- Department of Magnetic Resonance Imaging, the First Affiliated Hospital of Zhengzhou University, Zhengzhou, China
Background: The findings of many neuroimaging studies in patients with first-episode major depressive disorder (MDD), and even those of previous meta-analysis, are divergent. To quantitatively integrate these studies, we performed a meta-analysis of gray matter volumes using voxel-based morphometry (VBM).
Methods: We performed a comprehensive literature search for relevant studies and traced the references up to May 1, 2021 to select the VBM studies between first-episode MDD and healthy controls (HC). A quantitative meta-analysis of VBM studies on first-episode MDD was performed using the Seed-based d Mapping with Permutation of Subject Images (SDM-PSI) method, which allows a familywise error rate (FWE) correction for multiple comparisons of the results. Meta-regression was used to explore the effects of demographics and clinical characteristics.
Results: Nineteen studies, with 22 datasets comprising 619 first-episode MDD and 707 HC, were included. The pooled and subgroup meta-analysis showed robust gray matter reductions in the left insula, the bilateral parahippocampal gyrus extending into the bilateral hippocampus, the right gyrus rectus extending into the right striatum, the right superior frontal gyrus (dorsolateral part), the left superior frontal gyrus (medial part) and the left superior parietal gyrus. Meta-regression analyses showed that higher HDRS scores were significantly more likely to present reduced gray matter volumes in the right amygdala, and the mean age of MDD patients in each study was negatively correlated with reduced gray matter in the left insula.
Conclusions: The present meta-analysis revealed that structural abnormalities in the fronto-striatal-limbic and fronto-parietal networks are essential characteristics in first-episode MDD patients, which may become a potential target for clinical intervention.
Introduction
Major depressive disorder (MDD), the third leading cause of global disease burden and a leading cause of disability worldwide, is a prevalent mental disorder caused by many complex factors, such as trauma, stress, psychological, and even some social or genetic factors (1). The pathophysiology of MDD has not yet to be fully elucidated and no preventive treatments are currently available. Thus, the investigation of the etiology, therapy and relevant biomarkers of MDD is of high importance for society and current research.
As a non-invasive imaging technique, structural magnetic resonance (MR) imaging has shown great potential value in elucidating the neuropathogenesis of psychiatric disorders. Structural MR imaging studies, which are paradigm free, are potentially able to make comparisons containing fewer biases across studies (2). Voxel-based morphometry (VBM) is a whole-brain and automatic technique which has accuracy comparable with manual volumetry and overcomes the technical limitations of region-of-interest approaches (1, 3). Notably, VBM has become an established research method to detect neuromorphometric abnormalities in subjects with various psychiatric disorders, such as schizophrenia, bipolar disorder, and obsessive–compulsive disorder, including MDD (4–11).
Previous VBM studies have demonstrated alterations in gray matter volume in MDD patients, however, the results vary considerably across studies. For example, Serra-Blasco et al., Stratmann et al. and Lu et al. did not find any significant gray matter difference between MDD patients and HC, whereas Zhang et al. and Kong et al. have detected increased gray matter volume (12–16). While, most studies have identified decreased gray matter volume in a wide range of brain regions, including the pre-supplementary motor area, the parietal-temporal regions, the frontal cortex, the temporal cortex, the cingulate cortex, the insular cortex, the parahippocampal gyrus, the hippocampus, the cerebellum and the orbitofrontal cortex (12, 16–24). These inconsistencies mainly might be contributed to the heterogeneity of MDD patients, which came from clinical and demographic characteristics of patients, the used imaging protocols and devices, the sample sizes and the technical methods of data acquisition and analysis. Two of the most important interferences are medications and chronic episodes, which are resulting in difficulty explaining the core pathophysiology of this disease independently from the potentially confounding factors mentioned above. In addition, MDD patients with comorbidity (such as anxiety disorders) are among the most frequently occurring psychiatric conditions and commonly present occur together (25) and often undifferentiated in many studies (26). Thus, we speculate that the results may have been confounded when MDD patients with/without comorbidity were grouped together in previous meta-analysis studies (1, 27–29). Therefore, to better understand the whole brain primary morphometric changes in MDD patients, the above-mentioned limitations need to be overcome in research. For this aim, we conducted subgroup meta-analysis to evaluate the potentially confounding effect including medication and comorbidity in first-episode MDD patients. To our knowledge, this is the first meta-analysis of VBM studies in patients with MDD that examines whether comorbidity affect the gray matter volumes or not with the latest version of anisotropic effect size seed-based d mapping (ES-SDM).
The ES-SDM is a coordinate-based meta-analytic technique (10, 11), which has some advantages over the previous approaches. Firstly, it allows all the useful information from contributing studies to be used in the same map including both positive and negative differences, which can prevent a particular voxel from appearing to be significant in opposite directions. Secondly, SDM has some complementary analyses, such as jack-knife, subgroup and meta-regression analyses, which can be used to assess the robustness and heterogeneity of the results (30). Since the updated version of ES-SDM, namely, the Seed-based d Mapping with Permutation of Subject Images (SDM-PSI) allows a FWE correction for multiple comparisons of the results, we will conduct a quantitative analysis using the SDM-PSI method to reflect intrinsic brain structure of MDD.
Methods
Study Selection
On the basis of the Preferred Reporting Items for Systematic Reviews and Meta-Analyses (PRISMA) (see Supplementary Table 1) statement (31), we performed a systematic and comprehensive literature search in PubMed, Embase, Web of Science, and Ovid databases for relevant studies published up to May 1, 2021. The search strategy was: “VBM,” or “voxel-based,” or “morphometry” or “voxel-based morphometry” and “depression,” or “depressive disorder,” or “major depression,” or “major depressive disorder,” or “depressed.” We also checked the reference lists and review articles to identify studies that may have been missed in the original search. Two researchers (R.P.Z and Z.G.Y) independently searched the articles. A study was included if it was: (1) written in English and peer reviewed, (2) enrolled patients diagnosed with first-episode MDD and a matched HC group, (3) used VBM to analysis whole-brain gray matter volume changes, (4) clearly reported three coordinates (x, y, z) in a stereotactic space (Talairach or MNI). The exclusion criteria were as follows: (1) no first-episode MDD patients versus HC, (2) using ROI or seed voxel-based analysis, (3) missing important information on the results (e.g., coordinates of significant clusters [P < 0.05]) even after contacting the corresponding author. If there were some inconsistent opinions, two researchers reached a consensus results through the discussion, and then moved to the following steps.
Quality Assessment
The quality of all enrolled articles was independently accessed using a 10-point checklist by two authors (R.P.Z and Z.G.Y) (29, 32). The checklist included diagnostic criteria applied, demographic and clinical characteristics, the sample size, the quality of the reported results, the methods of image acquisition, imaging technique (see Supplementary Table 2). Although the checklist was not designed as an assessment tool, it still provided some objective indicators of the rigor of each study. If the rating results were inconsistent, the two authors reached a unified quality score by discussion. The final quality scores are shown in Supplementary Table 1.
Voxel-Wise Meta-Analysis
The coordinate-based meta-analysis was conducted by using SDM-PSI software version 6.21 (www.sdmproject.com/software/), which has been described in detail in some studies (33, 34) and the SDM-PSI reference manual (https://www.sdmproject.com/manual/), here we only described it briefly. Its standard procedures include: calculation of the maps of the lower and upper bounds of possible effect sizes for each study separately based on the peak information, full anisotropy = 1, isotropic full width half maximum = 20 mm, and voxel = 2 mm; the mean analysis: estimation of the map of most likely effect size and its standard error, conducting multiple imputations of the maps of effect size of the individual studies, meta-analysis of these maps using a standard random-effects model, and Rubin rules to pool the different meta-analyses resulting from the multiple imputations; FWE correction for multiple comparisons using common permutation tests (p < 0.05); and finally use of threshold-free cluster enhancement (TFCE) in the statistical thresholding (p < 0.05, voxel extent ≥10).
Moreover, we conducted jackknife sensitivity, heterogeneity and meta-regression analyses using SDM. Jackknife sensitivity analysis was conducted to assess the reproducibility of the results by the procedure of repeating the meta-analysis after discarding one study each time (30). Heterogeneity analysis was performed to explore unexplained inter-study variability of the results. Although I2 statistics were used widely to assess the heterogeneity (I2 < 50% indicates low heterogeneity) in previous meta-analysis, I2 has been questioned for accuracy and reliability by some researchers (35).Thus we combined tau2 and I2 to explore inter-study heterogeneity (30). Egger's tests were calculated to assess potential publication bias (p < 0.05 indicates obvious publication bias). Meta-regression analysis was conducted to explore the potential effects of clinical variables (p < 0.00005, uncorrected, voxels > 10 indicates statistical differences), such as gender rations, mean age, education duration, illness duration, and severity of depression symptoms, by means of simple linear regression. Additional, two subgroup meta-analyses were performed to eliminate the effect of medication and comorbidity. Firstly, we conducted a subgroup analysis of first-episode medication-naïve MDD patients (n = 477) to eliminate confounders such as illness duration and previous antidepressant treatment. Secondly, two subgroup analysis of first-episode MDD patients without comorbidity (n = 402) and with comorbidity (n = 149) were performed to control the confounding factors of comorbidity.
Results
Included Studies and Sample Characteristics
The identification and attrition of the studies are shown in Figure 1. Finally, the search identified 2,044 studies, and only 19 studies met the inclusion criteria (7, 12–24, 36–40), of which three reported two separate experiments (7, 20, 39). Our final sample consisted of 619 first-episode MDD patients (371 females and 248 males; mean age 33.43 ± 4.71 years) and 707 HCs (403 females and 304 males; mean age 32.90 ± 4.79 years). In addition, some more basic information about the subjects is shown in Table 1. There was no significant difference in age, sex, education duration between MDD patients and HCs (all p > 0.05). Sixteen out of 22 datasets included patients who were medication-naïve, which included 477 medication-naïve MDD (288 females and 189 males; mean age 32.02 ± 4.21 years) patients and 533 HCs (307 females and 226 males; mean age 31.52 ± 4.16 years). Thirteen out of 22 datasets included patients who were without comorbidities, which included 372 patients with first-episode MDD (213 females and 159 males; mean age 36.54 ± 4.69 years) and 431 HCs (231 females and 200 males; mean age 36.41 ± 4.32 years).
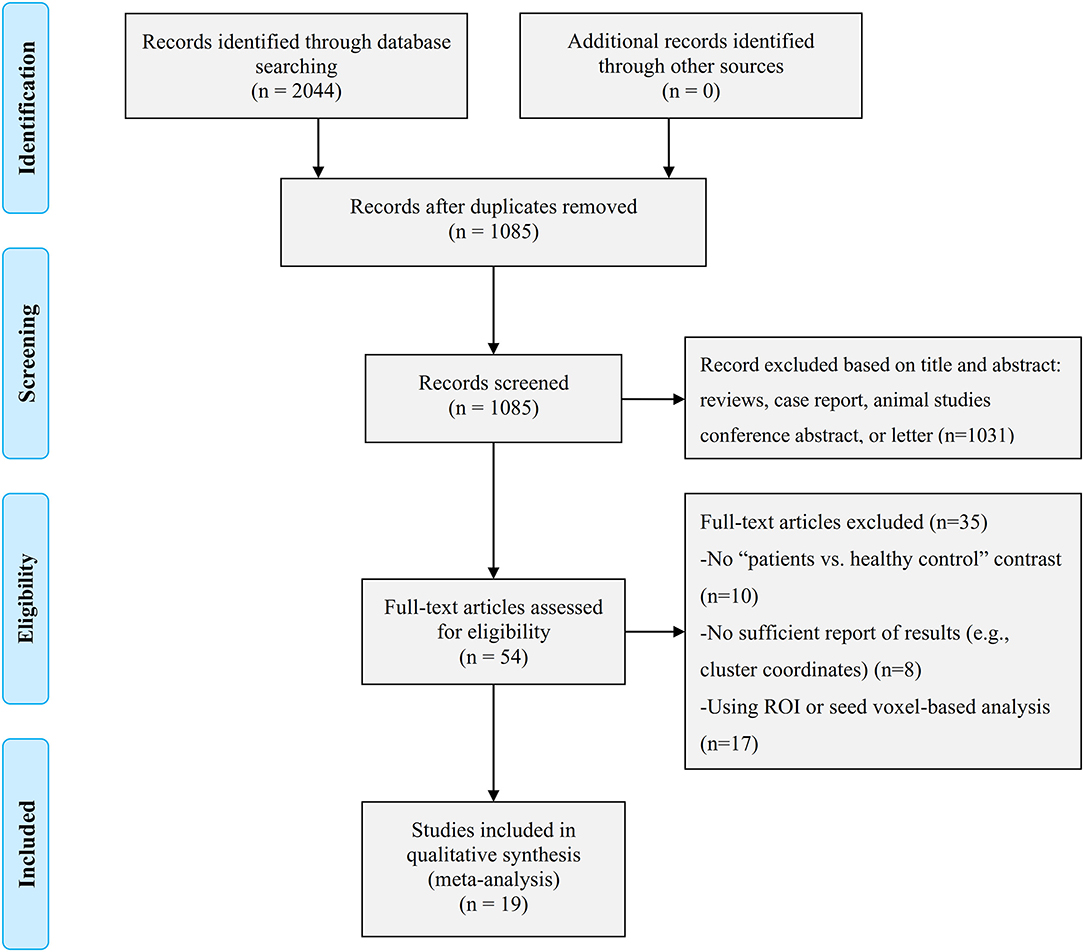
Figure 1. Search strategy used for the inclusion of the studies considered in the current meta-analysis.
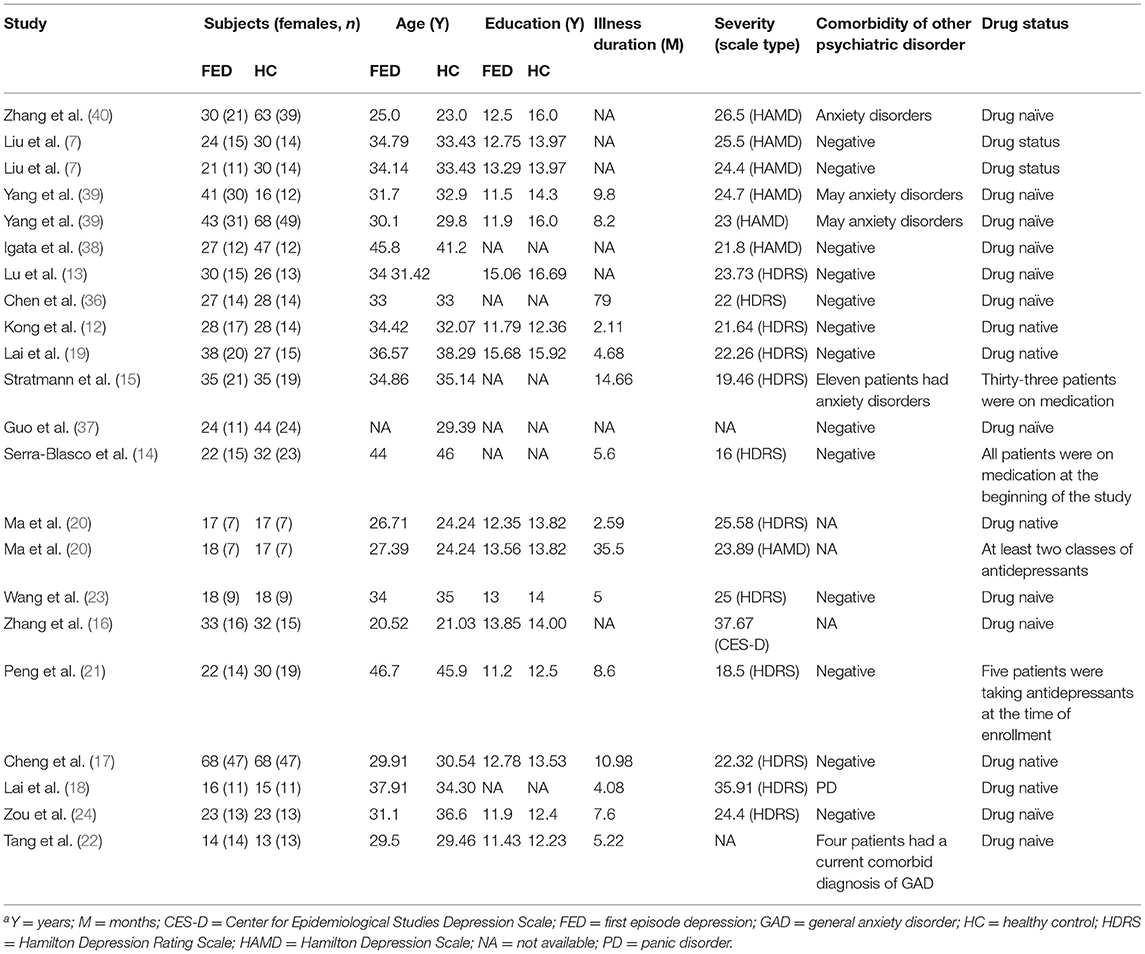
Table 1. Demographic and clinical characteristics of subjects in the 22 voxel-based morphometry datasets included in the meta-analysisa.
Pooled Meta-Analysis
In the pooled meta-analysis, using a threshold of FWE-corrected p < 0.05, first-episode MDD patients showed gray matter volume reduction in the left insula (INS), bilateral parahippocampal gyrus (PHG) extending into the bilateral hippocampus (HIP), right gyrus rectus (REC), right dorsolateral part of superior frontal gyrus (SFGdor), right striatum, left medial part of superior frontal gyrus (SFGmed), and left superior parietal gyrus (SPG), compared with HCs. No increased gray matter volume was found in MDD patients (Figure 2 and Table 2).
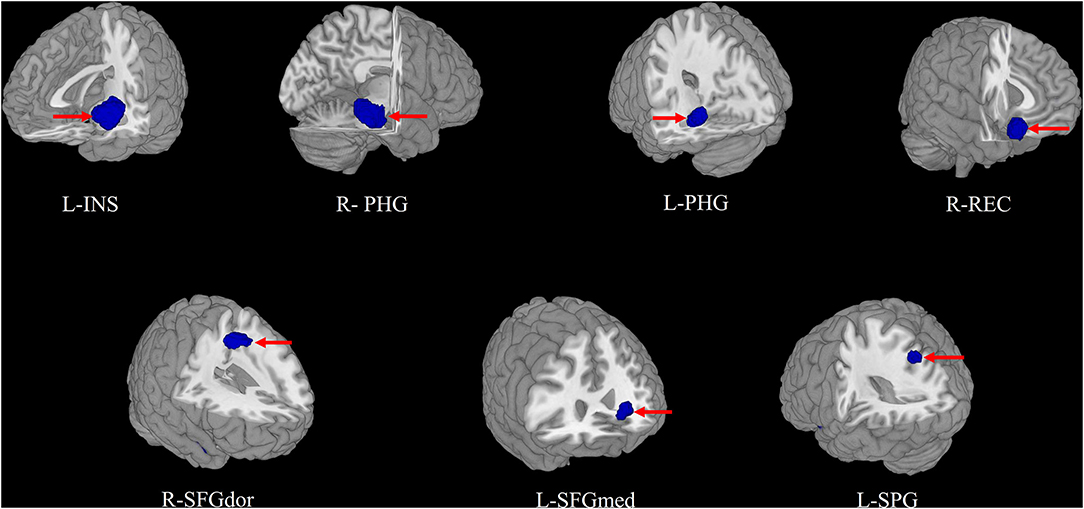
Figure 2. The areas of decreased (blue) gray matter volumes in patients with first episode depression (FED) compared with healthy controls in the pooled meta-analysis. INS, insula; PHG, parahippocampal gyrus; REC, gyrus rectus; SFGdor, superior frontal gyrus, dorsolateral; SFGmed, superior frontal gyrus, medial; SPG, superior parietal gyrus; L, left; R, right.
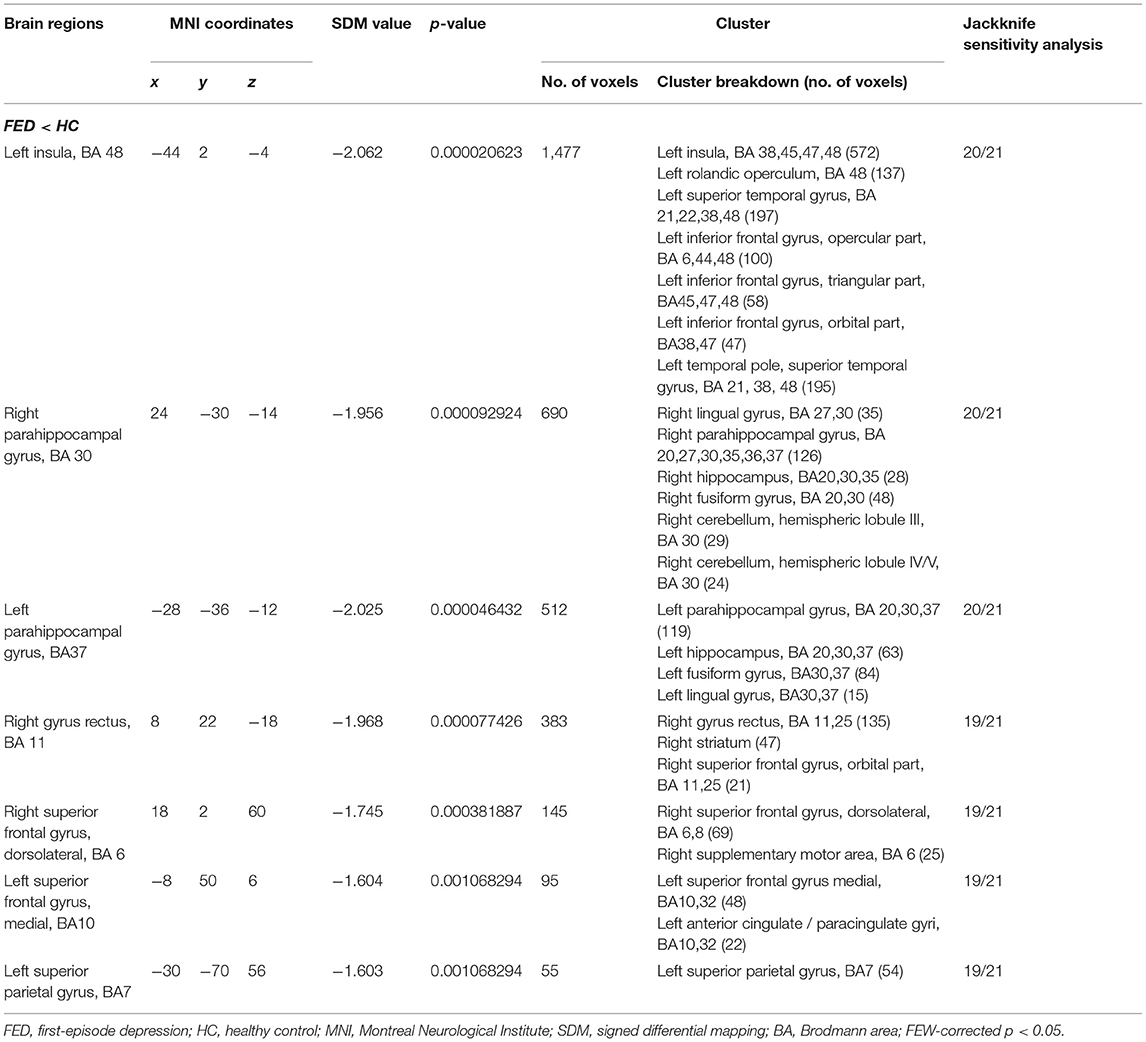
Table 2. Regional differences in gray matter volume between FED patients and healthy controls in the pooled meta-analysis.
Reliability Analyses
The results of whole-brain jackknife sensitivity analysis between first-episode MDD and HC (Supplementary Table 3) showed that the gray matter volume reduction in the left INS and left PHG was highly replicable, as this finding was replicated across all 21 combinations of the datasets. The gray matter volume reduction in the right PHG was also significant in all but one of the datasets. The gray matter volume reduction in the right GR, the right SFGdor, the left SFGmed, the left SPG was also significant in all but two of the datasets.
Subgroup Analysis of First-Episode, Medication-Naïve MDD (16 Datasets)
The subgroup analysis of first-episode, medication-naïve MDD included 16 datasets comprising 477 medication-naïve MDD patients and 533 HCs. This analysis revealed that MDD patients, relative to controls, showed decreased gray matter volume in the left INS, right REC, right SFGdor, left SFGmed, left SPG, and right amygdala.
Subgroup Analysis of First-Episode MDD Without Comorbidities (13 Datasets)
The subgroup analysis of first-episode MDD without comorbidities included 13 datasets comprising 372 patients with first-episode MDD and 431 HCs. This analysis revealed gray matter volume decreases in the left INS, bilateral PHG, and right SFGdor in first-episode MDD patients without comorbidities compared with HCs (Supplementary Table 4).
Subgroup Analysis of First-Episode MDD With Comorbidities (6 Datasets)
The subgroup analysis of first-episode MDD with comorbidities included 6 datasets comprising 179 patients with first-episode MDD and 210 HCs. This analysis showed gray matter volume decreases in the right SFGorb, left SFGmed, left SPG in first-episode MDD patients with comorbidities compared with HCs (Supplementary Table 4).
Analysis of Heterogeneity and Publication Bias
Heterogeneity analyses exhibited low inter-study variability in the left INS. Moreover, we used a funnel plot and Egger's test to assess potential publication biases for the brain regions identified in the meta-analysis. In all seven clusters, the funnel plots were found to be roughly symmetric, except for only one region (left PHG, Egger test: P = 0.027), and Egger's tests did not detect significant differences, suggesting that there was nearly no publication bias in our main findings (Supplementary Figure 1).
Meta-Regression Analyses
Meta-regression analyses showed that the HDRS score was negatively correlated with the gray matter volume in the right amygdala (BA 34; MNI coordinate: x = 28, y = 0, z = −20; SDM-Z = −1.906, P = 0.0001, voxel = 16) (Figure 3). The gray matter volume in the left insula (BA 48; MNI coordinate: x = −44, y = 10, z = −8; SDM-Z = −3.605, P = ~0, voxel = 1,055) was shown to be modulated by age (Figure 4). However, this result should be interpreted with caution as it was driven by only three studies. No linear associations with sex ratio, education duration and illness duration were observed.
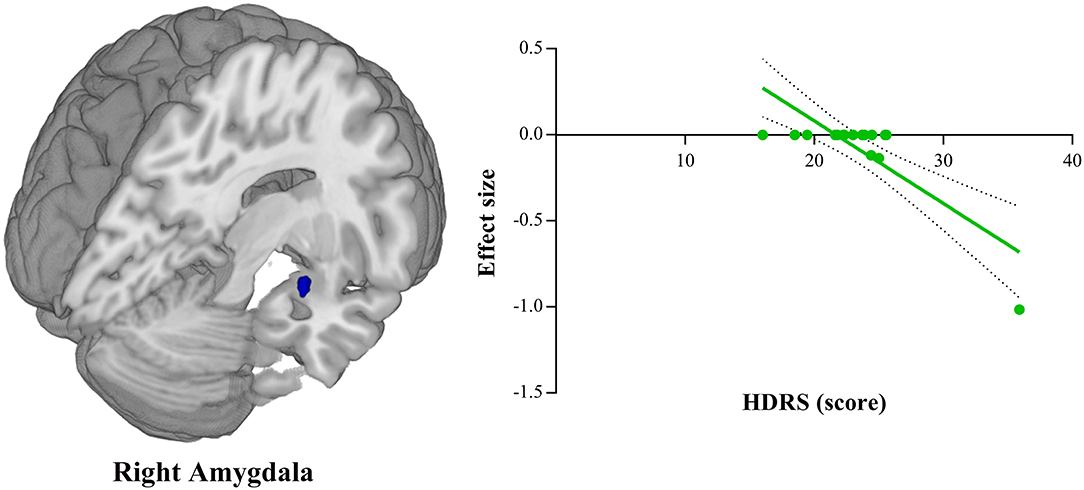
Figure 3. Meta-regression analysis shows that Hamilton Depression Rating Scale (HDRS) score of depressed patients is negatively correlated with gray matter volume in the right amygdala. In the graphs, the effect sizes needed to create this plot have been extracted from the peak of maximum slope significance, and each study is represented as a dot. The regression line (meta-regression signed differential mapping slope) is shown.
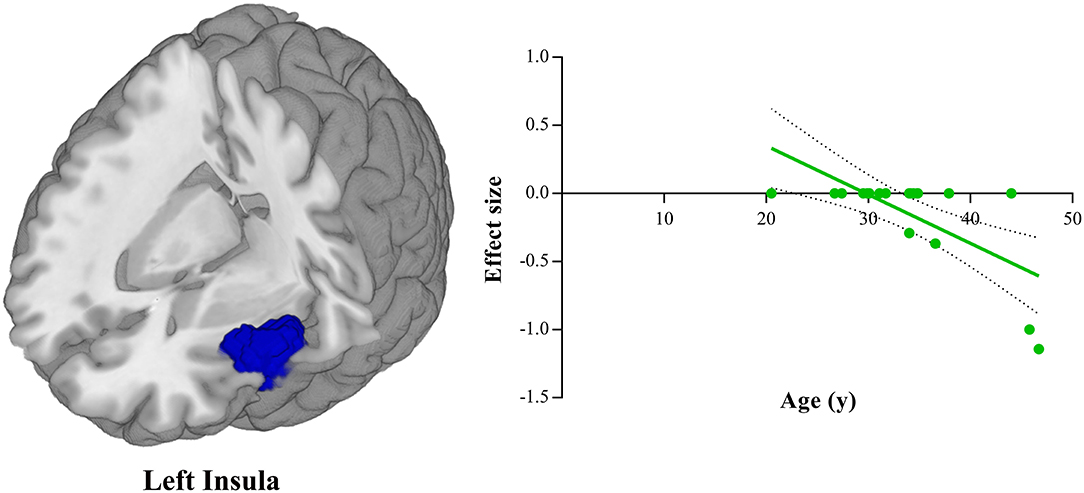
Figure 4. Meta-regression analysis shows that mean age of depressed patients is negatively correlated with gray matter volume in the left insula. In the graphs, the effect sizes needed to create this plot have been extracted from the peak of maximum slope significance, and each study is represented as a dot. The regression line (meta-regression signed differential mapping slope) is shown.
Discussion
VBM is the most common way to identify abnormal gray matter volume in the clinical diseases. Our study integrated the findings from 19 VBM studies using AES-SDM, in which first-episode MDD patients were compared with HC. Without the influence of recurrence and external tasks, this whole-brain meta-analysis, comparing gray matter volume between first-episode MDD patients and HC, could reflect the intrinsic relationship between structural changes and pathologic processes in MDD. Brain regions with increased gray matter volume were not observed. In addition, to clarify the pathological mechanisms of pure MDD patients without comorbidity, we further conduct a subgroup. Both pooled and subgroup meta-analysis identified decreased gray matter volume in the fronto-striatal-limbic circuits. And, this meta-analysis founded that the HDRS score was negatively correlated with the gray matter volume in the right amygdala and the age was negatively correlated with gray matter volume in the left insula.
Most of the brain regions with reduced gray matter volume were located in the limbic system, including the left INS, bilateral PHG, bilateral HIP. The limbic system has been theoretically identified to play an important role in the pathophysiology of MDD by regulating the balance between experience, emotion and behavior, motivation and long-term memory (41, 42), because it has widespread connections to extensive cortical areas known as the neuroanatomical circuits of mood regulation (43). As an important part of limbic system, the INS has extensive connectivity with fronto-limbic regions, which may partly explain the difficulties in cognitive and emotional integration that characterize the clinical manifestations of MDD (12, 15, 19, 38, 44–50). Although many neuroimaging studies, involving in VBM and fMRI, have demonstrated the INS was concerning with MDD patients, the findings were inconsistent. Peng et al. showed a volume reduction of the bilateral insular in first-episode MDD patients (28). Kong et al. founded gray matter volume of the right insular increased in medication-naïve MDD patients (12), while a reduction of gray matter volume in the left insular was founded in first-episode medication-naïve MDD patients in a study conducted by Lai and Wu (19). Our findings are supported by many previous VBM studies that demonstrated a volume reduction of the left insular lobe in MDD patients (19, 51). This finding may reflect the heterogeneity of the right insular in MDD. It is also likely reflecting differential responses to the confining environment, such as the MRI scanner, and the different characteristics of subjects. Further studies are needed to determine their relative contributions to MDD pathology. The HIP and PHG, involved in the pathophysiology of MDD, are also important components of limbic system and limbic-cortical-striatal-pallidal-thalamic networks (52). They are assigned a pivotal role in assessing novel items, information retrieval success, visual memory, spatial memory, and recollection memory (53–55), and therefore damages to these regions could lead to diverse symptoms in MDD. Numerous neuroimaging studies have consistently demonstrated the deficits in gray matter volume in the HIP or PHG in various MDD samples, involved in first-episode patients, recurrent patients, medicated patients and medication-naïve patients, suggesting that the altered volume of HIP or PHG might be a trait-related biomarker to characterize MDD (7, 15, 56–60). However, results from these study differ in localization of HIP or PHG volume reduction with studies showing bilateral (7, 17, 24, 61, 62), left unilateral (21, 63, 64), and right unilateral (15, 18, 49) atrophy, which might be attributed to some mixed factors, such as sex, age, recurrences, medication, illness severity, educational level, and the magnetic field strength of MR scanner. In the present study, we detected the decreased gray matter volume in the bilateral HIP and HPG in the first-episode patients, consistent with other studies (7, 17, 24). An explanation for HIP atrophy in MDD is provided by the vulnerability hypothesis, suggesting that HIP atrophy is a pre-existing risk factor for MDD (65), and is therefore already evident in first-episode patients. In conclusion, our results of the present study suggest that the reduced gray matter volume of left INS, bilateral HIP and HPG could serve as neuroimaging biomarker for diagnosing MDD.
The striatum (composed of the putamen, the caudate, and the ventral striatum) is an important part of basal ganglia (60, 66). The striatum is involved in the fronto-striatal-limbic circuitry, and it plays an important role in motor and cognitive control, social learning and reward processing (50). Converging evidence suggests that the gray matter volume of striatum was decreased in the initial presentation of MDD patients (67–69), although not all studies replicated this finding. In the present study, we detected a decrease in the right striatum. The role of the striatum in MDD is not only supported by VBM studies but also by rs-fMRI studies. Lai et al. showed that gray matter volume decreased in the bilateral striatum, and this study was included in the present meta-analysis (18). However, we observed the decreased gray matter volume in the striatum on the right side. It is noteworthy that their study included small sample size (16 MDD patients, 15 healthy controls), which may lead to inaccurate results. In addition, rs-fMRI has shown that striatal activity was reduced in reward system defects (60, 70), and decreased reward network connections were found to be associated with depression severity (71). Meanwhile, functional studies have also confirmed the presence of metabolic abnormalities in MDD in the striatum (71). A minority of results does not same in localization of striatum volume loss with studies showing bilateral and right unilateral atrophy, which might be due to potential influencing factors like sex. As the Dluzen et al. found, estrogen appears to have a neuroprotective effect on the striatum, implicating decreased vulnerability in this region in females and increased vulnerability in males (72). Taken together, these findings suggest that the altered volume of striatum may contribute to internal pathophysiology in MDD.
We also found decreased gray matter volume in the right SFGdor and left SFGmed in first-episode MDD patients compared with controls. The dorsolateral prefrontal cortex (DLPFC) and medial prefrontal cortex (MPFC) are important components of the prefrontal lobe (73–75). These findings were in accordance with two postmortem studies on depressive patients which found decreased neuronal and glial cells respect to density, number and size in the prefrontal cortex (76, 77). According to previous studies (60, 78), the prefrontal regions have been considered to be the most common regions to manifest anatomic abnormalities in MDD. The DLPFC is critical components of frontal lobe, and plays an essential role in emotional, motivational, attentional, and executive functions (60, 78). Some studies have proved that the reduced volume of DLPFC correlates well with the hypoactivation during working memory updating and during conscious negative emotion processing in fMRI studies (79–81). The MPFC is a crucial cortical region that integrates information from numerous cortical and subcortical areas and converges updated information to output structures. It has been implicated in a variety of social, cognitive, and affective functions that are commonly impaired in mental illness (82). In recent years, MPFC has aroused increasing attention for its role in depression (83). Previous studies have showed that abnormal functional activity of MPFC has related to altered self-reflection and rumination and MPFC has also been implicated in emotion-regulation process, particularly in the down-regulation of negative affect (84). Our findings of significant gray matter loss in the MPFC replicate previous data (68, 85). Some researchers even supposed that highly variable MPFC-to-DLPFC connectivity may signify weaknesses in brain circuits responsible for cognitive control, and could be related to depressive deficits in executive functioning such as difficulty inhibiting emotional distraction (86, 87). In line with these results, we may speculate that the atrophy of DLPFC and MPFC are some reasons of the occurrence of MDD, and could serve as a neuroimaging biomarker for diagnosing MDD.
We observed decreased gray matter volume in first-episode MDD patients in the left SPG compared with HC. The SPG is part of the default-mode network, and it is involved in the organization, decision making, emotional processing, cognitive changes and predictions of rewards and so on (88, 89). The SPG is also involved in fronto-parietal network. Lai et al. showed decreased gray matter volume has been noted in fronto-parietal regions in MDD patients compared to HC, and then the gray matter volume of fronto-parietal regions increased after medication treatment (90). Cole et al. showed fronto-parietal network (along with other cognitive control networks) plays an important role in against mental disease via its widespread functional connectivity with other networks (91). Alterations in fronto-parietal network functional connectivity have been identified in a number of mental disorders, including depression (92). Some researchers have proved that inefficiency of the fronto-parietal circuit results in lower cognitive control, and then leads to problems with flexible cognition and executive functions, and could be the cause of more typical symptoms of depression like persistent rumination which are very often present in depressive disorders (93). Together with our findings, this suggests that fronto-parietal circuit serves as an important role in cognitive control networks, and alteration of gray matter volume of fronto-parietal regions may lead severe mental disease, including MDD.
To clarify the pathological mechanisms of pure MDD patients without comorbidity, we further conduct a subgroup analysis. Both pooled and subgroup meta-analysis identified decreased gray matter volume in the fronto-striatal-limbic circuits. As we all know, MDD and some other psychiatric disorders, such as anxiety disorder, panic disorder and so on, have an overlap of clinical symptoms, which suggests that they may share similar neurological mechanisms (50, 94, 95). And, patients with MDD often have considerable comorbidity, such as anxiety (96, 97). Then we speculated the results may have been confounded when MDD patients with comorbidity were grouped together in the previous studies. However, despite the clinical importance of comorbidity in MDD, few neuroimaging studies have focused on its brain structural alterations, or its difference from pure depression and depression with comorbidity (98). Peng et al. found that anxious depression had smaller gray matter volume in the fronto-limbic circuits (right inferior frontal gyrus and orbital frontal gyrus), which were in line with our results partly, relative to both non-anxious depression and healthy controls. The part difference may result from that the MDD patients with comorbidity in our meta-analysis include not just anxiety, but other comorbidity, such as panic disorder. Above all, although it is difficult to determine the factor contributing most to the smaller gray matter volumes, some articles have showed that the presence of comorbidity did not affect the rate of response to pharmacotherapy for depression (99), which to some extent was in line with our results that MDD with or without comorbidity may share the similar neurological mechanisms.
Limitations
Some limitations of the current meta-analysis are well-acknowledged. Firstly, the sample size was not very large, especially in the subgroup analysis and meta-regressions, although they were more numerous than in many previous studies (26–29, 100). Secondly, despite controlling for the age and gender, the included studies in the present study varied in terms of the data acquisition, analysis techniques, demographic and clinical characteristics, which can lead to the impact of such heterogeneity on our meta-analysis. Thirdly, the accuracy of our voxel-wise meta-analysis may have been limited, because the accuracy was not derived from an original study rooted in raw statistical image but instead from published studies. Fourthly, due to the limitations of the current researches, we can't ensure all patients enrolled in comorbidity group were MDD patients with comorbidity. In the future study, more independent efforts about comorbidity should be made. Finally, to better understand the core mechanism of MDD, more longitudinal investigations of first-episode medication-naïve patients should be conducted to determine the causal relationships between clinical features and neuroimaging findings.
Conclusions
The results of meta-analysis of all VBM studies implicate regional gray matter reduction in first-episode MDD patients is neural networks of fronto-striatal-limbic and fronto-parietal involved in the emotional and cognitive processing. When the confounding influence of medication or comorbidity was excluded, the subgroup meta-analysis results still focused on the fronto-striatal-limbic and fronto-parietal networks. The meta-regression analysis suggested that structural abnormality in the right amygdala may also be associated with the severity of depressive symptoms and structural abnormality in the left insula may be modulated by age. As such, our findings strongly implicate that the cause of MDD may be the abnormal gray matter volume of some certain brain areas, but whether the abnormal function connection of fronto-striatal-limbic and fronto-parietal networks is still unknown. To understand the complex pathogenic mechanism of MDD associated with fronto-striatal-limbic and fronto-parietal networks in the brain, further neuroimaging studies with a large number of subjects and sophisticated design should be pursued.
Data Availability Statement
The original contributions presented in the study are included in the article/Supplementary Material, further inquiries can be directed to the corresponding author/s.
Author Contributions
RZ and YZ conceived and designed the study. RZ and ZY are responsible for data acquisition. RZ and SH drafted the initial manuscript. JC, YZ, and SH reviewed and revised the manuscript. RZ and JC took responsibility for the paper. All authors read and approved the final manuscript.
Funding
This research study was supported by the Natural Science Foundation of China (Nos. 81601467, 81871327, and 81601472) Medical science and technology research project of Henan province (201701011).
Conflict of Interest
The authors declare that the research was conducted in the absence of any commercial or financial relationships that could be construed as a potential conflict of interest.
Supplementary Material
The Supplementary Material for this article can be found online at: https://www.frontiersin.org/articles/10.3389/fpsyt.2021.671348/full#supplementary-material
References
1. Zhao YJ, Du MY, Huang XQ, Lui S, Chen ZQ, Liu J, et al. Brain grey matter abnormalities in medication-free patients with major depressive disorder: a meta-analysis. Psychol Med. (2014) 44:2927–37. doi: 10.1017/S0033291714000518
2. Webb CA, Weber M, Mundy EA, Killgore WD. Reduced gray matter volume in the anterior cingulate, orbitofrontal cortex and thalamus as a function of mild depressive symptoms: a voxel-based morphometric analysis. Psychol Med. (2014) 44:2833–43. doi: 10.1017/S0033291714000348
3. Ashburner J, Friston KJ. Voxel-based morphometry—the methods. Neuroimage. (2000) 11:805–21. doi: 10.1006/nimg.2000.0582
4. Bora E, Fornito A, Yücel M, Pantelis C. Voxelwise meta-analysis of gray matter abnormalities in bipolar disorder. Biol Psychiatry. (2010) 67:1097–105. doi: 10.1016/j.biopsych.2010.01.020
5. Ellison-Wright I, Bullmore E. Anatomy of bipolar disorder and schizophrenia: a meta-analysis. Schizophr Res. (2010) 117:1–12. doi: 10.1016/j.schres.2009.12.022
6. Fornito A, Yücel M, Patti J, Wood SJ, Pantelis C. Mapping grey matter reductions in schizophrenia: an anatomical likelihood estimation analysis of voxel-based morphometry studies. Schizophr Res. (2009) 108:104–13. doi: 10.1016/j.schres.2008.12.011
7. Liu PH, Li GZ, Zhang AX, Sun N, Kang LJ, Yang CX, et al. The prognosis and changes of regional brain gray matter volume in MDD with gastrointestinal symptoms. Neuropsychiatr Dis Treat. (2019) 15:1181–91. doi: 10.2147/NDT.S197351
8. Nickson T, Chan SW, Papmeyer M, Romaniuk L, Macdonald A, Stewart T, et al. Prospective longitudinal voxel-based morphometry study of major depressive disorder in young individuals at high familial risk. Psychol Med. (2016) 46:2351–61. doi: 10.1017/S0033291716000519
9. Niida R, Yamagata B, Matsuda H, Niida A, Uechi A, Kito S, et al. Regional brain volume reductions in major depressive disorder and bipolar disorder: an analysis by voxel-based morphometry. Int J Geriatr Psychiatry. (2019) 34:186–92. doi: 10.1002/gps.5009
10. Radua J, Mataix-Cols D. Voxel-wise meta-analysis of grey matter changes in obsessive-compulsive disorder. Br J Psychiatry. (2009) 195:393–402. doi: 10.1192/bjp.bp.108.055046
11. Radua J, van den Heuvel OA, Surguladze S, Mataix-Cols D. Meta-analytical comparison of voxel-based morphometry studies in obsessive-compulsive disorder vs other anxiety disorders. Arch Gen Psychiatry. (2010) 67:701–11. doi: 10.1001/archgenpsychiatry.2010.70
12. Kong L, Wu F, Tang Y, Ren L, Kong D, Liu Y, et al. Frontal-subcortical volumetric deficits in single episode, medication-naive depressed patients and the effects of 8 weeks fluoxetine treatment: a VBM-DARTEL study. PLoS ONE. (2014) 9:e79055. doi: 10.1371/journal.pone.0079055
13. Lu Y, Liang H, Han D, Mo Y, Li Z, Cheng Y, et al. The volumetric and shape changes of the putamen and thalamus in first episode, untreated major depressive disorder. Neuroimage Clin. (2016) 11:658–66. doi: 10.1016/j.nicl.2016.04.008
14. Serra-Blasco M, Portella MJ, Gomez-Anson B, de Diego-Adelino J, Vives-Gilabert Y, Puigdemont D, et al. Effects of illness duration and treatment resistance on grey matter abnormalities in major depression. Br J Psychiatry. (2013) 202:434–40. doi: 10.1192/bjp.bp.112.116228
15. Stratmann M, Konrad C, Kugel H, Krug A, Schoning S, Ohrmann P, et al. Insular and hippocampal gray matter volume reductions in patients with major depressive disorder. PLoS ONE. (2014) 9:e102692. doi: 10.1371/journal.pone.0102692
16. Zhang X, Yao S, Zhu X, Wang X, Zhu X, Zhong M. Gray matter volume abnormalities in individuals with cognitive vulnerability to depression: a voxel-based morphometry study. J Affect Disord. (2012) 136:443–52. doi: 10.1016/j.jad.2011.11.005
17. Cheng YQ, Xu J, Chai P, Li HJ, Luo CR, Yang T, et al. Brain volume alteration and the correlations with the clinical characteristics in drug-naive first-episode MDD patients: a voxel-based morphometry study. Neurosci Lett. (2010) 480:30–4. doi: 10.1016/j.neulet.2010.05.075
18. Lai CH, Hsu YY, Wu YT. First episode drug-naive major depressive disorder with panic disorder: gray matter deficits in limbic and default network structures. Eur Neuropsychopharmacol. (2010) 20:676–82. doi: 10.1016/j.euroneuro.2010.06.002
19. Lai CH, Wu YT. Frontal-insula gray matter deficits in first-episode medication-naive patients with major depressive disorder. J Affect Disord. (2014) 160:74–9. doi: 10.1016/j.jad.2013.12.036
20. Ma C, Ding J, Li J, Guo W, Long Z, Liu F, et al. Resting-state functional connectivity bias of middle temporal gyrus and caudate with altered gray matter volume in major depression. PLoS ONE. (2012) 7:e45263. doi: 10.1371/journal.pone.0045263
21. Peng J, Liu J, Nie B, Li Y, Shan B, Wang G, et al. Cerebral and cerebellar gray matter reduction in first-episode patients with major depressive disorder: a voxel-based morphometry study. Eur J Radiol. (2011) 80:395–9. doi: 10.1016/j.ejrad.2010.04.006
22. Tang Y, Wang F, Xie G, Liu J, Li L, Su L, et al. Reduced ventral anterior cingulate and amygdala volumes in medication-naive females with major depressive disorder: a voxel-based morphometric magnetic resonance imaging study. Psychiatry Res. (2007) 156:83–6. doi: 10.1016/j.pscychresns.2007.03.005
23. Wang L, Dai W, Su Y, Wang G, Tan Y, Jin Z, et al. Amplitude of low-frequency oscillations in first-episode, treatment-naive patients with major depressive disorder: a resting-state functional MRI study. PLoS ONE. (2012) 7:e48658. doi: 10.1371/journal.pone.0048658
24. Zou K, Deng W, Li T, Zhang B, Jiang L, Huang C, et al. Changes of brain morphometry in first-episode, drug-naive, non-late-life adult patients with major depression: an optimized voxel-based morphometry study. Biol Psychiatry. (2010) 67:186–8. doi: 10.1016/j.biopsych.2009.09.014
25. Beesdo K, Pine DS, Lieb R, Wittchen HU. Incidence and risk patterns of anxiety and depressive disorders and categorization of generalized anxiety disorder. Arch Gen Psychiatry. (2010) 67:47–57. doi: 10.1001/archgenpsychiatry.2009.177
26. Bora E, Fornito A, Pantelis C, Yucel M. Gray matter abnormalities in Major Depressive Disorder: a meta-analysis of voxel based morphometry studies. J Affect Disord. (2012) 138:9–18. doi: 10.1016/j.jad.2011.03.049
27. Lai CH. Gray matter volume in major depressive disorder: a meta-analysis of voxel-based morphometry studies. Psychiatry Res. (2013) 211:37–46. doi: 10.1016/j.pscychresns.2012.06.006
28. Peng W, Chen Z, Yin L, Jia Z, Gong Q. Essential brain structural alterations in major depressive disorder: a voxel-wise meta-analysis on first episode, medication-naive patients. J Affect Disord. (2016) 199:114–23. doi: 10.1016/j.jad.2016.04.001
29. Zhang H, Li L, Wu M, Chen Z, Hu X, Chen Y, et al. Brain gray matter alterations in first episodes of depression: a meta-analysis of whole-brain studies. Neurosci Biobehav Rev. (2016) 60:43–50. doi: 10.1016/j.neubiorev.2015.10.011
30. Radua J, Mataix-Cols D, Phillips ML, El-Hage W, Kronhaus DM, Cardoner N, et al. A new meta-analytic method for neuroimaging studies that combines reported peak coordinates and statistical parametric maps. Eur Psychiatry. (2012) 27:605–11. doi: 10.1016/j.eurpsy.2011.04.001
31. Moher D, Liberati A, Tetzlaff J, Altman DG. Preferred reporting items for systematic reviews and meta-analyses: the PRISMA statement. PLoS Med. (2009) 6:e1000097. doi: 10.1371/journal.pmed.1000097
32. Wang T, Liu J, Zhang J, Zhan W, Li L, Wu M, et al. Altered resting-state functional activity in posttraumatic stress disorder: a quantitative meta-analysis. Sci Rep. (2016) 6:27131. doi: 10.1038/srep27131
33. Albajes-Eizagirre A, Solanes A, Fullana MA, Ioannidis JPA, Fusar-Poli P, Torrent C, et al. Meta-analysis of voxel-based neuroimaging studies using seed-based d mapping with Permutation of Subject Images (SDM-PSI). J Vis Exp. (2019) e59841. doi: 10.3791/59841
34. Albajes-Eizagirre A, Solanes A, Vieta E, Radua J. Voxel-based meta-analysis via permutation of subject images (PSI): theory and implementation for SDM. Neuroimage. (2019) 186:174–84. doi: 10.1016/j.neuroimage.2018.10.077
35. Cao Y, Wu B, Chen T, Diao W, Jia Z. Altered intrinsic brain activity in patients with hepatic encephalopathy. J Neurosci Res. (2021) 99:1337–53. doi: 10.1002/jnr.24788
36. Chen Z, Peng W, Sun H, Kuang W, Li W, Jia Z, et al. High-field magnetic resonance imaging of structural alterations in first-episode, drug-naive patients with major depressive disorder. Transl Psychiatry. (2016) 6:e942. doi: 10.1038/tp.2016.209
37. Guo W, Liu F, Yu M, Zhang J, Zhang Z, Liu J, et al. Functional and anatomical brain deficits in drug-naive major depressive disorder. Prog Neuropsychopharmacol Biol Psychiatry. (2014) 54:1–6. doi: 10.1016/j.pnpbp.2014.05.008
38. Igata N, Kakeda S, Watanabe K, Ide S, Kishi T, Abe O, et al. Voxel-based morphometric brain comparison between healthy subjects and major depressive disorder patients in Japanese with the s/s genotype of 5-HTTLPR. Sci Rep. (2017) 7:3931. doi: 10.1038/s41598-017-04347-8
39. Yang S, Cheng Y, Mo Y, Bai Y, Shen Z, Liu F, et al. Childhood maltreatment is associated with gray matter volume abnormalities in patients with first-episode depression. Psychiatry Res Neuroimaging. (2017) 268:27–34. doi: 10.1016/j.pscychresns.2017.07.005
40. Zhang Y, Yang Y, Zhu L, Zhu Q, Jia Y, Zhang L, et al. Volumetric deficit within the fronto-limbic-striatal circuit in first-episode drug naïve patients with major depression disorder. Front Psychiatry. (2020) 11:600583. doi: 10.3389/fpsyt.2020.600583
41. Cui X, Abduljalil A, Manor BD, Peng CK, Novak V. Multi-scale glycemic variability: a link to gray matter atrophy and cognitive decline in type 2 diabetes. PLoS ONE. (2014) 9:e86284. doi: 10.1371/journal.pone.0086284
42. Morgane PJ, Galler JR, Mokler DJ. A review of systems and networks of the limbic forebrain/limbic midbrain. Prog Neurobiol. (2005) 75:143–60. doi: 10.1016/j.pneurobio.2005.01.001
43. Drevets WC. Functional anatomical abnormalities in limbic and prefrontal cortical structures in major depression. Prog Brain Res. (2000) 126:413–31. doi: 10.1016/S0079-6123(00)26027-5
44. Augustine JR. Circuitry and functional aspects of the insular lobe in primates including humans. Brain Res Brain Res Rev. (1996) 22:229–44. doi: 10.1016/S0165-0173(96)00011-2
45. Fitzgerald PB, Laird AR, Maller J, Daskalakis ZJ. A meta-analytic study of changes in brain activation in depression. Hum Brain Mapp. (2008) 29:683–95. doi: 10.1002/hbm.20426
46. Kaiser RH, Andrews-Hanna JR, Wager TD, Pizzagalli DA. Large-scale network dysfunction in major depressive disorder: a meta-analysis of resting-state functional connectivity. JAMA Psychiatry. (2015) 72:603–11. doi: 10.1001/jamapsychiatry.2015.0071
47. Nagai M, Kishi K, Kato S. Insular cortex and neuropsychiatric disorders: a review of recent literature. Eur Psychiatry. (2007) 22:387–94. doi: 10.1016/j.eurpsy.2007.02.006
48. Sliz D, Hayley S. Major depressive disorder and alterations in insular cortical activity: a review of current functional magnetic imaging research. Front Hum Neurosci. (2012) 6:323. doi: 10.3389/fnhum.2012.00323
49. Yang X, Peng Z, Ma X, Meng Y, Li M, Zhang J, et al. Sex differences in the clinical characteristics and brain gray matter volume alterations in unmedicated patients with major depressive disorder. Sci Rep. (2017) 7:2515. doi: 10.1038/s41598-017-02828-4
50. Zhao Y, Chen L, Zhang W, Xiao Y, Shah C, Zhu H, et al. Gray matter abnormalities in non-comorbid medication-naive patients with major depressive disorder or social anxiety disorder. EBioMedicine. (2017) 21:228–35. doi: 10.1016/j.ebiom.2017.06.013
51. Igata R, Katsuki A, Kakeda S, Watanabe K, Igata N, Hori H, et al. PCLO rs2522833-mediated gray matter volume reduction in patients with drug-naive, first-episode major depressive disorder. Transl Psychiatry. (2017) 7:e1140. doi: 10.1038/tp.2017.100
52. MacQueen G, Frodl T. The hippocampus in major depression: evidence for the convergence of the bench and bedside in psychiatric research? Mol Psychiatry. (2011) 16:252–64. doi: 10.1038/mp.2010.80
53. Diana RA, Yonelinas AP, Ranganath C. Imaging recollection and familiarity in the medial temporal lobe: a three-component model. Trends Cogn Sci. (2007) 11:379–86. doi: 10.1016/j.tics.2007.08.001
54. Ma X, Liu J, Liu T, Ma L, Wang W, Shi S, et al. Altered resting-state functional activity in medication-naive patients with first-episode major depression disorder vs. healthy control: a quantitative meta-analysis. Front Behav Neurosci. (2019) 13:89. doi: 10.3389/fnbeh.2019.00089
55. Milne AM, MacQueen GM, Hall GB. Abnormal hippocampal activation in patients with extensive history of major depression: an fMRI study. J Psychiatry Neurosci. (2012) 37:28–36. doi: 10.1503/jpn.110004
56. Cole J, Costafreda SG, McGuffin P, Fu CH. Hippocampal atrophy in first episode depression: a meta-analysis of magnetic resonance imaging studies. J Affect Disord. (2011) 134:483–7. doi: 10.1016/j.jad.2011.05.057
57. Frodl T, Meisenzahl EM, Zetzsche T, Born C, Groll C, Jäger M, et al. Hippocampal changes in patients with a first episode of major depression. Am J Psychiatry. (2002) 159:1112–8. doi: 10.1176/appi.ajp.159.7.1112
58. Kronmüller KT, Schröder J, Köhler S, Götz B, Victor D, Unger J, et al. Hippocampal volume in first episode and recurrent depression. Psychiatry Res. (2009) 174:62–6. doi: 10.1016/j.pscychresns.2008.08.001
59. McKinnon MC, Yucel K, Nazarov A, MacQueen GM. A meta-analysis examining clinical predictors of hippocampal volume in patients with major depressive disorder. J Psychiatry Neurosci. (2009) 34:41–54.
60. Zhang FF, Peng W, Sweeney JA, Jia ZY, Gong QY. Brain structure alterations in depression: psychoradiological evidence. CNS Neurosci Ther. (2018) 24:994–1003. doi: 10.1111/cns.12835
61. Lee HY, Tae WS, Yoon HK, Lee BT, Paik JW, Son KR, et al. Demonstration of decreased gray matter concentration in the midbrain encompassing the dorsal raphe nucleus and the limbic subcortical regions in major depressive disorder: an optimized voxel-based morphometry study. J Affect Disord. (2011) 133:128–36. doi: 10.1016/j.jad.2011.04.006
62. Wagner G, Koch K, Schachtzabel C, Schultz CC, Sauer H, Schlösser RG. Structural brain alterations in patients with major depressive disorder and high risk for suicide: evidence for a distinct neurobiological entity? Neuroimage. (2011) 54:1607–14. doi: 10.1016/j.neuroimage.2010.08.082
63. Frodl T, Koutsouleris N, Bottlender R, Born C, Jager M, Morgenthaler M, et al. Reduced gray matter brain volumes are associated with variants of the serotonin transporter gene in major depression. Mol Psychiatry. (2008) 13:1093–101. doi: 10.1038/mp.2008.62
64. Gerritsen L, Comijs HC, van der Graaf Y, Knoops AJ, Penninx BW, Geerlings MI. Depression, hypothalamic pituitary adrenal axis, and hippocampal and entorhinal cortex volumes–the SMART Medea study. Biol Psychiatry. (2011) 70:373–80. doi: 10.1016/j.biopsych.2011.01.029
65. Sheline YI. Depression and the hippocampus: cause or effect? Biol Psychiatry. (2011) 70:308–9. doi: 10.1016/j.biopsych.2011.06.006
66. Postuma RB, Dagher A. Basal ganglia functional connectivity based on a meta-analysis of 126 positron emission tomography and functional magnetic resonance imaging publications. Cereb Cortex. (2006) 16:1508–21. doi: 10.1093/cercor/bhj088
67. Kim MJ, Hamilton JP, Gotlib IH. Reduced caudate gray matter volume in women with major depressive disorder. Psychiatry Res. (2008) 164:114–22. doi: 10.1016/j.pscychresns.2007.12.020
68. Koolschijn PCMP, van Haren NE, Lensvelt-Mulders GJ, Hulshoff Pol HE, Kahn RS. Brain volume abnormalities in major depressive disorder: a meta-analysis of magnetic resonance imaging studies. Hum Brain Mapp. (2009) 30:3719–35. doi: 10.1002/hbm.20801
69. Pizzagalli DA, Holmes AJ, Dillon DG, Goetz EL, Birk JL, Bogdan R, et al. Reduced caudate and nucleus accumbens response to rewards in unmedicated individuals with major depressive disorder. Am J Psychiatry. (2009) 166:702–10. doi: 10.1176/appi.ajp.2008.08081201
70. Forbes EE, Hariri AR, Martin SL, Silk JS, Moyles DL, Fisher PM, et al. Altered striatal activation predicting real-world positive affect in adolescent major depressive disorder. Am J Psychiatry. (2009) 166:64–73. doi: 10.1176/appi.ajp.2008.07081336
71. Satterthwaite TD, Kable JW, Vandekar L, Katchmar N, Bassett DS, Baldassano CF, et al. Common and dissociable dysfunction of the reward system in bipolar and unipolar depression. Neuropsychopharmacology. (2015) 40:2258–68. doi: 10.1038/npp.2015.75
72. Dluzen DE. Neuroprotective effects of estrogen upon the nigrostriatal dopaminergic system. J Neurocytol. (2000) 29:387–99. doi: 10.1023/A:1007117424491
73. Gusnard DA, Akbudak E, Shulman GL, Raichle ME. Medial prefrontal cortex and self-referential mental activity: relation to a default mode of brain function. Proc Natl Acad Sci USA. (2001) 98:4259–64. doi: 10.1073/pnas.071043098
74. Ramnani N, Owen AM. Anterior prefrontal cortex: insights into function from anatomy and neuroimaging. Nat Rev Neurosci. (2004) 5:184–94. doi: 10.1038/nrn1343
75. Simons JS, Spiers HJ. Prefrontal and medial temporal lobe interactions in long-term memory. Nat Rev Neurosci. (2003) 4:637–48. doi: 10.1038/nrn1178
76. Rajkowska G. Postmortem studies in mood disorders indicate altered numbers of neurons and glial cells. Biol Psychiatry. (2000) 48:766–77. doi: 10.1016/S0006-3223(00)00950-1
77. Rajkowska G, Miguel-Hidalgo JJ, Wei J, Dilley G, Pittman SD, Meltzer HY, et al. Morphometric evidence for neuronal and glial prefrontal cell pathology in major depression. Biol Psychiatry. (1999) 45:1085–98. doi: 10.1016/S0006-3223(99)00041-4
78. Fettes P, Schulze L, Downar J. Cortico-striatal-thalamic loop circuits of the orbitofrontal cortex: promising therapeutic targets in psychiatric illness. Front Syst Neurosci. (2017) 11:25. doi: 10.3389/fnsys.2017.00025
79. Fitzgerald PB, Oxley TJ, Laird AR, Kulkarni J, Egan GF, Daskalakis ZJ. An analysis of functional neuroimaging studies of dorsolateral prefrontal cortical activity in depression. Psychiatry Res. (2006) 148:33–45. doi: 10.1016/j.pscychresns.2006.04.006
80. Korgaonkar MS, Grieve SM, Etkin A, Koslow SH, Williams LM. Using standardized fMRI protocols to identify patterns of prefrontal circuit dysregulation that are common and specific to cognitive and emotional tasks in major depressive disorder: first wave results from the iSPOT-D study. Neuropsychopharmacology. (2013) 38:863–71. doi: 10.1038/npp.2012.252
81. Zhong X, Pu W, Yao S. Functional alterations of fronto-limbic circuit and default mode network systems in first-episode, drug-naïve patients with major depressive disorder: a meta-analysis of resting-state fMRI data. J Affect Disord. (2016) 206:280–6. doi: 10.1016/j.jad.2016.09.005
82. Hiser J, Koenigs M. The multifaceted role of the ventromedial prefrontal cortex in emotion, decision making, social cognition, and psychopathology. Biol Psychiatry. (2018) 83:638–47. doi: 10.1016/j.biopsych.2017.10.030
83. Drevets WC, Price JL, Furey ML. Brain structural and functional abnormalities in mood disorders: implications for neurocircuitry models of depression. Brain Struct Funct. (2008) 213:93–118. doi: 10.1007/s00429-008-0189-x
84. Ochsner KN, Knierim K, Ludlow DH, Hanelin J, Ramachandran T, Glover G, et al. Reflecting upon feelings: an fMRI study of neural systems supporting the attribution of emotion to self and other. J Cogn Neurosci. (2004) 16:1746–72. doi: 10.1162/0898929042947829
85. Grieve SM, Korgaonkar MS, Koslow SH, Gordon E, Williams LM. Widespread reductions in gray matter volume in depression. Neuroimage Clin. (2013) 3:332–9. doi: 10.1016/j.nicl.2013.08.016
86. Banich MT, Milham MP, Atchley RA, Cohen NJ, Webb A, Wszalek T, et al. Prefrontal regions play a predominant role in imposing an attentional 'set': evidence from fMRI. Brain Res Cogn Brain Res. (2000) 10:1–9. doi: 10.1016/S0926-6410(00)00015-X
87. MacDonald AWIII, Cohen JD, Stenger VA, Carter CS. Dissociating the role of the dorsolateral prefrontal and anterior cingulate cortex in cognitive control. Science. (2000) 288:1835–8. doi: 10.1126/science.288.5472.1835
88. Liu X, Kakeda S, Watanabe K, Yoshimura R, Abe O, Ide S, et al. Relationship between the cortical thickness and serum cortisol levels in drug-naïve, first-episode patients with major depressive disorder: a surface-based morphometric study. Depress Anxiety. (2015) 32:702–8. doi: 10.1002/da.22401
89. Yang XH, Wang Y, Huang J, Zhu CY, Liu XQ, Cheung EF, et al. Increased prefrontal and parietal cortical thickness does not correlate with anhedonia in patients with untreated first-episode major depressive disorders. Psychiatry Res. (2015) 234:144–51. doi: 10.1016/j.pscychresns.2015.09.014
90. Lai CH, Wu YT, Chen CY, Hou YC. Gray matter increases in fronto-parietal regions of depression patients with aripiprazole monotherapy: an exploratory study. Medicine. (2016) 95:e4654. doi: 10.1097/MD.0000000000004654
91. Cole MW, Repovš G, Anticevic A. The frontoparietal control system: a central role in mental health. Neuroscientist. (2014) 20:652–64. doi: 10.1177/1073858414525995
92. Schultz DH, Ito T, Solomyak LI, Chen RH, Mill RD, Anticevic A, et al. Global connectivity of the fronto-parietal cognitive control network is related to depression symptoms in the general population. Netw Neurosci. (2019) 3:107–23. doi: 10.1162/netn_a_00056
93. Brzezicka A. Integrative deficits in depression and in negative mood states as a result of fronto-parietal network dysfunctions. Acta Neurobiol Exp. (2013) 73:313–25.
94. Koyuncu A, Ertekin E, Binbay Z, Ozyildirim I, Yüksel C, Tükel R. The clinical impact of mood disorder comorbidity on social anxiety disorder. Compr Psychiatry. (2014) 55:363–9. doi: 10.1016/j.comppsych.2013.08.016
95. Ohayon MM, Schatzberg AF. Social phobia and depression: prevalence and comorbidity. J Psychosom Res. (2010) 68:235–43. doi: 10.1016/j.jpsychores.2009.07.018
96. Bui E, Fava M. From depression to anxiety, and back. Acta Psychiatr Scand. (2017) 136:341–2. doi: 10.1111/acps.12801
97. Fava M, Rush AJ, Alpert JE, Balasubramani GK, Wisniewski SR, Carmin CN, et al. Difference in treatment outcome in outpatients with anxious versus nonanxious depression: a STAR*D report. Am J Psychiatry. (2008) 165:342–51. doi: 10.1176/appi.ajp.2007.06111868
98. Peng W, Jia Z, Huang X, Lui S, Kuang W, Sweeney JA, et al. Brain structural abnormalities in emotional regulation and sensory processing regions associated with anxious depression. Prog Neuropsychopharmacol Biol Psychiatry. (2019) 94:109676. doi: 10.1016/j.pnpbp.2019.109676
99. Hirschfeld RM. Personality disorders and depression: comorbidity. Depress Anxiety. (1999) 10:142–6.
Keywords: major depressive disorder, first-episode, voxel-based morphometry, signed differential mapping, meta-analysis
Citation: Zheng R, Zhang Y, Yang Z, Han S and Cheng J (2021) Reduced Brain Gray Matter Volume in Patients With First-Episode Major Depressive Disorder: A Quantitative Meta-Analysis. Front. Psychiatry 12:671348. doi: 10.3389/fpsyt.2021.671348
Received: 23 February 2021; Accepted: 02 June 2021;
Published: 01 July 2021.
Edited by:
Anthony L. Vaccarino, Indoc Research, CanadaCopyright © 2021 Zheng, Zhang, Yang, Han and Cheng. This is an open-access article distributed under the terms of the Creative Commons Attribution License (CC BY). The use, distribution or reproduction in other forums is permitted, provided the original author(s) and the copyright owner(s) are credited and that the original publication in this journal is cited, in accordance with accepted academic practice. No use, distribution or reproduction is permitted which does not comply with these terms.
*Correspondence: Jingliang Cheng, fccchengjl@zzu.edu.cn