- 1Department of Psychiatry, Chaohu Hospital of Anhui Medical University, Hefei, Anhui, China
- 2Anhui Psychiatric Center, Anhui Medical University, Hefei, Anhui, China
- 3Department of Psychiatry, Fuyang Third People’s Hospital, Fuyang, Anhui, China
- 4Department of Psychiatry, Bozhou People’s Hospital, Bozhou, Anhui, China
- 5Department of Psychiatry, The Second Affiliated Hospital of Bengbu Medical College, Bengbu, Anhui, China
- 6Department of Psychiatry, Ma’anshan Fourth People’s Hospital, Ma’anshan, Anhui, China
Background: Suicidal ideation is prevalent in major depressive disorder (MDD) and is closely related to empathy and alexithymia. While traditional approaches (e.g., regression models) focus on linear associations, network analysis provides unique advantages by mapping dynamic symptom interactions and identifying pivotal nodes that may drive suicidal risk. This study investigates these relationships through a network lens to reveal actionable intervention targets.
Methods: The study included 329 adolescents with MDD (ages 12–18). The Alexithymia Scale (TAS-20), Interpersonal Reactivity Index (IRI), and the Positive and Negative Suicide Ideation scale (PNSI) were used to assess alexithymia, empathy, and suicidal ideation levels, respectively. Network analysis was conducted to model the relationships between symptoms and calculate centrality and stability indices.
Results: Network analysis revealed strong stability with Emotional Identification Difficulty (DIF) and Personal Distress (PD) identified as the most influential core symptoms, exhibiting the strongest bridging roles between emotional dysfunction and suicidal ideation. DIF showed particularly robust connections to both PD and suicidal ideation, while comparative subgroup analyses indicated no significant differences in network patterns between first-episode and recurrent MDD patients, suggesting consistent symptom dynamics across illness stages.
Conclusion: By revealing DIF and PD as central therapeutic targets, this study demonstrates how network analysis can uncover intervention opportunities missed by traditional approaches. Clinically, targeting these nodes through emotion recognition training and distress tolerance interventions may disrupt the pathway to suicidality in adolescents with MDD.
1 Introduction
Major Depressive Disorder (MDD) is a severe mood disorder with an estimated global prevalence of approximately 229 million people, characterized by hallmark symptoms of persistent low mood and significant cognitive impairments (1). According to the Global Burden of Disease data, Depressive Disorder ranked 15th in disability-adjusted life years (DALYs) and 2nd in years lived with disability (YLDs), occupying the highest burden among mental disorders (1, 2). The reasons for this phenomenon, in addition to comorbidities between MDD and other diseases, include suicide as a significant contributing factor. The risk of suicide mortality for individuals with MDD was nearly 20 times higher compared to the general population (3). Previous studies emphasized that MDD were often accompanied by emotional dysregulation, which might have been an underlying mechanism contributing to the increased risk of suicide (4, 5). Emotion expression, identification, and empathy are crucial in initial emotional processing and play key roles in emotion regulation. When these abilities are impaired, it can lead to emotional dysregulation and may increase suicide risk in individuals with depression (6, 7).
Alexithymia is defined as a significant difficulty in recognizing, describing, and paying attention to one’s internal emotional states (8). The prevalence of alexithymia in MDD patients ranges from 40% to 70% (9, 10), significantly higher than the 12%–36% found in the general population (11–13), underscoring its significant impact on emotional regulation and its potential role in suicidal ideation. Research demonstrated that individuals with higher levels of alexithymia were more frequently associated with suicidal ideation, especially those with MDD (14, 15). Such as, an exploratory study had found that drug naïve patients with MDD were more likely to experience suicidal ideation compared to those without alexithymia (16). This phenomenon may be attributed to alexithymia leading to difficulties in emotion management, which in turn amplifies feelings of stress and helplessness in patients, intensifying thoughts of self-harm (14, 15). For example, mediation analysis indicated that alexithymia has a direct positive effect on suicidal ideation in patients with MDD and can additionally increase suicide risk indirectly by intensifying patients’ levels of stress and depression (6). Another correlational study also found that the severity of alexithymia in MDD patients was positively associated with feelings of helplessness and suicidal ideation (17). Moreover, difficulties in recognizing one’s own emotions may result in deficits in empathy toward others (18, 19). Recent research suggests that both alexithymia and empathy share common neural pathways, which could explain the interaction between these two constructs. For example, abnormalities in the connectivity between the right basolateral amygdala and the left precuneus, and the left-right asymmetry of the gyrus rectus (20, 21). Furthermore, affective temperament may also play a significant role in shaping an individual’s empathy, alexithymia, and their relationship with suicidal ideation (22). Studies have found that individuals with higher scores in cyclothymic, irritable, depressive, and anxious temperaments, or lower scores in hyperthymic temperament, tend to exhibit more severe emotional expression difficulties and empathy deficits, alongside higher levels of suicidal ideation and behavior (23–26).
In parallel with alexithymia, empathy defined as the ability to recognize and understand others’ emotions and respond appropriately is another key factor in emotional regulation and suicide risk (27). Unlike the internally emotion-oriented nature of alexithymia, empathy is externally emotion-oriented, involving a positive response to others’ emotions and comprising two components: cognitive empathy, the understanding of others’ emotional states, and emotional empathy, the resonance with others’ emotional states (28). Empathy in individuals with MDD may be impaired to varying degrees. Previous studies have indicated deficits in both cognitive and emotional empathy among MDD patients (29), while another study found a reduction solely in emotional empathy (30). Researches also suggested that although empathy decreased, depressed patients tended to experience others’ negative emotions due to a negativity bias in emotional processing (31, 32). This excessive empathetic experience of negative emotions may contribute to personal distress and emotional exhaustion, potentially fostering the development of suicidal ideation (31, 33). However, on the relationship between empathy and suicide in patients with MDD were currently limited. A cross-sectional survey of adult patients with depression who experienced lower cognitive empathy were associated with a higher risk of suicide (7). In contrast, no correlation was found between empathy and suicidal behavior in individuals with depression who attempted suicide (34). Additionally, research on empathy and alexithymia found that depressed patients with high levels of alexithymia scored significantly lower in emotional empathy and perspective-taking, and exhibited more personal distress. Nevertheless, the sample size was small, with only 29 participants, limiting its representativeness (35).
Overall, previous studies have primarily focused on the impact of either alexithymia or empathy deficits on suicidal ideation individually, with limited investigation into the specific connections and key factors among alexithymia, empathy, and suicidal ideation within adolescent patients with MDD. Network analysis in psychopathology, as a novel analytical approach, replaces traditional conceptualizations of mental disorders by focusing on the direct interactions between symptoms (36). This method visualizes and quantifies these connections, enabling researchers to identify central nodes or critical links that play a pivotal role in the overall network. Compared to traditional regression analysis, network analysis more effectively captures complex nonlinear relationships and interactions among multiple variables (37).
This study aims to use network analysis to elucidate the role of alexithymia and empathy disorders in suicidal ideation among patients with depression. We hypothesize that: (1) suicidal ideation is associated with both empathy and alexithymia, with a positive correlation between suicidal ideation and the personal distress dimension of empathy, as well as the difficulty in emotion recognition dimension of alexithymia; (2) there is a strong association between alexithymia and empathy; (3) the network structure will differ between first episode and non-first episode depression subgroups.
2 Method
2.1 Participants and procedure
This cross-sectional study was conducted from January to August 2021 in three general hospitals and four psychiatric hospitals in Anhui Province, China. Adolescent patients were eligible to participate if they met the following criteria: 1) diagnosed with major depressive disorder (MDD) based on a structured clinical interview according to DSM-5 guidelines; 2) aged between 12 and 18 years and of Han Chinese ethnicity; and 3) able to understand the questionnaires. Individuals with other psychiatric or neurological disorders or intellectual disabilities were excluded from the study. All enrolled patients were referred for inclusion after evaluation by trained professional psychiatrists.
Participants and their guardians gave written informed consent after being informed about the study’s objectives and procedures. The research was approved by the Medical Ethics Committee of Chaohu Hospital, Anhui Medical University (approval number: 202009-kyxm-04).
2.2 Materials and methods
Participants’ demographic characteristics were collected, including gender(male or female), age, body mass index (BMI), educational level and first-episode (yes or no).
2.2.1 The Toronto alexithymia scale-20
The TAS-20 is a widely used scale for assessing alexithymia, consisting of 20 items rated on a 5-point Likert scale, ranging from 1 (strongly disagree) to 5 (strongly agree) (38). The scale measures three key components: Difficulty Identifying Feelings (DIF), Difficulty Describing Feelings (DDF), and Externally Oriented Thinking (EOT). Higher TAS-20 scores indicate more pronounced alexithymic traits. Studies have shown that the TAS-20 demonstrates good reliability in Chinese populations, with a Cronbach’s alpha of 0.79 (39). In this study, the scale showed strong reliability, with a Cronbach’s alpha of 0.744.
2.2.2 Interpersonal reactivity index
IRI is a self-report tool used to assess an individual’s empathy and emotional responsiveness, consisting of 28 items rated on a five-point Likert scale, ranging from 1 to 5 points (28). The scale is divided into four dimensions: Fantasy (FS), Empathic Concern (EC), Personal Distress (PD), and Perspective Taking (PT). The total score ranges from 28 to 140 points. Higher scores typically indicate a stronger capacity for empathy and emotional sensitivity, suggesting that individuals are better able to understand and care for the emotional states of others. The IRI scale demonstrated good internal consistency in the Chinese population, with a Cronbach’s alpha of 0.70 (40). In this study, the scale demonstrated solid reliability, with a Cronbach’s alpha of 0.769.
2.2.3 Positive and negative suicide ideation
The Positive and Negative Suicide Ideation scale (PNSI) assesses suicidal ideation through 14 items scored on a five-point Likert scale from “strongly disagree” (1) to “strongly agree” (5) (41). It consists of two dimensions: positive suicidal ideation and negative suicidal ideation, with a total score ranging from 14 to 70 points. Higher scores indicate stronger positive and negative suicidal ideations, reflecting a higher suicide risk and complex psychological state. The PNSI scale has demonstrated good internal consistency in the Chinese population, with a Cronbach’s alpha of 0.88 (42), and a strong reliability in our sample (Cronbach’s alpha = 0.938). The total PANSI score was used as an indicator of empathy.
2.3 Statistical analysis
2.3.1 Network estimation
All analyses were conducted using RStudio version 4.4.0. The network structure was estimated using the Gaussian Graphical Model (GGM) with the Extended Bayesian Information Criterion Lasso (EBICglasso) regularization method, which serves to reduce redundant edges and increase network sparsity. The bootnet package (version 1.6.0) (43) was used to construct the network, applying Spearman correlation to calculate the relationships between variables, and the network was visualized using the qgraph package (version 1.9.8) (44), where blue edges indicate positive associations and red edges indicate negative associations. Additionally, the mgm package (version 1.2-14) was employed to perform node-wise predictions, with green circles representing the magnitude of the predicted values (45).
2.3.2 Network centrality
Network centrality was assessed using the qgraph package (version 1.9.8) (44). Indicators for identifying prominent nodes in the overall network included betweenness, closeness, strength, and expected influence (EI). Given that both positive and negative edges are present in the network analyzed in this study, EI serves as a more suitable measure. Additionally, bridge centrality (bridge expected influence, BEI) was assessed using the networktools package (version 1.5.2) to highlight nodes that act as bridges between groups (46). This analysis aids in identifying significant connections between different symptom groups.
2.3.3 Network accuracy and stability
The bootstrapping method was utilized to assess the accuracy and stability of the network, involving 1000 iterations with the bootnet package (version 1.6.0) (43). To evaluate the stability of network centrality, we used the case-dropping subset bootstrap approach, which systematically drops individual cases (i.e., participants) from the dataset to test whether centrality measures (e.g., EI, BEI) remain stable across different subsets of the data. This approach provides insight into the robustness of central nodes and edges in the network. The accuracy of the edges was assessed by calculating the 95% confidence interval (CI) of the bootstrapped edge weights, ensuring that we account for the variability in edge estimates across the resampling iterations. Finally, the correlation stability coefficient (CS coefficient) was calculated to assess the stability of the network’s centrality indices. A CS coefficient value above 0.5 indicates that the centrality indices are stable, meaning they are unlikely to change significantly with the exclusion of a small portion of the sample (43).
2.3.4 Network comparison test
To explore differences in network structures among patients at various stages of illness, this study compared first episode (FE) and non-first episode (NFE) subgroups using the Network Comparison Test (NCT) (version 2.2.2) (47). Primary indicators comprised global strength to assess overall network strength, structure invariance for consistency across phases, and edge weights to identify differences in symptom connections.
3 Result
3.1 Descriptive statistics
As shown in Table 1, a total of 329 participants were included in this study, with an average age of 15.33 years. Among them, 241 were female, and 43.8% were junior high school students. There were 193 first-episode patients (58.7%) and 136 non-first-episode patients (41.3%). The mean total score for suicide ideation, IRI, and TAS were 47.79, 94.21, and 67.90, respectively.
3.2 Edge weights and centrality
Figure 1A showed the overall structure of the network analysis. Overall, nodes representing various dimensions within each scale showed positive correlations, indicating strong “within-scale” edges. The network included a total of 28 edges, with 19 being non-zero edges (67.9%), indicating a densely connected structure. The highest edge weights between nodes were found between DIF and DDF (r = 0.381), PT and EC (r = 0.352), and PD and DIF (r = 0.300), while the strongest connections between scales were observed between PD and DIF, and DIF and PNSI (0.258). Additionally, negative correlations were found between EOT, EC, and PNSI. The size of edge weights and the significance of differences between them were shown in Supplementary Table S1, Supplementary Figure S1.
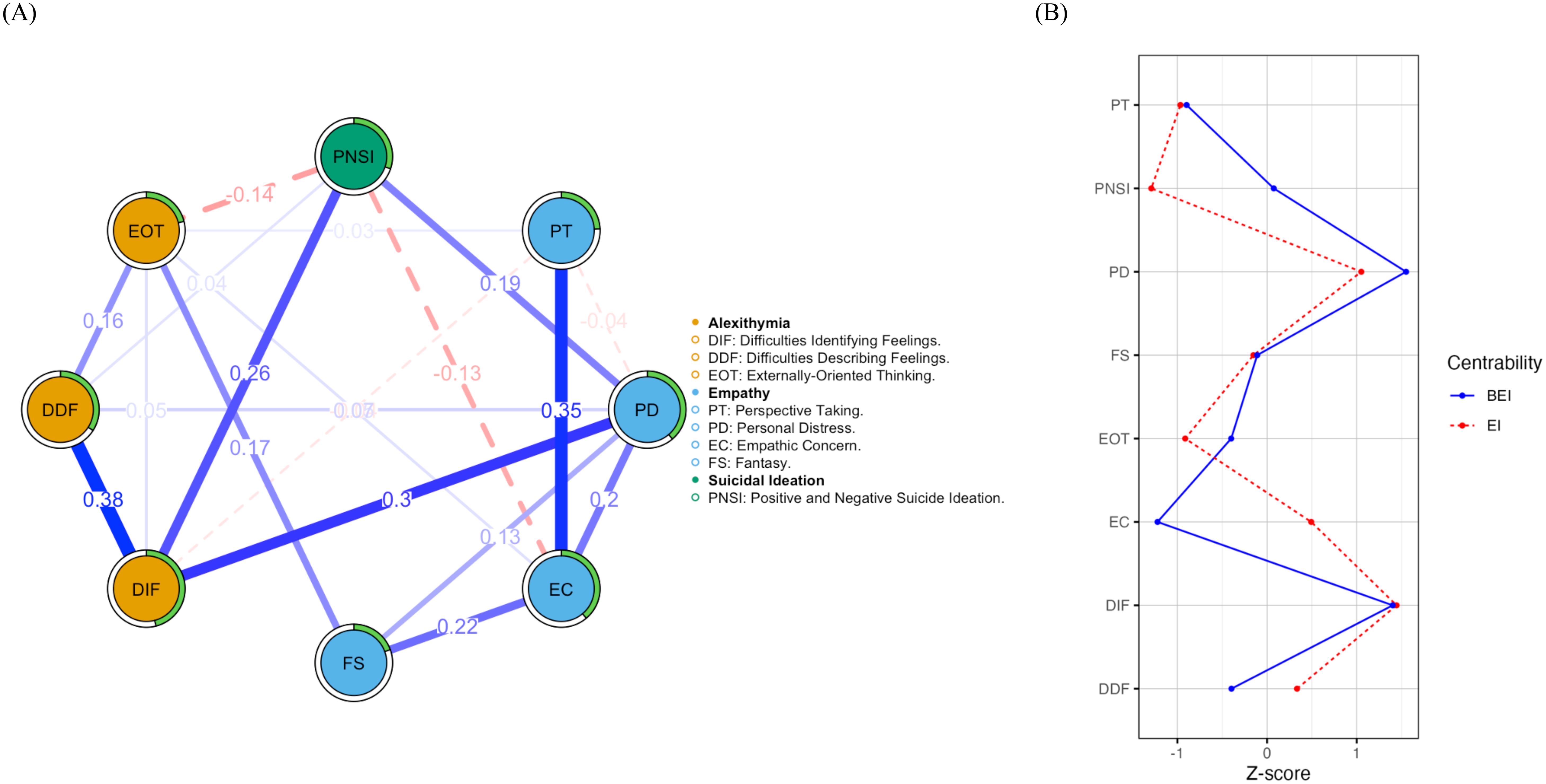
Figure 1. (A) Regularized partial correlation network for MDD patients (n = 329). Blue edges represent positive correlations, and red edges represent negative correlations. The green ring around each node indicates its predictability. Thicker edges represent stronger partial correlations. (B) Network centrality index. Centrality is expressed as a standardized z-score, with the red line representing the EI index and the blue line representing the BEI index. Nodes with a high EI index have significant overall influence, affecting many other nodes, while nodes with a high BEI index act as bridges, connecting different parts of the network and facilitating communication between clusters. EI, Expected Influence; BEI, Bridge Expected Influence.
Table 2, Figure 1B presented the specific values and visualization results of network centrality metrics EI and BEI for different nodes. The strongest EI node was DIF (0.95), followed by PD (0.84). For BEI, the strongest nodes were PD (1.55) and DIF (1.40). Supplementary Figures S3, S4 showed that the bootstrap difference test results indicated statistically significant differences between DIF, PD, and the remaining nodes. Additionally, DIF (0.39) and PD (0.46) had the highest predictability values. The results suggested that DIF and PD occupied central positions in the network, with the greatest influence on the other nodes in the network and the highest predictability by the remaining nodes.
3.3 Network stability and group comparison
Nonparametric bootstrap analysis of edge accuracy indicated that edge precision was acceptable, as shown in Figure 2A. The narrow gray areas represent the confidence interval (CI) range, with smaller CIs indicating more accurate edge estimates. The near overlap of the brown and black lines further suggests high consistency between the bootstrap edge means and the sample edge weights. Additionally, stability analysis of EI and BEI using case-dropping subset bootstrap showed that, although the correlation between centrality indices and those of the full sample slightly decreased as subset size reduced, overall stability remained high (Figure 2B). Finally, the CS coefficients confirmed this stability, with EI and BEI yielding CS coefficients of 0.596 and 0.672, respectively.
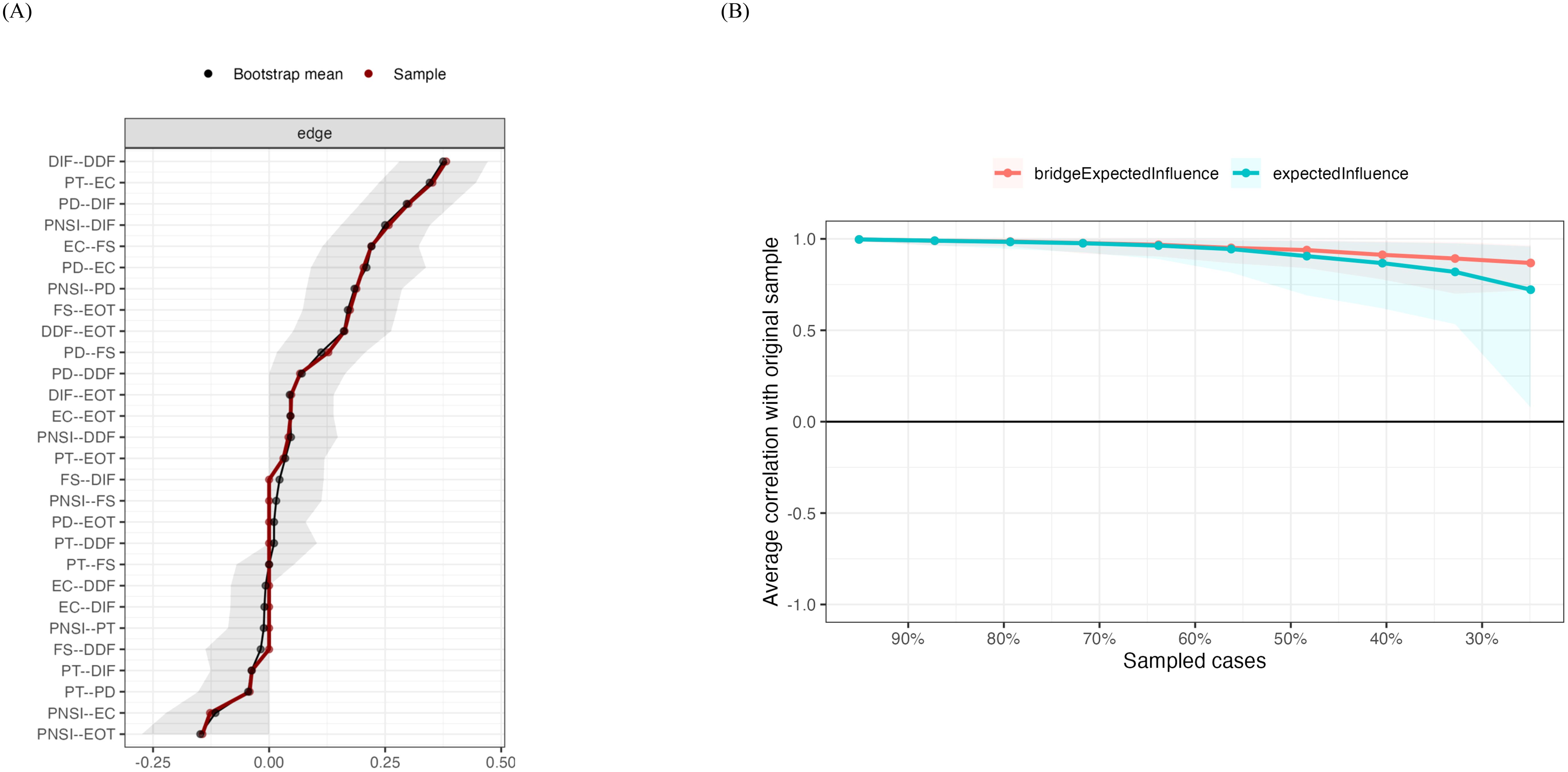
Figure 2. (A) Bootstrap Stability Test of Edge Weights. (B) Bootstrap Stability Test of Network Bridge and Expected Influence. DIF, Difficulty Identifying Feelings; DDF, Difficulty Describing Feelings; EOT, Externally Oriented Thinking; PD, Personal Distress; PT, Perspective Taking; EC, Empathic Concern; FS, Fantasy; PNSI, Positive and Negative Suicide Ideation.
The network analysis visualization and centrality indices for the NFE and FE subgroups are shown in Supplementary Figures S4 and S5. Comparisons of network estimates between subgroups revealed no statistical differences in global strength invariance (S = 0.52, p = 0.65), structural invariance (M = 0.19, p = 0.75), or edge weights.
4 Discussion
To our knowledge, this study was the first to apply network analysis to examine the complex associations among alexithymia, empathy, and suicidal ideation in adolescent patients with MDD. The primary findings are as follows. First, the network identified central nodes for alexithymia and empathy, particularly DIF and PD. Second, the strongest edge weight between symptom clusters was found between DIF and PD. Finally, network comparison analysis showed no significant structural differences between first-episode and recurrent MDD patients, suggesting a stable association structure among alexithymia, empathy deficits, and suicidal ideation across illness stages.
This study identified DIF as the strongest EI indicator and the most predictable node in the integrated network analysis of alexithymia, empathy, and suicidal ideation, underscoring its role as a core symptom of alexithymia with substantial direct impact on other nodes. According to emotion regulation theories, accurately identifying one’s emotions is foundational for selecting effective regulation strategies (48). Individuals with alexithymia were often positively associated with emotional dysregulation and tend to employ fewer adaptive emotion regulation strategies (e.g., cognitive reappraisal, problem-solving, and seeking social support) and more maladaptive strategies (e.g., emotional suppression, behavioral withdrawal, and neglect) (49). Similar studies have also found that DIF was directly positively correlated with anxiety and stress, and that it indirectly promoted the development of anxiety and depression through the suppression of reappraisal (50). This deficiency increases susceptibility to negative thoughts and actions, such as suicidal ideation and behaviors, under emotional stress, aligning with our finding of a positive association between DIF and suicidal ideation (6).The direct link between DIF and suicidal ideation in MDD patients has also been confirmed in previous studies, which have found a weaker or non-existent relationship between DDF and suicidal ideation. This phenomenon further supports the critical role of DIF within the overall network (6, 17). Currently, there is a lack of longitudinal studies examining the impact of treatment for alexithymia in patients with MDD on empathy and suicidal ideation. Previous research has shown that deep brain stimulation, art therapy, and clay art therapy can reduce the severity of emotional expression disorders in patients with schizophrenia, depression, and Parkinson’s disease (51–53). However, the effects on the depressive population and other nodes within the network still require further validation.
Furthermore, we found a significant positive correlation between DIF and PD, reflecting a potential link between emotional processing deficits and self-related emotional responses in empathy. Recent studies have revealed that, beyond the traditional definition of alexithymia, which focuses on internal emotional cognition deficits, individuals with this condition also exhibit impairments in recognizing and recalling external emotional information (54–56). Through facial expression recognition and recognition memory tests of real social videos, individuals showed a significant decrease in the accuracy of immediate recognition and recall of emotional expressions (relative to neutral expressions) in social activities, which was significantly negatively correlated with DIF (54). The foundation of empathy lies in the accurate perception of others’ emotions, so it is reasonable to assume that alexithymia is related to impaired empathy. In fact, this connection has been demonstrated in both the general population and individuals with mental disorders, particularly in autism (35, 57, 58). For example, studies on individuals with autism have shown that alexithymia impairs empathy, primarily manifesting as a decrease in emotional empathy and an increase in PD (57). Similarly, a study on a small sample of individuals with MDD found that, compared to the low alexithymia group, the high alexithymia group showed a decline in cognitive empathy and higher levels of PD (35). The strong correlation between alexithymia and empathy could be due to overlapping neural mechanisms, particularly in brain areas like anterior insula and the anterior cingulate cortex, which play pivotal roles in the shared processing of self and others’ emotions (59–61).
More importantly, PD serves as the variable with the highest cross-symptom group node (BEI) in the network, demonstrating a significant impact on suicidal ideation. Most studies have shown that patients with MDD often experience emotional exhaustion, primarily caused by specific negative cognitive biases, characterized by a reduced responsiveness to positive emotions and heightened sensitivity to negative emotions (31, 32, 62). Compared to healthy controls, individuals with MDD show stronger alter centric biases, being more influenced by others’ negative emotions, related to emotional contagion and increased PD (62). This empathy impairment may be related to deficits in executive function among patients with MDD. A meta-analysis revealed a strong association between empathy and executive function, with inhibitory control serving as a predictor of emotional empathy (63). Deficits in inhibitory control among MDD patients suggest that when empathizing with others, decreased emotional empathy was accompanied by reduced ability to regulate emotional contagion, leading to increased personal distress (63, 64). Additionally, studies suggest that disruptions in the excitation/inhibition balance of the anterior cingulate cortex and medial prefrontal cortex underlie the empathy impairments observed in MDD (65). The distress resulting from empathy impairment was thought to be linked to suicide (34, 66), consistent with our findings that PD was positively associated with suicidal ideation. PD, as a potential mediating node between alexithymia and suicidal ideation, underscores the importance of considering the entire network in interventions, rather than focusing solely on the effects of individual nodes such as alexithymia or empathy. This highlights the importance of personalized interventions in adolescent mental health care, while systemic mental health care can effectively manage adolescent patients and ensure continuity of care (67, 68).
Notable, the group analysis in this study did not reveal significant differences in network structure between FE and NFE patients, suggesting that the overall network model may be stable across different stages of depression, unaffected by the progression of the illness. This finding is of significant importance as it supports the idea of implementing unified interventions for adolescent depression patients, particularly those focusing on improving emotional recognition and regulation abilities, which may have positive effects regardless of the stage of illness. Due to the higher prevalence of depression in females, the proportion of females in this study was significantly higher than that of males (69). Studies have found that there are sex differences in the emotion regulation strategies used; for example, females may be more likely to use mindfulness, reflection, or emotional expression to cope with negative emotions, while males may tend to use suppression or avoidance strategies (70, 71). Additionally, female patients with depression exhibit significantly higher levels of emotional empathy and suicidal ideation compared to males (72, 73). Therefore, the underlying mechanisms linking these factors may differ by sex suggesting that the conclusions of this study may be more applicable to female populations with depression.
The limitations of this study are as follows: First, the sample was limited to adolescent depression patients, which may restrict the generalizability of the findings to other populations or age groups. Second, due to the cross-sectional design, we were unable to explore causal relationships. While network analysis revealed associations between variables, the directionality and causality of these relationships remain unclear. Longitudinal studies would be beneficial in uncovering the causal pathways between the variables and providing a more in-depth understanding of these relationships. Third, the network was constructed using self-reported measures, which are subject to recall bias and social desirability effects. These biases may compromise the accuracy of participants’ responses, potentially influencing the findings. Future studies could address this limitation by integrating biological measures or behavioral observations to complement the self-report data. Finally, the underrepresentation of male participants may have limited the ability to examine sex differences in alexithymia and suicidal ideation fully, suggesting the need for future studies with a larger male sample to investigate these potential differences.
5 Conclusion
This study, employing network analysis, underscores the intricate relationships among alexithymia, empathy, and suicidal ideation in adolescents with MDD. DIF was found to be closely associated with PD and suicidal ideation, emphasizing its pivotal role in emotional processing and interpersonal distress. Additionally, PD, as the strongest bridging node, serve as a mediator between alexithymia and suicidal ideation. The consistent network structure across FE and NFE patients with MDD suggests the stability of these associations across different stages of the illness. These findings highlight the importance of targeted interventions aimed at key points, such as improving emotional recognition, to alleviate emotional distress and reduce suicidal ideation. Moreover, they offer valuable directions for future longitudinal studies to investigate the causal pathways between these variables. Such research would allow for a deeper exploration of how these factors evolve and interact over time, thus enhancing our understanding of the temporal dynamics and potential causal relationships in MDD.
Data availability statement
The raw data supporting the conclusions of this article will be made available by the authors, without undue reservation.
Ethics statement
The studies involving humans were approved by The Medical Ethics Committee of Chaohu Hospital, Anhui Medical University (approval number: 202009-kyxm-04). The studies were conducted in accordance with the local legislation and institutional requirements. Written informed consent for participation in this study was provided by the participants’ legal guardians/next of kin.
Author contributions
YS: Conceptualization, Data curation, Formal Analysis, Investigation, Methodology, Resources, Software, Supervision, Validation, Visualization, Writing – original draft, Writing – review & editing. QZ: Conceptualization, Data curation, Formal Analysis, Investigation, Methodology, Software, Writing – original draft, Writing – review & editing. SW: Conceptualization, Data curation, Formal Analysis, Investigation, Methodology, Software, Writing – original draft, Writing – review & editing. LZ: Conceptualization, Data curation, Investigation, Methodology, Software, Writing – review & editing. ZL: Conceptualization, Data curation, Investigation, Methodology, Software, Writing – review & editing. JW: Conceptualization, Data curation, Investigation, Methodology, Software, Writing – review & editing. XL: Conceptualization, Data curation, Investigation, Methodology, Software, Writing – review & editing. XW: Conceptualization, Data curation, Investigation, Methodology, Software, Writing – review & editing. HL: Conceptualization, Data curation, Formal Analysis, Funding acquisition, Investigation, Methodology, Project administration, Resources, Software, Supervision, Validation, Visualization, Writing – review & editing.
Funding
The author(s) declare that financial support was received for the research and/or publication of this article. This study was supported by the National Clinical Key Specialty Project Foundation (CN); and Anhui Medical University Clinical Science Foundation; and Research Fund of Anhui Institute of translational medicine (2022zhyx-B01).
Conflict of interest
The authors declare that the research was conducted in the absence of any commercial or financial relationships that could be construed as a potential conflict of interest.
The reviewer KH declared a shared parent affiliation with the authors YS, QZ, SW, LZ, HL to the handling editor at the time of review.
Generative AI statement
The author(s) declare that no Generative AI was used in the creation of this manuscript.
Publisher’s note
All claims expressed in this article are solely those of the authors and do not necessarily represent those of their affiliated organizations, or those of the publisher, the editors and the reviewers. Any product that may be evaluated in this article, or claim that may be made by its manufacturer, is not guaranteed or endorsed by the publisher.
Supplementary material
The Supplementary Material for this article can be found online at: https://www.frontiersin.org/articles/10.3389/fpsyt.2025.1543651/full#supplementary-material
References
1. Ferrari AJ, Santomauro DF, Aali A, Abate YH, Abbafati C, Abbastabar H, et al. Global incidence, prevalence, years lived with disability (YLDs), disability-adjusted life-years (DALYs), and healthy life expectancy (HALE) for 371 diseases and injuries in 204 countries and territories and 811 subnational locations, 1990–2021: a systematic analysis for the Global Burden of Disease Study 2021. Lancet. (2024) 403:2133–61. doi: 10.1016/S0140-6736(24)00757-8
2. GBD 2019 Mental Disorders Collaborators. Global, regional, and national burden of 12 mental disorders in 204 countries and territories, 1990-2019: A systematic analysis for the global burden of disease study 2019. Lancet Psychiatry. (2022) 9:137–50. doi: 10.1016/S2215-0366(21)00395-3
3. Chesney E, Goodwin GM, Fazel S. Risks of all-cause and suicide mortality in mental disorders: a meta-review. World Psychiatry. (2014) 13:153–60. doi: 10.1002/wps.2012810.1002/w
4. LeMoult J, Gotlib IH. Depression: A cognitive perspective. Clin Psychol Rev. (2019) 69:51–66. doi: 10.1016/j.cpr.2018.06.008
5. Kneeland ET, Cunningham C, Lattuada I, Cwalina M, Shanahan M. How I think about how I feel: Personal beliefs about emotion prospectively predict suicide-related outcomes and depression symptoms. J Psychiatr Res. (2024) 179:330–40. doi: 10.1016/j.jpsychires.2024.09.03310.1016/j.jpsychires.2024
6. Li D, Gao Y, Li S, Zhou C, Wang Y, Li M, et al. Perceived stress and severity of depression mediate the association between alexithymia and suicidal ideation in patients with major depressive disorder. Heliyon. (2023) 9:e21986. doi: 10.1016/j.heliyon.2023.e21986
7. Ekinci O, Ekinci A. Relationship between empathic responding and its clinical characteristics in patients with major depressive disorder. Dusunen Adam J Psychiatry Neurological Sci. (2016) 29:145–54. doi: 10.5350/DAJPN2016290206
8. Jc N. Alexithymia: A view of the psychosomatic process. Modern Trends psychosomatic Med. (1976) 3:430–9.
9. Honkalampi K, Hintikka J, Laukkanen E, Viinamäki JLH. Alexithymia and depression: A prospective study of patients with major depressive disorder. Psychosomatics. (2001) 42:229–34. doi: 10.1176/appi.psy.42.3.229
10. Wang X, Li X, Guo C, Hu Y, Xia L, Geng F, et al. Prevalence and correlates of alexithymia and its relationship with life events in Chinese adolescents with depression during the COVID-19 pandemic. Front Psychiatry. (2021) 12:774952. doi: 10.3389/fpsyt.2021.774952
11. Sirri L. Prevalence and correlates of alexithymia in the general population. Mediterranean J Clin Psychol. (2023) 11:156–6.
12. McGillivray L, Becerra R, Harms C. Prevalence and demographic correlates of alexithymia: A comparison between Australian psychiatric and community samples. J Clin Psychol. (2017) 73:76–87. doi: 10.1002/jclp.22314
13. Ng CSM, Chan VCW. Prevalence and associated factors of alexithymia among Chinese adolescents in Hong Kong. Psychiatry Res. (2020) 290:113126. doi: 10.1016/j.psychres.2020.11312610.1016/j.psychres.20
14. Mohamed BES, Ahmed MGAE. Emotional intelligence, alexithymia and suicidal ideation among depressive patients. Arch Psychiatr Nurs. (2022) 37:33–8. doi: 10.1016/j.apnu.2021.12.00210.1016/j.apnu.2021.12.0
15. Iskric A, Ceniti AK, Bergmans Y, McInerney S, Rizvi SJ. Alexithymia and self-harm: A review of nonsuicidal self-injury, suicidal ideation, and suicide attempts. Psychiatry Res. (2020) 288:112920. doi: 10.1016/j.psychres.2020.112920
16. De Berardis D, Fornaro M, Valchera A, Rapini G, Di Natale S, De Lauretis I, et al. Alexithymia, resilience, somatic sensations and their relationships with suicide ideation in drug naïve patients with first-episode major depression: An exploratory study in the “real world” everyday clinical practice. Early Interv Psychia. (2020) 14:336–42. doi: 10.1111/eip.12863
17. Izci F, Zincir S, Bozkurt Zincir S, Bilici R, Gica S, Iris Koc MS, et al. Suicide attempt, suicidal ideation and hopelessness levels in major depressive patients with and without alexithymia. Dusunen Adam J Psychiatry Neurological Sci. (2015) 28:27. doi: 10.5350/DAJPN2015280103
18. Ursoniu S, Bredicean A-C, Serban CL, Rivis I, Bucur A, Papava I, et al. The interconnection between social media addiction, alexithymia and empathy in medical students. Front Psychiatry. (2024) 15:1467246. doi: 10.3389/fpsyt.2024.1467246
19. Burghart M, Sahm AHJ, Schmidt S, Bulla J, Mier D. Understanding empathy deficits and emotion dysregulation in psychopathy: The mediating role of alexithymia. PloS One. (2024) 19:e0301085. doi: 10.1371/journal.pone.0301085
20. Li W, Lou W, Zhang W, Tong RK-Y, Jin R, Peng W. Gyrus rectus asymmetry predicts trait alexithymia, cognitive empathy, and social function in neurotypical adults. Cereb Cortex. (2023) 33:1941–54. doi: 10.1093/cercor/bhac184
21. Li X, Peng C, Qin F, Luo Q, Ren Z, Wang X, et al. Basolateral amygdala functional connectivity in alexithymia: Linking interoceptive sensibility and cognitive empathy. Neuroscience. (2024) 539:12–20. doi: 10.1016/j.neuroscience.2023.12.014
22. Favaretto E, Bedani F, Brancati GE, De Berardis D, Giovannini S, Scarcella L, et al. Synthesizing 30 years of clinical experience and scientific insight on affective temperaments in psychiatric disorders: State of the art. J Affect Disord. (2024) 362:406–15. doi: 10.1016/j.jad.2024.07.011
23. Tanaka K, Ikeuchi S, Teranishi K, Oe M, Morikawa Y, Konya C. Temperament and professional quality of life among Japanese nurses. Nurs Open. (2020) 7:700–10. doi: 10.1002/nop2.441
24. Vecchiotti R, Mansueto G, Marziali RA, Marconi M, Valchera A, Cosci F. The mediating role of alexithymia in the relationship between affective temperament and craving: Cross-sectional study conducted in a sample of bipolar and alcohol use disorder patients. J Affect Disord. (2023) 325:110–8. doi: 10.1016/j.jad.2023.01.021
25. Luciano M, Sampogna G, Della Rocca B, Simonetti A, De Fazio P, Di Nicola M, et al. The impact of affective temperaments on suicidal ideation and behaviors: Results from an observational multicentric study on patients with mood disorders. Brain Sci. (2023) 13:117. doi: 10.3390/brainsci13010117
26. Madeira L, Moreira Pereira J, Figueira ML. Exploring relational and moral features in medical students. Int J Med Educ. (2021) 12:76–83. doi: 10.5116/ijme.606a.f16c
27. De Vignemont F, Singer T. The empathic brain: how, when and why? Trends Cognit Sci. (2006) 10:435–41. doi: 10.1016/j.tics.2006.08.008
28. Davis MH. Measuring individual differences in empathy: Evidence for a multidimensional approach. J Pers Soc Psychol. (1983) 44:113–26. doi: 10.1037/0022-3514.44.1.113
29. Cusi AM, MacQueen GM, Spreng RN, McKinnon MC. Altered empathic responding in major depressive disorder: Relation to symptom severity, illness burden, and psychosocial outcome. Psychiatry Res. (2011) 188:231–6. doi: 10.1016/j.psychres.2011.04.013
30. Kilian HM, Schiller B, Schläpfer TE, Heinrichs M. Impaired socio-affective, but intact socio-cognitive skills in patients with treatment-resistant, recurrent depression. J Psychiatr Res. (2022) 153:206–12. doi: 10.1016/j.jpsychires.2022.07.025
31. Hu S, Mo D, Guo P, Zheng H, Jiang X, Zhong H. Correlation between suicidal ideation and emotional memory in adolescents with depressive disorder. Sci Rep. (2022) 12:5470. doi: 10.1038/s41598-022-09459-4
32. Li L, Li R, Shen F, Wang X, Zou T, Deng C, et al. Negative bias effects during audiovisual emotional processing in major depression disorder. Hum Brain Mapp. (2022) 43:1449–62. doi: 10.1002/hbm.25735
33. Müller J, Herpertz J, Taylor J, Suslow T, Lane RD, Donges U-S. Emotional awareness for self and others and empathic abilities in clinical depression during acute illness and recovery. BMC Psychiatry. (2024) 24:488. doi: 10.1186/s12888-024-05877-y
34. Senna S, Schwab B, Melo HM, Diaz AP, Schwarzbold ML. Social cognition and suicide-related behaviors in depression: A cross-sectional, exploratory study. Braz J Psychiatry. (2022) 44:639–43. doi: 10.47626/1516-4446-2021-2407
35. Banzhaf C, Hoffmann F, Kanske P, Fan Y, Walter H, Spengler S, et al. Interacting and dissociable effects of alexithymia and depression on empathy. Psychiatry Res. (2018) 270:631–8. doi: 10.1016/j.psychres.2018.10.045
36. Borsboom D. A network theory of mental disorders. World Psychiatry. (2017) 16:5–13. doi: 10.1002/wps.20375
37. Robinaugh DJ, Hoekstra RHA, Toner ER, Borsboom D. The network approach to psychopathology: a review of the literature 2008–2018 and an agenda for future research. Psychol Med. (2020) 50:353–66. doi: 10.1017/S0033291719003404
38. Bagby RM, Parker JDA, Taylor GJ. The twenty-item Toronto Alexithymia scale—I. Item selection and cross-validation of the factor structure. J Psychosom Res. (1994) 38:23–32. doi: 10.1016/0022-3999(94)90005-1
39. Zhu X, Yi J, Yao S, Ryder AG, Taylor GJ, Bagby RM. Cross-cultural validation of a Chinese translation of the 20-item Toronto Alexithymia Scale. Compr Psychiatry. (2007) 48:489–96. doi: 10.1016/j.comppsych.2007.04.007
40. Liu F, Dong Y, Yuan Y, Jiang Y. Relationship between empathy and interpersonal distress of Chinese left-behind children: the role of emotion regulation and gender. Psychol Res Behav Manag. (2023) 16:3717–26. doi: 10.2147/PRBM.S406801
41. Osman A, Gutierrez PM, Kopper BA, Barrios FX, Chiros CE. The positive and negative suicide ideation inventory: development and validation. Psychol Rep. (1998) 82:783–93. doi: 10.2466/pr0.1998.82.3.783
42. Peng B, Hu N, Guan L, Chen C, Chen Z, Yu H. Family functioning and suicidal ideation in college students: a moderated mediation model of depression and acceptance. Front Public Health. (2023) 11:1137921. doi: 10.3389/fpubh.2023.1137921
43. Epskamp S, Borsboom D, Fried EI. Estimating psychological networks and their accuracy: A tutorial paper. Behav Res Methods. (2018) 50:195–212. doi: 10.3758/s13428-017-0862-1
44. Epskamp S, Cramer AOJ, Waldorp LJ, Schmittmann VD, Borsboom D. qgraph: network visualizations of relationships in psychometric data. J Stat Software. (2012) 48:1–18. doi: 10.18637/jss.v048.i04
45. Haslbeck JMB, Waldorp LJ. mgm: estimating time-varying mixed graphical models in high-dimensional data. J Stat Softw. (2020) 93:1–46. doi: 10.18637/jss.v093.i08
46. Jones P. networktools: Tools for identifying important nodes in networks (2025). Available online at: https://cran.r-project.org/web/packages/networktools/index.html (Accessed April 7, 2025).
47. Van Borkulo CD, Van Bork R, Boschloo L, Kossakowski JJ, Tio P, Schoevers RA, et al. Comparing network structures on three aspects: A permutation test. Psychol Methods. (2023) 28:1273–85. doi: 10.1037/met0000476
48. Gross JJ. The emerging field of emotion regulation: an integrative review. Rev Gen Psychol. (1998) 2:271–99. doi: 10.1037/1089-2680.2.3.271
49. Preece DA, Mehta A, Petrova K, Sikka P, Bjureberg J, Becerra R, et al. Alexithymia and emotion regulation. J Affect Disord. (2023) 324:232–8. doi: 10.1016/j.jad.2022.12.06510.1016/j.jad.2022
50. Morie KP, Lord KA, Diefenbach GJ, Basuchoudhary O, Lewis S, Assaf M. Subscales of alexithymia show unique pathways through reappraisal and suppression to anxiety, depression and stress. J Affect Disord. (2024) 347:445–52. doi: 10.1016/j.jad.2023.11.038
51. Dafsari HS, Ray-Chaudhuri K, Mahlstedt P, Sachse L, Steffen JK, Petry-Schmelzer JN, et al. Beneficial effects of bilateral subthalamic stimulation on alexithymia in parkinson’s disease. Eur J Neurol. (2019) 26:222–e17. doi: 10.1111/ene.13773
52. Utas-Akhan L, Avci D, Basak I. Art therapy as a nursing intervention for individuals with schizophrenia. J Psychosoc Nurs Ment Health Serv. (2024) 62:29–38. doi: 10.3928/02793695-20231025-02
53. Nan JKM, Ho RTH. Effects of clay art therapy on adults outpatients with major depressive disorder: A randomized controlled trial. J Affect Disord. (2017) 217:237–45. doi: 10.1016/j.jad.2017.04.013
54. Ridout N, Smith J, Hawkins H. The influence of alexithymia on memory for emotional faces and realistic social interactions. Cogn Emotion. (2021) 35:540–58. doi: 10.1080/02699931.2020.174799110.1080/02699931.2020.17479
55. Franz M, Nordmann MA, Rehagel C, Schäfer R, Müller T, Lundqvist D. It is in your face-alexithymia impairs facial mimicry. Emotion. (2021) 21:1537–49. doi: 10.1037/emo0001002
56. Gul H, Torun YT, Cakmak FH, Gul A. Facial emotion recognition in adolescent depression: The role of childhood traumas, emotion regulation difficulties, alexithymia and empathy. Indian J Psychiatry. (2023) 65:443–52. doi: 10.4103/Indianjpsychiatry.Indianjpsychiatry_284_22
57. Butera CD, Harrison L, Kilroy E, Jayashankar A, Shipkova M, Pruyser A, et al. Relationships between alexithymia, interoception, and emotional empathy in autism spectrum disorder. Autism. (2023) 27:690–703. doi: 10.1177/13623613221111310
58. Salles BM, Maturana W, Mograbi DC. Exploring the multidimensional relationship between alexithymia and empathy: A systematic review. Bruno Maciel Carvalho Pinto Salles. (2023) 143:143-235.
59. Meng F, Meng F, Cui Y, Li X, Huang W, Xue Q, et al. Neural mechanisms of social empathy in the anterior cingulate cortex. Advanced Neurol. (2023) 2:281. doi: 10.36922/an.281
60. Bernhardt BC, Singer T. The neural basis of empathy. Annu Rev Neurosci. (2012) 35:1–23. doi: 10.1146/annurev-neuro-062111-150536
61. Morr M, Lieberz J, Dobbelstein M, Philipsen A, Hurlemann R, Scheele D. Insula reactivity mediates subjective isolation stress in alexithymia. Sci Rep. (2021) 11:15326. doi: 10.1038/s41598-021-94799-w
62. Hoffmann F, Banzhaf C, Kanske P, Gärtner M, Bermpohl F, Singer T. Empathy in depression: Egocentric and altercentric biases and the role of alexithymia. J Affect Disord. (2016) 199:23–9. doi: 10.1016/j.jad.2016.03.007
63. Yan Z, Hong S, Liu F, Su Y. A meta-analysis of the relationship between empathy and executive function. Psych J. (2020) 9:34–43. doi: 10.1002/pchj.311
64. Schumacher A, Campisi SC, Khalfan AF, Merriman K, Williams TS, Korczak DJ. Cognitive functioning in children and adolescents with depression: A systematic review and meta-analysis. Eur Neuropsychopharmacol. (2024) 79:49–58. doi: 10.1016/j.euroneuro.2023.11.005
65. Tang Y, Wang C, Li Q, Liu G, Song D, Quan Z, et al. Neural network excitation/inhibition: A key to empathy and empathy impairment. Neuroscientist. (2024) 30:644–65. doi: 10.1177/10738584231223119
66. Liu J, Zhao K, Zhou S, Hong L, Xu Y, Sun S, et al. Suicidal ideation in Chinese adults with schizophrenia: associations with neurocognitive function and empathy. BMC Psychiatry. (2023) 23:311. doi: 10.1186/s12888-023-04739-3
67. Sampogna G, Della Rocca B, Di Vincenzo M, Catapano P, Del Vecchio V, Volpicelli A, et al. Innovations and criticisms of the organization of mental health care in Italy. Int Rev Psychiatry. (2024), 1–10. doi: 10.1080/09540261.2024.2391800
68. Das A, Singh P, Bruckner T. Continuity of mental health care at community health centers and reduced emergency department visits for suicidal ideation/self-harm. Community Ment Health J. (2021) 57:1142–50. doi: 10.1007/s10597-020-00745-x
69. Fox DJ, Hanes D. Prevalence and correlates of unmet mental health services need in adolescents with major depressive episode in 2019: An analysis of national survey on drug use and health data. J Adolesc Health. (2023) 72:182–8. doi: 10.1016/j.jadohealth.2022.10.001
70. Mink AJ, Maddox MM, Pinero AJZ, Crockett EE. Gender differences in the physiological effects of emotional regulation. J Soc Psychol. (2023) 163:256–68. doi: 10.1080/00224545.2022.2064732
71. Rogier G, Chiorri C, Beomonte Zobel S, Muzi S, Pace CS, Cheung MW-L, et al. The multifaceted role of emotion regulation in suicidality: Systematic reviews and meta-analytic evidence. Psychol Bull. (2024) 150:45–81. doi: 10.1037/bul0000415
72. Su Y, Ye C, Xin Q, Si T. Major depressive disorder with suicidal ideation or behavior in Chinese population: A scoping review of current evidence on disease assessment, burden, treatment and risk factors. J Affect Disord. (2023) 340:732–42. doi: 10.1016/j.jad.2023.08.106
Keywords: major depressive disorder, alexithymia, adolescents, empathy, network analysis, suicide ideation
Citation: Shi Y, Zhang Q, Wang S, Zhang L, Liu Z, Wang J, Luo X, Wen X and Liu H (2025) A network analysis of alexithymia, empathy, and suicidal ideation in Chinese adolescents with major depressive disorders. Front. Psychiatry 16:1543651. doi: 10.3389/fpsyt.2025.1543651
Received: 11 December 2024; Accepted: 28 March 2025;
Published: 16 April 2025.
Edited by:
Vassilis Martiadis, Asl Napoli 1 Centro, ItalyReviewed by:
Fabiola Raffone, Asl Napoli 1 Centro, ItalyKongliang He, Hefei Fourth People’s Hospital Affiliated to Anhui Medical University, China
Copyright © 2025 Shi, Zhang, Wang, Zhang, Liu, Wang, Luo, Wen and Liu. This is an open-access article distributed under the terms of the Creative Commons Attribution License (CC BY). The use, distribution or reproduction in other forums is permitted, provided the original author(s) and the copyright owner(s) are credited and that the original publication in this journal is cited, in accordance with accepted academic practice. No use, distribution or reproduction is permitted which does not comply with these terms.
*Correspondence: Huanzhong Liu, aHVhbnpob25nbGl1QGFobXUuZWR1LmNu
†These authors have contributed equally to the work