- Virtual Environments and Computer Graphics Group, Department of Computer Science, University College London, London, UK
Communication technologies are becoming increasingly diverse in form and functionality. A central concern is the ability to detect whether others are trustworthy. Judgments of trustworthiness rely, in part, on assessments of non-verbal cues, which are affected by media representations. In this research, we compared trust formation on three media representations. We presented 24 participants with advisors represented by two of the three alternate formats: video, avatar, or robot. Unknown to the participants, one was an expert, and the other was a non-expert. We observed participants’ advice-seeking behavior under risk as an indicator of their trust in the advisor. We found that most participants preferred seeking advice from the expert, but we also found a tendency for seeking robot or video advice. Avatar advice, in contrast, was more rarely sought. Users’ self-reports support these findings. These results suggest that when users make trust assessments, the physical presence of the robot representation might compensate for the lack of identity cues.
1. Introduction
In various types of interactions, such as advice seeking and job interviews, individuals may attempt to determine whether their communication partners are trustworthy (Mayer et al., 1995). Experiencing the consequences of misplaced trust can undermine future willingness to interact with their communication partners. However, if the interaction is mediated, trust could be affected due to the loss of some non-verbal cues. With the proliferation of cutting edge-mediated communication systems, from multiview videoconferencing units to humanlike robots [e.g., Spanlang et al. (2013)], the need to understand how their functionalities affect trust formation is critical (Bjørn et al., 2014).
As reviewed in the next section, many studies have shown the influence of media representations on trust formation [e.g., Bos et al. (2002), Nguyen (2007), and Rae et al. (2013)]. Video-mediated interaction can represent physical appearance, but it does a poor job of preserving some non-verbal cues, including eye gaze and deictic gestures that are important for trust formation (Nguyen, 2007). Compared to video-mediated interaction, avatars using computer-generated representations of the actors also provide channels for the transmission of non-verbal cues, such as gestures, postures, movements, and facial expressions (Bente et al., 2008; Weise et al., 2011; Trinh et al., 2015). Additionally, they possess the ability to systematically filter the physical appearance and behavioral actions in the eyes of the conversational partners, amplifying or suppressing features and non-verbal signals in real-time (Hyde et al., 2015). Recently, robot-mediated interaction has emerged as a viable option. These systems seek to increase the richness of the interaction and to improve communication channels by bringing them closer to face-to-face interaction (Bainbridge et al., 2011). The main distinctive feature of these systems is the physical presence of the robot.
In this paper, we compare trust development in video-, avatar-, and robot-mediated interaction. In particular, we examine how cues of expertise presented using the different media presentations affect trust. In many everyday situations, questions of trust do not arise from the risk of wilful deception, but because one is uncertain about the other’s expertise (Riegelsberger et al., 2006). We have followed previous work (Riegelsberger et al., 2006; Li et al., 2007; Pan et al., 2014) that has conceptualized trust in terms of individuals’ choice behavior in user–adviser relationships. In our experiment, participants were required to attempt to answer thirty difficult general-knowledge questions. For each question, participants could ask for advice from one of two advisers, which were presented on two different media. Unknown to the participants, one was an expert who responded with mainly correct information, and the other was a non-expert who provided mainly incorrect information. We measured participants’ advice-seeking behavior as an indicator of their trust in the adviser. Results show that participants were able to identify expert advice, but bias occurred due to media representation. There was no significant difference in advice-seeking behavior between video and robot; however, the use of an avatar negatively affected trust formation patterns. Participants’ subjective reports further supported the behavioral results. See Figure 1 for an overview of the experimental hypotheses, protocol, and results. These findings demonstrate how trust can be altered depending on representation, and they motivate the future study of mediated interaction.
2. Related Work
2.1. Trust, Non-Verbal Cues, and Mediated Communication
Trust has been defined as a willingness to be vulnerable, based on positive expectations (Mayer et al., 1995). It is a multidimensional construct that includes expertise and benevolence components. Expert-based trust refers to a rational judgment of the partner’s knowledge, competence, and dependability. Benevolence-based trust is described as an emotional bond between individuals or the confidence in the other that he/she is protective with respect to our interests and shows genuine care and concern for our welfare (Mayer et al., 1995).
Non-verbal cues, including visual cues (e.g., eye contact, head nods) and audio cues (e.g., pitch), can play an important role in the perception of trustworthiness in face-to-face situations. This is because these cues not only give information about an individual’s background (e.g., education, provenance) but also about intrinsic states, such as sincerity and confidence (Williams, 1977).
However, some non-verbal cues are lost in mediated communication [e.g., Horn et al. (2002), Nguyen (2007), and Teoh et al. (2010)]. Many theories have considered communication bandwidth and the function of non-verbal cues, such as social presence theory, cues-filtered-out and media richness theory, or social information processing theory. Despite a series of conceptual controversies and empirical disaccords, these theories at least implicitly share the assumption that the media-dependent loss of non-verbal channels influence users’ perception of trustworthiness (Bente et al., 2008).
2.2. Communication Channels
We briefly review research that specifically addresses video-, avatar-, and robot-mediated communications with a view to the characteristics of these three media representations and the influence factors for trust formation. These previous studies guided us when creating stimuli for our study.
2.2.1. Video
Videoconferencing is able to represent participants’ appearance across a distance. However, even minor physical movement of a user may introduce parallax between camera position and video display, resulting in loss of gaze awareness (Nguyen, 2007). Also, the 2D nature of standard video presents a compressed representation of 3D space, constraining the rich spatial cues common to collocated interaction, such as depth, resolution, and field of view (Steptoe et al., 2010).
Horn et al. (2002) found a non-linear relationship between video quality and lie detection performance. A slight distortion of the video signal impaired detection performance; however, the performance improved when the video was severely spatially degraded. Huang et al. (2002) found that when a videoconferencing system makes a person look artificially taller, and that person has more social impact than when the camera makes him or her look artificially shorter. Bekkering and Shim (2006) investigated the effect of eye contact in video-mediated communication on trust. Results revealed that videos that did not support eye contact resulted in lower perceived trust scores, compared to videos that enabled eye contact. Voice-mail enabled just as much trust as the video that created eye contact, perhaps because lack of eye contact cannot be perceived in audio-only communication. Nguyen (2007) showed that in group conferencing situations, the spatial arrangement of the conferencing environment affected trust in videoconferencing. They reported that gaze support and awareness were the main influencing factors on trust (Nguyen, 2007). Based on findings from this previous works, we created life-sized high-resolution video clips for our video stimuli.
2.2.2. Avatar
Avatars, artificial computer-animated representations of humans within virtual environments, are sometimes presented as simple means to enrich user experience and build trust (Yee and Bailenson, 2007; Bente et al., 2008; Steptoe et al., 2010; Junuzovic et al., 2012). The social communicative potential of avatars is similar to video, providing channels for the transmission of non-verbal cues, such as gestures, postures, movements, and facial expressions. However, the artificial nature of avatars and the non-disclosure of physical appearance and identity cues might run the risk of generating negative effects, such as loss of trust and relatedness (Bente et al., 2008).
Steptoe et al. (2010) assessed the impact of the addition of realistic eye motion in avatar-mediated communication. They found that the addition of eye movement increases participant accuracy in detecting truth and deception when interacting with virtual avatars. Hussain et al. (2011) investigated the impact that an avatar’s appearance may have on the credibility of the information it shares with Second Life® users. They observed high correlation between professional appearances in avatar design with high credibility measures. McDonnell et al. (2012) showed that small differences in rendering styles can influence perceptual judgments of computer graphic characters. Hyde et al. (2015) found that adjusting the expressiveness of interactive animated avatars may be a simple way to influence people’s social judgments and willingness to collaborate with animated avatars. The choice of an avatar and its animation techniques might have specific consequences on its own. For example, different appearances of avatars might result in different impression formation and activate social stereotypes. This implies that variations have to be included in cross media comparisons, as will be the case in the current study. We used a commercially available female Rocketbox® avatar and the Faceshift® animation software to create avatar clips.
2.2.3. Robot
Robot-mediated communication takes us one step closer to face to-face interaction by presenting the conversation partner’s motions via a physical embodiment. Some previous studies [e.g., Sakamoto et al. (2007)] have discussed the superiority of robot conferencing to videoconferencing. They showed that using a teleoperated robot that has a realistic human appearance enhances social telepresence compared with audio-only conferencing and videoconferencing (Sakamoto et al., 2007). However, it is difficult for each user to own a robot with his/her realistic appearance due to the high cost. Tanaka et al. (2014) used an anonymous teleoperated robot that had a human-like face without a specific age or gender, and compared it with life-sized communication media that reproduced the whole body of the conversational partner. They conclude that robot conferencing in the absence of presenting the remote persons appearance does not always result in superior social telepresence. Additionally, Morita et al. (2007) showed that the eye gaze of a remote person was more recognizable when reproduced by a robot than by a live video.
Bainbridge et al. (2011) explored how a robot’s physical presence affects human judgments of the robot as a social partner. They found that participants were more likely to fulfill a trust-related task when the robot was physically present, than when it was shown on live video. Rae et al. (2013) investigated the effects of two features, physical embodiment and control, on communicative and collaborative outcomes. They found that physical embodiment and control by the local user increased the amount of trust built between partners. Also, physical contact is particularly important in interactions that demand mutual cooperation and trust; it has been suggested that there can be no real trust without touch (Handy, 1999). Bevan and Stanton Fraser (2015) examined the effect of shaking hands prior to engaging in a single issue distributive negotiation, where one negotiator performed their role telepresently through a “Nao” humanoid robot. Results showed this increased cooperation between negotiators. Different from previous works (Rae et al., 2013; Bevan and Stanton Fraser, 2015), we used a life-size humanoid robot.
By integrating the above literature, it is possible to see how the characteristics of communication medium (summarized in Table 1) can impact trust formation. In this study, we investigated trust formation and discussed tradeoffs for avatar, video, and robot representations.
2.3. Measuring Trust
As a measure of trust, many studies [e.g., Bainbridge et al. (2011)] have combined behavioral observations with participants’ self-reported trust, because participants’ responses to questionnaires can be biased by factors outside of the intended experimental manipulation.
For task performance measures, a popular experimental paradigm currently employed by researchers has been social dilemma games based on the Prisoner’s Dilemma, such as the Daytrader game (Dawes, 1980). Social dilemma games vary in how difficult they are depending on the exact rules and pay off structure, but it generally takes some amount of time and some communication in order to reach the required level of trust (Bos et al., 2002; Nguyen, 2007; Rae et al., 2013). In these games, every participant is simultaneously trustor and trustee and incentives are symmetrical. However, in some everyday trust situations (e.g., a sale, acting on advice, and lending money to someone), it is an asynchronous and asymmetric exchange, where the trustor and trustee act sequentially and under different situational incentives. The Trust Game [e.g., Riegelsberger et al. (2005)] is a model of such situations. Additionally, many researchers have investigated how to maintain or increase levels of trust. However, it is also crucial to ensure that users are able to place trust correctly, avoiding overestimation of the trustworthiness of others (Riegelsberger et al., 2006).
In particular, Riegelsberger et al. (2006) compared photo/text, audio, video, and avatar communication in a quiz task with expert and non-expert advisors. In their scenario, participants were asked to participate in a quiz and financial incentives were given for good performance. The questions included in the quiz were extremely difficult, so that good performance required seeking advice. Participants had two advisers but could only ask one for each question. Thus, asking one adviser rather than the other can be understood as an indicator of trusting behavior. Their results showed that subjects sought advice from advisors significantly more through audio and video channels than text or avatar.
None of the above studies have systematically compared trust formation across avatar, video, and robot representations. In pursuing this question, we adapt previous studies to investigate how media representations affect trust. We focus on cues of expertise as a constituent of trustworthiness.
3. Experiment
We modeled our experiments on a user–adviser relationship (Riegelsberger et al., 2006). Participants were asked to answer 31 difficult general-knowledge questions, and they received chocolates depending on their performance. We gave participants two advisers presented on two different media representations. Unknown to participants, the two advisers had different levels of expertise. For each question, the participant could ask only one of the advisers for advice. Asking for advice did not cost the participants, and they did not need to ask advice for any question if they did not want to.
We measured participants’ advice-seeking behavior under risk as an indicator of trust in the adviser. People generally decide to trust others when facing situations involving risk and uncertainty. Uncertainty arises from the fact that the participants cannot directly observe the two advisers’ ability (e.g., expertise) and motivation (e.g., desire to deceive). They needed to infer those from interpersonal cues. In particular, as the questions were extremely difficult, it was unlikely that the participants would know the answer to more than one or two questions and thus would not immediately be able to tell expertise from the correctness of the answers. When recording the non-expert clips, the actor exhibited less direct eye contact. When recording the expert clips, the actor exhibited confidence through more positive facial expression (e.g., smiles) and body language. In our experiment, different media representations influence those interpersonal cues. Seeking advice from one adviser in preference over the other could be an indication of trust in that adviser, because receiving poor advice carried the risk of missing out on better advice and therefore the participant would be less likely to get the correct answer.
This study was approved by University College London Research Ethics Committee.
4. Hypotheses
We specifically make the following hypotheses based on previous findings (Kiesler et al., 2008; Bainbridge et al., 2011; Rae et al., 2013) that physical embodiment increased the amount of trust built between partners.
Hypothesis 1: for the robot vs. avatar setup, we expect that the avatar will be less trusted compared to the robot.
Hypothesis 2: for the robot vs. video setup, we expect that the video will be less trusted compared to the robot.
However, previous research (Tanaka et al., 2014) also found that robot conferencing was comparable to videoconferencing in the social telepresence, since the positive effect of the physical embodiment offset the negative effect of lacking appearance. Thus, we need to explore whether this is the case for trust formation.
Hypothesis 3: for the avatar vs. video setup, we expect that the avatar will be less trusted compared to the video.
Due to the choice of avatars, some researchers found that avatar and videoconferencing were similar with respect to user satisfaction, trust, and social presence (Bente et al., 2008), whereas others suggested that the avatar representation results in a lower level of trust than the video representation (Riegelsberger et al., 2006). Recently, many technologies, such as Faceshift, enable any user to control the facial expressions of a digital avatar in real time (Weise et al., 2011). The low cost and ease of setup of these technologies paves the way for avatar-mediated communication. Thus, it is interesting to re-evaluate how users trust assessments are affected by the use of avatar versus video representations.
5. Method
5.1. Participants
Twenty-four participants (12 males), students and staff at University College London, were recruited to take part in our user study. The median age was 23.25 (SD = 3.30). None of the participants had interacted with the actor in the video clips, the robot, or the animated character before.
5.2. Materials
5.2.1. Questions
We used 31 questions and answers in total: a main body of 30 with equal stakes and then a single final high-stakes question (Riegelsberger et al., 2006). The participant was offered four answers to choose from them. The questions are difficult general-knowledge questions, to minimize effects of participants’ prior knowledge. Examples of questions that were included are “What subject did Bill Gates study at Harvard?” and “How many US Presidents have been assassinated?” Based on the pretest results, the mean probability for pretesters giving a correct answer was 0.31 (SD = 0.11). This value was only marginally above chance (0.25), indicating that very difficult questions had been picked.
5.2.2. Expertise
The non-expert and expert advisors were created by recording advice from the same female actor before and after training, respectively. Thus, the expert and non-expert advisors only differed in the ratio of correct to incorrect advice and their interpersonal cues of expertise. Because for each question, the observer only had access to one of the advisers, they were unaware that both advisers were, in fact, the same individual recorded at different levels of expertise. For the non-expert adviser, the proportion of correct (i.e., confident) advice was 0.36. For the expert adviser, the proportion of correct (i.e., confident) advice was 0.93. Two incorrect (and less confident) pieces of advice from the untrained recording were added to the expert, in order to avoid artificial perfection.
5.2.3. Media Representations
The participant observes the prerecorded clips on different media representations. The participants were primed that the avatar and robot were representing someone, but were not autonomous and did not react to the user.
For the video representation (see Figures 2A,B), we used a Sony® NEX-3 video camera to record the actor standing in a natural environment with black background. We placed the camera 90 cm in front of the actor and adjusted the camera to look at the actor’s eyes, so that both entire upper-body and gaze cues could be captured. We then used an Optoma® ML1500e ultra-slim portable LED projector to project a life-sized image with a native resolution of 1280 × 800 pixels.
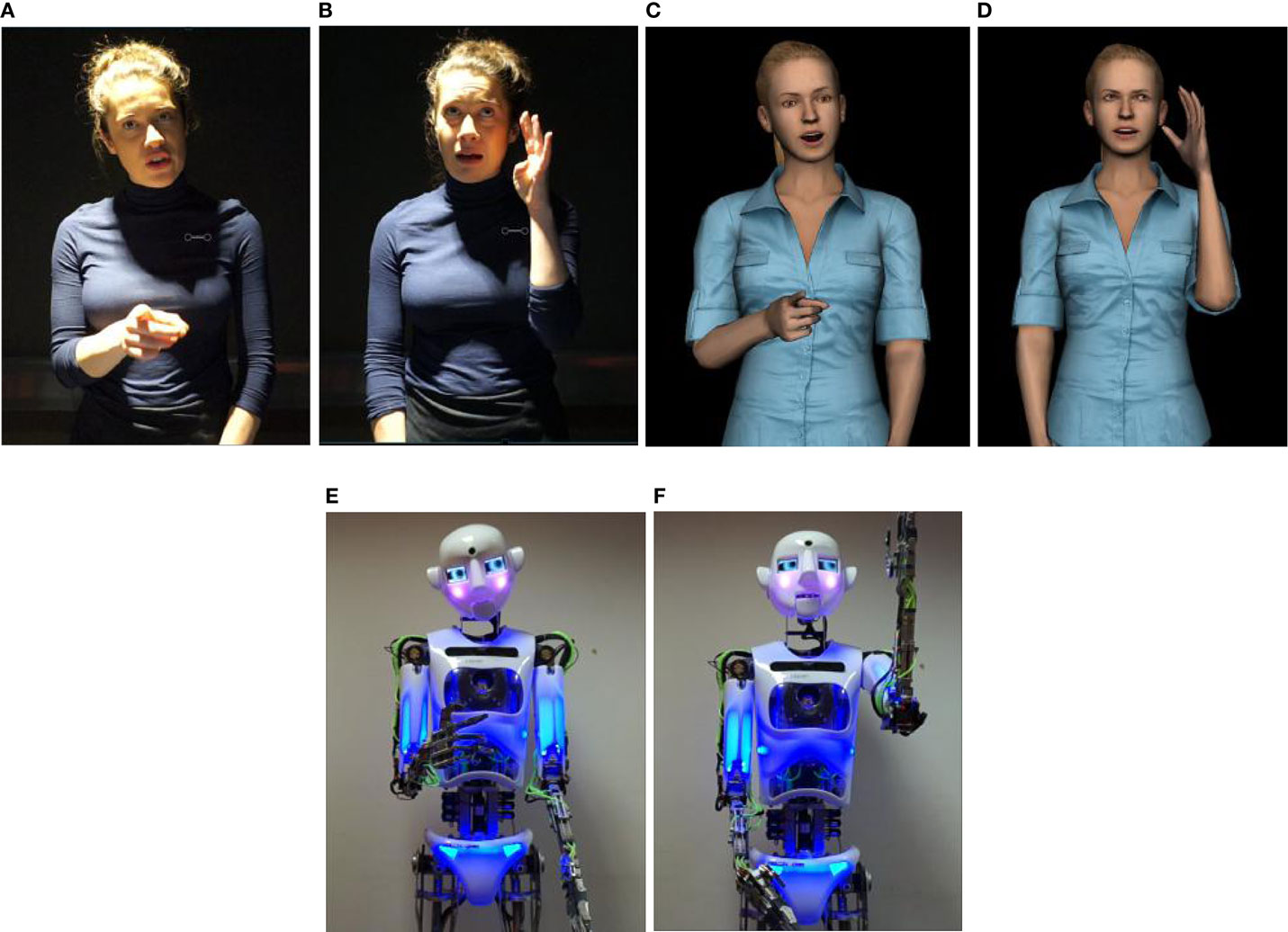
Figure 2. Examples of stimuli for different media representations and expertise. (A) Video expert, (B) video non-expert, (C) avatar expert, (D) avatar non-expert, (E) robot expert, and (F) robot non-expert.
For the avatar representation (see Figures 2C,D), we used Faceshift® with a Microsoft Xbox 360 Kinect™ to capture our actor’s facial performances and represent this on a Rocketbox® avatar. We set the Microsoft Kinect right above the video camera to simultaneously record the actor. The Microsoft Kinect captures a 640 × 480 2D color image and a 3D depth map at 30 Hz. Faceshift analyzes the facial movements of an actor and describes them as a mixture of basic expressions, plus head orientations, and eye gaze. This description is used to animate virtual characters. With the embedded plug-in of Faceshift in Maya®, we recorded the facial animations. We then used keyframe animations to animate the entire upper-body of the avatar. The clips of video of the avatar were projected in the same way, as the video clips discussed above.
For the robot representation (see Figures 2E,F), we used a commercial off-the-shelf robotic humanoid called the Robothespian™ from Engineered Arts. We used the Virtual Robothespian software to create the animation. Animations for the robot were created by importing the keyframes for the avatar animations. The Virtual Robothespian software then exports a sequence of control messages for the real robot that makes a physical animation as close as possible to the virtual avatar animation, given the joint structure and dynamics of the robot. The robot’s abilities include head movement, arm movements, finger flex (all four fingers together), lips movement, and eye graphics.
All media representations used the same audio tracks, which ranged from 1 to 8 s long. Since gender can influence trust in computer recommendation systems (Nass et al., 1997), we ensured that the advisors are female for all media representations (e.g., the same female audio tracks, female names). The sizes of the representations of the actor were made consistent in each media, by making sure that the video and avatar clips were projected at the same size, and at the same eye level, as the robot. The colors (e.g., blue clothing, plain background) used in each of the representations were made consistent as well. Image quality (e.g., resolution) was the same in both avatar and video representations.
5.2.4. Setup
The participant stood in front of a table about 2.5 m from the screen (see Figure 3). We ensured that the participants viewing distances to the two media representations were the same. We also ensured that the vertical alignment of the eye level of the participant and the eye level of the advisor were the same across the different media representations.
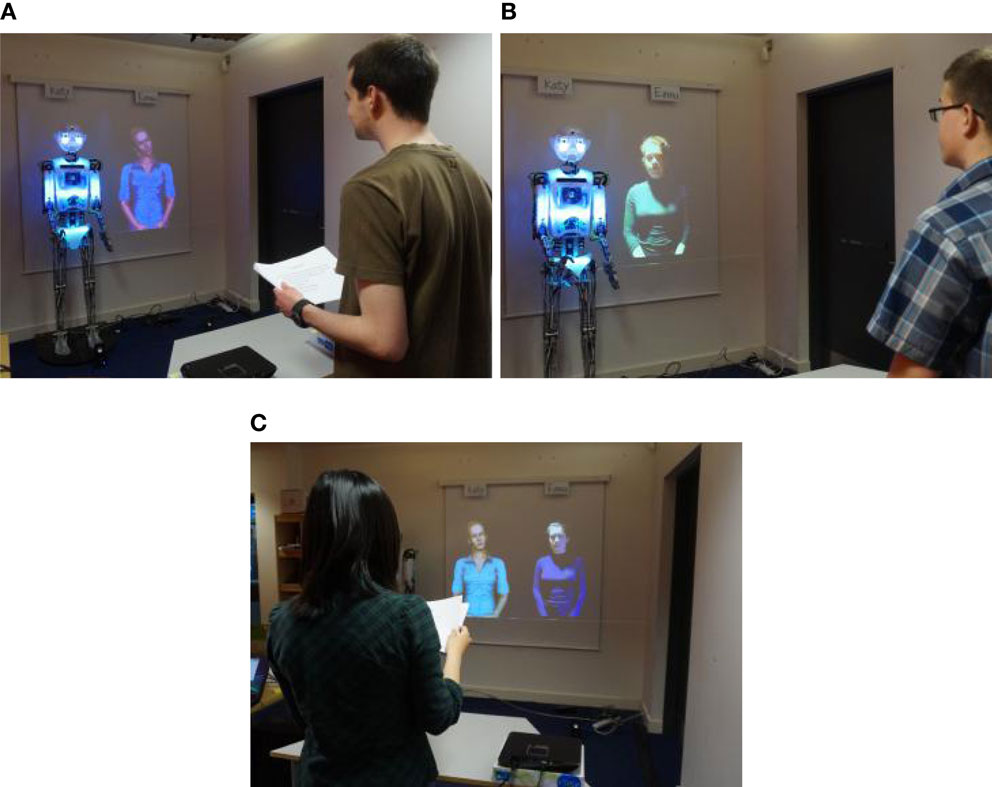
Figure 3. Pictures of experiment setup for different experimental conditions. (A) Robot vs. avatar, (B) robot vs. video, and (C) avatar vs. video.
5.2.5. Incentives and Risk
The number of chocolates that participants received was linked to the number of correctly answered questions. For the 30 assessed questions, the number of chocolates varied between one and six. A final high-stakes question was worth an additional three chocolates.
5.3. Design
The study had 6 between-subject conditions. In each condition, two advisors, one of which gave expert advice, whereas the other gave non-expert advice, were presented using two of the three possible media representations (robot, avatar, and video). Figure 1 shows our six experimental conditions, including robot vs. avatar (robot is expert), robot vs. avatar (avatar is expert), robot vs. video (robot is expert), robot vs. video (video is expert), avatar vs. video (avatar is expert), and avatar vs. video (video is expert) conditions.
The order of the questions and answer options (A–D) was randomized; the position (left, right) of media representations and names (Katy, Emma) of the advisors were counterbalanced.
5.4. Procedure
To moderate the effect introduced by evaluating a novel media representation, we asked each participant to complete a practice round prior to starting the assessed part of the experiment. For these, both advisors gave identical and correct advice. Then, participants answered 30 assessed questions, followed by a final high-stakes question. For each question, participants could ask for advice from one of the two advisers without knowing the adviser’s expertise. Next, they were presented with the postexperimental questionnaire and the open question, eliciting their subjective assessment of the advisors. Finally, the participants were compensated with chocolates based on their performance. The experiment took about 20 min.
5.5. Scoring
5.5.1. Task Performance Measure
We defined the advice-seeking rate to be the proportion of advice sought from an advisor out of the total number of times advice was sought by a participant. We did not include the no query situations, and only focus on the difference between the two advisers. This is because the focus of the experiment is on the variance of this difference due to the six experimental conditions. Also, presenting the data in this way simplifies the data analysis. As each participant had two advisers, but could only choose one of them to ask for advice on each question, the following relationships hold: expert advice-seeking rate = 1 − non-expert advice-seeking rate; one media representation’s advice-seeking rate = 1 − the other media representations.
5.5.2. Postquestionnaire
Participants presented with a postexperimental questionnaire with 6 items measuring trustworthiness (see Table 2) eliciting their subjective assessment of the two advisers. Agreement with the statements was elicited on 7-point Likert scales with the anchors 1 (Strongly disagree) to 7 (Strongly agree).
5.5.3. Open Question
Upon completion of the experiment, we interviewed each participant with an open question: “please describe how you decided which adviser to rely on.” The purpose of this open question was to understand participants’ general impressions of the advisers, any specific incidents in the experiment that stood out (e.g., the decision-making processes for the final high-stakes question), and any strategies that they used etc.
6. Results
6.1. Behavioral Measurements
6.1.1. Advice-Seeking Rate over Time
For 30 assessed questions, Participants sought advice on 27.42 out of 30 questions (91.39%), on average. Six participants (25%) sought advice for every question.
We analyzed how participants’ advice-seeking behavior changed over time. Figure 4 presents the mean advice-seeking rate of every five questions in chronological order. The participant should choose randomly between the two at the start, if the media representations were equally attractive. Then, the choice to seek advice from a specific adviser could be expected to depend upon the information (e.g., interpersonal cues) accumulated from previous pieces of advice. Figure 4 shows that participants would increasingly seek advice from the expert as they gained experience with the advisers.
We can interpret the high solid blue line as indicating that in the robot vs. avatar condition, either the participants were better at spotting the expert and thus preferentially sought its advice or that they generally preferred the robot to the avatar and thus were biased toward seeking advice from the robot. Conversely, the solid red line can be interpreted as indicating that despite the avatar being the expert, it is not questioned consistently until the final five questions. Thus, either the participants have trouble perceiving that the avatar was the expert or they are biased against the avatar toward the video. Given that the verbal and gestural content is very similar between the different media conditions, both interpretations are interesting.
A 6 experimental conditions × 6 time (batches of 5 questions, see Figure 4) mixed design ANOVA was conducted on the mean expert advice-seeking rate of every five questions, with experimental conditions as between-subjects factors and time as a within-subjects factor. There were no outliers in the data, as assessed by inspection of a boxplot for values >1.5 box lengths from the edge of the box. The expert advice-seeking rate was normally distributed for all experimental conditions at all time points, as assessed by Shapiro–Wilk’s test, p > 0.05. There was homogeneity of variances, as assessed by Levene’s test of homogeneity of variance, p > 0.05. Mauchly’s test indicated that the assumption of sphericity had not been violated, χ(14) = 11.383, p > 0.05.
The result revealed that there was no statistically significant interaction between the experimental conditions and time, F(25,90) = 0.326, p = 0.999. Therefore, we only need to concentrate on the main effects and main effects’ post hoc tests.
The main effect of the experimental conditions showed that there was a statistically significant difference in the mean advice-seeking rate of every five questions between these conditions, F(5,18) = 7.279, p = 0.001. Post hoc comparisons using the Tukey HSD test revealed that the mean expert advice-seeking rate for robot vs. avatar (robot is expert) condition was significantly higher than robot vs. avatar (avatar is expert) condition, p = 0.025. This supports hypothesis 1. The mean expert advice-seeking rate for the avatar vs. video (video is expert) condition was significantly higher than the avatar vs. video (avatar is expert) condition, p = 0.005. This supports hypothesis 3. However, there was no statistically significant difference in mean expert advice-seeking rate between the robot vs. video (robot is expert) condition and the robot vs. video (video is expert) condition, p = 0.983. This violates hypothesis 2.
The main effect of time showed a statistically significant difference at the different time points, F(5,90) = 5.45, p < 0.001.
6.1.2. Average Advice-Seeking Rate
Figure 5 shows the mean expert advice-seeking rate and the mean non-expert advice-seeking rate for the six experimental conditions. The overall expert advice-seeking rate (M = 0.615, 95% CI [0.575, 0.655]), was approximately 20% higher than the overall non-expert advice-seeking rate. Figure 5 also shows biases in the robot vs. avatar setup (left two blue bars) and the avatar vs. video setup (right two blue bars), but not in the robot vs. video setup (middle two blue bars), indicating that expert advice-seeking rate was different in that condition.
We investigated the non-expert advice-seeking rate for the six experimental conditions (unshaded red bar in Figure 5). A non-expert advice-seeking rate <0.5 would provide evidence for users’ ability to discriminate between expert and non-expert advisers, whereas a value >0.5 would be a sign of bias outweighing discrimination. Based on a one-sample t-test, the non-expert advice-seeking rate conditions were significantly below 0.5 for the following conditions: robot vs. avatar (robot is expert), robot vs. video (robot is expert), robot vs. video (video is expert), and avatar vs. video (video is expert), with t(3) = −12.187, p = 0.001; t(3) = −3.612, p = 0.036; t(3) = −3.465, p = 0.041; and t(3) = −6.527, p = 0.007, respectively. However, no such effect is presented for robot vs. avatar (avatar is expert) and avatar vs. video (avatar is expert) conditions with t(3) = −0.900, p > 0.05 and t(3) = 0.379, p > 0.05, respectively. Participants took longer and found it more difficult (see Figure 4) to identify the avatar as the expert. This indicated a bias in users’ ability to discriminate.
6.1.3. Final High-Stakes Question
We then explored advice-seeking behavior for the final high-stakes question. This question was worth an additional three chocolates, thus it has a subjectively important and risky outcome. As previously discussed, the longer participants spend with advisors, the more likely they are to have figured out who knows more answers. In the avatar vs. video (avatar is expert) condition, the expert advice-seeking rate had already reached nearly 60% by the final five questions (see Figure 4); however, for the final high-stakes question, the preference for seeking video advice almost matched the preference for avatar advice (see Figure 6).
6.2. Participants’ Self-Reports
We investigated participants’ subjective assessment of the two advisers (see Table 2). We calculated Cronbach’s α as the reliability test. The questionnaire measured the trustworthiness of the expert adviser (6 items, α = 0.871), and the trustworthiness of the non-expert adviser (6 items, α = 0.879).
We then compared self-reported trust measures by experimental conditions. The means are presented in Figure 7. A 6 experimental conditions × 2 expertise mixed design ANOVA was conducted on the participants’ self-reported score, with experimental conditions as between-subjects factors and expertise as a within-subjects factor. First, the main effect of expertise has two levels; therefore, the assumption of sphericity is not an issue. Results revealed that the mean score of the expert adviser was significantly higher than that of the non-expert adviser, F(1,18) = 68.751, p < 0.001. This result matches the results of the behavioral measurements that the participant generally would seek more advice from the expert adviser than the non-expert adviser. Second, the main effect of experimental conditions was not significant, F(5,18) = 1.414, p > 0.05. However, the experimental conditions × expertise interaction was significant, F(5,18) = 4.48, p = 0.008. This indicated that participants’ self-reported score due to experimental conditions were presented differently for expert and non-expert advisers. Since our results have a statistically significant interaction, reporting the main effects can be misleading, so we further explored the difference between experimental conditions at each level of expertise. Based on a paired samples t-test, the participants’ self-reported trust score for the non-expert adviser were significantly lower than the expert adviser in robot vs. avatar (robot is expert), robot vs. video (robot is expert), robot vs. video (video is expert), and avatar vs. video (video is expert) conditions, with t(3) = −4.656, p = 0.019; t(3) = −5.101, p = 0.015; t(3) = −5.355, p = 0.013; and t(3) = −4.073, p = 0.027, respectively. However, there were no significant differences between expert adviser and non-expert adviser in robot vs. avatar (avatar is expert) and avatar vs. video (avatar is expert) conditions with t(3) = −2.504, p > 0.05 and t(3) = −0.219, p > 0.05, respectively. This result also matched the findings for participant’s advice-seeking behavior shown in Figure 5.
7. Discussion
We compared the advice-seeking rate for six experimental conditions. We found that participants mostly chose expert advice for all conditions. This indicates that participants were able to discriminate between experts and non-experts, and accordingly, distributed more trust to the expert. However, there was also evidence that media representation can interfere with participants’ ability to discriminate effectively, as discussed below. Note that there are relatively few subjects in the experiment, so verbal reports should be taken as indicative of issues to explore.
7.1. Video
For the avatar vs. video setup, when the non-expert was represented via video, in many cases users’ preference for receiving video advice led them to disregard better advice from the avatar. This preference for video is particularly problematic, as it interfered with their ability to detect expertise. It might be because only video can present physical appearance and real dynamic visual interpersonal cues. In the words of one participant:
Katy (non-expert video) revealed her identity, so I trust her more.
Another interesting response was the following:
From the tone of voice, Emma (expert avatar) seems always certain about the answer, but Katy (non-expert video) seems to tell what she knows. But for the high stake question, I could look at Katy’s more detailed facial expressions while she answered. I guessed I could tell whether she is certain or not. So I chose Katy. If she is certain, I would follow her advice; if she is not, I would decide myself.
This indicated this participant believed that video would give them the more detailed insight into expertise, and thus explained participants’ advice-seeking behavior for the final high-stakes question. It is also supported by previous research (Horn et al., 2002; Riegelsberger et al., 2006) that participants would over-estimate their own ability in detecting lies or untrustworthy actors over video.
In this experiment, we placed the camera to directly look at the actors’ eyes while recording these video clips. However, we noted that spatial distortions and consequent loss of gaze reciprocity would apply when using standard videoconferencing systems. According to the previous research (Nguyen, 2007), this would reduce levels of trust. Therefore, for social interactions, the impact of spatial distortions should be considered before choosing video teleconferencing.
7.2. Avatar
For both robot vs. avatar and avatar vs. video setups, the avatar adviser generated a negative bias. The subjective assessments also corroborate the notion of a negative bias resulting from the avatar. One participant directly commented:
Emma (avatar expert) didn’t seem real. I don’t trust her.
Our findings indicate that due to masked identity and synthetic visual cues, avatars might not support trust building. However, these findings cannot necessarily be generalized to other avatar representations or contexts of use. The avatar used in our experiment was created with simple off-the-shelf tools and presented on a 2D screen. If the avatar had been in a 3D virtual environment (e.g., through a head mounted display) with more spatial information, the avatar advisor might have appeared to be more trustworthy. Also, many studies (Garau et al., 2001) have demonstrated strong differences in reactions to animated characters due to relatively small differences in behavior, appearance, or context of use.
7.3. Robot
For avatar vs. robot setup, participants had a tendency for seeking robot advice that interfered with participants’ preference for expert advice. This media bias could be caused by many factors. First, the robot resembles the avatar in transmitting user’s body motions without disclosing the user’s appearance, but differs in reflecting these movements onto a physical embodiment, instead of a computer graphics animation. Many previous studies (Bainbridge et al., 2011; Rae et al., 2013) showed that physical embodiment would increase the amount of trust built between partners. Second, compared with distributed teams or remote partners, colocated teams have less trouble developing trust, coordinating work, resolving conflict, and engendering high motivation (Hinds and Bailey, 2003). Third, it was also reported that social telepresence was enhanced when the remote person’s movement was augmented by a display’s physical movement (Adalgeirsson and Breazeal, 2010; Nakanishi et al., 2011; Sirkin, 2012). Finally, in robot-mediated communication, the depth from motion parallax could increase the visibility of body motions. The lack of the depth information might have made it hard for users to see facial movements of the avatar. Our postexperimental open question further supports our findings:
I feel Emma (robot non-expert) close to me. Hence, I chose Emma more times.
Emma (robot expert) is confident, expressive and influential. I feel she is reliable.
Participants’ answers also show that there were other factors influencing their decision-making:
Robot must be intelligent. So I tend to ask Emma (robot expert) more.
However, for the robot vs. video setup, no such bias was found. In this case, enhanced trust in the physical embodiment may have offset decreased trust due to the hiding of the partner’s identity. This may imply the possibility to increase the level of trust by using a robot that has a realistic appearance. In order to do this, we could use a robot system, such as Geminoid HI-1, Animatronic Shader Lamps Avatars (SLA) (Lincoln et al., 2011), or a screen-based telepresence robot. However, the uncanny valley hypothesis (Mori et al., 2012) suggests that care should be taken to avoid negative reactions.
Furthermore, two participants reported that the robot was distracting because of rigid and frozen postures. Additionally, in financial terms, robots are more expensive than videos and avatars. Nevertheless, we did observe a benefit of the physical presence of the robot.
7.4. Other Points
We acknowledge that there were other factors that might influence participants decision-making. For example, if participants knew the answer to the question and either of the advisers also told them the matched answer, the participants might tend to ask this advisor more. To moderate this effect, the questions we used were extremely difficult and thus relied on the participants’ ability to identify the expert advisor through the interpersonal cues.
We used chocolates as incentives and all the participants took the chocolates at the end of the experiments. However, we acknowledge that if participants are on a diet, or not fond of chocolates, they might have been less motivated than someone else who liked chocolates or was hungry. In related economic games [e.g., Bos et al. (2002) and Nguyen (2007)], researchers found significant effects based on the relative wealth or poverty of the players, where poorer players were more willing to accept unfair amounts of money because they needed the money more than wealthier players. Although none of our participants has reported these effects in this experiment, motivation and compensation should be taken into consideration when designing future studies.
Conclusion
In this study, we compared how the features of video, avatar, and robot representations influence trust formation in remote communication. Unlike previous work we attempted to fairly compare all three technologies. We analyzed participants’ advice-seeking behavior in a situation of limited advice and risk as an indicator of trust. We found that participants mainly sought advice from the expert advisor. However, bias occurred when advice was preferred due to its media representation. Participants were less likely to choose advice from the avatar, irrespective of whether or on the avatar was expert. Users’ self-reports are in agreement with behavioral findings. The avatar was rated lowest in trust assessment, whereas the robot and video were rated similarly.
Users, including system developers, might have strong preferences for video, avatar, and robot, based on esthetic or technical characteristics. However, we believe that it is important to explore how such systems can be compared in order to determine which may be more appropriate. For example, there is a temptation to reduce cost and maintenance by using avatar- or video-mediated communication systems. However, our findings suggest that designers and investigators should consider that the physical presence of robots appears to positively influence trust and thus might positively affect other aspects of social interaction.
This study mainly looked at cues for expertise in the context of a general-knowledge quiz. Future studies could usefully employ a similar paradigm to research media effects for cues of motivation (e.g., wilful deception) in different trust-requiring situations.
Author Contributions
YP wrote the code, ran the study, analyzed the data, and wrote the paper. AS posed the original hypothesis and supervised YP through each stage of the work including writing the code, running the study, analyzing the data, and writing the paper.
Conflict of Interest Statement
The authors declare that the research was conducted in the absence of any commercial or financial relationships that could be construed as a potential conflict of interest.
Acknowledgments
The authors would like to acknowledge UCL internal funding. We thank the UCL VECG group members for their general support including proofreading.
References
Adalgeirsson, S. O., and Breazeal, C. (2010). “Mebot: a robotic platform for socially embodied presence,” in HRI (Osaka: IEEE Press), 15–22.
Bainbridge, W. A., Hart, J. W., Kim, E. S., and Scassellati, B. (2011). The benefits of interactions with physically present robots over video-displayed agents. Int. J. Soc. Rob. 3, 41–52. doi:10.1007/s12369-010-0082-7
Bekkering, E., and Shim, J. (2006). Trust in videoconferencing. Commun. ACM 49, 103–107. doi:10.1145/1139922.1139925
Bente, G., Rüggenberg, S., Krämer, N. C., and Eschenburg, F. (2008). Avatar-mediated networking: increasing social presence and interpersonal trust in net-based collaborations. Hum. Commun. Res. 34, 287–318. doi:10.1111/j.1468-2958.2008.00322.x
Bevan, C., and Stanton Fraser, D. (2015). “Shaking hands and cooperation in tele-present human-robot negotiation,” in HRI (Portland: ACM) 247–254.
Bjørn, P., Esbensen, M., Jensen, R. E., and Matthiesen, S. (2014). Does distance still matter? Revisiting the CSCW fundamentals on distributed collaboration. ACM Trans. Comput. Hum. Interact. 21, 1–27. doi:10.1145/2670534
Bos, N., Olson, J., Gergle, D., Olson, G., and Wright, Z. (2002). “Effects of four computer-mediated communications channels on trust development,” in SIGCHI (Minneapolis: ACM), 135–140.
Dawes, R. M. (1980). Social dilemmas. Annu. Rev. Psychol. 31, 169–193. doi:10.1146/annurev.ps.31.020180.001125
Garau, M., Slater, M., Bee, S., and Sasse, M. A. (2001). “The impact of eye gaze on communication using humanoid avatars,” in SIGCHI (Seattle: ACM), 309–316.
Hinds, P. J., and Bailey, D. E. (2003). Out of sight, out of sync: understanding conflict in distributed teams. Organ. Sci. 14, 615–632. doi:10.1287/orsc.14.6.615.24872
Horn, D. B., Karasik, L., and Olsen, J. S. (2002). “The effects of spatial and temporal video distortion on lie detection performance,” in SIGCHI (Minneapolis: ACM), 714–715.
Huang, W., Olson, J. S., and Olson, G. M. (2002). “Camera angle affects dominance in video-mediated communication,” in CHI’02 Extended Abstracts on Human Factors in Computing Systems (Minneapolis: ACM), 716–717.
Hussain, M., Nakamura, B., and Marino, J. (2011). “Avatar appearance & information credibility in second life®,” in Proceedings of the 2011 iConference (Seattle: ACM), 682–683.
Hyde, J., Carter, E. J., Kiesler, S., and Hodgins, J. K. (2015). “Using an interactive avatar’s facial expressiveness to increase persuasiveness and socialness,” in Proceedings of the 33rd Annual ACM Conference on Human Factors in Computing Systems (Seoul: ACM), 1719–1728.
Junuzovic, S., Inkpen, K., Tang, J., Sedlins, M., and Fisher, K. (2012). “To see or not to see: a study comparing four-way avatar, video, and audio conferencing for work,” in Proceedings of the 17th ACM International Conference on Supporting Group Work (Sanibel Island: ACM), 31–34.
Kiesler, S., Powers, A., Fussell, S. R., and Torrey, C. (2008). Anthropomorphic interactions with a robot and robot-like agent. Soc. Cogn. 26, 169–181. doi:10.1521/soco.2008.26.2.169
Li, I., Forlizzi, J., Dey, A., and Kiesler, S. (2007). “My agent as myself or another: effects on credibility and listening to advice,” in Proceedings of the 2007 Conference on Designing Pleasurable Products and Interfaces (Helsinki: ACM), 194–208.
Lincoln, P., Welch, G., Nashel, A., State, A., Ilie, A., and Fuchs, H. (2011). Animatronic shader lamps avatars. Virtual Real. 15, 225–238. doi:10.1007/s10055-010-0175-5
Mayer, R. C., Davis, J. H., and Schoorman, F. D. (1995). An Integrative Model of Organizational Trust. Academy of Management Review, 709–734.
McDonnell, R., Breidt, M., and Bülthoff, H. H. (2012). Render me real?: investigating the effect of render style on the perception of animated virtual humans. ACM Trans. Graph. 31, 91. doi:10.1145/2185520.2185587
Mori, M., MacDorman, K. F., and Kageki, N. (2012). The uncanny valley [from the field]. IEEE Robot. Autom. Mag. 19, 98–100. doi:10.1109/MRA.2012.2192811
Morita, T., Mase, K., Hirano, Y., and Kajita, S. (2007). “Reciprocal attentive communication in remote meeting with a humanoid robot,” in Proceedings of the 9th International Conference on Multimodal Interfaces (Nagoya: ACM), 228–235.
Nakanishi, H., Kato, K., and Ishiguro, H. (2011). “Zoom cameras and movable displays enhance social telepresence,” in SIGCHI (Vancouver, BC: ACM), 63–72.
Nass, C., Moon, Y., and Green, N. (1997). Are machines gender neutral? Gender-stereotypic responses to computers with voices. J. Appl. Soc. Psychol. 27, 864–876. doi:10.1111/j.1559-1816.1997.tb00275.x
Nguyen, D. T. and Canny, J. (2007). “Multiview: improving trust in group video conferencing through spatial faithfulness,” in SIGCHI (San Jose: ACM), 1465–1474.
Pan, Y., Steptoe, W., and Steed, A. (2014). “Comparing flat and spherical displays in a trust scenario in avatar-mediated interaction,” in SIGCHI (Toronto: ACM), 1397–1406.
Rae, I., Takayama, L., and Mutlu, B. (2013). In-body experiences: embodiment, control, and trust in robot-mediated communication. Interaction 15, 36. doi:10.1145/2470654.2466253
Riegelsberger, J., Sasse, M. A., and McCarthy, J. D. (2005). The mechanics of trust: a framework for research and design. Int. J. Hum. Comput. Stud. 62, 381–422. doi:10.1016/j.ijhcs.2005.01.001
Riegelsberger, J., Sasse, M. A., and McCarthy, J. D. (2006). “Rich media, poor judgement? A study of media effects on users trust in expertise,” in People and Computers XIX – The Bigger Picture (London: Springer), 267–284.
Sakamoto, D., Kanda, T., Ono, T., Ishiguro, H., and Hagita, N. (2007). “Android as a telecommunication medium with a human-like presence,” in HRI (Arlington: IEEE), 193–200.
Sirkin, D. and Ju, W. (2012). “Consistency in physical and on-screen action improves perceptions of telepresence robots,” in HRI (Boston: ACM), 57–64.
Spanlang, B., Navarro, X., Normand, J.-M., Kishore, S., Pizarro, R., and Slater, M. (2013). “Real time whole body motion mapping for avatars and robots,” in VRST (Singapore: ACM), 175–178.
Steptoe, W., Steed, A., Rovira, A., and Rae, J. (2010). “Lie tracking: social presence, truth and deception in avatar-mediated telecommunication,” in SIGCHI (Atlanta: ACM), 1039–1048.
Tanaka, K., Nakanishi, H., and Ishiguro, H. (2014). “Comparing video, avatar, and robot mediated communication: pros and cons of embodiment,” in Collaboration Technologies and Social Computing (Berlin: Springer), 96–110.
Teoh, C., Regenbrecht, H., and O’Hare, D. (2010). “Investigating factors influencing trust in video-mediated communication,” in OzCHI (Brisbane: ACM), 312–319.
Trinh, H., Ring, L., and Bickmore, T. (2015). “Dynamicduo: co-presenting with virtual agents,” in SIGCHI (Seoul: ACM), 1739–1748.
Weise, T., Bouaziz, S., Li, H., and Pauly, M. (2011). Realtime performance-based facial animation. ACM Trans. Graph. 30, 1–10. doi:10.1145/2010324.1964972
Williams, E. (1977). Experimental comparisons of face-to-face and mediated communication: a review. Psychol. Bull. 84, 963. doi:10.1037/0033-2909.84.5.963
Keywords: computer supported collaborative work, video, avatar, robot, trust, expertise
Citation: Pan Y and Steed A (2016) A Comparison of Avatar-, Video-, and Robot-Mediated Interaction on Users’ Trust in Expertise. Front. Robot. AI 3:12. doi: 10.3389/frobt.2016.00012
Received: 15 January 2016; Accepted: 11 March 2016;
Published: 29 March 2016
Edited by:
Daniel Thalmann, Nanyang Technological University, SingaporeReviewed by:
Daniel Keefe, University of Minnesota, USAVictoria Interrante, University of Minnesota, USA
Copyright: © 2016 Pan and Steed. This is an open-access article distributed under the terms of the Creative Commons Attribution License (CC BY). The use, distribution or reproduction in other forums is permitted, provided the original author(s) or licensor are credited and that the original publication in this journal is cited, in accordance with accepted academic practice. No use, distribution or reproduction is permitted which does not comply with these terms.
*Correspondence: Ye Pan, eS5wYW5AY3MudWNsLmFjLnVr