- 1Department of Anatomy and Clinical Anatomy, School of Medicine, University of Zagreb, Zagreb, Croatia
- 2Department of Neuroscience, Croatian Institute for Brain Research, School of Medicine, University of Zagreb, Zagreb, Croatia
- 3Center of Excellence for Basic, Clinical and Translational Neuroscience, School of Medicine, University of Zagreb, Zagreb, Croatia
- 4Department of Neuroscience and Cell Biology, Rutgers University, Robert Wood Johnson Medical School, Piscataway, NJ, United States
- 5Department of Medical Biology, School of Medicine, University of Zagreb, Zagreb, Croatia
The human specific cognitive shift starts around the age of 2 years with the onset of self-awareness, and continues with extraordinary increase in cognitive capacities during early childhood. Diffuse changes in functional connectivity in children aged 2–6 years indicate an increase in the capacity of cortical network. Interestingly, structural network complexity does not increase during this time and, thus, it is likely to be induced by selective maturation of a specific neuronal subclass. Here, we provide an overview of a subclass of cortico-cortical neurons, the associative layer IIIC pyramids of the human prefrontal cortex. Their local axonal collaterals are in control of the prefrontal cortico-cortical output, while their long projections modulate inter-areal processing. In this way, layer IIIC pyramids are the major integrative element of cortical processing, and changes in their connectivity patterns will affect global cortical functioning. Layer IIIC neurons have a unique pattern of dendritic maturation. In contrast to other classes of principal neurons, they undergo an additional phase of extensive dendritic growth during early childhood, and show characteristic molecular changes. Taken together, circuits associated with layer IIIC neurons have the most protracted period of developmental plasticity. This unique feature is advanced but also provides a window of opportunity for pathological events to disrupt normal formation of cognitive circuits involving layer IIIC neurons. In this manuscript, we discuss how disrupted dendritic and axonal maturation of layer IIIC neurons may lead into global cortical disconnectivity, affecting development of complex communication and social abilities. We also propose a model that developmentally dictated incorporation of layer IIIC neurons into maturing cortico-cortical circuits between 2 to 6 years will reveal a previous (perinatal) lesion affecting other classes of principal neurons. This “disclosure” of pre-existing functionally silent lesions of other neuronal classes induced by development of layer IIIC associative neurons, or their direct alteration, could be found in different forms of autism spectrum disorders. Understanding the gene-environment interaction in shaping cognitive microcircuitries may be fundamental for developing rehabilitation and prevention strategies in autism spectrum and other cognitive disorders.
Quantitative Expansion of the Cerebral Cortex and Microcircuitry Changes: Role in the Appearance of Complex Human-specific Cognition
Increase in brain size, particularly an increase in the number of neuronal columns of the cerebral cortex, is the prerequisite enabling humans to achieve tremendous mental capabilities such as self-awareness, consciousness, language, abstract thinking, cognitive flexibility, mathematical abilities, as well as representational memory and complex social cognition (1–6). These abilities are not only species-specific features; the cognitive state achieved by humans represents a new qualitative level in mental functioning (7–9). It is correct that some animal species, in particular apes, are able to achieve a rudimentary level of some of these mental abilities (10–12). However, complex neuropsychiatric disorders as autism, schizophrenia or psychopathy are not present in any other species (13–17), which sets humans apart regarding cognitive and emotional features and capacities.
One of the most important human-specific abilities is complex social cognition, which includes processing, storing, and applying information about other people and social situations (18). Social cognition is the base for complex personal competencies, which are altered in the above mentioned diseases. From a neurobiological point of view, it is interesting that fundamental cognitive shift, which sets up human-specific cognitive abilities, the ability to understand the mental state of oneself or others (mentalization, i.e., “theory of mind”), appears in the period of transition from infancy to childhood (19–21). Humans and great apes (as our closest relatives) share roughly the same course of psychological development during the first 18 months of life (22). Around this age in both species, the brain nearly achieves adult neuronal composition, and even overall size (23–25). Nevertheless, in humans, intensive and diffuse changes in functional connectivity continue throughout the rest of childhood (26–31), while apes do not exhibit further important progress in cognitive capacities after the second postnatal year (22).
How did this unmatched shift in mental functioning between apes and humans appear without a robust quantitative increase in overall brain structure, i.e., overall increase in complexity of dendrites, or formation of new pathways and connections on most of the neurons? It should be noted, that a tremendous increase in the number of cortical neurons and connections, is a biological prerequisite to enabling high cognitive functioning (32–37). But at a certain point further quantitative expansion is not enough to initiate a more complex functional outcome, since the present pattern of organization does not allow proper integration inside numerically expanded circuitries. To make such an expanding system function properly, new microcircuitries that provide novel integrative properties are needed (31, 38–42). As such, the enhanced integration across cortical areas, along with an increase in network processing capacities, could come as a result of structural changes inside few selective microcircuitries (43–48).
It is possible that such changes are focused onto specific cortical areas. For example, the prefrontal cortex has abundant connections to most of the remaining cortical areas (49–53). Therefore, changes in functional properties of prefrontal cortex output (Figure 1) change the information processing throughout the whole brain (54). To produce considerable functional changes to the output, structural changes within the prefrontal cortex do not need to be “robust,” i.e., they do not need to include dendritic growth of a wide range of neurons. Even fine changes, e.g., growth focused on selective neuronal populations that have rich local connectivity, may cause dramatic changes in functional properties of the prefrontal cortex. Based on previous work by our and other research groups, we suggest that deep located large layer III(C) pyramidal neurons (L3N) of the human prefrontal cortex could perform such a role.
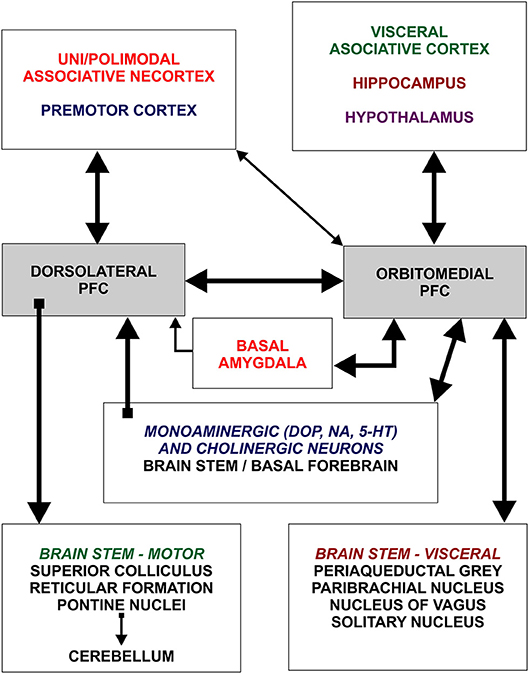
Figure 1. Schematic representation of two main subdivisions of the prefrontal cortex and general organization of their afferent and efferent connections. Dorsolateral prefrontal cortex establishes rich connections with all neocortical areas, except primary regions, whereas orbitomedial prefrontal cortex is mainly connected with hippocampus and cortical regions processing visceral information. Note that dorsolateral and orbitomedial prefrontal cortex are densely interconnected. Figure is based on Groenewegen and Uylings (49). DOP, dopamine; NA, noradrenaline; 5HT, serotonin.
The L3N have a unique developmental pattern during early childhood (55, 56) that correlates with the appearance and boost in the maturation of cognitive abilities, such as self-awareness and complex social cognition. With a detailed overview of mature connectivity patterns, here we present a possible integrative role of L3N in cortico-cortical network processing. Their selective alteration in pathological conditions could produce immense changes in mental capacities, due to the failure of proper integration (57, 58). Therefore, we suggest that alterations in mentalization and communication abilities found in the autism spectrum and social communication disorder are a result of disrupted development of circuitries established by L3N (59).
Molecular Features and Cytoarchitecture of Human Prefrontal Cortex: the Role of Associative Neurons in Inter-areal and Inter-columnar Connectivity
Evolution of the human brain (Figures 2A,B) is characterized by a sharp linear increase in the number of cortical neurons (67–69), but also with an exponential increase in the number of cortico-cortical projecting neurons (50, 67, 68). This leads to increased thickness of upper cortical layers (Figures 2C–E) which contain more neurons than lower layers populated by subcortically projecting neurons (3, 4, 39, 65, 66, 70, 71). So, the primate neocortex is characterized by a tremendous increase in the number of columns and changes to their internal neuronal composition (Figure 2F). Last but not least is the increase in width inside and between columns. The increase in width of columns is a result of increase in dendritic complexity of principal cells (72), i.e., more “space” between columns is a result of increased interconnectivity (73).
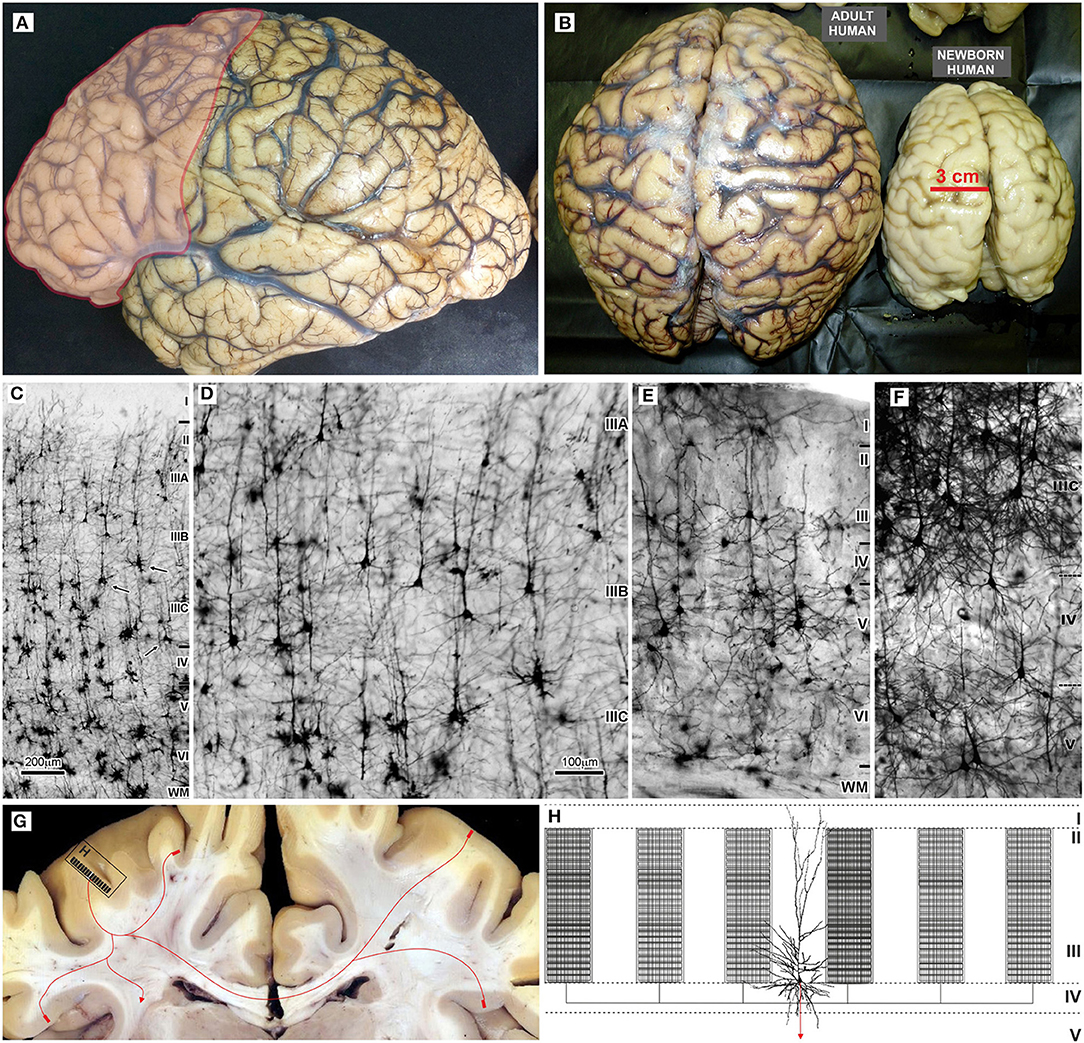
Figure 2. Macroscopic and microscopic features of the adult human brain, including schematic organization of extrinsic and intrinsic cortical projections of associative layer IIIC neurons. (A) In the adult human brain frontal granular cortex occupies 80% of the frontal lobe, and almost one third (red) of the total cortical surface. (B) Around the age of two, the brain is very close to its adult size (23). Microphotography of Golgi Cox (C–E) and rapid Golgi (F) impregnated sections of the associative areas in the human (C,D,F), and mice neocortex (E). (G) Large layer IIIC neurons are considered to be associative neurons, connecting several higher order areas in the ipsi- and contralateral hemisphere, with the columnar pattern of axon ramification (60, 61). Ipsilateral collaterals are much more numerous (H), and around 80% of synapses are established within the area of origin. Local axon branches are forming numerous terminal ramifications which have columnar distribution through layers II and III, and extend several millimeters around the cell body (62–64). The figure is a compilation of figures published by Hladnik et al. (65) (A,B) and Džaja et al. (66) (C–E). Scale bar 200 μm (C) and 100 μm (D–F).
These changes are present in a majority of cortical areas, but are most prominent in several prefrontal cortex areas and related with their important integrative function inside cortico-cortical network (52, 53, 74, 75). The connectivity pattern of human prefrontal cortex (Figure 1), particularly its highly expanded dorso-lateral part, is characterized by massive reciprocal projections to both, multimodal and unimodal-parasensory associative areas (44, 76). This allows the prefrontal cortex to have a major role in regulating synchronous and coordinated activity between cortical areas. Experimental studies in monkeys and functional studies in humans have confirmed that the prefrontal cortex is functionally the highest associative region of the primate brain (38, 49, 51).
In humans, some areas of the prefrontal cortex show a specific cytoarchitecture, the magnopyramidality of layer III (77), i.e., deep located large layer III neurons exceed the size of large layer V pyramids. When compared to other populations of cortico-cortical projecting neurons, the L3N show strong acetylcholinesterase (AChE) (78–80) and SMI32 (antibody against phosphorylated protein H) reactivity (81–83) as a result of higher metabolic rate and prominent axonal tree. The density of SMI32/AChE reactive L3N, as well as their size and intensity of staining, is higher in human associative areas than in monkeys. In lower mammals, SMI32/AChE reactivity in deep layer III was not found (84).
The L3N are a subset of cortico-cortical neurons with long ipsi- and contra-lateral projections (Figure 2G) (40, 60, 61, 85). Individual L3N establish projections to several different areas suggesting a major role in inter-areal integration that grants them the title “associative” neurons (86). They are characterized by an astonishing number of intracortical axonal collaterals (Figure 2H), that extend around the cell, having dense columnar distribution of their terminal ramification through layers II and III (62–64). Thus, L3N are playing the major role in intercolumnar connectivity within a particular cortical area. In monkeys, L3N are indeed the key element for processing working memory and other prefrontal cortex-dependent high cognitive functions (87–90).
Above mentioned features of connectivity, functional properties and evolutionary expansion support the idea that L3N underlie the highly efficient network integration throughout the human cerebral cortex. We propose that selective structural and molecular changes of associative L3N in the human prefrontal cortex around the age of 2 and several upcoming years (55, 56), change the properties of the whole prefrontal cortex output, and have a pivotal role in cognitive maturation characterizing the preschool period. Developmental changes selectively related to this neuron class may be crucial for the appearance of cognitive abilities needed for the understanding of higher levels of inter-personal interaction, and to lay foundation for a further increase in cognitive capacity observed later throughout the childhood and adolescence, that ultimately leads to socio-emotional maturity. Selective alterations of the L3N were described in neuropathological states characterized by intense and global changes in the efficiency of the cortical network (91–96). We propose that selective alterations of associative L3N have a pivotal role in the “dis-connectivity” of prefrontal cortex found in autism spectrum disorder (97, 98), but also in other prefrontal cortex-associated disorders, like schizophrenia (90, 99, 100). These two disorders share similarities in cognitive pathology, and are characterized by global cortical dysfunction, without concurrent structural alterations and specific structural pathology identified so far (16, 17).
Understanding the Mental States of Oneself or Others: Neuronal Development of Prefrontal Cortex During Early Childhood and Focal Disconnectivity in Autism
The capacity to attribute mental states (mentalization) remains one of the quintessential abilities that makes us human and is defined as the “theory-of-mind” (ToM) (20, 21, 101, 102). This ability appears during the second year of life and subsequently sophisticates through childhood with cognitive spurts at specific time points (103). Following temporal pattern at which various levels of ToM have been achieved is important since it reflects changes inside cortical circuitries which allow shifts in mental capacities.
Most data marks the infant to child transition as a point when first ToM abilities appear, i.e., during the second year of life. Infants around 12–15 months of age display behaviors that are prerequisites to ToM development (104–106). Nevertheless, it is difficult to talk about internalization of mental abilities before 18 months of age (107, 108). By the age of 2, children are clearly aware that there is a difference between thoughts in their mind, and things in their surroundings (109). An average 3-year-old knows that the brain has a set of mental functions, such as dreaming, wanting, thinking, and that different persons may want, like and feel different things. Further important cognitive twists occur around the age of 4, when children realize that thoughts might not be true (110). Also, a 4-year-old can remember that their own belief has changed which is not the case with a 3-year-old (111). By the end of early childhood (ages 5 to 6), children realize that people talk and act on the basis how they think the world is, even when it does not reflect the reality of the situation. They can keep secrets and understand that sometimes a person may believe something that is not true, and that what a person does or says, can be based on a false belief (112, 113).
The cortical areas related to ToM tasks typically activate the frontal lobe. In particular, neuroimaging studies of ToM showed activations in the dorsal prefrontal cortex (Brodmann area 9). However, other frontal regions were also involved in understanding and controlling oneself, as well as in interaction of thoughts about oneself and others (112, 114–117). Therefore, the prefrontal cortex can be considered as a region with a key role in social cognition, and it is assumed that pathological substrates in states characterized by disrupted social cognition, such as psychopathy personality (14) and autism spectrum disorder (59, 118), must be located within.
In autism spectrum disorder (ASD) and related social (pragmatic) communication disorders, social interactions are affected (119) due to difficulties in the aptitude for inferring other people's states of mind, such as intentions, beliefs, desires and wishes (120). When a false-belief test is applied to children with ASD, most of them fail even at the age of 11, in contrast to typically developed children who pass the test by the age of 4 (121). In a modified (122) and simplified (123) form of the false belief task, typically developing children show ToM abilities latest by the age of 2.5, while many of them show it already at the age of 1.5 years (107, 124). These abilities are lacking in ASD subjects, showing that deficiency in the ability to reflect on the contents of one's own and other's minds (101, 125) is a core cognitive feature of ASD. This lack is a consequence of a disturbed cognitive development at some point during the period of early childhood (1.5–6 years) (126–128). In search for neurobiological correlations, it is important to recognize that cognitive impairment in ASD is specific and different from learning difficulties of blind or deaf people (129–131).
Neuroimaging data show that dorsolateral and medial prefrontal cortex in ASD are hyperconnected during the second year of life (132–137). In parallel with hyperconnectivity, many of ASD individuals undergo brain overgrowth that is particularly pronounced in the frontal lobes (138–141). The hyperconnectivity later on changes to hypoconnectivity (142–144). Therefore, it is still debated if ASD should be considered as a disorder characterized by hyper- or hypoconnectivity (145, 146).
Nevertheless, the concept of hypo- or hyperconnectivity seems to be oversimplified. Neuroimaging data are in line with the view that ASD symptomatology is the result of disconnection in areas involved in the processing of language, executive and socioemotional reaction as well as in abstract and conceptual thinking. Furthermore, there is a disconnection in cortical regions that are highly evolved in humans and involve higher-order associative processing along with the prefrontal cortex (147–151). Alteration in ASD was also found in many regions of the temporal lobe and in adjoining parts of the occipital and parietal lobes, including the insula and regions important for ToM processing (117, 125, 152, 153). However, the majority of functional and structural connectivity studies in ASD suggest that the key disconnection must be between the frontal lobe and other higher order association cortices (154–160), and that the frontotemporal, frontolimbic, frontoparietal, and interhemispheric connections are altered. In addition, alterations in synaptic organization related to specific deficits were found (161–166). The type and range of cognitive pathology in ASD suggests that structural alterations are focused, as well as delicate, but present even among highly-functional adults with ASD (122, 167–169). Preservation (or even enhancement) of other mental functions (170, 171) suggests that development of certain circuitries is spared, supporting the model of “focal disconnections” which appear during development. Therefore, ASD should be considered as a form of “developmental disconnection syndrome” (172–174).
In conclusion, structural and functional data in ASD suggest that development of specific micro-circuitries is disrupted during the ToM acquiring stage of infant to child transition (second year of life), or in milder ASD forms during the upcoming years (118, 120, 175, 176). The role of distinct neuron classes in the prefrontal cortex for processing ToM and complex social cognition is not yet defined, and therefore the neuronal correlates of ASD pathology remain unknown (177).
Based on connectivity patterns, as well as the pattern of their development and maturation, selective changes of associative L3N microcircuits in the prefrontal cortex could represent one of the major biological substrates for normal cognitive development during early childhood. Consequently, abnormal L3N development could be associated with appearance of ASD symptomatology.
Sequential Differentiation of Principal Neurons in the Prefrontal Cortex And Early Development of Cortico-cortical Microcircuitry
Mechanism of Dendritic Growth
Development of dendrites is one of the essential processes in differentiation and maturation of neuronal circuitry (178–182). Developmental changes in dendritic size and complexity will define the total neuronal receptive field. Since dendritic development occurs in parallel with rapid synaptogenesis (183) and axon growth (184, 185), it will affect both the neuronal functional response to the input and the neuronal output (186). Dendritic development typically undergoes three phases:
The first phase of dendritic growth starts after the neuron arrives to its final position within the cortical plate (37, 187, 188). This phase is characterized by the protrusion of primary basal dendrites and apical dendrite, which arise from the cell body, including appearance of oblique dendrites which grow out on the proximal site of the apical dendrite (189). No significant outgrowth of additional branches on primary oblique and basal dendrites occurred during this phase (Figure 3).
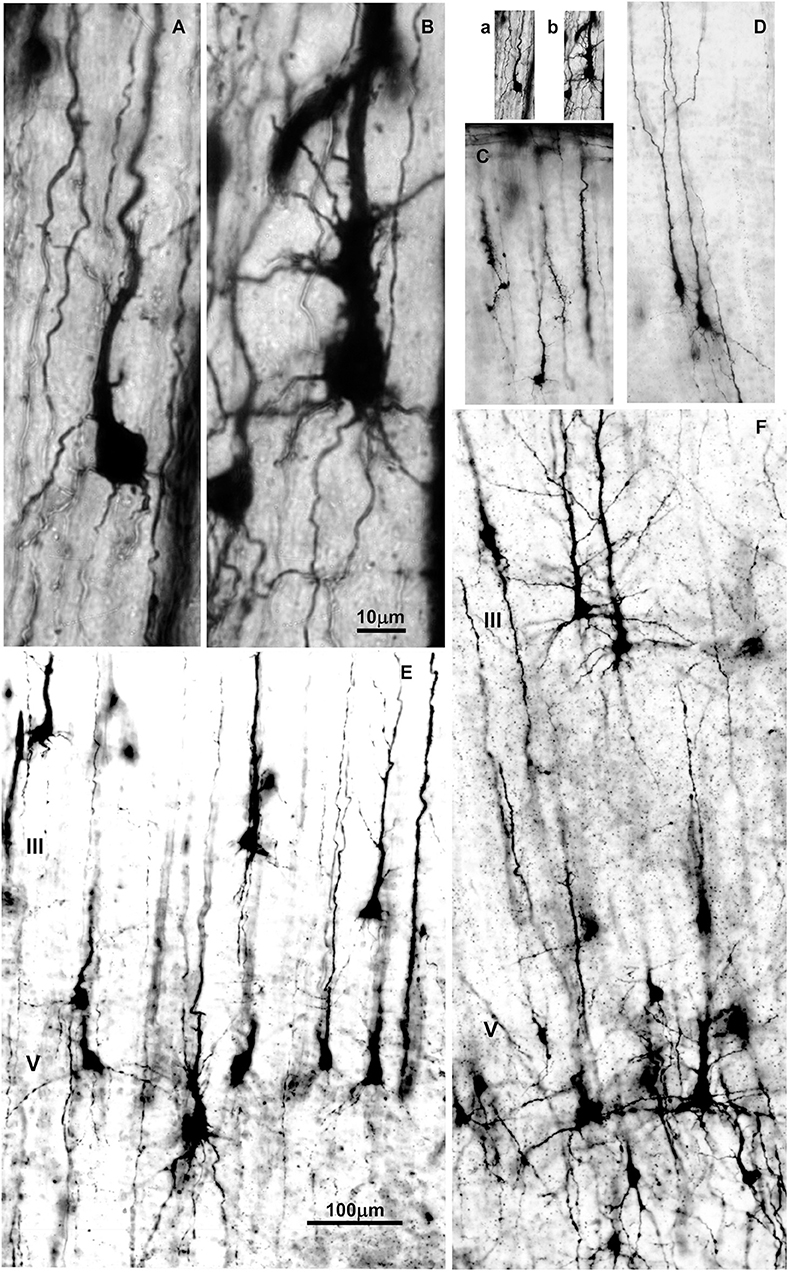
Figure 3. Changes in dendritic morphology of rapid-Golgi impregnated pyramidal neurons in the dorsolateral part of the prefrontal cortex during the second half of gestation (A–D) and first postnatal month (E,F). Microphotography of rapid Golgi impregnated sections in the human fetal prospective dorsolateral prefrontal cortex at 21 (A,B), and 32 postconceptional weeks (C,D), newborn (E) and one month old infant (F). Scale bar 10 μm (A,B) and 100 μm (C–F). (a,b) are (A,B) shown at the same magnification as (C–F).
The second phase is characterized by extensive and rapid growth of the dendritic tree. Initially, new segments grow out on the, during first phase formed primary basal and oblique dendrites (Figures 4A,B). This is followed by an increase in the size of the dendritic tree achieved mainly through elongation of present branches. Importantly, the appearance of functional glutamate receptors is crucial for inducement of rapid dendritic growth (183, 191–195). This strongly supports the view that ingrowth of glutamatergic thalamo-cortical and cortico-cortical afferents during the fetal and perinatal period, triggers the rapid dendritic growth of principal neurons (196).
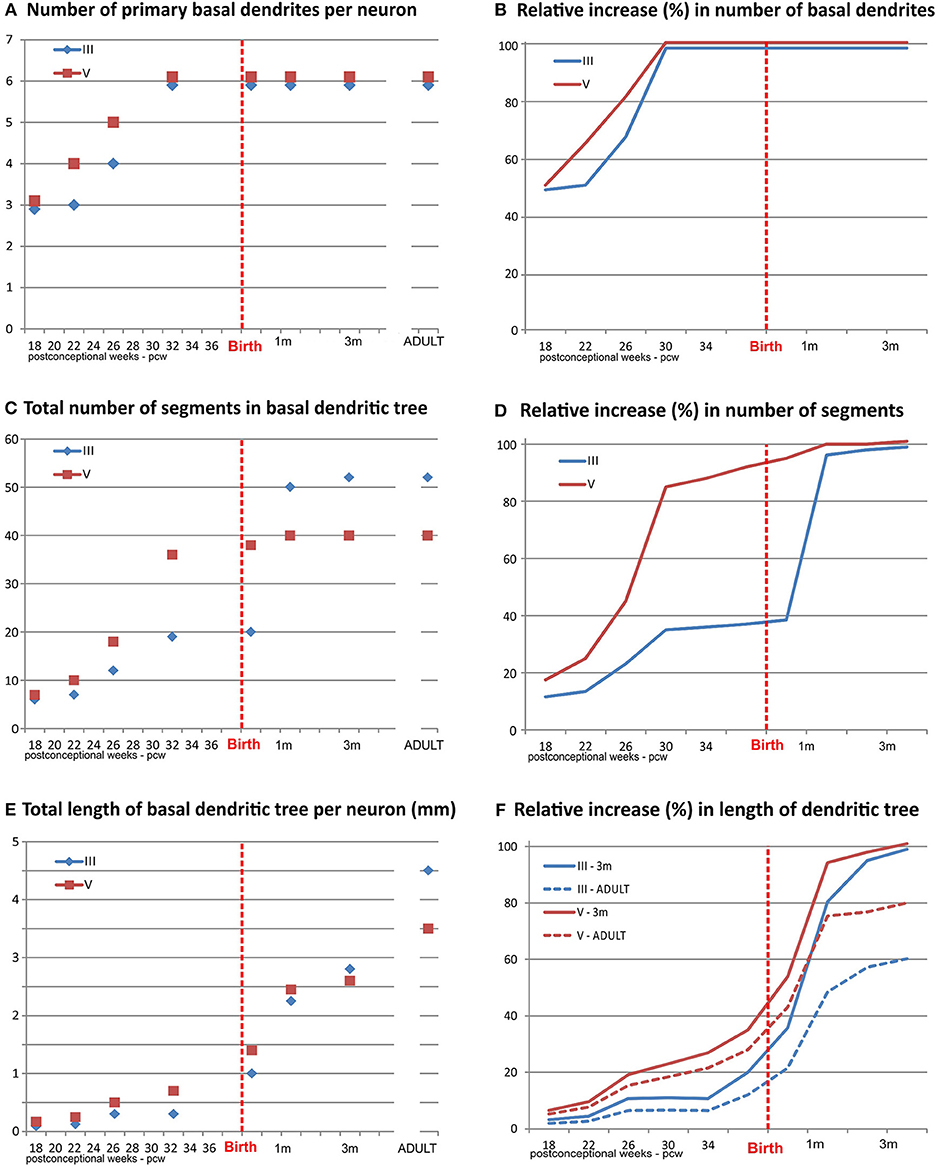
Figure 4. Graphical presentation of quantitative data from the basal dendritic tree of large layer III and V pyramidal neurons in the human dorsolateral prefrontal cortex, impregnated by a rapid Golgi method, in a period of rapid growth between 18 postconceptional weeks, and third postnatal month, compared to adult values. An outgrowth of primary basal dendrites (A) started earlier on layer V pyramidal neurons, during the middle trimester of gestation prior to layer III, but not later on (B). The number of basal dendritic segments (indicating frequency of bifurcation) shows a clear inside-out gradient until birth (C). A constant, slow outgrowth of new segments is present, for both classes, during the middle trimester of gestation, followed by rapid increase in period 26–32 PCW for layer V pyramidal neurons (D). The major outgrowth of new segments occurred for layer III pyramids during the first postnatal month. No additional segment outgrowth is observed after first postnatal month. Despite rapid segment outgrowth up to the 32 postconceptional weeks, the increase in total length for layer V pyramids (E) was rather slow. Most of the elongation occurred later, during the last 2 months of gestation and first postnatal month (F). At the same time, opposite to layer V, a considerable increase in length occurred for layer III during the period of rapid segment outgrowth. At the 3rd postnatal month, layer III rapid Golgi impregnated neurons have just exceeded 50%, whereas layer V pyramidal neurons exceed 80% of their adult length (dashed lines). Data shown here were extrapolated from the studies of Mrzljak et al. (190) and Petanjek et al. (55).
The final stage of dendritic growth (once up to 20% of total dendritic length is established) is characterized by significant, but much slower elongation of dendrites than during the second phase. Many connections established on developing dendrites are functional at the beginning of this stage, making dendritic development more sensitive to environmental influences (“nurture”). In contrast to the second phase, glutamatergic activity during the third phase stabilizes the dendritic tree instead of promoting its growth (199–201). As such, the last stage of dendritic development is the longest and is characterized by a slighter increase in the length of the dendritic tree (202, 203).
Intensive Perinatal Dendritic Growth of Associative Neurons Results in Early Functional Microcircuitries
Dendritic development and synaptic rearrangement of principal neurons have been extensively studied in the monkey and human prefrontal cortex (189, 190, 197, 199, 204–221). In the human fetal prefrontal cortex, intensive dendritic growth (second phase) of both deep layer III and V principal neurons, starts 12–15 weeks after they arrived into the cortical plate. However, beginning of the second phase differs between these two subpopulations of principal neurons. For the large layer V pyramidal neurons it coincides with the ingrowth of thalamo-cortical fibers into the cortical plate by the end of the middle trimester of gestation (188, 196, 222, 223). In contrast, intensive growth of the L3N begins with ingrowth of cortico-cortical fibers by the end of the last trimester of gestation (224, 225). Thus, for the two main classes of large pyramidal neurons in the human prefrontal cortex there is an inside-out gradient of differentiation during the prenatal period, and intensive growth seems to be induced by the arrival of specific glutamatergic afferents.
At birth, large layer V principal neurons have already attained their adult dendritic complexity (branching pattern), while L3N are not well developed (190), suggesting that the cortico-cortical network is not highly functional at that time (226–228). Indeed, the most intensive dendritic development of the L3N is the first postnatal month, when around 60% of basal dendritic segments appear (Figures 4C,D), and almost half of the total size is achieved (Figures 4E,F). Thus, the L3N dendritic tree “catch-up” large layer V pyramidal neurons in absolute values of their length and complexity soon after birth. By the third postnatal month both classes are equal in total dendritic tree length, and have achieved not only adult complexity, but also, adult-like overall dendritic three shape.
The maturity level of layer V principal cells reached soon after birth is not surprising, as these cells are key neuronal elements for processing early executive functions of the prefrontal cortex (43, 229–231). However, the maturity level of L3N reached between first and third postnatal month is somewhat surprising (55, 56), as these cells are believed to be the key elements involved in sophisticated, evolutionarily recent, and human-specific cognitive functions that develop later on (232, 233). Such an early functioning cortico-cortical neuronal network centered on L3N may represent a neurobiological basis for cognitive functions present already in the first months after birth (234–238). Behavioral and functional studies found that the perinatal period (32 week of gestation−3 months postnatal) is characterized by rapid transformation and disappearance of fetal patterns of behavior, but also with concomitant appearance of certain aspects of cognitive functions, which will intensively develop through infancy (124, 239–246).
Sequential Development of Microcircuitries in the Human Prefrontal Cortex During the First Postnatal Year
Not all classes of principal neurons in the prefrontal cortex undergo intensive dendritic growth during the prenatal and perinatal period as observed for large pyramidal neurons impregnated with the rapid Golgi method. Subpopulations of pyramidal neurons impregnated by the Golgi Cox method (197, 207) undergo a major dendritic growth after birth, mainly during the second half of the first postnatal year (Figure 5). Different modification of Golgi methods have a selective affinity to stain different neuronal subpopulations, i.e., the rapid Golgi method is more prone to impregnate large pyramidal cells. These differences in timing of intensive dendritic growth between different subclasses of principal neurons (247, 248) suggest that there is a different gradient of maturation for different subclasses of neurons, even within the same layer.
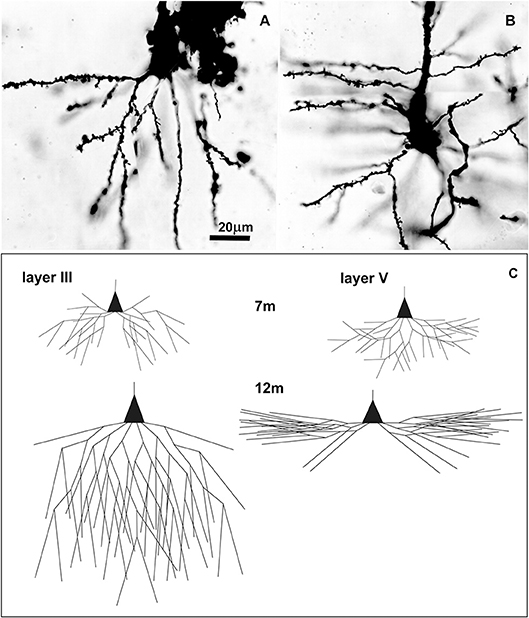
Figure 5. Golgi Cox impregnated pyramidal neurons in human Brodmann area 9 at 7 postnatal months and changes in basal dendritic tree between 7 and 12 months. Microphotography of Golgi Cox impregnated large pyramidal neurons in deep layer III (A) and layer V (B) at 7 postnatal months at higher magnification. Schematic drawings (C) indicating changes in length and complexity of dendritic tree of Golgi-Cox impregnated neurons between 7 and 12 months, showing that major dendritic growth for those two populations of neurons, occurred during the second half of the first postnatal year. A total number of segments is approximated on the basis of neuronal reconstructions (197), and percentage of segments cut at a particular dendritic order (198).
In our recent work, by using rapid Golgi method and encompassing broader population of the layer III impregnated principal neurons (Figure 6), we showed significant differences in the level of dendritic differentiation during the first postnatal month within frontal lobe, that includes dorsolateral part and Broca's region as well as primary motor and premotor cortices (249). The populations of L3N attained a highly developed dendritic tree in all analyzed areas, whereas dendrites of other principal neurons in layer III were less differentiated. Such findings show an asynchronous maturation of different microcircuitries throughout the cortico-cortical network: some of them reach functional level soon after birth, while others are still very immature (47, 250–252). This is in contrast to the traditional view of hierarchical neuronal development across the cerebral cortex, which suggests a sequential gradient of maturation from lower to higher order areas. We propose that there is a sequential maturation of distinct elements, forming cortico-cortical circuitries across all frontal areas (88). Such a pattern of development may represent a neurobiological basis for the sequential development of cognitive functions during the first and second postnatal year (124, 235, 253–256). Also, rapid dendritic growth is related with maturity of glutamatergic and GABA-ergic receptors (257, 258), making dendritic differentiation more prone to environmental influences. As such, for the development of early maturing neurons, as is the case for L3N, already during the perinatal and early postnatal period, the environment has an important role in regulating their morphological differentiation (259–263).
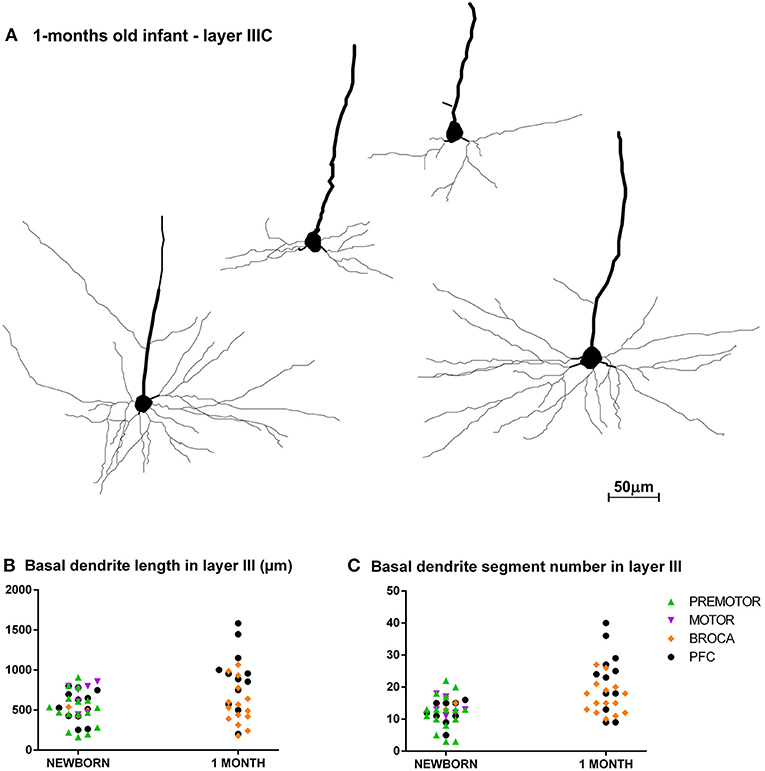
Figure 6. Asynchronous maturation of pyramidal neurons in layer IIIC during first postnatal month. (A) Rapid Golgi impregnated deep layer III pyramidal neurons in Brodmann area 9 of a 1-month-old infant in the prefrontal cortex show different stages of differentiation. Quantitative analysis of newborn and 1-month-old infant reveals large differences in total length (B), and number of segments (C) in basal dendritic tree of deep layer III pyramidal neurons, across different areas of the human frontal cortex. Each symbol represents mean values per individual neurons.
Selective Maturation of the Associative Large Layer IIIC Neurons During Early Childhood And Protracted Development of “Cognitive” Microcircuitry Through Adolescence
Unique Pattern of Dendritic Growth of Associative Neurons in Human Prefrontal Cortex
For most subpopulations of principal neurons in the prefrontal (197, 207, 208, 218, 219, 221, 264, 265), and other regions of the human cerebral cortex (266–276) major postnatal dendritic growth occurs during the first year in parallel with massive synaptogenesis (185, 205, 206, 226, 277–284).
An important exception from the typical temporal pattern of dendritic growth (see previous chapter) are associative L3N in the prefrontal cortex (Figures 7, 8). The L3N do not undergo the typical third stage of dendritic development like large layer V pyramidal neurons (55, 56). The layer V neurons attain more than 80% of their adult dendritic length by the third postnatal month. They then continue with further elongation during the third stage of dendritic development for roughly 1 year, and reach adult values around 15 months of age. In contrast, by the third postnatal month dendritic size of the L3N has reached only half of their adult values (Figures 7A–C, 8A,C,D). In addition, basal and oblique dendrites of the L3N have no significant growth (“dormant” period) until the middle of the second year (Figures 7A,C,D, 8A,D,E). Between 16 months and 2.5 years, length of L3N basal and oblique dendrites almost doubled, with growth rate higher than expected for the third stage (Figures 7A,D,E, 8A,E,F). To the best of our knowledge, this second L3N dendritic growth spurt represents an undescribed developmental feature for any class of cortical neurons. In the following period from 2 to 5 years, there is a further increase in synaptic spine density (285) at L3N dendrites, accompanied with molecular changes of this class of neurons. The L3N start to express strong AChE (78, 79) and SMI-32 (82, 265, 286) reactivity in their bodies and proximal apical and basal dendrites. This unique expression sets them apart from other classes of cortical neurons (Figure 9). Additionally, by the age of 5, the L3N show intensive Nissl staining paralleling transient somatic overgrowth (Figure 8B) (287–290).
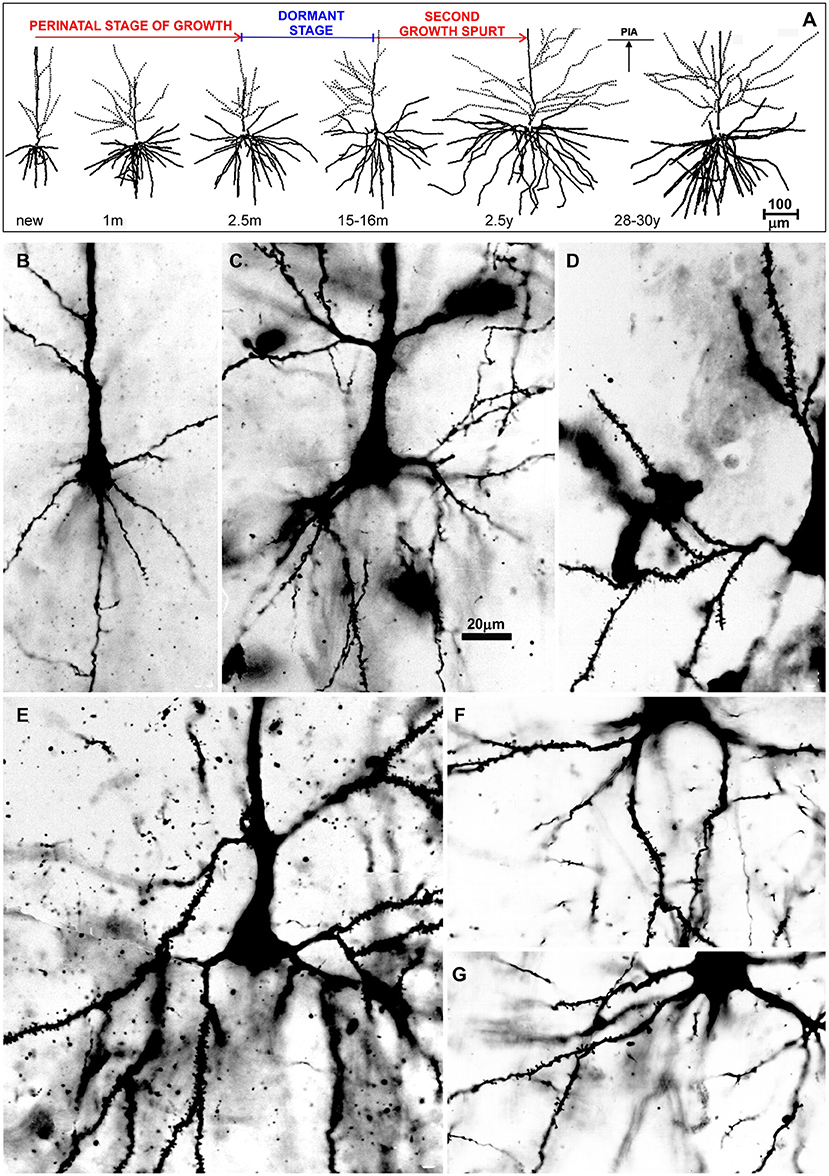
Figure 7. Postnatal development of rapid Golgi impregnated large layer IIIC pyramidal neurons in the magnopyramidal area 9 of the human prefrontal cortex. Three-dimensional reconstructions of basal and apical dendritic trees of rapid Golgi impregnated pyramidal cells in layer IIIC, projected onto the coronal plane (A). Orientation toward the pia is indicated by the arrow. Oblique dendrites originate from the apical dendrite, and are represented by dashed lines. All layer IIIC pyramidal cells are represented at the same magnification (scale bar 100 μm) and at the following ages: newborn, 1-month-old, 2.5-months-old, 15-months-old infants, 2.5-year-old child, and 28-year-old adult. Dendritic trees of layer IIIC pyramidal cells increased between 16 months and 2.5 years of age. Note that there are no obvious differences between layer IIIC pyramidal cells of 2.5-month-old and 16-month-old infants (dormant stage), as well as 2.5-year-old and 28-year-old subjects. Microphotographs showing changes in morphology of rapid Golgi impregnated layer IIIC pyramidal cells of the Brodmann area 9 between: newborn (B), infants aged 1 (C) and 16 (D) months, 2.5-year-old child (E), 19-year-old (F), and 73-year-old (G) adults (the magnification is same for all microphotographs; scale bar –20 μm). Even in these high-power microphotographs, the increase in dendritic complexity (an outgrowth of new segments) between newborn (B), and 1-month-old infant (C) is obvious. The figure is taken from Petanjek et al. (55) with permission.
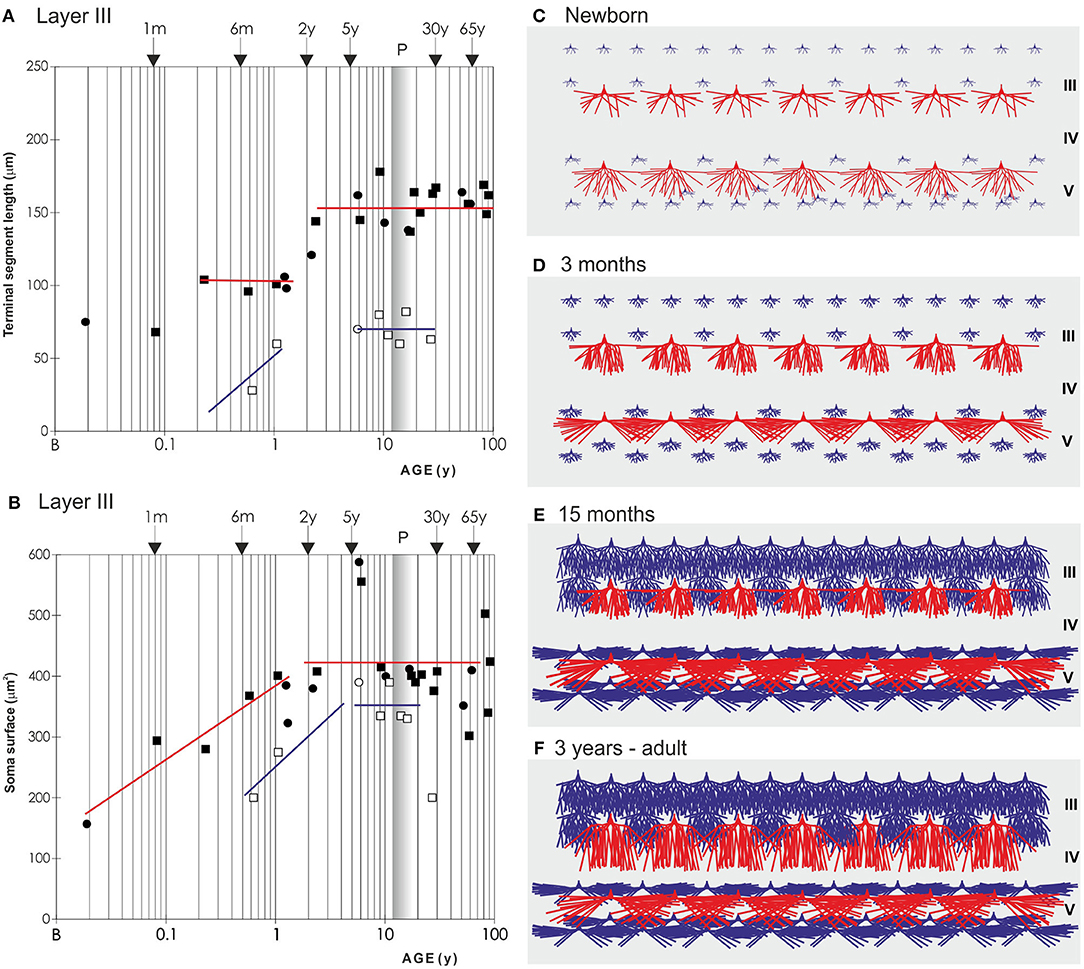
Figure 8. Graphical and schematic presentation of quantitative morphological data from basal dendritic tree of deep layer III and layer V pyramidal neurons, in magnopyramidal area 9 of the human dorsolateral prefrontal cortex impregnated by the Golgi method during postnatal development. Changes in terminal segment length (A) and soma size (B), from birth until 91 years of age on layer III pyramidal neurons impregnated with rapid Golgi (full marks—red line), and Golgi Cox method (open marks—blue line). Data about terminal segment length (A) are shown here as they participate with 90% in the total length of dendrites. Data from rapid Golgi stained slides, regarding terminal segment length confirm, that the period between 3 and 16 months, is a dormant stage in basal dendritic growth for layer III pyramidal neurons, and that further growth occurs between 16 months and 2.5 years (at least 1/3 of total length). Large temporal overgrowth in cell body size was present in subjects 5 to 6 years old (B). Data shown here were extrapolated from Koenderink et al. (197) and Petanjek et al. (55) studies. Squares represent males and circles represent females. The age is shown in postnatal years on a logarithmic scale. Puberty is marked by a shaded bar. Schematic drawings (C–F) of changes in length and complexity of dendritic tree of deep layer III and layer V pyramidal neurons, illustrate changes occurring in the cytoarchitecture and overall neuronal morphology. Total number of segments is estimated on neuronal reconstructions (based on real data) (55, 197) and values of missing dendrites were calculated by formula (198). Red represents rapid-Golgi impregnated neurons, and blue Golgi Cox impregnated pyramidal neurons. Illustrations for Golgi Cox neurons at newborn (C) and 3-months-old infant (D) stage are prediction based on dendrite growth pattern in the period 7–15 months. By the 15 months (E) most of the neurons have achieved an adult level of dendritic size, except large layer IIIC pyramidal cells impregnated by the rapid Golgi method (F).
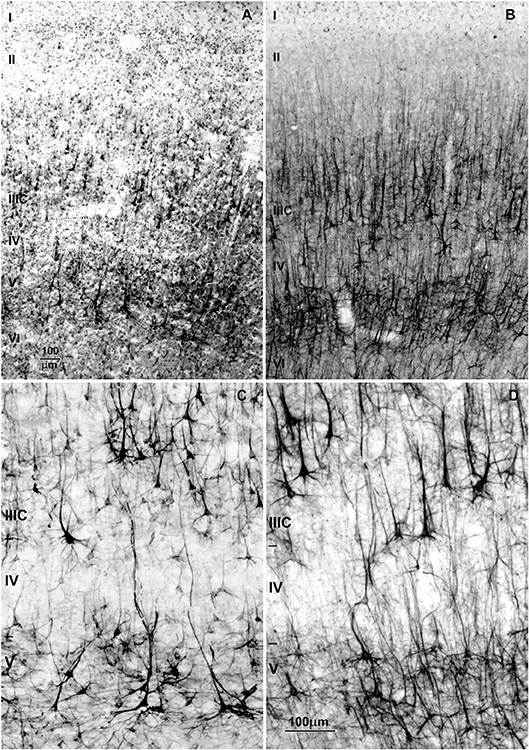
Figure 9. Neurofilament staining (SMI32) in Brodmann area 9 of child and adult human cortex. Microphotography of SMI32 (non-phosphorylated filament H) stained sections in Brodmann area 9 of the human prefrontal cortex at the age of 3 (A,C) and 38 years (B,D). The deep part of layer III and layer V are sub-laminas most densely populated with reactive neurons. There are no differences in staining intensity of layer V neurons at both ages, however in the adult subject layer III, the amount of intensely stained pyramidal neurons with clearly visible dendrites has increased. Accumulation of neurofilaments in dendrites is corresponding to the length and complexity of axon, suggesting that pyramidal neurons located deeper in layer III continue with axon growth after the age of 3. Scale bar at 100 μm (A–D).
Thus, the L3N acquire a significant portion of their maturity after infancy. We suggest that morphological and molecular changes on dendrites of the L3N in the period from 2 to 6 years are related to the growth and synaptogenesis of their own local intracortical projections, which establish very dense innervation on all cortico-cortical projecting neurons (62–64). Thus, changes in L3N intracortical projections will affect function of all prefrontal cortex neurons that project to other cortical areas. Consequently, L3N changes will be reflected on network processing throughout the whole cerebral cortex. Intracortical projections in experimental studies on rhesus monkey were found to be the last maturing part of the cortical excitatory network (209), whereas basic architecture for cortico-cortical projections is established earlier during infancy (41, 124, 243, 291–294). This leads us to conclude that large scale functional changes in the cerebral cortex, starting around the age of 2, are related with maturation of excitatory intracortical connections. Still, further maturation of cortico-cortical projections could not be excluded (116).
Protracted and Environmentally Driven Synaptic Pruning of Associative Microcircuitries
Structural changes through the cortical network are not finished by the age of 5–6 years, while the circuitry reorganization continues throughout the rest of childhood and adolescence (28, 33, 36, 76, 90, 205, 216, 283, 295–317). Molecular tuning of synaptic strength during development, when synaptic numbers exceed adult values, is proposed to be a major mechanism for the environmental effect on circuitry reorganization. The period of overproduction and elimination of supernumerary synaptic spines corresponds to the developmental stage when principal neurons have the highest magnitude of plasticity (185, 201, 260, 277, 304, 318–326). In the prefrontal cortex, the stage of developmental plasticity is highly prolonged and extends even up to the third decade of life (Figure 10). Concomitantly, there is a prolonged peak in expression of genes regulating neuronal development, including those associated with schizophrenia (298, 307, 327, 328). The comparative analysis of mRNA expression in the prefrontal cortex shows that in the human brain, relative to non-human primates, the dramatic changes in transcriptome profiles are delayed (283, 304, 307, 329, 330). So, extraordinary protracted circuitry reorganization is a specific feature of human higher-order associative areas.
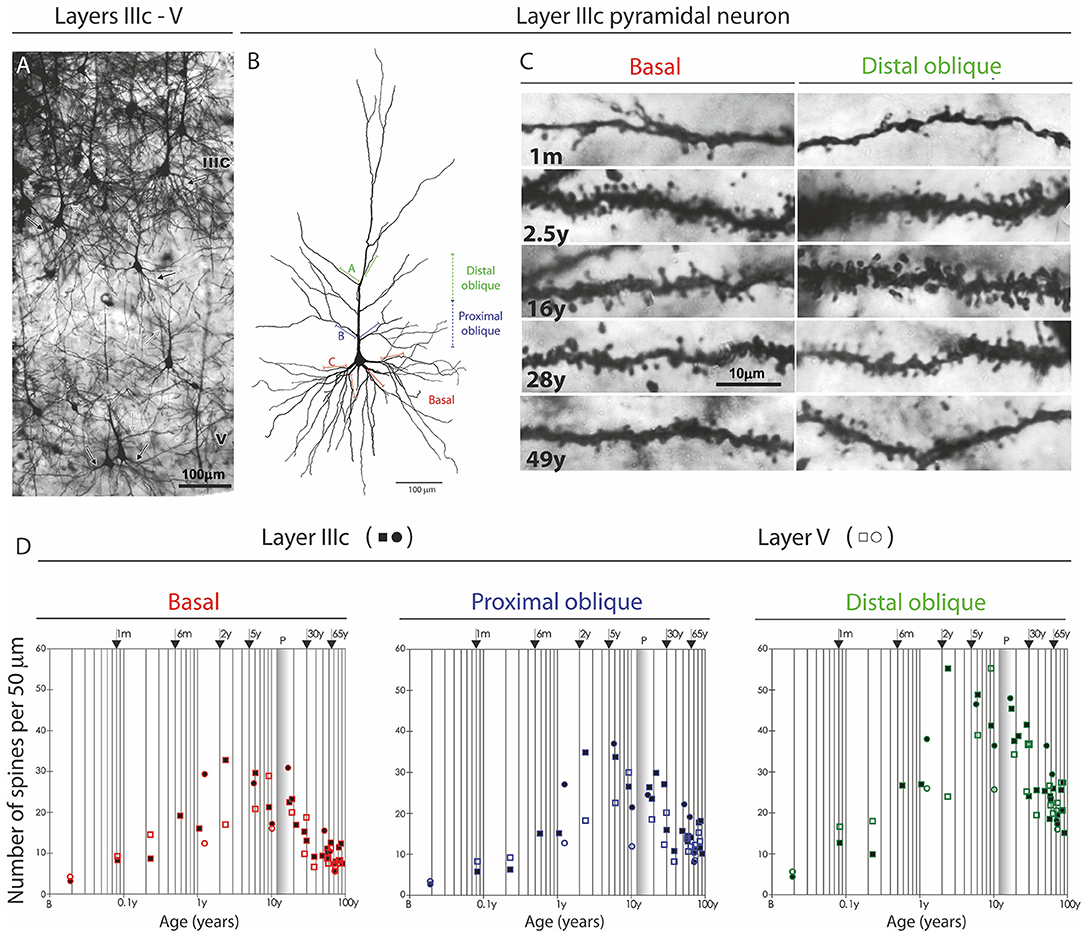
Figure 10. Changes in dendritic synaptic spine density on rapid Golgi impregnated large layer IIIC and layer V pyramidal neurons in magnopyramidal area 9 of the human prefrontal cortex from birth until 86 years of age. (A) Representative low magnification photographs of the rapid Golgi-impregnated layer IIIC and V pyramidal cells in the dorsolateral prefrontal cortex of a 16-year-old subject. (B) Neurolucida reconstruction of layer IIIC pyramidal neuron of a 49-year-old subject, illustrating dendrites selected for counting spines. (C) Representative high power magnification images of layer IIIC pyramidal neuron dendrites. (D) Graphs represent changes in spine numbers per 50 μm of dendritic length. The age is presented in postnatal years on a logarithmic scale. Puberty is marked by a shaded bar. Squares represent males, circles females. P, puberty; B, birth (fourth postnatal day); m, months; y, years. Figure published by Petanjek et al. (285).
It is possible however, that distinct types of microcircuitries may have different rates of synaptic formation and elimination. The pruning of supernumerary dendritic spines during the third decade of life is most pronounced and protracted on the L3N. It starts earlier on segments that are targeted by thalamo-cortical, rather than on those targeted by cortico-cortical projections (285). Studies obtained in monkeys and humans show somewhat higher synaptic overproduction in supragranular (including L3N and cortico-cortical projecting neurons) vs. infragranular (including layer V and subcortical projecting neurons) layers (205, 226). Regional differences in the number of grown and pruned spines on the layer III neurons have been described in the monkey and human cortex, with highest spine overproduction in the prefrontal cortex and lowest in the primary sensory regions (208, 221, 250, 279, 282, 331). The level and duration of synaptic overproduction, and consequently the level and duration of developmental plasticity (332), increases within increasing functional hierarchy of distinct microcircuits. Thus, microcircuits that are processing the highest cognitive functions, such as social abilities, are subject to the highest developmental remodeling induced by psycho-social and emotional environment (32, 39, 250, 252, 260, 305, 333, 334).
The Development of Prefrontal Cortex Associative Neurons in Autism: a Model of Selective Neuronal Vulnerability in Global Cortical Network Disconnectivity
Based on the above, we suggest that selective disruption of L3N could cause global cortical network disconnectivity, underlying ASD cognitive symptomatology. The protracted and biphasic pattern of L3N dendritic growth, coupled to the intensive molecular maturation after infancy, is not described for any other population of principal neurons. This developmental timing overlaps with the period when specific ASD symptomatology becomes evident.
Thus, alteration of the specific neuronal population with “strategic” position inside cortical circuitry, like L3N, could lead to global cortical network dysfunction. The timing of appearance and severity of symptoms in ASD might depend on the affected level of structural and molecular maturation of associative neurons during early childhood (2–6 years). For example, if development of the L3N is affected during the second year of life, it is most likely that the elongation of dendrites would be altered. This possible reduction in dendritic size would result in abnormal input on the L3N, and would change their functional properties. Consequently, as the L3N densely innervate all layer II/III cortico-cortical neurons, a robust alteration in dendritic morphology and consequently possible out-growth of intracortical projections of L3N, would lead to disrupted inter-columnar processing (335). We would expect such changes in subjects with a more serious form of ASD, where cognitive pathology already emerged during the second year of life.
In some ASD cases, specific cognitive symptoms are not apparent during the second, and even third year of life. Those subjects only show a subtle deflection of ToM, with no signs of any other neurological or psychiatric comorbidity, like in Asperger's syndrome (122, 167, 168). We hypothesize that in these forms of disorder the L3N alterations are not as robust. Here, the development of the L3N is probably affected later, after the age of 2.5 years, when further molecular maturation of the L3N occurs. Cognitive pathology is in such cases related to synaptic network architecture or/and fine molecular deflection at the level of individual synapse, without any dendritic and axonal changes that can be defined as pathological.
However, complex cognitive processing is disturbed in most cases of ASD at earlier stages of development (336, 337). Neurological and cognitive pathology is frequently present during the first year, sometimes even at birth (338, 339). In the most serious cases, there is ASD comorbidity with the intellectual developmental disorder (340) or/and epilepsy (341). In cases with absent comorbidity, many parents report a concern about socio-emotional interactions during the first year of life (342–345). Neuroimaging further revealed network inefficiencies during the first year of life (337, 346, 347). However, although specific ASD symptoms are not seen before the age of two, epidemiological and genetic studies support the idea, that alterations of neuronal development occur during the prenatal or perinatal time (348–355).
Thus, the “uniquely biphasic” pattern of dendritic growth of the L3N, with delayed structural and molecular maturation during the post-infant period (after 1.5 years), indicates that this neuronal class is particularly vulnerable during the perinatal period, when harmful events related to ASD are expected to occur. Pathological alterations induced at that stage may not be severe enough to affect functional demands of the L3N at the early postnatal period. However, the perinatal lesion could manifest after the second year, since the role of L3N, in now much complex microcircuitries, becomes more demanding at this time. In this view, the earlier “perinatal lesion” becomes visible as notable functional impairments after the second year (327, 356).
Most ASD cases will be diagnosed earliest by the age of 18 months (103). However, social cognition is often altered earlier, even in infants that do not show neither neurological, nor intellectual impairment. Retrospective studies have shown that ASD affected children clearly have different behavior related to social responses by 12 months, like gazing in a single direction and the way how they respond to their name (357). On functional magnetic resonance imaging (fMRI) altered connectivity in ASD was found by the 6 months (127, 346) and EEG signal was found to be altered by the 3 months (358). Above mentioned behavioral and functional aberrations present at early stages of infancy (337), further suggest that ASD symptoms are related with structural alterations from the early postnatal period. Interestingly, EEG pathology of ASD is first observed in the left temporal electrode (T7), and the frontal lobe starts to differ between 15 and 18 months of age, which corresponds to the beginning of the second L3N growth spurt. The developmental (“biphasic”) pattern of L3N, with intensive perinatal dendritic growth (suggesting high vulnerability), and second growth spurt around the age of 2, corresponds to the timing of alterations in cortical functioning and appearance of symptoms in infants with ASD. Understanding relations between L3N development and functional changes of cortical activity is important in early detection of ASD and might help to develop algorithms as combination of functional imaging methods and focused behavioral testing.
Gene-Environment Interaction During Postnatal Development Affects Associative Circuitry Architecture And May Contribute to Autistic Traits
Architecture of the mature cortical network is determined through complex gene-environment interactions during intricate developmental steps (1, 260, 330, 359–365). Given the unimaginable number of possible interactions between genes and environment, there are large interindividual differences in the size of particular areas, and even in internal cytoarchiteconics, particularly within the prefrontal cortex (77, 366–368). Large interindividual differences were found among the dendritic structure of cortico-cortical projecting neurons in high associative areas of the human brain, correlating with the level of education (369). All mentioned interindividual differences point to a strong environmental impact on cortical development.
The mechanism of developmental plasticity leading to interindividual differences in cytoarchitectonics and neuron morphology is related to synaptic overproduction. By activity-dependent molecular tuning of synaptic spines, it is determined which synapse will remain and which will be removed from the network during the pruning process (370, 371). This model is defined as selective stabilization hypothesis (318) and proposes that through synaptic tuning the environment is shaping the architecture of the neural network. The highest degree and longest period of synaptic overproduction are so far described for the L3N in the prefrontal cortex, particularly at dendritic compartments (distal side branches of apical dendrite) targeted by cortico-cortical and intra-cortical projections (285). Altogether, psychological, educational, social and emotional milieu has a predominant influence in reshaping circuitries which are involved in processing the most complex cognitive functions (29, 76, 226, 309, 332, 372–379).
Taken together, these findings suggest that human-specific cognitive functions and circuitry specializations (1) have foundation in interactions between genes (25, 380–382) and environment (242, 363, 383, 384) during the period of synaptic overproduction and pruning. In humans, the period of synaptic overproduction and pruning is the period of the highest magnitude of acquisitions of new knowledge. It might look paradoxical that this occurs with a decrease in the number of synapses, but the final functional outcome of pruning leads to increased connectivity of the cortical network. Therefore, this process allows humans to acquire the highest level of cognition, but it also prolongs the vulnerability period, increasing the chance for the formation of abnormal circuitry (296, 306, 327, 364, 385–387).
Such developmental reshaping could be an important factor in the development of ASD, particularly in subjects with a mild form of the autism spectrum phenotype. Recognition that ToM deficit is a core psycho-pathological substrate of ASD, has allowed better diagnosing of both patients with mild impairment and individuals with atypical symptoms or personality traits associated with ASD, which fall under the broad autism phenotype (388–390). It is possible that the “pathological” substrate can be found only in the circuitry architecture, without any structural or molecular impairment of neurons and their pathways. In line with this possibility is a higher incidence of ASD in school rather than preschool children (391), suggesting that in some cases, “autistic” circuitry architecture is established through late childhood, or even adolescence (103, 392, 393). So, the broad or subthreshold autism spectrum conditions, could be used as a model to understand trajectories of “nature-nurture” interactions, guiding neurodevelopment toward, or away from ASD.
We propose that in such individuals, the emotional and psycho-social environment during infancy and childhood is crucial for the appearance of ASD or autistic traits (387, 394, 395). Vice versa, in individuals with genetic backgrounds to develop ASD or autistic traits, this opens a huge window of opportunity for cognitive rehabilitation, particularly considering the highly extended period of circuitry reorganization in the human prefrontal cortex. At an epidemiological level, mild and atypical cases should be far more numerous then serious cases of ASD, and are therefore of much higher societal impact. Second, enhanced emotional and psychological interaction in infants and children, have been shown to have a stimulating effect on the development of ToM (396, 397), suggesting that those with mild symptomatology and subtle alterations in circuitry organization have a greater chance for a positive outcome of early intervention (398).
Overview of Reported Cortical Neuronal Pathology in Autism
Despite pathological changes observed in the cerebellum, amygdala and brainstem (399–402), imaging data and studies on post-mortem material are implying that cerebral cortex circuitries are the most plausible candidate to produce core deficits of autism. However, specific cortical neuronal alterations are yet to be described and present data are often contradictory. Some ASD cases have reduced neuronal cell body size but increased number of minicolumns and increased neuronal density (403, 404). These findings suggest an increased number of neurons in frontal, temporal and parietal regions of ASD cortices (405, 406). In other ASD cases, neuron numbers and density were unchanged (407), while some have a reduction in the number of neurons (408). Cellular patches were found in prefrontal and temporal cortices of ASD patients, while again no change in in neuron density was described (409).
Cell body size was unaltered in the dorsolateral medial prefrontal cortex (405), superior temporal gyrus (407), anterior cingulate cortex (410), hippocampus (411), and amygdala (412). Smaller cell size was found in cortical regions with identified minicolumnar pathologies in ASD, i.e., frontal regions (403, 406, 413), as well as primary motor, sensory and visual cortices (414). In the fusiform gyrus (415), hippocampus (416) and portions of the anterior cingulate cortex (417, 418), smaller neuron cell bodies of varying types have also been reported. These changes in cell body size are considered to be present in preadolescent stages between ASD and controls, and this effect becomes diminished later on (400, 410). Importantly, changes in cell body size are usually related with changes in dendritic morphology. However, Golgi studies on ASD neocortices, showed that there is no dendritic pathology in neocortical pyramidal and non-pyramidal neurons (411, 419), but increased density of dendritic spines was found in layer II of temporal, parietal and frontal region (420). Higher spine density suggests impaired synaptic pruning, and is correlated to decreased brain weight and lower levels of cognitive functioning in ASD (164, 421–424).
Reduction in neuronal size and loss of neurons in ASD suggests a bias in connectional abnormalities present in multiple areas of the association cortex, specifically within layers that are involved in long-range connectivity (406, 414). The alteration of neuronal classes essential to these circuitries is expected to be the main correlate of altered cognitive processing. In line with that, it was suggested that the total number of a special neuronal subtypes found only in species with highly developed social cognition, von Economo spindle cells, is decreased in autism, but stereological analysis in the frontal part of the insula could not confirm that assumption (425).
Based on the level of gene expression, a reduced number of distinct cell subtypes in layers IV and V, the calbindin and parvalbumin neurons, was suggested (426, 427). So far the only neuron-specific pathology documented histologically in ASD is a decreased number of parvalbumin interneurons in medial prefrontal cortex (428). Parvalbumin expressing cortical neurons provide inhibitory input to cortico-cortical projecting principal cells (429–431). The temporal pattern of change in axon terminals of parvalbumin interneurons parallels the changes in dendritic spine density on layer III principal cells (206, 432). The chandelier subpopulation of parvalbumin neurons, which is projecting to axon initial segment of principal neurons, is found to be affected in prefrontal cortex of ASD subjects (433). Therefore, decreased number of parvalbumin neurons in ASD may be related to alterations of postnatal refinements in cortical circuitry related with associative pyramidal neurons.
In conclusion, despite no direct evidence of L3N pathology in ASD being found, already mentioned findings that in ASD there is a higher spine density in layer II (420), could suggest an altered synaptic pruning of projections arising from associative L3N.
Disclosure of Pre-Existing Lesion Through Late Maturation of Associative Neurons in Autism
In this manuscript we present an interesting observation about neuron pathology of an ASD case from Zagreb's Neuroembryological Collection (434–436), and evaluate the possibility that appearance of ASD symptoms is correlated with maturation of associative L3N during early childhood, but without disruption of their development.
We performed an in depth analysis of brain tissue from a 23-year-old female with ASD (based on DSM-III-R criteria) (437), with mild intellectual disability and epilepsy. We did not observe changes in the brain's gross morphology, cytoarchitectonic structure, nor expression of non-phosphorylated-neurofilament H (SMI32) which is highly expressed in L3N (Figure 11). Unchanged intensity of neurofilament staining (81, 438) suggests normal axonal development of associative neurons. On rapid Golgi impregnated sections of prefrontal cortex (Brodmann area 9), primary motor cortex (Brodmann area 4) and primary visual cortex (Brodmann area 17), we did not observe changes in dendritic size or in spine density of L3N or other classes of principal neurons. However, a small fraction of neurons in the layer II and upper part of layer III in the analyzed areas exhibited abnormalities of dendritic morphology (Figure 12).
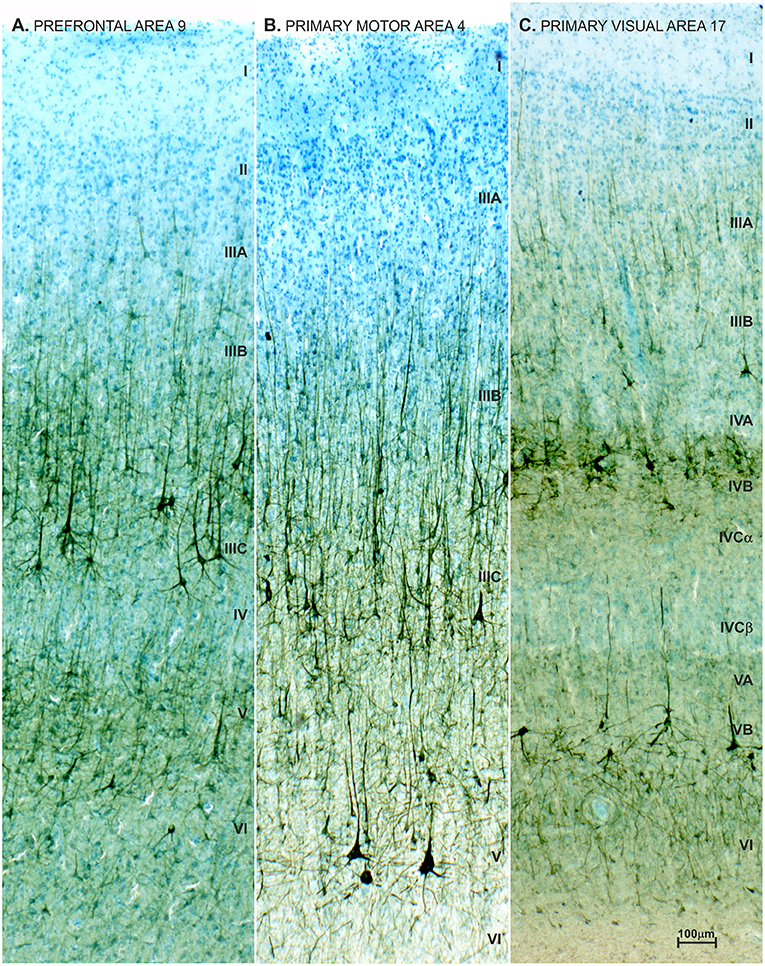
Figure 11. Neurofilament staining (SMI32) in neocortex of adult female with autism. Pattern of SMI32 immunostaining counterstained with Giemsa in the prefrontal area 9 (A), primary motor area 4 (B) and primary visual area 17 (C), in a 21-year-old autistic female with a comorbidity in the form of epilepsy and intellectual disability (according to DSMIII classification). The analyzed material is a part of Zagreb's Neuroembryiological Collection (434–436). The distribution, density and level of neurofilament (SMI32) expression did not differ from normative control (81, 438), and no obvious disruption of cytoarchitecture was observed (68, 266). SMI32 stained sections were compared to normative control specimens obtained from the Zagreb Neuroembryological Collection, which includes 29 specimens, with an age span of 19 to 57 years.
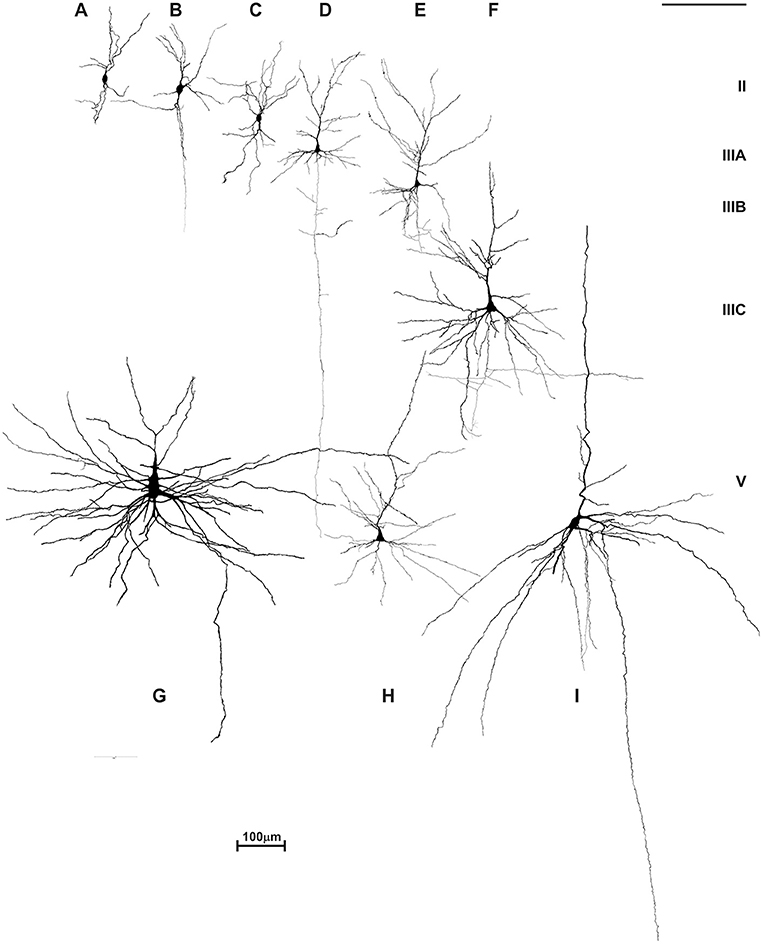
Figure 12. Reconstructions of rapid Golgi impregnated principal neurons in the neocortex of an adult female with autism. Neurons are marked in order of their laminar position: atypical pyramid-like cells (A–C) and typical pyramidal cells in analyzed neocortices were shown: layer II (D), layer IIIA/B pyramidal cells (E), layer IIIC pyramidal cells in prefrontal cortex (F), giant Betz pyramidal cells in layer V of motor cortex (G), small pyramidal cells of layer V (H) and large Meynert pyramidal cells of layer V/VI in area striata (I). Atypical pyramid-like cells (A–C) were mainly located in the layer II, and some in the layer IIIA, but not in any other layers. They have oval cell bodies with bipolar orientation of dendrites, low spine density, and axons directed toward white matter. Such cell types were not observed in Golgi sections of a normal, adult human cerebral cortex. Golgi sections from this case were compared to 29 rapid Golgi stained specimens, with an age span of 19 to 57 years. No other qualitative signs of dendritic, nor spine pathology and density could be found on rapid Golgi slices, and morphology, cell body size, dendritic extent, and complexity (D–I) corresponded to pyramidal neurons of the same laminar position in an aged-matched controls.
Alongside well developed and regularly oriented principal neurons, a subset of neurons in layers II/IIIA had spiny dendrites, whose morphology resembled those of immature principal cells (Figure 13A). Such dendritic morphology with low spine density is characteristic for developing principal neurons at initial stages of their dendritic differentiation. Developing neurons with similar morphology are found in the neocortex of healthy neonates (Figure 13B), but not later on. Since only part of the neurons had the immature dendritic morphology (Figures 13C,D) (204, 439, 440), we concluded that in the analyzed subject a selective fraction of cortico-cortically projecting neurons is affected. In particular, layer II and upper part of layer III contain cortico-cortical neurons that have relatively short axons, and participated in local microcircuits between neighboring areas (44, 74, 441–445).
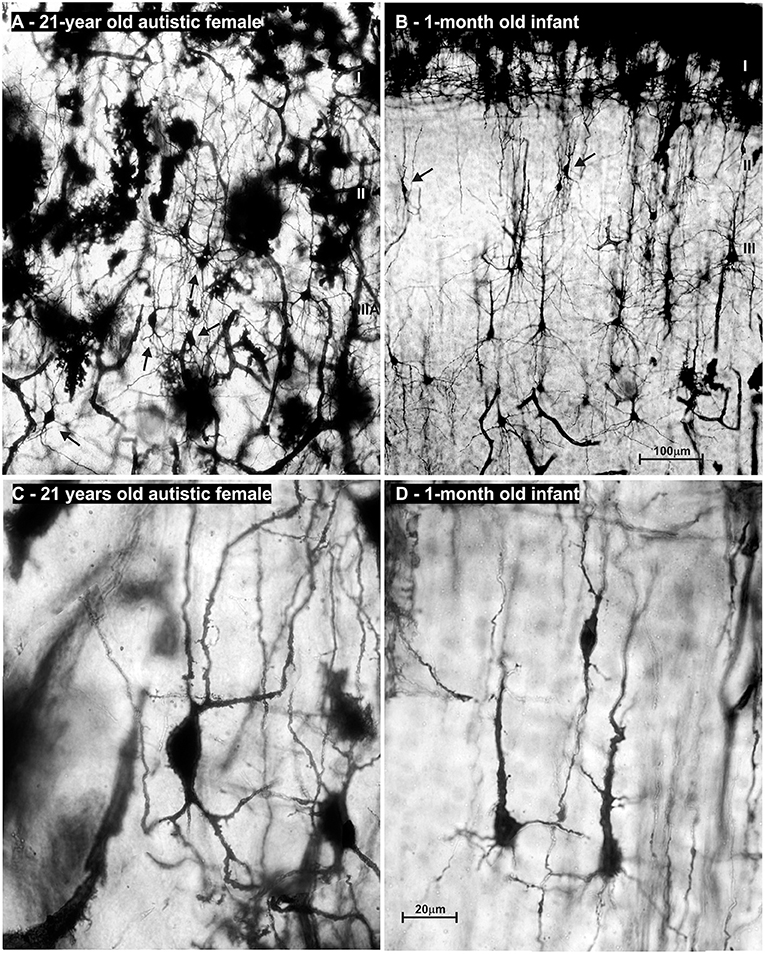
Figure 13. Microphotography of rapid Golgi sections in the prefrontal cortex of an adult female with autism, compared to a normal 1-month-old infant. On low power microphotography of layer II/IIIA in the prefrontal cortex of a 21-year-old autistic female, numerous bipolar—pyramid-like cells were found (A). In the prefrontal cortex of a normal 1-month old infant (B), numerous neurons with similar morphology were found in the same position (arrows). High power microphotography shows layer II bipolar-pyramid like cells in the analyzed subject (C). Note that dendrites had spines, but their density was low. Upper dendrites were directed to the layer I, resembling apical dendrite morphology. On the other pole, two dendrites resembled basal dendrite morphology. An axon arose from one of those dendrites and was directed to the white matter. In the prefrontal cortex of a newborn infant, immature pyramidal neurons with similar morphology are found (D). In the adult autistic subject neuron morphology of spiny bipolar neurons regarding cell body shape, and dendritic complexity is similar only larger when compared to healthy neonate. The presented material is a part of Zagreb's Neuroembryological Collection, which also includes normative control specimens of 31 rapid Golgi specimens, lifespan from infant to adolescent. Scale bar indicates 100 μm (A,B) and 20 μm (C,D).
Thus, their abnormal development in ASD may be a result of harmful events (including those induced genetically) occurring during the perinatal period. In this manner, development of microcircuitries established by short cortico-cortical neurons would be stalled at the neonatal stage. Importantly, this neuron class is not expected to go through distinct structural complexification after the first year. Therefore, we hypothesed that they will achieve adult structure by the age of 1, but full functional capacity will be achieved through maturation of associative neurons and related circuitries during early childhood (2–6 years). By having subtle alterations of the short cortico-cortical network, first symptoms, in general, would not appear before final maturation of local intracortical connections which occur later during childhood. It means that development of L3N and their projections in ASD could take a fully regular course, but may trigger appearance of symptoms.
Neurodevelopmental model with early structural lesions and a delayed appearance of symptoms is already established for schizophrenia (385, 446). Typical schizophrenia symptoms occur predominantly during late adolescence or early adulthood. Such timing is linked to massive synaptic pruning in the prefrontal cortex that occurs as part of normal development. So, in schizophrenia the appearance of symptoms is not a result of disrupted development at that time (90, 99, 447, 448). In fact, events occurring through the course of regular development are a trigger which may cause an already present, but for a long time asymptomatic impairment, to become eminent. Direct evidence for such a hypothesis comes from patients with metachromatic leukodystrophy, a disorder characterized by demyelination present at birth. The lesion remains without exacerbation up until late adolescence, when a schizophrenia-like psychosis will emerge (449, 450). So, a fixed “lesion” from earlier in development has been silent for decades, and interacts with normal brain maturational events that manifest much later in life. Despite the causative process not being obvious, it is still present long before the symptoms appear and any diagnosis is made (327, 360, 451–454).
Development of Associative Neurons During Childhood and Relation to ASD Symptoms: Altered Development or Trigger For Pre-existing Lesion?
In this manuscript, we hypothesize that selective alteration of a specific subset of principal neurons could lead to global changes in cortical network connectivity. We applied this model to the ASD and social (pragmatic) communication disorders, which include disrupted social and communication functioning, with more or less severe global disconnectivity.
We propose that contrary to normally developing children (Figure 14A), there might be a disrupted development of inter-collumnar connectivity within the prefrontal cortex of ASD patients, as these microciruitries undergo intensive maturation in the period between 2 and 6 years when ASD manifests. Associative L3N, which are the main source of local excitatory cortico-cortical connections, and are thus responsible for inter-columnar connectivity, undergo intensive structural and molecular changes during the same time (Figure 14B). Disrupted maturation of intracortical connectivity may then consequently alter outputs from the prefrontal cortex. The severity of this pathology would depend on the extent and timing of disruption within those microcircuitries.
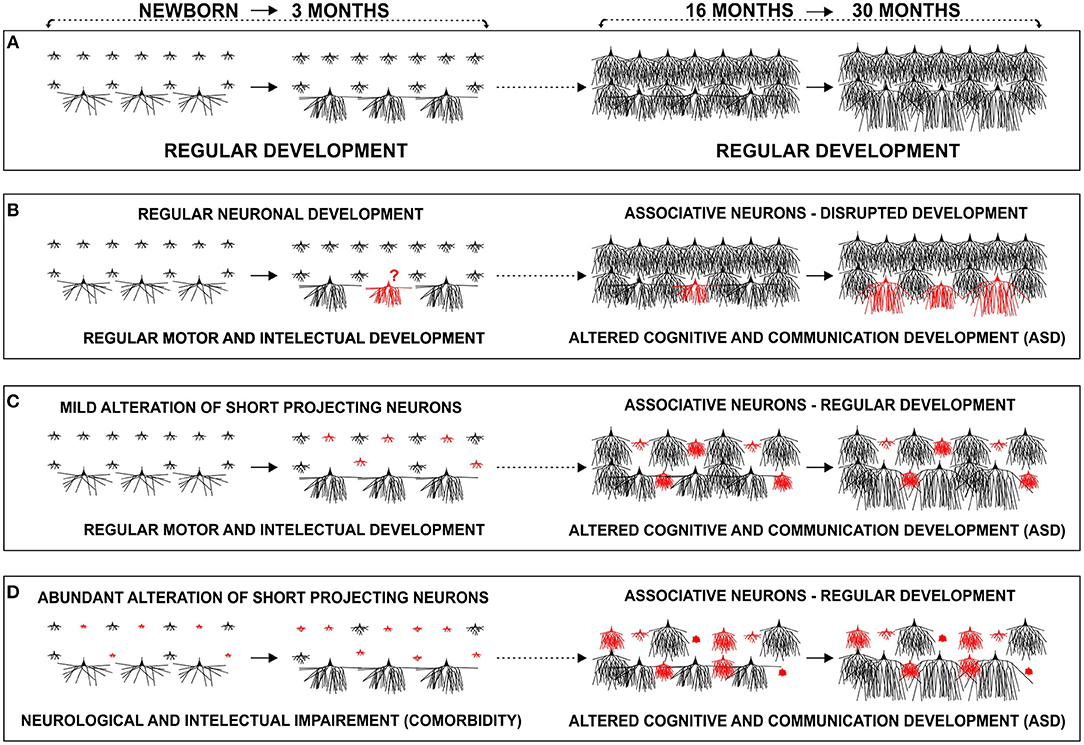
Figure 14. Proposed developmental models of cortico-cortical neuron alterations in autism: schematic representation of changes in dendritic morphology of layer III in the human prefrontal cortex during the early postnatal period (newborn–3 months) and around the second year (16–30 months). (A) In a typically developing infant, associative neurons undergo intensive dendritic differentiation during the first 3 postnatal months, whereas other classes of cortico-cortical projecting neurons, undergo major dendritic growth later during their first postnatal year. A late, second growth spurt, with large dendritic elongation in the period from 18–30 months, is so far described only in associative neurons. We propose that maturation of associative neurons during early childhood (1.5–6 years), plays a major role in global, functional changes of the cortical network, related with tremendous cognitive development. (B) Disrupted development of associative neurons (red), around age of 2, does not allow enhancement in inter-columnar and inter-areal connectivity, leading to cortical network impairment. Development of associative neurons does not have to be disrupted (C,D). There might be a preexisting (perinatal) alteration, in a subset of short cortico-cortical neurons (red) that would become evident around the age of 2, during typical maturation of intra-cortical connections established by associative neurons. (C) In the case of mild perinatal disruption of short cortico-cortical neurons, the lesion would remain functionally silent until late differentiation of associative neurons, whereas in (D) cases where alterations are more robust, comorbidities in form of neurological, and intellectual impairment, manifest earlier. We suggest that alteration can be present in various forms thus creating many phenotypes of ASD.
Lacking evidence for structural pathology of the L3N in ASD both in our reported case and overall in the literature, opens the possibility that differentiation of this neuron class takes a regular course. Thus, their developmental incorporation into maturing circuits during childhood will reveal a pre-existing (perinatal) lesion in other neuronal classes and microciruitries. Despite regular development of intracortical connections during the second year, and throughout the rest of early childhood, the cortical network will not be able to reach a new/higher level of information processing, as there is a pre-existing alteration in other classes of projection neurons, e.g., a subset of cortico-cortical neurons with short projections. We propose that in the case of a more subtle disruption of neurons, which are a source of short cortico-cortical circuitries, the lesion remains fully silent until the age of 2 (Figure 14C), whereas in the case of more robust alteration, neurological, and intellectual comorbidity may appear earlier (Figure 14D).
These two proposed models are also not mutually exclusive. Direct alteration of L3N or “disclosure” of pre-existing lesions on other neuronal classes during differentiation of associative neurons around the age of 2, could be present in different phenotypes of ASD, or even act at the same time (455, 456).
The important concept in understanding the mechanism of ASD is gene-environment interaction in shaping the architecture of the developing neuronal network (457, 458). The environmental factor may induce or prevent appearance of the ASD pathological functioning, like infection, malnutrition, toxins, or vascular insult (227, 354, 356, 459–462).
While not specifically recognized yet, structural and molecular alteration of mirocircuitry is clearly related with ASD, but in the subthreshold autism phenotype there might be “autistic architecture” of the cortical network, without evident structural or molecular pathology. It is intriguing that in such conditions, psychosocial ambience is exclusively related with appearance of autistic traits, particularly taking into consideration that associative and intracortical circuitries have the highest rate, and most protracted period of synaptic overproduction. Finally, the protracted period of highly plastic circuits involved in ASD pathology opens a new potential in rehabilitation strategies, particularly if early clinical detection approaches are applied (348, 351, 358, 463–472).
Ethics Statement
This study was carried out in accordance with Croatian legislation and the approval of the Ethical Committee School of Medicine, University of Zagreb (number: 380-59-10106-14-55/152; class 641-01/14-02/01; 1 July 2014).
Author Contributions
ZP, DS, and DD contributed equally to the work. ZP designed concept of research, performed Golgi experiments, analyzed Golgi and SMI32 sections, wrote the manuscript, prepared figures. DS analyzed Golgi and SMI32 sections, performed quantitative analysis of neuron morphology in the neonate, prepared figures, wrote and structured the manuscript, and the literature. DD analyzed Golgi and SMI32 sections, wrote and revised the manuscript, and the literature. AH interpretated the data, revised the manuscript. MRR reconstructed Golgi impregnated neurons, revised the manuscript. NJ-M analyzed Golgi and SMI32 sections, performed immunohistochemical experiments, revised manuscript. All authors read and approved the final version.
Funding
This work is supported by the Croatian Science Foundation grant no. 5943 (Microcircuitry of higher cognitive functions, PI: ZP), and work of AH was co-supported by grant no. 1245 (Biomarkers in schizophrenia-integration of complementary methods in longitudinal follow up of first episode psychosis patients). This publication was co-financed by the European Union through the European Regional Development Fund, Operational Programme Competitiveness and Cohesion, grant agreement No. KK.01.1.1.01.0007, CoRE—Neuro.
Conflict of Interest Statement
The authors declare that the research was conducted in the absence of any commercial or financial relationships that could be construed as a potential conflict of interest.
Acknowledgments
Authors would like to thank Tin Luka Petanjek for helpful comments while this manuscript was in the final stages of writing.
References
1. Rakic P. Evolution of the neocortex: a perspective from developmental biology. Nat Rev Neurosci. (2009) 10:724–35. doi: 10.1038/nrn2719
2. Rakic P, Ayoub AE, Breunig JJ, Dominguez MH. Decision by division: making cortical maps. Trends Neurosci. (2009) 32:291–301. doi: 10.1016/j.tins.2009.01.007
3. Defelipe J. The evolution of the brain, the human nature of cortical circuits, and intellectual creativity. Front Neuroanat. (2011) 5:29. doi: 10.3389/fnana.2011.00029
4. Defelipe J, Markram H, Rockland KS. The neocortical column. Front Neuroanat. (2012) 6:22. doi: 10.3389/fnana.2012.00022
5. Soligo C, Smaers JB. Contextualising primate origins - an ecomorphological framework. J Anat. (2016) 228:608–29. doi: 10.1111/joa.12441
6. Skulachev VP, Holtze S, Vyssokikh MY, Bakeeva LE, Skulachev MV, Markov AV, et al. Neoteny, prolongation of youth: from naked mole rats to “Naked Apes” (Humans). Phys Rev. (2017) 97:699–720. doi: 10.1152/physrev.00040.2015
7. Rosati AG, Wobber V, Hughes K, Santos LR. Comparative developmental psychology: how is human cognitive development unique? Evol Psychol. (2014) 12:448–73. doi: 10.1177/147470491401200211
8. Santos LR, Rosati AG. The evolutionary roots of human decision making. Annu Rev Psychol. (2015) 66:321–47. doi: 10.1146/annurev-psych-010814-015310
9. Semendeferi K. Why do we want to talk? Evolution of neural substrates of emotion and social cognition. Interact Stud. (2018) 19:102–20. doi: 10.1075/is.17046.sem
10. Krupenye C, Kano F, Hirata S, Call J, Tomasello M. Great apes anticipate that other individuals will act according to false beliefs. Science. (2016) 354:110–4. doi: 10.1126/science.aaf8110
11. Martin A, Santos LR. What cognitive representations support primate theory of mind? Trends Cogn Sci. (2016) 20:375–82. doi: 10.1016/j.tics.2016.03.005
12. Whiten A, Van De Waal E. Social learning, culture and the ‘socio-cultural brain’ of human and non-human primates. Neurosci Biobehav Rev. (2017) 82:58–75. doi: 10.1016/j.neubiorev.2016.12.018
13. Arguello PA, Gogos JA. Genetic and cognitive windows into circuit mechanisms of psychiatric disease. Trends Neurosci. (2012) 35:3–13. doi: 10.1016/j.tins.2011.11.007
14. Koenigs M. The role of prefrontal cortex in psychopathy. Rev Neurosci. (2012) 23:253–62. doi: 10.1515/revneuro-2012-0036
15. Hanson KL, Hrvoj-Mihic B, Semendeferi K. A dual comparative approach: integrating lines of evidence from human evolutionary neuroanatomy and neurodevelopmental disorders. Brain Behav Evolut. (2014) 84:135–55. doi: 10.1159/000365409
16. Brainstorm C, Anttila V, Bulik-Sullivan B, Finucane HK, Walters RK, Bras J, et al. Analysis of shared heritability in common disorders of the brain. Science. (2018) 360:eaap8757. doi: 10.1126/science.aap8757
17. Park MTM, Raznahan A, Shaw P, Gogtay N, Lerch JP, Chakravarty MM. Neuroanatomical phenotypes in mental illness: identifying convergent and divergent cortical phenotypes across autism, ADHD and schizophrenia. J Psychiatry Neurosci. (2018) 43:201–12. doi: 10.1503/jpn.170094
18. Carlson SM, Koenig MA, Harms MB. Theory of mind. Wiley Interdiscip Rev Cogn Sci. (2013) 4:391–402. doi: 10.1002/wcs.1232
19. Povinelli DJ, Preuss TM. Theory of mind: evolutionary history of a cognitive specialization. Trends Neurosci. (1995) 18:418–24. doi: 10.1016/0166-2236(95)93939-U
20. Schurz M, Perner J. An evaluation of neurocognitive models of theory of mind. Front Psychol. (2015) 6:1610. doi: 10.3389/fpsyg.2015.01610
21. Estes D, Bartsch K. Theory of mind: a foundational component of human general intelligence. Behav Brain Sci. (2017) 40:e201. doi: 10.1017/S0140525X16001618
22. Wobber V, Herrmann E, Hare B, Wrangham R, Tomasello M. Differences in the early cognitive development of children and great apes. Dev Psychobiol. (2014) 56:547–73. doi: 10.1002/dev.21125
23. Dobbing J, Sands J. Quantitative growth and development of human brain. Arch Dis Child. (1973) 48:757–67. doi: 10.1136/adc.48.10.757
24. Shi F, Yap PT, Wu G, Jia H, Gilmore JH, Lin W, et al. Infant brain atlases from neonates to 1- and 2-year-olds. PLoS ONE. (2011) 6:e18746. doi: 10.1371/journal.pone.0018746
25. Reardon PK, Seidlitz J, Vandekar S, Liu S, Patel R, Park MTM, et al. Normative brain size variation and brain shape diversity in humans. Science. (2018) 360:1222–7. doi: 10.1126/science.aar2578
26. Kuzawa CW, Chugani HT, Grossman LI, Lipovich L, Muzik O, Hof PR, et al. Metabolic costs and evolutionary implications of human brain development. Proc Natl Acade Sci USA. (2014) 111:13010–5. doi: 10.1073/pnas.1323099111
27. Mills KL, Lalonde F, Clasen LS, Giedd JN, Blakemore SJ. Developmental changes in the structure of the social brain in late childhood and adolescence. Soc Cogn Affect Neurosci. (2014) 9:123–31. doi: 10.1093/scan/nss113
28. Amlien IK, Fjell AM, Tamnes CK, Grydeland H, Krogsrud SK, Chaplin TA, et al. Organizing principles of human cortical development-thickness and area from 4 to 30 years: insights from comparative primate neuroanatomy. Cereb Cortex. (2016) 26:257–67. doi: 10.1093/cercor/bhu214
29. Johnson CM, Loucks FA, Peckler H, Thomas AW, Janak PH, Wilbrecht L. Long-range orbitofrontal and amygdala axons show divergent patterns of maturation in the frontal cortex across adolescence. Dev Cogn Neurosci. (2016) 18:113–20. doi: 10.1016/j.dcn.2016.01.005
30. Geng X, Li G, Lu Z, Gao W, Wang L, Shen D, et al. Structural and maturational covariance in early childhood brain development. Cereb Cortex. (2017) 27:1795–807. doi: 10.1093/cercor/bhw022
31. Vasa F, Seidlitz J, Romero-Garcia R, Whitaker KJ, Rosenthal G, Vertes PE, et al. Adolescent Tuning of Association Cortex in Human Structural Brain Networks. Cereb Cortex. (2018) 28:281–94. doi: 10.1093/cercor/bhx249
32. Semendeferi K, Teffer K, Buxhoeveden DP, Park MS, Bludau S, Amunts K, et al. Spatial organization of neurons in the frontal pole sets humans apart from great apes. Cereb Cortex. (2011) 21:1485–97. doi: 10.1093/cercor/bhq191
33. Charvet CJ, Finlay BL. Embracing covariation in brain evolution: large brains, extended development, and flexible primate social systems. Prog Brain Res. (2012) 195:71–87. doi: 10.1016/B978-0-444-53860-4.00004-0
34. Preuss TM. Human brain evolution: from gene discovery to phenotype discovery. Proc Natl Acad Sci USA. (2012) 109 (Suppl. 1):10709–16. doi: 10.1073/pnas.1201894109
35. Sherwood CC, Bauernfeind AL, Bianchi S, Raghanti MA, Hof PR. Human brain evolution writ large and small. Prog Brain Res. (2012) 195:237–54. doi: 10.1016/B978-0-444-53860-4.00011-8
36. Somel M, Liu X, Khaitovich P. Human brain evolution: transcripts, metabolites and their regulators. Nat Rev Neurosci. (2013) 14:112–27. doi: 10.1038/nrn3372
37. Kostović I, Judaš M. Embryonic and Fetal Development of the Human Cerebral Cortex. In: Toga AW, editor. Brain Mapping - An Encyclopedic Reference. London: Academic Press. (2015). p. 167–77.
38. Elston GN, Benavides-Piccione R, Elston A, Zietsch B, Defelipe J, Manger P, et al. Specializations of the granular prefrontal cortex of primates: implications for cognitive processing. Anat Rec A Discov Mol Cell Evol Biol. (2006) 288:26–35. doi: 10.1002/ar.a.20278
39. Shepherd GM. The microcircuit concept applied to cortical evolution: from three-layer to six-layer cortex. Front Neuroanat. (2011) 5:30. doi: 10.3389/fnana.2011.00030
40. Zaitsev AV, Povysheva NV, Gonzalez-Burgos G, Lewis DA. Electrophysiological classes of layer 2/3 pyramidal cells in monkey prefrontal cortex. J Neurophysiol. (2012) 108:595–609. doi: 10.1152/jn.00859.2011
41. Buckner RL, Krienen FM. The evolution of distributed association networks in the human brain. Trends Cogn Sci. (2013) 17:648–65. doi: 10.1016/j.tics.2013.09.017
42. Goulas A, Bastiani M, Bezgin G, Uylings HB, Roebroeck A, Stiers P. Comparative analysis of the macroscale structural connectivity in the macaque and human brain. PLoS Comput Biol. (2014) 10:e1003529. doi: 10.1371/journal.pcbi.1003529
43. Zhong JD, Rifkin-Graboi A, Ta AT, Yap KL, Chuang KH, Meaney MJ, et al. Functional networks in parallel with cortical development associate with executive functions in children. Cereb Cortex. (2014) 24:1937–47. doi: 10.1093/cercor/bht051
44. Barbas H. General cortical and special prefrontal connections: principles from structure to function. Annu Rev Neurosci. (2015) 38:269–89. doi: 10.1146/annurev-neuro-071714-033936
45. Hage SR, Nieder A. Dual neural network model for the evolution of speech and language. Trends Neurosci. (2016) 39:813–29. doi: 10.1016/j.tins.2016.10.006
46. Wendelken C, Ferrer E, Whitaker KJ, Bunge SA. Fronto-parietal network reconfiguration supports the development of reasoning ability. Cereb Cortex. (2016) 26:2178–90. doi: 10.1093/cercor/bhv050
47. Krongold M, Cooper C, Bray S. Modular development of cortical gray matter across childhood and adolescence. Cereb Cortex. (2017) 27:1125–36. doi: 10.1093/cercor/bhv307
48. Guzman GEC, Sato JR, Vidal MC, Fujita A. Identification of alterations associated with age in the clustering structure of functional brain networks. PLoS ONE. (2018) 13:e0195906. doi: 10.1371/journal.pone.0195906
49. Groenewegen HJ, Uylings HB. The prefrontal cortex and the integration of sensory, limbic and autonomic information. Prog Brain Res. (2000) 126:3–28. doi: 10.1016/S0079-6123(00)26003-2
50. Schoenemann PT, Sheehan MJ, Glotzer LD. Prefrontal white matter volume is disproportionately larger in humans than in other primates. Nat Neurosci. (2005) 8:242–52. doi: 10.1038/nn1394
51. Teffer K, Semendeferi K. Human prefrontal cortex: Evolution, development, and pathology. In: Hofman MA, Falk D, editors. Evolution of the Primate Brain: From Neuron to Behavior. Oxford: Elsevier (2012). p. 191–218.
52. Barbas H, Garcia-Cabezas MA. How the prefrontal executive got its stripes. Curr Opin Neurobiol. (2016) 40:125–34. doi: 10.1016/j.conb.2016.07.003
53. Snow PJ. The structural and functional organization of cognition. Front Hum Neurosci. (2016) 10:501. doi: 10.3389/fnhum.2016.00501
54. Teffer K, Buxhoeveden DP, Stimpson CD, Fobbs AJ, Schapiro SJ, Baze WB, et al. Developmental changes in the spatial organization of neurons in the neocortex of humans and common chimpanzees. J Compar Neurol. (2013) 521:4249–59. doi: 10.1002/cne.23412
55. Petanjek Z, Judas M, Kostovic I, Uylings HB. Lifespan alterations of basal dendritic trees of pyramidal neurons in the human prefrontal cortex: a layer-specific pattern. Cereb Cortex. (2008) 18:915–29. doi: 10.1093/cercor/bhm124
56. Sedmak D, Hrvoj-Mihic B, Dzaja D, Habek N, Uylings HB, Petanjek Z. Biphasic dendritic growth of dorsolateral prefrontal cortex associative neurons and early cognitive development. Croat Med J. (2018) 59:189–202. doi: 10.3325/cmj.2018.59.189
57. Poggi G, Boretius S, Mobius W, Moschny N, Baudewig J, Ruhwedel T, et al. Cortical network dysfunction caused by a subtle defect of myelination. Glia. (2016) 64:2025–40. doi: 10.1002/glia.23039
58. Espinoza FA, Vergara VM, Reyes D, Anderson NE, Harenski CL, Decety J, et al. Aberrant functional network connectivity in psychopathy from a large (N = 985) forensic sample. Hum Brain Mapp. (2018) 39:2624–34. doi: 10.1002/hbm.24028
59. Zikopoulos B, Garcia-Cabezas MA, Barbas H. Parallel trends in cortical gray and white matter architecture and connections in primates allow fine study of pathways in humans and reveal network disruptions in autism. PLoS Biol. (2018) 16:e2004559. doi: 10.1371/journal.pbio.2004559
60. Schwartz ML, Goldman-Rakic PS. Single cortical neurones have axon collaterals to ipsilateral and contralateral cortex in fetal and adult primates. Nature. (1982) 299:154–5. doi: 10.1038/299154a0
61. Barbas H, Hilgetag CC, Saha S, Dermon CR, Suski JL. Parallel organization of contralateral and ipsilateral prefrontal cortical projections in the rhesus monkey. BMC Neurosci. (2005) 6:32. doi: 10.1186/1471-2202-6-32
62. Barbas H, Pandya DN. Architecture and intrinsic connections of the prefrontal cortex in the rhesus monkey. J Comp Neurol. (1989) 286:353–75. doi: 10.1002/cne.902860306
63. Kritzer MF, Goldman-Rakic PS. Intrinsic circuit organization of the major layers and sublayers of the dorsolateral prefrontal cortex in the rhesus monkey. J Comp Neurol. (1995) 359:131–43. doi: 10.1002/cne.903590109
64. Melchitzky DS, Gonzalez-Burgos G, Barrionuevo G, Lewis DA. Synaptic targets of the intrinsic axon collaterals of supragranular pyramidal neurons in monkey prefrontal cortex. J Comp Neurol. (2001) 430:209–21. doi: 10.1002/1096-9861(20010205)430:2<209::AID-CNE1026>3.0.CO;2-%23
65. Hladnik A, Dzaja D, Darmopil S, Jovanov-Milosevic N, Petanjek Z. Spatio-temporal extension in site of origin for cortical calretinin neurons in primates. Front Neuroanat. (2014) 8:50. doi: 10.3389/fnana.2014.00050
66. Džaja D, Hladnik A, Bicanic I, Bakovic M, Petanjek Z. Neocortical calretinin neurons in primates: increase in proportion and microcircuitry structure. Front Neuroanat. (2014) 8:103. doi: 10.3389/fnana.2014.00103
67. Uylings HB, Van Eden CG. Qualitative and quantitative comparison of the prefrontal cortex in rat and in primates, including humans. Prog Brain Res. (1990) 85:31–62. doi: 10.1016/S0079-6123(08)62675-8
68. Petrides M, Tomaiuolo F, Yeterian EH, Pandya DN. The prefrontal cortex: comparative architectonic organization in the human and the macaque monkey brains. Cortex. (2012) 48:46–57. doi: 10.1016/j.cortex.2011.07.002
69. Hrvoj-Mihic B, Bienvenu T, Stefanacci L, Muotri AR, Semendeferi K. Evolution, development, and plasticity of the human brain: from molecules to bones. Front Hum Neurosci. (2013) 7:707. doi: 10.3389/fnhum.2013.00707
70. Wang GZ, Konopka G. Differential functional constraints on the evolution of postsynaptic density proteins in neocortical laminae. PLoS ONE. (2012) 7:e39686. doi: 10.1371/journal.pone.0039686
71. Krienen FM, Yeo BTT, Ge T, Buckner RL, Sherwood CC. Transcriptional profiles of supragranular-enriched genes associate with corticocortical network architecture in the human brain. Proc Natl Acade Sci USA. (2016) 113:E469–78. doi: 10.1073/pnas.1510903113
72. Mohan H, Verhoog MB, Doreswamy KK, Eyal G, Aardse R, Lodder BN, et al. Dendritic and axonal architecture of individual pyramidal neurons across layers of adult human neocortex. Cereb Cortex. (2015) 25:4839–53. doi: 10.1093/cercor/bhv188
73. Spocter MA, Hopkins WD, Barks SK, Bianchi S, Hehmeyer AE, Anderson SM, et al. Neuropil distribution in the cerebral cortex differs between humans and chimpanzees. J Comp Neurol. (2012) 520:2917–29. doi: 10.1002/cne.23074
74. Fuster J. The Prefrontal Cortex. London; Burlington, NJ; San Diego: Academic Press (2008). doi: 10.1016/B978-0-12-373644-4.00002-5
75. Donahue CJ, Glasser MF, Preuss TM, Rilling JK, Van Essen DC. Quantitative assessment of prefrontal cortex in humans relative to nonhuman primates. Proc Natl Acad Sci USA. (2018) 115:E5183–92. doi: 10.1073/pnas.1721653115
76. Werchan DM, Amso D. A novel ecological account of prefrontal cortex functional development. Psychol Rev. (2017) 124:720–39. doi: 10.1037/rev0000078
77. Rajkowska G, Goldman-Rakic PS. Cytoarchitectonic definition of prefrontal areas in the normal human cortex: I. Remapping of areas 9 and 46 using quantitative criteria. Cereb Cortex. (1995) 5:307–22. doi: 10.1093/cercor/5.4.307
78. Kostovic I, Skavic J, Strinovic D. Acetylcholinesterase in the human frontal associative cortex during the period of cognitive development: early laminar shifts and late innervation of pyramidal neurons. Neurosci Lett. (1988) 90:107–12. doi: 10.1016/0304-3940(88)90795-1
79. Mesulam MM, Geula C. Acetylcholinesterase-rich neurons of the human cerebral cortex: cytoarchitectonic and ontogenetic patterns of distribution. J Comp Neurol. (1991) 306:193–220. doi: 10.1002/cne.903060202
80. Mrzljak L, Goldman-Rakic PS. Acetylcholinesterase reactivity in the frontal cortex of human and monkey: contribution of AChE-rich pyramidal neurons. J Comp Neurol. (1992) 324:261–81. doi: 10.1002/cne.903240208
81. Hof PR, Nimchinsky EA, Morrison JH. Neurochemical phenotype of corticocortical connections in the macaque monkey: quantitative analysis of a subset of neurofilament protein-immunoreactive projection neurons in frontal, parietal, temporal, and cingulate cortices. J Comp Neurol. (1995) 362:109–33. doi: 10.1002/cne.903620107
82. Koenderink MJT. (1996). Postnatal Neuronal Maturation of the Human Prefrontal Cortex. [dissertation thesis]. Amsterdam, NL: Vrije Universiteit Amsterdam.
83. Geyer S, Zilles K, Luppino G, Matelli M. Neurofilament protein distribution in the macaque monkey dorsolateral premotor cortex. Eur J Neurosci. (2000) 12:1554–66. doi: 10.1046/j.1460-9568.2000.00042.x
84. Hof PR, Sherwood CC. Morphomolecular neuronal phenotypes in the neocortex reflect phylogenetic relationships among certain mammalian orders. Anat Rec A Discov Mol Cell Evol Biol. (2005) 287:1153–63. doi: 10.1002/ar.a.20252
85. Campbell MJ, Hof PR, Morrison JH. A subpopulation of primate corticocortical neurons is distinguished by somatodendritic distribution of neurofilament protein. Brain Res. (1991) 539:133–6. doi: 10.1016/0006-8993(91)90695-R
86. Goldman-Rakic PS. The “psychic” neuron of the cerebral cortex. Ann N Y Acad Sci. (1999) 868:13–26. doi: 10.1111/j.1749-6632.1999.tb11270.x
87. Wang Y, Markram H, Goodman PH, Berger TK, Ma J, Goldman-Rakic PS. Heterogeneity in the pyramidal network of the medial prefrontal cortex. Nat Neurosci. (2006) 9:534–42. doi: 10.1038/nn1670
88. Verduzco-Flores S, Bodner M, Ermentrout B, Fuster JM, Zhou Y. Working memory cells' behavior may be explained by cross-regional networks with synaptic facilitation. PLoS ONE. (2009) 4:e6399. doi: 10.1371/journal.pone.0006399
89. Elston GN, Benavides-Piccione R, Elston A, Manger PR, Defelipe J. Pyramidal cells in prefrontal cortex of primates: marked differences in neuronal structure among species. Front Neuroanat. (2011) 5:2. doi: 10.3389/fnana.2011.00002
90. Hoftman GD, Datta D, Lewis DA. Layer 3 excitatory and inhibitory circuitry in the prefrontal cortex: developmental trajectories and alterations in schizophrenia. Biol Psychiat. (2017) 81:862–73. doi: 10.1016/j.biopsych.2016.05.022
91. Hof PR, Cox K, Morrison JH. Quantitative analysis of a vulnerable subset of pyramidal neurons in Alzheimer's disease: I. Superior frontal and inferior temporal cortex. J Comp Neurol. (1990) 301:44–54. doi: 10.1002/cne.903010105
92. Belichenko PV, Hagberg B, Dahlstrom A. Morphological study of neocortical areas in Rett syndrome. Acta Neuropathol. (1997) 93:50–61. doi: 10.1007/s004010050582
93. Glantz LA, Lewis DA. Decreased dendritic spine density on prefrontal cortical pyramidal neurons in schizophrenia. Arch Gen Psychiatry. (2000) 57:65–73. doi: 10.1001/archpsyc.57.1.65
94. Schwarcz R, Eid T, Du F. Neurons in layer III of the entorhinal cortex. A role in epileptogenesis and epilepsy? Ann N Y Acad Sci. (2000) 911:328–42. doi: 10.1111/j.1749-6632.2000.tb06735.x
95. Dorph-Petersen KA, Delevich KM, Marcsisin MJ, Zhang W, Sampson AR, Gundersen HJ, et al. Pyramidal neuron number in layer 3 of primary auditory cortex of subjects with schizophrenia. Brain Res. (2009) 1285:42–57. doi: 10.1016/j.brainres.2009.06.019
96. Glausier JR, Lewis DA. Selective pyramidal cell reduction of GABA(A) receptor alpha1 subunit messenger RNA expression in schizophrenia. Neuropsychopharmacology. (2011) 36:2103–10. doi: 10.1038/npp.2011.102
97. Kana RK, Libero LE, Moore MS. Disrupted cortical connectivity theory as an explanatory model for autism spectrum disorders. Phys Life Rev. (2011) 8:410–37. doi: 10.1016/j.plrev.2011.10.001
98. Zikopoulos B, Barbas H. Altered neural connectivity in excitatory and inhibitory cortical circuits in autism. Front Hum Neurosci. (2013) 7:609. doi: 10.3389/fnhum.2013.00609
99. Catts VS, Fung SJ, Long LE, Joshi D, Vercammen A, Allen KM, et al. Rethinking schizophrenia in the context of normal neurodevelopment. Front Cell Neurosci. (2013) 7:60. doi: 10.3389/fncel.2013.00060
100. Glausier JR, Lewis DA. Dendritic spine pathology in schizophrenia. Neuroscience. (2013) 251:90–107. doi: 10.1016/j.neuroscience.2012.04.044
101. Mitchell P. Introduction to the Theory of Mind: Children, Autism and Apes. New York, NY: Oxford University Press Inc (1997).
102. Frith CD, Frith U. Mechanisms of social cognition. Annu Rev Psychol. (2012) 63:287–313. doi: 10.1146/annurev-psych-120710-100449
103. Peterson CC, Wellman HM. Longitudinal Theory of Mind (ToM) development from preschool to adolescence with and without ToM delay. Child Dev. (2018). doi: 10.1111/cdev.13064
104. Colonnesi C, Rieffe C, Koops W, Perucchini P. Precursors of a theory of mind: a longitudinal study. Br J Dev Psychol. (2008) 26:561–77. doi: 10.1348/026151008X285660
105. Upshaw MB, Sommerville JA. Twelve-month-old infants anticipatorily plan their actions according to expected object weight in a novel motor context. Front Public Health. (2015) 3:32. doi: 10.3389/fpubh.2015.00032
106. Schieler A, Koenig M, Buttelmann D. Fourteen-month-olds selectively search for and use information depending on the familiarity of the informant in both laboratory and home contexts. J Exp Child Psychol. (2018) 174:112–29. doi: 10.1016/j.jecp.2018.05.010
107. Knudsen B, Liszkowski U. Eighteen- and 24-month-old infants correct others in anticipation of action mistakes. Dev Sci. (2012) 15:113–22. doi: 10.1111/j.1467-7687.2011.01098.x
108. Wade M, Moore C, Astington JW, Frampton K, Jenkins JM. Cumulative contextual risk, maternal responsivity, and social cognition at 18 months. Dev Psychopathol. (2015) 27:189–203. doi: 10.1017/S0954579414000674
109. Eggebrecht AT, Elison JT, Feczko E, Todorov A, Wolff JJ, Kandala S, et al. Joint attention and brain functional connectivity in infants and toddlers. Cereb Cortex. (2017) 27:1709–20. doi: 10.1093/cercor/bhw403
110. Lillard AS, Kavanaugh RD. The contribution of symbolic skills to the development of an explicit theory of mind. Child Dev. (2014) 85:1535–51. doi: 10.1111/cdev.12227
111. Cvencek D, Greenwald AG, Meltzoff AN. Measuring implicit attitudes of 4-year-olds: the preschool implicit association test. J Exp Child Psychol. (2011) 109:187–200. doi: 10.1016/j.jecp.2010.11.002
112. Bowman LC, Liu D, Meltzoff AN, Wellman HM. Neural correlates of belief- and desire-reasoning in 7- and 8-year-old children: an event-related potential study. Dev Sci. (2012) 15:618–32. doi: 10.1111/j.1467-7687.2012.01158.x
113. Kushnir T, Gopnik A, Chernyak N, Seiver E, Wellman HM. Developing intuitions about free will between ages four and six. Cognition. (2015) 138:79–101. doi: 10.1016/j.cognition.2015.01.003
114. Lieberman MD. Social cognitive neuroscience: a review of core processes. Annu Rev Psychol. (2007) 58:259–89. doi: 10.1146/annurev.psych.58.110405.085654
115. Schurz M, Radua J, Aichhorn M, Richlan F, Perner J. Fractionating theory of mind: a meta-analysis of functional brain imaging studies. Neurosci Biobehav Rev. (2014) 42:9–34. doi: 10.1016/j.neubiorev.2014.01.009
116. Grosse Wiesmann C, Schreiber J, Singer T, Steinbeis N, Friederici AD. White matter maturation is associated with the emergence of Theory of Mind in early childhood. Nat Commun. (2017) 8:14692. doi: 10.1038/ncomms14692
117. Thye MD, Ammons CJ, Murdaugh DL, Kana RK. Differential recruitment of theory of mind brain network across three tasks: an independent component analysis. Behav Brain Res. (2018) 347:385–93. doi: 10.1016/j.bbr.2018.03.041
118. Lewis JD, Evans AC, Pruett JR, Botteron K, Zwaigenbaum L, Estes A, et al. Network inefficiencies in autism spectrum disorder at 24 months. Transl Psychiatry. (2014) 4:e388. doi: 10.1038/tp.2014.24
119. Mehling MH, Tasse MJ. Severity of autism spectrum disorders: current conceptualization, and transition to DSM-5. J Autism Dev Disord. (2016) 46:2000–16. doi: 10.1007/s10803-016-2731-7
120. Colle L, Baron-Cohen S, Hill J. Do children with autism have a theory of mind? A non-verbal test of autism vs specific language impairment. J Autism Dev Disord. (2007) 37:716–23. doi: 10.1007/s10803-006-0198-7
121. Happe FG. The role of age and verbal ability in the theory of mind task performance of subjects with autism. Child Dev. (1995) 66:843–55. doi: 10.2307/1131954
122. Baron-Cohen S, Jolliffe T, Mortimore C, Robertson M. Another advanced test of theory of mind: evidence from very high functioning adults with autism or asperger syndrome. J Child Psychol Psychiatry. (1997) 38:813–22. doi: 10.1111/j.1469-7610.1997.tb01599.x
123. Setoh P, Scott RM, Baillargeon R. Two-and-a-half-year-olds succeed at a traditional false-belief task with reduced processing demands. Proc Natl Acad Sci USA. (2016) 113:13360–5. doi: 10.1073/pnas.1609203113
124. Gilmore JH, Knickmeyer RC, Gao W. Imaging structural and functional brain development in early childhood. Nat Rev Neurosci. (2018) 19:123–37. doi: 10.1038/nrn.2018.1
125. Kana RK, Maximo JO, Williams DL, Keller TA, Schipul SE, Cherkassky VL, et al. Aberrant functioning of the theory-of-mind network in children and adolescents with autism. Mol Autism. (2015) 6:59. doi: 10.1186/s13229-015-0052-x
126. Baron-Cohen S. The development of a theory of mind in autism: deviance and delay? Psychiatr Clin North Am. (1991) 14:33–51. doi: 10.1016/S0193-953X(18)30323-X
127. Estes A, Zwaigenbaum L, Gu H, St John T, Paterson S, Elison JT, et al. Behavioral, cognitive, and adaptive development in infants with autism spectrum disorder in the first 2 years of life. J Neurodev Disord. (2015) 7:24. doi: 10.1186/s11689-015-9117-6
128. Shen MD, Piven J. Brain and behavior development in autism from birth through infancy. Dialogues Clin Neurosci. (2017) 19:325–33.
129. Jure R, Pogonza R, Rapin I. Autism Spectrum Disorders (ASD) in blind children: very high prevalence, potentially better outlook. J Autism Dev Disord. (2016) 46:749–59. doi: 10.1007/s10803-015-2612-5
130. Peterson C, Slaughter V, Moore C, Wellman HM. Peer social skills and theory of mind in children with autism, deafness, or typical development. Dev Psychol. (2016) 52:46–57. doi: 10.1037/a0039833
131. Denmark T, Atkinson J, Campbell R, Swettenham J. Signing with the face: emotional expression in narrative production in deaf children with autism spectrum disorder. J Autism Dev Disord. (2018) 49:294–306. doi: 10.1007/s10803-018-3756-x
132. Supekar K, Uddin LQ, Khouzam A, Phillips J, Gaillard WD, Kenworthy LE, et al. Brain hyperconnectivity in children with autism and its links to social deficits. Cell Rep. (2013) 5:738–47. doi: 10.1016/j.celrep.2013.10.001
133. Orekhova EV, Elsabbagh M, Jones EJ, Dawson G, Charman T, Johnson MH, et al. EEG hyper-connectivity in high-risk infants is associated with later autism. J Neurodev Disord. (2014) 6:40. doi: 10.1186/1866-1955-6-40
134. Dajani DR, Uddin LQ. Local brain connectivity across development in autism spectrum disorder: a cross-sectional investigation. Autism Res. (2016) 9:43–54. doi: 10.1002/aur.1494
135. Solso S, Xu R, Proudfoot J, Hagler DJJr, Campbell K, Venkatraman V, et al. Diffusion tensor imaging provides evidence of possible axonal overconnectivity in frontal lobes in autism spectrum disorder toddlers. Biol Psychiatry. (2016) 79:676–84. doi: 10.1016/j.biopsych.2015.06.029
136. Conti E, Mitra J, Calderoni S, Pannek K, Shen KK, Pagnozzi A, et al. Network over-connectivity differentiates autism spectrum disorder from other developmental disorders in toddlers: A diffusion MRI study. Hum Brain Mapp. (2017) 38:2333–44. doi: 10.1002/hbm.23520
137. Chen H, Wang J, Uddin LQ, Wang X, Guo X, Lu F, et al. Aberrant functional connectivity of neural circuits associated with social and sensorimotor deficits in young children with autism spectrum disorder. Autism Res. (2018) 11:1643–52. doi: 10.1002/aur.2029
138. Carper RA, Courchesne E. Localized enlargement of the frontal cortex in early autism. Biol Psychiatry. (2005) 57:126–33. doi: 10.1016/j.biopsych.2004.11.005
139. Courchesne E, Pierce K. Why the frontal cortex in autism might be talking only to itself: local over-connectivity but long-distance disconnection. Curr Opin Neurobiol. (2005) 15:225–30. doi: 10.1016/j.conb.2005.03.001
140. Chawarska K, Campbell D, Chen L, Shic F, Klin A, Chang J. Early generalized overgrowth in boys with autism. Arch Gen Psychiatry. (2011) 68:1021–31. doi: 10.1001/archgenpsychiatry.2011.106
141. Hazlett HC, Poe MD, Gerig G, Styner M, Chappell C, Smith RG, et al. Early brain overgrowth in autism associated with an increase in cortical surface area before age 2 years. Arch Gen Psychiatry. (2011) 68:467–76. doi: 10.1001/archgenpsychiatry.2011.39
142. Abrams DA, Lynch CJ, Cheng KM, Phillips J, Supekar K, Ryali S, et al. Underconnectivity between voice-selective cortex and reward circuitry in children with autism. Proc Natl Acad Sci USA. (2013) 110:12060–5. doi: 10.1073/pnas.1302982110
143. Falahpour M, Thompson WK, Abbott AE, Jahedi A, Mulvey ME, Datko M, et al. Underconnected, but not broken? dynamic functional connectivity MRI shows underconnectivity in autism is linked to increased intra-individual variability across time. Brain Connect. (2016) 6:403–14. doi: 10.1089/brain.2015.0389
144. Chen H, Nomi JS, Uddin LQ, Duan X, Chen H. Intrinsic functional connectivity variance and state-specific under-connectivity in autism. Hum Brain Mapp. (2017) 38:5740–55. doi: 10.1002/hbm.23764
145. Dinstein I, Pierce K, Eyler L, Solso S, Malach R, Behrmann M, et al. Disrupted neural synchronization in toddlers with autism. Neuron. (2011) 70:1218–25. doi: 10.1016/j.neuron.2011.04.018
146. Uddin LQ, Supekar K, Menon V. Reconceptualizing functional brain connectivity in autism from a developmental perspective. Front Hum Neurosci. (2013) 7:458. doi: 10.3389/fnhum.2013.00458
147. Kana RK, Uddin LQ, Kenet T, Chugani D, Muller RA. Brain connectivity in autism. Front Hum Neurosci. (2014) 8:349. doi: 10.3389/fnhum.2014.00349
148. Vasa RA, Mostofsky SH, Ewen JB. The disrupted connectivity hypothesis of autism spectrum disorders: time for the next phase in research. Biol Psychiatry Cogn Neurosci Neuroimaging. (2016) 1:245–52. doi: 10.1016/j.bpsc.2016.02.003
149. Lombardo MV, Courchesne E, Lewis NE, Pramparo T. Hierarchical cortical transcriptome disorganization in autism. Mol Autism. (2017) 8:29. doi: 10.1186/s13229-017-0147-7
150. Mash LE, Reiter MA, Linke AC, Townsend J, Muller RA. Multimodal approaches to functional connectivity in autism spectrum disorders: An integrative perspective. Dev Neurobiol. (2018) 78:456–73. doi: 10.1002/dneu.22570
151. Muller RA, Fishman I. Brain connectivity and neuroimaging of social networks in autism. Trends Cogn Sci. (2018) 22:1103–16. doi: 10.1016/j.tics.2018.09.008
152. Yang DY, Beam D, Pelphrey KA, Abdullahi S, Jou RJ. Cortical morphological markers in children with autism: a structural magnetic resonance imaging study of thickness, area, volume, and gyrification. Mol Autism. (2016) 7:11. doi: 10.1186/s13229-016-0076-x
153. Fingher N, Dinstein I, Ben-Shachar M, Haar S, Dale AM, Eyler L, et al. Toddlers later diagnosed with autism exhibit multiple structural abnormalities in temporal corpus callosum fibers. Cortex. (2017) 97:291–305. doi: 10.1016/j.cortex.2016.12.024
154. Griebling J, Minshew NJ, Bodner K, Libove R, Bansal R, Konasale P, et al. Dorsolateral prefrontal cortex magnetic resonance imaging measurements and cognitive performance in autism. J Child Neurol. (2010) 25:856–63. doi: 10.1177/0883073809351313
155. Kumar A, Sundaram SK, Sivaswamy L, Behen ME, Makki MI, Ager J, et al. Alterations in frontal lobe tracts and corpus callosum in young children with autism spectrum disorder. Cereb Cortex. (2010) 20:2103–13. doi: 10.1093/cercor/bhp278
156. Catani M, Dell'acqua F, Budisavljevic S, Howells H, Thiebaut De Schotten M, Froudist-Walsh S, et al. Frontal networks in adults with autism spectrum disorder. Brain. (2016) 139:616–30. doi: 10.1093/brain/awv351
157. Kana RK, Patriquin MA, Black BS, Channell MM, Wicker B. Altered medial frontal and superior temporal response to implicit processing of emotions in autism. Autism Res. (2016) 9:55–66. doi: 10.1002/aur.1496
158. Khundrakpam BS, Lewis JD, Kostopoulos P, Carbonell F, Evans AC. Cortical thickness abnormalities in autism spectrum disorders through late childhood, adolescence, and adulthood: a large-scale MRI study. Cereb Cortex. (2017) 27:1721–31. doi: 10.1093/cercor/bhx038
159. Ameis SH, Kassee C, Corbett-Dick P, Cole L, Dadhwal S, Lai MC, et al. Systematic review and guide to management of core and psychiatric symptoms in youth with autism. Acta Psychiatr Scand. (2018) 138:379–400. doi: 10.1111/acps.12918
160. Dickie EW, Ameis SH, Shahab S, Calarco N, Smith DE, Miranda D, et al. Personalized intrinsic network topography mapping and functional connectivity deficits in autism spectrum disorder. Biol Psychiatry. (2018) 84:278–86. doi: 10.1016/j.biopsych.2018.02.1174
161. Zoghbi HY, Bear MF. Synaptic dysfunction in neurodevelopmental disorders associated with autism and intellectual disabilities. Cold Spring Harb Perspect Biol. (2012) 4:a009886. doi: 10.1101/cshperspect.a009886
162. Hahamy A, Behrmann M, Malach R. The idiosyncratic brain: distortion of spontaneous connectivity patterns in autism spectrum disorder. Nat Neurosci. (2015) 18:302–9. doi: 10.1038/nn.3919
163. Habela CW, Song HJ, Ming GL. Modeling synaptogenesis in schizophrenia and autism using human iPSC derived neurons. Mol Cell Neurosci. (2016) 73:52–62. doi: 10.1016/j.mcn.2015.12.002
164. Thomas MSC, Davis R, Karmiloff-Smith A, Knowland VCP, Charman T. The over-pruning hypothesis of autism. Dev Sci. (2016) 19:284–305. doi: 10.1111/desc.12303
165. Romero-Garcia R, Warrier V, Bullmore ET, Baron-Cohen S, Bethlehem RAI. Synaptic and transcriptionally downregulated genes are associated with cortical thickness differences in autism. Mol Psychiatry. (2018). doi: 10.1038/s41380-018-0023-7. [Epub ahead of print].
166. Schwede M, Nagpal S, Gandal MJ, Parikshak NN, Mirnics K, Geschwind DH, et al. Strong correlation of downregulated genes related to synaptic transmission and mitochondria in post-mortem autism cerebral cortex. J Neurodev Disord. (2018) 10:18. doi: 10.1186/s11689-018-9237-x
167. Happe F, Ehlers S, Fletcher P, Frith U, Johansson M, Gillberg C, et al. 'Theory of mind' in the brain. Evidence from a PET scan study of Asperger syndrome. Neuroreport. (1996) 8:197–201. doi: 10.1097/00001756-199612200-00040
168. Goldstein G, Allen DN, Minshew NJ, Williams DL, Volkmar F, Klin A, et al. The structure of intelligence in children and adults with high functioning autism. Neuropsychology. (2008) 22:301–12. doi: 10.1037/0894-4105.22.3.301
169. Eilam-Stock T, Wu T, Spagna A, Egan LJ, Fan J. Neuroanatomical alterations in high-functioning adults with autism spectrum disorder. Front Neurosci. (2016) 10:237. doi: 10.3389/fnins.2016.00237
170. Vissers ME, Cohen MX, Geurts HM. Brain connectivity and high functioning autism: a promising path of research that needs refined models, methodological convergence, and stronger behavioral links. Neurosci Biobehav Rev. (2012) 36:604–25. doi: 10.1016/j.neubiorev.2011.09.003
171. Iuculano T, Rosenberg-Lee M, Supekar K, Lynch CJ, Khouzam A, Phillips J, et al. Brain organization underlying superior mathematical abilities in children with autism. Biol Psychiatry. (2014) 75:223–30. doi: 10.1016/j.biopsych.2013.06.018
172. Geschwind N, Kaplan E. A human cerebral deconnection syndrome: a preliminary report. 1962. Neurology. (1998) 50:1201–212. doi: 10.1212/WNL.50.5.1201-a
173. Geschwind DH, Levitt P. Autism spectrum disorders: developmental disconnection syndromes. Curr Opin Neurobiol. (2007) 17:103–11. doi: 10.1016/j.conb.2007.01.009
174. Geschwind N. Disconnexion syndromes in animals and man: part, I. 1965. Neuropsychol Rev. (2010) 20:128–57. doi: 10.1007/s11065-010-9131-0
175. Xiao Z, Qiu T, Ke X, Xiao X, Xiao T, Liang F, et al. Autism spectrum disorder as early neurodevelopmental disorder: evidence from the brain imaging abnormalities in 2-3 years old toddlers. J Autism Dev Disord. (2014) 44:1633–40. doi: 10.1007/s10803-014-2033-x
176. Smith E, Thurm A, Greenstein D, Farmer C, Swedo S, Giedd J, et al. Cortical thickness change in autism during early childhood. Hum Brain Mapp. (2016) 37:2616–29. doi: 10.1002/hbm.23195
177. Herringshaw AJ, Kumar SL, Rody KN, Kana RK. Neural correlates of social perception in children with autism: local versus global preferences. Neuroscience. (2018) 395:49–59. doi: 10.1016/j.neuroscience.2018.10.044
178. Uylings HB, Van Pelt J, Parnavelas JG, Ruiz-Marcos A. Geometrical and topological characteristics in the dendritic development of cortical pyramidal and non-pyramidal neurons. Prog Brain Res. (1994) 102:109–23. doi: 10.1016/S0079-6123(08)60535-X
179. Emoto K. Dendrite remodeling in development and disease. Dev Growth Diff. (2011) 53:277–86. doi: 10.1111/j.1440-169X.2010.01242.x
180. Bicanic I, Hladnik A, Petanjek Z. A quantitative golgi study of dendritic morphology in the mice striatal medium spiny neurons. Front Neuroanat. (2017) 11:37. doi: 10.3389/fnana.2017.00037
181. Papoutsi A, Kastellakis G, Poirazi P. Basal tree complexity shapes functional pathways in the prefrontal cortex. J Neurophysiol. (2017) 118:1970–83. doi: 10.1152/jn.00099.2017
182. Jose R, Santen L, Shaebani MR. Trapping in and escape from branched structures of neuronal dendrites. Biophys J. (2018) 115:2014–25. doi: 10.1016/j.bpj.2018.09.029
183. Cline HT. Dendritic arbor development and synaptogenesis. Curr Opin Neurobiol. (2001) 11:118–26. doi: 10.1016/S0959-4388(00)00182-3
184. Mcallister AK. Conserved cues for axon and dendrite growth in the developing cortex. Neuron. (2002) 33:2–4. doi: 10.1016/S0896-6273(01)00577-3
185. Elston GN, Fujita I. Pyramidal cell development: postnatal spinogenesis, dendritic growth, axon growth, and electrophysiology. Front Neuroanat. (2014) 8:78. doi: 10.3389/fnana.2014.00078
186. Khazipov R, Esclapez M, Caillard O, Bernard C, Khalilov I, Tyzio R, et al. Early development of neuronal activity in the primate hippocampus in utero. J Neurosci. (2001) 21:9770–81. doi: 10.1523/JNEUROSCI.21-24-09770.2001
187. Bystron I, Blakemore C, Rakic P. Development of the human cerebral cortex: boulder Committee revisited. Nat Rev Neurosci. (2008) 9:110–22. doi: 10.1038/nrn2252
188. Kostović I, Judaš M, Petanjek Z. Structural development of the human prefrontal cortex. In: Nelson CA, Luciana M, editors. Handbook of Developmental Cognitive Neuroscience. Cambridge: MIT Press (2008). p. 213–35.
189. Mrzljak L, Uylings HB, Van Eden CG, Judas M. Neuronal development in human prefrontal cortex in prenatal and postnatal stages. Prog Brain Res. (1990) 85:185–222. doi: 10.1016/S0079-6123(08)62681-3
190. Mrzljak L, Uylings HB, Kostovic I, Van Eden CG. Prenatal development of neurons in the human prefrontal cortex. II A quantitative Golgi study. J Comp Neurol. (1992) 316:485–96. doi: 10.1002/cne.903160408
191. Groc L, Petanjek Z, Gustafsson B, Ben-Ari Y, Hanse E, Khazipov R. In vivo blockade of neural activity alters dendritic development of neonatal CA1 pyramidal cells. Eur J Neurosci. (2002) 16:1931–8. doi: 10.1046/j.1460-9568.2002.02264.x
192. Sin WC, Haas K, Ruthazer ES, Cline HT. Dendrite growth increased by visual activity requires NMDA receptor and Rho GTPases. Nature. (2002) 419:475–80. doi: 10.1038/nature00987
193. Pratt KG, Hiramoto M, Cline HT. An evolutionarily conserved mechanism for activity-dependent visual circuit development. Front Neural Circuits. (2016) 10:79. doi: 10.3389/fncir.2016.00079
194. Bustos FJ, Jury N, Martinez P, Ampuero E, Campos M, Abarzua S, et al. NMDA receptor subunit composition controls dendritogenesis of hippocampal neurons through CAMKII, CREB-P, and H3K27ac. J Cell Physiol. (2017) 232:3677–92. doi: 10.1002/jcp.25843
195. Sudhof TC. Towards an understanding of synapse formation. Neuron. (2018) 100:276–93. doi: 10.1016/j.neuron.2018.09.040
196. Kostovic I, Jovanov-Milosevic N. The development of cerebral connections during the first 20-45 weeks' gestation. Semin Fetal Neonatal Med. (2006) 11:415–22. doi: 10.1016/j.siny.2006.07.001
197. Koenderink MJ, Uylings HB, Mrzljak L. Postnatal maturation of the layer III pyramidal neurons in the human prefrontal cortex: a quantitative Golgi analysis. Brain Res. (1994) 653:173–82. doi: 10.1016/0006-8993(94)90387-5
198. Petanjek Z. (1998). Postnatal maturation of projection neurons in the human prefrontal cortex. [dissertation thesis]. [Zagreb (CRO)]: University of Zagreb.
199. Gonzalez-Burgos G, Kroener S, Zaitsev AV, Povysheva NV, Krimer LS, Barrionuevo G, et al. Functional maturation of excitatory synapses in layer 3 pyramidal neurons during postnatal development of the primate prefrontal cortex. Cereb Cortex. (2008) 18:626–37. doi: 10.1093/cercor/bhm095
200. Hanse E, Taira T, Lauri S, Groc L. Glutamate synapse in developing brain: an integrative perspective beyond the silent state. Trends Neurosci. (2009) 32:532–7. doi: 10.1016/j.tins.2009.07.003
201. Dupuis JP, Ladepeche L, Seth H, Bard L, Varela J, Mikasova L, et al. Surface dynamics of GluN2B-NMDA receptors controls plasticity of maturing glutamate synapses. EMBO J. (2014) 33:842–61. doi: 10.1002/embj.201386356
202. Groc L, Petanjek Z, Gustafsson B, Ben-Ari Y, Khazipov R, Hanse E. Compensatory dendritic growth of CA1 pyramidal cells following growth impairment in the neonatal period. Eur J Neurosci. (2003) 18:1332–6. doi: 10.1046/j.1460-9568.2003.02839.x
203. Koleske AJ. Molecular mechanisms of dendrite stability. Nat Rev Neurosci. (2013) 14:536–50. doi: 10.1038/nrn3486
204. Mrzljak L, Uylings HB, Kostovic I, Van Eden CG. Prenatal development of neurons in the human prefrontal cortex: I. A qualitative Golgi study. J Comp Neurol. (1988) 271:355–86. doi: 10.1002/cne.902710306
205. Bourgeois JP, Goldman-Rakic PS, Rakic P. Synaptogenesis in the prefrontal cortex of rhesus monkeys. Cereb Cortex. (1994) 4:78–96. doi: 10.1093/cercor/4.1.78
206. Anderson SA, Classey JD, Condé F, Lund JS, Lewis DA. Synchronous development of pyramidal neuron dendritic spines and parvalbumin-immunoreactive chandelier neuron axon terminals in layer III of monkey prefrontal cortex. Neuroscience. (1995) 67:7–22. doi: 10.1016/0306-4522(95)00051-J
207. Koenderink MJ, Uylings HB. Postnatal maturation of layer V pyramidal neurons in the human prefrontal cortex. A quantitative Golgi analysis. Brain Res. (1995) 678:233–43. doi: 10.1016/0006-8993(95)00206-6
208. Jacobs B, Driscoll L, Schall M. Life-span dendritic and spine changes in areas 10 and 18 of human cortex: a quantitative Golgi study. J Comp Neurol. (1997) 386:661–80. doi: 10.1002/(SICI)1096-9861(19971006)386:4<661::AID-CNE11>3.0.CO;2-N
209. Woo TU, Pucak ML, Kye CH, Matus CV, Lewis DA. Peripubertal refinement of the intrinsic and associational circuitry in monkey prefrontal cortex. Neuroscience. (1997) 80:1149–58. doi: 10.1016/S0306-4522(97)00059-6
210. De Brabander JM, Kramers RJ, Uylings HB. Layer-specific dendritic regression of pyramidal cells with ageing in the human prefrontal cortex. Eur J Neurosci. (1998) 10:1261–9. doi: 10.1046/j.1460-9568.1998.00137.x
211. Lewis DA, Sesack SR, Levey AI, Rosenberg DR. Dopamine axons in primate prefrontal cortex: specificity of distribution, synaptic targets, and development. Adv Pharmacol. (1998) 42:703–6. doi: 10.1016/S1054-3589(08)60845-5
212. Petanjek Z, Rašin MR, Jovanov N, Krsnik Ž. Magnopyramidal neurons in the area 9 of the human prefrontal cortex. A quantitative rapid Golgi study. Period Biol. (1998) 100:221–30.
213. Petanjek Z, Rašin MR, Darmopil S, Jovanov N, Ivkić G. Morphology of layer V pyramidal neurons in area 9 of the human prefrontal cortex in adulthood and during aging. A quantitative rapid Golgi study. Period Biol. (2000) 102:411–9.
214. Jacobs B, Schall M, Prather M, Kapler E, Driscoll L, Baca S, et al. Regional dendritic and spine variation in human cerebral cortex: a quantitative golgi study. Cereb Cortex. (2001) 11:558–71. doi: 10.1093/cercor/11.6.558
215. Soloway AS, Pucak ML, Melchitzky DS, Lewis DA. Dendritic morphology of callosal and ipsilateral projection neurons in monkey prefrontal cortex. Neuroscience. (2002) 109:461–71. doi: 10.1016/S0306-4522(01)00507-3
216. Cruz DA, Lovallo EM, Stockton S, Rasband M, Lewis DA. Postnatal development of synaptic structure proteins in pyramidal neuron axon initial segments in monkey prefrontal cortex. J Comp Neurol. (2009) 514:353–67. doi: 10.1002/cne.22006
217. Vuksic M, Petanjek Z, Kostovic I. Development of prefrontal layer Iii pyramidal neurons in infants with down syndrome. Trans Neurosci. (2011) 2:225–32. doi: 10.2478/s13380-011-0027-0
218. Oga T, Aoi H, Sasaki T, Fujita I, Ichinohe N. Postnatal development of layer III pyramidal cells in the primary visual, inferior temporal, and prefrontal cortices of the marmoset. Front Neural Circ. (2013) 7:31. doi: 10.3389/fncir.2013.00031
219. Sasaki T, Aoi H, Oga T, Fujita I, Ichinohe N. Postnatal development of dendritic structure of layer III pyramidal neurons in the medial prefrontal cortex of marmoset. Brain Struct Funct. (2015) 220:3245–58. doi: 10.1007/s00429-014-0853-2
220. Hrvoj-Mihic B, Hanson KL, Lew CH, Stefanacci L, Jacobs B, Bellugi U, et al. Basal dendritic morphology of cortical pyramidal neurons in williams syndrome: prefrontal cortex and beyond. Front Neurosci. (2017) 11:419. doi: 10.3389/fnins.2017.00419
221. Oga T, Elston GN, Fujita I. Postnatal dendritic growth and spinogenesis of layer-V pyramidal cells differ between visual, inferotemporal, and prefrontal cortex of the macaque monkey. Front Neurosci. (2017) 11:118. doi: 10.3389/fnins.2017.00118
222. Kostovic I, Rakic P. Developmental history of the transient subplate zone in the visual and somatosensory cortex of the macaque monkey and human brain. J Compar Neurol. (1990) 297:441–70. doi: 10.1002/cne.902970309
223. Duque A, Krsnik Z, Kostovic I, Rakic P. Secondary expansion of the transient subplate zone in the developing cerebrum of human and nonhuman primates. Proc Natl Acad Sci USA. (2016) 113:9892–7. doi: 10.1073/pnas.1610078113
224. Jovanov-Milosevic N, Culjat M, Kostovic I. Growth of the human corpus callosum: modular and laminar morphogenetic zones. Front Neuroanat. (2009) 3:6. doi: 10.3389/neuro.05.006.2009
225. Vasung L, Huang H, Jovanov-Milosevic N, Pletikos M, Mori S, Kostovic I. Development of axonal pathways in the human fetal fronto-limbic brain: histochemical characterization and diffusion tensor imaging. J Anat. (2010) 217:400–17. doi: 10.1111/j.1469-7580.2010.01260.x
226. Rakic P, Bourgeois JP, Goldman-Rakic PS. Synaptic development of the cerebral cortex: implications for learning, memory, and mental illness. Prog Brain Res. (1994) 102:227–43. doi: 10.1016/S0079-6123(08)60543-9
227. Batalle D, Hughes EJ, Zhang H, Tournier JD, Tusor N, Aljabar P, et al. Early development of structural networks and the impact of prematurity on brain connectivity. Neuroimage. (2017) 149:379–92. doi: 10.1016/j.neuroimage.2017.01.065
228. Shi F, Salzwedel AP, Lin W, Gilmore JH, Gao W. Functional brain parcellations of the infant brain and the associated developmental trends. Cereb Cortex. (2018) 28:1358–68. doi: 10.1093/cercor/bhx062
229. Goldman-Rakic PS. The prefrontal landscape: implications of functional architecture for understanding human mentation and the central executive. Philos Trans R Soc Lond B Biol Sci. (1996) 351:1445–53. doi: 10.1098/rstb.1996.0129
230. Zelazo PD, Carlson SM, Kesek A. The development of executive function in childhood. In: Nelson CA, Luciana M, editors. Handbook of Developmental Cognitive Neuroscience. Cambridge: MIT Press (2008). p. 553–574.
231. Meltzoff AN, Saby JN, Marshall PJ. Neural representations of the body in 60-day-old human infants. Dev Sci. (2019) 22:e12698. doi: 10.1111/desc.12698
232. Davidson MC, Amso D, Anderson LC, Diamond A. Development of cognitive control and executive functions from 4 to 13 years: evidence from manipulations of memory, inhibition, and task switching. Neuropsychologia. (2006) 44:2037–78. doi: 10.1016/j.neuropsychologia.2006.02.006
233. Murty VP, Calabro F, Luna B. The role of experience in adolescent cognitive development: Integration of executive, memory, and mesolimbic systems. Neurosci Biobehav Rev. (2016) 70:46–58. doi: 10.1016/j.neubiorev.2016.07.034
234. Li G, Wang L, Shi F, Lyall AE, Lin WL, Gilmore JH, et al. Mapping longitudinal development of local cortical gyrification in infants from birth to 2 years of age. J Neurosci. (2014) 34:4228–38. doi: 10.1523/JNEUROSCI.3976-13.2014
235. Skeide MA, Friederici AD. The ontogeny of the cortical language network. Nat Rev Neurosci. (2016) 17:323–32. doi: 10.1038/nrn.2016.23
236. Sours C, Raghavan P, Foxworthy WA, Meredith MA, El Metwally D, Zhuo J, et al. Cortical multisensory connectivity is present near birth in humans. Brain Imaging Behav. (2017) 11:1207–13. doi: 10.1007/s11682-016-9586-6
237. Batalle D, Edwards AD, O'muircheartaigh J. Annual research review: not just a small adult brain: understanding later neurodevelopment through imaging the neonatal brain. J Child Psychol Psychiatry. (2018) 59:350–71. doi: 10.1111/jcpp.12838
238. Dean DCIII, Planalp EM, Wooten W, Schmidt CK, Kecskemeti SR, Frye C, et al. Investigation of brain structure in the 1-month infant. Brain Struct Funct. (2018) 223:1953–70. doi: 10.1007/s00429-018-1643-z
239. Kostovic I, Judas M, Petanjek Z, Simic G. Ontogenesis of goal-directed behavior: anatomo-functional considerations. Int J Psychophysiol. (1995) 19:85–102. doi: 10.1016/0167-8760(94)00081-O
240. Einspieler C, Prechtl HF. Prechtl's assessment of general movements: a diagnostic tool for the functional assessment of the young nervous system. Ment Retard Dev Disabil Res Rev. (2005) 11:61–7. doi: 10.1002/mrdd.20051
241. Hadders-Algra M. Development of postural control during the first 18 months of life. Neural Plast. (2005) 12:99–108; discussion 263-172. doi: 10.1155/NP.2005.99
242. Gao W, Alcauter S, Elton A, Hernandez-Castillo CR, Smith JK, Ramirez J, et al. Functional network development during the first year: relative sequence and socioeconomic correlations. Cereb Cortex. (2015) 25:2919–28. doi: 10.1093/cercor/bhu088
243. Gao W, Lin W, Grewen K, Gilmore JH. Functional connectivity of the infant human brain: plastic and modifiable. Neuroscientist. (2016) 23:169–84. doi: 10.1177/1073858416635986
244. Tousignant B, Eugene F, Jackson PL. A developmental perspective on the neural bases of human empathy. Infant Behav Dev. (2017) 48:5–12. doi: 10.1016/j.infbeh.2015.11.006
245. Marrus N, Eggebrecht AT, Todorov A, Elison JT, Wolff JJ, Cole L, et al. Walking, gross motor development, and brain functional connectivity in infants and toddlers. Cereb Cortex. (2018) 28:750–63. doi: 10.1093/cercor/bhx313
246. Shultz S, Klin A, Jones W. Neonatal transitions in social behavior and their implications for autism. Trends Cogn Sci. (2018) 22:452–69. doi: 10.1016/j.tics.2018.02.012
247. Williams RS, Ferrante RJ, Caviness VSJr. The Golgi rapid method in clinical neuropathology: the morphologic consequences of suboptimal fixation. J Neuropathol Exp Neurol. (1978) 37:13–33. doi: 10.1097/00005072-197801000-00002
248. Braak H, Braak E. Golgi preparations as a tool in neuropathology with particular reference to investigations of the human telencephalic cortex. Prog Neurobiol. (1985) 25:93–139. doi: 10.1016/0301-0082(85)90001-2
249. Sedmak D, Hladnik A, Kostović I, Petanjek Z. Synchronous development of pyramidal neurons across the human frontal cortex during perinatal period. 10th FENS forum of Neuroscience (Index no. 2355) (2016).
250. Goulas A, Uylings HB, Stiers P. Mapping the hierarchical layout of the structural network of the macaque prefrontal cortex. Cereb Cortex. (2014) 24:1178–94. doi: 10.1093/cercor/bhs399
251. Kipping JA, Tuan TA, Fortier MV, Qiu A. Asynchronous development of cerebellar, cerebello-cortical, and cortico-cortical functional networks in infancy, childhood, and adulthood. Cereb Cortex. (2017) 27:5170–84. doi: 10.1093/cercor/bhw298
252. Pendl SL, Salzwedel AP, Goldman BD, Barrett LF, Lin W, Gilmore JH, et al. Emergence of a hierarchical brain during infancy reflected by stepwise functional connectivity. Hum Brain Mapp. (2017) 38:2666–82. doi: 10.1002/hbm.23552
253. Vacc NA, Greenleaf W. Sequential development of cognitive complexity. Percept Mot Skills. (1975) 41:319–22. doi: 10.2466/pms.1975.41.1.319
254. Damaraju E, Caprihan A, Lowe JR, Allen EA, Calhoun VD, Phillips JP. Functional connectivity in the developing brain: a longitudinal study from 4 to 9 months of age. Neuroimage. (2014) 84:169–80. doi: 10.1016/j.neuroimage.2013.08.038
255. Gao W, Alcauter S, Smith JK, Gilmore JH, Lin W. Development of human brain cortical network architecture during infancy. Brain Struct Funct. (2015) 220:1173–86. doi: 10.1007/s00429-014-0710-3
256. Hodel AS. Rapid infant prefrontal cortex development and sensitivity to early environmental experience. Dev Rev. (2018) 48:113–44. doi: 10.1016/j.dr.2018.02.003
257. Ben-Ari Y. The GABA excitatory/inhibitory developmental sequence: a personal journey. Neuroscience. (2014) 279:187–219. doi: 10.1016/j.neuroscience.2014.08.001
258. Datta D, Arion D, Lewis DA. Developmental expression patterns of GABAA receptor subunits in layer 3 and 5 pyramidal cells of monkey prefrontal cortex. Cereb Cortex. (2015) 25:2295–305. doi: 10.1093/cercor/bhu040
259. Grossman AW, Churchill JD, Mckinney BC, Kodish IM, Otte SL, Greenough WT. Experience effects on brain development: possible contributions to psychopathology. J Child Psychol Psychiatry. (2003) 44:33–63. doi: 10.1111/1469-7610.t01-1-00102
260. Uylings HBM. Development of the human cortex and the concept of ‘critical’ or ‘sensitive’ periods. In: Gullberg M, Indefrey P, editors. Series in Cognitive Neuroscience and Language Learning and Processing. Oxford: Blackwell PublCy (2006). p. 59–90.
261. Lewis DA, Gonzalez-Burgos G. Neuroplasticity of neocortical circuits in schizophrenia. Neuropsychopharmacology. (2008) 33:141–65. doi: 10.1038/sj.npp.1301563
262. Jung CKE, Herms J. Structural dynamics of dendritic spines are influenced by an environmental enrichment: an in vivo imaging study. Cereb Cortex. (2014) 24:377–84. doi: 10.1093/cercor/bhs317
263. Nozari M, Suzuki T, Rosa MGP, Yamakawa K, Atapour N. The impact of early environmental interventions on structural plasticity of the axon initial segment in neocortex. Dev Psychobiol. (2017) 59:39–47. doi: 10.1002/dev.21453
264. Schade JP, Van Groenigen W. Structural organization of the human cerebral cortex. 1. Maturation of the middle frontal gyrus. Acta Anat. (1961) 47:74–111. doi: 10.1159/000141802
265. Uylings HBM, Delalle I, Petanjek Z, Koenderink MJT. Structural and immunocytochemical differentiation of neurons in prenatal and postnatal human prefrontal cortex. Neuroembryology. (2002) 1:176–86. doi: 10.1159/000066268
266. Conel L. The Postnatal Development of the Human Cerebral Cortex. Cambridge: Harvard Univ Press (1939-1967).
267. Marin-Padilla M. Prenatal and early postnatal ontogenesis of the human motor cortex: a golgi study. II The basket-pyramidal system. Brain Res. (1970) 23:185–91. doi: 10.1016/0006-8993(70)90038-7
268. Kemper TL, Caveness WF, Yakovlev PI. The neuronographic and metric study of the dendritic arbours of neurons in the motor cortex of Macaca mulatta at birth and at 24 months of age. Brain. (1973) 96:765–82. doi: 10.1093/brain/96.4.765
269. Purpura DP. Normal and aberrant neuronal development in the cerebral cortex of human fetus and young infant. UCLA Forum Med Sci. (1975) 141–69. doi: 10.1016/B978-0-12-139050-1.50014-8
270. Lund JS, Boothe RG, Lund RD. Development of neurons in the visual cortex (area 17) of the monkey (Macaca nemestrina): a Golgi study from fetal day 127 to postnatal maturity. J Comp Neurol. (1977) 176:149–88. doi: 10.1002/cne.901760203
271. Boothe RG, Greenough WT, Lund JS, Wrege K. A quantitative investigation of spine and dendrite development of neurons in visual cortex (area 17) of Macaca nemestrina monkeys. J Comp Neurol. (1979) 186:473–89. doi: 10.1002/cne.901860310
272. Takashima S, Chan F, Becker LE, Armstrong DL. Morphology of the developing visual cortex of the human infant: a quantitative and qualitative Golgi study. J Neuropathol Exp Neurol. (1980) 39:487–501. doi: 10.1097/00005072-198007000-00007
273. Garey LJ, De Courten C. Structural development of the lateral geniculate nucleus and visual cortex in monkey and man. Behav Brain Res. (1983) 10:3–13. doi: 10.1016/0166-4328(83)90145-6
274. Becker LE, Armstrong DL, Chan F, Wood MM. Dendritic development in human occipital cortical neurons. Brain Res. (1984) 315:117–24. doi: 10.1016/0165-3806(84)90083-X
275. Jacobs B, Scheibel AB. A quantitative dendritic analysis of Wernicke's area in humans. I Lifespan changes. J Comp Neurol. (1993) 327:83–96. doi: 10.1002/cne.903270107
276. Bianchi S, Stimpson CD, Duka T, Larsen MD, Janssen WGM, Collins Z, et al. Synaptogenesis and development of pyramidal neuron dendritic morphology in the chimpanzee neocortex resembles humans. Proc Natl Acade Sci USA. (2013) 110:10395–401. doi: 10.1073/pnas.1301224110
277. Rakic P, Bourgeois JP, Eckenhoff MF, Zecevic N, Goldman-Rakic PS. Concurrent overproduction of synapses in diverse regions of the primate cerebral cortex. Science. (1986) 232:232–5. doi: 10.1126/science.3952506
278. Lidow MS, Goldman-Rakic PS, Rakic P. Synchronized overproduction of neurotransmitter receptors in diverse regions of the primate cerebral cortex. Proc Natl Acad Sci USA. (1991) 88:10218–21. doi: 10.1073/pnas.88.22.10218
279. Huttenlocher PR, Dabholkar AS. Regional differences in synaptogenesis in human cerebral cortex. J Comp Neurol. (1997) 387:167–78. doi: 10.1002/(SICI)1096-9861(19971020)387:2<167::AID-CNE1>3.0.CO;2-Z
280. Chugani HT. A critical period of brain development: studies of cerebral glucose utilization with PET. Prev Med. (1998) 27:184–8. doi: 10.1006/pmed.1998.0274
281. Eastwood SL, Weickert CS, Webster MJ, Herman MM, Kleinman JE, Harrison PJ. Synaptophysin protein and mRNA expression in the human hippocampal formation from birth to old age. Hippocampus. (2006) 16:645–54. doi: 10.1002/hipo.20194
282. Elston GN, Oga T, Fujita I. Spinogenesis and pruning scales across functional hierarchies. J Neurosci. (2009) 29:3271–5. doi: 10.1523/JNEUROSCI.5216-08.2009
283. Somel M, Franz H, Yan Z, Lorenc A, Guo S, Giger T, et al. Transcriptional neoteny in the human brain. Proc Natl Acad Sci USA. (2009) 106:5743–8. doi: 10.1073/pnas.0900544106
284. Elston GN, Oga T, Okamoto T, Fujita I. Spinogenesis and pruning from early visual onset to adulthood: an intracellular injection study of layer III pyramidal cells in the ventral visual cortical pathway of the macaque monkey. Cereb Cortex. (2010) 20:1398–408. doi: 10.1093/cercor/bhp203
285. Petanjek Z, Judas M, Simic G, Rasin MR, Uylings HB, Rakic P, et al. Extraordinary neoteny of synaptic spines in the human prefrontal cortex. Proc Natl Acad Sci USA. (2011) 108:13281–6. doi: 10.1073/pnas.1105108108
286. Ang LC, Munoz DG, Shul D, George DH. SMI-32 immunoreactivity in human striate cortex during postnatal development. Brain Res Dev Brain Res. (1991) 61:103–9. doi: 10.1016/0165-3806(91)90119-4
287. Aldama J. Cytoarchitektonik der Grosshirnrinde eines 5-jähriges und eines 1-jähriges Kindes. Z Ges Neurol Psychiat. (1930) 130:532–630. doi: 10.1007/BF02865942
288. Kononova EP. [The development of the frontal region in postnatal period] (Russian). Contr Moscow Brain Inst. (1940) 5:73–124.
289. Amunts K, Schleicher A, Ditterich A, Zilles K. Broca's region: cytoarchitectonic asymmetry and developmental changes. J Comp Neurol. (2003) 465:72–89. doi: 10.1002/cne.10829
290. Judas M, Cepanec M. Adult structure and development of the human fronto-opercular cerebral cortex (Broca's region). Clin Linguist Phon. (2007) 21:975–89. doi: 10.1080/02699200701617175
291. Webster MJ, Ungerleider LG, Bachevalier J. Connections of inferior temporal areas TE and TEO with medial temporal-lobe structures in infant and adult monkeys. J Neurosci. (1991) 11:1095–116. doi: 10.1523/JNEUROSCI.11-04-01095.1991
292. Burkhalter A. Development of forward and feedback connections between areas V1 and V2 of human visual cortex. Cereb Cortex. (1993) 3:476–87. doi: 10.1093/cercor/3.5.476
293. Innocenti GM. Development and evolution: two determinants of cortical connectivity. Prog Brain Res. (2011) 189:65–75. doi: 10.1016/B978-0-444-53884-0.00018-X
294. Webster MJ, Elashoff M, Weickert CS. Molecular evidence that cortical synaptic growth predominates during the first decade of life in humans. Int J Dev Neurosci. (2011) 29:225–36. doi: 10.1016/j.ijdevneu.2010.09.006
295. Gogtay N, Giedd JN, Lusk L, Hayashi KM, Greenstein D, Vaituzis AC, et al. Dynamic mapping of human cortical development during childhood through early adulthood. Proc Natl Acad Sci USA. (2004) 101:8174–9. doi: 10.1073/pnas.0402680101
296. Shaw P, Greenstein D, Lerch J, Clasen L, Lenroot R, Gogtay N, et al. Intellectual ability and cortical development in children and adolescents. Nature. (2006) 440:676–9. doi: 10.1038/nature04513
297. Whitford TJ, Rennie CJ, Grieve SM, Clark CR, Gordon E, Williams LM. Brain maturation in adolescence: concurrent changes in neuroanatomy and neurophysiology. Hum Brain Mapp. (2007) 28:228–37. doi: 10.1002/hbm.20273
298. Harris LW, Lockstone HE, Khaitovich P, Weickert CS, Webster MJ, Bahn S. Gene expression in the prefrontal cortex during adolescence: implications for the onset of schizophrenia. BMC Med Genomics. (2009) 2:28. doi: 10.1186/1755-8794-2-28
299. Asato MR, Terwilliger R, Woo J, Luna B. White matter development in adolescence: a DTI study. Cereb Cortex. (2010) 20:2122–31. doi: 10.1093/cercor/bhp282
300. Feinberg I, Campbell IG. Sleep EEG changes during adolescence: an index of a fundamental brain reorganization. Brain Cogn. (2010) 72:56–65. doi: 10.1016/j.bandc.2009.09.008
301. Luna B, Padmanabhan A, O'hearn K. What has fMRI told us about the development of cognitive control through adolescence? Brain Cogn. (2010) 72:101–13. doi: 10.1016/j.bandc.2009.08.005
302. Jolles DD, Van Buchem MA, Crone EA, Rombouts SA. A comprehensive study of whole-brain functional connectivity in children and young adults. Cereb Cortex. (2011) 21:385–91. doi: 10.1093/cercor/bhq104
303. Sakai T, Mikami A, Tomonaga M, Matsui M, Suzuki J, Hamada Y, et al. Differential prefrontal white matter development in chimpanzees and humans. Curr Biol. (2011) 21:1397–402. doi: 10.1016/j.cub.2011.07.019
304. Liu X, Somel M, Tang L, Yan Z, Jiang X, Guo S, et al. Extension of cortical synaptic development distinguishes humans from chimpanzees and macaques. Genome Res. (2012) 22:611–22. doi: 10.1101/gr.127324.111
305. Brant AM, Munakata Y, Boomsma DI, Defries JC, Haworth CMA, Keller MC, et al. The Nature and nurture of high IQ: an extended sensitive period for intellectual development. Psychol Sci. (2013) 24:1487–95. doi: 10.1177/0956797612473119
306. Kadosh KC, Linden DEJ, Lau JYF. Plasticity during childhood and adolescence: innovative approaches to investigating neurocognitive development. Dev Sci. (2013) 16:574–83. doi: 10.1111/desc.12054
307. Beveridge NJ, Santarelli DM, Wang X, Tooney PA, Webster MJ, Weickert CS, et al. Maturation of the human dorsolateral prefrontal cortex coincides with a dynamic shift in microRNA expression. Schizophr Bull. (2014) 40:399–409. doi: 10.1093/schbul/sbs198
308. Blakemore SJ, Mills KL. Is adolescence a sensitive period for sociocultural processing? Ann Rev Psychol. (2014) 65:187–207. doi: 10.1146/annurev-psych-010213-115202
309. Caballero A, Granberg R, Tseng KY. Mechanisms contributing to prefrontal cortex maturation during adolescence. Neurosci Biobehav Rev. (2016) 70:4–12. doi: 10.1016/j.neubiorev.2016.05.013
310. Mills KL, Goddings AL, Herting MM, Meuwese R, Blakemore SJ, Crone EA, et al. Structural brain development between childhood and adulthood: convergence across four longitudinal samples. Neuroimage. (2016) 141:273–81. doi: 10.1016/j.neuroimage.2016.07.044
311. Morita T, Asada M, Naito E. Contribution of neuroimaging studies to understanding development of human cognitive brain functions. Front Hum Neurosci. (2016) 10:464. doi: 10.3389/fnhum.2016.00464
312. Clawson A, Clayson PE, Keith CM, Catron C, Larson MJ. Conflict and performance monitoring throughout the lifespan: an event-related potential (ERP) and temporospatial component analysis. Biol Psychol. (2017) 124:87–99. doi: 10.1016/j.biopsycho.2017.01.012
313. Forde NJ, Ronan L, Zwiers MP, Schweren LJS, Alexander-Bloch AF, Franke B, et al. Healthy cortical development through adolescence and early adulthood. Brain Struct Funct. (2017) 222:3653–63. doi: 10.1007/s00429-017-1424-0
314. Tamnes CK, Herting MM, Goddings AL, Meuwese R, Blakemore SJ, Dahl RE, et al. Development of the cerebral cortex across adolescence: a multisample study of inter-related longitudinal changes in cortical volume, surface area, and thickness. J Neurosci. (2017) 37:3402–12. doi: 10.1523/JNEUROSCI.3302-16.2017
315. Larsen B, Luna B. Adolescence as a neurobiological critical period for the development of higher-order cognition. Neurosci Biobehav Rev. (2018) 94:179–95. doi: 10.1016/j.neubiorev.2018.09.005
316. Sousa SS, Amaro E, Crego A, Goncalves OF, Sampaio A. Developmental trajectory of the prefrontal cortex: a systematic review of diffusion tensor imaging studies. Brain Imaging Behav. (2018) 12:1197–210. doi: 10.1007/s11682-017-9761-4
317. Tousignant B, Eugene F, Sirois K, Jackson PL. Difference in neural response to social exclusion observation and subsequent altruism between adolescents and adults. Neuropsychologia. (2018) 116:15–25. doi: 10.1016/j.neuropsychologia.2017.04.017
318. Changeux JP, Danchin A. Selective stabilisation of developing synapses as a mechanism for the specification of neuronal networks. Nature. (1976) 264:705–12. doi: 10.1038/264705a0
319. Purves D, Lichtman JW. Elimination of synapses in the developing nervous system. Science. (1980) 210:153–7. doi: 10.1126/science.7414326
320. He H, Mahnke AH, Doyle S, Fan N, Wang CC, Hall BJ, et al. Neurodevelopmental role for VGLUT2 in pyramidal neuron plasticity, dendritic refinement, and in spatial learning. J Neurosci. (2012) 32:15886–901. doi: 10.1523/JNEUROSCI.4505-11.2012
321. Selemon LD. A role for synaptic plasticity in the adolescent development of executive function. Transl Psychiatry. (2013) 3:e238. doi: 10.1038/tp.2013.7
322. Selemon LD. Frontal lobe synaptic plasticity in development and disease: modulation by the dopamine D1 receptor. Curr Pharm Des. (2014) 20:5194–201. doi: 10.2174/1381612819666140110122307
323. Barger N, Sheley MF, Schumann CM. Stereological study of pyramidal neurons in the human superior temporal gyrus from childhood to adulthood. J Comp Neurol. (2015) 523:1054–72. doi: 10.1002/cne.23707
324. Navlakha S, Barth AL, Bar-Joseph Z. Decreasing-rate pruning optimizes the construction of efficient and robust distributed networks. PLoS Comput Biol. (2015) 11:e1004347. doi: 10.1371/journal.pcbi.1004347
325. Hoel EP, Albantakis L, Cirelli C, Tononi G. Synaptic refinement during development and its effect on slow-wave activity: a computational study. J Neurophysiol. (2016) 115:2199–213. doi: 10.1152/jn.00812.2015
326. Ju HR, Colbert CM, Levy WB. Limited synapse overproduction can speed development but sometimes with long-term energy and discrimination penalties. PLoS Comput Biol. (2017) 13:e1005750. doi: 10.1371/journal.pcbi.1005750
327. Selemon LD, Zecevic N. Schizophrenia: a tale of two critical periods for prefrontal cortical development. Transl Psychiatry. (2015) 5:e623. doi: 10.1038/tp.2015.115
328. Breen MS, Ozcan S, Ramsey JM, Wang Z, Ma'ayan A, Rustogi N, et al. Temporal proteomic profiling of postnatal human cortical development. Transl Psychiatry. (2018) 8:267. doi: 10.1038/s41398-018-0306-4
329. Shapiro LP, Parsons RG, Koleske AJ, Gourley SL. Differential expression of cytoskeletal regulatory factors in the adolescent prefrontal cortex: implications for cortical development. J Neurosci Res. (2017) 95:1123–43. doi: 10.1002/jnr.23960
330. Kroeze Y, Oti M, Van Beusekom E, Cooijmans RHM, Van Bokhoven H, Kolk SM, et al. Transcriptome analysis identifies multifaceted regulatory mechanisms dictating a genetic switch from neuronal network establishment to maintenance during postnatal prefrontal cortex development. Cereb Cortex. (2018) 28:833–51. doi: 10.1093/cercor/bhw407
331. Jacobs B, Chugani HT, Allada V, Chen S, Phelps ME, Pollack DB, et al. Developmental changes in brain metabolism in sedated rhesus macaques and vervet monkeys revealed by positron emission tomography. Cereb Cortex. (1995) 5:222–33. doi: 10.1093/cercor/5.3.222
332. Sherwood CC, Gomez-Robles A. Brain plasticity and human evolution. Annu Rev Anthropol Vol. (2017) 46:399–419. doi: 10.1146/annurev-anthro-102215-100009
333. Defelipe J, Elston GN, Fujita I, Fuster J, Harrison KH, Hof PR, et al. Neocortical circuits: evolutionary aspects and specificity versus non-specificity of synaptic connections. Remarks, main conclusions and general comments and discussion J Neurocytol. (2002) 31:387–416. doi: 10.1023/A:1024142513991
334. Datta D, Arnsten AFT. Unique molecular regulation of higher-order prefrontal cortical circuits: insights into the neurobiology of Schizophrenia. ACS Chem Neurosci. (2018) 9:2127–45. doi: 10.1021/acschemneuro.7b00505
335. Cossart R, Petanjek Z, Dumitriu D, Hirsch JC, Ben-Ari Y, Esclapez M, et al. Interneurons targeting similar layers receive synaptic inputs with similar kinetics. Hippocampus. (2006) 16:408–20. doi: 10.1002/hipo.20169
336. Minshew NJ, Johnson C, Luna B. The cognitive and neural basis of autism: a disorder of complex information processing and dysfunction of neocortical systems. Int Rev Res Mental Retard. (2000) 23:111–138. doi: 10.1016/S0074-7750(00)80008-7
337. Lewis JD, Evans AC, Pruett JRJr, Botteron KN, Mckinstry RC, Zwaigenbaum L, et al. The emergence of network inefficiencies in infants with autism spectrum disorder. Biol Psychiatry. (2017) 82:176–85. doi: 10.1016/j.biopsych.2017.03.006
338. Maski KP, Jeste SS, Spence SJ. Common neurological co-morbidities in autism spectrum disorders. Curr Opin Pediatr. (2011) 23:609–15. doi: 10.1097/MOP.0b013e32834c9282
339. Rosen TE, Mazefsky CA, Vasa RA, Lerner MD. Co-occurring psychiatric conditions in autism spectrum disorder. Int Rev Psychiatry. (2018) 30:40–61. doi: 10.1080/09540261.2018.1450229
340. Carulla LS, Reed GM, Vaez-Azizi LM, Cooper S-A, Leal RM, Bertelli M, et al. Intellectual developmental disorders: towards a new name, definition and framework for “mental retardation/intellectual disability” in ICD-11. World Psychiatry. (2011) 10:175–80. doi: 10.1002/j.2051-5545.2011.tb00045.x
341. Viscidi EW, Triche EW, Pescosolido MF, Mclean RL, Joseph RM, Spence SJ, et al. Clinical characteristics of children with autism spectrum disorder and co-occurring epilepsy. PLoS ONE. (2013) 8:e67797. doi: 10.1371/journal.pone.0067797
342. Ventola P, Saulnier CA, Steinberg E, Chawarska K, Klin A. Early-emerging social adaptive skills in toddlers with autism spectrum disorders: an item analysis. J Autism Dev Disord. (2014) 44:283–93. doi: 10.1007/s10803-011-1278-x
343. Costanzo V, Chericoni N, Amendola FA, Casula L, Muratori F, Scattoni ML, et al. Early detection of autism spectrum disorders: from retrospective home video studies to prospective 'high risk' sibling studies. Neurosci Biobehav Rev. (2015) 55:627–35. doi: 10.1016/j.neubiorev.2015.06.006
344. Bedford R, Gliga T, Shephard E, Elsabbagh M, Pickles A, Charman T, et al. Neurocognitive and observational markers: prediction of autism spectrum disorder from infancy to mid-childhood. Mol Autism. (2017) 8:49. doi: 10.1186/s13229-017-0167-3
345. Bacon EC, Courchesne E, Barnes CC, Cha D, Pence S, Schreibman L, et al. Rethinking the idea of late autism spectrum disorder onset. Dev Psychopathol. (2018) 30:553–69. doi: 10.1017/S0954579417001067
346. Emerson RW, Adams C, Nishino T, Hazlett HC, Wolff JJ, Zwaigenbaum L, et al. Functional neuroimaging of high-risk 6-month-old infants predicts a diagnosis of autism at 24 months of age. Sci Transl Med. (2017) 9:eaag2882. doi: 10.1126/scitranslmed.aag2882
347. Levin AR, Varcin KJ, O'leary HM, Tager-Flusberg H, Nelson CA. EEG power at 3 months in infants at high familial risk for autism. J Neurodev Disord. (2017) 9:34. doi: 10.1186/s11689-017-9214-9
348. Raznahan A. Sizing up the search for autism spectrum disorder (ASD) risk markers during prenatal and early postnatal life. J Am Acad Child Adolesc Psychiatry. (2014) 53:1045–7. doi: 10.1016/j.jaac.2014.07.010
349. Ben-Ari Y. Is birth a critical period in the pathogenesis of autism spectrum disorders? Nat Rev Neurosci. (2015) 16:498–505. doi: 10.1038/nrn3956
350. Kostovic I, Sedmak G, Vuksic M, Judas M. The relevance of human fetal subplate zone for developmental neuropathology of neuronal migration disorders and cortical dysplasia. CNS Neurosci Ther. (2015) 21:74–82. doi: 10.1111/cns.12333
351. Pineda R, Melchior K, Oberle S, Inder T, Rogers C. Assessment of autism symptoms during the neonatal period: is there early evidence of autism risk? Am J Occup Ther. (2015) 69:6904220010p1-11. doi: 10.5014/ajot.2015.015925
352. Munoz-Moreno E, Fischi-Gomez E, Batalle D, Borradori-Tolsa C, Eixarch E, Thiran JP, et al. Structural brain network reorganization and social cognition related to adverse perinatal condition from infancy to early adolescence. Front Neurosci. (2016) 10:560. doi: 10.3389/fnins.2016.00560
353. Hazlett HC, Gu H, Munsell BC, Kim SH, Styner M, Wolff JJ, et al. Early brain development in infants at high risk for autism spectrum disorder. Nature. (2017) 542:348–51. doi: 10.1038/nature21369
354. Guisso DR, Saadeh FS, Saab D, El Deek J, Chamseddine S, El Hassan HA, et al. Association of autism with maternal infections, perinatal and other risk factors: a case-control study. J Autism Dev Disord. (2018) 48:2010–21. doi: 10.1007/s10803-017-3449-x
355. Sestan N, State MW. Lost in translation: traversing the complex path from genomics to therapeutics in autism spectrum disorder. Neuron. (2018) 100:406–23. doi: 10.1016/j.neuron.2018.10.015
356. Raznahan A, Greenstein D, Lee NR, Clasen LS, Giedd JN. Prenatal growth in humans and postnatal brain maturation into late adolescence. Proc Natl Acad Sci USA. (2012) 109:11366–71. doi: 10.1073/pnas.1203350109
357. Vivanti G, Mccormick C, Young GS, Abucayan F, Hatt N, Nadig A, et al. Intact and impaired mechanisms of action understanding in autism. Dev Psychol. (2011) 47:841–56. doi: 10.1037/a0023105
358. Bosl WJ, Tager-Flusberg H, Nelson CA. EEG analytics for early detection of autism spectrum disorder: a data-driven approach. Sci Rep. (2018) 8:6828. doi: 10.1038/s41598-018-24318-x
359. Ben-Ari Y, Spitzer NC. Nature and nurture in brain development. Trends Neurosci. (2004) 27:361. doi: 10.1016/j.tins.2004.05.007
360. Petanjek Z, Kostovic I. Epigenetic regulation of fetal brain development and neurocognitive outcome. Proc Natl Acad Sci USA. (2012) 109:11062–3. doi: 10.1073/pnas.1208085109
361. Sheridan MA, Mclaughlin KA. Dimensions of early experience and neural development: deprivation and threat. Trends Cogn Sci. (2014) 18:580–5. doi: 10.1016/j.tics.2014.09.001
362. D'ambrosio P, Colage I. Extending epigenesis: from phenotypic plasticity to the bio-cultural feedback. Biol Philos. (2017) 32:705–28. doi: 10.1007/s10539-017-9581-3
363. Jha SC, Xia K, Ahn M, Girault JB, Li G, Wang L, et al. Environmental influences on infant cortical thickness and surface area. Cereb Cortex. (2018) 29:1139–49. doi: 10.1093/cercor/bhy020
364. Leijser LM, Siddiqi A, Miller SP. Imaging evidence of the effect of socio-economic status on brain structure and development. Semin Pediatr Neurol. (2018) 27:26–34. doi: 10.1016/j.spen.2018.03.004
365. St Pourcain B, Eaves LJ, Ring SM, Fisher SE, Medland S, Evans DM, et al. Developmental changes within the genetic architecture of social communication behavior: a multivariate study of genetic variance in unrelated individuals. Biol Psychiatry. (2018) 83:598–606. doi: 10.1016/j.biopsych.2017.09.020
366. Rajkowska G, Goldman-Rakic PS. Cytoarchitectonic definition of prefrontal areas in the normal human cortex: II. variability in locations of areas 9 and 46 and relationship to the talairach coordinate system. Cereb Cortex. (1995) 5:323–37. doi: 10.1093/cercor/5.4.323
367. Amunts K, Schleicher A, Burgel U, Mohlberg H, Uylings HB, Zilles K. Broca's region revisited: cytoarchitecture and intersubject variability. J Comp Neurol. (1999) 412:319–41. doi: 10.1002/(SICI)1096-9861(19990920)412:2<319::AID-CNE10>3.0.CO;2-7
368. Uylings HB, Rajkowska G, Sanz-Arigita E, Amunts K, Zilles K. Consequences of large interindividual variability for human brain atlases: converging macroscopical imaging and microscopical neuroanatomy. Anat Embryol. (2005) 210:423–31. doi: 10.1007/s00429-005-0042-4
369. Jacobs B, Schall M, Scheibel AB. A quantitative dendritic analysis of Wernicke's area in humans. II gender, hemispheric, and environmental factors J Comp Neurol. (1993) 327:97–111. doi: 10.1002/cne.903270108
370. Yang G, Pan F, Gan WB. Stably maintained dendritic spines are associated with lifelong memories. Nature. (2009) 462:920–4. doi: 10.1038/nature08577
371. Lin YC, Koleske AJ. Mechanisms of synapse and dendrite maintenance and their disruption in psychiatric and neurodegenerative disorders. Annu Rev Neurosci. (2010) 33:349–78. doi: 10.1146/annurev-neuro-060909-153204
372. Chugani HT, Behen ME, Muzik O, Juhasz C, Nagy F, Chugani DC. Local brain functional activity following early deprivation: a study of postinstitutionalized Romanian orphans. Neuroimage. (2001) 14:1290–301. doi: 10.1006/nimg.2001.0917
373. Bruno RM, Hahn TT, Wallace DJ, De Kock CP, Sakmann B. Sensory experience alters specific branches of individual corticocortical axons during development. J Neurosci. (2009) 29:3172–81. doi: 10.1523/JNEUROSCI.5911-08.2009
374. Kolb B, Mychasiuk R, Muhammad A, Li YL, Frost DO, Gibb R. Experience and the developing prefrontal cortex. Proc Natl Acad Sci USA. (2012) 109:17186–93. doi: 10.1073/pnas.1121251109
375. Mills KL, Goddings AL, Clasen LS, Giedd JN, Blakemore SJ. The developmental mismatch in structural brain maturation during adolescence. Dev Neurosci. (2014) 36:147–60. doi: 10.1159/000362328
376. Kok R, Thijssen S, Bakermans-Kranenburg MJ, Jaddoe VW, Verhulst FC, White T, et al. Normal variation in early parental sensitivity predicts child structural brain development. J Am Acad Child Adolesc Psychiatry. (2015) 54:824–31 e1. doi: 10.1016/j.jaac.2015.07.009
377. Lee YA, Goto Y. Prefrontal cortical dopamine from an evolutionary perspective. Neurosci Bull. (2015) 31:164–74. doi: 10.1007/s12264-014-1499-z
378. Leblanc E, Degeilh F, Daneault V, Beauchamp MH, Bernier A. Attachment security in infancy: a preliminary study of prospective links to brain morphometry in late childhood. Front Psychol. (2017) 8:2141. doi: 10.3389/fpsyg.2017.02141
379. Sethna V, Pote I, Wang S, Gudbrandsen M, Blasi A, Mccusker C, et al. Mother-infant interactions and regional brain volumes in infancy: an MRI study. Brain Struct Funct. (2017) 222:2379–88. doi: 10.1007/s00429-016-1347-1
380. Shen KK, Dore V, Rose S, Fripp J, Mcmahon KL, De Zubicaray GI, et al. Heritability and genetic correlation between the cerebral cortex and associated white matter connections. Hum Brain Mapp. (2016) 37:2331–47. doi: 10.1002/hbm.23177
381. Van Dyck LI, Morrow EM. Genetic control of postnatal human brain growth. Curr Opin Neurol. (2017) 30:114–24. doi: 10.1097/WCO.0000000000000405
382. Patel S, Patel R, Park MTM, Masellis M, Knight J, Chakravarty MM. Heritability estimates of cortical anatomy: the influence and reliability of different estimation strategies. Neuroimage. (2018) 178:78–91. doi: 10.1016/j.neuroimage.2018.05.014
383. Parsons CE, Young KS, Murray L, Stein A, Kringelbach ML. The functional neuroanatomy of the evolving parent-infant relationship. Prog Neurobiol. (2010) 91:220–41. doi: 10.1016/j.pneurobio.2010.03.001
384. Shaw P, Gilliam M, Malek M, Rodriguez N, Greenstein D, Clasen L, et al. Parental age effects on cortical morphology in offspring. Cereb Cortex. (2012) 22:1256–62. doi: 10.1093/cercor/bhr194
385. Weinberger DR. Implications of normal brain development for the pathogenesis of schizophrenia. Arch Gen Psychiatry. (1987) 44:660–9. doi: 10.1001/archpsyc.1987.01800190080012
386. Kishiyama MM, Boyce WT, Jimenez AM, Perry LM, Knight RT. Socioeconomic disparities affect prefrontal function in children. J Cogn Neurosci. (2009) 21:1106–15. doi: 10.1162/jocn.2009.21101
387. Braun K, Bock J. The experience-dependent maturation of prefronto-limbic circuits and the origin of developmental psychopathology: implications for the pathogenesis and therapy of behavioural disorders. Dev Med Child Neurol. (2011) 53 (Suppl. 4):14–8. doi: 10.1111/j.1469-8749.2011.04056.x
388. Billeci L, Calderoni S, Conti E, Gesi C, Carmassi C, Dell'osso L, et al. The broad autism (endo)phenotype: neurostructural and neurofunctional correlates in parents of individuals with autism spectrum disorders. Front Neurosci. (2016) 10:346. doi: 10.3389/fnins.2016.00346
389. Dell'osso L, Luche RD, Gesi C, Moroni I, Carmassi C, Maj M. From Asperger's autistischen psychopathen to DSM-5 autism spectrum disorder and beyond: a subthreshold autism spectrum model. Clin Pract Epidemiol Ment Health. (2016) 12:120–31. doi: 10.2174/1745017901612010120
390. Rubenstein E, Chawla D. Broader autism phenotype in parents of children with autism: a systematic review of percentage estimates. J Child Fam Stud. (2018) 27:1705–20. doi: 10.1007/s10826-018-1026-3
391. Ozonoff S, Young GS, Brian J, Charman T, Shephard E, Solish A, et al. Diagnosis of autism spectrum disorder after age 5 in children evaluated longitudinally since infancy. J Am Acad Child Adolesc Psychiatry. (2018) 57:849–57 e2. doi: 10.1016/j.jaac.2018.06.022
392. Karahanoglu FI, Baran B, Nguyen QTH, Meskaldji DE, Yendiki A, Vangel M, et al. Diffusion-weighted imaging evidence of altered white matter development from late childhood to early adulthood in autism spectrum disorder. Neuroimage Clin. (2018) 19:840–7. doi: 10.1016/j.nicl.2018.06.002
393. Mooney-Leber SM, Gould TJ. The long-term cognitive consequences of adolescent exposure to recreational drugs of abuse. Learn Mem. (2018) 25:481–91. doi: 10.1101/lm.046672.117
394. Stein DS, Welchons LW, Corley KB, Dickinson H, Levin AR, Nelson CA, et al. Autism associated with early institutionalization, high intelligence, and naturalistic behavior therapy in a 7-year-old boy. J Dev Behav Pediatr. (2015) 36:53–5. doi: 10.1097/DBP.0000000000000120
395. Piccolo LR, Merz EC, He XF, Sowell ER, Noble KG, Pediat Imaging N. Age-related differences in cortical thickness vary by socioeconomic status. PLoS ONE. (2016) 11:e0162511. doi: 10.1371/journal.pone.0162511
396. Meins E, Fernyhough C, Arnott B, Leekam SR, De Rosnay M. Mind-mindedness and theory of mind: mediating roles of language and perspectival symbolic play. Child Dev. (2013) 84:1777–90. doi: 10.1111/cdev.12061
397. Longobardi E, Spataro P, Colonnesi C. Maternal communicative functions and mind-mindedness at 16 months as predictors of children's internal and non-internal language at 20 months. Infant Behav Dev. (2018) 50:52–63. doi: 10.1016/j.infbeh.2017.11.003
398. Hoogenhout M, Malcolm-Smith S. Theory of mind predicts severity level in autism. Autism. (2017) 21:242–52. doi: 10.1177/1362361316636758
399. Hashimoto T, Tayama M, Murakawa K, Yoshimoto T, Miyazaki M, Harada M, et al. Development of the brainstem and cerebellum in autistic patients. J Autism Dev Disord. (1995) 25:1–18. doi: 10.1007/BF02178163
400. Kemper TL, Bauman M. Neuropathology of infantile autism. J Neuropathol Exp Neurol. (1998) 57:645–52. doi: 10.1097/00005072-199807000-00001
401. Skefos J, Cummings C, Enzer K, Holiday J, Weed K, Levy E, et al. Regional alterations in purkinje cell density in patients with autism. PLoS ONE. (2014) 9:e81255. doi: 10.1371/journal.pone.0081255
402. Shen MD, Li DD, Keown CL, Lee A, Johnson RT, Angkustsiri K, et al. Functional connectivity of the amygdala is disrupted in preschool-aged children with autism spectrum disorder. J Am Acad Child Adolesc Psychiatry. (2016) 55:817–24. doi: 10.1016/j.jaac.2016.05.020
403. Casanova MF, El-Baz A, Vanbogaert E, Narahari P, Switala A. A topographic study of minicolumnar core width by lamina comparison between autistic subjects and controls: possible minicolumnar disruption due to an anatomical element in-common to multiple laminae. Brain Pathol. (2010) 20:451–8. doi: 10.1111/j.1750-3639.2009.00319.x
404. Morgan JT, Chana G, Abramson I, Semendeferi K, Courchesne E, Everall IP. Abnormal microglial-neuronal spatial organization in the dorsolateral prefrontal cortex in autism. Brain Res. (2012) 1456:72–81. doi: 10.1016/j.brainres.2012.03.036
405. Courchesne E, Mouton PR, Calhoun ME, Semendeferi K, Ahrens-Barbeau C, Hallet MJ, et al. Neuron number and size in prefrontal cortex of children with autism. JAMA. (2011) 306:2001–10. doi: 10.1001/jama.2011.1638
406. Karst AT, Hutsler JJ. Two-dimensional analysis of the supragranular layers in autism spectrum disorder. Res Autism Spectr Disord. (2016) 32:96–105. doi: 10.1016/j.rasd.2016.09.004
407. Kim E, Camacho J, Combs Z, Ariza J, Lechpammer M, Noctor SC, et al. Preliminary findings suggest the number and volume of supragranular and infragranular pyramidal neurons are similar in the anterior superior temporal area of control subjects and subjects with autism. Neurosci Lett. (2015) 589:98–103. doi: 10.1016/j.neulet.2015.01.021
408. Sparks BF, Friedman SD, Shaw DW, Aylward EH, Echelard D, Artru AA, et al. Brain structural abnormalities in young children with autism spectrum disorder. Neurology. (2002) 59:184–92. doi: 10.1212/WNL.59.2.184
409. Stoner R, Chow ML, Boyle MP, Sunkin SM, Mouton PR, Roy S, et al. Patches of disorganization in the neocortex of children with autism. N Engl J Med. (2014) 370:1209–19. doi: 10.1056/NEJMoa1307491
410. Simms ML, Kemper TL, Timbie CM, Bauman ML, Blatt GJ. The anterior cingulate cortex in autism: heterogeneity of qualitative and quantitative cytoarchitectonic features suggests possible subgroups. Acta Neuropathol. (2009) 118:673–84. doi: 10.1007/s00401-009-0568-2
411. Williams RS, Hauser SL, Purpura DP, Delong GR, Swisher CN. Autism and mental retardation: neuropathologic studies performed in four retarded persons with autistic behavior. Arch Neurol. (1980) 37:749–53. doi: 10.1001/archneur.1980.00500610029003
412. Schumann CM, Amaral DG. Stereological analysis of amygdala neuron number in autism. J Neurosci. (2006) 26:7674–9. doi: 10.1523/JNEUROSCI.1285-06.2006
413. Jacot-Descombes S, Uppal N, Wicinski B, Santos M, Schmeidler J, Giannakopoulos P, et al. Decreased pyramidal neuron size in Brodmann areas 44 and 45 in patients with autism. Acta Neuropathol. (2012) 124:67–79. doi: 10.1007/s00401-012-0976-6
414. Casanova MF, Van Kooten IA, Switala AE, Van Engeland H, Heinsen H, Steinbusch HW, et al. Minicolumnar abnormalities in autism. Acta Neuropathol. (2006) 112:287–303. doi: 10.1007/s00401-006-0085-5
415. Van Kooten I. A. J., Palmen, S. J. M. C., Von Cappeln P, Steinbusch HWM, Korr H, et al. Neurons in the fusiform gyrus are fewer and smaller in autism. Brain. (2008) 131:987–99. doi: 10.1093/brain/awn033
416. Raymond GV, Bauman ML, Kemper TL. Hippocampus in autism: a Golgi analysis. Acta Neuropathol. (1996) 91:117–9. doi: 10.1007/s004010050401
417. Uppal N, Wicinski B, Buxbaum JD, Heinsen H, Schmitz C, Hof PR. Neuropathology of the anterior midcingulate cortex in young children with autism. J Neuropathol Exp Neurol. (2014) 73:891–902. doi: 10.1097/NEN.0000000000000108
418. Wegiel J, Flory M, Schanen NC, Cook EH, Nowicki K, Kuchna I, et al. Significant neuronal soma volume deficit in the limbic system in subjects with 15q11.2-q13 duplications. Acta Neuropathol Commun. (2015) 3:63. doi: 10.1186/s40478-015-0241-z
419. Bailey A, Luthert P, Dean A, Harding B, Janota I, Montgomery M, et al. A clinicopathological study of autism. Brain. (1998) 121:889–905. doi: 10.1093/brain/121.5.889
420. Hutsler JJ, Zhang H. Increased dendritic spine densities on cortical projection neurons in autism spectrum disorders. Brain Res. (2010) 1309:83–94. doi: 10.1016/j.brainres.2009.09.120
421. Frith C. Is autism a disconnection disorder? Lancet Neurol. (2004) 3:577. doi: 10.1016/S1474-4422(04)00875-0
422. Lee S, Zhang HY, Webb DJ. Dendritic spine morphology and dynamics in health and disease. Cell Health Cytoskeleton. (2015) 7:121–31. doi: 10.2147/CHC.S82214
423. Martinez-Cerdeno V. Dendrite and spine modifications in autism and related neurodevelopmental disorders in patients and animal models. Dev Neurobiol. (2017) 77:393–404. doi: 10.1002/dneu.22417
424. Forrest MP, Parnell E, Penzes P. Dendritic structural plasticity and neuropsychiatric disease. Nat Rev Neurosci. (2018) 19:215–34. doi: 10.1038/nrn.2018.16
425. Kennedy DP, Semendeferi K, Courchesne E. No reduction of spindle neuron number in frontoinsular cortex in autism. Brain Cogn. (2007) 64:124–9. doi: 10.1016/j.bandc.2007.01.007
426. Blatt GJ, Fatemi SH. Alterations in GABAergic biomarkers in the autism brain: research findings and clinical implications. Anat Rec (Hoboken). (2011) 294:1646–52. doi: 10.1002/ar.21252
427. Filice F, Vörckel KJ, Sungur AÖ, Wöhr M, Schwaller B. Reduction in parvalbumin expression not loss of the parvalbumin-expressing GABA interneuron subpopulation in genetic parvalbumin and shank mouse models of autism. Mol Brain. (2016) 9:10. doi: 10.1186/s13041-016-0192-8
428. Hashemi E, Ariza J, Rogers H, Noctor SC, Martinez-Cerdeno V. The number of parvalbumin-expressing interneurons is decreased in the prefrontal cortex in autism. Cereb Cortex. (2018) 28:690. doi: 10.1093/cercor/bhx063
429. Melchitzky DS, Sesack SR, Lewis DA. Parvalbumin-immunoreactive axon terminals in macaque monkey and human prefrontal cortex: laminar, regional, and target specificity of type I and type II synapses. J Comp Neurol. (1999) 408:11–22. doi: 10.1002/(SICI)1096-9861(19990524)408:1<11::AID-CNE2>3.0.CO;2-T
430. Povysheva NV, Zaitsev AV, Rotaru DC, Gonzalez-Burgos G, Lewis DA, Krimer LS. Parvalbumin-positive basket interneurons in monkey and rat prefrontal cortex. J Neurophysiol. (2008) 100:2348–60. doi: 10.1152/jn.90396.2008
431. Dienel SJ, Lewis DA. Alterations in cortical interneurons and cognitive function in schizophrenia. Neurobiol Dis. (2018) doi: 10.1016/j.nbd.2018.06.020. [Epub ahead of print].
432. Chung DW, Wills ZP, Fish KN, Lewis DA. Developmental pruning of excitatory synaptic inputs to parvalbumin interneurons in monkey prefrontal cortex. Proc Natl Acad Sci USA. (2017) 114:E629–37. doi: 10.1073/pnas.1610077114
433. Ariza J, Rogers H, Hashemi E, Noctor SC, Martinez-Cerdeno V. The number of chandelier and basket cells are differentially decreased in prefrontal cortex in autism. Cereb Cortex. (2018) 28:411–20. doi: 10.1093/cercor/bhw349
434. Kostovic I, Judas M, Kostovic-Knezevic L, Simic G, Delalle I, Chudy D, et al. Zagreb research collection of human brains for developmental neurobiologists and clinical neuroscientists. Int J Dev Biol. (1991) 35:215–30.
435. Judas M, Simic G, Petanjek Z, Jovanov-Milosevic N, Pletikos M, Vasung L, et al. The Zagreb Collection of human brains: a unique, versatile, but underexploited resource for the neuroscience community. Ann N Y Acad Sci. (2011) 1225 (Suppl. 1):E105–30. doi: 10.1111/j.1749-6632.2011.05993.x
436. Kruslin B, Dzombeta T, Bezjak M, Sedmak G, Petanjek Z, Simic G, et al. Congenital brain anomalies and chromosomal aberrations from the Zagreb collection of human brains. Transl Neurosci. (2014) 5:293–301. doi: 10.2478/s13380-014-0231-9
437. Szatmari P. A review of the DSM-III-R criteria for autistic disorder. J Autism Dev Disord. (1992) 22:507–23. doi: 10.1007/BF01046325
438. Zeba M, Jovanov-Milosevic N, Petanjek Z. Quantitative analysis of basal dendritic tree of layer III pyramidal neurons in different areas of adult human frontal cortex. Coll Antropol. (2008) 32 (Suppl. 1):161–9.
439. Marin-Padilla M. Abnormal neuronal differentiation (functional maturation) in mental retardation. Birth Defects Orig Artic Ser. (1975) 11:133–53.
440. Purpura DP. Normal and abnormal development of cerebral cortex in man. Neurosci Res Program Bull. (1982) 20:569–77.
441. Defelipe J, Fariñas I. The pyramidal neuron of the cerebral cortex: morphological and chemical characteristics of the synaptic inputs. Prog Neurobiol. (1992) 39:563–607. doi: 10.1016/0301-0082(92)90015-7
442. Nieuwenhuys R. The neocortex. an overview of its evolutionary development, structural organization and synaptology Anat Embryol. (1994) 190:307–37.
443. Shukla DK, Keehn B, Smylie DM, Muller RA. Microstructural abnormalities of short-distance white matter tracts in autism spectrum disorder. Neuropsychologia. (2011) 49:1378–82. doi: 10.1016/j.neuropsychologia.2011.02.022
444. Ouyang MH, Jeon T, Mishra V, Du HX, Wang Y, Peng Y, et al. Global and regional cortical connectivity maturation index (CCMI) of developmental human brain with quantification of short-range association tract. In: Gimi B, Krol A, editors. Medical Imaging 2016-Biomedical Applications in Molecular, Structural, and Functional Imaging. Washington, USA: SPIE (2016). p. 97881B.
445. Hong SJ, Hyung B, Paquola C, Bernhardt BC. The superficial white matter in autism and its role in connectivity anomalies and symptom severity. Cereb Cortex. (2018). doi: 10.1093/cercor/bhy321. [Epub ahead of print].
446. Rapoport JL, Giedd JN, Gogtay N. Neurodevelopmental model of schizophrenia: update 2012. Mol Psychiatry. (2012) 17:1228–38. doi: 10.1038/mp.2012.23
447. Bennett MR. Synapse formation and regression in the cortex during adolescence and in schizophrenia. Med J Aust. (2009) 190:S14–6. doi: 10.5694/j.1326-5377.2009.tb02368.x
448. Boksa P. Abnormal synaptic pruning in schizophrenia: urban myth or reality? J Psychiatry Neurosci. (2012) 37:75–7. doi: 10.1503/jpn.120007
449. Hyde TM, Ziegler JC, Weinberger DR. Psychiatric disturbances in metachromatic leukodystrophy. Insights into the neurobiology of psychosis. Arch Neurol. (1992) 49:401–6. doi: 10.1001/archneur.1992.00530280095028
450. Keshavan MS, Giedd J, Lau JY, Lewis DA, Paus T. Changes in the adolescent brain and the pathophysiology of psychotic disorders. Lancet Psychiatry. (2014) 1:549–58. doi: 10.1016/S2215-0366(14)00081-9
451. Cannon TD. How Schizophrenia Develops: Cognitive and Brain Mechanisms Underlying Onset of Psychosis. Trends Cogn Sci. (2015) 19:744–56. doi: 10.1016/j.tics.2015.09.009
452. Davis J, Eyre H, Jacka FN, Dodd S, Dean O, Mcewen S, et al. A review of vulnerability and risks for schizophrenia: beyond the two hit hypothesis. Neurosci Biobehav Rev. (2016) 65:185–94. doi: 10.1016/j.neubiorev.2016.03.017
453. Forsyth JK, Lewis DA. Mapping the Consequences of Impaired Synaptic Plasticity in schizophrenia through development: an integrative model for diverse clinical features. Trends Cogn Sci. (2017) 21:760–78. doi: 10.1016/j.tics.2017.06.006
454. Maccari S, Polese D, Reynaert ML, Amici T, Morley-Fletcher S, Fagioli F. Early-life experiences and the development of adult diseases with a focus on mental illness: the human birth theory. Neuroscience. (2017) 342:232–51. doi: 10.1016/j.neuroscience.2016.05.042
455. Jones W, Klin A. Heterogeneity and homogeneity across the autism spectrum: the role of development. J Am Acad Child Adolesc Psychiatry. (2009) 48:471–3. doi: 10.1097/CHI.0b013e31819f6c0d
456. Crespi B. Developmental heterochrony and the evolution of autistic perception, cognition and behavior. BMC Med. (2013) 11:119. doi: 10.1186/1741-7015-11-119
457. Ben-Ari Y. Neuro-archaeology: pre-symptomatic architecture and signature of neurological disorders. Trends Neurosci. (2008) 31:626–36. doi: 10.1016/j.tins.2008.09.002
458. Hong SJ, Valk SL, Di Martino A, Milham MP, Bernhardt BC. Multidimensional neuroanatomical subtyping of autism spectrum disorder. Cereb Cortex. (2018) 28:3578–88. doi: 10.1093/cercor/bhx229
459. Pineda RG, Neil J, Dierker D, Smyser CD, Wallendorf M, Kidokoro H, et al. Alterations in brain structure and neurodevelopmental outcome in preterm infants hospitalized in different neonatal intensive care unit environments. J Pediatr. (2014) 164:52–60 e2. doi: 10.1016/j.jpeds.2013.08.047
460. Heijtz RD. Fetal, neonatal, and infant microbiome: perturbations and subsequent effects on brain development and behavior. Semin Fetal Neonatal Med. (2016) 21:410–7. doi: 10.1016/j.siny.2016.04.012
461. Rogers GB, Keating DJ, Young RL, Wong ML, Licinio J, Wesselingh S. From gut dysbiosis to altered brain function and mental illness: mechanisms and pathways. Mol Psychiatry. (2016) 21:738–48. doi: 10.1038/mp.2016.50
462. O'Mahony SM, Clarke G, Dinan TG, Cryan JF. Early-life adversity and brain development: is the microbiome a missing piece of the puzzle? Neuroscience. (2017) 342:37–54. doi: 10.1016/j.neuroscience.2015.09.068
463. Hasan KM, Walimuni IS, Frye RE. Global cerebral and regional multimodal neuroimaging markers of the neurobiology of autism: development and cognition. J Child Neurol. (2013) 28:874–85. doi: 10.1177/0883073812452917
464. Casanova MF, Sokhadze E, Opris I, Wang Y, Li X. Autism spectrum disorders: linking neuropathological findings to treatment with transcranial magnetic stimulation. Acta Paediatr. (2015) 104:346–55. doi: 10.1111/apa.12943
465. Ameis SH, Daskalakis ZJ, Blumberger DM, Desarkar P, Drmic I, Mabbott DJ, et al. Repetitive transcranial magnetic stimulation for the treatment of executive function deficits in autism spectrum disorder: clinical trial approach. J Child Adolesc Psychopharmacol. (2017) 27:413–21. doi: 10.1089/cap.2016.0146
466. Gurau O, Bosl WJ, Newton CR. How useful is electroencephalography in the diagnosis of autism spectrum disorders and the delineation of subtypes: a systematic review. Front Psychiatry. (2017) 8:121. doi: 10.3389/fpsyt.2017.00121
467. Magan-Maganto M, Bejarano-Martin A, Fernandez-Alvarez C, Narzisi A, Garcia-Primo P, Kawa R, et al. Early detection and intervention of ASD: a european overview. Brain Sci. (2017) 7:E159. doi: 10.3390/brainsci7120159
468. Maximo JO, Murdaugh DL, O'kelley S, Kana RK. Changes in intrinsic local connectivity after reading intervention in children with autism. Brain Lang. (2017) 175:11–17. doi: 10.1016/j.bandl.2017.08.008
469. Sathe N, Andrews JC, Mcpheeters ML, Warren ZE. Nutritional and dietary interventions for autism spectrum disorder: a systematic review. Pediatrics. (2017) 139:e20170346. doi: 10.1542/peds.2017-0346
470. Goldberg SB, Tucker RP, Greene PA, Davidson RJ, Wampold BE, Kearney DJ, et al. Mindfulness-based interventions for psychiatric disorders: a systematic review and meta-analysis. Clin Psychol Rev. (2018) 59:52–60. doi: 10.1016/j.cpr.2017.10.011
471. Keefer A, White SW, Vasa RA, Reaven J. Psychosocial interventions for internalizing disorders in youth and adults with ASD. Int Rev Psychiatry. (2018) 30:62–77. doi: 10.1080/09540261.2018.1432575
Keywords: cerebral cortex, theory of mind, cortico-cortical neurons, dendritic development, schizophrenia, excitatory transmission, glutamate
Citation: Petanjek Z, Sedmak D, Džaja D, Hladnik A, Rašin MR and Jovanov-Milosevic N (2019) The Protracted Maturation of Associative Layer IIIC Pyramidal Neurons in the Human Prefrontal Cortex During Childhood: A Major Role in Cognitive Development and Selective Alteration in Autism. Front. Psychiatry 10:122. doi: 10.3389/fpsyt.2019.00122
Received: 30 June 2018; Accepted: 18 February 2019;
Published: 14 March 2019.
Edited by:
Tanja Maria Michel, University of Southern Denmark, DenmarkReviewed by:
Mirolyuba Ilieva, University of Southern Denmark, DenmarkJu Wang, Tianjin Medical University, China
Copyright © 2019 Petanjek, Sedmak, Džaja, Hladnik, Rašin and Jovanov-Milosevic. This is an open-access article distributed under the terms of the Creative Commons Attribution License (CC BY). The use, distribution or reproduction in other forums is permitted, provided the original author(s) and the copyright owner(s) are credited and that the original publication in this journal is cited, in accordance with accepted academic practice. No use, distribution or reproduction is permitted which does not comply with these terms.
*Correspondence: Zdravko Petanjek, emRyYXZrby5wZXRhbmpla0BtZWYuaHI=
†These authors have contributed equally to this work