China: A descriptive study. Lancet 395 (10223), 507–513. doi:10.1016/s0140-6736(20)30211-7
Chen, X., Ji, Z. L., and Chen, Y. Z. (2002). Ttd: Therapeutic target database. Nucleic Acids Res. 30 (1), 412–415. doi:10.1093/nar/30.1.412
Cheng, Y., Luo, R., Wang, K., Zhang, M., Wang, Z., Dong, L., et al. (2020). Kidney disease is associated with in-hospital death of patients with COVID-19. Kidney Int. 97 (5), 829–838. doi:10.1016/j.kint.2020.03.005
Consortium, U. (2015). UniProt: A hub for protein information. Nucleic Acids Res. 43, D204–D212. Database issue. doi:10.1093/nar/gku989
Coomes, E. A., and Haghbayan, H. (2020). Interleukin-6 in covid-19: A systematic review and meta-analysis. Rev. Med. Virol. 30 (6), 1–9. doi:10.1002/rmv.2141
Coperchini, F., Chiovato, L., Croce, L., Magri, F., and Rotondi, M. (2020). The cytokine storm in COVID-19: An overview of the involvement of the chemokine/chemokine-receptor system. Cytokine Growth Factor Rev. 53, 25–32. doi:10.1016/j.cytogfr.2020.05.003
Daniels, G. E., and Morton, M. H. (2023). COVID-19 recession: Young adult food insecurity, racial disparities, and correlates. J. Adolesc. Health 72 (2), 237–245. doi:10.1016/j.jadohealth.2022.09.008
Derosa, G., Maffioli, P., D'angelo, A., and Di Pierro, F. (2021). A role for quercetin in coronavirus disease 2019 (COVID-19). Phytother. Res. 35 (3), 1230–1236. doi:10.1002/ptr.6887
Dong, E., Du, H., and Gardner, L. (2020). An interactive web-based dashboard to track COVID-19 in real time. Lancet Infect. Dis. 20 (5), 533–534. doi:10.1016/s1473-3099(20)30120-1
Gajewski, A., Kośmider, A., Nowacka, A., Puk, O., and Wiciński, M. (2021). Potential of herbal products in prevention and treatment of COVID-19. Literature review. Biomed. Pharmacother. 143, 112150. doi:10.1016/j.biopha.2021.112150
Guan, W. J., Ni, Z. Y., Hu, Y., Liang, W. H., Ou, C. Q., He, J. X., et al. (2020). Clinical characteristics of coronavirus disease 2019 in China. N. Engl. J. Med. 382 (18), 1708–1720. doi:10.1056/NEJMoa2002032
Han, X., and Ye, Q. (2021). Kidney involvement in COVID-19 and its treatments. J. Med. Virol. 93 (3), 1387–1395. doi:10.1002/jmv.26653
Hariharan, A., Hakeem, A. R., Radhakrishnan, S., Reddy, M. S., and Rela, M. (2021). The role and therapeutic potential of NF-kappa-B pathway in severe COVID-19 patients. Inflammopharmacology 29 (1), 91–100. doi:10.1007/s10787-020-00773-9
Hayden, M. S., and Ghosh, S. (2011). NF-κB in immunobiology. Cell Res. 21 (2), 223–244. doi:10.1038/cr.2011.13
Hu, B., Guo, H., Zhou, P., and Shi, Z. L. (2021a). Characteristics of SARS-CoV-2 and COVID-19. Nat. Rev. Microbiol. 19 (3), 141–154. doi:10.1038/s41579-020-00459-7
Hu, K., Guan, W. J., Bi, Y., Zhang, W., Li, L., Zhang, B., et al. (2021b). Efficacy and safety of lianhuaqingwen capsules, a repurposed Chinese herb, in patients with coronavirus disease 2019: A multicenter, prospective, randomized controlled trial. Phytomedicine 85, 153242. doi:10.1016/j.phymed.2020.153242
Hu, Y., Gui, Z., Zhou, Y., Xia, L., Lin, K., and Xu, Y. (2019). Quercetin alleviates rat osteoarthritis by inhibiting inflammation and apoptosis of chondrocytes, modulating synovial macrophages polarization to M2 macrophages. Free Radic. Biol. Med. 145, 146–160. doi:10.1016/j.freeradbiomed.2019.09.024
Huang, C., Wang, Y., Li, X., Ren, L., Zhao, J., Hu, Y., et al. (2020a). Clinical features of patients infected with 2019 novel coronavirus in Wuhan, China. Lancet 395 (10223), 497–506. doi:10.1016/s0140-6736(20)30183-5
Huang, Y. F., Bai, C., He, F., Xie, Y., and Zhou, H. (2020b). Review on the potential action mechanisms of Chinese medicines in treating Coronavirus Disease 2019 (COVID-19). Pharmacol. Res. 158, 104939. doi:10.1016/j.phrs.2020.104939
Jia, Z., Nallasamy, P., Liu, D., Shah, H., Li, J. Z., Chitrakar, R., et al. (2015). Luteolin protects against vascular inflammation in mice and TNF-alpha-induced monocyte adhesion to endothelial cells via suppressing IΚBα/NF-κB signaling pathway. J. Nutr. Biochem. 26 (3), 293–302. doi:10.1016/j.jnutbio.2014.11.008
Kang, K. P., Park, S. K., Kim, D. H., Sung, M. J., Jung, Y. J., Lee, A. S., et al. (2011). Luteolin ameliorates cisplatin-induced acute kidney injury in mice by regulation of p53-dependent renal tubular apoptosis. Nephrol. Dial. Transpl. 26 (3), 814–822. doi:10.1093/ndt/gfq528
Kudose, S., Batal, I., Santoriello, D., Xu, K., Barasch, J., Peleg, Y., et al. (2020). Kidney biopsy findings in patients with COVID-19. J. Am. Soc. Nephrol. 31 (9), 1959–1968. doi:10.1681/asn.2020060802
Li, H. D., Meng, X. M., Huang, C., Zhang, L., Lv, X. W., and Li, J. (2019). Application of herbal traditional Chinese medicine in the treatment of acute kidney injury. Front. Pharmacol. 10, 376. doi:10.3389/fphar.2019.00376
Li, R., Li, Y., Liang, X., Yang, L., Su, M., and Lai, K. P. (2021). Network Pharmacology and bioinformatics analyses identify intersection genes of niacin and COVID-19 as potential therapeutic targets. Brief. Bioinform 22 (2), 1279–1290. doi:10.1093/bib/bbaa300
Li, Y., Yao, J., Han, C., Yang, J., Chaudhry, M. T., Wang, S., et al. (2016). Quercetin, inflammation and immunity. Nutrients 8 (3), 167. doi:10.3390/nu8030167
Lin, L., Deng, J., Li, J., Zheng, L., Wu, Z., Tan, W., et al. (2022). Pathogenesis and histological changes of nephropathy associated with COVID-19. J. Med. Virol. 95, e28311. doi:10.1002/jmv.28311
Lin, L., Wang, X., Ren, J., Sun, Y., Yu, R., Li, K., et al. (2020). Risk factors and prognosis for COVID-19-induced acute kidney injury: A meta-analysis. BMJ Open 10 (11), e042573. doi:10.1136/bmjopen-2020-042573
Liu, B., Li, M., Zhou, Z., Guan, X., and Xiang, Y. (2020). Can we use interleukin-6 (IL-6) blockade for coronavirus disease 2019 (COVID-19)-induced cytokine release syndrome (CRS)? J. Autoimmun. 111, 102452. doi:10.1016/j.jaut.2020.102452
Liu, J., Sun, T., Liu, S., Liu, J., Fang, S., Tan, S., et al. (2022). Dissecting the molecular mechanism of cepharanthine against COVID-19, based on a network pharmacology strategy combined with RNA-sequencing analysis, molecular docking, and molecular dynamics simulation. Comput. Biol. Med. 151, 106298. doi:10.1016/j.compbiomed.2022.106298
Luo, L., Jiang, J., Wang, C., Fitzgerald, M., Hu, W., Zhou, Y., et al. (2020). Analysis on herbal medicines utilized for treatment of COVID-19. Acta Pharm. Sin. B 10 (7), 1192–1204. doi:10.1016/j.apsb.2020.05.007
Martonik, D., Parfieniuk-Kowerda, A., Rogalska, M., and Flisiak, R. (2021). The role of Th17 response in COVID-19. Cells 10 (6), 1550. doi:10.3390/cells10061550
Matsumoto, K., and Prowle, J. R. (2022). COVID-19-associated AKI. Curr. Opin. Crit. Care 28 (6), 630–637. doi:10.1097/mcc.0000000000000988
Morris, G. M., Huey, R., Lindstrom, W., Sanner, M. F., Belew, R. K., Goodsell, D. S., et al. (2009). AutoDock4 and AutoDockTools4: Automated docking with selective receptor flexibility. J. Comput. Chem. 30 (16), 2785–2791. doi:10.1002/jcc.21256
Nadim, M. K., Forni, L. G., Mehta, R. L., Connor, M. J., Liu, K. D., Ostermann, M., et al. (2020). COVID-19-associated acute kidney injury: Consensus report of the 25th acute disease quality initiative (ADQI) workgroup. Nat. Rev. Nephrol. 16 (12), 747–764. doi:10.1038/s41581-020-00356-5
Namgung, S., Yoon, J. J., Yoon, C. S., Han, B. H., Choi, E. S., Oh, H., et al. (2017). Prunella vulgaris attenuates diabetic renal injury by suppressing glomerular fibrosis and inflammation. Am. J. Chin. Med. 45 (3), 475–495. doi:10.1142/s0192415x1750029x
Nechemia-Arbely, Y., Barkan, D., Pizov, G., Shriki, A., Rose-John, S., Galun, E., et al. (2008). IL-6/IL-6R axis plays a critical role in acute kidney injury. J. Am. Soc. Nephrol. 19 (6), 1106–1115. doi:10.1681/asn.2007070744
Nogales, C., Mamdouh, Z. M., List, M., Kiel, C., Casas, A. I., and Schmidt, H. (2022). Network pharmacology: Curing causal mechanisms instead of treating symptoms. Trends Pharmacol. Sci. 43 (2), 136–150. doi:10.1016/j.tips.2021.11.004
Ozyel, B., Le Gall, G., Needs, P. W., and Kroon, P. A. (2021). Anti-inflammatory effects of quercetin on high-glucose and pro-inflammatory cytokine challenged vascular endothelial cell metabolism. Mol. Nutr. Food Res. 65 (6), e2000777. doi:10.1002/mnfr.202000777
Peng, J., Ren, X., Lan, T., Chen, Y., Shao, Z., and Yang, C. (2016). Renoprotective effects of ursolic acid on ischemia/reperfusion-induced acute kidney injury through oxidative stress, inflammation and the inhibition of STAT3 and NF-κB activities. Mol. Med. Rep. 14 (4), 3397–3402. doi:10.3892/mmr.2016.5654
Piñero, J., Bravo, À., Queralt-Rosinach, N., Gutiérrez-Sacristán, A., Deu-Pons, J., Centeno, E., et al. (2017). DisGeNET: A comprehensive platform integrating information on human disease-associated genes and variants. Nucleic Acids Res. 45 (D1), D833–D839. doi:10.1093/nar/gkw943
Rebhan, M., Chalifa-Caspi, V., Prilusky, J., and Lancet, D. (1997). GeneCards: Integrating information about genes, proteins and diseases. Trends Genet. 13 (4), 163. doi:10.1016/s0168-9525(97)01103-7
Rovas, A., Osiaevi, I., Buscher, K., Sackarnd, J., Tepasse, P. R., Fobker, M., et al. (2021). Microvascular dysfunction in COVID-19: The MYSTIC study. Angiogenesis 24 (1), 145–157. doi:10.1007/s10456-020-09753-7
Ru, J., Li, P., Wang, J., Zhou, W., Li, B., Huang, C., et al. (2014). Tcmsp: A database of systems pharmacology for drug discovery from herbal medicines. J. Cheminform 6, 13. doi:10.1186/1758-2946-6-13
Runfeng, L., Yunlong, H., Jicheng, H., Weiqi, P., Qinhai, M., Yongxia, S., et al. (2020). Lianhuaqingwen exerts anti-viral and anti-inflammatory activity against novel coronavirus (SARS-CoV-2). Pharmacol. Res. 156, 104761. doi:10.1016/j.phrs.2020.104761
Shannon, P., Markiel, A., Ozier, O., Baliga, N. S., Wang, J. T., Ramage, D., et al. (2003). Cytoscape: A software environment for integrated models of biomolecular interaction networks. Genome Res. 13 (11), 2498–2504. doi:10.1101/gr.1239303
Shohan, M., Nashibi, R., Mahmoudian-Sani, M. R., Abolnezhadian, F., Ghafourian, M., Alavi, S. M., et al. (2022). The therapeutic efficacy of quercetin in combination with antiviral drugs in hospitalized COVID-19 patients: A randomized controlled trial. Eur. J. Pharmacol. 914, 174615. doi:10.1016/j.ejphar.2021.174615
Szklarczyk, D., Gable, A. L., Lyon, D., Junge, A., Wyder, S., Huerta-Cepas, J., et al. (2019). STRING v11: Protein-protein association networks with increased coverage, supporting functional discovery in genome-wide experimental datasets. Nucleic Acids Res. 47 (1), D607–D613. doi:10.1093/nar/gky1131
Tabba, H. D., Chang, R. S., and Smith, K. M. (1989). Isolation, purification, and partial characterization of prunellin, an anti-HIV component from aqueous extracts of Prunella vulgaris. Antivir. Res. 11 (5-6), 263–273. doi:10.1016/0166-3542(89)90036-3
Tan, R. Z., Wang, C., Deng, C., Zhong, X., Yan, Y., Luo, Y., et al. (2020). Quercetin protects against cisplatin-induced acute kidney injury by inhibiting Mincle/Syk/NF-κB signaling maintained macrophage inflammation. Phytother. Res. 34 (1), 139–152. doi:10.1002/ptr.6507
Tian, C., Kasavajhala, K., Belfon, K. A., Raguette, L., Huang, H., Migues, A. N., et al. (2019). ff19SB: Amino-acid-specific protein backbone parameters trained against quantum mechanics energy surfaces in solution. J. Chem. theory Comput. 16 (1), 528–552. doi:10.1021/acs.jctc.9b00591
Wang, W., Chen, J., Hu, D., Pan, P., Liang, L., Wu, W., et al. (2022). SARS-CoV-2 N protein induces acute kidney injury via smad3-dependent G1 cell cycle arrest mechanism. Adv. Sci. (Weinh) 9 (3), e2103248. doi:10.1002/advs.202103248
Wang, Y., Bryant, S. H., Cheng, T., Wang, J., Gindulyte, A., Shoemaker, B. A., et al. (2017). PubChem BioAssay: 2017 update. Nucleic Acids Res. 45 (D1), D955–D963. doi:10.1093/nar/gkw1118
Wang, Y., Quan, F., Cao, Q., Lin, Y., Yue, C., Bi, R., et al. (2021). Quercetin alleviates acute kidney injury by inhibiting ferroptosis. J. Adv. Res. 28, 231–243. doi:10.1016/j.jare.2020.07.007
Weiser, J., Shenkin, P. S., and Still, W. C. (1999). Approximate atomic surfaces from linear combinations of pairwise overlaps (LCPO). J. Comput. Chem. 20 (2), 217–230. doi:10.1002/(sici)1096-987x(19990130)20:2<217::aid-jcc4>3.0.co;2-a
Wu, W. F., Wang, J. N., Li, Z., Wei, B., Jin, J., Gao, L., et al. (2020). 7-Hydroxycoumarin protects against cisplatin-induced acute kidney injury by inhibiting necroptosis and promoting Sox9-mediated tubular epithelial cell proliferation. Phytomedicine 69, 153202. doi:10.1016/j.phymed.2020.153202
Xia, Q. D., Xun, Y., Lu, J. L., Lu, Y. C., Yang, Y. Y., Zhou, P., et al. (2020). Network pharmacology and molecular docking analyses on Lianhua Qingwen capsule indicate Akt1 is a potential target to treat and prevent COVID-19. Cell Prolif. 53 (12), e12949. doi:10.1111/cpr.12949
Xu, H. X., Lee, S. H., Lee, S. F., White, R. L., and Blay, J. (1999). Isolation and characterization of an anti-HSV polysaccharide from Prunella vulgaris. Antivir. Res. 44 (1), 43–54. doi:10.1016/s0166-3542(99)00053-4
Yang, X., Yu, Y., Xu, J., Shu, H., Xia, J., Liu, H., et al. (2020). Clinical course and outcomes of critically ill patients with SARS-CoV-2 pneumonia in wuhan, China: A single-centered, retrospective, observational study. Lancet Respir. Med. 8 (5), 475–481. doi:10.1016/s2213-2600(20)30079-5
Yao, H., Sun, J., Wei, J., Zhang, X., Chen, B., and Lin, Y. (2020). Kaempferol protects blood vessels from damage induced by oxidative stress and inflammation in association with the Nrf2/HO-1 signaling pathway. Front. Pharmacol. 11, 1118. doi:10.3389/fphar.2020.01118
Yao, X. J., Wainberg, M. A., and Parniak, M. A. (1992). Mechanism of inhibition of HIV-1 infection in vitro by purified extract of Prunella vulgaris. Virology 187 (1), 56–62. doi:10.1016/0042-6822(92)90294-y
Yu, R., Chen, L., Lan, R., Shen, R., and Li, P. (2020). Computational screening of antagonists against the SARS-CoV-2 (COVID-19) coronavirus by molecular docking. Int. J. Antimicrob. Agents 56 (2), 106012. doi:10.1016/j.ijantimicag.2020.106012
Yuan, P., Sun, X., Liu, X., Hutterer, G., Pummer, K., Hager, B., et al. (2021). Kaempferol alleviates calcium oxalate crystal-induced renal injury and crystal deposition via regulation of the AR/NOX2 signaling pathway. Phytomedicine 86, 153555. doi:10.1016/j.phymed.2021.153555
Zhang, X., Ao, Z., Bello, A., Ran, X., Liu, S., Wigle, J., et al. (2016). Characterization of the inhibitory effect of an extract of Prunella vulgaris on Ebola virus glycoprotein (GP)-mediated virus entry and infection. Antivir. Res. 127, 20–31. doi:10.1016/j.antiviral.2016.01.001
Keywords: COVID-19, acute kidney injury, cytokine storm, network pharmacology, molecular docking
Citation: Yang X-L, Wang C-X, Wang J-X, Wu S-M, Yong Q, Li K and Yang J-R (2023) In silico evidence implicating novel mechanisms of Prunella vulgaris L. as a potential botanical drug against COVID-19-associated acute kidney injury. Front. Pharmacol. 14:1188086. doi: 10.3389/fphar.2023.1188086
Received: 16 March 2023; Accepted: 09 May 2023;
Published: 18 May 2023.
Edited by:
Xuezhong Gong, Shanghai Municipal Hospital of Traditional Chinese Medicine, ChinaReviewed by:
Lu Zhang, First Affiliated Hospital of Xiamen University, ChinaQihe Xu, King’s College London, United Kingdom
Copyright © 2023 Yang, Wang, Wang, Wu, Yong, Li and Yang. This is an open-access article distributed under the terms of the Creative Commons Attribution License (CC BY). The use, distribution or reproduction in other forums is permitted, provided the original author(s) and the copyright owner(s) are credited and that the original publication in this journal is cited, in accordance with accepted academic practice. No use, distribution or reproduction is permitted which does not comply with these terms.
*Correspondence: Ke Li, ke.li@mail.xjtu.edu.cn; Ju-Rong Yang, yjr923@163.com
†These authors have contributed equally to this work and share first authorship
‡These authors share senior authorship