- 1School of Automation, Beijing University of Posts and Telecommunications, Beijing, China
- 2School of Economics and Management, Minjiang University, Fuzhou, China
- 3School of Electrical and Information Engineering, Beijing University of Civil Engineering and Architecture, Beijing, China
Introduction: Investigating the neuroimaging changes from mild cognitive impairment (MCI) to Alzheimer's disease (AD) is of great significance. However, the details about the distinct functional characteristics of AD and MCI remain unknown.
Methods: In this study, we investigated distinct profiles of functional connectivity density (FCD) differences between AD and MCI compared with the normal population, aiming to depict the progressive brain changes from MCI to AD. As a data-driven method, FCD measures the profiles of FC for the given voxel at different scales. Resting-state functional magnetic resonance imaging (fMRI) images were obtained from patients with AD and MCI and matched healthy controls (HCs). One-way ANCOVA was used to investigate (global, long-range, and local) FCD differences among the three groups followed by post-hoc analysis controlling age, sex, and head motion.
Results: The three groups exhibited significant global FCD differences in the superior frontal gyrus. The post-hoc results further showed that patients with AD had a significant increase in global FCD values than those with MCI and HCs. Patients with MCI exhibited an increased trend compared with HCs. We further identified brain regions contributing to the observed global FCD differences by conducting seed-based FC analysis. We also identified that the observed global FCD differences were the additive effects of altered FC between the superior frontal gyrus and the posterior default model network.
Discussion: These results depicted the global information communication capability impairment in AD and MCI providing a new insight into the progressive brain changes from MCI to AD.
Introduction
As an irreversible neurodegenerative brain disorder, Alzheimer's disease (AD) leads to severe mental dysfunction and ultimately death in patients (1). AD is characterized by two main pathological changes, namely, amyloid-beta plaques and neurofibrillary tangles that finally lead to neuronal degeneration and loss (2). To date, there is no effective approach to early diagnosis and treatment that can stop or hinder this progression in the world (3). The identification of predictors at the beginning of AD, termed mild cognitive impairment (MCI) with an annual progression rate, from MCI to AD reaches 10 to 15% (4), which is of great significance in the clinic (5). Discovering potential biomarkers for identifying patients with MCI who are most likely to develop AD supports early diagnosis and medical intervention.
Advances in modern neuroimaging technologies, especially magnetic resonance imaging (MRI) technology, motivate researchers to identify distinct neuroimaging phenotypes between AD and MCI. Among these technologies, resting-state functional MRI turns out to be a powerful tool to investigate the progressive brain changes from MCI to AD (6). Studies recognized that both tau and amyloid-beta accumulation can affect neuronal activity and ultimately impair neuronal network (7, 8). Using the functional MRI, researchers consistently recognized that AD is accompanied by disruption of large-scale brain systems supporting a variety of cognitive abilities that were observed to decline with the disease progression (9). In other words, AD is a neurodegeneration featured with altered large-scale brain networks (10). These are two main methods to investigate intrinsic brain networks using resting-state functional MRI data, namely, independent component analysis (ICA) and seed-based functional connectivity (FC) (11). However, they have many problems. Specifically, seed-based approach relies on a predefined region of interest that is difficult to determine if the underlying pathology is unclear (12). As for ICA, there is no effective method to determine the appropriate number of independent components (13). In response to these problems, a novel method named FC density (FCD) mapping is proposed (14). As a data-driven method, FCD measures the number of functional connections between the given voxel and other voxels in the brain at different scales. Especially, the global FCD value is found to reflect the glucose metabolism (15) and the global information communication capability of the given voxel (16). Brain regions with high global FCD values are usually considered to be hubs of functional systems. At the same time, cascading network failure hypothesis postulates that tau deposition and amyloid lead to larger-scale brain network abnormalities, especially for functional hubs (9). In this regard, FCD is well suited to investigate brain disorders, including AD.
In this study, we aimed to investigate FCD differences among patients with AD and MCI and matched healthy controls (HCs) to depict the progressive brain changes from MCI to AD. Resting-state functional MRI images were obtained from patients with AD (n = 33) and MCI (n = 88) and HCs (n = 30). First, we calculated FCD (e.g., global FCD, long-range FCD, and local FCD) values for each subject. Then, we investigated FCD differences among the three groups. Previous studies found that MCI could be further divided into early MCI (EMCI) and late MCI (LMCI) on the basis of the severity of impaired delayed recall of logical memory (17). We also investigated whether EMCI and LMCI exhibited differences with regard to FCD. Finally, to further determine brain regions contributing to the observed FCD differences, seed-based FC maps were constructed where brain regions showing FCD aberrance were treated as seeds and compared among the three groups.
Materials and methods
Subjects
All subjects used in this study come from the Alzheimer's Disease Neuroimaging Initiative (ADNI) open database (http://adni.loni.usc.edu/). This project recruited more than 1,500 adults aged between 55 and 90 years since 2013, comprising patients with AD, those with MCI, and healthy population. We downloaded the dataset from ADNI phase 2. All subjects fulfilled the following inclusion criteria: (1) with no comorbidity with depression; (2) having no other kinds of dementia; (3) having clinical scales; and (4) having no obvious artifacts. In this dataset, cognitive function and degree of dementia were evaluated using the Mini-Mental State Examination (MMSE) and the Clinical Dementia Rating Scale-Sum of Boxes (CDR_SB) (18, 19). More details are included in Table 1.
Ethical review and approval were not required for the current study in accordance with the local legislation and institutional requirements. The datasets on which this article relies on were reviewed and approved by the Cleveland Clinic Institutional Review Board ADNI Individual Site Institutional Review Board. Written informed consent for participation was not required for this study in accordance with national legislation and institutional requirements.
Data acquisition
Resting-state functional MRI images were acquired using a 3.0 T Philips Healthcare MRI scanner. All subjects were asked to keep their eyes closed during the scan. Images were obtained by echo-planar imaging (EPI) sequence. The scanning parameters were as follows: repetition time = 3 s, echo time = 30 ms, flip angle = 80°, acquisition matrix = 64 × 64, number of volumes = 140, slice thickness = 3.3 mm, and voxel size = 3 × 3 × 3 mm.
Data preprocessing
The preprocessing of functional MRI images was performed using the Data Processing Assistant for Resting-State fMRI package (http://www.restfmri.net). The following steps were included: First, the first 10 scans with time point correction and realignment were removed. Then, images were normalized to the standard EPI template and resampled to 3 mm3. In this step, to control the head motion, subjects would be excluded if the translational/rotational displacement exceeded 3.0 mm/3.0°. Next, images were smoothed with 6 mm3 full-width at half maximum Gaussian kernel, detrended, and filtered with bandpass (0.01–0.1 Hz). Nuisance covariates including white matter signal, cerebrospinal fluid signal, and Friston 24 motion parameters (20) were regressed out. Especially, the global signal was not included as another covariate, as previous studies consistently recognized that the global signal bore physiological signification and was altered in mental disorders (21–23). Finally, to further remove the effect of head motion, scrubbing with cubic spline interpolation was used. The “bad” points were identified with a threshold of frame displacement larger than 0.5 mm as well as one-forward and two-back neighbors (24). The mean frame-wise displacement (FD) for each subject was also calculated (25, 26).
Calculation of FCD
For each subject, we calculated (global, long-range, and local) FCD maps according to the previous study (14). In this study, we briefly described the calculation process, and more details could be referred to in the study of Tomasi and Volkow (14). For each voxel, the global FCD value was defined as the number of significant functional connections between it with other voxels in the gray matter. The local FCD value of one voxel was defined as the size of a continuous cluster of spatially connected voxels (voxel number) that were significantly correlated with it (27). The long-range FCD value of voxel i was obtained using the equation: long-range FCDi = gFCDi – local FCDi (27). Some studies set the threshold with a predefined correlation r (e.g., 0.6). We did not adopt this strategy, as there was no clue to choose the optimal threshold (28). In this study, the significance of one connection was determined according to its p-value (p < 0.05, Bonferroni correction for all voxels in the gray matter) (29). Finally, all FCD maps were transformed to Z-scores by subtracting the mean and dividing the value by the standard deviation across gray matter voxels (30).
Statistical analysis
We obtained (global, local, or long-range) FCD map differences among the three groups using one-way ANOVA equipped in SPM12 (http://www.fil.ion.ucl.ac.uk/spm). To exclude the effects of factors including age, sex, and mean FD, they were included as covariates in this step. The results were controlled with multiple comparisons with Gaussian random field (GRF) where voxel-wise threshold was a p-value of < 0.005 and cluster-level threshold was a p-value of < 0.05. To determine the details about between-group differences, we extracted the mean (global, local, or long-range) FCD values of each peak coordinate with a spherical radius of 6 mm that demonstrated significant differences among the three groups and compared them between each pair of groups with two-sample t test. As previous studies identified that MCI could be further divided into EMCI and LMCI (17), we also investigated whether FCD values (extracted before) exhibited significant differences between EMCI and LMCI with a two-sample t test.
Identification of brain regions contributing to the FCD aberrance
To further identify which brain regions contributed to the observed FCD differences among the three groups, we calculated seed-based FC maps where peak coordinates of identified clusters showing FCD differences with a spherical radius of 6 mm were treated as seeds. The obtained FC values were transformed into Fisher Z-scores and then compared among the three groups followed by post-hoc analysis. This procedure was not designed to find significant FC differences connected to seeds but to identify brain regions contributing to the observed FCD differences. Thus, we reported uncorrected results with a loose threshold (voxel-wise p < 0.05, cluster size > 100).
Association with symptom severity
To associate altered FCD values with symptom severity, Pearson's correlation coefficients between altered FCD values (extracted before) and symptom scores (CDR_SB/MMSE) were calculated.
Head motion analysis
As head motion had a strong impact on FC, we adopted a number of strategies to exclude the effects of head motion on our results. First, subjects would be excluded if the translational and rotational displacement exceeded 3.0 mm or 3.0°. Second, mean FD was calculated for each subject and compared among the three groups. Third, we also calculated Pearson's correlation coefficients between the FCD values of brain regions exhibiting aberrance among the three groups and mean FD.
Results
Clinical demographics
The demographical and clinical information is included in Table 1. As we could see, the three groups demonstrated no significant differences in age and sex.
ANOVA and post-hoc results of FCD
The three groups exhibited significant global FCD differences in the superior frontal gyrus (voxel-wise p < 0.005, cluster p < 0.05, GRF corrected; Figure 1, Table 2). The post-hoc results demonstrated that AD exhibited a significant increase of global FCD in the superior frontal gyrus than MCI (t = 2.948, p = 0.002, Cohen's d = 0.602) and HCs (t = 3.276, p = 0.004, Cohen's d =0.827). Patients with MCI exhibited an increased trend compared with HCs (t = 1.002, p = 0.318, Cohen's d = 0.212). There was no significant difference between patients with EMCI and LMCI (all p values > 0.05).
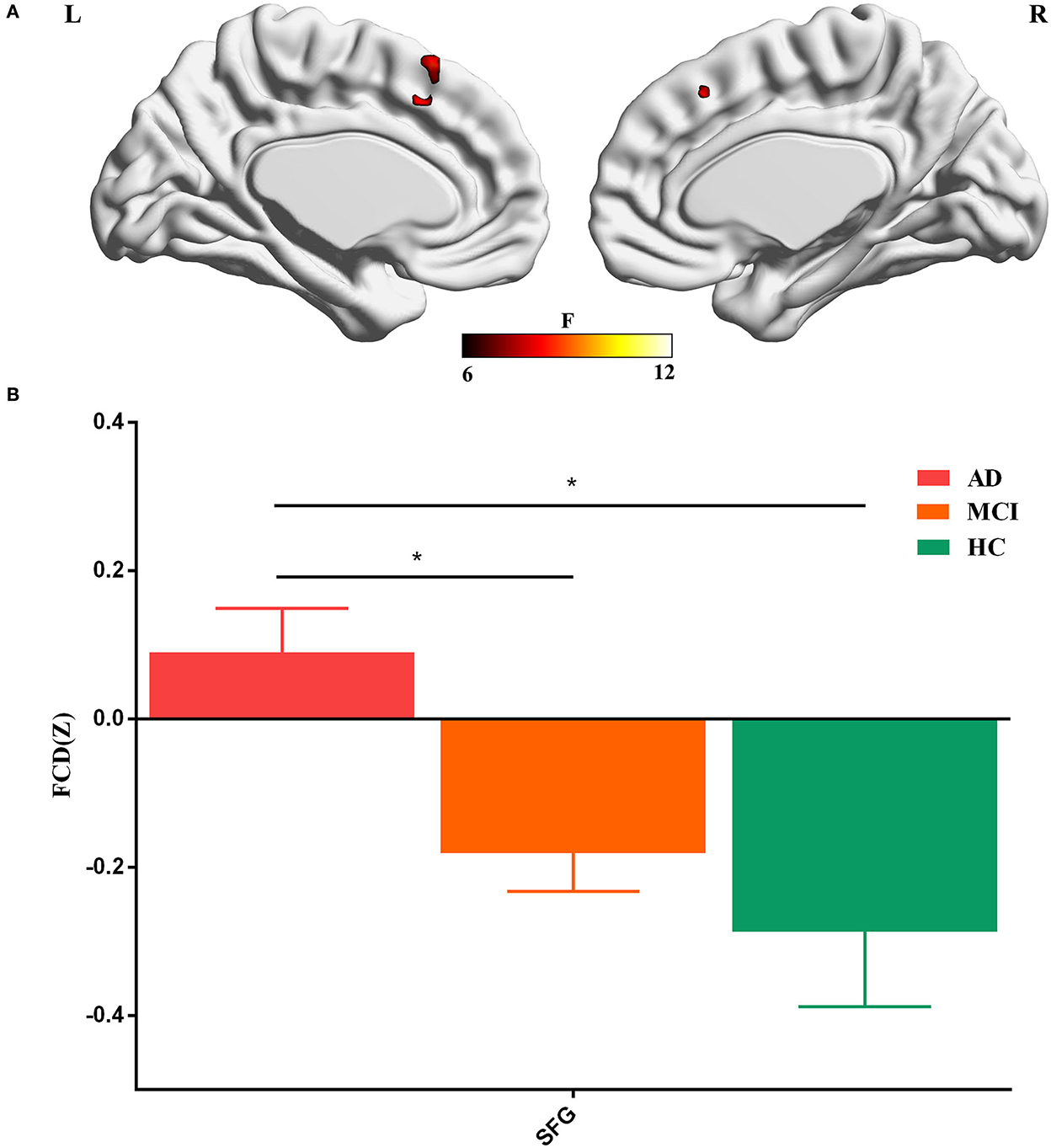
Figure 1. (A) Global FCD aberrance among the three groups. (B) Post-hoc results. SFG, superior frontal gyrus. The “*” means that the difference is significant (p < 0.05).
Brain regions contributing to the FCD aberrance
To further identify which brain regions contributed to the observed FCD differences, we constructed seed-based FC maps where the superior frontal gyrus was treated as the seed. The results demonstrated that distributed brain regions showed altered FC with the superior frontal gyrus, including the middle cingulum, the precuneus, the thalamus, the parahippocampus, the superior temporal gyrus, and the occipital lobe. The post-hoc results further showed that AD exhibited increased FC of these regions connected to the superior frontal gyrus than MCI and HCs (Figure 2). In addition, MCI exhibited increased FC linking the right cerebellum posterior lobe and the superior frontal gyrus than HCs. The post-hoc results are shown in Figure 3, Table 3.
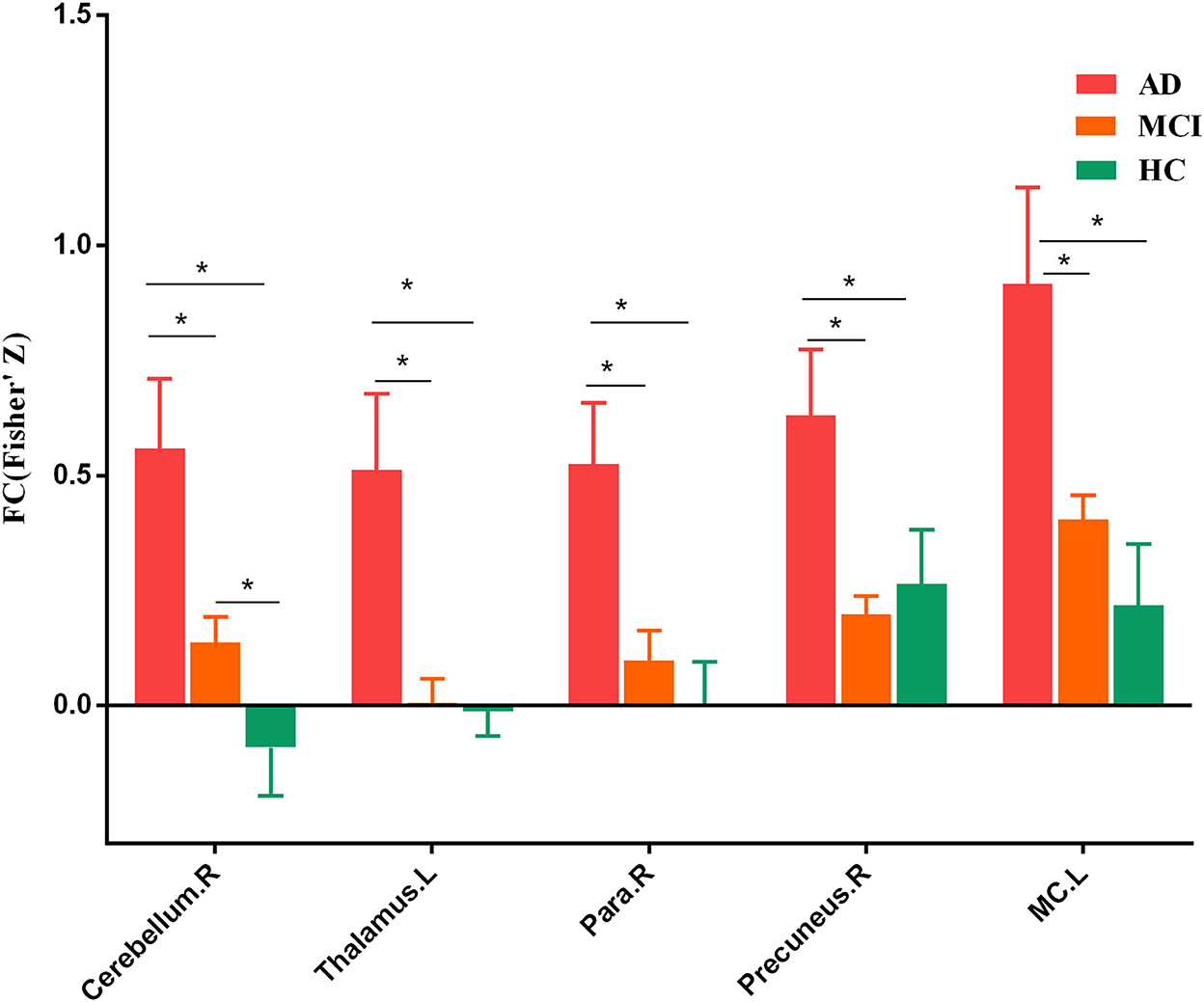
Figure 3. Post-hoc results of seed-based functional connectivity. Para, parahippocampus. MC, middle cingulum. The “*” means that the difference is significant (p < 0.05).
Association with symptom severity
There was no significant correlation between global FCD values of the superior frontal gyrus and CDR_SB/MMSE scores (all p-values > 0.05).
Head motion analysis results
We adopted a series of strategies to exclude the effects of head motion on our results. Four subjects (one AD, one MCI, and two HCs) were excluded if their translational and rotational displacement exceeded 3.0 mm or 3.0°. The three groups did not exhibit a significant difference in mean FD (p = 0.164). In addition, there was no significant correlation between mean FD and FCD values (all p-values > 0.05). These results indicated that our results were not obtained from head motion.
Discussion
In this study, we investigated distinct profiles of FCD aberrance between AD and MCI, compared with matched HCs aiming to depict the progressive brain changes from MCI to AD. First, we found that the three groups exhibited a significant global FCD difference in the superior frontal gyrus. The post-hoc results further showed us that patients with AD had a significant increase in global FCD than those with MCI and HCs. Patients with MCI exhibited an increased trend compared with HCs. There was no significant difference between subtypes of MCI (EMCI and LMCI) in terms of global FCD values. We further identified brain regions contributing to observed global FCD aberrance. As a result, we identified that the observed global FCD aberrance was the additive effects of FC between the superior frontal gyrus and brain regions mainly located in the posterior DMN including the middle cingulum, the precuneus, the thalamus, the parahippocampus, the superior temporal gyrus, and the occipital lobe.
Implicated in a variety of cognitive processes and motor functions, the superior frontal gyrus was one of the brain regions showing the greatest age-related volume reduction and surface area reductions, which further predicts the risk of cognitive decline and dementia (31–33). Connected with distributed brain regions with white matter, the superior frontal gyrus was implicated in spatial working memory (34, 35). Lesion studies recognized that subjects with the superior frontal gyrus lesion exhibited impaired working memory performance, especially for spatial working memory (36). Decreased volume of the superior frontal gyrus was associated with disinhibited behavior in patients with AD (37). The intrinsic brain activity of the superior frontal gyrus was also found in AD (38). Coinciding with cascading network failure hypothesis, we observed increased global FCD values in AD, possibly reflecting a compensatory phenomenon in response to local network failure resulted from tau accumulation (9). The compensation mechanism was often reported and accompanied by impairments during the progression from MCI to AD (39–42). Patients with MCI also exhibited an increased trend of global FCD aberrance compared with HCs. These results suggested that the compensatory increase of the global information communication capability in the superior frontal gyrus might be related to the conversion from MCI to AD. Another possible explanation of the increased global FCD was the heterogeneity in the pathology of AD. Although functional dysfunction of the superior frontal gyrus was widely reported, the findings were conflicting (43, 44). This might have resulted from the high interindividual heterogeneity among individuals with AD. The high individual variation in etiology and clinical manifestations was increasingly acknowledged and was thought to be one of the leading causes resulting in conflicting findings in neuroimaging studies in brain disorders (45–49). In addition, we did not observe significant differences between EMCI and LMCI. A number of previous studies found that these two subtypes exhibited structural and functional aberrance differences (50–52). The disagreement between our results and previous studies might also be attributed to the heterogeneity. More future studies were needed to investigate the heterogeneity in AD and MCI.
Another finding was that the FC between the superior frontal gyrus and the brain regions mainly in the posterior default mode network (DMN) contributed to the observed global FCD aberrance. Although the pathology of AD was found to be related to a variety of brain networks, the dysfunction of DMN was the most consistent and frequent findings in AD (53). Compared with other brain networks, the DMN was preferentially studied for two main reasons. First, converging evidence recognized the linear association between the amyloid deposition and the dysfunction of DMN (54) whose core regions were associated with episodic memory retrieval (53, 55). Second, the dysfunction of DMN was related to the disease progression from MCI to AD (42, 56). For example, the hippocampus, playing a vital role in declarative memory, was identified as the anatomical signature of AD (57). The neuroanatomical aberrance of AD was thought to stem from the hippocampus and then spread to other brain regions (58). The hippocampal atrophy along with its atrophy rate was consistently found in AD and MCI and turned out to be potential biomarkers to forecast the conversion from MCI to AD (59, 60). Apart from brain regions in the DMN, the thalamus was found to play an important role in AD. The thalamus, receiving and integrating information from widespread brain regions, was of importance in cognitive processes, memory, and attention (61, 62). The microstructural change, volume atrophy, and functional decline led to a deficit in cognitive ability with age, as observed in the thalamus (63, 64). The volume atrophy and dysfunction of the thalamus were related to memory dysfunction in AD (65). Among these brain regions contributing to the observed global FCD aberrance, the cerebellum was noteworthy as its FC connected to the superior frontal gyrus differed between MCI and HCs. In addition to the motor function, recent evidence pointed out that the cerebellum was also implicated in working memory (66) and that working memory impairment was one of the dominating symptoms in AD and MCI (67). Consistent with these results, we found that FC between these brain regions and the superior frontal gyrus was altered in subjects with AD, helping to depict the progressive brain changes from MCI to AD.
This study has a number of limitations. First, all results were obtained in one single dataset, whether our conclusions held true in another independent dataset should be tested in the future. Second, longitudinal data declaring which subjects with MCI would develop AD were needed to further confirm our results. Third, another reason that we did not observe a significant difference between subtypes of MCI was the limited sample size. Future studies could confirm this by using datasets with large sample size.
Conclusion
In this study, we investigated distinct profiles of FCD aberrance between AD and MCI compared with the normal population, aiming to depict the progressive brain changes from MCI to AD. Patients with AD exhibited a significant increase in global FCD than HCs/MCI and patients with MCI demonstrated an increased trend of global FCD compared with HCs. Further results identified that brain regions mainly located in the posterior DMN contributed to the observed global FCD aberrance. These results depicted the global information communication capability impairment in AD and MCI and provided a new insight into the progressive brain changes from MCI to AD.
Data availability statement
The original contributions presented in the study are included in the article/supplementary material, further inquiries can be directed to the corresponding authors.
Ethics statement
Ethical review and approval was not required for the study on human participants in accordance with the local legislation and institutional requirements. Written informed consent for participation was not required for this study in accordance with the national legislation and the institutional requirements.
Author contributions
DM designed the research, analyzed the data, and wrote the manuscript. XW analyzed the data and wrote the manuscript. CC searched the literature and downloaded the data. LT provided suggestions and modified the language. XZ directed the research program and provided guidance and suggestions for the study. All authors read and approved the final manuscript.
Funding
All data used in this study come from the ADNI, and it was funded by the National Institutes of Health Grant (U01AG024904) and DOD ADNI (Department of Defense, W81XWH-12-2-0012).
Conflict of interest
The authors declare that the research was conducted in the absence of any commercial or financial relationships that could be construed as a potential conflict of interest.
Publisher's note
All claims expressed in this article are solely those of the authors and do not necessarily represent those of their affiliated organizations, or those of the publisher, the editors and the reviewers. Any product that may be evaluated in this article, or claim that may be made by its manufacturer, is not guaranteed or endorsed by the publisher.
References
1. Hett K, Ta V-T, Oguz I, Manjón JV, Coupé P, Initiative ADN. Multi-scale graph-based grading for Alzheimer's disease prediction. Med Image Anal. (2021) 67:101850. doi: 10.1016/j.media.2020.101850
2. Bero AW, Yan P, Roh JH, Cirrito JR, Stewart FR, Raichle ME, et al. Neuronal activity regulates the regional vulnerability to amyloid-β deposition. Nat Neurosci. (2011) 14:750–6. doi: 10.1038/nn.2801
3. Perry RJ, Watson P, Hodges JR. The nature and staging of attention dysfunction in early (minimal and mild) Alzheimer's disease: relationship to episodic and semantic memory impairment. Neuropsychologia. (2000) 38:252–71. doi: 10.1016/S0028-3932(99)00079-2
4. Petersen RC, Doody R, Kurz A, Mohs RC, Morris JC, Rabins PV, et al. Current concepts in mild cognitive impairment. Arch Neurol. (2001) 58:1985–92. doi: 10.1001/archneur.58.12.1985
5. Talwar P, Kushwaha S. Systematic review of different neuroimaging correlates in mild cognitive impairment and Alzheimer's disease. Clin Neuroradiol. (2021) 31:953–67. doi: 10.1007/s00062-021-01057-7
6. Fayed N, Modrego PJ, Salinas GR, Gazulla J. Magnetic resonance imaging based clinical research in Alzheimer's disease. J Alzheimers Dis. (2012) 31 Suppl 3:S5–18. doi: 10.3233/JAD-2011-111292
7. Busche MA, Wegmann S, Dujardin S. Tau impairs neural circuits, dominating amyloid-β effects, in Alzheimer models in vivo. Nat Neurosci. (2019) 22:57–64. doi: 10.1038/s41593-018-0289-8
8. Sintini I, Graff-Radford J, Jones DT, Botha H, Martin PR, Machulda MM, et al. Tau and amyloid relationships with resting-state functional connectivity in atypical Alzheimer's disease. Cereb Cortex. (2021) 31:1693–706. doi: 10.1093/cercor/bhaa319
9. Jones DT, Knopman DS, Gunter JL, Graff-Radford J, Vemuri P, Boeve BF, et al. Cascading network failure across the Alzheimer's disease spectrum. Brain. (2016) 139(Pt 2):547–62. doi: 10.1093/brain/awv338
10. Greicius MD, Kimmel DL. Neuroimaging insights into network-based neurodegeneration. Curr Opin Neurol. (2012) 25:727–34. doi: 10.1097/WCO.0b013e32835a26b3
11. Kaiser RH, Andrews-Hanna JR, Wager TD, Pizzagalli DA. Large-scale network dysfunction in major depressive disorder: a meta-analysis of resting-state functional connectivity. JAMA Psychiatry. (2015) 72:603–11. doi: 10.1001/jamapsychiatry.2015.0071
12. Nair A, Keown CL, Datko M, Shih P, Keehn B, Müller R-A. Impact of methodological variables on functional connectivity findings in autism spectrum disorders. Hum Brain Mapp. (2014) 35:4035–48. doi: 10.1002/hbm.22456
13. Hobson AR, Hillebrand A. Independent component analysis of the EEG: is this the way forward for understanding abnormalities of brain-gut signalling? Gut. (2006) 55:597–600. doi: 10.1136/gut.2005.081703
14. Tomasi D, Volkow ND. Functional connectivity density mapping. Proc Natl Acad Sci U S A. (2010) 107:9885–90. doi: 10.1073/pnas.1001414107
15. Thompson GJ, Riedl V, Grimmer T, Drzezga A, Herman P, Hyder F. The whole-brain “global” signal from resting state fMRI as a potential biomarker of quantitative state changes in glucose metabolism. Brain Connect. (2016) 6:435–47. doi: 10.1089/brain.2015.0394
16. Zuo X-N, Ehmke R, Mennes M, Imperati D, Castellanos FX, Sporns O, et al. Network centrality in the human functional connectome. Cereb Cortex. (2012) 22:1862–75. doi: 10.1093/cercor/bhr269
17. Aisen PS, Petersen RC, Donohue MC, Gamst A, Raman R, Thomas RG, et al. Clinical core of the Alzheimer's Disease Neuroimaging Initiative: progress and plans. Alzheimers Dement. (2010) 6:239–46. doi: 10.1016/j.jalz.2010.03.006
18. Folstein MF, Folstein SE, McHugh PR. “Mini-mental state”. A practical method for grading the cognitive state of patients for the clinician. J Psychiatr Res. (1975) 12:189–98. doi: 10.1016/0022-3956(75)90026-6
19. O'Bryant SE, Waring SC, Cullum CM, Hall J, Lacritz L, Massman PJ, et al. Staging dementia using clinical dementia rating scale sum of boxes scores: a Texas Alzheimer's research consortium study. Arch Neurol. (2008) 65:1091–5. doi: 10.1001/archneur.65.8.1091
20. Satterthwaite TD, Wolf DH, Loughead J, Ruparel K, Elliott MA, Hakonarson H, et al. Impact of in-scanner head motion on multiple measures of functional connectivity: relevance for studies of neurodevelopment in youth. Neuroimage. (2012) 60:623–32. doi: 10.1016/j.neuroimage.2011.12.063
21. Han S, Wang X, He Z, Sheng W, Zou Q, Li L, et al. Decreased static and increased dynamic global signal topography in major depressive disorder. Prog Neuropsychopharmacol Biol Psychiatry. (2019) 94:109665. doi: 10.1016/j.pnpbp.2019.109665
22. Yang GJ, Murray JD, Glasser M, Pearlson GD, Krystal JH, Schleifer C, et al. Altered global signal topography in schizophrenia. Cereb Cortex. (2017) 27:5156–69. doi: 10.1093/cercor/bhw297
23. Han S, Chen Y, Zheng R, Li S, Jiang Y, Wang C, et al. The stage-specifically accelerated brain aging in never-treated first-episode patients with depression. Hum Brain Mapp. (2021) 42:3656–66. doi: 10.1002/hbm.25460
24. Power JD, Barnes KA, Snyder AZ, Schlaggar BL, Petersen SE. Spurious but systematic correlations in functional connectivity MRI networks arise from subject motion. Neuroimage. (2012) 59:2142–54. doi: 10.1016/j.neuroimage.2011.10.018
25. Power JD, Barnes KA, Snyder AZ, Schlaggar BL, Petersen SE. Spurious but systematic correlations in functional connectivity MRI networks arise from subject motion (vol 59, pg 2142, 2012). Neuroimage. (2012) 63:999–999. doi: 10.1016/j.neuroimage.2012.01.069
26. Han S, Cui Q, Wang X, Li L, Li D, He Z, et al. Resting state functional network switching rate is differently altered in bipolar disorder and major depressive disorder. Hum Brain Mapp. (2020) 41:3295–304. doi: 10.1002/hbm.25017
27. Tomasi D, Volkow ND. Abnormal functional connectivity in children with attention-deficit/hyperactivity disorder. Biol Psychiatry. (2012) 71:443–50. doi: 10.1016/j.biopsych.2011.11.003
28. Zhang B, Li M, Qin W, Demenescu LR, Metzger CD, Bogerts B, et al. Altered functional connectivity density in major depressive disorder at rest. Eur Arch Psychiatry Clin Neurosci. (2016) 266:239–48. doi: 10.1007/s00406-015-0614-0
29. Zou K, Gao Q, Long Z, Xu F, Sun X, Chen H, et al. Abnormal functional connectivity density in first-episode, drug-naive adult patients with major depressive disorder. J Affect Disord. (2016) 194:153–8. doi: 10.1016/j.jad.2015.12.081
30. Liao X-H, Xia M-R, Xu T, Dai Z-J, Cao X-Y, Niu H-J, et al. Functional brain hubs and their test-retest reliability: a multiband resting-state functional MRI study. Neuroimage. (2013) 83:969–82. doi: 10.1016/j.neuroimage.2013.07.058
31. Li W, Qin W, Liu H, Fan L, Wang J, Jiang T, et al. Subregions of the human superior frontal gyrus and their connections. Neuroimage. (2013) 78:46–58. doi: 10.1016/j.neuroimage.2013.04.011
32. Gourley D, Pasha EP, Kaur SS, Haley AP, Tanaka H. Association of dementia and vascular risk scores with cortical thickness and cognition in low-risk middle-aged adults. Alzheimer Dis Assoc Disord. (2020) 34:313–7. doi: 10.1097/WAD.0000000000000392
33. Lemaitre H, Goldman AL, Sambataro F, Verchinski BA, Meyer-Lindenberg A, Weinberger DR, et al. Normal age-related brain morphometric changes: nonuniformity across cortical thickness, surface area and gray matter volume? Neurobiol Aging. (2012) 33:617.e1–9. doi: 10.1016/j.neurobiolaging.2010.07.013
34. Courtney SM, Petit L, Maisog JM, Ungerleider LG, Haxby JV. An area specialized for spatial working memory in human frontal cortex. Science. (1998) 279:1347–51. doi: 10.1126/science.279.5355.1347
35. Owen AM, Evans AC, Petrides M. Evidence for a two-stage model of spatial working memory processing within the lateral frontal cortex: a positron emission tomography study. Cereb Cortex. (1996) 6:31–8. doi: 10.1093/cercor/6.1.31
36. du Boisgueheneuc F, Levy R, Volle E, Seassau M, Duffau H, Kinkingnehun S, et al. Functions of the left superior frontal gyrus in humans: a lesion study. Brain. (2006) 129(Pt 12):3315–28. doi: 10.1093/brain/awl244
37. Hornberger M, Geng J, Hodges JR. Convergent grey and white matter evidence of orbitofrontal cortex changes related to disinhibition in behavioural variant frontotemporal dementia. Brain. (2011) 134(Pt 9):2502–12. doi: 10.1093/brain/awr173
38. Song Y, Xu W, Chen S, Hu G, Ge H, Xue C, et al. Functional MRI-specific alterations in salience network in mild cognitive impairment: an ALE meta-analysis. Front Aging Neurosci. (2021) 13:695210. doi: 10.3389/fnagi.2021.695210
39. Cai S, Huang L, Zou J, Jing L, Zhai B, Ji G, et al. Changes in thalamic connectivity in the early and late stages of amnestic mild cognitive impairment: a resting-state functional magnetic resonance study from ADNI. PLoS ONE. (2015) 10:e0115573. doi: 10.1371/journal.pone.0115573
40. Wang Z, Xia M, Dai Z, Liang X, Song H, He Y, et al. Differentially disrupted functional connectivity of the subregions of the inferior parietal lobule in Alzheimer's disease. Brain Struct Funct. (2015) 220:745–62. doi: 10.1007/s00429-013-0681-9
41. Jacobs HIL, Van Boxtel MPJ, Heinecke A, Gronenschild EHBM, Backes WH, Ramakers IHGB, et al. Functional integration of parietal lobe activity in early Alzheimer disease. Neurology. (2012) 78:352–60. doi: 10.1212/WNL.0b013e318245287d
42. Wang Z, Liang P, Jia X, Jin G, Song H, Han Y, et al. The baseline and longitudinal changes of PCC connectivity in mild cognitive impairment: a combined structure and resting-state fMRI study. PLoS ONE. (2012) 7:e36838. doi: 10.1371/journal.pone.0036838
43. Gramespacher H, Richter N, Thanarajah SE, Jacobs HIL, Dillen KNH, Nellessen N, et al. Aberrant frontostriatal connectivity in Alzheimer's disease with positive palmomental reflex. Eur J Neurol. (2020) 27:2405–14. doi: 10.1111/ene.14443
44. Zhao Q, Sang X, Metmer H, Swati ZNNK, Lu J, Initiative ADN. Functional segregation of executive control network and frontoparietal network in Alzheimer's disease. Cortex. (2019) 120:36–48. doi: 10.1016/j.cortex.2019.04.026
45. Badhwar A, McFall GP, Sapkota S, Black SE, Chertkow H, Duchesne S, et al. A multiomics approach to heterogeneity in Alzheimer's disease: focused review and roadmap. Brain. (2020) 143:1315–31. doi: 10.1093/brain/awz384
46. Habes M, Grothe MJ, Tunc B, McMillan C, Wolk DA, Davatzikos C. Disentangling heterogeneity in Alzheimer's disease and related dementias using data-driven methods. Biol Psychiatry. (2020) 88:70–82. doi: 10.1016/j.biopsych.2020.01.016
47. Han S, Xu Y, Guo H-R, Fang K, Wei Y, Liu L, et al. Two distinct subtypes of obsessive compulsive disorder revealed by heterogeneity through discriminative analysis. Hum Brain Mapp. (2022) 43:3037–46. doi: 10.1002/hbm.25833
48. Han S, Xu Y, Guo H-R, Fang K, Wei Y, Liu L, et al. Resolving heterogeneity in obsessive-compulsive disorder through individualized differential structural covariance network analysis. Cereb Cortex. (2022). doi: 10.1093/cercor/bhac163. [Epub ahead of print].
49. Han S, Xu Y, Guo H-R, Fang K, Wei Y, Liu L, et al. Two distinct subtypes of obsessive compulsive disorder revealed by a framework integrating multimodal neuroimaging information. Hum Brain Mapp. (2022) 43:4254–65. doi: 10.1002/hbm.25951
50. Tang X, Liu J. Comparing different algorithms for the course of Alzheimer's disease using machine learning. Ann Palliat Med. (2021) 10:9715–24. doi: 10.21037/apm-21-2013
51. Xu W, Rao J, Song Y, Chen S, Xue C, Hu G, et al. Altered functional connectivity of the basal nucleus of meynert in subjective cognitive impairment, early mild cognitive impairment, and late mild cognitive impairment. Front Aging Neurosci. (2021) 13:671351. doi: 10.3389/fnagi.2021.671351
52. Fang F, Gao Y, Schulz PE, Selvaraj S, Zhang Y. Brain controllability distinctiveness between depression and cognitive impairment. J Affect Disord. (2021) 294:847–56. doi: 10.1016/j.jad.2021.07.106
53. Greicius MD, Srivastava G, Reiss AL, Menon V. Default-mode network activity distinguishes Alzheimer's disease from healthy aging: evidence from functional MRI. Proc Natl Acad Sci U S A. (2004) 101:4637–42. doi: 10.1073/pnas.0308627101
54. Schultz AP, Buckley RF, Hampton OL, Scott MR, Properzi MJ, Peña-Gómez C, et al. Longitudinal degradation of the default/salience network axis in symptomatic individuals with elevated amyloid burden. Neuroimage Clin. (2020) 26:102052. doi: 10.1016/j.nicl.2019.102052
55. Lundstrom BN, Ingvar M, Petersson KM. The role of precuneus and left inferior frontal cortex during source memory episodic retrieval. Neuroimage. (2005) 27:824–34. doi: 10.1016/j.neuroimage.2005.05.008
56. Zhu H, Zhou P, Alcauter S, Chen Y, Cao H, Tian M, et al. Changes of intranetwork and internetwork functional connectivity in Alzheimer's disease and mild cognitive impairment. J Neural Eng. (2016) 13:046008. doi: 10.1088/1741-2560/13/4/046008
57. Schröder J, Pantel J. Neuroimaging of hippocampal atrophy in early recognition of Alzheimer's disease–a critical appraisal after two decades of research. Psychiatry Res Neuroimaging. (2016) 247:71–8. doi: 10.1016/j.pscychresns.2015.08.014
58. Braak H, Braak E. Development of Alzheimer-related neurofibrillary changes in the neocortex inversely recapitulates cortical myelogenesis. Acta Neuropathol. (1996) 92:197–201. doi: 10.1007/s004010050508
59. Machado A, Ferreira D, Grothe MJ, Eyjolfsdottir H, Almqvist PM, Cavallin L, et al. The cholinergic system in subtypes of Alzheimer's disease: an in vivo longitudinal MRI study. Alzheimers Res Ther. (2020) 12:51–51. doi: 10.1186/s13195-020-00620-7
60. Henneman WJP, Sluimer JD, Barnes J, van der Flier WM, Sluimer IC, Fox NC, et al. Hippocampal atrophy rates in Alzheimer disease: added value over whole brain volume measures. Neurology. (2009) 72:999–1007. doi: 10.1212/01.wnl.0000344568.09360.31
61. Postuma RB, Dagher A. Basal ganglia functional connectivity based on a meta-analysis of 126 positron emission tomography and functional magnetic resonance imaging publications. Cereb Cortex. (2006) 16:1508–21. doi: 10.1093/cercor/bhj088
62. Ystad M, Eichele T, Lundervold AJ, Lundervold A. Subcortical functional connectivity and verbal episodic memory in healthy elderly–a resting state fMRI study. Neuroimage. (2010) 52:379–88. doi: 10.1016/j.neuroimage.2010.03.062
63. Cherubini A, Péran P, Caltagirone C, Sabatini U, Spalletta G. Aging of subcortical nuclei: microstructural, mineralization and atrophy modifications measured in vivo using MRI. Neuroimage. (2009) 48:29–36. doi: 10.1016/j.neuroimage.2009.06.035
64. Ota M, Obata T, Akine Y, Ito H, Matsumoto R, Ikehira H, et al. Laterality and aging of thalamic subregions measured by diffusion tensor imaging. Neuroreport. (2007) 18:1071–5. doi: 10.1097/WNR.0b013e3281c10e27
65. Di Paola M, Macaluso E, Carlesimo GA, Tomaiuolo F, Worsley KJ, Fadda L, et al. Episodic memory impairment in patients with Alzheimer's disease is correlated with entorhinal cortex atrophy. A voxel-based morphometry study. J Neurol. (2007) 254:774–81. doi: 10.1007/s00415-006-0435-1
66. Peterburs J, Blevins LC, Sheu Y-S, Desmond JE. Cerebellar contributions to sequence prediction in verbal working memory. Brain Struct Funct. (2019) 224:485–99. doi: 10.1007/s00429-018-1784-0
Keywords: Alzheimer's disease, mild cognitive impairment, fMRI, functional connectivity, default mode network
Citation: Miao D, Zhou X, Wu X, Chen C and Tian L (2022) Distinct profiles of functional connectivity density aberrance in Alzheimer's disease and mild cognitive impairment. Front. Psychiatry 13:1079149. doi: 10.3389/fpsyt.2022.1079149
Received: 25 October 2022; Accepted: 23 November 2022;
Published: 15 December 2022.
Edited by:
Xiao Wang, Chengdu University of Traditional Chinese Medicine, ChinaReviewed by:
Jianfeng Bao, Zhengzhou University, ChinaZhengui Yang, First Affiliated Hospital of Zhengzhou University, China
Copyright © 2022 Miao, Zhou, Wu, Chen and Tian. This is an open-access article distributed under the terms of the Creative Commons Attribution License (CC BY). The use, distribution or reproduction in other forums is permitted, provided the original author(s) and the copyright owner(s) are credited and that the original publication in this journal is cited, in accordance with accepted academic practice. No use, distribution or reproduction is permitted which does not comply with these terms.
*Correspondence: Xiaoguang Zhou, enhnQGJ1cHQuZWR1LmNu; Le Tian, dGlhbmxlQGJ1Y2VhLmVkdS5jbg==