- 1Pharmaceutical Analysis Department, King Abdullah International Medical Research Center (KAIMRC), Riyadh, Saudi Arabia
- 2King Saud Bin Abdulaziz University for Health Sciences (KSAU-HS), Ministry of National Guard Health Affairs (MNGHA), Riyadh, Saudi Arabia
- 3Medical Genomics Research Department, KAIMRC, Riyadh, Saudi Arabia
- 4Department of Pharmacy Practice, College of Pharmacy, Princess Nourah bint Abdulrahman University, Riyadh, Saudi Arabia
- 5Department of Pharmaceutical Sciences, College of Pharmacy, Princess Nourah bint Abdulrahman University, Riyadh, Saudi Arabia
- 6Clinical Pharmacy Department, University of Hail, Hail, Saudi Arabia
- 7Department of Pharmacotherapy and Outcomes Science, School of Pharmacy, Virginia Commonwealth University, Richmond, VA, United States
Background: Pharmacogenomic (PGx) variants can significantly impact drug response, but limited data exists on their prevalence in Middle Eastern populations. This study aimed to investigate the inheritance of certain markers in candidate pharmacogenes among healthy Saudis.
Methods: DNA samples from 95 unrelated healthy Saudi participants were genotyped using the Affymetrix Axiom Precision Medicine Diversity Array. Thirty-eight variants in 15 pharmacogenes were analyzed based on their clinical relevance and lack of previous reporting in Saudi populations.
Results: Twenty-six of the 37 tested markers were undetected in the cohort. The selected variants in six genes [DPYD (rs1801268), CACNA1S (rs772226819), EGFR (rs121434568), RYR1 (rs193922816), CYP2B6 (rs3826711), and MT-RNR1 (rs267606617, rs267606618, rs267606619)] were found to be non-existing among Saudis. In contrast, 11 variants and alleles in nine pharmacogenes were detected at varying frequencies. Notable findings included high frequencies of variants in ATIC [rs4673993, minor allele frequency (MAF) = 0.71)] and SLC19A1 (rs1051266, MAF = 0.48) affecting methotrexate efficacy. Three alleles were identified in CYP3A4, including a common (CYP3A4 rs2242480) and two rare alleles (*3 and *22). Another three markers [rs16969968 in CHRNA5, rs11881222 in IFNL3 (IL28B), and SLCO1B1*14] were found to be highly distributed among the participants (MAF = 0.35, 0.30, and 0.14, respectively). Conversely, three rare markers: CYP2A6*2, NAT2*14, and rs115545701 in CFTR, were identified at low-frequency levels (MAF = 0.021, 0.011, 0.005, respectively). Statistically significant differences in allele frequencies were observed for eight variants between Saudi and African populations, five variants compared to East Asians, and two variants compared to Europeans.
Conclusion: This study provides novel insights into the distribution of clinically relevant PGx variants in the Saudi population. The findings have implications for personalizing treatments for various conditions, including rheumatoid arthritis, cystic fibrosis, and hepatitis C. These data contribute to the development of population-specific PGx testing panels and treatment guidelines.
1 Introduction
An individual’s genetic composition can affect their pharmacological responses, including variations in drug metabolism, therapeutic efficacy, and susceptibility to adverse drug reactions (ADRs) (Pirmohamed, 2023). Different populations can have unique genetic variations that influence drug response (Biswas et al., 2023). Over the past 2 decades, a large number of studies have been published describing the pharmacogenomic (PGx) variants and their allele frequencies among different populations, particularly in European, American, African, and Southeast Asian nations (e.g., Japanese and Chinese). While Europe and the United States have well-established research infrastructures (van der Wouden et al., 2017; McDermott et al., 2022), very limited PGx research has been performed to identify ancestral diversity and differences in variants inheritance across Middle Eastern populations (Jithesh et al., 2022; Abou Tayoun et al., 2021). Understanding the prevalence and distribution of PGx variants among different populations is crucial for precision medicine practice. Thus, testing of these variants can ensure patients receive optimal treatment based on their genetic profile (Sarwar, 2023). Several cost-effectiveness studies have shown that genetic testing can help reduce healthcare expenditure by minimizing the costs related to preventable ADRs and treatment failures (Angamo et al., 2016; Brixner et al., 2016; Deenen et al., 2016).
Few reports have investigated the carriage of common and rare variants in 35 pharmacogenes among the Saudi population (Mizzi et al., 2016; Goljan et al., 2022; Alshabeeb et al., 2022b). The common risk alleles were noticed in several genes encoding cytochrome P450 enzymes such as CYP2D6 (*2, *4,*10, and *41), CYP2C9 (*2 and *3), CYP2C19 (*2 and *17), CYP3A5 (*3), CYP4F2 (*3). Other common mutations in different genes [e.g., ABCG2 (rs2231142), ACE (rs1799752), ADD1 (rs4961), ADRB2 (rs1042713), APOE (rs7412), HLA-A (*31:01), and HLA-C (*04:01)] were also detected. In contrast, multiple rare mutations were reported in various genetic loci, for example, CES1 (rs71647871), CYP2D6 (*5, *6, *17, and *29), CYP3A5*3, DPYD (1236G>A), TPMT (*3A and *3C), NUDT15*3. Additionally, further important PGx markers in genes with known impact on therapeutic outcome have not yet been investigated (Alshabeeb et al., 2022a). This study aimed to investigate the inheritance level of selected variants and star alleles in 15 candidate pharmacogenes for the first time among Saudis. Verification of the mutations in these genes would help in designing a suitable gene panel that outfits the Saudi patients’ need for PGx testing implementation in clinical practice (Alshabeeb et al., 2022b).
2 Materials and methods
The DNA of healthy unrelated Saudi participants (n = 95), who voluntarily provided their samples for research purposes and agreed to sign informed consent, was collected from the Biobank at King Abdullah International Medical Research Center (KAIMRC), Ministry of National Guard health Affairs (MNGHA), Riyadh, Saudi Arabia. The total genomic DNA was extracted from whole blood using Puregene Blood Kits (Qiagen, Hilden, Germany, Catalog Number #158389) according to the supplier’s instructions. An automated DNA extraction system (KingFisher™ magnetic system, Thermo Fisher Scientific, Fresno, CA, United States) was used. A DanoDrop (2000/2000c), Thermo Fisher Scientific, Fresno, CA, United States, was used to measure the absorbance of DNA at 260 nm. Working DNA stocks were aliquoted at a concentration of 50 ng/ul and stored at 4 C. The samples were genotyped using the 10x Affymetrix Axiom™ Precision Medicine Diversity Array (PMDA) Plus Kit designed by Thermo Fisher Scientific, United States, (catalog number 951961). The genetic markers to be tested are illustrated in Table 1 and Table 2 as shown in the latest human reference genome version GRCh38.p14. These include ATIC (rs4673993), CACNA1S (rs772226819), CFTR [11 single-nucleotide polymorphisms (SNPs)], CHRNA5 (rs16969968), CYP2A6 (6 SNPs), CYP2B6 (rs3826711), CYP3A4 (5 SNPs), DPYD (rs1801268), EGFR (rs121434568), IFNL3 (rs11881222), MT-RNR1 (rs267606617, rs267606618, rs267606619), NAT2 (*14, rs1801279 + rs1208), RYR1 (rs193922816), SLC19A1 (rs1051266), and SLCO1B1 [*9 (rs59502379), *14 (rs11045819 + rs2306283)]. The official full names of the selected pharmacogenes, their ontology, biological function, and gene identification (ID) number are described in the Supplementary Table S1. The given names of the genes match the reported names in the National Center for Biotechnology Information (NCBI) database. The selected markers met two criteria: (1) they showed strong associations with drug response outcome according to well-established PGx databases [levels A–B in the Clinical Pharmacogenetics Implementation Consortium (CPIC), 3–4 in the Dutch Pharmacogenetics Working Group (DPWG), or 1–2 levels in the Pharmacogenomics Knowledgebase (PharmGKB), and (2) their frequency distributions were not previously reported among Saudis. SNPs that showed a genotyping call rate <95% or a Hardy-Weinberg equilibrium (HWE) p-value <0.05 were removed from the study. Linkage disequilibrium was assessed using r2 (a squared correlation coefficient). Only markers with r2 ≥ 0.6, reflecting moderate-to-strong allelic correlation, were retained for discussion to prioritize robust associations. Allele frequencies of the identified markers in our Saudi cohort were compared with those of other populations (Africans, East Asians, and Europeans) reported in Ensembl and PharmGKB databases (Harrison et al., 2024; Whirl-Carrillo et al., 2021). Fisher’s exact test was used to determine statistically significant differences in allele frequencies between the tested group and other populations. A haplotype analysis software tool version 1.05, prepared by Eliades N-G. and Eliades D. G., was used to determine haplotype frequency of both NAT2*14 and SLCO1B1*14 (Eliades and Eliades, 2009).
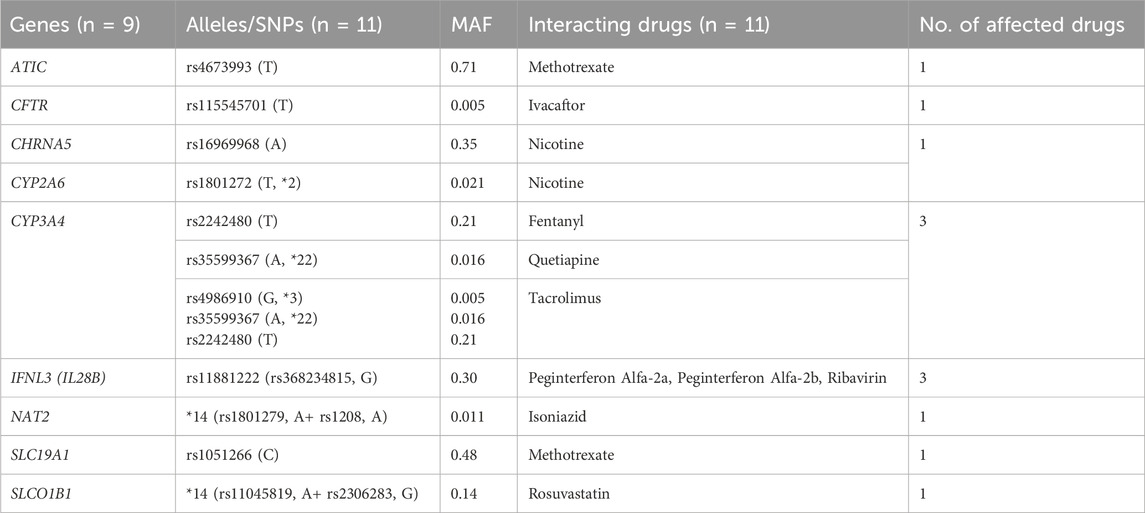
Table 2. Detected variants and alleles in nine pharmacogenes and their associated therapeutic agents. Drug/gen associations were derived from PharmGKB and CPIC databases.
3 Results
The enrolled healthy participants were 60 males and 35 females, aged 30.4 ± 7.4 years versus 32.3 ± 8.9 years (P-value = 0.87). The average sample genotyping call rate was 99.8%, and none of the samples had a call rate <96.5%. As shown in Table 1, the selected variants in six genes were not detected among the screened individuals [DPYD (rs1801268), CACNA1S (rs772226819), EGFR (rs121434568), RYR1 (rs193922816), CYP2B6 (rs3826711), and MT-RNR1 (rs267606617, rs267606618, rs267606619)]. Also, other markers were found to be non-existing in the tested cohort [rs28371759 and rs67666821 in CYP3A4, 10 SNPs (see Table 1) in CFTR, rs59502379in SLCO1B1, and 5 alleles (*20, *24, *27, *28, *38) in CYP2A6]. Of the 37 tested markers, 26 were found to be not inherited by the examined participants. In contrast, 11 variants and alleles in nine pharmacogenes were found to be carried by the investigated Saudi cohort. Eight markers were identified in eight different pharmacogenes: ATIC (rs4673993, minor allele frequency (MAF) = 0.71), CFTR (rs115545701, MAF = 0.005), CHRNA5 (rs16969968, MAF = 0.35), CYP2A6 [*2 (rs1801272), MAF = 0.021], IFNL3 (rs11881222, MAF = 0.30), NAT2 [*14 (rs1801279, A+ rs1208, A), MAF = 0.011], SLC19A1 (rs1051266, MAF = 0.48), and SLCO1B1 [*14, (rs11045819, A+ rs2306283, G), MAF = 0.14)], Table 2. Whereas, three alleles were detected in CYP3A4 [*3 (rs4986910, MAF = 0.005); rs2242480 (MAF = 0.21); *22 (rs35599367, MAF = 0.016)]. Linkage analysis between rs11881222 and another previously well-studied variant (rs12979860) in IFNL3 showed a strong correlation (r2 = 0.97). Significant differences in allele frequencies were detected for eight variants between the Saudi and African populations, five variants compared to East Asians, and two variants compared to Europeans, as shown in Figure 1 and the Supplementary Table S2. No significant differences were noted for the rare markers rs115545701 in CFTR and rs4986910 in CYP3A4. The identified mutations in our study cohort have the potential to influence patients’ responses to 11 different therapeutic agents including antimicrobials [n = 4 agents (peginterferon alfa-2a and alfa-2b, and ribavirin affected by IFNL3, and isoniazid affected by NAT2)], psychiatric [n = 2 agents (quetiapine affected by CYP3A4, and nicotine affected by CHRNA5 and CYP2A6)], immunosuppressants/oncology [n = 2 agents (methotrexate affected by ATIC and SLC19A1, and tacrolimus affected by CYP3A4)], analgesic (fentanyl affected by CYP3A4), cardiovascular (rosuvastatin affected by SLCO1B1), and respiratory (ivacaftor affected by CFTR) medications.
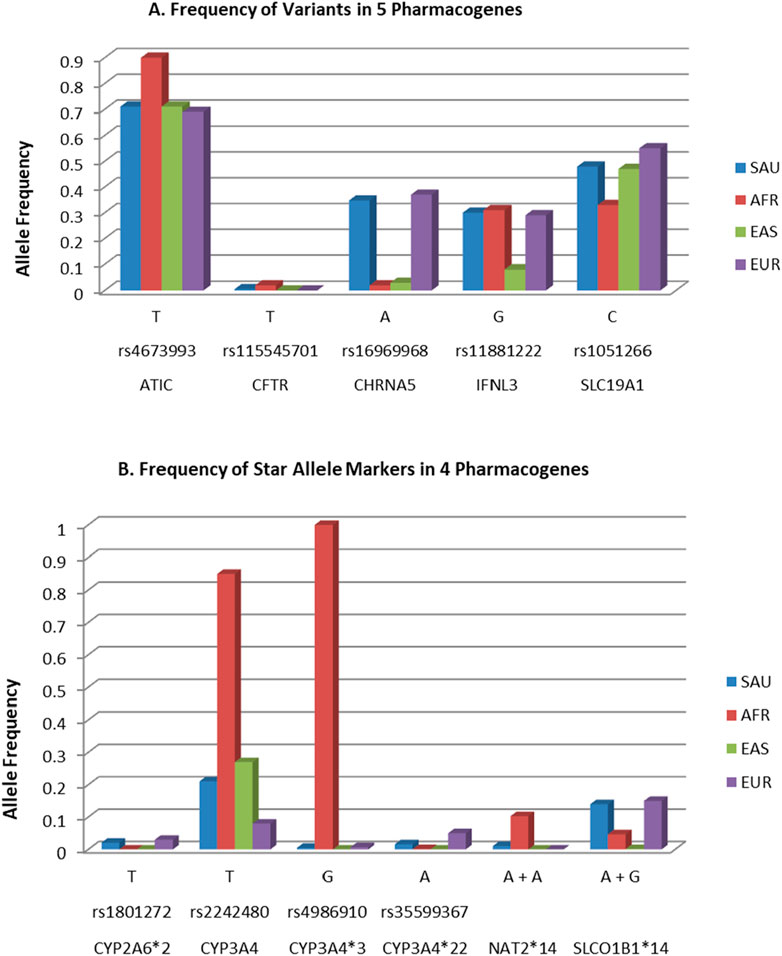
Figure 1. Allele frequency of the detected variants and alleles among Saudis in comparison to previously reported frequencies in Ensembl and PharmGKB databases for African, East Asians, and European populations (Harrison et al., 2024; Whirl-Carrillo et al., 2021). The PGx variants were ploted in (A) while the star allele markers are shown in (B). NAT2*14 = (rs1801279, A+ rs1208, A); SLCO1B1*14 = (rs11045819, A+ rs2306283, G).
4 Discussion
This study investigated several variants in 15 genes known to impact patients’ responses to many drugs based on high-quality evidence derived from multiple studies on various populations (Jithesh et al., 2022; Thorn et al., 2013; Alshabeeb et al., 2022a). The selected variants in this study are prevalent in populations with various ancestral backgrounds. However, due to population-specificity and variability of genetic mutations, we intended to identify whether these variants are also common among Saudis. To the best of our knowledge, the tested variants have not yet been screened in the Saudi population, which makes it difficult to design an appropriate gene panel for PGx testing that suits Saudi society’s needs.
The variants in the DPYD gene can substantially affect a patient’s response to fluoropyrimidine drugs, potentially leading to severe toxic reactions (Larrue et al., 2024; Da Rocha et al., 2021). Patients carrying certain DPYD variants are at an increased risk of experiencing severe toxicity (e.g., gastrointestinal and hematological toxicities) when receiving standard doses of capecitabine or fluorouracil (Wigle et al., 2021). Previously, Goljan et al. reported eight rare variants (rs56038477 was the most frequent mutation, MAF = 0.005) in DPYD among the Saudi population (Goljan et al., 2022). One further DPYD variant (rs1801268) was tested in the current study, and none of the participants were found to carry the variant allele. According to a previous report (Mizzi et al., 2016), DPYD*13 (rs55886062, c.1679T>G) was also found to be non-inherited by Saudis. We also demonstrated a non-existence of two SNPs: rs121434569 in EGFR and rs267606617 in MT-RNR1, in a previous study (Alshabeeb et al., 2022b), and similarly, a SNP (rs121434568) in EGFR and three (rs267606617, rs267606618, rs267606619) in MT-RNR1 could not be detected in the current study. These preliminary findings do not confirm the safety or efficacy of drug substrates of EGFR (e.g., gefitinib) and MT-RNR1 (e.g., aminoglycosides) in Saudi populations as a larger sample size cohort need to be tested to replicate our results.
RYR1 and CACNA1S function together to regulate calcium release from calcium channels in the skeletal muscles (Gonsalves et al., 2019). Selected variants in these genes are associated primarily with malignant hyperthermia and muscle disorders when patients are exposed to anesthetic agents and statins (Alvarellos et al., 2016; Sangkuhl et al., 2020; Beam et al., 2017; Haseeb and Thompson, 2021; Isackson et al., 2018). The current Saudi cohort was genotyped for rs772226819 in CACNA1S and rs193922816 in RYR1, and previously for 20 SNPs in RYR1 (Alshabeeb et al., 2022b). These variants were absent among the tested Saudi cohort. However, this does not necessarily imply reduced susceptibility to anesthesia-related risks associated with CACNA1S and RYR1 genes among Saudi patients. Further study with a larger sample size is needed to confirm these findings. Another gene is CYP2B6, a highly polymorphic cytochrome P450 enzyme that plays a significant role in the drug metabolism of several drugs such as efavirenz and methadone (Desta et al., 2007; Kharasch et al., 2015). A rare variant (rs28399499, MAF = 0.005) in CYP2B6, which is known to impact enzyme activity, was reported previously by our team among healthy Saudis (Alshabeeb et al., 2022a). Thus, we subsequently tested another marker (rs3826711) in this study, but it was found to be absent. Further eleven selected variants in CFTR commonly associated with cystic fibrosis (CF) in Caucasian and African populations, were explored, and a single marker (rs115545701) was found to be carried by only one participant (MAF = 0.005). These data emphasize the extreme rarity of CFTR variants among healthy individuals, but they are common in CF patients. A previous study conducted on 396 CF patients across different regions of Saudi Arabia indicated a carriage of different ten CFTR variants (Banjar et al., 2021). Ivacaftor is a novel drug designed to treat cystic fibrosis, targeting only those who carry disease-causative CFTR mutations (Anderson et al., 2023).
Carriage of ten other mutations in nine genes was confirmed in the tested Saudi participants. The mutations have the potential to affect the response of patients to 10 drugs commonly used in the country’s healthcare system. Two variants in ATIC (rs4673993, T allele) and SLC19A1 (rs1051266, C allele), which impact methotrexate (MTX) efficacy in rheumatoid arthritis (Chen et al., 2017), demonstrated high prevalence among the enrolled Saudis with allele frequencies of 71% and 48%, respectively. These high-frequency distributions within our population necessitate prior genetic testing for the variants preemptively for each patient predicted to be exposed to MTX. Carriage of these variants is associated with decreased treatment efficacy (Lima et al., 2016). Thus, higher doses of MTX might be necessary to achieve satisfactory therapeutic outcome. Clinicians may also consider using alternative or additional disease-modifying antirheumatic drugs to improve treatment results (Qiu et al., 2017). Additionally, two markers in CHRNA5 (rs16969968) and CYP2A6 (rs1801272) genes, associated with increased risk for nicotine dependence in smokers (Lee et al., 2018; Pianezza et al., 1998), were proven to be carried by the Saudis involved in our study. These mutations have significant implications for smoking behavior and cessation. Another mutation in our population is NAT2*14, a slow acetylator SNP which affects isoniazid (antituberculosis) metabolism. This allele was reported with an increased risk of isoniazid-induced liver injury (McDonagh et al., 2014).
In this study, a large portion of the Saudi participants (30%) was found to be carriers of the IFNL3 variant (rs11881222). This mutation is associated with poorer antiviral response in hepatitis C or human immunodeficiency virus (HIV) disease management (Chen et al., 2011). However, we noticed a strong LD (r2 = 0.97) between rs11881222 and a previously reported IFNL SNP (rs12979860) (Al-Qahtani et al., 2015). This linkage was also indicated in East Asians and Europeans (r2 = 0.95 and 0.91, respectively) (Prokunina-Olsson, 2019). CYP3A4 is a crucial enzyme in drug metabolism, responsible for metabolizing approximately 50%–60% of clinically prescribed drugs. Thus, it has significant implications for personalized medicine and drug therapy optimization (Zhang et al., 2024). Two markers in CYP3A4 (−290A < G and 902 T > C, predicted to impact the metabolism of nifedipine and vitamin D, respectively) were previously reported among Saudis (Tayeb et al., 2000; Al-Ashwal et al., 2023). Further, our study identified two rare (*3 and *22) and one common (rs2242480) CYP3A4 alleles. These alleles have the potential to impact tacrolimus metabolism and affect its blood concentrations (Hesselink et al., 2003; Oetting et al., 2018). In addition, increased exposure to quetiapine (an antipsychotic) and fentanyl (an opioid painkiller) was reported in patients carrying CYP3A4*22 and rs2242480, respectively (Mulder et al., 2021; Zhang et al., 2018).
Furthermore, statin-induced myopathy and rhabdomyolysis in association with various markers in SLCO1B1 were extensively studied (Vladutiu and Isackson, 2009). Previous reports confirmed the existence of SLCO1B1*5 among Saudis (Mizzi et al., 2016; Alshabeeb et al., 2023). This study also detected the SLCO1B1*14 genetic allele in 14% of the tested Saudi cohort. This marker is associated with increased OATP1B1 transporter expression and activity causing profound loss of rosuvastatin efficacy (Ho et al., 2006). SLCO1B1*14 is a haplotype mutation comprised of two variants: rs11045819 and rs2306283 variants. The variant rs11045819 was independently found to be associated with decreased exposure to simvastatin and rifampin in single reports that need further replication (Mykkänen et al., 2022; Kwara et al., 2014).
These data shed light on the missing heritability related to PGx markers across our underrepresented Saudi population, though further studies are needed to screen larger sample size. Identifying the level of distribution of genetic factors that influence drug safety and efficacy can lead to more informed treatment decisions (Chenchula et al., 2024). The distinct genetic makeup of the Saudi population, shaped by unique geographic and demographic factors (Khubrani, 2020; Aleissa et al., 2022), necessitates studies on their drug response variability. Our study confirmed the uniqueness of the Saudi genome, as nine out of the 11 identified markers showed statistically significant differences in their allele frequencies between the Saudi group and at least one of the populations with different ancestral backgrounds. The variant selection in this study was informed by prior findings from Caucasian population studies, necessitating complementary next-generation sequencing (NGS) approaches [whole-genome sequencing, exome sequencing, or targeted sequencing of regions near actionable SNPs] to fully characterize Saudi-specific genetic associations. While the Axiom PMD Research Array provided cost-effective detection of shared variants between Saudis and other populations, three key limitations emerged: 1) its coverage is limited to pre-designed probes (fails to detect novel variants), 2) covers <5% of regulatory regions, and 3) unable to detect structural variants larger than 50 kilobases (kb)] (Wheelan et al., 2008; Miller and Tang, 2009).
5 Conclusion
Eleven variants in nine genes, with the potential to impact 11 therapeutic agents, were found to be inherited among the enrolled Saudi cohort. The identified data can be integrated into clinical practice in Saudi Arabia. This includes developing guidelines for healthcare providers on using genetic information to tailor drug therapy.
Data availability statement
The datasets presented in this study can be found in online repositories. The names of the repository/repositories and accession number(s) can be found in the article/Supplementary Material.
Ethics statement
The study involved humans and was approved by the Institutional Review Board (IRB) Committee (reference protocol number: NRR24/011/12) at King Abdullah International Medical Research Center (KAIMRC). The study was conducted in accordance with the local legislation and institutional requirements. The participants provided their written informed consent to participate in this study.
Author contributions
MA: Conceptualization, Data curation, Formal Analysis, Funding acquisition, Methodology, Validation, Writing – original draft, Writing – review and editing. DA: Methodology, Resources, Writing – review and editing. SS: Data curation, Funding acquisition, Resources, Validation, Writing – review and editing. FA: Data curation, Investigation, Writing – review and editing. AA: Formal Analysis, Validation, Writing – review and editing.
Funding
The author(s) declare that financial support was received for the research and/or publication of this article. The financial support for this research project was obtained from KAIMRC (reference protocol number: NRR24/011/12), Riyadh, Saudi Arabia.
Acknowledgments
The current work was also supported by Princess Nourah bint Abdulrahman University Researchers Supporting Project number: PNURSP2025R814, Princess Nourah bint Abdulrahman University, Riyadh, Saudi Arabia.
Conflict of interest
The authors declare that the research was conducted in the absence of any commercial or financial relationships that could be construed as a potential conflict of interest.
Generative AI statement
The author(s) declare that no Gen AI was used in the creation of this manuscript.
Publisher’s note
All claims expressed in this article are solely those of the authors and do not necessarily represent those of their affiliated organizations, or those of the publisher, the editors and the reviewers. Any product that may be evaluated in this article, or claim that may be made by its manufacturer, is not guaranteed or endorsed by the publisher.
Supplementary material
The Supplementary Material for this article can be found online at: https://www.frontiersin.org/articles/10.3389/fphar.2025.1559399/full#supplementary-material
References
Abou Tayoun, A. N., Fakhro, K. A., Alsheikh-Ali, A., and Alkuraya, F. S. (2021). Genomic medicine in the Middle East. Genome Med. 13, 184–193. doi:10.1186/s13073-021-01003-9
Al-Ashwal, A., Al Zahrani, A., Dammas, N., Aletani, L., and Alhuthil, R. (2023). CYP3A4 mutation causes vitamin D-dependent rickets type 3: a case report in Saudi Arabia. Cureus 15, e49976–e49977. doi:10.7759/cureus.49976
Aleissa, M., Aloraini, T., Alsubaie, L. F., Hassoun, M., Abdulrahman, G., Swaid, A., et al. (2022). Common disease-associated gene variants in a Saudi Arabian population. Ann. Saudi Med. 42, 29–35. doi:10.5144/0256-4947.2022.29
Al-Qahtani, A., Al-Anazi, M., Abdo, A. A., Sanai, F. M., Al-Hamoudi, W., Alswat, K. A., et al. (2015). Correlation between genetic variations and serum level of interleukin 28B with virus genotypes and disease progression in chronic hepatitis C virus infection. J. Immunol. Res. 2015, 768470–768510. doi:10.1155/2015/768470
Alshabeeb, M. A., Alwadaani, D., Al Qahtani, F. H., Abohelaika, S., Alzahrani, M., Al Zayed, A., et al. (2023). Impact of genetic variations on thromboembolic risk in Saudis with sickle cell disease. Genes. 14, 1919–2016. doi:10.3390/genes14101919
Alshabeeb, M. A., Alyabsi, M., Aziz, M. A., and Abohelaika, S. (2022a). Pharmacogenes that demonstrate high association evidence according to CPIC, DPWG, and PharmGKB. Front. Med. 9, 1001876–1001912. doi:10.3389/fmed.2022.1001876
Alshabeeb, M. A., Alyabsi, M., and Paras, B. (2022b). Prevalence of exposure to pharmacogenetic drugs by the Saudis treated at the health care centers of the Ministry of National Guard. Saudi Pharm. J. 30, 1181–1192. doi:10.1016/j.jsps.2022.06.013
Alvarellos, M. L., Krauss, R. M., Wilke, R. A., Altman, R. B., and Klein, T. E. (2016). PharmGKB summary: very important pharmacogene information for: RYR1. Pharmacogenetics genomics 26, 138–144. doi:10.1097/FPC.0000000000000198
Anderson, J. D., Davis, B. H., Giang, G., Jones, A., Lee, C. R., Parker, K., et al. (2023). Pharmacogenetic actionability and medication prescribing in people with cystic fibrosis. Clin. Transl. Sci. 16, 662–672. doi:10.1111/cts.13479
Angamo, M. T., Chalmers, L., Curtain, C. M., and Bereznicki, L. R. (2016). Adverse-drug-reaction-related hospitalisations in developed and developing countries: a review of prevalence and contributing factors. Drug Saf. 39, 847–857. doi:10.1007/s40264-016-0444-7
Banjar, H., Al-Mogarri, I., Nizami, I., Al-Haider, S., Almaghamsi, T., Alkaf, S., et al. (2021). Geographic distribution of cystic fibrosis transmembrane conductance regulator (CFTR) gene mutations in Saudi Arabia. Int. J. Pediatr. Adolesc. Med. 8, 25–28. doi:10.1016/j.ijpam.2019.12.002
Beam, T. A., Loudermilk, E. F., and Kisor, D. F. (2017). Pharmacogenetics and pathophysiology of CACNA1S mutations in malignant hyperthermia. Physiol. Genomics 49, 81–87. doi:10.1152/physiolgenomics.00126.2016
Biswas, M., Jinda, P., and Sukasem, C. (2023). Pharmacogenomics in Asians: differences and similarities with other human populations. Expert Opin. Drug Metabolism and Toxicol. 19, 27–41. doi:10.1080/17425255.2023.2178895
Brixner, D., Biltaji, E., Bress, A., Unni, S., Ye, X., Mamiya, T., et al. (2016). The effect of pharmacogenetic profiling with a clinical decision support tool on healthcare resource utilization and estimated costs in the elderly exposed to polypharmacy. J. Med. Econ. 19, 213–228. doi:10.3111/13696998.2015.1110160
Chen, J., Lin, C., Wang, C., Lin, Y., Kuo, S., Shiu, C., et al. (2011). IL28B genetic variations are associated with high sustained virological response (SVR) of interferon-α plus ribavirin therapy in Taiwanese chronic HCV infection. Genes. and Immun. 12, 300–309. doi:10.1038/gene.2011.1
Chen, Y., Zou, K., Sun, J., Yang, Y., and Liu, G. (2017). Are gene polymorphisms related to treatment outcomes of methotrexate in patients with rheumatoid arthritis? A systematic review and meta-analysis. Pharmacogenomics 18, 175–195. doi:10.2217/pgs-2016-0158
Chenchula, S., Atal, S., and Uppugunduri, C. R. S. (2024). A review of real-world evidence on preemptive pharmacogenomic testing for preventing adverse drug reactions: a reality for future health care. Pharmacogenomics J. 24, 9–6. doi:10.1038/s41397-024-00326-1
Da Rocha, J. E., Lombard, Z., and Ramsay, M. (2021). Potential impact of DPYD variation on fluoropyrimidine drug response in sub-Saharan African populations. Front. Genet. 12, 626954–626958. doi:10.3389/fgene.2021.626954
Deenen, M. J., Meulendijks, D., Cats, A., Sechterberger, M. K., Severens, J. L., Boot, H., et al. (2016). Upfront genotyping of DPYD* 2A to individualize fluoropyrimidine therapy: a safety and cost analysis. J. Clin. Oncol. 34, 227–234. doi:10.1200/JCO.2015.63.1325
Desta, Z., Saussele, T., Ward, B., Blievernicht, J., Li, L., Klein, K., et al. (2007). Impact of CYP2B6 polymorphism on hepatic efavirenz metabolism in vitro. Pharmacogenomics 8, 547–558. doi:10.2217/14622416.8.6.547
Eliades, N., and Eliades, D. (2009). Haplotype analysis: software for analysis of haplotype data. Forest Goettingen (Germany): Genetics and Forest Tree Breeding. Georg-August University Goettingen.
Goljan, E., Abouelhoda, M., Elkalioby, M. M., Jabaan, A., Alghithi, N., Meyer, B. F., et al. (2022). Identification of pharmacogenetic variants from large scale next generation sequencing data in the Saudi population. Plos one 17, 02631377–e263215. doi:10.1371/journal.pone.0263137
Gonsalves, S. G., Dirksen, R. T., Sangkuhl, K., Pulk, R., Alvarellos, M., Vo, T., et al. (2019). Clinical Pharmacogenetics Implementation Consortium (CPIC) guideline for the use of potent volatile anesthetic agents and succinylcholine in the context of RYR 1 or CACNA 1S genotypes. Clin. Pharmacol. and Ther. 105, 1338–1344. doi:10.1002/cpt.1319
Harrison, P. W., Amode, M. R., Austine-Orimoloye, O., Azov, A. G., Barba, M., Barnes, I., et al. (2024). Ensembl 2024. Nucleic acids Res. 52, D891–D899. doi:10.1093/nar/gkad1049
Haseeb, M., and Thompson, P. D. (2021). The effect of statins on RyR and RyR-associated disease. J. Appl. Physiology 131, 661–671. doi:10.1152/japplphysiol.01003.2020
Hesselink, D. A., Van Schaik, R. H., Van Der Heiden, I. P., Van Der Werf, M., Gregoor, P. J. S., Lindemans, J., et al. (2003). Genetic polymorphisms of the CYP3A4, CYP3A5, and MDR-1 genes and pharmacokinetics of the calcineurin inhibitors cyclosporine and tacrolimus. Clin. Pharmacol. and Ther. 74, 245–254. doi:10.1016/S0009-9236(03)00168-1
Ho, R. H., Tirona, R. G., Leake, B. F., Glaeser, H., Lee, W., Lemke, C. J., et al. (2006). Drug and bile acid transporters in rosuvastatin hepatic uptake: function, expression, and pharmacogenetics. Gastroenterology 130, 1793–1806. doi:10.1053/j.gastro.2006.02.034
Isackson, P. J., Wang, J., Zia, M., Spurgeon, P., Levesque, A., Bard, J., et al. (2018). RYR1 and CACNA1S genetic variants identified with statin-associated muscle symptoms. Pharmacogenomics 19, 1235–1249. doi:10.2217/pgs-2018-0106
Jithesh, P. V., Abuhaliqa, M., Syed, N., Ahmed, I., El Anbari, M., Bastaki, K., et al. (2022). A population study of clinically actionable genetic variation affecting drug response from the Middle East. NPJ genomic Med. 7, 10. doi:10.1038/s41525-022-00281-5
Kharasch, E. D., Regina, K. J., Blood, J., and Friedel, C. (2015). Methadone pharmacogenetics: CYP2B6 polymorphisms determine plasma concentrations, clearance and metabolism. Anesthesiology 123, 1142–1153. doi:10.1097/ALN.0000000000000867
Khubrani, Y. (2020). Genetic diversity and population structure of Saudi Arabia. Leicester: University of Leicester. PhD. 2020-01-02.
Kwara, A., Cao, L., Yang, H., Poethke, P., Kurpewski, J., Tashima, K. T., et al. (2014). Factors associated with variability in rifampin plasma pharmacokinetics and the relationship between rifampin concentrations and induction of efavirenz clearance. Pharmacother. J. Hum. Pharmacol. Drug Ther. 34, 265–271. doi:10.1002/phar.1388
Larrue, R., Fellah, S., Hennart, B., Sabaouni, N., Boukrout, N., Van Der Hauwaert, C., et al. (2024). Integrating rare genetic variants into DPYD pharmacogenetic testing may help preventing fluoropyrimidine-induced toxicity. Pharmacogenomics J. 24, 1–9. doi:10.1038/s41397-023-00322-x
Lee, S.-H., Ahn, W.-Y., Seweryn, M., and Sadee, W. (2018). Combined genetic influence of the nicotinic receptor gene cluster CHRNA5/A3/B4 on nicotine dependence. BMC genomics 19, 826–911. doi:10.1186/s12864-018-5219-3
Lima, A., Bernardes, M., Azevedo, R., Seabra, V., and Medeiros, R. (2016). Moving toward personalized medicine in rheumatoid arthritis: SNPs in methotrexate intracellular pathways are associated with methotrexate therapeutic outcome. Pharmacogenomics 17, 1649–1674. doi:10.2217/pgs-2016-0067
Mcdermott, J. H., Wright, S., Sharma, V., Newman, W. G., Payne, K., and Wilson, P. (2022). Characterizing pharmacogenetic programs using the consolidated framework for implementation research: a structured scoping review. Front. Med. 9, 945352–945414. doi:10.3389/fmed.2022.945352
Mcdonagh, E. M., Boukouvala, S., Aklillu, E., Hein, D. W., Altman, R. B., and Klein, T. E. (2014). PharmGKB summary: very important pharmacogene information for: N:-acetyltransferase 2. Pharmacogenetics genomics 24, 409–425. doi:10.1097/FPC.0000000000000062
Miller, M. B., and Tang, Y.-W. (2009). Basic concepts of microarrays and potential applications in clinical microbiology. Clin. Microbiol. Rev. 22, 611–633. doi:10.1128/CMR.00019-09
Mizzi, C., Dalabira, E., Kumuthini, J., Dzimiri, N., Balogh, I., Başak, N., et al. (2016). A European spectrum of pharmacogenomic biomarkers: implications for clinical pharmacogenomics. PloS one 11, 01628666–e162919. doi:10.1371/journal.pone.0162866
Mulder, T. A., Van Eerden, R. A., De With, M., Elens, L., Hesselink, D. A., Matic, M., et al. (2021). CYP3A4∗ 22 genotyping in clinical practice: ready for implementation? Front. Genet. 12, 1–10. doi:10.3389/fgene.2021.711943
Mykkänen, A. J., Taskinen, S., Neuvonen, M., Paile-Hyvärinen, M., Tarkiainen, E. K., Lilius, T., et al. (2022). Genomewide association study of simvastatin pharmacokinetics. Clin. Pharmacol. and Ther. 112, 676–686. doi:10.1002/cpt.2674
Oetting, W. S., Wu, B., Schladt, D. P., Guan, W., Remmel, R. P., Dorr, C., et al. (2018). Attempted validation of 44 reported SNPs associated with tacrolimus troughs in a cohort of kidney allograft recipients. Pharmacogenomics 19, 175–184. doi:10.2217/pgs-2017-0187
Pianezza, M. L., Sellers, E. M., and Tyndale, R. F. (1998). Nicotine metabolism defect reduces smoking. Nature 393, 750. doi:10.1038/31623
Pirmohamed, M. (2023). Pharmacogenomics: current status and future perspectives. Nat. Rev. Genet. 24, 350–362. doi:10.1038/s41576-022-00572-8
Prokunina-Olsson, L. (2019). Genetics of the human interferon lambda region. J. Interferon and Cytokine Res. 39, 599–608. doi:10.1089/jir.2019.0043
Qiu, Q., Huang, J., Shu, X., Fan, H., Zhou, Y., and Xiao, C. (2017). Polymorphisms and pharmacogenomics for the clinical efficacy of methotrexate in patients with rheumatoid arthritis: a systematic review and meta-analysis. Sci. Rep. 7, 44015–44024. doi:10.1038/srep44015
Sangkuhl, K., Dirksen, R. T., Alvarellos, M. L., Altman, R. B., and Klein, T. E. (2020). PharmGKB summary: very important pharmacogene information for CACNA1S. Pharmacogenetics genomics 30, 34–44. doi:10.1097/FPC.0000000000000393
Sarwar, E. (2023). “Clinical significance of precision medicine–genomics and pharmacogenomics (PGx),” in Global perspectives on precision medicine: ethical, social and public health implications. Editor E. SARWAR, 1st ed. (San Bernardino, USA: California State University).
Tayeb, M. T., Clark, C., Ameyaw, M.-M., Haites, N. E., Evans, D. P., Tariq, M., et al. (2000). CYP3A4 promoter variant in Saudi, Ghanaian and Scottish Caucasian populations. Pharmacogenetics Genomics 10, 753–756. doi:10.1097/00008571-200011000-00009
Thorn, C. F., Klein, T. E., and Altman, R. B. (2013). PharmGKB: the pharmacogenomics knowledge base. Methods Mol. Biol. 1015, 311–320. doi:10.1007/978-1-62703-435-7_20
Van Der Wouden, C. H., Cambon-Thomsen, A., Cecchin, E., Cheung, K.-C., Dávila-Fajardo, C. L., Deneer, V. H., et al. (2017). Implementing pharmacogenomics in Europe: design and implementation strategy of the ubiquitous pharmacogenomics consortium. Clin. Pharmacol. and Ther. 101, 341–358. doi:10.1002/cpt.602
Vladutiu, G. D., and Isackson, P. J. (2009). SLCO1B1 variants and statin-induced myopathy. N. Engl. J. Med. 360, 304. doi:10.1056/NEJMc081975
Wheelan, S. J., Murillo, F. M., and Boeke, J. D. (2008). The incredible shrinking world of DNA microarrays. Mol. Biosyst. 4, 726–732. doi:10.1039/b706237k
Whirl-Carrillo, M., Huddart, R., Gong, L., Sangkuhl, K., Thorn, C. F., Whaley, R., et al. (2021). An evidence-based framework for evaluating pharmacogenomics knowledge for personalized medicine. Clin. Pharmacol. and Ther. 110, 563–572. doi:10.1002/cpt.2350
Wigle, T. J., Povitz, B. L., Medwid, S., Teft, W. A., Legan, R. M., Lenehan, J., et al. (2021). Impact of pretreatment dihydropyrimidine dehydrogenase genotype-guided fluoropyrimidine dosing on chemotherapy associated adverse events. Clin. Transl. Sci. 14, 1338–1348. doi:10.1111/cts.12981
Zhang, J., Zhang, L., Zhao, X., Shen, S., Luo, X., and Zhang, Y. (2018). Association between MDR1/CYP3A4/OPRM1 gene polymorphisms and the post-caesarean fentanyl analgesic effect on Chinese women. Gene 661, 78–84. doi:10.1016/j.gene.2018.03.081
Keywords: Pharmacogenomics (PGx), ATIC, CHRNA5, CYP3A4, IFNL3, SLC19A1, SLCO1B1, Saudis
Citation: Alshabeeb MA, Alwadaani D, Shilbayeh SAR, Alherz FA and Alghubayshi A (2025) Unveiling the heritability of selected unexplored pharmacogenetic markers in the Saudi population. Front. Pharmacol. 16:1559399. doi: 10.3389/fphar.2025.1559399
Received: 14 January 2025; Accepted: 31 March 2025;
Published: 01 May 2025.
Edited by:
Henk-Jan Guchelaar, Leiden University Medical Center (LUMC), NetherlandsReviewed by:
Dora Janeth Fonseca, Rosario University, ColombiaMaha Mohamed Saber-Ayad, University of Sharjah, United Arab Emirates
Shivashankar Nagaraj, Queensland University of Technology, Australia
Copyright © 2025 Alshabeeb, Alwadaani, Shilbayeh, Alherz and Alghubayshi. This is an open-access article distributed under the terms of the Creative Commons Attribution License (CC BY). The use, distribution or reproduction in other forums is permitted, provided the original author(s) and the copyright owner(s) are credited and that the original publication in this journal is cited, in accordance with accepted academic practice. No use, distribution or reproduction is permitted which does not comply with these terms.
*Correspondence: Mohammad A. Alshabeeb, c2hhYmVlYm1vQG5naGEubWVkLnNh