- Federal State Budgetary Institution Centre for Strategic Planning and Management of Biomedical Health Risks of the Federal Medical Biological Agency, Moscow, Russia
Background: Psycho-emotional well-being is essential for living a life of satisfaction and fulfillment. However, depression and anxiety have become the leading mental health issues worldwide, according to the World Health Organization. Both disorders have been linked to stress and other psychological factors. Their genetic basis remains understudied.
Methods: In 2020–2021, the psycho-emotional well-being of 30,063 Russians with no known psychiatric history was assessed using the Hospital Anxiety and Depression Scale (HADS) for general mental health and the HADS subscale A (anxiety) for anxiety. Following the original instructions, an anxiety score of ≥11 points was used as the anxiety threshold. A genome-wide association study was performed to find associations between anxiety and HADS/HADS-A scores using linear and logistic regressions based on HADS/HADS-A scores as binary and continuous variables, respectively. In addition, the links between anxiety, sociodemographic factors (such as age, sex, and employment), lifestyle (such as physical activity, sleep duration, and smoking), and markers of caffeine and alcohol metabolism were analyzed. To assess the risk of anxiety, polygenic risk score modeling was carried out using open-access software and principal component analysis (PCA) to simplify the calculations (ROC AUC = 89.4 ± 2.2% on the test set).
Results: There was a strong positive association between HADS/HADS-A scores and sociodemographic factors and lifestyle. New single-nucleotide polymorphisms (SNPs) with genome-wide significance were discovered, which had not been associated with anxiety or other stress-related conditions but were located in genes previously associated with bipolar disorder, schizophrenia, or emotional instability. The CACNA1C variant rs1205787230 was associated with clinical anxiety (a HADS-A score of ≥11 points). There was an association between anxiety levels (HADS-A scores) and genes involved in the activity of excitatory neurotransmitters: PTPRN2 (rs3857647), DLGAP4 (rs8114927), and STK24 (rs9517326).
Conclusion: Our results suggest that calcium channels and monoamine neurotransmitters, as well as SNPs in genes directly or indirectly affecting neurogenesis and synaptic functions, may be involved in the development of increased anxiety. The role of some non-genetic factors and the clinical significance of physiological markers such as lifestyle were also demonstrated.
1 Introduction
Psycho-emotional well-being is essential for functioning effectively in all areas of life. Anxiety and depression are the most common mental disorders worldwide. According to the World Health Organization (WHO), approximately 264 million people suffered from anxiety disorders in 2017. In 2015, the overall prevalence of mental illness was on the rise, and the number of people with anxiety disorders increased by 14.9% since 2005 (1). The COVID-19 pandemic sparked a global mental health crisis in 2020, with 25% of the world’s population affected by a phobia or an anxiety disorder (2).
Anxiety is of particular interest among all stress-related mental disorders due to its complex psychophysiological nature. Fear and anxiety are natural physiological responses to certain situations. However, anxiety that is overwhelming, persistent, or disproportionate to the actual threat is a psychological condition that can disrupt personal, social, and professional lives.
Anxiety is a term used in psychiatry to describe a variety of disorders. The difference between them is not obvious. Furthermore, anxiety is often associated with other mental disorders, such as depression (3). Some people are anxiety sensitive; they are afraid of feeling anxious and the physical sensations that come with it. Anxiety sensitivity is generally considered to be a personality trait.
According to the National Institute of Mental Health (NIMH), 31.1% of US adults will experience at least one episode of anxiety in their lifetime (4). Despite its prevalence, there are still gaps in our understanding of the causes and underlying mechanisms of anxiety. Its development appears to be triggered by biopsychosocial factors. When stressed or traumatized, genetically predisposed individuals may exhibit clinically significant anxiety symptoms similar to those of other mental disorders (5).
Further research is needed to understand the role of genetic factors in the development of anxiety and the predisposition to excessive pathological anxiety and anxiety sensitivity. According to genetic epidemiological studies, 30–50% of all anxiety disorders are hereditary (6). However, many authors believe that all mental illnesses, not just anxiety, have a genetic component.
One of the least understood aspects of anxiety is the genetic mechanisms underlying its development and progression. Attempts have been made to examine candidate genes encoding monoamine transporters (7, 8). Several cohort studies, such as a recent GWAS involving over 25,000 people with anxiety disorders or a history of episodic anxiety, have examined the genetic basis of anxiety. Five loci of genome-wide significance have been found, including an intergenic region (IGR) on chromosome 9, previously associated with neuroticism, and a locus overlapping neurotrophic receptor tyrosine kinase type 2 (NTRK2), the receptor gene for brain-derived neurotrophic factor (BDNF) (9). Anxiety disorders have been found to have a significant genetic component. However, additional research is needed for a more comprehensive understanding of the relationship between an individual’s genetics and the development and clinical manifestations of anxiety.
Most research on the genetics of anxiety has focused on specific disorders. Therefore, people who are prone to anxiety but do not have a clinically diagnosable pathological condition are of particular interest. Levey et al. used the Generalized Anxiety Disorder 2-item (GAD-2) screening scale to assess anxiety in 200,000 participants in the Million Veterans Program (MVP, USA) (10). They performed a GWAS based on GAD-2 scores and discovered five significant signals for European Americans and one for African Americans throughout the genome. The strongest signals were found on chromosome 1, in the intron of MAD1L, and near SATB1 and SATB1-ASI. Approximately 90% of patients were male; however, one of the strongest signals was in the intron of the estrogen receptor gene (ESR1), previously implicated in anxiety-like behavior in animal models. These results suggest that the mere presence of symptoms could be of value for identifying the genetic factors underlying anxiety. In addition, most anxiety patients are diagnosed with a mild disorder (43.5%). Many people with anxiety are unlikely to seek medical attention and stay outside the scope of anxiety studies.
Unfortunately, there is not much research on the psycho-emotional well-being of the Russian population. Therefore, assessing the prevalence of anxiety in Russia is a challenging task. To better understand the genetics of anxiety, we evaluated data from an epidemiological study (conducted between 2020 and 2021) of 30,063 Russian adults who had never sought psychiatric treatment. To assess overall mental distress and anxiety, we used the Hospital Anxiety and Depression Scale (HADS) and its HADS-A subscale, a widely used and internationally validated screening tool for anxiety and depression. We examined the participants’ caffeine and alcohol consumption, sleep duration, and physical activity as potential factors contributing to increased anxiety levels. We also analyzed the effects of genetic variants associated with alcohol and caffeine metabolism to determine whether tolerance to these substances contributes to anxiety. The collected data were used in a genome-wide association study and a polygenic risk score model of increased anxiety levels (Figure 1).
2 Methods
2.1 Study design and participants
In this study, we used data from an epidemiological study (conducted in 2020–2021) of 30,063 randomly selected adults at least 18 years of age registered in outpatient medical facilities in 8 federal districts of Russia. The regional distribution of participants is shown in Supplementary Table S1.
Whole blood samples were collected from all participants for genome-wide sequencing. The Hospital Anxiety and Depression Scale (HADS) and its HADS-A subscale (anxiety) were used to measure psycho-emotional well-being and anxiety levels. Based on the participants’ responses, medical professionals filled out the questionnaires. The original instructions by Zigmond et al. indicate that a HADS-A score of 8–10 is a borderline case (sub-clinically/clinically insignificant); a HADS-A score of ≥11 is an abnormal case (clinical/clinically significant). However, a series of HADS sensitivity studies has shown that the interpretation of HADS-A scores may vary depending on the disorder (11, 12). Therefore, to avoid overdiagnosis, only participants with a score of ≥11 were classified as showing clinically significant anxiety symptoms. Despite the subclinical anxiety symptoms, those who scored ≤10 were not considered to have anxiety. In some cases, the total score from the HADS was used to measure psychological distress and overall stress.
To analyze alcohol consumption, the participants were divided into three groups based on their drinking behavior and signs of alcohol abuse: Group 1 abstained from alcohol; Group 2 drank alcohol but showed no signs of alcohol abuse; and Group 3 drank alcohol (once a month or more) and displayed signs of alcohol abuse, such as uncontrolled intake, withdrawal syndrome, inability to meet personal and professional commitments, feeling shameful and guilty, alcohol-induced blackouts, etc.
Physical activity was assessed using the Global Physical Activity Questionnaire (GPAQ). The GPAQ measures the intensity, duration, and frequency in three domains of physical activity: work-related activity, travel to and from locations, and recreational activities. The participants’ weekly physical activity levels, or metabolic equivalent task units (METs), were calculated. In line with the WHO recommendations, participants with less than 600 METs a week were considered sedentary. The non-sedentary participants were divided into low-, moderate-, and high-intensity groups using the techniques described in previous studies (13, 14).
2.2 Ethical considerations
All participants gave their informed consent after full disclosure of the scope, methodology, and expected outcomes of the study. The study was approved by the local ethics committee of the Centre for Strategic Planning and Management of Biomedical Health Risks of the Federal Medical Biological Agency (FMBA) (Protocol No. 02, May 28, 2020).
2.3 DNA extraction and genome-wide sequencing
DNA was extracted from whole blood samples using the QIAamp DNA Mini Kit (Qiagen, Germany). A whole-genome sequencing (WGS) library was prepared using the Nextera DNA Flex kit (Illumina, USA), following the manufacturer’s instructions. The samples were sequenced to 150 bp reads with a minimum of 30× mean depth of coverage. The sequencing data from NovaSeq 6000 were demultiplexed using the bcl2fastq2 Conversion Software v2.20 (Illumina) (15). The quality of the generated FASTQ.GZ files was checked using FastQC v0.11.9, a quality control tool for high throughput sequence data (16). Only samples meeting the quality filtration criteria, such as distribution uniformity of nucleotides and G/C composition, were examined. The total size of the Undetermined*.fastq.gz files matched the size settings.
The reads were aligned to the reference genome (GRCh38.d1.vd1) using the Illumina Dragen Bio-IT platform (Illumina, USA). The quality of the generated BAM files was checked using the DRAGEN module of FastQC v0.11.9 (16), SAMtools v1.13 (17), and Mosdepth v0.3.1 (18). Only the samples meeting the quality filtration criteria, such as duplicates and unmapped reads, were examined. Strelka2 (v2.9.10) with default settings was used for small variant calling (19). Only variants with “PASS” status were used in further analyses. Variants violating the Hardy–Weinberg equilibrium (p < 10−6), multiallelic variants, and variants with a minor allele frequency of less than 1% were removed. Supplementary Table S4 provides information on the whole-genome sequence quality metrics.
2.4 Statistical analysis
Python 3.6.9 was used for data analysis. Relative and absolute value frequencies were calculated for categorical variables. Median values, standard deviations, and interquartile ranges were used for numerical variables.
The significance of associations between the variables and anxiety levels was tested by modeling a binary outcome for HADS scores using logistic regression (Statsmodels package). Sex and age were used as covariates.
A value of p of <0.05 was used as the significance threshold. Odds ratios were interpreted as the likelihood of developing anxiety. To address multiple testing, the Bonferroni adjustment (correction) was applied to all test results.
2.5 Genome-wide association study
Logistic regression was used to test genome-wide associations as a binary variable:
where β0 is the constant; βc is the vector of the covariate effect; C is the covariate vector;
βg is the vector of the genotype effect; and G is the genotype vector.
Linear regression was used to test genome-wide associations as a continuous variable:
where β0 is the constant; βc is the coefficient of the covariate effect; C is the vector of the covariates; βg is the vector of the genotype effect vector; and G is the genotype vector.
To account for population structure, Scikit-learn, a free machine learning package for the Python programming language, was used to perform a principal component analysis (PCA) on 15,000 SNPs from the Human Core Exome SNP Array (Illumina) with a minimum frequency of 5%. Over 50 simulations verified the stability of the results with less than 5% variability. The first 10 principal components were used as covariates in the genome-wide association studies. For variant annotation, we used dbSNP build 155.
Each position passing the filtering procedures was optimized. The target variable (anxiety) was encoded as a binary variable (1 for patients with anxiety). Calculations were performed using the Python library (statsmodels v0.12.2) parallelized on the Spark Cluster. Age, sex, and the first 10 principal components were used as covariates. After quality control filtration, 7,710,227 variants were tested. The variants passing a Bonferroni threshold of p < 5,0 × 10−8 were considered significant. The results were visualized using the LocusZoom JavaScript library. All significant variant sites were further analyzed for the quality of sequencing at each position. Information on the quality distribution is provided in the Supplement (Supplementary Figure S5).
2.6 Polygenic risk scores
For proper testing, 10% of the data were excluded from PRS modeling to be used as a test set in assessing the accuracy of the final model.
For the low-and high-anxiety groups, a polygenic risk score model was built using ridge regression in the scikit-learn library (Python v3.8). The regression minimizes the squared difference between the observed and predicted values and penalizes it with the sum of the squared coefficients:
where is the observed value; is the estimated value; β is the ridge regression coefficient, and is the regularization parameter.
SNPs for the regression model were pre-selected based on their GWAS scores calculated as follows:
where is the SNP coefficient; is the coefficient of the age variable; is the coefficient of the sex variable; is the intercept of the model; and is the value of p of the SNP.
The threshold for GWAS scores was chosen at random. SNPs passing the threshold were included in the model along with the following covariates: age, sex, and the first 10 principal components. To train the model, the data were divided into a training set (80%) and a validation set (20%). ROC AUC was calculated based on the performance of the model on the validation set.
The training procedure was repeated with a new threshold and set of SNPs. After a number of repetitions, the model with the highest ROC AUC on the validation set was selected as the final model. This approach allows for the selection of the optimal number of SNPs and provides the highest accuracy (20).
The accuracy of the final model, or ROC AUC, was tested on the test set.
3 Results
3.1 The study cohort
The study included 30,063 Russian adults aged 18 years and older (mean = 50 years) from 8 federal districts of Russia, who had no medical history of anxiety or other psychiatric disorders. Men made up 37.4% of the cohort (n = 11,232); women, 62.6% (n = 18,831). Detailed information on data completeness (sociodemographic factors, lifestyle, questionnaires, etc.) is presented in Supplementary Table S2.
3.1.1 Sociodemographic factors of anxiety
3.1.1.1 Anxiety prevalence
The mean HADS-A score was 6 points [3.0, 9.0]. Overall, 14% of the cohort had anxiety (≥ 11 points, HADS-A). The highest mean score was recorded in the North Caucasian Federal District (6 points); the lowest, in the Far Eastern, Northwestern, Siberian, and Ural Federal Districts (3 points). Supplementary Table S1 provides information on the overall level of stress and psychological distress (HADS) and the mean level of anxiety (HADS-A). Supplementary Figure S1 shows the distribution of HADS-A scores.
Expectedly, sex and age were associated with anxiety levels. Clinical anxiety was twice as prevalent in women as in men (OR = 2.14, value of p = 9.06*10−18). Age had less of an effect (Table 1). Figure 2 illustrates how the mean HADS-A scores varied depending on age in both men and women. The 20–30-year age group had the highest mean score, which then gradually decreased.
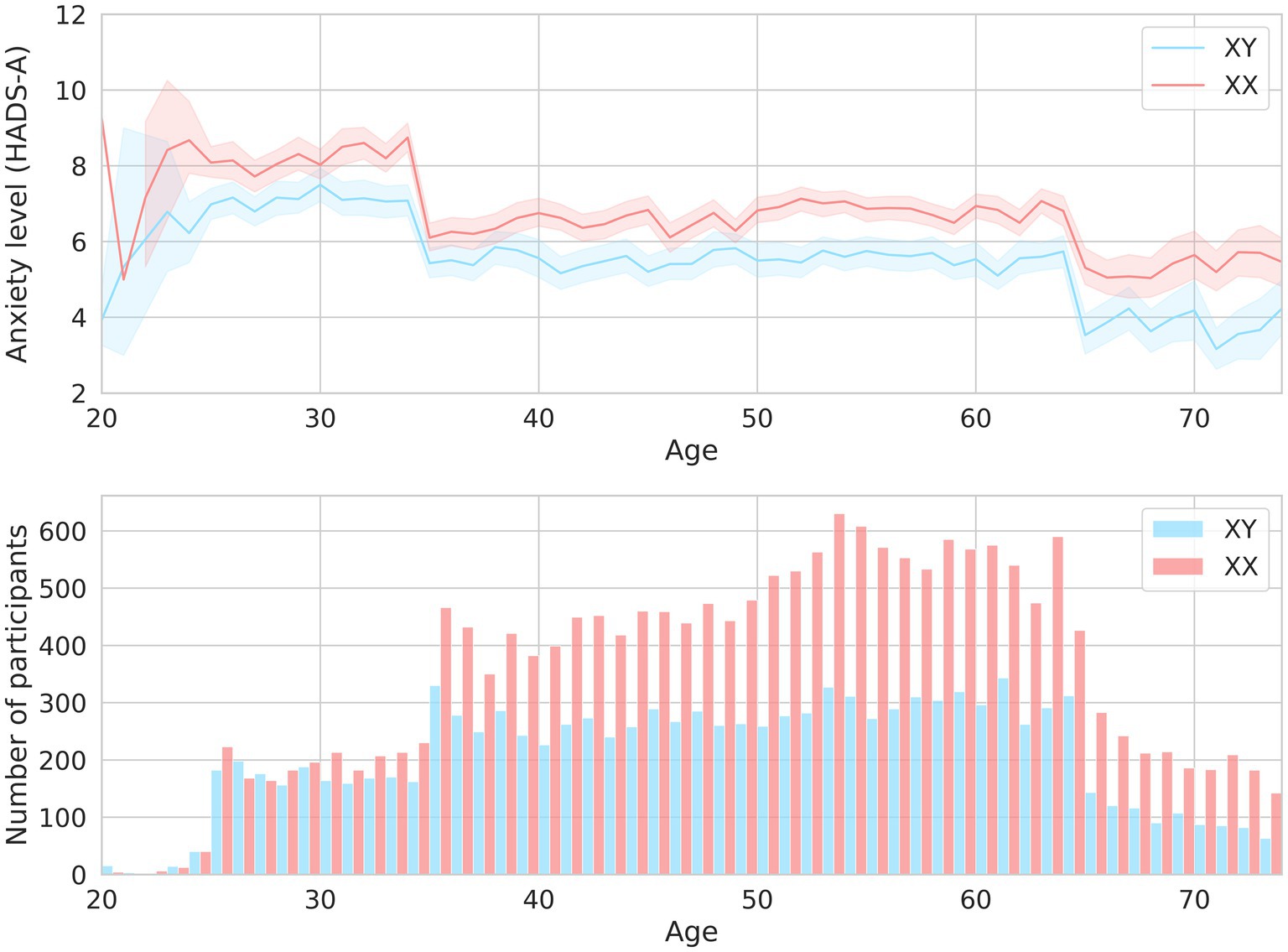
Figure 2. The mean HADS-A scores in men and women of different ages are shown in the upper graph. The men-to-women ratios in different age groups are shown in the lower graph.
Unsurprisingly, there was a strong association between marital status and anxiety levels. Anxiety levels were marginally lower in married participants. Widowhood increased anxiety levels by 40%, while divorce did not have such an effect. Although the number of children had a variety of effects, anxiety levels were markedly higher in the participants with two children (OR = 2.2, value of p = 4.33*10−112, Table 1).
Anxiety levels were also associated with employment: they were higher in the temporarily unemployed, unemployed due to disability, and non-working pensioners and almost three times as high in the unemployed due to a disability as in women.
Thus, there were significant associations between anxiety levels and marital, parental, and employment status. All sociodemographic factors significantly associated with anxiety are listed in Table 1.
3.1.2 Associations between behavioral patterns and anxiety levels
3.1.2.1 Sleep duration
The association between sleep and anxiety was examined in 16,518 participants who reported their average nightly sleep duration in the previous month. Expectedly, there was a negative association between sleep duration and anxiety (coef = −0.21; value of p = 1.2*10−20), even after adjustments for age and sex (coef = −0.2; value of p = 1.08*10−29). Sleep reduced by one hour resulted in a 13.5% increase in anxiety (OR = 0.865 [0.838, 0.891]). Figure 3A shows the associations between sleep duration and anxiety levels (HADS-A). Men experienced increased anxiety only when they slept less than 4 h, while women experienced subclinical anxiety (8–10 points, HADS-A) when they slept 6 h or less.
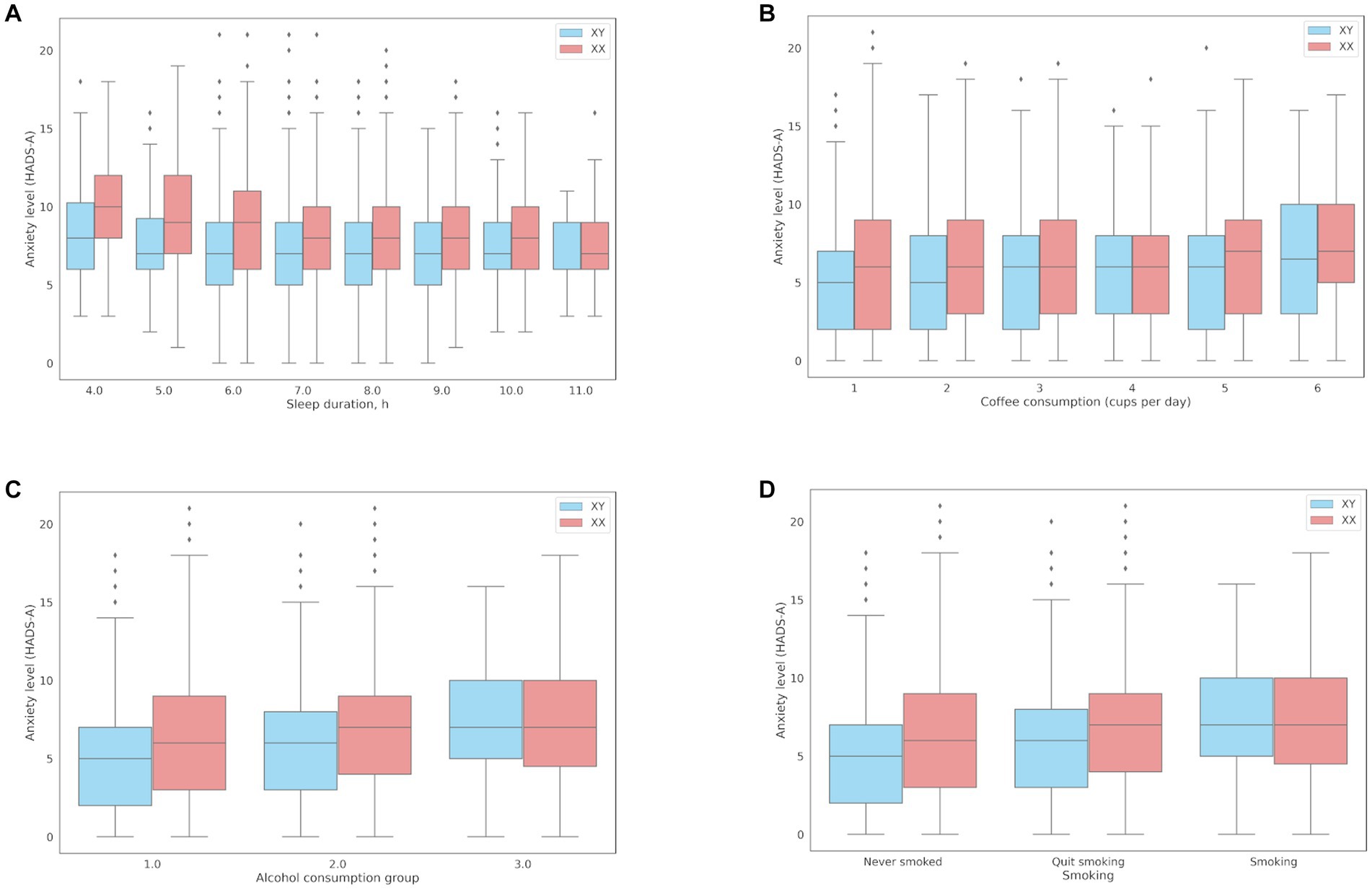
Figure 3. Associations between anxiety levels (HADS-A) and behavioral patterns: (A) Sleep duration; (B) Coffee consumption; (C) The median HADS-A anxiety scores in three groups of alcohol consumption: Group 1, median = 5 points [2.00, 9.00]; Group 2, median = 6 points [4.00, 9.00]; Group 3, median = 7 points [5.00, 10.00]; (D) Smoking.
3.1.2.2 Coffee consumption
The association between coffee consumption and anxiety was examined in 18,293 participants who reported their daily coffee consumption. Compared to the participants with lower anxiety levels, the participants with clinical anxiety (≥ 11 points) on average drank more cups of coffee per day (1.31 ± 1.4 and 1.23 ± 1.28, respectively; value of p = 2.70*10−08). There was a positive association between coffee consumption and anxiety levels (coef = 0.1254; OR = 1.13 per cup; value of p = 2.70*10−08). However, the effects of coffee consumption were somewhat reduced after adjustments for age and sex (coef = 0.0686, OR = 1.07, value of p = 2.33*10−03). Figure 3B shows the associations between daily coffee consumption and anxiety levels.
3.1.2.3 Alcohol consumption
The association between alcohol consumption and anxiety was assessed in 26,864 participants who reported their alcohol consumption. Anxiety levels were more than twice as high in the participants who showed signs of alcohol abuse (ОR = 2.3; value of p = 6.3*10−09). The results are presented in Table 2.
The mean HADS scores were identical in Groups 1 and 2 and matched the mean score in the entire cohort (10 points); the mean HADS score in Group 3 was higher (13 points). Meanwhile, the mean HADS-A scores varied across all three groups, with the lowest in Group 1 and the highest in Group 3, which was also higher than the mean score in the entire cohort. Anxiety levels in Groups 1–3 are shown in Figure 3C.
3.1.2.4 Smoking
The association between smoking and anxiety levels was examined in all participants (Table 3). There was a positive correlation between smoking and anxiety (OR = 1.15). The lowest anxiety levels were found in lifelong non-smokers.
Panel D in Figure 3 shows that anxiety levels were higher in smokers, including former smokers. This association, however, was more common in women.
3.1.2.5 Physical activity
The association between physical activity and anxiety levels was assessed in 27,320 participants. No linear relationship was found between the level of anxiety and physical activity. However, anxiety levels differed between the low-, moderate-, and high-intensity groups in both men and women (Table 4; Figure 4). The high-intensity group had the highest HADS-A scores.

Table 4. Association between physical activity and increased anxiety levels, adjusted for age and sex.
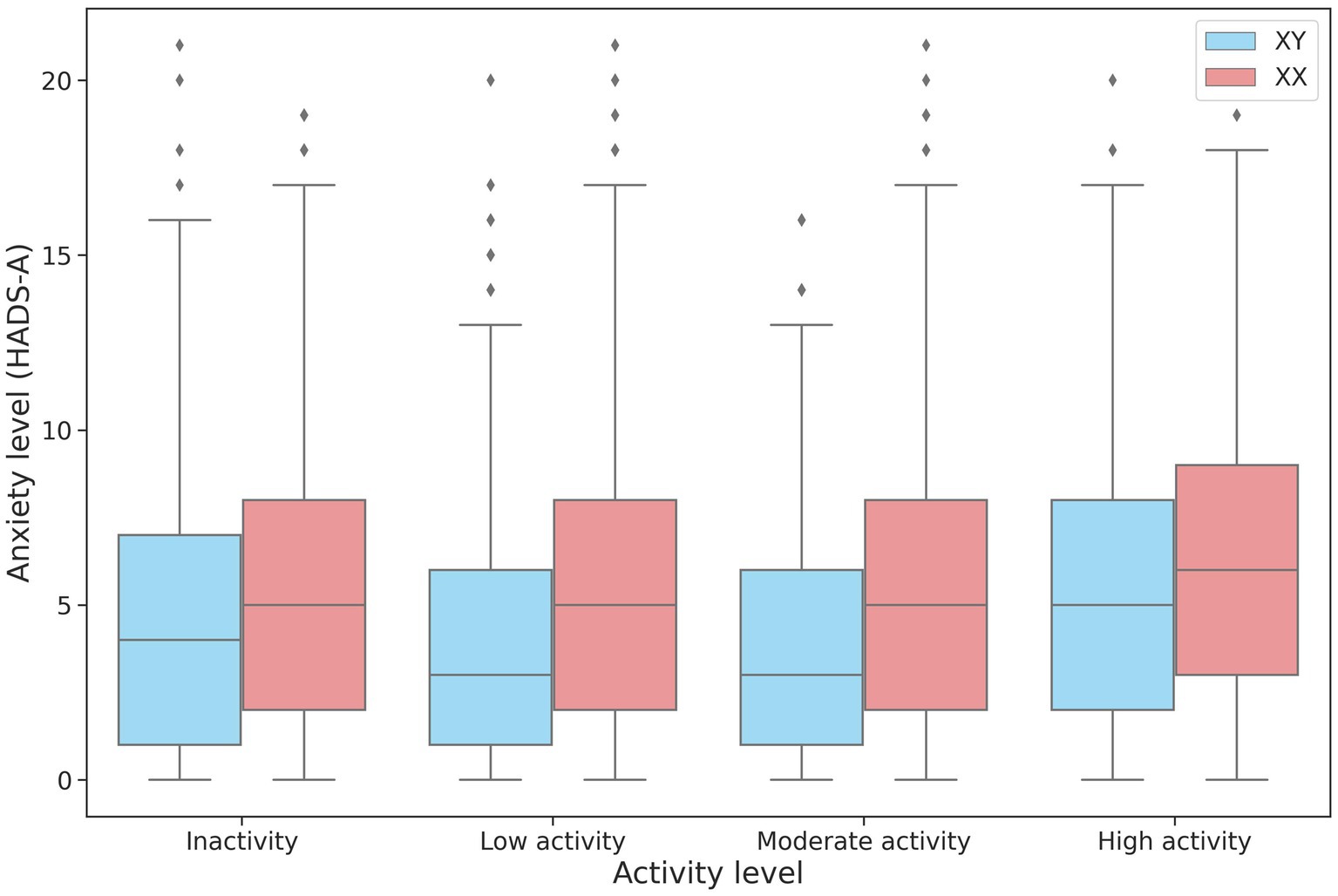
Figure 4. Changes in HADS-A scores depending on the intensity of physical activity in men and women.
Additionally, we conducted a multivariate analysis of the following parameters: age, sex, number of children, marital status, alcohol consumption, coffee consumption, and sleep duration. The final model included all of the above parameters and generated a ROC AUC of 63.2% (Supplementary Figure S3). The multivariate and univariate analyses (Supplementary Figure S2) produced largely similar results, with minor differences due to a strong correlation between the parameters.
3.2 Specific genetic markers
Based on the behavioral patterns, we examined specific genetic markers of caffeine and alcohol metabolism and assessed their association with anxiety.
3.2.1 Markers of caffeine metabolism
The genetic variants rs762551 (C > A) in CYP1A2 and rs5751876 (T > C) in ADORA2A were examined as genetic markers of caffeine metabolism. According to the Pharmacogenomics Knowledge Base (PharmGKB), rs762551 АС and СС genotype carriers are “slower” caffeine metabolizers than АА genotype carriers, while rs5751876 TT genotype carriers are more likely to experience changes in anxiety levels in response to caffeine than СС and СТ genotype carriers. The participants were divided based on their caffeine intolerance: Group 0, no caffeine intolerance (rs762551 АА and rs5751876 СС or СТ); Group 1, carriers of one substitution (rs762551 АС or СС or rs5751876 ТТ); Group 2, carriers of two substitutions (rs762551 АС and СС and rs5751876 ТТ). The daily coffee consumption for each group is presented in Table 5. Figure 5A shows the effects of daily coffee consumption in these three groups. Anxiety levels were higher in the participants who consumed more coffee. However, this association was significant only in Group 1 (value of p = 4*10−6). It was insignificant in Group 2, probably due to the small number of participants. The significance of this association may increase for a larger sample.
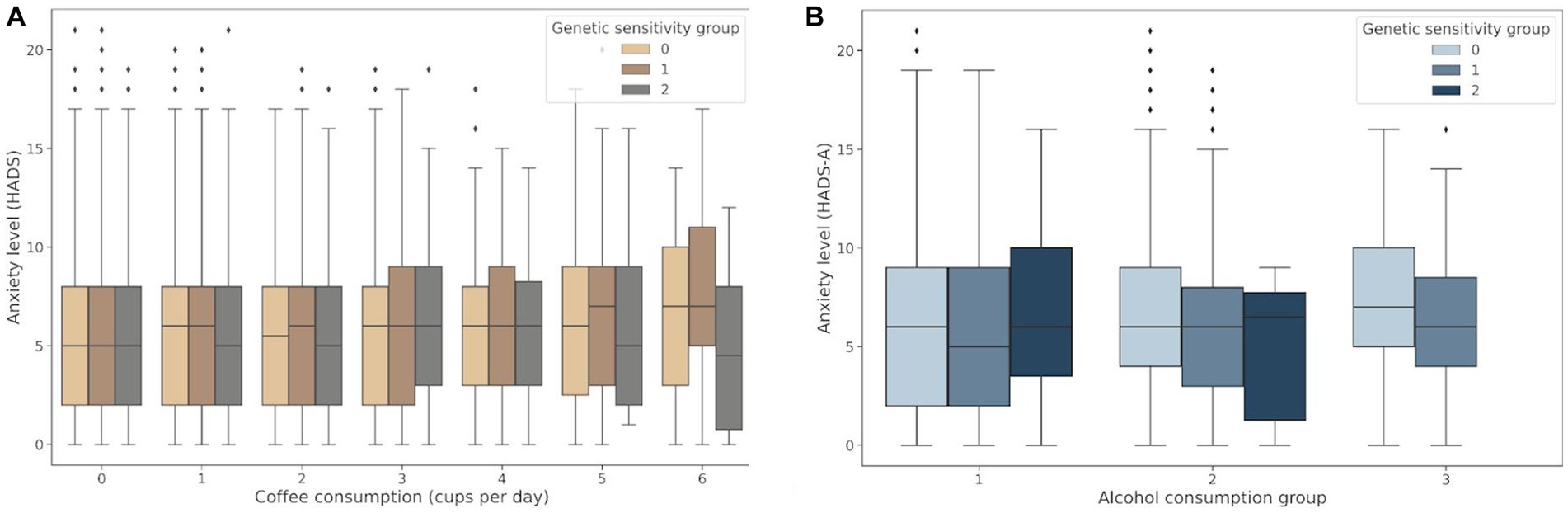
Figure 5. (A) Association between daily coffee consumption and anxiety levels in Groups 0–2; (B) Changes in anxiety levels in response to alcohol consumption in Groups 0–2: Group 0, no alcohol intolerance; Group 1, one marker of alcohol intolerance (rs671 or rs1229984); Group 2, two markers of alcohol intolerance (rs671 and rs1229984).
3.2.2 Markers of alcohol metabolism
Both rs671 (G > A) in ALDH2 and rs1229984 (T > C) in ADH1B were examined as markers of alcohol intolerance. The rs671 GG genotype has been linked to an increased risk of excessive alcohol consumption and alcohol dependence (21). However, this genotype is uncommon in the European population. Therefore, the rs1229984 CC genotype was also examined as another marker of predisposition to alcohol abuse, previously detected in the European population (22). The participants were divided based on their alcohol intolerance: Group 0, no alcohol intolerance (carriers of rs671 GG and rs1229984 CC); Group 1, carriers of one substitution associated with alcohol intolerance (rs671 AA or AG or rs1229984 TT or СТ); Group 2, carriers of two substitutions associated with alcohol intolerance (rs671 AA or AG and rs1229984 TT or СТ). Table 6 details alcohol consumption patterns in Groups 0–2. The graph in Figure 5B shows changes in the HADS-A scores in Groups 0–2 depending on the alcohol consumption patterns.
Alcohol consumption increased anxiety levels both in non-carriers and one substitution carriers (value of p = 1.48*10−39 and value of p = 4.8*10−3, respectively). However, anxiety levels were higher only in non-carriers with a tendency for alcohol abuse. Meanwhile, one substitution carriers had elevated anxiety levels even without signs of alcohol abuse. In two substitution carriers, anxiety levels also trended upward in response to alcohol consumption; however, this association was not significant, probably due to the small number of people in the group. There were no two substitution carriers showing signs of alcohol abuse.
The results of the analysis (Supplementary Table S3) suggest that caffeine/alcohol intolerance in combination with excessive alcohol/caffeine consumption may be linked to increased anxiety levels.
3.3 GWAS results
We performed a genome-wide association study (GWAS) to assess the role of genetic variants in the development of anxiety. The comparison of the participants with clinical anxiety (≥ 11, HADS-A) and participants with no clinical anxiety (< 11, HADS-A) revealed 27 polymorphisms significantly associated with clinical anxiety (p < 5.0 × 10−8). These variants were located on different chromosomes (Figure 6A).
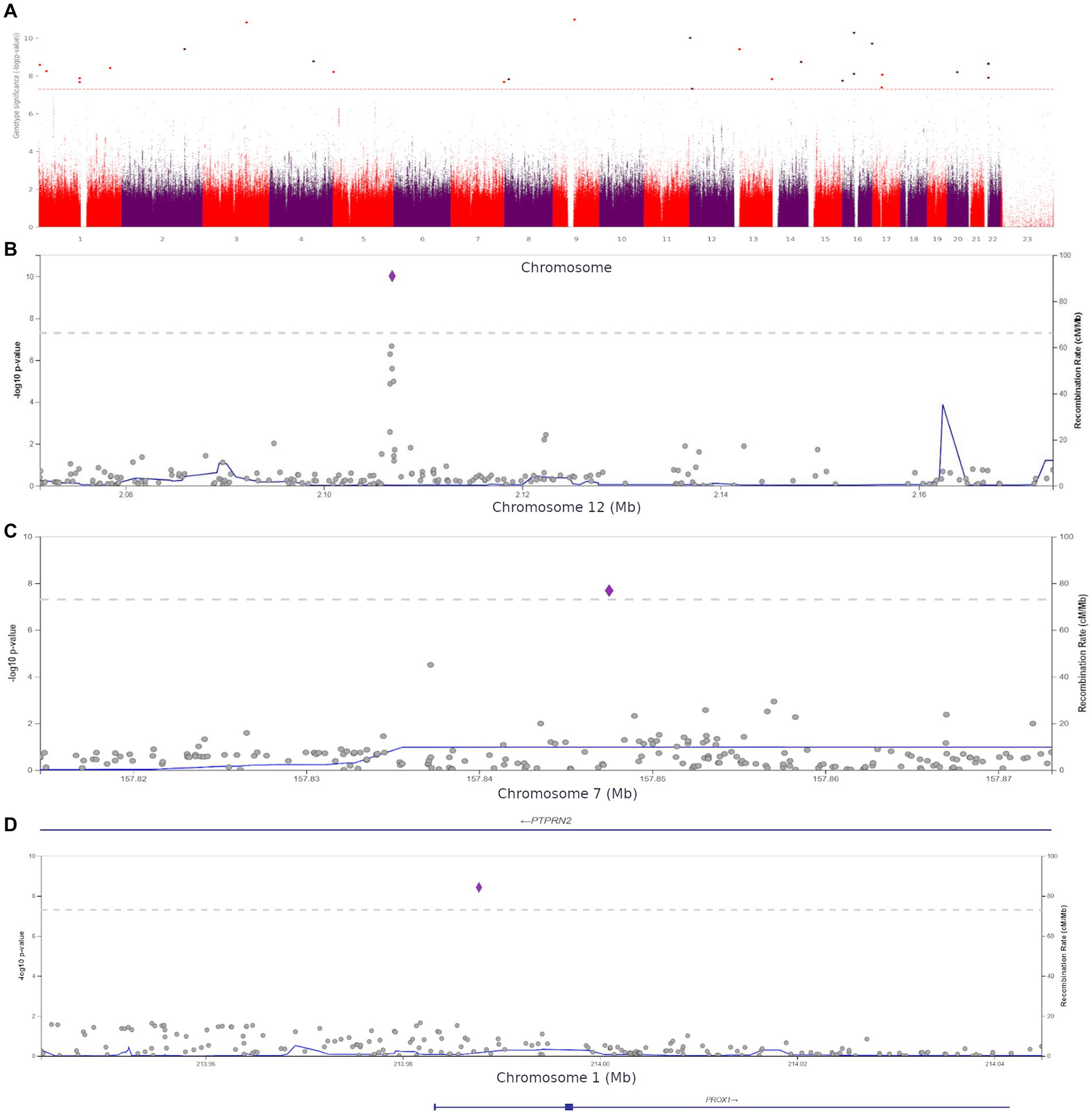
Figure 6. (A) Manhattan plot for associations between the detected variants and clinical anxiety (HADS-A, ≥11 points). The 5.0 × 10−8 and 1 × 10−6 significance thresholds are represented by gray dotted lines. (B) Association plot for the locus on chromosome 12 (chr12:2071357–2,173,517). The 5.0 × 10−8 significance threshold is represented by a gray dotted line. The SNP rs1205787230 with the strongest association with clinical anxiety is represented by a purple diamond. (C) Association plot for the locus on chromosome 7 (chr7:157814623–157,873,078). The 5.0 × 10−8 significance threshold is represented by a gray dotted line. The SNP rs3857647 with the strongest association with clinical anxiety is represented by a purple diamond. (D) Association plot for the locus on chromosome 1 (chr1:213943304–214,044,772). The 5.0 × 10−8 significance threshold is represented by a gray dotted line. The SNP rs941727476 with the strongest association with clinical anxiety is represented by a purple diamond. Linkage data were obtained from the 1,000 Genomes Project (1KGP). Color codes: red, r2 ≥ 0.8; yellow, r2 ≥ 0.6; green, r2 ≥ 0.4; blue, r2 ≥ 0.2; dark blue, r2 ≥ 0.0; gray, no data available.
The Supplement (Supplementary Table S5) contains all variants with genome-wide significance. The discovered SNPs had not been previously associated with anxiety. Some of them were located in genes previously associated with several other psychiatric disorders, while others were in intergenic regions. For instance, rs1205787230 was found in the calcium channel gene CACNA1C. Loci containing significant variants are shown in Figures 6B–D.
Using HADS-A scores as a continuous variable, we discovered 15 more variants (Supplementary Table S6) shown in Figure 7A.
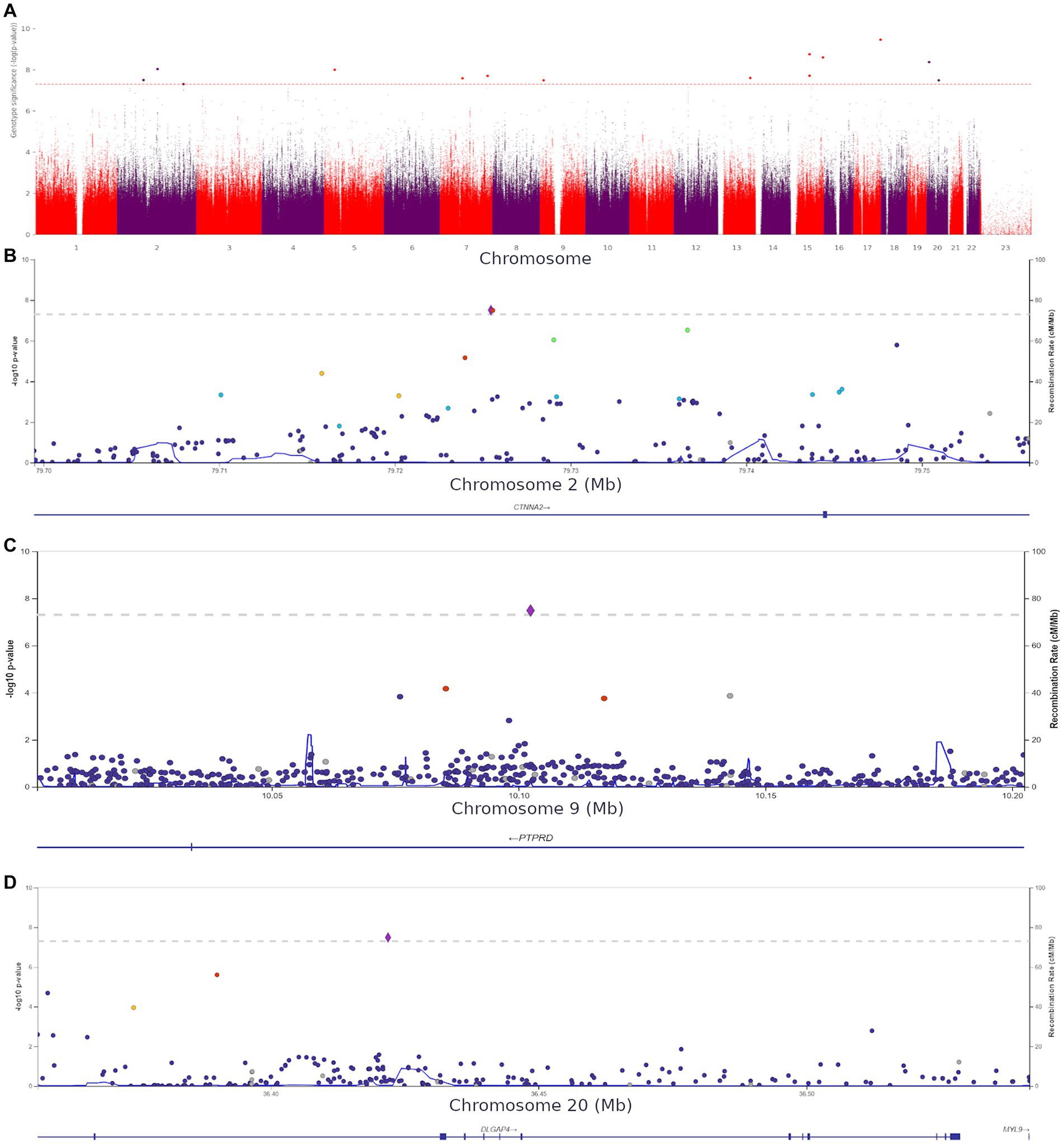
Figure 7. (A) Manhattan plot for associations between the detected variants and HADS-A scores. The 5.0 × 10−8 and 1 × 10−6 significance thresholds are represented by gray dotted lines. (B) Association plot for the locus on chromosome 2 (chr2:79699464–79,756,118). A 5.0 × 10−8 threshold for significance is represented by a gray dotted line. The SNP rs72927416 with the strongest association with clinical anxiety is represented by the purple diamond. (C) Association plot for the locus on chromosome 9 (chr9:10002424–10,202,424). The 5.0 × 10−8 significance threshold is represented by a gray dotted line. The SNP rs832264 with the strongest association with clinical anxiety is represented by a purple diamond. (D) Association plot for the locus on chromosome 20 (chr20:36356540–36,541,606). The 5.0 × 10−8 significance threshold is represented by a gray dotted line. The SNP rs832264 with the strongest association with clinical anxiety is represented by a purple diamond. Linkage data were obtained from the 1,000 Genomes Project (1KGP). Color codes: red, r2 ≥ 0.8; yellow, r2 ≥ 0.6; green, r2 ≥ 0.4; blue, r2 ≥ 0.2; dark blue, r2 ≥ 0.0; gray, no data available.
We detected two CTNNA2 polymorphisms significantly associated with high HADS-A scores: rs72927416 and rs7558324 (Figure 7B). Notably, many of the discovered variants, including one DLGAP4 variant and PTPRD (rs832264) on chromosome 9, were associated with lower HADS-A scores, i.e., with the absence of clinical anxiety symptoms (Figures 7C,D).
These variants have not been previously linked to anxiety or psychiatric disorders. However, some of them might be crucial for better understanding the neurobiological basis of anxiety. The detection of CACNA1C rs1205787230 supports the hypothesis that calcium channels are involved in the development of increased anxiety in non-psychiatric patients. The detection of PTPRN2, which is required for the accumulation of noradrenaline, dopamine, and serotonin in the brain, adds to the evidence that monoamine neurotransmitters play a role in the development of anxiety. Moreover, some of the variants were located in genes directly or indirectly affecting neurogenesis and synaptic functions. Supplementary Figures S6, S7 show QQ plots and lambda values for both GWASs.
3.4 Polygenic risk score model of anxiety
To predict the risk of increased anxiety (≥ 11 points, HADS-A), we built polygenic risk score (PRS) models. The ROC AUC of the final model was 97.66% ± 1.86% on the training set and 89.44% ± 2.24% on the test set (Figure 8). In addition, we built models based on two types of data: phenotypes, such as sex and age, which were used as covariates in the general model; and phenotypes combined with the first 10 principal components. This combination eliminated the effect of population structure. The accuracy values of these models were 59.15 and 61.38%, respectively.
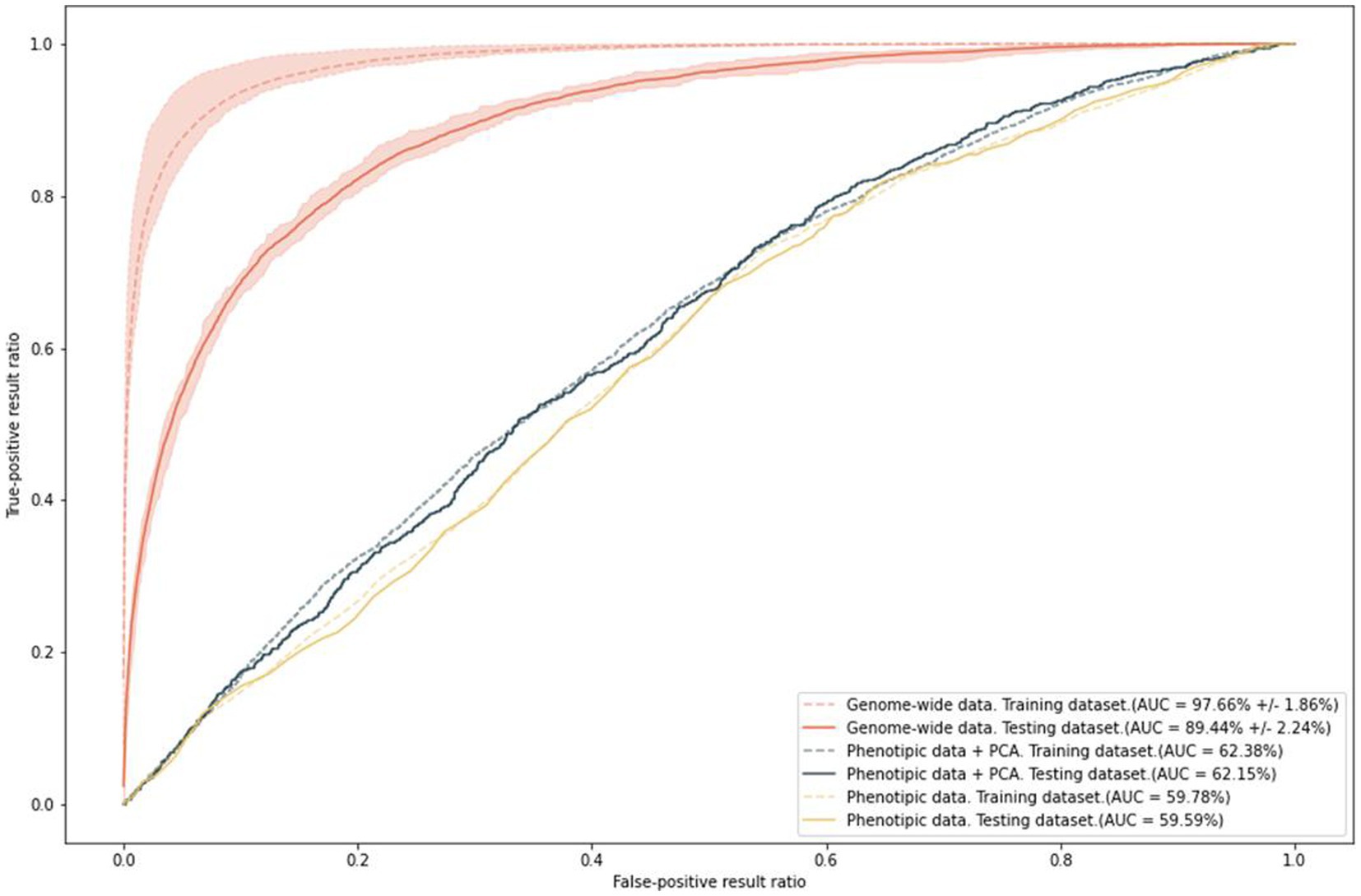
Figure 8. Results of PRS model training with anxiety as a binary variable; ROC AUC on the two types of data.
The final model included 9,535 SNPs. Notably, the SNPs that did not reach the standard significant threshold in the GWAS had the largest “weights” in the model. These SNPs are listed in Supplementary Table S7.
The ROC AUC of the models on the test set slightly decreased. Nevertheless, the final model is extremely sensitive and specific for predicting the risk of anxiety. In contrast, age and sex, which are known to be significantly associated with anxiety, can only generate a prediction accuracy of about 60%.
4 Discussion
Anxiety is currently one of the most prevalent psycho-emotional disorders, associated with both genetic and non-genetic factors. This study assessed the prevalence of anxiety in Russia and examined its genetic architecture and risk factors.
We found associations between psycho-emotional well-being and sex, marital status, parental status, employment status, and lifestyle. According to the National Comorbidity Survey, anxiety is 1.5 times more common in women (23). In our study, anxiety levels were twice as high in women as in men. Studies on other populations have also found that social and marital well-being are associated with mental health (24). In married participants and participants with one child, anxiety levels were lower; in participants with two children and widows/widowers, anxiety levels were increased. Anxiety levels were higher among the unemployed, particularly due to disability or retirement.
Notably, young participants aged 20–30 had the highest level of anxiety. Previous epidemiological research has also shown that anxiety is more common in younger individuals (25). Participants in this age group were most likely going through a “critical period” of establishing themselves in their professional, personal, and social lives, which may have affected their psycho-emotional state.
Some unhealthy habits and lifestyle choices can affect psycho-emotional well-being. Alternatively, psycho-emotional distress can lead to lifestyle changes or addictions. Short sleep duration has been linked to reduced stress resilience and mood swings in healthy individuals (26). The National Institutes of Health (NIH) estimates that over 60% of sleep disorders in the United States are associated with increased levels of anxiety. In our study, there was an inverse association between sleep duration and anxiety levels. Women were more sensitive to a sleep deficit: ≤ 6 h of sleep per day increased anxiety to the subclinical level (HADS-A).
Smoking was another factor associated with increased anxiety, which is generally consistent with previous studies (27, 28). We found that increased anxiety was 15% more common in smokers and 13% less common in lifelong non-smokers. Importantly, anxiety levels were lower in former smokers.
We also found an association between anxiety and caffeine/alcohol consumption. Anxiety has been identified as a comorbid disorder or symptom of alcohol dependence in several studies (29). Caffeine has a very individual effect and can cause side effects, such as anxiety, even in safe amounts (30). In our study, alcohol consumption patterns indicative of alcohol abuse or dependence were associated with increased anxiety. However, the mean HADS-A score was also higher in alcohol consumers with no signs of abuse than in non-drinkers. Individuals prone to anxiety may turn to alcohol as a form of self-medication (31). Some authors believe that increased anxiety in alcohol abusers could be managed by treating their alcohol dependence (29). Notably, the participants with normal and increased anxiety levels did not show significant differences in the prevalence of genetic traits associated with alcohol intolerance caused by reduced enzymatic activity of human aldehyde dehydrogenase 2 (ALDH2). The study cohort did not include carriers of two substitutions with signs of alcohol abuse; nonetheless, the alcohol-consuming carriers of one substitution had increased anxiety even without signs of alcohol abuse. In non-carriers of intolerance-related variants, the mean HADS-A anxiety score increased only in response to alcohol abuse.
According to the Food and Drug Administration (FDA), 400 mg of caffeine per day, or 4–5 cups of coffee, is safe for most people. In our cohort, increased coffee consumption was associated with increased anxiety levels; however, the average daily coffee intake was still at an acceptable and safe level. It should be noted that several factors affect caffeine metabolism, processing, and excretion, including age, sex, hormones, and obesity. Genetic predisposition also significantly contributes to the rate of caffeine metabolism (32). Genetic variants associated with slow caffeine metabolism (33) have been linked to increased anxiety levels in response to coffee consumption (34). In our study, however, we found no compelling evidence that caffeine increased anxiety levels in carriers of the genetic markers of slow caffeine metabolism. Nonetheless, drinking four or more cups of coffee per day only marginally increased anxiety levels in all participants.
Many studies have examined the link between physical activity and mental health. However, the data on this subject is still very limited. Nevertheless, there is evidence that a sedentary lifestyle may be associated with anxiety (35). In our cohort, a sedentary lifestyle led to a 16% increase in anxiety levels. HADS-A scores showed an upward trend only in the male participants who engaged in high-intensity physical activity. Thus, the impact of daily physical activity and exercise is not straightforward and needs further research.
4.1 Genome-wide association study
We identified associations between genetic traits and anxiety in both binary and continuous trait tests. Although these genetic traits had not been associated with anxiety, some of them were within genes associated with other mental disorders and anxiety-like behavior in animal models.
Certain substitutions were of special interest since they were located within regions previously associated with mental disorders. We detected an SNP within a gene encoding subunits of calcium channels—CACNA1C (rs1205787230). The Cav1.2 channel, the α1C subunit of which is encoded by CACNA1C, has also been implicated in fear-related memory formation and pathway activation (36); however, its role in the brain and other tissues needs further research. CACNA1C SNPs have been repeatedly detected in studies on bipolar affective disorder (BAD) (37). However, functional magnetic resonance imaging (fMRI) revealed amygdala activation during emotional processing both in healthy carriers of the CACNA1C rs1006737 risk allele and people with BAD and schizophrenia (38). This finding is especially important, since amygdala subregions are known to play a critical role in mediating anxiety (39). When anxiety patients were exposed to a trigger, their amygdalae became hyper-activated (40). A recent study showed that impaired expression of CACNA1C disrupts spontaneous Ca2+ activity, leading to abnormal brain development and increased anxiety (41).
Another Ca2+ activity-associated gene, PIEZO1 (rs371838333), also contained a significant SNP. The expression of PIEZO1 was studied in adult rats: in the cerebral cortex after ischemia/reperfusion injury (IRI) and in PC12 neuron-like cells after oxygen–glucose deprivation/reoxygenation (OGD/R) injury (42). The study found that the inhibition of PIEZO1 could decrease intracellular Ca2+ concentrations following OGD/R. Hence, there is evidence that PIEZO1 may be involved in brain injury via the activation of Ca2+/calpain signaling, while its abnormal expression may play a role in neuronal apoptosis after OGD/R.
Anxiety has traditionally been associated with the dysfunction of monoaminergic systems. Dopaminergic (DA), noradrenergic (NA), and serotonergic (5-HT) circuitries play an essential role in the neurobiology of major depression disorder (MDD) and anxiety disorders (43). We found no significant SNPs in the genes regulating monoamine neurotransmitters. However, there was a strong association between clinical anxiety and PTPRN2 (rs3857647), which is required for noradrenaline, dopamine, and serotonin accumulation in the brain. Hypermethylated cytosine sites of PTPRN2 have been linked to depression in trauma survivors (44).
We detected many significant genetic variants associated with HADS-A scores. For instance, STK24 (rs9517326), involved in excitatory synaptic transmission, was associated with higher scores. Previously, STK24 overexpression was found to increase neuronal excitability in a hippocampal neuronal culture model. Moreover, STK24 was identified as a possible binding partner of N-methyl-D-aspartate (NMDA) (glutamate receptor) in a study on epilepsy in mice (45).
High HADS-A scores in our study were significantly associated with two SNPs, CTNNA2 (rs72927416), and CTNNA2 (rs7558324). CTNNA2 encodes a protein essential for normal cortical neuronal migration and neurite outgrowth. It is involved in intrauterine brain development and has been implicated in several psychiatric disorders, such as BAD (46), ADHD (47), alcoholism, schizophrenia, and general cognitive dysfunction (48). The detected variants have not been previously studied; other CTNNA2 and PTPRD variants, however, have been linked to personality traits, such as impulsivity, schizophrenia, and autism, which manifest as unresponsiveness to visual stimuli (49). Alpha N-catenin is thought to be critical to synaptic contact stability and dendritic spine motility, which are crucial for neural plasticity. In mice, alpha N-catenin deficiency disrupts axon migration and causes abnormal startle responses and behaviors consistent with prepulse inhibition (50), which is believed to be an endophenotype of human psychosis. Monkeys classified as inhibited in infancy were more likely to be overly cautious in adulthood, while inhibited temperament (IT) was found to be hereditary. Moreover, an SNV with genome-wide significance was detected near CTNNA2 (51).
Conversely, PTPRD (rs832264) was associated with lower HADS-A scores. This gene encodes the receptor-type protein tyrosine phosphatase, which interacts with several postsynaptic cell adhesion molecules via synapses. It has been associated with restless legs syndrome (RLS) (52) and psychoactive drug addiction. It has been hypothesized that lower PTPRD expression is associated with a reduced predisposition to psychoactive drug addiction, such as opioids (53). There is further evidence that PTPRD expression may affect the risk of Alzheimer’s disease and other neurodegenerative diseases (54, 55). Moreover, PTPRD variants have been recently implicated in schizophrenia (56, 57) and obsessive–compulsive disorder (OCD) (58). It is worth noting that PTPRD polymorphisms have been linked to mood instability based on the responses from the UK Biobank participants (59). In some cases, mood swings can be exacerbated by stress-related anxiety and persistent nervousness. Hence, the effects of the receptor-type protein tyrosine phosphatase on mental health are complex and should be studied further as a promising research topic.
DLGAP4 (rs6039798) was another variant associated with lower HADS-A scores. DLGAP4 encodes the membrane-associated guanylate kinase, which is localized in the postsynaptic density in neural cells. DLGAP proteins bind to three main glutamate receptors: NMDAR, AMPAR, and mGluR. They have been associated with numerous psychiatric and neurological disorders, including schizophrenia, OCD, and cerebellar ataxia (60). The role of glutamate in anxiety has been investigated in studies on the therapeutic effects of drugs that modulate its function (61). Pathological anxiety could also be caused by a GABA-glutamate imbalance leading to increased neuronal excitation (62). Supplementary Table S8 provides descriptions of other genes that contain significant associations. Functional changes in these genes might underlie many mental and neurological disorders and should be further studied. Their involvement in the development of anxiety also requires a more detailed examination.
4.2 Polygenic risk score
The genetic predisposition to anxiety is polygenic. The identified genes only partially explain the genetic risk. Therefore, we built a polygenic risk model of increased (clinical) anxiety that could be used to screen and identify risk groups. We analyzed 50 positions with the lowest p-values and selected the ones located in coding regions. The most significant were downstream gene variant KLRB1 and some of the variants in the introns of UBE2G1, SRRM4, TRIM2, SAMD12, SRRM4, SEMA5B, ANKFY1, WWOX, SLCO1B1, TENM3, MAGI2, and GSE1.
SRRM4 regulates neuronal differentiation and the splicing of protrudin gene transcripts, which are involved in neurite outgrowth during neurogenesis (63). Ubiquitin Conjugating Enzyme E2 G1 (UBE2G1) transcripts in the blood could be used as biomarkers of Alzheimer’s disease (64). The ubiquitin ligase TRIM2 is involved in axon growth, polarization, and specification and has neuroprotection properties. Changes in TRIM2 function have been linked to progressive neurodegeneration in Alzheimer’s patients, while its variations have been associated with childhood-onset axonal neuropathy (65). Semaphorin 5B (SEMA5B) functions as a guidance cue and is essential for axon guidance and growth. Semaphorins have been linked to synaptic rearrangement (66). The SOX-6 transcription factor is involved in cortical interneuron differentiation. Cortical interneurons have been linked to several disorders, including epilepsy, autism, and schizophrenia; therefore, understanding the molecular basis of their variability may aid in developing new treatment options for these conditions (67). Variations in the scaffold protein coding gene MAGUK Inverted 2 (MAGI-2), which may be implicated in postsynaptic density and neurotransmission, have been found in patients with neurological disorders such as schizophrenia (68).
We also detected significant regions, which were cis-regulatory elements (CREs): chr1:244164789 (a promoter flanking region), which is located near ZBTB18 and ADSS; ZBTB18, which encodes a DNA-binding transcription factor coordinating neurodevelopment during the formation of the mammalian cerebral cortex (69); ADSS (a catalyst in the early stages of adenosine monophosphate synthesis), the overexpression of which has been implicated in schizophrenia, suggesting that it plays an important role in the development of this condition.
5 Conclusion
The identification of the patterns linking lifestyle and genetic predisposition to psycho-emotional disorders and anxiety could provide new treatment and prevention options. Polygenic risk scores as part of screening procedures will enable the identification of high-risk individuals, which is crucial to developing individualized prevention strategies. In genetically predisposed individuals, lifestyle changes could be effective for both preventing and reducing anxiety.
Data availability statement
The original contributions presented in the study are publicly available. This data can be found here: https://www.ebi.ac.uk/gwas/studies/GCP000797; accession number: GCP000797.
Ethics statement
The studies involving human participants were reviewed and approved by the Local Ethics Committee of the Centre for Strategic Planning of FMBA of Russia (Protocol No. 02; May 28, 2020). The patients/participants provided their written informed consent to participate in this study.
Author contributions
AY, DT, DK, AM, and VE: conceptualization ideas and validation. AR, OB, ES, MI, and SM: data curation. ES and MI: formal analysis and software. AA, AR, and DK: investigation. AY, DT, DK, VE, AM, and VY: methodology. VY, VM, AK, SK, and SY: project administration. AA, AR, and OB: resources. DK, VY, AK, SK, and SY: supervision. ES, MI, and MT: visualization. AY, DT, DK, AM, ES, VE, MI, LM, VY, and AR: writing – original draft and writing – review and editing. All authors contributed to the article and approved the submitted version.
Funding
The study was funded by the Centre for Strategic Planning and Management of Biomedical Health Risks.
Acknowledgments
The authors would like to thank the study participants and hospital staff who were integral to the successful completion of this study.
Conflict of interest
The authors declare that the research was conducted in the absence of any commercial or financial relationships that could be construed as a potential conflict of interest.
Publisher’s note
All claims expressed in this article are solely those of the authors and do not necessarily represent those of their affiliated organizations, or those of the publisher, the editors and the reviewers. Any product that may be evaluated in this article, or claim that may be made by its manufacturer, is not guaranteed or endorsed by the publisher.
Supplementary material
The Supplementary material for this article can be found online at: https://www.frontiersin.org/articles/10.3389/fpsyt.2023.1188427/full#supplementary-material
References
1. World Health Organization. (2017). Depression and other common mental disorders: global health estimates. World health Organization; Available at: https://apps.who.int/iris/bitstream/handle/10665/254610/W?sequence=1
2. Health Organization. (2022). Mental health and COVID-19: early evidence of the pandemic’s impact: scientific brief. Available at: https://pesquisa.bvsalud.org/portal/resource/pt/who-352189
4. NIH. (2022). Any anxiety disorder. National Institute of Mental Health (NIMH) [internet]. Available at: https://www.nimh.nih.gov/health/statistics/any-anxiety-disorder
5. Auxéméry, Y. Posttraumatic stress disorder (PTSD) as a consequence of the interaction between an individual genetic susceptibility, a traumatogenic event and a social context. Encéphale. (2012) 38:373–80. doi: 10.1016/j.encep.2011.12.003
6. Hettema, JM, Neale, MC, and Kendler, KS. A review and meta-analysis of the genetic epidemiology of anxiety disorders. Am J Psychiatry. (2001) 158:1568–78. doi: 10.1176/appi.ajp.158.10.1568
7. Meier, SM, and Deckert, J. Genetics of anxiety disorders. Curr Psychiatry Rep. (2019) 21:16. doi: 10.1007/s11920-019-1002-7
8. Domschke, K, and Maron, E. Genetic factors in anxiety disorders. Mod trends Pharmacopsychiatry. (2013) 29:24–46. doi: 10.1159/000351932
9. Purves, KL, JRI, C, Meier, SM, Rayner, C, KAS, D, Cheesman, R, et al. A major role for common genetic variation in anxiety disorders. Mol Psychiatry. (2020) 25:3292–303. doi: 10.1038/s41380-019-0559-1
10. Levey, DF, Gelernter, J, Polimanti, R, Zhou, H, Cheng, Z, Aslan, M, et al. Reproducible genetic risk loci for anxiety: results from ∼200, 000 participants in the million veteran program. Am J Psychiatry. (2020) 177:223–32. doi: 10.1176/appi.ajp.2019.19030256
11. Terluin, B, Brouwers, EPM, van Marwijk, HWJ, Verhaak, PFM, and van der Horst, HE. Detecting depressive and anxiety disorders in distressed patients in primary care; comparative diagnostic accuracy of the four-dimensional symptom questionnaire (4DSQ) and the hospital anxiety and depression scale (HADS). BMC Fam Pract. (2009) 10:58. doi: 10.1186/1471-2296-10-58
12. Cosco, TD, Doyle, F, Ward, M, and McGee, H. Latent structure of the hospital anxiety and depression scale: a 10-year systematic review. J Psychosom Res. (2012) 72:180–4. doi: 10.1016/j.jpsychores.2011.06.008
13. Kyu, HH, Bachman, VF, Alexander, LT, Mumford, JE, Afshin, A, Estep, K, et al. Physical activity and risk of breast cancer, colon cancer, diabetes, ischemic heart disease, and ischemic stroke events: systematic review and dose-response meta-analysis for the global burden of disease study 2013. BMJ. (2016) 354:i3857. doi: 10.1136/bmj.i3857
14. GBD 2013 Risk Factors Collaborators Forouzanfar, MH, Alexander, L, Anderson, HR, Bachman, VF, Biryukov, S, et al. Global, regional, and national comparative risk assessment of 79 behavioural, environmental and occupational, and metabolic risks or clusters of risks in 188 countries, 1990-2013: a systematic analysis for the global burden of disease study 2013. Lancet. (2015) 386:2287–323. doi: 10.1016/S0140-6736(15)00128-2
15. EMEA. (2022). bcl2fastq and bcl2fastq2 Conversion Software Downloads https://emea.support.illumina.com/sequencing/sequencing_software/bcl2fastq-conversion-software/downloads.html (Accessed November 21, 2022)
16. Babraham Bioinformatics (2022). FastQC a quality control tool for high throughput sequence data. https://www.bioinformatics.babraham.ac.uk/projects/fastqc/ (Accessed November 21, 2022)
17. Li, H, Handsaker, B, Wysoker, A, Fennell, T, Ruan, J, Homer, N, et al. The sequence alignment/map format and SAMtools. Bioinformatics. (2009) 25:2078–9. doi: 10.1093/bioinformatics/btp352
18. Pedersen, BS, and Quinlan, AR. Mosdepth: quick coverage calculation for genomes and exomes. Bioinformatics. (2018) 34:867–8. doi: 10.1093/bioinformatics/btx699
19. Kim, S, Scheffler, K, Halpern, AL, Bekritsky, MA, Noh, E, Källberg, M, et al. Strelka 2: fast and accurate calling of germline and somatic variants. Nat Methods. (2018) 15:591–4. doi: 10.1038/s41592-018-0051-x
20. Liu, W, Zhuang, Z, Wang, W, Huang, T, and Liu, Z. An improved genome-wide polygenic score model for predicting the risk of type 2 diabetes. Front Genet. (2021) 12:632385. doi: 10.3389/fgene.2021.632385
21. Jorgenson, E, Thai, KK, Hoffmann, TJ, Sakoda, LC, Kvale, MN, Banda, Y, et al. Genetic contributors to variation in alcohol consumption vary by race/ethnicity in a large multi-ethnic genome-wide association study. Mol Psychiatry. (2017) 22:1359–67. doi: 10.1038/mp.2017.101
22. Walters, RK, Polimanti, R, Johnson, EC, McClintick, JN, Adams, MJ, Adkins, AE, et al. Transancestral GWAS of alcohol dependence reveals common genetic underpinnings with psychiatric disorders. Nat Neurosci. (2018) 21:1656–69. doi: 10.1038/s41593-018-0275-1
23. Kessler, RC, McGonagle, KA, Zhao, S, Nelson, CB, Hughes, M, Eshleman, S, et al. Lifetime and 12-month prevalence of DSM-III-R psychiatric disorders in the United States. Results from the National Comorbidity Survey. Arch Gen Psychiatry. (1994) 51:8–19. doi: 10.1001/archpsyc.1994.03950010008002
24. Spiker, RL. Mental health and marital status. The Wiley Blackwell encyclopedia of health, illness, behavior, and society. Chichester, UK: John Wiley & Sons, Ltd. (2014). pp. 1485–1489.
25. Flint, AJ, Peasley-Miklus, C, Papademetriou, E, Meyers, BS, Mulsant, BH, Rothschild, AJ, et al. Effect of age on the frequency of anxiety disorders in major depression with psychotic features. Am J Geriatr Psychiatry. (2010) 18:404–12. doi: 10.1097/JGP.0b013e3181c294ac
26. Kalmbach, DA, Fang, Y, Arnedt, JT, Cochran, AL, Deldin, PJ, Kaplin, AI, et al. Effects of sleep, physical activity, and shift work on daily mood: a prospective Mobile monitoring study of medical interns. J Gen Intern Med. (2018) 33:914–20. doi: 10.1007/s11606-018-4373-2
27. Moylan, S, Jacka, FN, Pasco, JA, and Berk, M. How cigarette smoking may increase the risk of anxiety symptoms and anxiety disorders: a critical review of biological pathways. Brain Behav. (2013) 3:302–26. doi: 10.1002/brb3.137
28. Morissette, SB, Tull, MT, Gulliver, SB, Kamholz, BW, and Zimering, RT. Anxiety, anxiety disorders, tobacco use, and nicotine: a critical review of interrelationships. Psychol Bull. (2007) 133:245–72. doi: 10.1037/0033-2909.133.2.245
29. Gallagher, C, Radmall, Z, O’Gara, C, and Burke, T. Anxiety and depression among patients with alcohol dependence: co-morbid or substance-related problems? Ir J Psychol Med. (2018) 35:121–6. doi: 10.1017/ipm.2017.25
30. Kaplan, GB, Greenblatt, DJ, Ehrenberg, BL, Goddard, JE, Cotreau, MM, Harmatz, JS, et al. Dose-dependent pharmacokinetics and psychomotor effects of caffeine in humans. J Clin Pharmacol. (1997) 37:693–703. doi: 10.1002/j.1552-4604.1997.tb04356.x
31. Chavarria, J, Allan, NP, Boffa, JW, Albanese, BJ, Schmidt, NB, and Zvolensky, MJ. Decomposing the relationship between anxiety sensitivity and alcohol use. J Stud Alcohol Drugs. (2015) 76:957–61. doi: 10.15288/jsad.2015.76.957
32. Nehlig, A. Interindividual differences in caffeine metabolism and factors driving caffeine consumption. Pharmacol Rev. (2018) 70:384–411. doi: 10.1124/pr.117.014407
33. Southward, K, Rutherfurd-Markwick, K, Badenhorst, C, and Ali, A. The role of genetics in moderating the inter-individual differences in the Ergogenicity of caffeine. Nutrients. (2018) 10:10. doi: 10.3390/nu10101352
34. Childs, E, Hohoff, C, Deckert, J, Xu, K, Badner, J, and de Wit, H. Association between ADORA2A and DRD2 polymorphisms and caffeine-induced anxiety. Neuropsychopharmacology. (2008) 33:2791–800. doi: 10.1038/npp.2008.17
35. Vancampfort, D, Stubbs, B, and Koyanagi, A. Physical activity correlates in people with anxiety: data from 46 low-and middle-income countries. Gen Hosp Psychiatry. (2017) 49:26–31. doi: 10.1016/j.genhosppsych.2017.04.007
36. Bhat, S, Dao, DT, Terrillion, CE, Arad, M, Smith, RJ, Soldatov, NM, et al. CACNA1C (Cav 1.2) in the pathophysiology of psychiatric disease. Prog Neurobiol. (2012) 99:1–14. doi: 10.1016/j.pneurobio.2012.06.001
37. Ferreira, M, O’Donovan, M, Meng, Y, Jones, IR, Ruderfer, DM, Jones, L, et al. Collaborative genome-wide association analysis supports a role for ANK3 and CACNA1C in bipolar disorder. Nat Genet. (2008) 40:1056–8. doi: 10.1038/ng.209
38. Tesli, M, Skatun, KC, Ousdal, OT, Brown, AA, Thoresen, C, Agartz, I, et al. CACNA1C risk variant and amygdala activity in bipolar disorder, schizophrenia and healthy controls. PLoS One. (2013) 8:e56970. doi: 10.1371/journal.pone.0056970
39. Forster, G, Novick, AM, Scholl, J, and Watt, M. The role of the amygdala in anxiety disorders In: B Ferry (editor). The amygdala – a discrete multitasking manager Ch. 3. Rijeka: InTech (2012)
40. Etkin, A, and Wager, TD. Functional neuroimaging of anxiety: a meta-analysis of emotional processing in PTSD, social anxiety disorder, and specific phobia. Am J Psychiatry. (2007) 164:1476–88. doi: 10.1176/appi.ajp.2007.07030504
41. Smedler, E, Louhivuori, L, Romanov, RA, Masini, D, Dehnisch Ellström, I, Wang, C, et al. Disrupted gene expression perturbs spontaneous ca activity causing abnormal brain development and increased anxiety. Proc Natl Acad Sci U S A. (2022) 119:119. doi: 10.1073/pnas.2108768119
42. Wang, Y-Y, Zhang, H, Ma, T, Lu, Y, Xie, H-Y, Wang, W, et al. Piezo1 mediates neuron oxygen-glucose deprivation/reoxygenation injury via ca/calpain signaling. Biochem Biophys Res Commun. (2019) 513:147–53. doi: 10.1016/j.bbrc.2019.03.163
43. Liu, Y, Zhao, J, and Guo, W. Emotional roles of mono-Aminergic neurotransmitters in major depressive disorder and anxiety disorders. Front Psychol. (2018) 9:2201. doi: 10.3389/fpsyg.2018.02201
44. Martin, CA, Vorn, R, Schrieber, M, Lai, C, Yun, S, Kim, H-S, et al. Identification of DNA methylation changes that predict onset of post-traumatic stress disorder and depression following physical trauma. Front Neurosci. (2021) 15:347. doi: 10.3389/fnins.2021.738347
45. Yang, J, Jiang, Q, Yu, X, Xu, T, Wang, Y, Deng, J, et al. STK24 modulates excitatory synaptic transmission in epileptic hippocampal neurons. CNS Neurosci Ther. (2020) 26:851–61. doi: 10.1111/cns.13391
46. Scott, LJ, Muglia, P, Kong, XQ, Guan, W, Flickinger, M, Upmanyu, R, et al. Genome-wide association and meta-analysis of bipolar disorder in individuals of European ancestry. Proc Natl Acad Sci U S A. (2009) 106:7501–6. doi: 10.1073/pnas.0813386106
47. Lesch, K-P, Timmesfeld, N, Renner, TJ, Halperin, R, Röser, C, Nguyen, TT, et al. Molecular genetics of adult ADHD: converging evidence from genome-wide association and extended pedigree linkage studies. J Neural Transm. (2008) 115:1573–85. doi: 10.1007/s00702-008-0119-3
48. Smeland, OB, Frei, O, Kauppi, K, Hill, WD, Li, W, Wang, Y, et al. Identification of genetic loci jointly influencing schizophrenia risk and the cognitive traits of verbal-numerical reasoning, reaction time, and general cognitive function. JAMA Psychiat. (2017) 74:1065–75. doi: 10.1001/jamapsychiatry.2017.1986
49. Eszlari, N, Bagyura, Z, Millinghoffer, A, Nagy, T, Juhasz, G, Antal, P, et al. Catenin alpha 2 may be a biomarker or potential drug target in psychiatric disorders with perseverative negative thinking. Pharmaceuticals. (2021) 14:14. doi: 10.3390/ph14090850
50. Park, C, Falls, W, Finger, JH, Longo-Guess, CM, and Ackerman, SL. Deletion in Catna 2, encoding alpha N-catenin, causes cerebellar and hippocampal lamination defects and impaired startle modulation. Nat Genet. (2002) 31:279–84. doi: 10.1038/ng908
51. Fox, AS, Harris, RA, Rosso, LD, Raveendran, M, Kamboj, S, Kinnally, EL, et al. Infant inhibited temperament in primates predicts adult behavior, is heritable, and is associated with anxiety-relevant genetic variation. Mol Psychiatry. (2021) 26:6609–18. doi: 10.1038/s41380-021-01156-4
52. Jiménez-Jiménez, FJ, Alonso-Navarro, H, García-Martín, E, and Agúndez, JAG. Genetics of restless legs syndrome: an update. Sleep Med Rev. (2018) 39:108–21. doi: 10.1016/j.smrv.2017.08.002
53. Li, D, Zhao, H, Kranzler, HR, Li, MD, Jensen, KP, Zayats, T, et al. Genome-wide association study of copy number variations (CNVs) with opioid dependence. Neuropsychopharmacology. (2015) 40:1016–26. doi: 10.1038/npp.2014.290
54. Uhl, GR, and Martinez, MJ. PTPRD: neurobiology, genetics, and initial pharmacology of a pleiotropic contributor to brain phenotypes. Ann N Y Acad Sci. (2019) 1451:112–29. doi: 10.1111/nyas.14002
55. Chibnik, LB, White, CC, Mukherjee, S, Raj, T, Yu, L, Larson, EB, et al. Susceptibility to neurofibrillary tangles: role of the PTPRD locus and limited pleiotropy with other neuropathologies. Mol Psychiatry. (2018) 23:1521–9. doi: 10.1038/mp.2017.20
56. Liu, J, Li, S, Li, X, Li, W, Yang, Y, Guo, S, et al. Genome-wide association study followed by trans-ancestry meta-analysis identify 17 new risk loci for schizophrenia. BMC Med. (2021) 19:177. doi: 10.1186/s12916-021-02039-9
57. Trubetskoy, V, Pardiñas, AF, Qi, T, Panagiotaropoulou, G, Awasthi, S, Bigdeli, TB, et al. Mapping genomic loci implicates genes and synaptic biology in schizophrenia. Nature. (2022) 604:502–8. doi: 10.1038/s41586-022-04434-5
58. International Obsessive Compulsive Disorder Foundation Genetics Collaborative (IOCDF-GC) and OCD Collaborative Genetics Association Studies (OCGAS). Revealing the complex genetic architecture of obsessive-compulsive disorder using meta-analysis. Mol Psychiatry. (2018) 23:1181–8. doi: 10.1038/mp.2017.154
59. Ward, J, Strawbridge, RJ, Bailey, MES, Graham, N, Ferguson, A, Lyall, DM, et al. Genome-wide analysis in UK biobank identifies four loci associated with mood instability and genetic correlation with major depressive disorder, anxiety disorder and schizophrenia. Transl Psychiatry. (2017) 7:1264. doi: 10.1038/s41398-017-0012-7
60. Rasmussen, AH, Rasmussen, HB, and Silahtaroglu, A. The DLGAP family: neuronal expression, function and role in brain disorders. Mol Brain. (2017) 10:43. doi: 10.1186/s13041-017-0324-9
61. Cortese, BM, and Phan, KL. The role of glutamate in anxiety and related disorders. CNS Spectr. (2005) 10:820–30. doi: 10.1017/S1092852900010427
62. Wierońska, JM, Stachowicz, K, Nowak, G, and Pilc, A. The loss of glutamate-GABA harmony in anxiety disorders. Anxiety disorders. Intech. (2011). doi: 10.5772/19919
63. Ohnishi, T, Shirane, M, and Nakayama, KI. SRRM4-dependent neuron-specific alternative splicing of protrudin transcripts regulates neurite outgrowth. Sci Rep. (2017) 7:41130. doi: 10.1038/srep41130
64. Florentinus-Mefailoski, A, Bowden, P, Scheltens, P, Killestein, J, Teunissen, C, and Marshall, JG. The plasma peptides of Alzheimer’s disease. Clin Proteomics. (2021) 18:17. doi: 10.1186/s12014-021-09320-2
65. Lokapally, A, Neuhaus, H, Herfurth, J, and Hollemann, T. Interplay of TRIM2 E3 ubiquitin ligase and ALIX/ESCRT complex: control of developmental plasticity during early neurogenesis. Cells. (2020) 9:9. doi: 10.3390/cells9071734
66. O’Connor, TP, Cockburn, K, Wang, W, Tapia, L, Currie, E, and Bamji, SX. Semaphorin 5B mediates synapse elimination in hippocampal neurons. Neural Dev. (2009) 4:18. doi: 10.1186/1749-8104-4-18
67. Azim, E, Jabaudon, D, Fame, RM, and Macklis, JD. SOX6 controls dorsal progenitor identity and interneuron diversity during neocortical development. Nat Neurosci. (2009) 12:1238–47. doi: 10.1038/nn.2387
68. Danielson, E, Zhang, N, Metallo, J, Kaleka, K, Shin, SM, Gerges, N, et al. S-SCAM/MAGI-2 is an essential synaptic scaffolding molecule for the Glu A2-containing maintenance pool of AMPA receptors. J Neurosci. (2012) 32:6967–80. doi: 10.1523/JNEUROSCI.0025-12.2012
Keywords: anxiety, anxiety symptoms, genome-wide association study, HADS, polygenic risk score
Citation: Yakovchik AY, Tolynyova DV, Kashtanova DA, Sutulova ER, Ivanov MV, Mamchur AA, Erema VV, Matkava LR, Terekhov MV, Rumyantseva AM, Blinova OI, Akinshina AI, Mitrofanov SI, Yudin VS, Makarov VV, Keskinov AA, Kraevoy SA and Yudin SM (2024) Genetics of psycho-emotional well-being: genome-wide association study and polygenic risk score analysis. Front. Psychiatry. 14:1188427. doi: 10.3389/fpsyt.2023.1188427
Edited by:
Sergi Papiol, Ludwig Maximilian University of Munich, GermanyReviewed by:
Zhongshan Cheng, St. Jude Children’s Research Hospital, United StatesMaxim Ivanov, Moscow Institute of Physics and Technology, Russia
Copyright © 2024 Yakovchik, Tolynyova, Kashtanova, Sutulova, Ivanov, Mamchur, Erema, Matkava, Terekhov, Rumyantseva, Blinova, Akinshina, Mitrofanov, Yudin, Makarov, Keskinov, Kraevoy and Yudin. This is an open-access article distributed under the terms of the Creative Commons Attribution License (CC BY). The use, distribution or reproduction in other forums is permitted, provided the original author(s) and the copyright owner(s) are credited and that the original publication in this journal is cited, in accordance with accepted academic practice. No use, distribution or reproduction is permitted which does not comply with these terms.
*Correspondence: Anna Yurievna Yakovchik, eWFrb3ZjaGlrYW5uYTEzMDdAZ21haWwuY29t