- 1School of Physical Education and Health, Zhaoqing University, Zhaoqing, China
- 2School of Health Sciences and Sports, Macau Polytechnic University, Macau, China
- 3Day Surgery Centre, Shenzhen Nanshan People's Hospital, Shenzhen, China
- 4School of Social Sciences, Vitebsk State University, Vitebsk, Belarus
- 5School of Physical Education, Guangzhou Sport University, Guangzhou, China
Background: The Sustainable Development Goals (SDGs) call for systematic monitoring to optimize child development outcomes. As a developmental disorder affecting children and adults, Autism Spectrum Disorder (ASD) not only impacts individual social functioning but also places a burden on families and society. A detailed analysis of the latest global burden data on ASD can assist stakeholders in formulating support policies and interventions, thereby helping to meet the health needs of ASD.
Methods: We used data from the Global Burden of Disease Study 2021 (GBD 2021), compiled by the Institute for Health Metrics and Evaluation (IHME). Data were obtained through the Global Health Data Exchange (GHDx) and covered 204 countries and territories from 1990 to 2019. Variables included ASD-related prevalence, mortality, disability-adjusted life years (DALYs), age-standardized rates, and the sociodemographic index (SDI).
Results: Exposure to autism spectrum disorders contributed to 61823540 prevalence and 11544038 DALYs globally in 2021. Males and younger adults were high-risk populations. Higher socio-demographic index (SDI) regions were high-risk areas. The disease burden varied considerably across the GBD regions and the countries. From 1990 to 2021, the number of cases increased. The predicted results showed that the disease burden for both genders would still increase from 2022 to 2046. Countries or regions with a higher SDI have greater burden improvement potential.
Conclusion: The global burden of ASD has shown a continuous upward trend, with some differences observed across gender, age groups, and SDI regions. In terms of gender, the burden of ASD among females may be underestimated. Regarding age groups, the aging process has highlighted the urgent need to address ASD in the elderly population. High-SDI regions should place greater emphasis on improving diagnostic methods and implementing precise interventions, while middle- and low-SDI regions should focus on raising public awareness and enhancing screening capabilities.
1 Introduction
Autism Spectrum Disorder (ASD) is a complex developmental disorder characterized by difficulties in social interaction, restricted interests, and repetitive behaviors (1, 2). It typically manifests in early childhood and can have lifelong effects on an individual’s social functioning, including reduced opportunities for education, employment, and social inclusion (3). Additionally, individuals with ASD often experience comorbid conditions such as anxiety, depression, and attention-deficit hyperactivity disorder (ADHD) (4), which further exacerbate the decline in their quality of life and difficulties in social integration (5, 6). However, the specific causes and mechanisms underlying ASD remain unclear, potentially involving complex interactions between genetic, environmental, and social factors (7). With the global prevalence of ASD continuing to rise, particularly in high-income countries, the disorder has become a significant challenge in the field of global public health (8–10). Therefore, a comprehensive understanding of the prevalence and disease burden of ASD across different regions and populations is crucial for developing effective public health policies and interventions.
Recent studies have explored the use of machine learning models for the early prediction of autism in children, highlighting the potential of computational approaches in improving ASD diagnosis and monitoring (11). The Global Burden of Disease (GBD) study is a global health research project that aggregates the burden of various diseases, injuries, and risk factors across different regions and populations (12). Data for the GBD study come from multiple sources, including hospital records, disease surveillance systems, community surveys, health service usage, and demographic data (13). The GBD study also provides estimates of the prevalence, incidence, mortality, and disability-adjusted life years (DALYs) for ASD, offering valuable data to researchers for in-depth analysis of ASD’s global and regional impacts (14). The development of the Global Burden of Disease (GBD) database has advanced researchers’ ability to identify disease-specific patterns and has provided objective and reliable evidence for future studies (15).
Disability-adjusted life years (DALYs) are a comprehensive metric for measuring the burden of disease, proposed and widely adopted by the World Health Organization (WHO) and the GBD study. DALYs consist of two components: years of life lost due to premature mortality (YLL) and years lived with disability (YLD), and are used to assess the overall health loss caused by different diseases in populations (16). This indicator reflects both the fatal and non-fatal burden of diseases and serves as an important tool internationally for informing public health policies and setting intervention priorities (17).
In the GBD study, DALYs have been widely applied in health assessments and disease trend monitoring across numerous countries and regions, particularly in evaluating the societal burden of long-term health conditions such as chronic diseases, mental disorders, and developmental disorders (17). Therefore, incorporating DALYs into the burden assessment of ASD is beneficial for understanding the impact of this condition on public health systems from a macro-level perspective. However, there is still a lack of up-to-date information on the spatial distribution, temporal trends, and future projections of ASD burdens at the global, regional, and national levels, particularly concerning the differentiated impacts across regions with varying levels of social demographic development (SDI). This is crucial for formulating targeted public policies and reducing public health expenditures in regions with different development levels.
To better understand the temporal trends in the incidence and mortality of ASD, the Age-Period-Cohort (APC) model has been widely applied in epidemiological and public health research. This model separates the effects of three key time-related factors—age effects, period effects, and cohort effects—to help researchers identify changes in risk across different age groups, the influence of external events or environmental changes during specific historical periods, and the unique health risks faced by individuals born in different time cohorts (18, 19). Due to the strong linear dependency among the three factors (Cohort = Period – Age), traditional statistical methods struggle to simultaneously identify their independent effects. To address this “identifiability problem,” recent studies have developed various approaches such as constraint-based methods, decomposition techniques, and Bayesian models (20). In disease surveillance and forecasting, the APC model provides reliable evidence-based support for policymakers and has proven particularly valuable in the fields of mental disorders, chronic diseases, and developmental disorders in children, such as ASD.
To more accurately predict the future burden of ASD, this study aims to leverage data from GBD 2021 to conduct a comprehensive analysis of ASD prevalence and DALYs at global, regional, and national levels from 1990 to 2021. By exploring temporal trends and regional disparities in ASD burden, the study will identify key factors driving these trends. Additionally, the APC model will be employed to forecast changes in ASD burden over the next 25 years. Cutting-edge analytical methods will be utilized to assess the relationship between disease burden and social demographic development, revealing potential opportunities for health improvement across regions with varying development levels. These findings will help visualize global and regional epidemiological trends and burden changes of ASD, providing critical reference points for the development of targeted intervention strategies and resource allocation plans. ASD Differences in disease burden across regions may be associated with variations in access to healthcare resources, diagnostic capacity, and public awareness. Li et al. (2019) found that regions with higher socioeconomic status and more developed healthcare systems tend to report higher prevalence rates of ASD, which may be closely related to improved diagnostic capabilities and increased public awareness (10). Therefore, when evaluating the global burden of ASD, it is essential to consider contextual factors such as regional levels of social development and healthcare system capacity.
Although several studies have utilized the GBD database to analyze the burden of ASD, most have focused on descriptive analyses within the 1990–2019 period, primarily addressing temporal trends or regional disparities, without employing predictive models or advanced statistical approaches (21). Solmi et al. (2022) analyzed global and regional ASD burden trends using GBD 2019 data but did not incorporate advanced methods such as the APC model (21). Similarly, the study by Li et al. (2022) provided insights into the distribution of ASD burden in China and globally but did not project future disease burden or explore its associations with sociodemographic factors (22). Therefore, this study integrates GBD 2021 data with APC modeling, ARIMA forecasting, exponential smoothing, and frontier analysis to conduct a comprehensive temporal assessment and prediction of the ASD burden. This multi-method, advanced analytical approach offers a deeper understanding of historical changes and future trends in ASD burden and provides novel evidence to inform public health policies at both global and regional levels (23).
The GBD study was initially launched by the World Health Organization and is currently led by the Institute for Health Metrics and Evaluation (IHME) at the University of Washington. It is one of the most authoritative and comprehensive global health data projects to date. Since its inception in the 1990s, the GBD has evolved into a standardized assessment framework covering 204 countries and territories and encompassing 369 diseases and injuries. It provides estimates of incidence, prevalence, mortality, and DALYs, stratified by age, sex, region, and year (24). In recent years, GBD data have been widely applied in burden assessments of neurodevelopmental and mental disorders, including ASD (17, 22). Existing studies have explored temporal trends, regional disparities, and associations with sociodemographic factors; however, few have incorporated predictive models or in-depth decomposition methods. This study, based on the latest GBD 2021 data, aims to comprehensively evaluate and forecast the burden of ASD across regions by integrating APC analysis, ARIMA forecasting, exponential smoothing, and frontier efficiency analysis, thereby addressing existing research gaps.
2 Methods
2.1 Data sources
The present study is based on the GBD 2021, which represents the most up-to-date and comprehensive dataset currently available for estimating global and regional disease burden (25). Compared to previous GBD iterations (e.g., GBD 2019), GBD 2021 incorporates updated data sources, revised modeling strategies, and extended time series, thereby providing a more accurate and contemporary picture of ASD-related disability and mortality. To ensure the rigor and reliability of the statistical analyses applied in this study, including the APC model, ARIMA forecasting, exponential smoothing, and frontier efficiency analysis, all methods were reviewed and validated by an experienced statistician.
The primary data sources for this study were the Global Health Data Exchange (GHDx) and the IHME database (26, 27). These databases compile comprehensive information on autism spectrum disorders prevalence and DALYs from various countries and regions globally. We accessed the most recent estimates of autism spectrum disorders prevalence and DALYs, spanning from 1990 to 2021.
To ensure the robustness of our analyses, the GBD 2021 dataset already incorporates standardized protocols for dealing with missing or incomplete data through advanced statistical modeling. In this study, we adhered to the default data preprocessing methods provided by the Institute for IHME. Additionally, for country-year-age groups with missing estimates, the GBD database provides modeled estimates with corresponding 95% uncertainty intervals (UIs), which were used directly in our analyses to maintain data consistency and comparability. No further manual imputation was conducted, and sensitivity analyses using ARIMA and ES models were applied to validate the stability of the results derived from modeled inputs.
For the purpose of geographical analysis, the global encompassing 204 countries was systematically partitioned into 54 distinct GBD regions (27). Additionally, these 204 countries and territories were stratified into five SDI tiers: low, low-middle, middle, high-middle, and high, providing a nuanced understanding of socioeconomic disparities (28). The methodological underpinnings of the GBD 2021 framework have been thoroughly detailed in preceding publications (29, 30), ensuring reproducibility and transparency. Data acquisition was multifaceted, drawing upon an array of reputable sources such as cancer registries, peer-reviewed studies, surveillance systems, census records, and other pertinent databases (31). Each dataset was accompanied by its corresponding 95% uncertainty intervals (UIs), facilitating robust error propagation and assessment of the estimates’ precision. To quantify the overall burden of disease, DALYs were computed by the aggregation of Years of Life Lost (YLLs) due to premature mortality and Years Lived with Disability (YLDs) attributed to each individual cause of health loss (32).
2.2 Statistical analyses
First, the prevalence and causes of DALYs attributable to autism spectrum disorders, along with their Age-Standardized Rates (ASRs), were assessed for 2021 on a global scale, further stratified by sex, age, SDI regions, GBD regions, and countries. Next, a linear regression model was used to calculate the Estimated Annual Percentage Change (EAPC) values and to evaluate the temporal trends in the global burden from 1990 to 2021. Additionally, the association between the EAPCs in 1990 and the ASRs related to autism spectrum disorders, as well as the Human Development Index (HDI) values in 2021, was assessed. Given that these three factors do not follow a normal distribution, Spearman correlation analysis was employed to explore the associations among them. Furthermore, hierarchical clustering analysis was conducted using EAPC values, categorizing the 54 GBD regions into four distinct groups: significantly increasing, slightly increasing, stable or slightly decreasing, and significantly decreasing, to identify evolving patterns of disease burden in different GBD regions. To project the future disease burden from 2022 to 2046, an APC model integrated with nested Laplace approximations was employed. Sensitivity analyses were performed using the Autoregressive Integrated Moving Average (ARIMA) model and Exponential Smoothing (ES) model. These two time-series forecasting models are widely used in the field of epidemiology, as they can reveal data trends from different perspectives, complement each other, and enhance the scientific rigor and credibility of the projections. The ARIMA model is well-suited for handling non-stationary data by capturing linear temporal dependencies through autoregressive, differencing, and moving average components. It has been extensively applied in disease trend forecasting, including studies on mental disorders and developmental conditions (33). ES model is more suitable for forecasting data with relatively stable trends or short-term fluctuations, making it particularly useful for rapid response needs in public health management (34). In this study, the use of both ARIMA and ES models not only validated the stability of the APC model’s predictions but also provided a more comprehensive perspective for evaluating future trends in the burden of autism.
Finally, frontier analysis was used to assess the association between disease burden and socioeconomic development. A nonlinear frontier was established using a nonparametric Data Envelopment Analysis (DEA) method to determine the minimum achievable burden based on the current development levels of countries or regions. The efficiency gap represents the difference between observed ASRs and the frontier, indicating the potential for health improvements under current development conditions.
If the P-value was under 0.05, it was deemed statistically significant. R software (version 4.0.2) was employed for database creation, organization, and analysis.
3 Results
3.1 The disease burden attributable to autism spectrum disorders in 2021
In 2021, the number of autism spectrum disorders-related prevalence was 61823540 [95% uncertainty intervals (UI): 52067673-72711238], with the corresponding age-standardized prevalence rate (ASPR) was 788.34 (95% UI: 663.76-927.21) per 100,000 population. The number of DALYs cases was 11544038 (95% UI: 7842315-16288865), and the corresponding ASR of DALYs was 147.56 (95% UI: 100.21-208.16) per 100,000 population (Tables 1, 2).
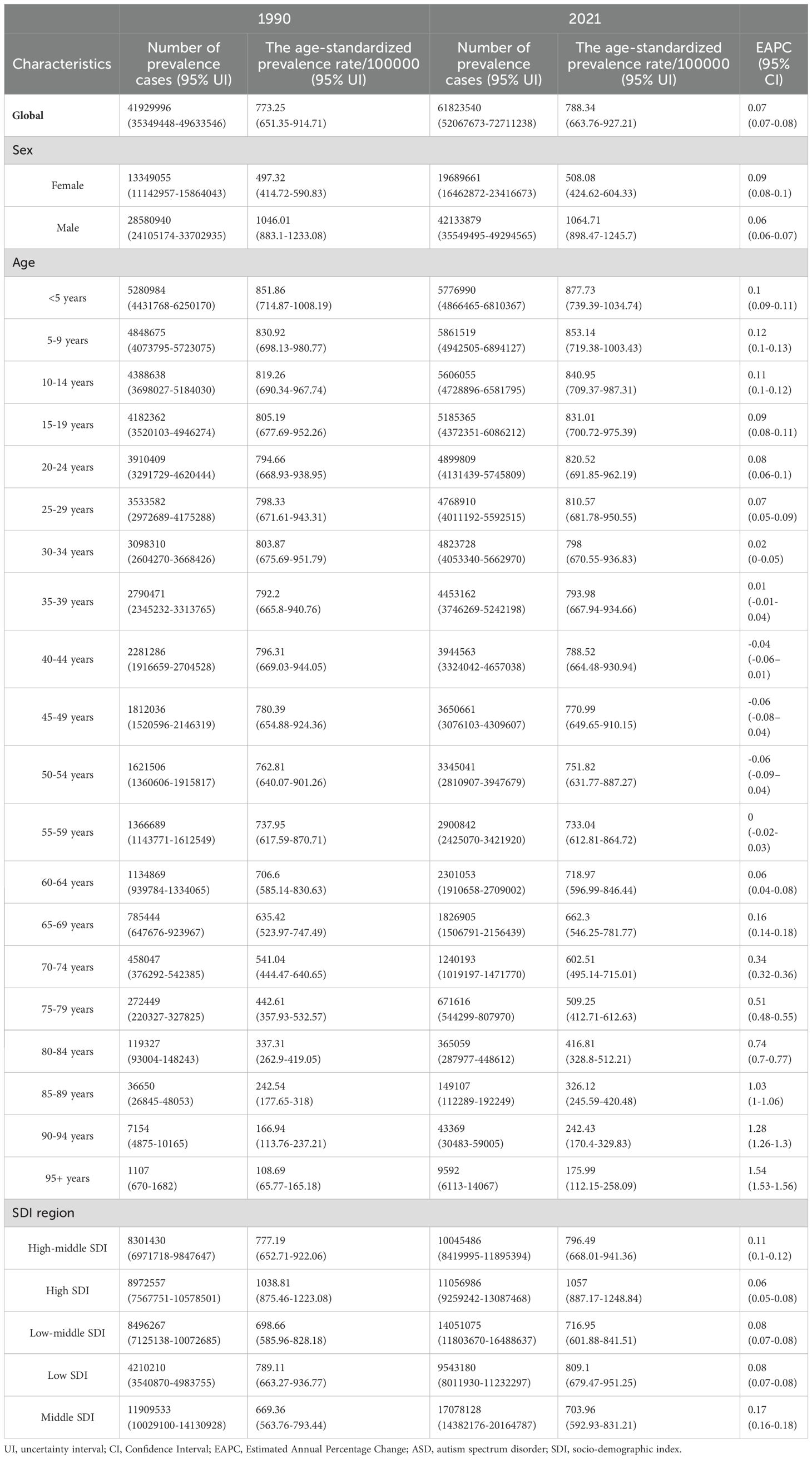
Table 1. The number of prevalence cases and the age-standardized prevalence rate attributable to autism spectrum disorders in 1990 and 2021, and its trends from 1990 to 2021 globally.
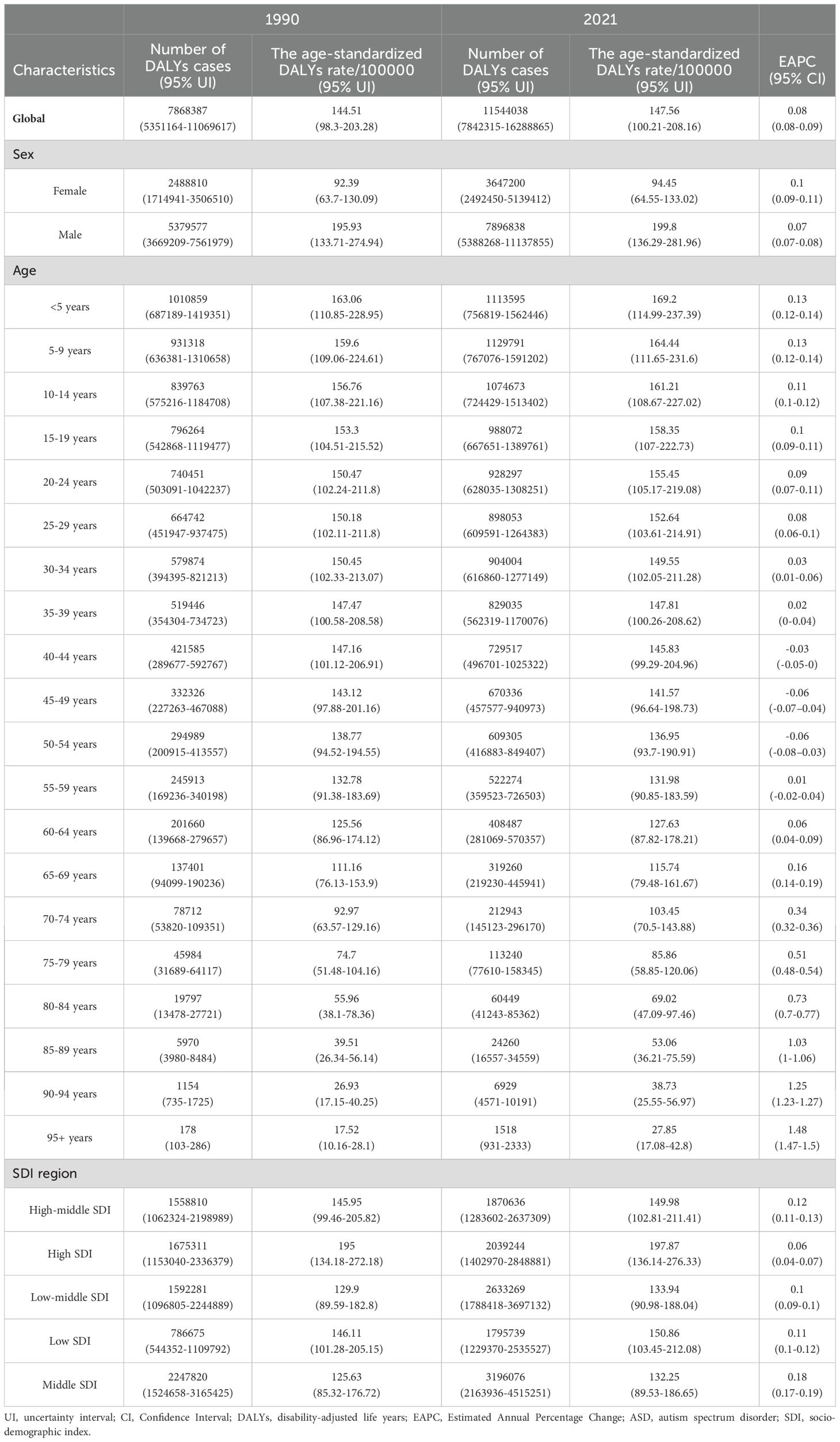
Table 2. The number of DALYs cases and the age-standardized DALYs rate attributable to autism spectrum disorders in 1990 and 2021, and its trends from 1990 to 2021 globally.
In 2021, the disease burden was higher in males than females. (Supplementary Figure S1, Tables 1, 2). Prevalence and DALYs across age groups separately in 2021 were available in Supplementary Figure S2. The disease burden decreased with age (Supplementary Figure S2, Tables 1, 2).
At the SDI region level, the high SDI region had the most ASRs, and the lowest ASPR and ASDAR were observed in the middle SDI regions (Supplementary Figure S3, Tables 1, 2). As for the number of cases, the middle SDI region had the highest number of cases and the low SDI regions had the lowest number of cases. The regular pattern of SDI levels and disease burden was stable across countries and territories. From Supplementary Figure S4, the ASRs for prevalence and DALYs showed a “U” relationship with SDI, with the rate being lowest when the SDI was around 0.50.
Across the 54 GBD regions, High-income Asia Pacific ranked the top one in autism spectrum disorders-related ASPR and ASDAR (ASPR: 1559.53, 95% UI: 1311.3-1832.39; ASDAR: 293.92, 95% UI: 203.17-413.01), followed by Australasia and High-income North America. While Tropical Latin America ranked the bottom one (ASPR: 614.52, 95% UI: 514.73-732.29; ASDAR: 114.44, 95% UI: 77.06-160.26), followed by East Asia and Oceania. For the number of cases, the top GBD region was Asia (prevalence: 33291200, 95% UI: 27983185-39014585; DALYs: 6224807, 95% UI: 4229135-8809052), followed by World Bank Lower Middle Income and Limited Health System. The bottom number of cases was observed in Oceania (prevalence: 96966, 95% UI: 81551-116331; DALYs: 18288, 95% UI: 12475-25630), followed by the Caribbean and Australasia (Supplementary Figure S5, Supplementary Tables S1-S2).
The disease burden of autism spectrum disorders varied considerably across the world, with the top ASPR and ASDAR observed in Japan (ASPR: 1586.87, 95% UI: 1333.18-1864.1; ASDAR: 299.14, 95% UI: 207.18-420.04), followed by Korea and Singapore. The bottom ASRs of prevalence and DALYs observed in Bangladesh (ASPR: 588.23, 95% UI: 486.73-696.61; ASDAR: 110.26, 95% UI: 75.38-154.62), followed by Brazil. As for the absolute number, the highest number of prevalence (10120042, 95% UI: 8503425-11876619) and DALYs cases (1887143, 95% UI: 1285675-2649048) were observed in India, followed by China and America. And the lowest number of prevalence (10, 95% UI: 8-12) and DALYs cases (2, 95% UI: 1-3) was observed in Tokelau in 2021, followed by Niue and Nauru (Figure 1, Supplementary Tables S1-S2).
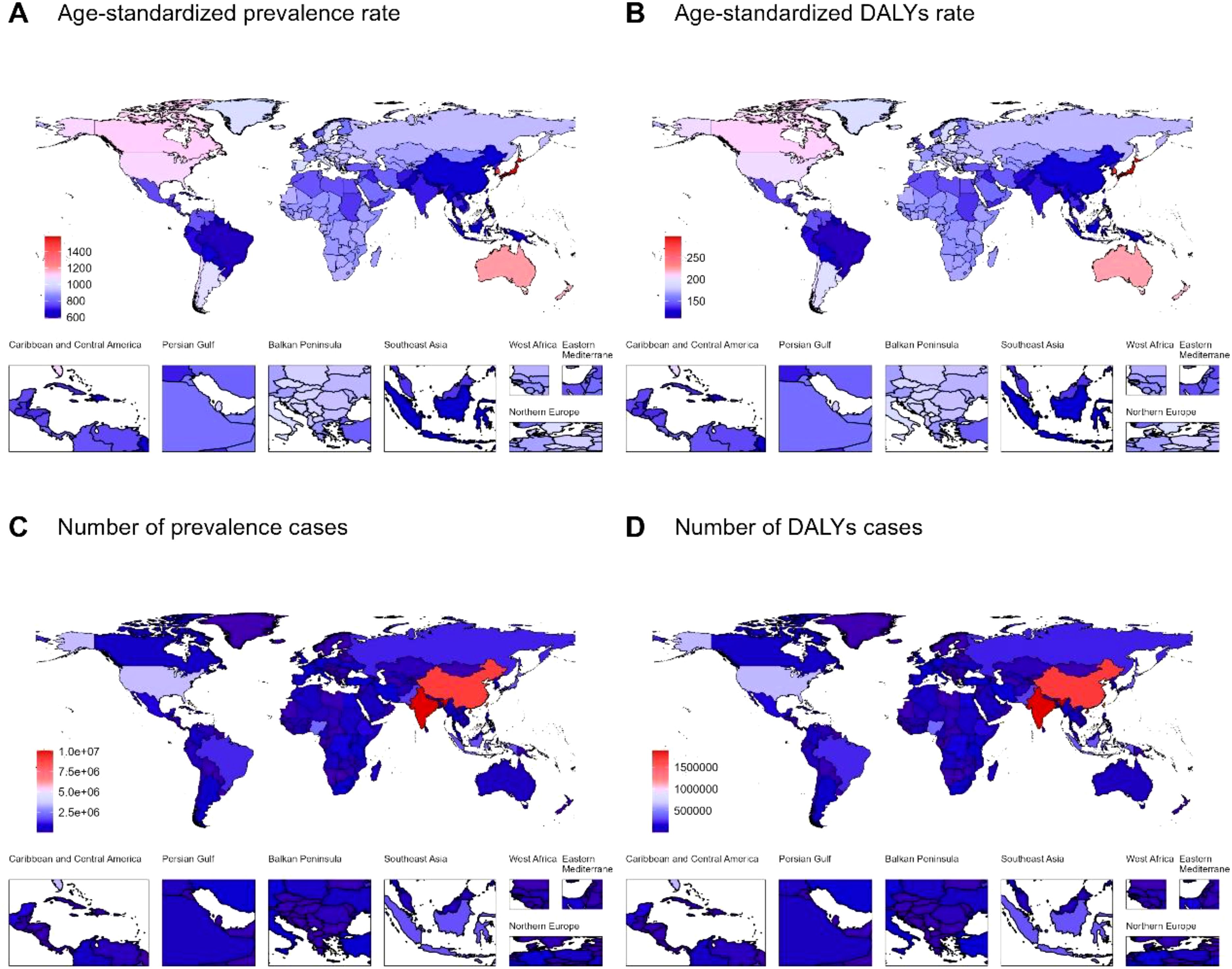
Figure 1. (A) Age-standardized prevalence rate, (B) Age-standardized DALYs rate, (C) Number of prevalence cases, and (D) Number of DALYs cases of autism spectrum disorders across countries and territories in 2021. DALYs: disability-adjusted life years.
3.2 Temporal trend for autism spectrum disorders-related disease burden from 1990 to 2021
The number of prevalence cases of autism spectrum disorders increased from 41929996 (95% UI: 35349448-49633546) in 1990 to 61823540 (95% UI: 52067673-72711238) in 2021 globally. However, the corresponding ASPR changed in the same direction, increasing from 773.25 (95% UI: 651.35-914.71) to 788.34 (95% UI: 663.76-927.21). However, the prevalence estimate followed a different pattern, with an increase in the number of DALYs cases from 7868387 (95% UI: 5351164-11069617) to 11544038 (95% UI: 7842315-16288865), and an increase in ASDAR from 144.51 (95% UI: 98.3-203.28) to 147.56 (95% UI: 100.21-208.16) (Supplementary Figure S6, Tables 1, 2).
The trends in males and females alone were consistent with the whole population (Supplementary Figure S7, Tables 1, 2). As for the subtype of age group, for the number of cases, the trends for all age groups were the same as global. For ASRs, the trend was also consistent for most age groups except for 40-44 years, 45-49 years, and 50-54 years (Supplementary Figure S8, Tables 1, 2). At the SDI region level, the trends for all SDI regions were also consistent with the whole population (Supplementary Figure S9, Tables 1, 2).
Across GBD regions, the autism spectrum disorders-related disease burden varied. To find areas with similar variation in disease burden, a hierarchical clustering analysis was conducted in this study. The results are shown in Supplementary Figure S8. The significant increase in ASRs occurred in World Bank Upper Middle Income, Commonwealth low-income, Basic Health System, High-income Asia Pacific, East Asia, and Commonwealth High Income, while the significant decrease was in Oceania, Caribbean, Region of the Americas, America, Tropical Latin America, Latin America & Caribbean -WB, Western Sub-Saharan Africa, Western Africa, South Asia -WB, and South Asia (Supplementary Figure S10).
Across countries and territories, the most pronounced increase in ASPR from 1990 to 2021 was observed in Equatorial Guinea [EAPC=0.34, 95% confidence interval (CI): 0.32-0.35]. And the most pronounced increase in ASDAR was also in Equatorial Guinea (EAPC=0.39, 95% CI: 0.37-0.41). The most pronounced decrease was observed in Zimbabwe for both ASPR and ASDAR (ASPR: EAPC=-0.09, 95% CI: -0.11–0.08; ASDAR: EAPC=-0.09, 95% CI: -0.11–0.08) (Supplementary Tables S1-S2).
3.3 The influential factors for EAPC
The association between EAPCs and ASRs in 1990, and HDIs in 2021 was detected, respectively (Supplementary Figure S11). The ASRs of autism spectrum disorders in 1990 reflected the disease pool at baseline, and the HDIs in 2021 could be used as the availability of health care and a proxy for the level in each country. A significant association between EAPCs and ASRs of prevalence and DALYs was discovered (prevalence: ρ=0.34, p<0.01; DALYs: ρ=0.32, p<0.01). Moreover, the positive association was also significant between EAPCs and HDIs (prevalence: ρ =0.59, p<0.01; DALYs: ρ=0.56, p<0.01) (Supplementary Figure S11).
3.4 The predicted results from 2022 to 2046
The predicted results of the APC model showed that the number of prevalence and DALYs cases for both genders would increase from 2022 to 2046. However, the corresponding ASRs will remain relatively stable, with little change, over the next 25 years (Figure 2, Supplementary Table S3). The results of the ARIMA and ES models remained consistent with the APC model, verifying the stability of the results (Figure 2, Supplementary Tables S4-S5).
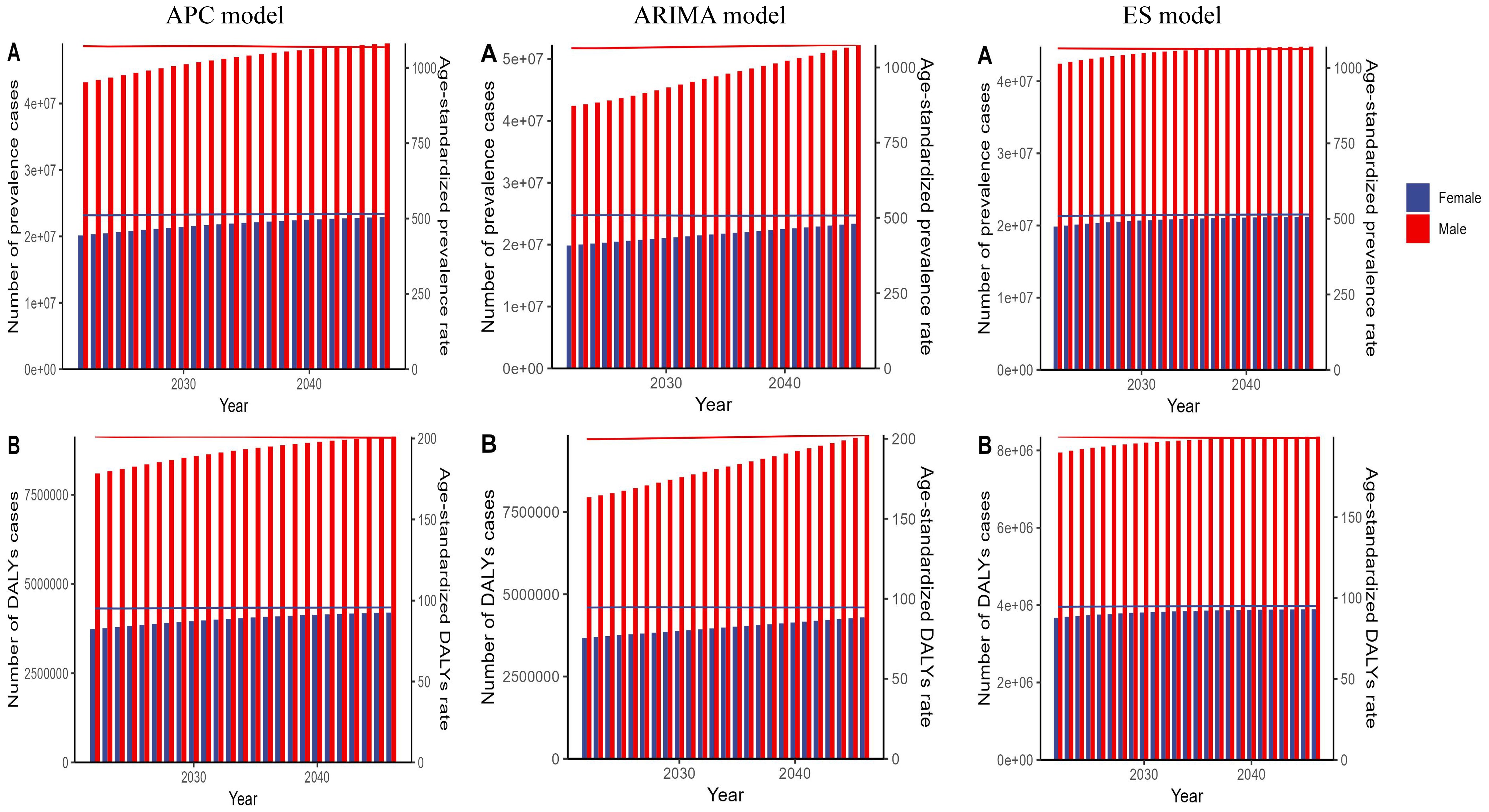
Figure 2. The predicted global results for autism spectrum disorder (ASD)-related numbers and age-standardized rates of prevalence and DALYs by sex from 2022 to 2046, based on the APC model, ARIMA model, and ES model. Panel (A) shows the projected number of prevalence cases and age-standardized prevalence rate for ASD. Panel (B) shows the projected number of DALYs cases and age-standardized DALYs rate for ASD. DALYs, disability-adjusted life years; APC, age–period–cohort; ARIMA, autoregressive integrated moving average; ES, exponential smoothing.
3.5 Frontier analysis for ASRs of autism spectrum disorders
Supplementary Figure S12 presents the unrealized health advancements in ASRs of autism spectrum disorders, stratified by sociodemographic development levels across various countries and regions from 1990 to 2021. Our analysis reveals discernible patterns in the and their respective efficacy disparities across these strata. Notably, as sociodemographic development advances, the effective difference in autism spectrum disorders burden generally escalates, suggesting a heightened potential for improvement among higher SDI nations or regions in 2021. This observation underscores the intricate interplay between societal advancement and autism spectrum disorders health outcomes, necessitating targeted interventions tailored to the unique challenges faced by each developmental tier.
4 Discussion
This study utilizes data from GBD 2021 to conduct a comprehensive analysis of the prevalence and DALYs of ASD at global, regional, and national levels from 1990 to 2021 and applies the APC model to predict trends in the disease burden over the next 25 years.
The results indicate that the global prevalence and DALYs of ASD have significantly increased over the past three decades, with the number of ASD cases worldwide rising from 41.93 million in 1990 to 61.82 million in 2021. Although the age-standardized prevalence rate and age-standardized DALY rate have remained relatively stable, the overall increase in the burden suggests that the diagnosis and reporting of ASD have improved globally. This trend reflects positive developments, such as increased public awareness of ASD diagnosis and improvements in healthcare services. However, it also highlights the various challenges countries face in terms of resource allocation and public health policies to address the growing burden of ASD.
From the perspective of global and regional trends in the ASD burden, there has been an overall increasing trend in the prevalence and DALYs of ASD from 1990 to 2021. This increase is closely related to the SDI level, being particularly evident in high-SDI regions, while the increase in ASD burden is less significant in middle and low-SDI regions, with some low-SDI areas even experiencing a decline. The findings of this study are consistent with previous research, which has generally suggested that regional differences in the burden of ASD are closely related to the distribution of healthcare resources, diagnostic capacity, and the level of public awareness about ASD (35, 36). Li et al. (2019) emphasized that socioeconomic development and the quality of healthcare systems significantly influence the reported prevalence of ASD (10).
The global burden of autism spectrum disorder (ASD) has shown a continuous upward trend, particularly in high-SDI regions. Solmi et al. (2022), using GBD 2019 data, reported a consistent increase in ASD prevalence and disease burden worldwide, especially in high-income countries (9). However, their study did not incorporate predictive modeling. In contrast, our study integrated the APC, ARIMA, and Exponential Smoothing models to provide a forward-looking assessment of the ASD burden through 2046. While Li et al. (2022) described the distribution of ASD burden in China and globally, their study did not forecast future trends or conduct stratified analyses by age and gender (10). Our study addressed these gaps, revealing persistent gender differences in ASD burden and a rising trend among the elderly population, particularly among older females. Baxter et al. (2015) highlighted the high rate of underdiagnosis of ASD in low-income countries, which our study further confirmed through frontier efficiency analysis (37). In addition, Lai et al. (2014) pointed out that females with ASD are more likely to engage in “social camouflaging” behaviors, leading to lower diagnostic rates—an observation that aligns closely with our findings on gender differences (38). Elsabbagh et al. (2012) emphasized the lack of ASD screening and surveillance systems in many resource-limited countries, which supports our recommendation to strengthen diagnostic capacity and public awareness in middle- and low-SDI regions (35). Overall, our study expands the analytical depth and temporal scope of ASD burden research and provides more comprehensive evidence to support the development of targeted public health policies across regions with varying levels of social development.
High-SDI regions benefit from better economic conditions, sufficient healthcare resources, and greater public awareness of ASD (39), leading to higher attention to and feasibility of diagnosing the disorder. Additionally, due to improvements in diagnostic capabilities, high-SDI areas have not only correctly reclassified individuals who were previously misdiagnosed with personality disorders as having ASD (40), but they have also extended the diagnostic scope to adults (41) while identifying some common comorbidities associated with ASD. In contrast, low-SDI regions often face various challenges, such as insufficient healthcare resources and lower public awareness (42), resulting in lower diagnostic rates. Therefore, it is necessary for relevant authorities to enhance the accessibility of healthcare services and improve public education to narrow the gap.
From the perspective of gender and age, the prevalence and DALYs of ASD are higher in males than in females, although this gap has gradually narrowed over the past three decades, it still remains significant. This gender difference may be attributed to higher rates of internal difficulties and social camouflaging behaviors in female ASD patients (43, 44), making the diagnosis of ASD in females more challenging. With increasing attention to female ASD in recent years (45, 46), the diagnostic rate for female patients has shown an upward trend. There are also significant differences across age groups. Due to improvements in early screening, early diagnosis of ASD has led to an increased diagnostic rate in children (41, 47), with the 0-4 age group having the highest confirmation rate of ASD. As age increases, the prevalence of ASD gradually decreases, however, the diagnostic rate and DALYs of ASD among individuals aged 60 and older have shown an increasing trend globally. Therefore, it is necessary to develop targeted diagnostic, treatment, and support measures for ASD patients across different age groups in the future, with a particular focus on enhancing the management and care of elderly ASD patients in the context of an aging population.
The prediction results based on the APC model indicate that from 2022 to 2046, the global prevalence and DALYs of ASD will continue to increase in absolute numbers, while the age-standardized rates will remain relatively stable. This trend aligns with population growth and aging, suggesting that the overall burden of ASD will increase in the future, although the distribution across different age groups and regions may remain relatively balanced. However, high-SDI regions will face a greater burden of ASD due to the intensifying aging trend. Therefore, policymakers in high-SDI areas should prioritize increasing the accessibility of ASD diagnosis and treatment services, as well as strengthening targeted support services. Meanwhile, middle- and low-SDI regions need to enhance early diagnosis and intervention measures in the future to mitigate the potential impact of ASD on society.
5 Limitations
Although this study provides a detailed analysis of the burden of ASD at global, regional, and national levels, there are some limitations. First, differences in data sources and quality may affect the accuracy of the results, especially in middle- and low-SDI regions where many ASD cases remain undiagnosed and untreated. Second, the significant variation in diagnostic criteria, data collection methods, and public awareness across countries and regions may increase the heterogeneity in estimating the ASD burden. Third, this study does not fully account for the issue of comorbidity between ASD and other mental and neurodevelopmental disorders, which are common among ASD patients (48–50), potentially leading to an underestimation of the ASD burden. Fourth, gender-related differences may contribute to the underdiagnosis and lack of intervention for females with ASD (51, 52), resulting in a possible underestimation of the burden among this group. Therefore, future research should further explore the relationship between ASD and other comorbidities, place more emphasis on the diagnosis and intervention of females with ASD, and incorporate more detailed data from various regions and countries to analyze the specific impact on the burden of ASD.
6 Conclusion
This study, by analyzing the burden of ASD (Autism Spectrum Disorder) at global, regional, and national levels from 1990 to 2021, elucidates the temporal trends and key determinants influencing ASD burden across these levels. It provides crucial insights for formulating targeted public health policies and interventions tailored to regions with varying levels of development. The findings indicate that, over the past three decades, the prevalence and associated burden of ASD have significantly increased in high-SDI regions. Although some progress has been made in management and intervention strategies, these efforts have not been sufficient to effectively curb the disability burden associated with ASD.
Future strategies should be tailored to the specific characteristics of ASD, with a focus on disease screening, comorbidities, social support, and public awareness. In high-SDI regions, there is a need to actively develop age-specific treatment strategies that not only address the high prevalence among children but also cater to the treatment needs of ASD patients across other age groups. Conversely, middle- and low-SDI regions should prioritize enhancing public awareness and improving ASD screening efforts to mitigate the associated burden. At the same time, it is recommended that future research adopt interdisciplinary approaches that integrate clinical, social, and policy perspectives to develop more effective strategies for early screening, diagnosis, and intervention (53). Attention should also be given to evaluating the long-term effects of public health interventions across different gender and age groups (54), as well as examining the impact of sociocultural factors and healthcare accessibility on disparities in ASD diagnosis (55, 56), in order to provide a foundation for more inclusive and targeted policy development. Moreover, recent developments in intelligent diagnostic systems demonstrate that hybrid ensemble learning techniques can enhance the accuracy of ASD detection and risk stratification, offering promising directions for personalized screening tools in future research (57).
Author’s note
The inclusion of any map (including the depiction of any boundaries therein), or of any geographic or locational reference, does not imply the expression of any opinion whatsoever on the part of BMJ concerning the legal status of any country, territory, jurisdiction or area or of its authorities. Any such expression remains solely that of the relevant source and is not endorsed by BMJ. Maps are provided without any warranty of any kind, either express or implied.
Data availability statement
The original contributions presented in the study are included in the article/Supplementary Material. Further inquiries can be directed to the corresponding author.
Ethics statement
This study was based on publicly available data from the Global Burden of Disease (GBD) study provided by the Institute for Health Metrics and Evaluation (https://vizhub.healthdata.org/gbd-results/). As the study utilized secondary data without directly involving human participants, no specific institutional ethics approval was required. Additionally, the study complies with the principles of the Declaration of Helsinki (http://www.wma.net/en/30publications/10policies/b3/index.html).
Author contributions
DL: Conceptualization, Data curation, Formal Analysis, Methodology, Project administration, Resources, Software, Validation, Writing – original draft, Writing – review & editing. ZL: Conceptualization, Data curation, Formal Analysis, Investigation, Methodology, Project administration, Software, Writing – original draft, Writing – review & editing. CM: Conceptualization, Data curation, Formal Analysis, Methodology, Project administration, Writing – original draft. LL: Methodology, Supervision, Formal Analysis, Validation, Visualization, Writing – review & editing. CL: Conceptualization, Formal Analysis, Funding acquisition, Investigation, Methodology, Resources, Software, Validation, Visualization, Writing – original draft, Writing – review & editing.
Funding
The author(s) declare that no financial support was received for the research and/or publication of this article.
Conflict of interest
The authors declare that the research was conducted in the absence of any commercial or financial relationships that could be construed as a potential conflict of interest.
Generative AI statement
The author(s) declare that no Generative AI was used in the creation of this manuscript.
Publisher’s note
All claims expressed in this article are solely those of the authors and do not necessarily represent those of their affiliated organizations, or those of the publisher, the editors and the reviewers. Any product that may be evaluated in this article, or claim that may be made by its manufacturer, is not guaranteed or endorsed by the publisher.
Supplementary material
The Supplementary Material for this article can be found online at: https://www.frontiersin.org/articles/10.3389/fpsyt.2025.1570276/full#supplementary-material
Abbreviations
ADHD, Attention-Deficit/Hyperactivity Disorder; APC, Age-Period-Cohort; ARIMA, Autoregressive Integrated Moving Average; ASD, Autism Spectrum Disorder; ASPR, Age-Standardized Prevalence Rate; BMJ, British Medical Journal; CI, Confidence Interval; DEA, Data Envelopment Analysis; EAPC, Estimated Annual Percentage Change; ES, Exponential Smoothing; GBD, Global Burden of Disease; GHDx, Global Health Data Exchange; HALE, Health-Adjusted Life Expectancy; HDI, Human Development Index; IHME, Institute for Health Metrics and Evaluation; RRBI, Restricted and Repetitive Behavior Interests; SDG, Sustainable Development Goal; SDI, Socio-Demographic Index; UI, Uncertainty Interval; WHO, World Health Organization;YLD, Years Lived with Disability; YLL, Years of Life Lost.
References
1. Lefebvre A, Traut N, Pedoux A, Maruani A, Beggiato A, Elmaleh M, et al. Exploring the multidimensional nature of repetitive and restricted behaviors and interests (RRBI) in autism: neuroanatomical correlates and clinical implications. Mol Autism. (2023) 14:45.
2. Thom RP, McDougle CJ. Repetitive thoughts and behaviors in autism spectrum disorder: A symptom-based framework for novel therapeutics. ACS Chem Neurosci. (2023) 14:1007–16.
3. Lord C, Brugha TS, Charman T, Cusack J, Dumas G, Frazier T, et al. Autism spectrum disorder. Nat Rev Dis Primers. (2020) 6:1–23.
4. Li D, Miao C, Wang D, Li C. Effect of physical activity interventions on executive functions in school-age children with ADHD: A meta-analysis of randomized controlled trials. (2025). doi: 10.1016/j.jad.2025.01.155
5. Howlin P, Magiati I. Autism spectrum disorder: Outcomes in adulthood. Curr Opin Psychiatry. (2017) 30:69–76.
6. Li D, Li L, Zang W, Wang D, Miao C, Li C, et al. Effect of physical activity on attention in school-age children with ADHD: a systematic review and meta-analysis of randomized controlled trials. Front Physiol. (2023) 14:1189443.
7. Lyall K, Croen L, Daniels J, Fallin MD, Ladd-Acosta C, Lee BK, et al. The changing epidemiology of autism spectrum disorders. Annu Rev Public Health. (2017) 38:81–102.
8. Dinstein I, Solomon S, Zats M, Shusel R, Lottner R, Gershon BB, et al. Large increase in ASD prevalence in Israel between 2017 and 2021. Autism Res. (2024) 17:410–8.
9. Solmi M, Song M, Yon DK, Lee SW, Fombonne E, Kim MS, et al. Incidence, prevalence, and global burden of autism spectrum disorder from 1990 to 2019 across 204 countries. Mol Psychiatry. (2022) 27:4172–80. doi: 10.1038/s41380-022-01630-7
10. Li Z, Yang L, Chen H, Fang Y, Zhang T, Yin X, et al. Global, regional and national burden of autism spectrum disorder from 1990 to 2019: results from the Global Burden of Disease Study 2019. Epidemiol Psychiatr Sci. (2022) 31:e33. doi: 10.1017/S2045796022000178
11. Ridouh A, Imedjdouben F, Mahi S. Autism spectrum disorder detection using machine learning techniques. Int J Bioinf Res Appl. (2024) 20:495–516. doi: 10.1504/IJBRA.2024.141770
12. G.B.D. Collaborators, Ärnlöv J. Global burden of 87 risk factors in 204 countries and territories, 1990–2019: a systematic analysis for the Global Burden of Disease Study 2019. Lancet. (2020) 396:1223–49.
13. Feigin VL, Vos T, Alahdab F, Amit AML, Bärnighausen TW, Beghi E, et al. Burden of neurological disorders across the US from 1990-2017: a global burden of disease study. JAMA Neurol. (2021) 78:165–76.
14. G.B.D.M.D. Collaborators. Global, regional, and national burden of 12 mental disorders in 204 countries and territories, 1990–2019: a systematic analysis for the Global Burden of Disease Study 2019. Lancet Psychiatry. (2022) 9:137–50.
15. Wang Y, Wang J, He J, Ji B, Pang Z, Wang J, et al. Comprehensive analysis of PRPF19 immune infiltrates, DNA methylation, senescence-associated secretory phenotype and ceRNA network in bladder cancer. Front Immunol. (2023) 14:1289198. doi: 10.3389/fimmu.2023.1289198
16. Murray CJ. A Comprehensive Assessment of Mortality and Disability from Diesease, Injuries and Risk factors in 1990 and projected to 2020. The Global Burden of Disease. (1994) 1:.
17. Vos T, Lim SS, Abbafati C, Abbas KM, Abbasi M, Abbasifard M, et al. Global burden of 369 diseases and injuries in 204 countries and territories, 1990–2019: a systematic analysis for the Global Burden of Disease Study 2019. The lancet. (2020) 396:1204–22.
18. Audirac M, Tec M, Meyers LA, Fox S, Zigler C. Impact of the timing of stay-at-home orders and mobility reductions on first-wave COVID-19 deaths in US counties. Am J Epidemiology. (2022) 191:900–7. doi: 10.1093/aje/kwac027
19. Holford TR. Understanding the effects of age, period, and cohort on incidence and mortality rates. Annu Rev Public Health. (1991) 12:425–57. doi: 10.1146/annurev.publhealth.12.1.425
20. Perales F, Todd AJSS. and Medicine, Structural stigma and the health and wellbeing of Australian LGB populations: Exploiting geographic variation in the results of the 2017 same-sex marriage plebiscite. Soc Sci Med. (2018) 208:190–9. doi: 10.1016/j.socscimed.2018.05.015
21. Solmi M, Radua J, Olivola M, Croce E, Soardo L, Salazar de Pablo G, et al. Age at onset of mental disorders worldwide: large-scale meta-analysis of 192 epidemiological studies. Mol Psychiatry. (2022) 27:281–95. doi: 10.1038/s41380-021-01161-7
22. Zhang Q, Li Q, Yang T, Chen L, Dai Y, Wei H, et al. Neurodevelopmental domain characteristics and their association with core symptoms in preschoolers with autism spectrum disorder in China: a nationwide multicenter study. BMC psychiatry. (2022) 22:393. doi: 10.1186/s12888-022-04028-5
23. Bernabe E, Marcenes W, Abdulkader RS, Abreu LG, Afzal S, Alhalaiqa FN, et al. Trends in the global, regional, and national burden of oral conditions from 1990 to 2021: a systematic analysis for the Global Burden of Disease Study 2021. (2025).
24. Murray CJ, Lopez AD. Global mortality, disability, and the contribution of risk factors: Global Burden of Disease Study. The lancet. (1997) 349:1436–42. doi: 10.1016/S0140-6736(96)07495-8
26. Wu A-M, Cross M, Elliott JM, Culbreth GT, Haile LM, Steinmetz JD, et al. Global, regional, and national burden of neck pain, 1990–2020, and projections to 2050: a systematic analysis of the Global Burden of Disease Study 2021. Lancet Rheumatol. (2024) 6:e142–55. doi: 10.1016/S2665-9913(23)00321-1
27. Murray CJL. Findings from the global burden of disease study 2021. Lancet. (2024) 403:2259–62. doi: 10.1016/S0140-6736(24)00769-4
28. Brauer M, Roth GA, Aravkin AY, Zheng P, Abate KH, Abate YH, et al. Global burden and strength of evidence for 88 risk factors in 204 countries and 811 subnational locations, 1990–2021: a systematic analysis for the Global Burden of Disease Study 2021. Lancet. (2024) 403:2162–203. doi: 10.1016/S0140-6736(24)00933-4
29. Naghavi M, Ong KL, Aali A, Ababneh HS, Abate YH, Abbafati C, et al. Global burden of 288 causes of death and life expectancy decomposition in 204 countries and territories and 811 subnational locations, 1990–2021: a systematic analysis for the Global Burden of Disease Study 2021. Lancet. (2024) 403:2100–32. doi: 10.1016/S0140-6736(24)00367-2
30. Ferrari AJ, Santomauro DF, Aali A, Abate YH, Abbafati C, Abbastabar H, et al. Global incidence, prevalence, years lived with disability (YLDs), disability-adjusted life-years (DALYs), and healthy life expectancy (HALE) for 371 diseases and injuries in 204 countries and territories and 811 subnational locations, 1990–2021: a systematic analysis for the Global Burden of Disease Study 2021. Lancet. (2024) 403:2133–61.
32. Kyu HH, Abate D, Abate KH, Abay SM, Abbafati C, Abbasi N, et al. Global, regional, and national disability-adjusted life-years (DALYs) for 359 diseases and injuries and healthy life expectancy (HALE) for 195 countries and territories, 1990–2017: a systematic analysis for the Global Burden of Disease Study 2017. Lancet. (2018) 392:1859–922.
33. Box GE, Jenkins GM, Reinsel GC, Ljung GM. Time series analysis: forecasting and control. Hoboken, NJ: John Wiley & Sons (2015).
35. Elsabbagh M, Divan G, Koh YJ, Kim YS, Kauchali S, Marcín C, et al. Global prevalence of autism and other pervasive developmental disorders. Autism Res. (2012) 5:160–79. doi: 10.1002/aur.v5.3
36. Durkin MS, Elsabbagh M, Barbaro J, Gladstone M, Happe F, Hoekstra RA, et al. Autism screening and diagnosis in low resource settings: Challenges and opportunities to enhance research and services worldwide. Autism Res. (2015) 8:473–6. doi: 10.1002/aur.2015.8.issue-5
37. Baxter AJ, Brugha T, Erskine HE, Scheurer RW, Vos T, Scott JG. The epidemiology and global burden of autism spectrum disorders. Psychol Med. (2015) 45:601–13. doi: 10.1017/S003329171400172X
38. Lai M-C, Lombardo MV, Auyeung B, Chakrabarti B, Baron-Cohen S. Sex/gender differences and autism: setting the scene for future research. J Am Acad Child Adolesc Psychiatry. (2015) 54:11–24. doi: 10.1016/j.jaac.2014.10.003
39. Li X, Wei N, Song J, Liu J, Yuan J, Song R, et al. The global burden of schizophrenia and the impact of urbanization during 1990–2019: an analysis of the global burden of disease study 2019. Environ Res. (2023) 232:116305. doi: 10.1016/j.envres.2023.116305
40. Mazza M, Pino MC, Keller R, Vagnetti R, Attanasio M, Filocamo A, et al. Qualitative differences in attribution of mental states to other people in autism and schizophrenia: what are the tools for differential diagnosis? J Autism Dev Disord. (2022) 52:1283–98. doi: 10.1007/s10803-021-05035-3
41. Schendel DE, Thorsteinsson EJJ. Cumulative incidence of autism into adulthood for birth cohorts in Denmark, 1980-2012. Jama. (2018) 320:1811–3. doi: 10.1001/jama.2018.11328
42. Bai Z, Wang H, Shen C, An J, Yang Z, Mo X. The global, regional, and national patterns of change in the burden of non-malignant upper gastrointestinal diseases from 1990 to 2019 and the forecast for the next decade. Int J Surg. (2024) 10:1097.
43. Halladay AK, Bishop S, Constantino JN, Daniels AM, Koenig K, Palmer K, et al. Sex and gender differences in autism spectrum disorder: summarizing evidence gaps and identifying emerging areas of priority. Mol Autism. (2015) 6:1–5. doi: 10.1186/s13229-015-0019-y
44. Weir E, Allison C, Baron-Cohen S. Understanding the substance use of autistic adolescents and adults: a mixed-methods approach. The Lancet Psychiatry. (2021) 8:673–85. doi: 10.1016/S2215-0366(21)00160-7
45. Lai M-C, Amestoy A, Bishop S, Brown HM, Onaiwu MG, Halladay A, et al. Improving autism identification and support for individuals assigned female at birth: clinical suggestions and research priorities. Lancet Child Adolesc Health. (2023) 7:897–908. doi: 10.1016/S2352-4642(23)00221-3
46. Horwitz E, Vos M, De Bildt A, Greaves-Lord K, Rommelse N, Schoevers R, et al. Sex differences in the course of autistic and co-occurring psychopathological symptoms in adolescents with and without autism spectrum disorder. Autism. (2023) 27:1716–29. doi: 10.1177/13623613221146477
47. Sasayama D, Kuge R, Toibana Y, Honda H. Trends in autism spectrum disorder diagnoses in Japan, 2009 to 2019. JAMA Network Open. (2021) . 4:e219234–e219234.
48. Salazar F, Baird G, Chandler S, Tseng E, O’sullivan T, Howlin P, et al. Co-occurring psychiatric disorders in preschool and elementary school-aged children with autism spectrum disorder. J Autism Dev Disord. (2015) 45:2283–94. doi: 10.1007/s10803-015-2361-5
49. Joshi G, Faraone SV, Wozniak J, Tarko L, Fried R, Galdo M, et al. Symptom profile of ADHD in youth with high-functioning autism spectrum disorder: a comparative study in psychiatrically referred populations. J Atten Disord. (2017) 21:846–55. doi: 10.1177/1087054714543368
50. Antshel KM, Russo N. Autism spectrum disorders and ADHD: Overlapping phenomenology, diagnostic issues, and treatment considerations. Curr Psychiatry Rep. (2019) 21:1–11. doi: 10.1007/s11920-019-1020-5
51. Oates M, Bean AJA. Intersecting effects of sex/gender and autism on structural language: A scoping review. Autism. (2023) 27:1876–90. doi: 10.1177/13623613221151095
52. Bourson L, Prevost CJEC, Psychiatry A. Characteristics of restricted interests in girls with ASD compared to boys: A systematic review of the literature. Eur Child Adolesc Psychiatry. (2024) 33:987–1004. doi: 10.1007/s00787-022-01998-5
53. Zwaigenbaum L, Bauman ML, Choueiri R, Fein D, Kasari C, Pierce K, et al. Early identification and interventions for autism spectrum disorder: executive summary. Pediatrics. (2015) 136:S1–9. doi: 10.1542/peds.2014-3667B
54. Tick B, Bolton P, Happé F, Rutter M, Rijsdijk F. Heritability of autism spectrum disorders: a meta-analysis of twin studies. J Child Psychol Psychiatry. (2016) 57:585–95. doi: 10.1111/jcpp.2016.57.issue-5
55. Daniels AM, Mandell DS. Explaining differences in age at autism spectrum disorder diagnosis: A critical review. Autism. (2014) 18:583–97. doi: 10.1177/1362361313480277
56. McKenzie K, Milton M, Smith G, Ouellette-Kuntz H. Systematic review of the prevalence and incidence of intellectual disabilities: current trends and issues. Curr Developmental Dis Reports. (2016) 3:104–15. doi: 10.1007/s40474-016-0085-7
Keywords: autism spectrum disorder, developmental disorder, global burden, incidence, health policy
Citation: Li D, Liang Z, Miao C, Li L and Li C (2025) Age-period-cohort analysis of autism spectrum disorders-related prevalence and DALYs: based on the Global Burden Of Disease Study 2021. Front. Psychiatry 16:1570276. doi: 10.3389/fpsyt.2025.1570276
Received: 04 February 2025; Accepted: 27 March 2025;
Published: 25 April 2025.
Edited by:
YaXuan Wang, First Affiliated Hospital of Harbin Medical University, ChinaReviewed by:
Ailton Barcelos Da Costa, Federal University of São Carlos, BrazilRidouh Abdelhakim, Centre de Recherche en Technologies Agro-Alimentaires, Algeria
Copyright © 2025 Li, Liang, Miao, Li and Li. This is an open-access article distributed under the terms of the Creative Commons Attribution License (CC BY). The use, distribution or reproduction in other forums is permitted, provided the original author(s) and the copyright owner(s) are credited and that the original publication in this journal is cited, in accordance with accepted academic practice. No use, distribution or reproduction is permitted which does not comply with these terms.
*Correspondence: Chenmu Li, NTEyMjlAZ3pzcG9ydC5lZHUuY24=; Lan Li, 18296393901@163.com
†These authors share first authorship