- 1Huaxi MR Research Center (HMRRC), Department of Radiology, West China Hospital, Sichuan University, Chengdu, China
- 2Department of Radiology, West China Hospital, Sichuan University, Chengdu, China
- 3Department of Psychoradiology, Chengdu Mental Health Center, Chengdu, China
- 4Department of Geriatric Psychiatry, The Fourth People’s Hospital of Chengdu, Chengdu, China
- 5Department of Psychiatry, Zigong Mental Health Center, Zigong, China
Background: Seed-based studies on resting-state functional connectivity (rsFC) in schizophrenia have shown disrupted connectivity involving a number of brain networks; however, the results have been controversial.
Methods: We conducted a meta-analysis based on independent component analysis (ICA) brain templates to evaluate dysconnectivity within resting-state brain networks in patients with schizophrenia. Seventy-six rsFC studies from 70 publications with 2,588 schizophrenia patients and 2,567 healthy controls (HCs) were included in the present meta-analysis. The locations and activation effects of significant intergroup comparisons were extracted and classified based on the ICA templates. Then, multilevel kernel density analysis was used to integrate the results and control bias.
Results: Compared with HCs, significant hypoconnectivities were observed between the seed regions and the areas in the auditory network (left insula), core network (right superior temporal cortex), default mode network (right medial prefrontal cortex, and left precuneus and anterior cingulate cortices), self-referential network (right superior temporal cortex), and somatomotor network (right precentral gyrus) in schizophrenia patients. No hyperconnectivity between the seed regions and any other areas within the networks was detected in patients, compared with the connectivity in HCs.
Conclusions: Decreased rsFC within the self-referential network and default mode network might play fundamental roles in the malfunction of information processing, while the core network might act as a dysfunctional hub of regulation. Our meta-analysis is consistent with diffuse hypoconnectivities as a dysregulated brain network model of schizophrenia.
Introduction
Disrupted resting-state functional connectivity (rsFC) involving a number of brain networks has been suggested as the core pathogenesis of schizophrenia (1–3). Most previous studies tried to identify the specific deficits in neural networks related to the disease, which is crucial not only to understand the mechanism of schizophrenia but also to provide potential biomarkers for clinical use. As the most widely used and simply operated analytic technique, seed-based functional connectivity analysis has demonstrated widespread dysconnectivity in schizophrenia, based on the blood oxygen level-dependent (BOLD) time series extracted from regions of interest (ROIs) as seeds (4).
Despite the growing number of seed-based studies on rsFC in patients with schizophrenia (5–8), the results have been controversial regarding either the location of key regions or the effect of dysconnectivity (i.e., increased or decreased connectivity). Taking the default mode network (DMN) for example, some studies have reported decreased rsFC in schizophrenia patients compared with healthy controls (HCs) between the seed ROI at the posterior cingulate cortex (PCC) and other areas within the same network, including the medial prefrontal, lateral parietal, cerebellar, and insular regions (9, 10). In contrast, other researchers have found increased connectivity within the DMN of patients, although the same seed region had been defined (11, 12). Inconsistency might be affected by studied samples and methodologies; however, seed-based studies pave a promising way to reveal the mechanisms of and biomarkers for schizophrenia (13). Therefore, it is necessary to merge divergent findings into a unified model of network functioning for a better characterization of the rsFC patterns. To fill this gap, meta-analysis could be a robust incorporation strategy by classifying seed ROIs and effects within brain networks (14), which has been proven effective to various studies in psychiatric imaging (15, 16). Recently, Dong and colleagues (17) conducted the first meta-analysis to illustrate rsFC within large-scale brain networks in schizophrenia patients. Based on a priori templates in which specific seed ROIs were selected before analysis (14, 18–20), they found dominant hypoconnectivities within multiple networks. This study helped to conceptualize schizophrenia in terms of whole-brain rsFC; however, it was inherently constrained by a dependence on a priori data and a lack of the simultaneous investigation of brain systems (4, 13, 21).
Alternatively, independent component analysis (ICA) is a common blind source separation approach applied to functional MRI (fMRI) data (22, 23), allowing a fully data-driven exploration of spatiotemporal patterns of synchronized neuronal activity without any predefined seed region (24–26). It creates a reproducible parcellation of functional brain systems, so that bias is reduced while the consideration of data obtained using various atlases is more flexible (25). Using ICA, Mantini and colleagues identified a collection of brain networks based on the correlation between BOLD signals and electroencephalography rhythms (22, 23). These ICA-based networks have previously been used as brain templates in fMRI studies (27, 28), which decomposed the resting-state brain networks into eight spatiotemporal components, as follows: the dorsal attention network (DAN), the central executive network (CEN), the DMN, the core network (CN), the self-referential network (SRN), the somatomotor network (SMN), the visual network (VN), and the auditory network (AN). To our knowledge, no meta-analysis of large-scale rsFC studies in schizophrenia patients has been based on brain network maps using ICA. We aimed to conduct the present meta-analysis to integrate the results of these studies in schizophrenia using the ICA-based brain templates. Our hypothesis was that distributed dysconnectivity would exist within multiple resting-state brain networks in patients with schizophrenia.
Methods
Literature Search
Literature searches were performed by four independent reviewers (SLi, NH, JD, and YG) in PubMed (https://www.ncbi.nlm.nih.gov/pubmed) and Embase (https://www.embase.com) for relevant original articles from January 1, 2007, to April 1, 2018. Earlier studies were excluded to reduce potential confounds due to low gradient fields or traditional analysis methods. The keywords included schizophrenia, rest*(-ing), connect* (-ivity), and functional magnetic resonance imaging, or functional MRI, or fMRI, or magnetic resonance imaging or MRI. In addition, the references within each article were carefully checked for further recruitment. Studies were included if they were 1) original MR studies evaluating seed-based rsFC of whole brain or a specified network to compare schizophrenia patients with HCs and 2) studies that provided seed ROI and peak effect coordinates using Montreal Neurological Institute (MNI) or Talairach coordinates. The exclusion criteria were as follows: 1) the field strength of the MR scanner was less than 1.5 T; 2) seed ROI or peak effect coordinates could not be retrieved; 3) entirely identical samples and seed ROIs were reported in distinct articles; or 4) seed ROIs or investigated networks were beyond the abovementioned eight brain-network templates (22, 23). Reports utilizing the same sample but different seed ROIs were labeled as distinct studies. With the exception of one publication comparing combined patients of two groups (schizophrenia with and without hallucinations) with HCs (29), publications in which different subgroups of schizophrenia were each compared with a single HC group were identified as separate studies.
Data Extraction
The included studies contained the locations and peak effects of significant intergroup differences in rsFC, so the data were extracted and coded by three reviewers (SLi, YT, and DC), as follows, for subsequent meta-analysis. Inconsistencies were resolved by a third assessor (NH).
First, coordinates of each seed ROI and peak effect of each significant intergroup comparison were extracted. If the seed ROI was a spherical area or an anatomical domain from a standard atlas, the region center was calculated to retrieve representative coordinates. For those studies targeting specified networks rather than whole-brain systems, coordinates of each peak location were also collected. All the coordinates and peak effects were converted to the MNI space. Second, we classified the peak coordinates into eight resting-state networks based on previous brain-network templates (22, 23): 1) the DAN, primarily including the left middle and superior occipital gyri, parietal gyrus, inferior and superior parietal gyri, and middle and superior frontal gyri; 2) the CEN, involving the dorsal lateral prefrontal and posterior parietal cortices; 3) the DMN, comprising the PCC/precuneus and the bilateral inferior parietal, middle temporal, angular, superior frontal, and medial frontal gyri; 4) the CN, the key regions of which were the anterior cingulate, bilateral insular cortices, and dorsolateral prefrontal cortex; 5) the SRN, referring to the ventromedial prefrontal and medial orbital prefrontal cortices, gyrus rectus, and pregenual anterior cingulate gyrus; 6) the SMN, relating to the primary sensory-motor cortices, precentral and postcentral gyri, and the supplementary motor area; 7) the VN, encompassing the occipital gyrus and the temporal–occipital regions along with superior parietal gyrus; and 8) the AN, including the bilateral middle and superior temporal gyri, Heschl’s gyrus, and temporal pole (Supplementary Table 1). Third, peak effects were classified into hyperconnectivity or hypoconnectivity in the schizophrenia groups based on the effect direction. Hyperconnectivity was defined as increased rsFC (higher positivity or less negativity of connectivity) in schizophrenia patients compared with HCs; hypoconnectivity was defined as decreased rsFC (less positivity or higher negativity of connectivity) in schizophrenia patients compared with rsFC in HCs.
Multilevel Kernel Density Analysis and Post Hoc Tests
To generalize the results of various studies and control publication bias, two reviewers (WZ and BT) conducted multilevel kernel density analysis (MKDA) using the MKDA toolbox (https://canlabweb.colorado.edu/fmri-resources.html) by treating the proportion of studies that were activated in a region rather than the number of peaks as the test statistic (30). In the MKDA procedure, the multilevel nature of the data was taken into account, and then the comparison indicator maps (CIMs) were weighted by study quality and sample size (31). As a consequence, no single CIM could disproportionately influence the meta-analytic results, while more rigorous and larger studies would contribute more to the results (32–35).
First, the peak coordinates of each comparison map from the included studies were separately convolved with a spherical kernel (r = 15 mm) to generate the CIMs (30). The CIMs were limited to a maximum value of 1 so that the values across brain voxels were either 1 or 0, which represented a significant effect or no significant effect in the neighborhood, respectively. Second, the proportion of the study comparison maps (activation maps) that indicated hyperconnectivity or hypoconnectivity for each network within a 15-mm radius of each voxel was produced by averaging the CIMs weighted by sample size and a discounting factor (30). Third, differences between the resulting density maps were computed to test for two activated effects: hyperconnectivity and hypoconnectivity. Therefore, activation maps were summarized based on the height mode or extent mode: the height-based activation maps reflected the activation of voxels that exceeded the maximum expected across the whole brain or within specified network by chance only 5% of the time; the extent-based activation maps revealed the activation of clusters of contiguous voxels above the maximum expected in a cluster of that size by chance.
To correct for multiple comparisons, a Monte Carlo simulation was used to establish a familywise error rate threshold of p < 0.05 (14, 30). A jack-knife analysis was carried out to test the replicability of the results. We also performed Fisher’s exact tests to assess whether a specific anatomical region accounted for more of a significant effect than other regions from the same network by comparing the differences in effect likelihood ratio (LR) among regions in the same network.
Results
The search protocol of meta-analysis followed the Preferred Reporting Items for Systematic Reviews and Meta-Analyses (PRISMA) guidelines (36). The literature searches and criteria yielded a sample of 76 studies from 70 publications (9–12, 29, 37–101) that reported on 2,588 schizophrenia patients and 2,567 HCs. Demographic characteristics were calculated including gender ratio defined as male/female (patients, 1.39; HCs, 1.20), and weighted mean age (patients, 31.00; HCs, 31.17). Weighted mean duration of illness for patients was 89.79 months, and the Positive and Negative Syndrome Scale (PANSS) scores were evaluated (total, 73.46; positive, 18.80; negative, 18.27; general, 34.89). Fifty-five studies provided medication information, and antipsychotic dosages were converted into chlorpromazine equivalents (mean chlorpromazine dosage, 478.58 mg/day) (Figure 1, Supplementary Table 2).
The extracted locations and activation effects of significant intergroup comparisons were classified into the eight networks based on the ICA-based brain templates: the DAN, CEN, DMN, CN, SRN, SMN, VN, and AN (Supplementary Table 3). The MKDA showed significant hypoconnectivity between the seed regions and additional areas within the AN, CN, DMN, SRN, and SMN (Table 1, Figure 2). No hyperconnectivity was detected between the seeds and any other regions within the networks. The jack-knife analysis showed that our results were reliable.
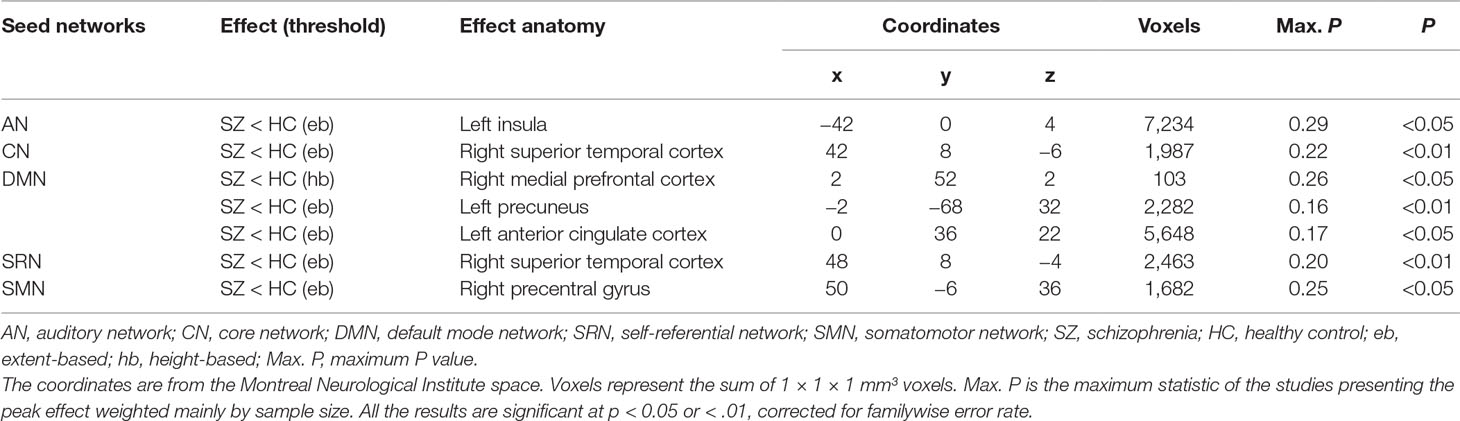
Table 1 Results of the meta-analysis of resting-state functional connectivity in patients with schizophrenia.
In the AN, schizophrenia was associated with decreased rsFC using extent-based threshold between the seed regions and the left insula, which is involved in processing emotional and self-attributed sensory stimuli (102). These seeds included the regions of the bilateral middle and superior temporal gyri, Heschl’s gyrus, and temporal pole. Post hoc tests revealed that seeds in the superior temporal gyrus (STG) were more likely than those in the medial prefrontal cortex (MPFC) and frontal pole to exhibit hypoconnectivity with the left insula (LR = 11.76; p = 0.003).
In the CN, seed regions were defined as areas within the anterior cingulate, bilateral insular, and dorsolateral prefrontal cortices. Hypoconnectivity was observed in the patients with schizophrenia based on the height threshold between the seeds and the right STG cortex related to the production, interpretation, and self-monitoring of language (103). The likelihood of hypoconnectivity did not differ among anatomical regions within the CN (p > 0.05; Supplementary Table 4).
In the DMN, seed regions were selected in the PCC/precuneus, bilateral inferior parietal gyrus, angular gyrus, middle temporal gyrus, and superior and medial frontal gyri. The characteristic activated effect in schizophrenia was represented as hypoconnectivity between the seeds and the right MPFC based on the height threshold and the left precuneus and anterior cingulate cortex (ACC) based on the extent threshold. These areas mainly contribute to internal mental participation in self-referential thinking and emotion processing (104–106). Post hoc tests indicated that seeds in the ACC, MPFC, and medial cingulate cortex (MCC)/PCC using height-based threshold (LR = 7.11∼10.14; p = 0.006∼0.026) and seeds in the MPFC, medial temporal gyrus (MTG)/STG, MCC/PCC, cerebellum, and precuneus on the extent mode (LR = 5.53∼11.23; p = 0.003∼0.048) were more likely to exhibit hypoconnectivity either with the right MPFC or with the left ACC and precuneus (Supplementary Table 4).
In the SRN, rsFC in patients with schizophrenia was reduced using extent-based threshold between the right STG specialized for self-referential processing (107, 108) and the seeds that included the ventromedial prefrontal cortex, medial orbital prefrontal cortex, gyrus rectus, and pregenual anterior cingulate gyrus. No differences among anatomical seed effects within the SRN were found in post hoc tests (p > 0.05; Supplementary Table 4).
In the SMN, schizophrenia was linked to hypoconnectivity based on the extent threshold between the right precentral gyrus, involved in motor function, and the SMN seeds, including the postcentral and precentral gyri and cerebellum. Compared with the seeds in the cerebellum and Broca’s area, the precentral seeds were more likely to show hypoconnectivity with the right precentral gyrus (LR = 6.90; p = 0.041).
Discussion
The present meta-analysis systematically integrated a sum of 76 studies reporting rsFC of seed-based whole-brain networks and specified networks in patients with schizophrenia based on ICA brain templates, and the meta-analysis consistently indicated that individuals with schizophrenia exhibited large-scale hypoconnectivity involving the auditory system (AN), task and cognitive control (CN), self-referential processing (SRN), episodic memory and self-projection (DMN), and sensory-motor function (SMN). These diffuse disconnections across these brain networks suggest a dysregulated brain network model of schizophrenia in which network dysfunction is associated with deficits in sensory and associated self-referential processing. In this model, decreased coordination within the SRN and DMN might play core roles in the malfunction of information processing, while the CN might act as a dysfunctional hub of regulation.
As a key component of the AN, the STG contains primary auditory cortex and auditory association cortical areas (109, 110). The morphological and functional abnormalities of the STG may be related to the experience of auditory verbal hallucinations (AVHs) and being less responsive to external auditory stimuli during ongoing AVHs compared with when they are absent (111, 112). On the other hand, the SRN is crucial in self-referential processing per se, accounting for differentiating stimuli related to one’s own self from those that are not relevant to inner concerns. The SRN receives information from exteroceptive stimuli through extensive connections from areas associated with primary and secondary auditory sensory modalities (113–118), and it has a role in both bottom-up and top-down modulation between sensory, self-referential, and higher-order processing (119). Furthermore, resting-state networks display a dominant direction of causal influence from the SRN to the AN (28). The dysconnectivity between the SRN seeds and the right STG suggested that the frontotemporal pathways may be a neural substrate underlying dysfunction of auditory and language processing in schizophrenia, i.e., AVHs that are core symptoms of the disease (120, 121). Our findings are in accord with many prior structural and functional neuroimaging studies of hallucinations (121), leading to cognitive models that have implicated disrupted frontotemporal pathways as a potential cause of AVHs and the failure to appropriately monitor inner speech generation.
Decreased rsFC was present in patients with schizophrenia between the DMN seeds and the regions within the right MPFC and left ACC, which is in line with the results of the DMN regarding the within-network connectivity by Dong and colleagues (17). Reduced synchronized neuronal activity within the DMN may support the dysfunctional pattern of schizophrenia in self-related processes relevant to emotional processing, self-referential mental activity, and the recollection of prior experiences (122). The reduced connectivity across the DMN, salience network (SN), and central executive network (CEN) further supports a triple network model in psychopathology (17). Such an imbalanced system of networks fails in dynamic regulation across salience processes, internal mental processes, and goal-relevant external stimuli (123), which is related to multiple phenomenological domains and the total symptom burden of schizophrenia (124, 125). Moreover, our analysis revealed hypoconnectivity between the seeds and the left precuneus, which was beyond the extent of hypoconnectivity described in the DMN by Dong and colleagues (17). This might be due to differences in brain-parcellation modes. It should be noted that the DMN is also thought to be related to AVHs (126, 127), and the anatomical regions of the DMN and SRN partially overlap. However, the SRN exerts a strong directional causal influence over the DMN (28), and the SRN is represented by distinct power spectra of electroencephalography (23) and spatial patterns of the BOLD signal from the DMN (23, 27, 28). Future research designs and phenomenological subcategorizations of individuals with AVHs might facilitate discrimination of different neurocognitive mechanisms (127).
Decreased mutual cross-network effects were exhibited between the AN involving the STG and the CN involving the insula, suggesting weakened synchronization between resting-state networks. Our results are in line with previous structural (128, 129) and functional (130, 131) MR studies in patients with schizophrenia that reported a direct association between the indices of auditory cortex connectivity and psychotic symptoms. Acting as a hub within the CN, the insula is not only connected with the wide array of regions processing external sensory stimuli but also responsible for interoceptive awareness of the body’s internal state, which is uniquely distinct from the external environment. Disrupted interoception processing within the insula would be expected to distort the evaluation of stimuli and boundaries of the self, resulting in allowing internal sensory information to be attributed to an external source (102). Taken together, our results suggest an etiology of AVHs in schizophrenia, leading to dysfunction in distinguishing an internally generated interoception from an external sensory experience (132, 133).
We also detected hypoconnectivity between the SMN seeds and the right precentral gyrus, which implies diminished activity within this network. Compared with HCs, schizophrenia patients showed limited connectivity in areas more strongly related to motor functions, such as the lateral M1 with the supplementary motor and medial motor areas (134). In addition, reduced cortical volume and attenuated activation of the precentral gyrus have been associated with motor-related cognitive dysfunction and impaired behavioral performance in the emotional face task (134–136), which is correlated with the clinical severity of schizophrenia. Although these findings corroborate the dysconnectivity hypothesis of schizophrenia, there are also patterns of increased connectivity within extensive motor networks of the patients (134, 137) and ultrahigh-risk youths (138). Despite the mixed findings, our analysis could be beneficial for better understanding of the relationships across the motor systems.
Further, we analyzed the data with other two organizations of resting state networks (20, 139) to test the repeatability of results (Supplementary Table 5). The hypoconnectivity in the DMN is consistent with our results between the seeds and the left precuneus/ACC using Yeo’s and Power’s templates, which makes internal mental participation in self-referential thinking and emotion processing more convincing (122). We also found a similar hypoconnectivity within the SMN in the right precentral gyrus using Yeo’s template, instead of the left postcentral gyrus with Power’s template; changes in different brain regions within the same network may reflect the extensiveness of the dysconnectivity in motor system. Meanwhile, we detected the decreased connectivity across the SMN in schizophrenia in the left ACC both in Yeo’s and Power’s network organizations. This might be due to different network classifications, in which our template included brain regions relating to auditory and visual systems; and functional alternations in the ACC have been found to be associated with attention and sensorimotor processes in patients (140, 141). Using Yeo’s and Power’s templates, we found extensive hypoconnectivities in individually defined networks. The hypoconnectivities between seeds in the limbic network (LN) and left STG/left inferior parietal gyrus were detected using Yeo’s templates, which is consistent with findings in the frontoparietal network (FPN) and DMN in Power’s classification. We also found decreased connectivity in the ventral attention network (VAN) between the seeds and the right middle temporal cortex using Yeo’s template rather than ours and Power’s. In addition to common network definitions including the DMN, SMN, DAN, and FPN, we had some findings in distinctive network definitions using Power’s template. The hypoconnectivities in the left insula within the cingulo-opercular network (CON) and the left parahippocampal gyrus within unknown network (UnN; mostly related to the memory retrieval process) were detected, and the findings in the left insula are consistent with our findings in the AN, while the parahippocampal gyrus participated in the key process of memory (142).
Our study reflected decreased rsFC with affected anatomic regions including left ACC, insula, precuneus and right STG, MPFC, and precentral gyrus. Previous large meta-analyses in cortical and subcortical changes using MRI (143, 144) have revealed structural alternations including widespread thinner cortex and smaller surface area in frontal and temporal regions, as well as reduced volume in the hippocampus, which is mostly consistent with our functional findings in the CN, SRN, and part of the DMN, while changes in precuneus and some subcortical regions are not consistent with our results in other networks. Moreover, we detected different performance of the left and right hemispheres, which was not shown in structural changes. These overlaps and diversities of brain alternations in structure and function existed in different networks and might suggest different pathophysiological process in different networks affected by schizophrenia.
Although our findings support the dysconnectivity hypothesis of schizophrenia (145) and identify regions associated with the pathogenesis of the disease, limitations should be considered in three perspectives. First, the exclusion of negative findings may have biased the results. This should motivate studies using improved methodology beyond MKDA, thereby both positive and negative findings could be matched. Second, the network spatial maps used in our analysis did not give consider the epencephalon, despite its potential role in the cerebello-thalamo-cortical circuit in psychosis (134, 146). A new parcellation template would benefit from more comprehensive network mapping. Third, we did not test whether the results are independent from the different types of schizophrenia patients due to limited number of studies included in the context of each brain network. Analysis with subtypes of schizophrenia would be a strong supplement, and more studies of specific networks will be needed in the future.
In conclusion, the present meta-analysis provides insight into rsFC within multiple brain networks based on ICA templates in schizophrenia and suggests diffuse hypoconnectivities as a dysregulated brain network model of the disease. Future systematic analyses including more homogenous samples are demanded, in view of the relationships across brain networks, and correlations between the levels of connectivity and clinical severity of schizophrenia.
Data Availability
The datasets generated for this study are available on request to the corresponding author.
Author Contributions
SLu contributed to the conceptual design and constructive discussion. SLi and NH searched the literature, extracted the data, and wrote the manuscript. WZ and BT performed the MKDA and post hoc tests. JD, YG, YT, and DC assisted in data analysis.
Funding
This study was supported by the National Natural Science Foundation of China (Project Nos. 81671664 and 81621003), National Program for Support of Top-notch Young Professionals (Project No. W02070140), Fundamental Research Funds for the Central Universities (Project No. 2018SCUH0011), Science and Technology Project of the Health Commission of Sichuan Province (Project No. 18ZD035), Sichuan Science and Technology Program (Project No. 2019YJ0155), and 1.3.5 Project for Disciplines of Excellence, West China Hospital, Sichuan University (Project Nos. ZYYC08001 and ZYJC18020).
Conflict of Interest Statement
The authors declare that the research was conducted in the absence of any commercial or financial relationships that could be construed as a potential conflict of interest.
Supplementary Material
The Supplementary Material for this article can be found online at: https://www.frontiersin.org/articles/10.3389/fpsyt.2019.00482/full#supplementary-material.
References
1. Northoff G, Duncan NW. How do abnormalities in the brain’s spontaneous activity translate into symptoms in schizophrenia? From an overview of resting state activity findings to a proposed spatiotemporal psychopathology. Prog Neurobiol (2016) 145-146:26–45. doi: 10.1016/j.pneurobio.2016.08.003
2. Li T, Wang Q, Zhang J, Rolls ET, Yang W, Palaniyappan L, et al. Brain-Wide Analysis of functional connectivity in first-episode and chronic stages of schizophrenia. Schizophr Bull (2017) 43:436–48. doi: 10.1093/schbul/sbw099
3. Curcic-Blake B, Ford JM, Hubl D, Orlov ND, Sommer IE, Waters F, et al. Interaction of language, auditory and memory brain networks in auditory verbal hallucinations. Prog Neurobiol (2017) 148:1–20. doi: 10.1016/j.pneurobio.2016.11.002
4. Yu Q, Allen EA, Sui J, Arbabshirani MR, Pearlson G, Calhoun VD. Brain connectivity networks in schizophrenia underlying resting state functional magnetic resonance imaging. Curr Top Med Chem (2012) 12:2415–25. doi: 10.2174/1568026611212210012
5. Fitzsimmons J, Kubicki M, Shenton ME. Review of functional and anatomical brain connectivity findings in schizophrenia. Curr Opin Psychiatry (2013) 26:172–87. doi: 10.1097/YCO.0b013e32835d9e6a
6. Fornito A, Zalesky A, Pantelis C, Bullmore ET. Schizophrenia, neuroimaging and connectomics. Neuroimage (2012) 62:2296–314. doi: 10.1016/j.neuroimage.2011.12.090
7. Pettersson-Yeo W, Allen P, Benetti S, McGuire P, Mechelli A. Dysconnectivity in schizophrenia: where are we now? Neurosci Biobehav Rev (2011) 35:1110–24. doi: 10.1016/j.neubiorev.2010.11.004
8. van den Heuvel MP, Fornito A. Brain networks in schizophrenia. Neuropsychol Rev (2014) 24:32–48. doi: 10.1007/s11065-014-9248-7
9. Bluhm RL, Miller J, Lanius RA, Osuch EA, Boksman K, Neufeld RW, et al. Spontaneous low-frequency fluctuations in the BOLD signal in schizophrenic patients: anomalies in the default network. Schizophr Bull (2007) 33:1004–12. doi: 10.1093/schbul/sbm052
10. Moran LV, Tagamets MA, Sampath H, O’Donnell A, Stein EA, Kochunov P, et al. Disruption of anterior insula modulation of large-scale brain networks in schizophrenia. Biol Psychiatry (2013) 74:467–74. doi: 10.1016/j.biopsych.2013.02.029
11. Woodward ND, Rogers B, Heckers S. Functional resting-state networks are differentially affected in schizophrenia. Schizophr Res (2011) 130:86–93. doi: 10.1016/j.schres.2011.03.010
12. He Z, Deng W, Li M, Chen Z, Jiang L, Wang Q, et al. Aberrant intrinsic brain activity and cognitive deficit in first-episode treatment-naive patients with schizophrenia. Psychol Med (2013) 43:769–80. doi: 10.1017/S0033291712001638
13. Cao H, Dixson L, Meyer-Lindenberg A, Tost H. Functional connectivity measures as schizophrenia intermediate phenotypes: advances, limitations, and future directions. Curr Opin Neurobiol (2016) 36:7–14. doi: 10.1016/j.conb.2015.07.008
14. Kaiser RH, Andrews-Hanna JR, Wager TD, Pizzagalli DA. Large-scale network dysfunction in major depressive disorder: a meta-analysis of resting-state functional connectivity. JAMA Psychiatry (2015) 72:603–11. doi: 10.1001/jamapsychiatry.2015.0071
15. Kleikers PW, Hooijmans C, Gob E, Langhauser F, Rewell SS, Radermacher K, et al. A combined pre-clinical meta-analysis and randomized confirmatory trial approach to improve data validity for therapeutic target validation. Sci Rep (2015) 5:13428. doi: 10.1038/srep13428
16. Neurology Working Group of the Cohorts for Heart and Aging Research in Genomic Epidemiology (CHARGE) Consortium, the Stroke Genetics Network (SiGN), and the International Stroke Genetics Consortium (ISGC). Identification of additional risk loci for stroke and small vessel disease: a meta-analysis of genome-wide association studies. Lancet Neurol (2016) 15:695–707. doi: 10.1016/S1474-4422(16)00102-2
17. Dong D, Wang Y, Chang X, Luo C, Yao D. Dysfunction of large-scale brain networks in schizophrenia: a meta-analysis of resting-state functional connectivity. Schizophr Bull (2018) 44:168–81. doi: 10.1093/schbul/sbx034
18. Buckner RL, Krienen FM, Castellanos A, Diaz JC, Yeo BT. The organization of the human cerebellum estimated by intrinsic functional connectivity. J Neurophysiol (2011) 106:2322–45. doi: 10.1152/jn.00339.2011
19. Choi EY, Yeo BT, Buckner RL. The organization of the human striatum estimated by intrinsic functional connectivity. J Neurophysiol (2012) 108:2242–63. doi: 10.1152/jn.00270.2012
20. Yeo BT, Krienen FM, Sepulcre J, Sabuncu MR, Lashkari D, Hollinshead M, et al. The organization of the human cerebral cortex estimated by intrinsic functional connectivity. J Neurophysiol (2011) 106:1125–65. doi: 10.1152/jn.00338.2011
21. Fox MD, Raichle ME. Spontaneous fluctuations in brain activity observed with functional magnetic resonance imaging. Nat Rev Neurosci (2007) 8:700–11. doi: 10.1038/nrn2201
22. Mantini D, Corbetta M, Perrucci MG, Romani GL, Del Gratta C. Large-scale brain networks account for sustained and transient activity during target detection. Neuroimage (2009) 44:265–74. doi: 10.1016/j.neuroimage.2008.08.019
23. Mantini D, Perrucci MG, Del Gratta C, Romani GL, Corbetta M. Electrophysiological signatures of resting state networks in the human brain. PNAS (2007) 104:13170–5. doi: 10.1073/pnas.0700668104
24. Amico E, Marinazzo D, Di Perri C, Heine L, Annen J, Martial C, et al. Mapping the functional connectome traits of levels of consciousness. Neuroimage (2017) 148:201–11. doi: 10.1016/j.neuroimage.2017.01.020
25. Calhoun VD, de Lacy N. Ten key observations on the analysis of resting-state functional MR imaging data using independent component analysis. Neuroimaging Clin N Am (2017) 27:561–79. doi: 10.1016/j.nic.2017.06.012
26. Lu J, Zhang H, Hameed NUF, Zhang J, Yuan S, Qiu T, et al. An automated method for identifying an independent component analysis-based language-related resting-state network in brain tumor subjects for surgical planning. Sci Rep (2017) 7:13769. doi: 10.1038/s41598-017-14248-5
27. Liao W, Chen H, Feng Y, Mantini D, Gentili C, Pan Z, et al. Selective aberrant functional connectivity of resting state networks in social anxiety disorder. Neuroimage (2010) 52:1549–58. doi: 10.1016/j.neuroimage.2010.05.010
28. Liao W, Mantini D, Zhang Z, Pan Z, Ding J, Gong Q, et al. Evaluating the effective connectivity of resting state networks using conditional Granger causality. Biol Cybern (2010) 102:57–69. doi: 10.1007/s00422-009-0350-5
29. Hoffman RE, Fernandez T, Pittman B, Hampson M. Elevated functional connectivity along a corticostriatal loop and the mechanism of auditory/verbal hallucinations in patients with schizophrenia. Biol Psychiatry (2011) 69:407–14. doi: 10.1016/j.biopsych.2010.09.050
30. Wager TD, Lindquist M, Kaplan L. Meta-analysis of functional neuroimaging data: current and future directions. Soc Cogn Affect Neurosci (2007) 2:150–8. doi: 10.1093/scan/nsm015
31. Kober H, Wager TD. Meta-analysis of neuroimaging data. Wiley Interdiscip Rev Cogn Sci (2010) 1:293–300. doi: 10.1002/wcs.41
32. Cacioppo S, Frum C, Asp E, Weiss RM, Lewis JW, Cacioppo JT. A quantitative meta-analysis of functional imaging studies of social rejection. Sci Rep (2013) 3:2027. doi: 10.1038/srep02027
33. Mende-Siedlecki P, Said CP, Todorov A. The social evaluation of faces: a meta-analysis of functional neuroimaging studies. Soc Cogn Affect Neurosci (2013) 8:285–99. doi: 10.1093/scan/nsr090
34. Fan Y, Duncan NW, de Greck M, Northoff G. Is there a core neural network in empathy? An fMRI based quantitative meta-analysis. Neurosci Biobehav Rev (2011) 35:903–11. doi: 10.1016/j.neubiorev.2010.10.009
35. Adolfi F, Couto B, Richter F, Decety J, Lopez J, Sigman M, et al. Convergence of interoception, emotion, and social cognition: a twofold fMRI meta-analysis and lesion approach. Cortex (2017) 88:124–42. doi: 10.1016/j.cortex.2016.12.019
36. Moher D. Preferred reporting items for systematic reviews and meta-analyses: the PRISMA statement (vol 8, pg 336, 2010). Int J Surg (2010) 8:658–. doi: 10.1016/j.ijsu.2010.07.299
37. Mier D, Eisenacher S, Rausch F, Englisch S, Gerchen MF, Zamoscik V, et al. Aberrant activity and connectivity of the posterior superior temporal sulcus during social cognition in schizophrenia. Eur Arch Psychiatry Clin Neurosci (2017) 267:597–610. doi: 10.1007/s00406-016-0737-y
38. Wang S, Zhang Y, Lv L, Wu R, Fan X, Zhao J, et al. Abnormal regional homogeneity as a potential imaging biomarker for adolescent-onset schizophrenia: a resting-state fMRI study and support vector machine analysis. Schizophr Res (2018) 192:179–84. doi: 10.1016/j.schres.2017.05.038
39. Anticevic A, Tang Y, Cho YT, Repovs G, Cole MW, Savic A, et al. Amygdala connectivity differs among chronic, early course, and individuals at risk for developing schizophrenia. Schizophr Bull (2014) 40:1105–16. doi: 10.1093/schbul/sbt165
40. Hoptman MJ, D’Angelo D, Catalano D, Mauro CJ, Shehzad ZE, Kelly AM, et al. Amygdalofrontal functional disconnectivity and aggression in schizophrenia. Schizophr Bull (2010) 36:1020–8. doi: 10.1093/schbul/sbp012
41. Oertel-Knochel V, Knochel C, Matura S, Stablein M, Prvulovic D, Maurer K, et al. Association between symptoms of psychosis and reduced functional connectivity of auditory cortex. Schizophr Res (2014) 160:35–42. doi: 10.1016/j.schres.2014.10.036
42. Guo Q, Tang Y, Li H, Zhang T, Li J, Sheng J, et al. Both volumetry and functional connectivity of Heschl’s gyrus are associated with auditory P300 in first episode schizophrenia. Schizophr Res (2014) 160:57–66. doi: 10.1016/j.schres.2014.10.006
43. Tian L, Meng C, Yan H, Zhao Q, Liu Q, Yan J, et al. Convergent evidence from multimodal imaging reveals amygdala abnormalities in schizophrenic patients and their first-degree relatives. PloS One (2011) 6:e28794. doi: 10.1371/journal.pone.0028794
44. Berman RA, Gotts SJ, McAdams HM, Greenstein D, Lalonde F, Clasen L, et al. Disrupted sensorimotor and social-cognitive networks underlie symptoms in childhood-onset schizophrenia. Brain (2016) 139:276–91. doi: 10.1093/brain/awv306
45. Chang X, Xi YB, Cui LB, Wang HN, Sun JB, Zhu YQ, et al. Distinct inter-hemispheric dysconnectivity in schizophrenia patients with and without auditory verbal hallucinations. Sci Rep (2015) 5:11218. doi: 10.1038/srep11218
46. Ganella EP, Bartholomeusz CF, Seguin C, Whittle S, Bousman C, Phassouliotis C, et al. Functional brain networks in treatment-resistant schizophrenia. Schizophr Res (2017) 184:73–81. doi: 10.1016/j.schres.2016.12.008
47. Shinn AK, Baker JT, Cohen BM, Ongur D. Functional connectivity of left Heschl’s gyrus in vulnerability to auditory hallucinations in schizophrenia. Schizophr Res (2013) 143:260–8. doi: 10.1016/j.schres.2012.11.037
48. Oertel-Knochel V, Knochel C, Matura S, Prvulovic D, Linden DE, de Ven V. Reduced functional connectivity and asymmetry of the planum temporale in patients with schizophrenia and first-degree relatives. Schizophr Res (2013) 147:331–8. doi: 10.1016/j.schres.2013.04.024
49. Xu L, Qin W, Zhuo C, Zhu J, Liu H, Liu X, et al. Selective functional disconnection of the dorsal subregion of the temporal pole in schizophrenia. Sci Rep (2015) 5:11258. doi: 10.1038/srep11258
50. Wei S, Womer F, Geng H, Jiang X, Zhou Q, Chang M, et al. Similarities and differences of functional connectivity in drug-naive, first-episode adolescent and young adult with major depressive disorder and schizophrenia. Sci Rep (2017) 7:44316. doi: 10.1038/srep44316
51. Hoptman MJ, Antonius D, Mauro CJ, Parker EM, Javitt DC. Cortical thinning, functional connectivity, and mood-related impulsivity in schizophrenia: relationship to aggressive attitudes and behavior. Am J Psychiatry (2014) 171:939–48. doi: 10.1176/appi.ajp.2014.13111553
52. Anticevic A, Hu X, Xiao Y, Hu J, Li F, Bi F, et al. Early-course unmedicated schizophrenia patients exhibit elevated prefrontal connectivity associated with longitudinal change. J Neurosci (2015) 35:267–86. doi: 10.1523/JNEUROSCI.2310-14.2015
53. Yan H, Tian L, Yan J, Sun W, Liu Q, Zhang YB, et al. Functional and anatomical connectivity abnormalities in cognitive division of anterior cingulate cortex in schizophrenia. PLoS One (2012) 7:e45659. doi: 10.1371/journal.pone.0045659
54. Zhou Y, Liang M, Jiang T, Tian L, Liu Y, Liu Z, et al. Functional dysconnectivity of the dorsolateral prefrontal cortex in first-episode schizophrenia using resting-state fMRI. Neurosci Lett (2007) 417:297–302. doi: 10.1016/j.neulet.2007.02.081
55. Mothersill O, Tangney N, Morris DW, McCarthy H, Frodl T, Gill M, et al. Further evidence of alerted default network connectivity and association with theory of mind ability in schizophrenia. Schizophr Res (2017) 184:52–8. doi: 10.1016/j.schres.2016.11.043
56. Penner J, Ford KA, Taylor R, Schaefer B, Theberge J, Neufeld RW, et al. Medial prefrontal and anterior insular connectivity in early schizophrenia and major depressive disorder: a resting functional MRI evaluation of large-scale brain network models. Front Hum Neurosci (2016) 10:132. doi: 10.3389/fnhum.2016.00132
57. Salvador R, Landin-Romero R, Anguera M, Canales-Rodriguez EJ, Radua J, Guerrero-Pedraza A, et al. Non redundant functional brain connectivity in schizophrenia. Brain Imaging Behav (2017) 11:552–64. doi: 10.1007/s11682-016-9535-4
58. Su TW, Lan TH, Hsu TW, Biswal BB, Tsai PJ, Lin WC, et al. Reduced neuro-integration from the dorsolateral prefrontal cortex to the whole brain and executive dysfunction in schizophrenia patients and their relatives. Schizophr Res (2013) 148:50–8. doi: 10.1016/j.schres.2013.05.005
59. Chen YL, Tu PC, Lee YC, Chen YS, Li CT, Su TP. Resting-state fMRI mapping of cerebellar functional dysconnections involving multiple large-scale networks in patients with schizophrenia. Schizophr Res (2013) 149:26–34. doi: 10.1016/j.schres.2013.05.029
60. Tu PC, Lee YC, Chen YS, Li CT, Su TP. Schizophrenia and the brain’s control network: aberrant within- and between-network connectivity of the frontoparietal network in schizophrenia. Schizophr Res (2013) 147:339–47. doi: 10.1016/j.schres.2013.04.011
61. Chen X, Liu C, He H, Chang X, Jiang Y, Li Y, et al. Transdiagnostic differences in the resting-state functional connectivity of the prefrontal cortex in depression and schizophrenia. J Affect Disord (2017) 217:118–24. doi: 10.1016/j.jad.2017.04.001
62. Cole MW, Anticevic A, Repovs G, Barch D. Variable global dysconnectivity and individual differences in schizophrenia. Biol Psychiatry (2011) 70:43–50. doi: 10.1016/j.biopsych.2011.02.010
63. Guo W, Yao D, Jiang J, Su Q, Zhang Z, Zhang J, et al. Abnormal default-mode network homogeneity in first-episode, drug-naive schizophrenia at rest. Prog Neuropsychopharmacol Biol Psychiatry (2014) 49:16–20. doi: 10.1016/j.pnpbp.2013.10.021
64. Wang S, Zhan Y, Zhang Y, Lyu L, Lyu H, Wang G, et al. Abnormal long- and short-range functional connectivity in adolescent-onset schizophrenia patients: a resting-state fMRI study. Prog Neuropsychopharmacol Biol Psychiatry (2018) 81:445–51. doi: 10.1016/j.pnpbp.2017.08.012
65. Chai XJ, Whitfield-Gabrieli S, Shinn AK, Gabrieli JD, Nieto Castanon A, McCarthy JM, et al. Abnormal medial prefrontal cortex resting-state connectivity in bipolar disorder and schizophrenia. Neuropsychopharmacology (2011) 36:2009–17. doi: 10.1038/npp.2011.88
66. Liu Y, Zhang Y, Lv L, Wu R, Zhao J, Guo W. Abnormal neural activity as a potential biomarker for drug-naive first-episode adolescent-onset schizophrenia with coherence regional homogeneity and support vector machine analyses. Schizophr Res (2018) 192:408–15. doi: 10.1016/j.schres.2017.04.028
67. Iwabuchi SJ, Palaniyappan L. Abnormalities in the effective connectivity of visuothalamic circuitry in schizophrenia. Psychol Med (2017) 47:1300–10. doi: 10.1017/S0033291716003469
68. Duan M, Chen X, He H, Jiang Y, Jiang S, Xie Q, et al. Altered basal ganglia network integration in schizophrenia. Front Hum Neurosci (2015) 9:561. doi: 10.3389/fnhum.2015.00561
69. Chang X, Shen H, Wang L, Liu Z, Xin W, Hu D, et al. Altered default mode and fronto-parietal network subsystems in patients with schizophrenia and their unaffected siblings. Brain Res (2014) 1562:87–99. doi: 10.1016/j.brainres.2014.03.024
70. Zhang Q, Shen J, Wu J, Yu X, Lou W, Fan H, et al. Altered default mode network functional connectivity in schizotypal personality disorder. Schizophr Res (2014) 160:51–6. doi: 10.1016/j.schres.2014.10.013
71. Wang D, Zhou Y, Zhuo C, Qin W, Zhu J, Liu H, et al. Altered functional connectivity of the cingulate subregions in schizophrenia. Transl Psychiatry (2015) 5:e575. doi: 10.1038/tp.2015.69
72. Zhou Y, Shu N, Liu Y, Song M, Hao Y, Liu H, et al. Altered resting-state functional connectivity and anatomical connectivity of hippocampus in schizophrenia. Schizophr Res (2008) 100:120–32. doi: 10.1016/j.schres.2007.11.039
73. Holt DJ, Cassidy BS, Andrews-Hanna JR, Lee SM, Coombs G, Goff DC, et al. An anterior-to-posterior shift in midline cortical activity in schizophrenia during self-reflection. Biol Psychiatry (2011) 69:415–23. doi: 10.1016/j.biopsych.2010.10.003
74. Zhou B, Tan C, Tang J, Chen X. Brain functional connectivity of functional magnetic resonance imaging of patients with early-onset schizophrenia. Zhong Nan Da Xue Xue Bao Yi Xue Ban (2010) 35:17–24. doi: 10.3969/j.issn.1672-7347.2010.01.003
75. Jiang Y, Duan M, Chen X, Chang X, He H, Li Y, et al. Common and distinct dysfunctional patterns contribute to triple network model in schizophrenia and depression: a preliminary study. Prog Neuropsychopharmacol Biol Psychiatry (2017) 79:302–10. doi: 10.1016/j.pnpbp.2017.07.007
76. Tu PC, Hsieh JC, Li CT, Bai YM, Su TP. Cortico-striatal disconnection within the cingulo-opercular network in schizophrenia revealed by intrinsic functional connectivity analysis: a resting fMRI study. Neuroimage (2012) 59:238–47. doi: 10.1016/j.neuroimage.2011.07.086
77. Zheng J, Zhang Y, Guo X, Duan X, Zhang J, Zhao J, et al. Disrupted amplitude of low-frequency fluctuations in antipsychotic-naive adolescents with early-onset schizophrenia. Psychiatry Res Neuroimaging (2016) 249:20–6. doi: 10.1016/j.pscychresns.2015.11.006
78. Zhang Y, Zheng J, Fan X, Guo X, Guo W, Yang G, et al. Dysfunctional resting-state connectivities of brain regions with structural deficits in drug-naive first-episode schizophrenia adolescents. Schizophr Res (2015) 168:353–9. doi: 10.1016/j.schres.2015.07.031
79. Guo W, Liu F, Chen J, Wu R, Li L, Zhang Z, et al. Family-based case–control study of homotopic connectivity in first-episode, drug-naive schizophrenia at rest. Sci Rep (2017) 7:43312. doi: 10.1038/srep43312
80. Wang X, Zhang Y, Long Z, Zheng J, Zhang Y, Han S, et al. Frequency-specific alteration of functional connectivity density in antipsychotic-naive adolescents with early-onset schizophrenia. J Psychiatr Res (2017) 95:68–75. doi: 10.1016/j.jpsychires.2017.07.014
81. Kraguljac NV, White DM, Hadley J, Reid MA, Lahti AC. Hippocampal-parietal dysconnectivity and glutamate abnormalities in unmedicated patients with schizophrenia. Hippocampus (2014) 24:1524–32. doi: 10.1002/hipo.22332
82. Guo W, Liu F, Chen J, Wu R, Li L, Zhang Z, et al. Hyperactivity of the default-mode network in first-episode, drug-naive schizophrenia at rest revealed by family-based case–control and traditional case–control designs. Medicine (Baltimore) (2017) 96:e6223. doi: 10.1097/MD.0000000000006223
83. Du Y, Pearlson GD, Yu Q, He H, Lin D, Sui J, et al. Interaction among subsystems within default mode network diminished in schizophrenia patients: a dynamic connectivity approach. Schizophr Res (2016) 170:55–65. doi: 10.1016/j.schres.2015.11.021
84. Orliac F, Naveau M, Joliot M, Delcroix N, Razafimandimby A, Brazo P, et al. Links among resting-state default-mode network, salience network, and symptomatology in schizophrenia. Schizophr Res (2013) 148:74–80. doi: 10.1016/j.schres.2013.05.007
85. Jiang L, Xu Y, Zhu XT, Yang Z, Li HJ, Zuo XN. Local-to-remote cortical connectivity in early- and adulthood-onset schizophrenia. Transl Psychiatry (2015) 5:e566. doi: 10.1038/tp.2015.59
86. Duan HF, Gan JL, Yang JM, Cheng ZX, Gao CY, Shi ZJ, et al. A longitudinal study on intrinsic connectivity of hippocampus associated with positive symptom in first-episode schizophrenia. Behav Brain Res (2015) 283:78–86. doi: 10.1016/j.bbr.2015.01.022
87. Knochel C, Stablein M, Storchak H, Reinke B, Jurcoane A, Prvulovic D, et al. Multimodal assessments of the hippocampal formation in schizophrenia and bipolar disorder: evidences from neurobehavioral measures and functional and structural MRI. Neuroimage Clin (2014) 6:134–44. doi: 10.1016/j.nicl.2014.08.015
88. Salvador R, Sarro S, Gomar JJ, Ortiz-Gil J, Vila F, Capdevila A, et al. Overall brain connectivity maps show cortico-subcortical abnormalities in schizophrenia. Hum Brain Mapp (2010) 31:2003–14. doi: 10.1002/hbm.20993
89. Jung WH, Jang JH, Shin NY, Kim SN, Choi CH, An SK, et al. Regional brain atrophy and functional disconnection in Broca’s area in individuals at ultra-high risk for psychosis and schizophrenia. PloS One (2012) 7:e51975. doi: 10.1371/journal.pone.0051975
90. Alonso-Solis A, Vives-Gilabert Y, Grasa E, Portella MJ, Rabella M, Sauras RB, et al. Resting-state functional connectivity alterations in the default network of schizophrenia patients with persistent auditory verbal hallucinations. Schizophr Res (2015) 161:261–8. doi: 10.1016/j.schres.2014.10.047
91. Bluhm RL, Miller J, Lanius RA, Osuch EA, Boksman K, Neufeld RW, et al. Retrosplenial cortex connectivity in schizophrenia. Psychiatry Res (2009) 174:17–23. doi: 10.1016/j.pscychresns.2009.03.010
92. Zhou Y, Ma X, Wang D, Qin W, Zhu J, Zhuo C, et al. The selective impairment of resting-state functional connectivity of the lateral subregion of the frontal pole in schizophrenia. PloS One (2015) 10:e0119176. doi: 10.1371/journal.pone.0119176
93. Kirino E, Tanaka S, Fukuta M, Inami R, Arai H, Inoue R, et al. Simultaneous resting-state functional MRI and electroencephalography recordings of functional connectivity in patients with schizophrenia. Psychiatry Clin Neurosci (2017) 71:262–70. doi: 10.1111/pcn.12495
94. Schilbach L, Hoffstaedter F, Muller V, Cieslik EC, Goya-Maldonado R, Trost S, et al. Transdiagnostic commonalities and differences in resting state functional connectivity of the default mode network in schizophrenia and major depression. Neuroimage Clin (2016) 10:326–35. doi: 10.1016/j.nicl.2015.11.021
95. Guo W, Liu F, Chen J, Wu R, Li L, Zhang Z, et al. Using short-range and long-range functional connectivity to identify schizophrenia with a family-based case–control design. Psychiatry Res Neuroimaging (2017) 264:60–7. doi: 10.1016/j.pscychresns.2017.04.010
96. Fan FM, Tan SP, Yang FD, Tan YL, Zhao YL, Chen N, et al. Ventral medial prefrontal functional connectivity and emotion regulation in chronic schizophrenia: a pilot study. Neurosci Bull (2013) 29:59–74. doi: 10.1007/s12264-013-1300-8
97. Hadley JA, Nenert R, Kraguljac NV, Bolding MS, White DM, Skidmore FM, et al. Ventral tegmental area/midbrain functional connectivity and response to antipsychotic medication in schizophrenia. Neuropsychopharmacology (2014) 39:1020–30. doi: 10.1038/npp.2013.305
98. Lui S, Yao L, Xiao Y, Keedy SK, Reilly JL, Keefe RS, et al. Resting-state brain function in schizophrenia and psychotic bipolar probands and their first-degree relatives. Psychol Med (2015) 45:97–108. doi: 10.1017/S003329171400110X
99. Lang X, Wang L, Zhuo CJ, Jia F, Wang LN, Wang CL. Reduction of interhemispheric functional connectivity in sensorimotor and visual information processing pathways in schizophrenia. Chin Med J (Engl) (2016) 129:2422–6. doi: 10.4103/0366-6999.191758
100. Fang X, Wang Y, Cheng L, Zhang Y, Zhou Y, Wu S, et al. Prefrontal dysconnectivity links to working memory deficit in first-episode schizophrenia. Brain Imaging Behav (2018) 12:335–44. doi: 10.1007/s11682-017-9692-0
101. Liu X, Zhuo C, Qin W, Zhu J, Xu L, Xu Y, et al. Selective functional connectivity abnormality of the transition zone of the inferior parietal lobule in schizophrenia. Neuroimage Clin (2016) 11:789–95. doi: 10.1016/j.nicl.2016.05.021
102. Wylie KP, Tregellas JR. The role of the insula in schizophrenia. Schizophr Res (2010) 123:93–104. doi: 10.1016/j.schres.2010.08.027
103. Sun J, Maller JJ, Guo L, Fitzgerald PB. Superior temporal gyrus volume change in schizophrenia: a review on region of interest volumetric studies. Brain Res Rev (2009) 61:14–32. doi: 10.1016/j.brainresrev.2009.03.004
104. Andrews-Hanna JR, Reidler JS, Sepulcre J, Poulin R, Buckner RL. Functional-anatomic fractionation of the brain’s default network. Neuron (2010) 65:550–62. doi: 10.1016/j.neuron.2010.02.005
105. Fransson P, Marrelec G. The precuneus/posterior cingulate cortex plays a pivotal role in the default mode network: evidence from a partial correlation network analysis. Neuroimage (2008) 42:1178–84. doi: 10.1016/j.neuroimage.2008.05.059
106. Etkin A, Egner T, Kalisch R. Emotional processing in anterior cingulate and medial prefrontal cortex. Trends Cogn Sci (2011) 15:85–93. doi: 10.1016/j.tics.2010.11.004
107. Bai F, Shi Y, Yuan Y, Wang Y, Yue C, Teng Y, et al. Altered self-referential network in resting-state amnestic type mild cognitive impairment. Cortex (2012) 48:604–13. doi: 10.1016/j.cortex.2011.02.011
108. Ohi K, Matsuda Y, Shimada T, Yasuyama T, Oshima K, Sawai K, et al. Structural alterations of the superior temporal gyrus in schizophrenia: detailed subregional differences. Eur Psychiatry (2016) 35:25–31. doi: 10.1016/j.eurpsy.2016.02.002
109. Kasai K, Shenton ME, Salisbury DF, Hirayasu Y, Onitsuka T, Spencer MH, et al. Progressive decrease of left Heschl gyrus and planum temporale gray matter volume in first-episode schizophrenia: a longitudinal magnetic resonance imaging study. Arch Gen Psychiatry (2003) 60:766–75. doi: 10.1001/archpsyc.60.8.766
110. Pearlson GD. Superior temporal gyrus and planum temporale in schizophrenia: a selective review. Prog Neuropsychopharmacol Biol Psychiatry (1997) 21:1203–29. doi: 10.1016/S0278-5846(97)00159-0
111. Woodruff PW, Wright IC, Bullmore ET, Brammer M, Howard RJ, Williams SC, et al. Auditory hallucinations and the temporal cortical response to speech in schizophrenia: a functional magnetic resonance imaging study. Am J Psychiatry (1997) 154:1676–82. doi: 10.1176/ajp.154.12.1676
112. David AS, Woodruff PW, Howard R, Mellers JD, Brammer M, Bullmore E, et al. Auditory hallucinations inhibit exogenous activation of auditory association cortex. Neuroreport (1996) 7:932–6. doi: 10.1097/00001756-199603220-00021
113. Kringelbach ML, Rolls ET. The functional neuroanatomy of the human orbitofrontal cortex: evidence from neuroimaging and neuropsychology. Prog Neurobiol (2004) 72:341–72. doi: 10.1016/j.pneurobio.2004.03.006
114. Damasio A. Feelings of emotion and the self. Ann N Y Acad Sci (2003) 1001:253–61. doi: 10.1196/annals.1279.014
116. Rolls ET. The orbitofrontal cortex and reward. Cereb Cortex (2000) 10:284–94. doi: 10.1093/cercor/10.3.284
117. Rolls ET. Precis of the brain and emotion. Behav Brain Sci (2000) 23:177–91; discussion 92–233. doi: 10.1017/S0140525X00002429
118. Barbas H. Connections underlying the synthesis of cognition, memory, and emotion in primate prefrontal cortices. Brain Res Bull (2000) 52:319–30. doi: 10.1016/S0361-9230(99)00245-2
119. Wolf ND, Sambataro F, Vasic N, Frasch K, Schmid M, Schonfeldt-Lecuona C, et al. Dysconnectivity of multiple resting-state networks in patients with schizophrenia who have persistent auditory verbal hallucinations. J Psychiatry Neurosci (2011) 36:366–74. doi: 10.1503/jpn.110008
120. Moseley P, Fernyhough C, Ellison A. Auditory verbal hallucinations as atypical inner speech monitoring, and the potential of neurostimulation as a treatment option. Neurosci Biobehav Rev (2013) 37:2794–805. doi: 10.1016/j.neubiorev.2013.10.001
121. Allen P, Laroi F, McGuire PK, Aleman A. The hallucinating brain: a review of structural and functional neuroimaging studies of hallucinations. Neurosci Biobehav Rev (2008) 32:175–91. doi: 10.1016/j.neubiorev.2007.07.012
122. Raichle ME. The brain’s default mode network. Annu Rev Neurosci (2015) 38:433–47. doi: 10.1146/annurev-neuro-071013-014030
123. Menon V. Large-scale brain networks and psychopathology: a unifying triple network model. Trends Cogn Sci (2011) 15:483–506. doi: 10.1016/j.tics.2011.08.003
124. Palaniyappan L, Mallikarjun P, Joseph V, White TP, Liddle PF. Regional contraction of brain surface area involves three large-scale networks in schizophrenia. Schizophr Res (2011) 129:163–8. doi: 10.1016/j.schres.2011.03.020
125. Mishara A, Bonoldi I, Allen P, Rutigliano G, Perez J, Fusar-Poli P, et al. Neurobiological models of self-disorders in early schizophrenia. Schizophr Bull (2016) 42:874–80. doi: 10.1093/schbul/sbv123
126. Allen P, Modinos G, Hubl D, Shields G, Cachia A, Jardri R, et al. Neuroimaging auditory hallucinations in schizophrenia: from neuroanatomy to neurochemistry and beyond. Schizophr Bull (2012) 38:695–703. doi: 10.1093/schbul/sbs066
127. Jones SR. Do we need multiple models of auditory verbal hallucinations? Examining the phenomenological fit of cognitive and neurological models. Schizophr Bull (2010) 36:566–75. doi: 10.1093/schbul/sbn129
128. Skelly LR, Calhoun V, Meda SA, Kim J, Mathalon DH, Pearlson GD. Diffusion tensor imaging in schizophrenia: relationship to symptoms. Schizophr Res (2008) 98:157–62. doi: 10.1016/j.schres.2007.10.009
129. Mitelman SA, Brickman AM, Shihabuddin L, Newmark RE, Hazlett EA, Haznedar MM, et al. A comprehensive assessment of gray and white matter volumes and their relationship to outcome and severity in schizophrenia. Neuroimage (2007) 37:449–62. doi: 10.1016/j.neuroimage.2007.04.070
130. Repovs G, Csernansky JG, Barch DM. Brain network connectivity in individuals with schizophrenia and their siblings. Biol Psychiatry (2011) 69:967–73. doi: 10.1016/j.biopsych.2010.11.009
131. Jang JH, Jung WH, Choi JS, Choi CH, Kang DH, Shin NY, et al. Reduced prefrontal functional connectivity in the default mode network is related to greater psychopathology in subjects with high genetic loading for schizophrenia. Schizophr Res (2011) 127:58–65. doi: 10.1016/j.schres.2010.12.022
132. Ford JM, Mathalon DH. Electrophysiological evidence of corollary discharge dysfunction in schizophrenia during talking and thinking. J Psychiatr Res (2004) 38:37–46. doi: 10.1016/S0022-3956(03)00095-5
133. Frith CD, Done DJ. Towards a neuropsychology of schizophrenia. Br J Psychiatry (1988) 153:437–43. doi: 10.1192/bjp.153.4.437
134. Bernard JA, Goen JRM, Maldonado T. A case for motor network contributions to schizophrenia symptoms: evidence from resting-state connectivity. Hum Brain Mapp (2017) 38:4535–45. doi: 10.1002/hbm.23680
135. Watanuki T, Matsuo K, Egashira K, Nakashima M, Harada K, Nakano M, et al. Precentral and inferior prefrontal hypoactivation during facial emotion recognition in patients with schizophrenia: a functional near-infrared spectroscopy study. Schizophr Res (2016) 170:109–14. doi: 10.1016/j.schres.2015.11.012
136. Zhou SY, Suzuki M, Hagino H, Takahashi T, Kawasaki Y, Matsui M, et al. Volumetric analysis of sulci/gyri-defined in vivo frontal lobe regions in schizophrenia: precentral gyrus, cingulate gyrus, and prefrontal region. Psychiatry Res (2005) 139:127–39. doi: 10.1016/j.pscychresns.2005.05.005
137. Woodward ND, Karbasforoushan H, Heckers S. Thalamocortical dysconnectivity in schizophrenia. Am J Psychiatry (2012) 169:1092–9. doi: 10.1176/appi.ajp.2012.12010056
138. Bernard JA, Orr JM, Mittal VA. Cerebello-thalamo-cortical networks predict positive symptom progression in individuals at ultra-high risk for psychosis. Neuroimage Clin (2017) 14:622–8. doi: 10.1016/j.nicl.2017.03.001
139. Power JD, Cohen AL, Nelson SM, Wig GS, Barnes KA, Church JA, et al. Functional network organization of the human brain. Neuron (2011) 72:665–78. doi: 10.1016/j.neuron.2011.09.006
140. Alderson-Day B, Lima CF, Evans S, Krishnan S, Shanmugalingam P, Fernyhough C, et al. Distinct processing of ambiguous speech in people with non-clinical auditory verbal hallucinations. Brain (2017) 140:2475–89. doi: 10.1093/brain/awx206
141. Lavigne KM, Menon M, Woodward TS. Functional brain networks underlying evidence integration and delusions in schizophrenia. Schizophr Bull (2019). doi: 10.1093/schbul/sbz032 [Epub ahead of print]
142. Liu ZX, Grady C, Moscovitch M. Effects of prior-knowledge on brain activation and connectivity during associative memory encoding. Cereb Cortex (2017) 27:1991–2009. doi: 10.1093/cercor/bhw047
143. van Erp TGM, Walton E, Hibar DP, Schmaal L, Jiang W, Glahn DC, et al. Cortical brain abnormalities in 4474 individuals with schizophrenia and 5098 control subjects via the Enhancing Neuro Imaging Genetics Through Meta Analysis (ENIGMA) Consortium. Biol Psychiatry (2018) 84:644–54. doi: 10.1016/j.biopsych.2018.04.023
144. van Erp TG, Hibar DP, Rasmussen JM, Glahn DC, Pearlson GD, Andreassen OA, et al. Subcortical brain volume abnormalities in 2028 individuals with schizophrenia and 2540 healthy controls via the ENIGMA consortium. Mol Psychiatry (2016) 21:585. doi: 10.1038/mp.2015.118
Keywords: schizophrenia, resting state, magnetic resonance imaging, functional connectivity, brain network, meta-analysis
Citation: Li S, Hu N, Zhang W, Tao B, Dai J, Gong Y, Tan Y, Cai D and Lui S (2019) Dysconnectivity of Multiple Brain Networks in Schizophrenia: A Meta-Analysis of Resting-State Functional Connectivity. Front. Psychiatry 10:482. doi: 10.3389/fpsyt.2019.00482
Received: 22 February 2019; Accepted: 19 June 2019;
Published: 12 July 2019.
Edited by:
André Schmidt, University of Basel, SwitzerlandReviewed by:
Matthias Kirschner, Psychiatrische Klinik der Universität Zürich, SwitzerlandDebo Dong, University of Electronic Science and Technology of China, China
Copyright © 2019 Li, Hu, Zhang, Tao, Dai, Gong, Tan, Cai and Lui. This is an open-access article distributed under the terms of the Creative Commons Attribution License (CC BY). The use, distribution or reproduction in other forums is permitted, provided the original author(s) and the copyright owner(s) are credited and that the original publication in this journal is cited, in accordance with accepted academic practice. No use, distribution or reproduction is permitted which does not comply with these terms.
*Correspondence: Su Lui, lusuwcums@tom.com
†These authors have contributed equally to this work.