- 1Department of Pharmacy, The People’s Hospital of Jianyang City, Chengdu, China
- 2Department of Pharmacy, Sichuan Academy of Medical Sciences and Sichuan Provincial People’s Hospital, School of Medicine, University of Electronic Science and Technology of China, Chengdu, China
- 3Department of Infection, Ziyang Central Hospital, Ziyang, China
Objective: Analyze the operation mode of the prescription pre-audit intelligent decision system in a county-level hospital, evaluate its intervention effects on outpatient and emergency operations, thus providing references for similar hospitals to carry out pre-audit intelligent decision system and promote rational drug use.
Methods: Utilizing evidence-based approaches, system rule modifications have been refined and synergized with AI-driven decision-making analytics to examine the operational framework of pre-audit prescription decision system. Additionally, retrospectively analyze the types and levels of problems triggered by outpatient and emergency prescriptions from October 2022 to August 2023, as well as the rationality of prescriptions in the system.
Results: According to the clinical operation of the hospital, problems triggered by unreasonable prescriptions have been finely classified into different levels according to the severity of prescription problems. From October 2022 to August 2023, the number of prescriptions triggering issues such as indications, dosage, special populations, compatibility, administration, and contraindications showed a decreasing trend compared with October 2022 before the intervention. For example, the number of prescriptions with unreasonable routes of administration decreased from 1,745 to 20, and the number of contraindicated prescriptions decreased from 1,399 to 16. The prescriptions triggering Level 5 alerts decreased from 5.609% to 1.793% and the prescription compliance rate increased from 92.20% to 95.98%.
Conclusion: The prescription pre-audit intelligent decision system enhances patient safety and promotes rational drug use. However, the system requires fine-tuning and continuous improvement of the system rule library to effectively validate prescriptions and improve prescription accuracy. In the future, integrating big data, artificial intelligence and other technologies for secondary system development will be a model worthy of consideration. In addition, promoting this system to medical federation to establish a regional prescription review model will further promote the high-quality development of pharmaceutical services.
1 Introduction
Medication errors may occur at any stage of patient medication use, such as when a doctor prescribes medication, a pharmacist dispenses medication and other actions by hospital personnel (Pais et al., 2024; Bowdle et al., 2023; Subramanian et al., 2022). Globally, medication errors cause 5%–41.3% of all hospitalizations and 22% of readmissions after discharge (Tariq et al., 2024). The annual cost of treating patients affected by medication errors exceeds $40 billion (Wang et al., 2022). Additionally, a total of 27,460 cases of medication error were collected in the China National Monitoring Network for Clinical Safe Medication in 2023, of which the main people who triggered by physicians (19,655, 71.58%) and pharmacists (5,688, 20.71%) (Zhang et al, 2024a). This greatly impacts patients’ health and hospitals’ reputation.
In most grassroots hospitals in China, patients typically go to the pharmacy to collect medication after the doctor has issued a prescription, and then the pharmacist dispenses the medication after verifying the prescription information. Creating prescriptions is an early step in patients’ medication process. As prescription errors are common and preventable medication errors, pharmacists’ review of prescriptions is crucial for detecting errors and preventing patient adverse effects (Ben Natan et al., 2017; Lee et al., 2022; Bu et al., 2022). However, due to the large number of prescriptions and a shortage of pharmacists in Chinese hospitals, there may be omissions during pharmacist review of prescriptions (Zhao et al., 2021). Additionally, due to differences in the professional abilities of each pharmacist, there is a lack of consistency in prescription review. Currently, the informatization and intelligent construction of hospitals in China are being vigorously promoted (Wang et al., 2024). Against the backdrop of new policies, the development of patient-centered hospital pharmacy requires more refined informatization systems as support, while also placing higher demands on hospital pharmacy services (Young et al., 2023; Allen et al., 2024a). Therefore, embedding intelligent rational drug use software in hospital information systems to intelligently review prescriptions when doctors create prescriptions, which may effectively avoid prescription errors (Westbrook et al., 2022; Pereira et al., 2023).
In recent decades, the gradual advancement of Health Information Systems (HIS) has significantly enhanced patients’ medical experiences and the quality of care (Tamrat et al., 2022; Tummers et al., 2021; Seth et al., 2024). Electronic medical records, electronic prescribing, and artificial intelligence-based decision support systems are pivotal in refining HIS (Nimri et al., 2024). The Prescription Pre-Audit Intelligent Decision System (PPIDS) is a rational medication support tool integrated into our HIS (Wang et al., 2022; Kassem et al., 2024). It is designed to provide intelligent decision support to physicians during medication prescribing, aiming to effectively reduce prescription errors. This system is constructed and continuously updated based on current drug instructions, guidelines, and peer-reviewed research (Wang et al., 2022; Li et al., 2022). Consequently, it assesses drug usage information when physicians issue prescriptions, intervening in inappropriate prescriptions according to varying safety levels (Westbrook et al., 2022). This process helps identify prescription errors early, enhances the consistency of medical practices, and promotes medication safety. Despite the integration of intelligent decision support in hospital systems, there is limited research evaluating its real-world effectiveness in reducing prescription errors at county-level hospitals. This study aims to bridge that gap by assessing the impact of PPIDS in clinical practice.
2 Methodology
2.1 The prescription pre-audit intelligent decision system
This study was conducted at the People’s Hospital of Jianyang City, which is a comprehensive tertiary grassroot hospital in Sichuan, China (Figure 1). The PPIDS was designed by Hangzhou Yiyao Co., Ltd. The system was embedded into the HIS, followed by system debugging and integration. Subsequently, the system underwent silent operation without prompts or interventions to collect debugging data. The collected trigger rule information was organized, and pharmacists retrieved relevant drug instructions, guidelines, and online databases (such as Micromedex, Uptodate, Drugs) based on the triggered drug rule information. An intelligent prescription knowledge database was constructed and embedded into the PPIDS to provide drug rule suggestions (i.e., different levels). Finally, after communication and discussion with doctors, the system began to operate (Supplementary Figures 1, 2).
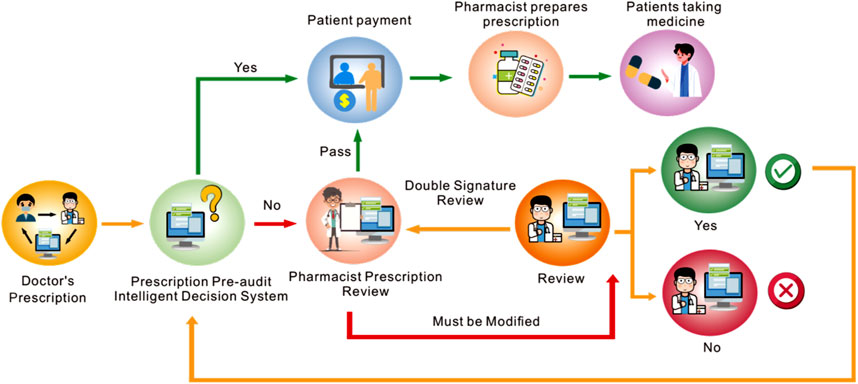
Figure 1. Operation of the Pre-audit Intelligent Decision System. In essence, all prescriptions issued to patients following a medical consultation must be reviewed by the Pre-audit Intelligent Decision System. If a prescription is approved, the patient can proceed with payment and receive the medication. If a prescription is not approved, it must be either double-signed or modified; otherwise, it cannot be processed.
2.2 Warning levels of system
All prescriptions are issued by physicians and stored in the electronic medical record system. Prescription orders are automatically sent to the prescription PPIDS for review. Drug alert messages are categorized into levels 1–8 based on literature review, consensus guidelines, and clinical discussions. Briefly, levels 1–4 are advisory messages (not shown to physicians, e.g., downgraded from higher alert levels after clinical discussion and evidence collection). Level 5 warnings are advisory messages shown to physicians (indicating potential medication error risks, e.g., “unclear,” “use with caution,” “weigh risks and benefits”). Level 6 warnings require dual signing by physicians (physicians must confirm before saving, e.g., severe hepatic or renal impairment requiring clinical assessment before use). Level 7 warnings require modification by physicians (system identifies prescription errors that need correction before saving, e.g., inappropriate dosage for traditional Chinese medicine, inappropriate administration frequency). Level 8 warnings prevent physicians from submitting prescription information (clear errors preventing prescription issuance, e.g., exceeding maximum dosage, obvious route of administration errors) (Figure 2). It is of significance to note that the false positive alerts caused by problematic rules must be treated with prudence. After obtaining rigorous evidence-based proof, it is essential to modify the rules to ensure their authority and effectiveness (Supplementary Figure 2). On the premise of guaranteeing the rational use of medications by patients, this also serves to prevent the addition of unnecessary prompts in the clinical setting.
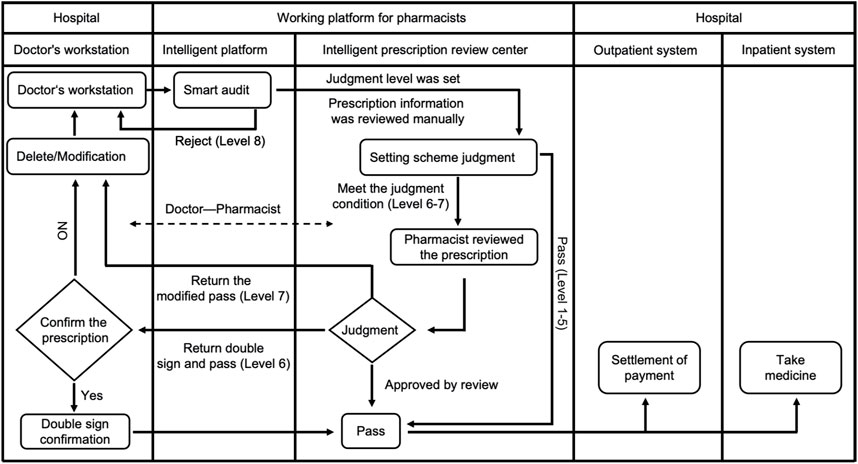
Figure 2. Flow chart for prescription review. During the prescription issuing process, the Prescription Pre-audit Intelligent Decision System automatically identifies the medication information prescribed by the doctor, evaluates its appropriateness, and conducts a review. Pre-audit of medical orders mainly includes steps: processing prescriptions based on different warning levels; when Levels 6 or 7 warnings appear, pharmacists conduct manual reviews of prescriptions, while prescriptions with Level 8 warnings cannot be issued. In summary, if there are no errors in the medical orders or prescriptions issued by the doctor, the medication will be successfully packaged and dispensed. Otherwise, it will not pass.
2.3 Study design and data collection
This study is a retrospective study that collected outpatient and emergency prescriptions at levels 5–8 were collected from Jianyang People’s Hospital from October 2022 to August 2023. Data were collected and exported using the Hangzhou Yiyao Rational Drug Use Management System (Hangzhou Yiyao Co., Ltd.) for analysis and processing. Pharmacists conducted to double check on the safety of medications, and then responded according to different warning levels (Wang et al., 2022). This study is mainly a retrospective descriptive analysis, focusing on orders that evoke alerts, with detailed information that was collected and entered into Microsoft Excel 2024 and GraphPad 9.0 software for further analyses. The trend of data changes was compared with the initial observation data (October 2022), and the pre-intervention data (October 2022 to March 2023) and post-intervention data (April 2023 to August 2023) were statistically analyzed before and after. The unpaired sample t-test was used to compare the means of the two groups, and the Wilcoxon test of paired samples was used for non-normally distributed data. A two-sided P < 0.05 was considered statistically significant.
2.4 Ethics
This study protocol was approved by the Ethics Committee of Jianyang People’s Hospital (ethical approval number: JYL2024004Z). As it is a retrospective study, written form informed consent was not required.
3 Results
3.1 Analysis of prescription problems
Collect data of rule-triggered type after the system goes live for analysis (Supplementary Table 1). From October 2022 to August 2023, there has been a general decrease in problematic prescriptions in terms of indications, dosage, special populations in outpatient and emergency prescriptions (Supplementary Figures 3A, B). For example, in October 2022, the number of prescriptions with dosage issues was 3,588, accounting for 2.912% of that month. By August 2023, the number decreased to 83, representing 0.071% of that month, a decrease of 2.841%. It is worth noting that there have been significant changes in the administration route and contraindications during this period (Figure 3A), decreasing from 1,745 to 20, and from 1,399 to 16, respectively. This change may be attributed to the initial in the system rules, which have been subsequently improved and modified in the rule library. Moreover, the frequency of alerts for most drugs exhibited a decline from March to August 2024. This phenomenon may be attributable to the refinement of our algorithm system, which consistently prompted physicians to improved prescribing behavior (Supplementary Table 2). Therefore, grounded in evidence-based medicine, the continuous enhancement of the system rule library in integration with clinical practice assumes a pivotal role in guaranteeing the rational utilization of medications and the seamless operation of the system (Ibáñez-Garcia et al., 2019; Tantray et al., 2024).
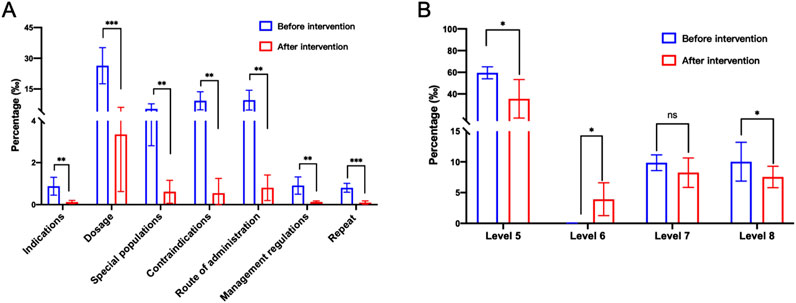
Figure 3. Pre-audit Intelligent Decision System intervention alert types and levels. (A) Pre-audit Intelligent Decision System intervention alert types. (B) Pre audit Intelligent Decision System intervention alert level 5 -8. Statistical significance was calculated via t-test, *p < 0.05; **p < 0.01; ***p < 0.001.
3.2 Level analysis of triggering questions
By collecting data through the system, different alert levels are classified (Supplementary Table 3). In October 2022, the trigger rate of level 5 warning in the system rule base is 5.609%. As the system rule library continues to be improved, the rules are gradually classified into different levels to achieve different warning effects. As of August 2023, the triggering rate of level 5 alerts has decreased to 1.793%, while level 6 alerts have significantly increased (Figure 3B; Supplementary Figure 3C). This suggests that through continuous improvement of the system and fine-grained classification of levels, using different alert levels not only increases doctors’ attention to prescription standards but also avoids ineffective pop-up alerts, thereby facilitating rational medication use for patients.
3.3 Analysis of prescription rationality
The rationality of prescriptions is significant importance in safeguarding patient safety, enhancing treatment efficacy and preventing drug abuse. The warning levels in this study are classified into levels 1–8. Levels 1–4 do not indicate any warning, and levels 5 and above may involve potential medication errors or even worse. Therefore, we define levels 5–8 as inappropriate medication prescriptions and further infer rational medication prescriptions. By analyzing the system data exported from PPIDS from October 2022 to August 2023, the results show a gradual increase in the prescription rationality rate (Table 1; Figure 4; Supplementary Figure 3D). Noteworthy, the system determined the prescription rationality rate to be 91.32% in January 2023. This is due to the tight supply of drugs and the severe epidemic situation of COVID-19 in China in early 2023, which led to the restriction of related drugs in short supply, resulting in an increase in alerts triggered by doctors and a significant decline in the rationality of prescriptions. Subsequently, with rapid adjustments and the introduction of relevant management policies, the prescription rationality rate gradually improved. Additionally, the system’s rationality rate may be subject to false negatives and false positives. Therefore, further analysis of the rationality of prescriptions prompted by the system needs to be conducted in conjunction with clinical practical issues and cannot be generalized.
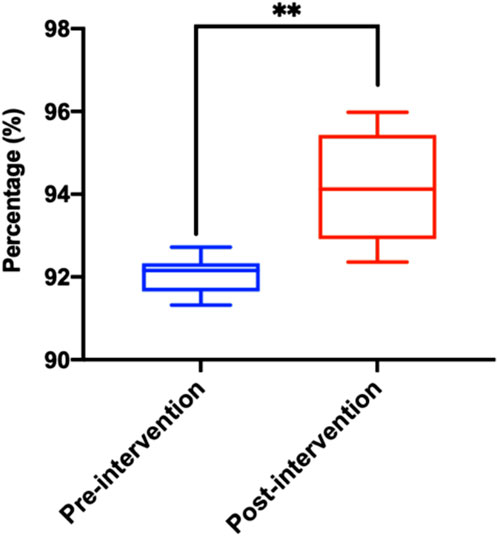
Figure 4. Distribution of reasonable prescription rate after Pre-audit Intelligent Decision System intervention. Statistical significance was calculated via t-test, *p < 0.05; **p < 0.01; ***p < 0.001.
4 Discussion
Our study indicates that PIDDS can providing real-time prescription feedback to physicians through intelligent review can reduce medication errors. This integration offers substantial benefits for enhancing diagnostic and treatment plans. For instance, the system can mitigate medication errors by alerting physicians with warning information about drugs (Supplementary Table 2). Among these alerts, dosage-related warnings are a frequent cause of medication errors, consistent with findings from a study conducted in Denmark (Rishoej et al., 2017). Furthermore, configuring abnormal dosage alerts within the system is crucial for preventing medication errors due to physician oversight (Wu et al., 2020).
It is noteworthy that incorrect routes of administration were the second type of error in this study, accounting for 1.416% of all prescription that month. Variations in drug preparation processes can lead to different routes of administration for the same medication (Liu et al., 2023). Errors in the route of administration can have serious adverse effects on patient safety (Gualano et al., 2021), such as prescribing “oral” medications as “nebulized” or “topical” medications as “oral,” among other issues. In this study, 1,745 alerts for “route of administration” errors were triggered in October 2022; however, this number decreased to 20 by August 2023 (Supplementary Table 1). This reduction may be attributed to initial discrepancies between the HIS’s “routes of administration” and at the time of system installation. Additionally, as medications are continually updated, ongoing system optimization is necessary.
In this study, medication errors are more prevalent in the hospital’s convenience outpatient clinics. In August 2023, the highest number of rule-triggered alerts of severity level 7 or above were recorded, accounting for approximately 4.82% of the total alerts (Supplementary Table 4). This trend may be attributed to grassroot hospitals offering services in non-specialized departments to accommodate patients’ needs for convenient access to care. Consequently, prescribing physicians, who may lack familiarity with specialized medications, are more likely to trigger medication warnings. Nevertheless, this observation underscores the critical role of the warning system in reducing the likelihood of medication errors. Notably, in cases involving critical care patients with clinical pharmacist involvement, only 6 alerts of level 7 or above were triggered, representing approximately 0.13% of the total (Supplementary Table 4). This contrasts with findings from Alghamdi et al. (2019). And underscores the significant role of clinical pharmacists in clinical practice and their contribution to assisting physicians in managing patient care.
The “Management Regulations” governing the review system are commendable, which are not only derived from hospital-specific policies but also from government guidelines. By embedding PPIDS in a structured manner, they play an important role in hospital drug and prescription management. They standardize physician prescribing practices and enhance the rationality of prescriptions. Furthermore, these regulations facilitate the dissemination of pertinent policies and guidelines to frontline clinicians, thereby aiding the execution of related tasks. Additionally, the “Management Regulations” provisions ensure drug supply under exceptional circumstances. For instance, during the COVID-19 pandemic, when drug production was insufficient to meet demand, regulations such as restricting acetaminophen prescriptions to 10 tablets per person per prescription were implemented to better ensure patient access to essential medications.
In recent decades, remarkable technological progressions have opened novel avenues for safeguarding patient safety (Li et al., 2024; Kurniawan et al., 2024). The integration of technology to digitize healthcare processes the potential to enhance the standardization and efficiency of clinical work processes, which mitigate medication errors across all medical institutions. Specifically, the shift from paper-based prescriptions to commercial computerized provider order entry (CPOE) systems has been demonstrated to reduce MEs and hospital mortality rates (Holmgren et al., 2023). The utilization of effective CPOE and clinical decision support system (CDSS) can avert or decrease medication errors (Ciapponi et al., 2021). One study showed that well-implemented CPOE systems led to a significant reduction in both serious and common prescription-related and procedural errors (Sheikh et al., 2022). However, systems with suboptimal designs are ill-suited to existing workflows, which may engender user dissatisfaction and heighten the likelihood of errors (Bocknek et al., 2022). Consequently, it is imperative to continuously optimize the rules to align with the existing workflows.
With the emergence of artificial intelligence systems, integrating PPIDS with intelligent platforms plays an important role in facilitating prescription review in clinical practice (Toffaha et al., 2023; Zhang et al, 2024b; Mohsen et al., 2023). Currently, the updating of the review system’s rule database often requires extensive evidence-based collection and processing (Grammatikopoulou et al., 2024a), followed by discussions before further modifications can be made (Lester et al., 2021). This not only increases the workload of pharmacists and physicians, but also makes problem resolution more cumbersome (Grammatikopoulou et al., 2024b). Therefore, the application of machine learning and big data analysis processing systems becomes particularly important. Such as building interconnected data processing centers across multiple databases, machines automatically upgrade the system based on new instructions, guidelines, and other databases to achieve precise prompts. Additionally, the establishment of intelligent review centers is crucial for prescription review in grassroots hospitals and ensuring medication safety (Rojas Garcia and Antonanzas Villar et al., 2021).
The effective operation of the review system relies heavily on the refinement and optimization of the core rule database (Hegde et al., 2022). As AI-based systems continue to evolve, special medications require specific management settings to enhance patient medication safety (Allen et al., 2024b). Furthermore, management settings for off-label drug use, prescription rights, and emergency drug supply management are beneficial for fine-tuning clinical decision-making. Strengthening communication with clinical staff, providing continuous personnel training, and building an evidence-based database can promote to constructing a refined drug rule database.
Notably, the PPIDS not only efficiently enhances the efficiency of pharmacists’ prescription review via prior intervention, but also furnishes a basis for judgment in clinical drug management decision-making. Nevertheless, it still has deficiencies in data updating, refinement, and coverage. False alerts may engender inconvenience and necessitate continuous attention. Furthermore, the system regulates pharmacists’ prescription behavior through prompt alerts. The incessant pop-up box alerts may require optimization in the future, as they might trigger pharmacists’ resistance to the system. The principal limitation of this study was its single-center nature. This descriptive retrospective study was carried out in only one setting and may not be generalizable to large-scale healthcare settings in China. Another limitation is the dearth of in-depth analysis of the causes of these MEs and the absence of rigorous statistical analysis.
5 Conclusion
In summary, under the supervision of trained pharmacists, PPIDS effectively verifies prescriptions, thereby improving prescription accuracy and medication safety. The application of the PPIDS enables hospital pharmacists to conduct prescription reviews more efficiently and accurately. This is of substantial significance for the future development and construction of intelligent hospitals. Moreover, in the future, greater emphasis should be placed on the AI integration. The utilization of big-data models to conduct in-depth research on hospital prescription information will encounter both opportunities and challenges in the process of providing more intelligent and individualized solutions.
Data availability statement
The original contributions presented in the study are included in the article/Supplementary Material, further inquiries can be directed to the corresponding author.
Ethics statement
The studies involving humans were approved by the Ethics Committee of Jianyang People’s Hospital. The studies were conducted in accordance with the local legislation and institutional requirements. Written informed consent for participation was not required from the participants or the participants’ legal guardians/next of kin in accordance with the national legislation and institutional requirements.
Author contributions
X-HY: Conceptualization, Data curation, Formal Analysis, Investigation, Methodology, Writing – original draft, Writing – review and editing. LY: Conceptualization, Data curation, Investigation, Methodology, Software, Writing – original draft, Writing – review and editing. J-JZ: Data curation, Investigation, Methodology, Software, Writing – original draft, Writing – review and editing. H-ML: Conceptualization, Data curation, Formal Analysis, Writing – review and editing. DW: Conceptualization, Data curation, Formal Analysis, Investigation, Software, Writing – review and editing. XT: Conceptualization, Data curation, Formal Analysis, Investigation, Methodology, Software, Writing – review and editing. G-FZ: Conceptualization, Data curation, Formal Analysis, Funding acquisition, Investigation, Methodology, Project administration, Resources, Software, Writing – original draft, Writing – review and editing.
Funding
The author(s) declare that financial support was received for the research and/or publication of this article. This study was supported by the Research Project on Pharmaceutical Sciences by the 5th Chengdu Pharmaceutical Commission (cdyxky5036); Research Project of Jianyang People’s Hospital (JY202349); Sichuan Pharmaceutical Association New Quality Pharmacy Start-up Plan Youth Project (scyxh20240709); Research and Development Project of Chengdu Science and Technology Bureau (2024-YF05-02542-SN).
Acknowledgments
The authors thank all the clinical pharmacists at the Jianyang People’s Hospital for prescription checking.
Conflict of interest
The authors declare that the research was conducted in the absence of any commercial or financial relationships that could be construed as a potential conflict of interest.
Publisher’s note
All claims expressed in this article are solely those of the authors and do not necessarily represent those of their affiliated organizations, or those of the publisher, the editors and the reviewers. Any product that may be evaluated in this article, or claim that may be made by its manufacturer, is not guaranteed or endorsed by the publisher.
Supplementary material
The Supplementary Material for this article can be found online at: https://www.frontiersin.org/articles/10.3389/fphar.2025.1491810/full#supplementary-material
References
Alghamdi, A. A., Keers, R. N., Sutherland, A., and Ashcroft, D. M. (2019). Prevalence and nature of medication errors and preventable adverse drug events in paediatric and neonatal intensive care settings: a systematic review. Drug Saf. 42 (12), 1423–1436. doi:10.1007/s40264-019-00856-9
Allen, L. A., Thompson, J. S., and Stehlik, J. (2024a). STRONG-HF evidence for proactive, patient-centered prescribing. JAMA Cardiol. 9 (2), 103–104. doi:10.1001/jamacardio.2023.4565
Allen, L. D., Pollini, R. A., Vaglienti, R., and Powell, D. (2024b). Opioid prescribing patterns after imposition of setting-specific limits on prescription duration. JAMA Health Forum 5 (1), e234731. doi:10.1001/jamahealthforum.2023.4731
Ben Natan, M., Sharon, I., Mahajna, M., and Mahajna, S. (2017). Factors affecting nursing students' intention to report medication errors: an application of the theory of planned behavior. Nurse Educ. Today 58, 38–42. doi:10.1016/j.nedt.2017.07.017
Bocknek, L., Kim, T., Spaar, P., Russell, J., Busog, D. N., Howe, J., et al. (2022). Duplicate medication order errors: safety gaps and recommendations for improvement. Patient Saf. 4 (3), 39–47. doi:10.33940/data/2022.9.6
Bowdle, T. A., Jelacic, S., Webster, C. S., and Merry, A. F. (2023). Take action now to prevent medication errors: lessons from a fatal error involving an automated dispensing cabinet. Br. J. Anaesth. 130 (1), 14–16. doi:10.1016/j.bja.2022.09.017
Bu, F., Sun, H., Li, L., Tang, F., Zhang, X., Yan, J., et al. (2022). Artificial intelligence-based internet hospital pharmacy services in China: perspective based on a case study. Front. Pharmacol. 13, 1027808. doi:10.3389/fphar.2022.1027808
Ciapponi, A., Fernandez, S. E., Seijo, M., Rodriguez, M. B., Vietto, V., Garcia-Perdomo, H. A., et al. (2021). Reducing medication errors for adults in hospital settings. Cochrane Database Syst. Rev. 11 (11), CD009985. doi:10.1002/14651858.CD009985.pub2
Grammatikopoulou, M., Lazarou, I., Giannios, G., Kakalou, C. A., Zachariadou, M., Zande, M., et al. (2024a). Electronic prescription systems in Greece: a large-scale survey of healthcare professionals' perceptions. Arch. Public Health 82 (1), 68. doi:10.1186/s13690-024-01304-6
Grammatikopoulou, M., Zachariadou, M., Zande, M., Giannios, G., Chytas, A., Karanikas, H., et al. (2024b). Evaluation of an electronic prescription platform: clinicians' feedback on three distinct services aiming to facilitate clinical decision and safer e-prescription. Res. Soc. Adm. Pharm. 20 (7), 640–647. doi:10.1016/j.sapharm.2024.04.004
Gualano, M. R., Lo Moro, G., Voglino, G., Catozzi, D., Bert, F., and Siliquini, R. (2021). Wrong administration route of medications in the domestic setting: a review of an underestimated public health topic. Expert Opin. Pharmacother. 22 (8), 1051–1059. doi:10.1080/14656566.2021.1876027
Hegde, H., Glurich, I., Panny, A., Vedre, J. G., VanWormer, J. J., Berg, R., et al. (2022). Identifying pneumonia subtypes from electronic health records using rule-based algorithms. Methods Inf. Med. 61 (1-02), 29–37. doi:10.1055/a-1801-2718
Holmgren, A. J., McBride, S., Gale, B., and Mossburg, S. (2023). Technology as a tool for improving patient safety. United States: Department of Health & Human Services. Available online at: https://psnet.ahrq.gov/perspective/technology-tool-improving-patient-safety.
Ibáñez-Garcia, S., Rodriguez-Gonzalez, C., Escudero-Vilaplana, V., Martin-Barbero, M. L., Marzal-Alfaro, B., De la Rosa-Triviño, J. L., et al. (2019). Development and evaluation of a clinical decision support system to improve medication safety. Appl Clin Inform. 10 (3), 513–520. doi:10.1055/s-0039-1693426
Kassem, K., Sperti, M., Cavallo, A., Vergani, A. M., Fassino, D., Moz, M., et al. (2024). An innovative artificial intelligence-based method to compress complex models into explainable, model-agnostic and reduced decision support systems with application to healthcare (NEAR). Artif. Intell. Med. 151, 102841. doi:10.1016/j.artmed.2024.102841
Kurniawan, M. H., Handiyani, H., Nuraini, T., Hariyati, R. T. S., and Sutrisno, S. (2024). A systematic review of artificial intelligence-powered (AI-powered) chatbot intervention for managing chronic illness. Ann. Med. 56 (1), 2302980. doi:10.1080/07853890.2024.2302980
Lee, S., Shin, J., Kim, H. S., Lee, M. J., Yoon, J. M., Lee, S., et al. (2022). Hybrid method incorporating a rule-based approach and deep learning for prescription error prediction. Drug Saf. 45 (1), 27–35. doi:10.1007/s40264-021-01123-6
Lester, C. A., Ding, Y., Li, J., Jiang, Y., Rowell, B., and Vydiswaran, V. G. V. (2021). Human versus machine editing of electronic prescription directions. J. Am. Pharm. Assoc. 61 (4), 484–491.e1. doi:10.1016/j.japh.2021.02.006
Li, J. K., Tang, T., Zong, H., Wu, E. M., Zhao, J., Wu, R. R., et al. (2024). Intelligent medicine in focus: the 5 stages of evolution in robot-assisted surgery for prostate cancer in the past 20 years and future implications. Mil. Med. Res. 11 (1), 58. doi:10.1186/s40779-024-00566-z
Li, S., Huang, L., Zeng, L., Yu, D., Jia, Z. J., Cheng, G., et al. (2022). A tool for screening potentially inappropriate prescribing in Chinese children. Front. Pharmacol. 13, 1019795. doi:10.3389/fphar.2022.1019795
Liu, H., Li, W., Zhang, W., Sun, S., and Chen, C. (2023). Levothyroxine: conventional and novel drug delivery formulations. Endocr. Rev. 44 (3), 393–416. doi:10.1210/endrev/bnac030
Mohsen, F., Al-Absi, H. R. H., Yousri, N. A., El Hajj, N., and Shah, Z. (2023). A scoping review of artificial intelligence-based methods for diabetes risk prediction. NPJ Digit. Med. 6 (1), 197. doi:10.1038/s41746-023-00933-5
Nimri, R., Phillip, M., Clements, M. A., and Kovatchev, B. (2024). Closed-loop control, artificial intelligence-based decision-support systems, and data science. Diabetes Technol. Ther. 26 (S1), S68–S89. doi:10.1089/dia.2024.2505
Pais, C., Liu, J., Voigt, R., Gupta, V., Wade, E., and Bayati, M. (2024). Large language models for preventing medication direction errors in online pharmacies. Nat. Med. 30 (6), 1574–1582. doi:10.1038/s41591-024-02933-8
Pereira, J., Antunes, N., Rosa, J., Ferreira, J. C., Mogo, S., and Pereira, M. (2023). Intelligent clinical decision support system for managing COPD patients. J. Pers. Med. 13 (9), 1359. doi:10.3390/jpm13091359
Rishoej, R. M., Almarsdottir, A. B., Christesen, H. T., Hallas, J., and Kjeldsen, L. J. (2017). Medication errors in pediatric inpatients: a study based on a national mandatory reporting system. Eur. J. Pediatr. 176 (12), 1697–1705. doi:10.1007/s00431-017-3023-8
Rojas Garcia, P., and Antonanzas Villar, F. (2021). Assessment of the quality of antibiotics prescription in a regional health system. Rev. Clin. Esp. 221 (9), 497–508. doi:10.1016/j.rceng.2020.04.013
Seth, M., Jalo, H., Lee, E., Bakidou, A., Medin, O., Bjorner, U., et al. (2024). Reviewing challenges in specifying interoperability requirement in procurement of health information systems. Stud. Health Technol. Inf. 310, 8–12. doi:10.3233/SHTI230917
Sheikh, A., Coleman, J., Chuter, A., Williams, R., Lilford, R., Slee, A., et al. (2022). Electronic prescribing systems in hospitals to improve medication safety: a multimethods research programme. Programme Grants Appl. Res. 10 (7), 1–164. doi:10.3310/ksrs2009
Subramanian, S., Biswas, A., Alves, C., Sudhakar, S. V., Shekdar, K. V., Krishnan, P., et al. (2022). ACTA2-Related dysgyria: an under-recognized malformation of cortical development. AJNR Am. J. Neuroradiol. 43 (1), 146–150. doi:10.3174/ajnr.A7364
Tantray, J., Patel, A., Wani, S. N., Kosey, S., and Prajapati, B. G. (2024). Prescription precision: a comprehensive review of intelligent prescription systems. Curr. Pharm. Des. 30(34), 2671–2684. doi:10.2174/0113816128321623240719104337
Tamrat, T., Chandir, S., Alland, K., Pedrana, A., Shah, M. T., Footitt, C., et al. (2022). Digitalization of routine health information systems: Bangladesh, Indonesia, Pakistan. Bull. World Health Organ 100 (10), 590–600. doi:10.2471/BLT.22.287816
Tariq, R. A., Vashisht, R., Sinha, A., and Scherbak, Y. (2024) Medication Dispensing Errors and Prevention. In: StatPearls. https://www.ncbi.nlm.nih.gov/books/NBK519065/
Toffaha, K. M., Simsekler, M. C. E., and Omar, M. A. (2023). Leveraging artificial intelligence and decision support systems in hospital-acquired pressure injuries prediction: a comprehensive review. Artif. Intell. Med. 141, 102560. doi:10.1016/j.artmed.2023.102560
Tummers, J., Tekinerdogan, B., Tobi, H., Catal, C., and Schalk, B. (2021). Obstacles and features of health information systems: a systematic literature review. Comput. Biol. Med. 137, 104785. doi:10.1016/j.compbiomed.2021.104785
Wang, G., Zheng, F., Zhang, G., Huang, Y., Ye, Q., Zhang, X., et al. (2022). Intercepting medication errors in pediatric in-patients using a prescription pre-audit intelligent decision system: a single-center study. Paediatr. Drugs 24 (5), 555–562. doi:10.1007/s40272-022-00521-2
Wang, Q., Ma, Y. J., Mao, J., Song, J. Y., Xiao, M. Z., Zhao, Q. H., et al. (2024). Driving the implementation of hospital examination reservation system through hospital management. BMC Health Serv. Res. 24 (1), 44. doi:10.1186/s12913-023-10467-x
Westbrook, J. I., Li, L., Raban, M. Z., Mumford, V., Badgery-Parker, T., Gates, P., et al. (2022). Short- and long-term effects of an electronic medication management system on paediatric prescribing errors. NPJ Digit. Med. 5 (1), 179. doi:10.1038/s41746-022-00739-x
Wu, D., Xu, Y., Zhang, X. H., Zheng, F., Chen, H., and Li, Z. P. (2020). Construction of pediatric prescription knowledge database facilitates prescription pre-audit intelligent decision. Chin. Pharm. J. 55 (12), 1046–1050. doi:10.2146/ajhp170346
Young, H. N., Pathan, F. S., Hudson, S., Mott, D., Smith, P. D., and Schellhase, K. G. (2023). Impact of patient-centered prescription medication labels on adherence in community pharmacy. J. Am. Pharm. Assoc. (2003) 63 (3), 785–792. doi:10.1016/j.japh.2023.01.004
Zhang, Q. X., Li, S. Y., Bai, X. R., Wang, Z. M., Yan, S. Y., Wang, Y. Q., et al. (2024a). Annual report of national monitoring Network for clinical Safe medication. Adverse Drug React. J. 26 (7), 390–398. doi:10.3760/cma.j.cn114015-20240617-00454
Zhang, Y., Deng, Z., Xu, X., Feng, Y., and Junliang, S. (2024b). Application of artificial intelligence in drug-drug interactions prediction: a review. J. Chem. Inf. Model. 64 (7), 2158–2173. doi:10.1021/acs.jcim.3c00582
Keywords: medication errors, pre-prescription review, prescription pre-audit intelligent decision system, evidence-based practice, rational drug use
Citation: Yue X-H, Yang L, Zhong J-J, Liu H-M, Wang D, Tao X and Zheng G-F (2025) Optimization and impact of an evidence-based pre-audit prescription decision system in primary healthcare settings. Front. Pharmacol. 16:1491810. doi: 10.3389/fphar.2025.1491810
Received: 05 September 2024; Accepted: 31 March 2025;
Published: 14 April 2025.
Edited by:
Saad Saeed Alqahtani, King Khalid University, Saudi ArabiaReviewed by:
Seerat Shahzad, Lahore University of Biological and Applied Sciences, PakistanMuhammad Iqbal, AIMST University, Malaysia
Rabeel Khan, Lahore University of Biological and Applied Sciences, Pakistan
Copyright © 2025 Yue, Yang, Zhong, Liu, Wang, Tao and Zheng. This is an open-access article distributed under the terms of the Creative Commons Attribution License (CC BY). The use, distribution or reproduction in other forums is permitted, provided the original author(s) and the copyright owner(s) are credited and that the original publication in this journal is cited, in accordance with accepted academic practice. No use, distribution or reproduction is permitted which does not comply with these terms.
*Correspondence: Gao-Feng Zheng, OTc4OTQ3MEBxcS5jb20=