The potential of robot eyes as predictive cues in HRI—an eye-tracking study
- 1Technische Universität Berlin, Berlin, Germany
- 2HFC Human-Factors-Consult GmbH, Berlin, Germany
- 3Land in Sicht—PROWO gGmbH, Eberswalde, Germany
Robots currently provide only a limited amount of information about their future movements to human collaborators. In human interaction, communication through gaze can be helpful by intuitively directing attention to specific targets. Whether and how this mechanism could benefit the interaction with robots and how a design of predictive robot eyes in general should look like is not well understood. In a between-subjects design, four different types of eyes were therefore compared with regard to their attention directing potential: a pair of arrows, human eyes, and two anthropomorphic robot eye designs. For this purpose, 39 subjects performed a novel, screen-based gaze cueing task in the laboratory. Participants’ attention was measured using manual responses and eye-tracking. Information on the perception of the tested cues was provided through additional subjective measures. All eye models were overall easy to read and were able to direct participants’ attention. The anthropomorphic robot eyes were most efficient at shifting participants’ attention which was revealed by faster manual and saccadic reaction times. In addition, a robot equipped with anthropomorphic eyes was perceived as being more competent. Abstract anthropomorphic robot eyes therefore seem to trigger a reflexive reallocation of attention. This points to a social and automatic processing of such artificial stimuli.
1 Introduction
Industrial collaborative robots, or cobots for short, interact in direct temporal and physical proximity with a human partner (Restrepo et al., 2017). The accompanying elimination of safety barriers creates new requirements for coordination and action prediction between humans and robots. To date, however, cobots only provide limited information about future motion sequences, making it a hard task for humans to coordinate their behavior around the cobot–especially compared to how easy it is for humans to coordinate their interpersonal behavior. Explicit predictive cues would seem to be a good idea to make the robot’s movements easier to understand. Yet, compared to social robots or other service robots, the design space offered by industrial cobots is quite a narrow one, as it is bounded rather by the specifications of the industrial task, performance metrics and a functional design, than by the affordances of a fluent human-robot interaction (HRI). If we want to implement predictive cues, we argue they have to meet at least three requirements. First, their implementation must not conflict with the robot’s performance: e.g., Faria et al. (2021) proposed a solution to make robotic movements more legible to the operator, but this was at the expense of extra costs in motion planning. Second, the predictive cues need to fit into the functionalist design scope. Thus, a simple, straight forward approach would come to mind, like the use of arrows on a screen to indicate motion intention of a mobile robot (Shrestha et al., 2016), or projected arrows on the ground (Hetherington et al., 2021). Another design option that has been explored in this regard are moving lightbands to indicate motion intents of a mobile factory robot (Bacula et al., 2020). Third, as industrial human-robot coordination is not the main part of task fulfillment but rather a means to an end, the predictive cues should trigger resource-efficient mechanisms that do not require additional cognitive resources (Neider et al., 2010). This means that humans’ attention shifts required to predict the robotic motion should happen as effortlessly as possible, i.e., automatically (Onuki et al., 2013; Khoramshahi et al., 2016). Arrows as indicators for robot movements might not fulfill this requirement as the interpretation of these cues needs an active consideration and therefore additional cognitive resources.
To find a solution integrating all these requirements, we think that functional anthropomorphic features, i.e., abstract forms of anthropomorphism that only aim to mimic certain functional aspects of human-likeness, are a promising suspect (Onnasch and Roesler, 2021). One such feature is the attention directing function of eyes and gaze. In human interaction, eye gaze is a key mechanism to engage in joint attention, which describes an automatic reallocation of one’s attention to an object that another individual is attending to (Shepherd, 2010). This, in turn, enables us to understand, predict and adapt to the situation. The automaticity in joint attention is very resource efficient as it does not require an active interpretation of the directional gaze information and thereby does not interfere with other cognitively demanding activities. Accordingly, the implementation of abstract anthropomorphic eyes into robot design might be a resource efficient option to make robot movements more predictable. However, there is evidence that only social stimuli evoke joint attention in contrast to non-social stimuli like arrows (Ricciardelli et al., 2002; Friesen et al., 2004; Ristic and Kingstone, 2005). Whether abstract anthropomorphic eyes like robot eyes, trigger joint attention has therefore been the subject of several studies, which point to a great potential (Admoni and Scassellati, 2017). People have no problems reliably following a robot’s gaze (e.g., Wiese et al., 2018; Onnasch et al., 2022), and use it to predict target positions before these are verbalized (Boucher et al., 2012). Even people’s decision-making can be influenced by a robot’s gaze. Mutlu et al. (2009) and Staudte and Crocker (2008) could show that although participants were told to only consider verbal cues, their attention allocation and object selection was biased by a robot briefly gazing at a certain object. Furthermore, human-like gaze trajectories implemented on a robot’s display have the potential to make object handovers of a robotic arm more pleasant and fluid as well as time-efficient (Moon et al., 2014). Similarly, supportive gaze has been shown to improve performance in an interactive map-drawing task and to reduce the cognitive resources required by the human interaction partner (Skantze et al., 2013). However, also detrimental effects of robot eyes are possible when the eyes and according gaze behavior are purely decorative features and do not correspond to the robot’s motion (Onnasch and Hildebrandt, 2021). In such cases, implementing abstract anthropomorphic eyes into robot design has the potential to distract people from their main task and to make interaction more difficult instead of supporting it.
Besides the growing body of evidence showing the effectiveness (or at least attention-grabbing effect) of robotic gaze, it is still unclear to what extent it is really automatic, i.e., to what extent abstract anthropomorphic gaze triggers reflexive attentional shifts. For example, Admoni et al. (2011) could not find a reflexive cueing for robotic stimuli. The study used the Posner paradigm (Posner, 1980), an experimental set-up for spatial cueing. Participants had to look at a fixation cross, which was then replaced by a spatial cue indicating the position of a subsequently following target stimulus to which participants had to react by an according key press as fast as possible (see also Figure 1). Results showed that participants could infer directional information from the robot’s gaze, but they did not reflexively reallocate their attention to the cued position (Admoni et al., 2011). Other studies suggest an automatic attention cueing of robot gaze (e.g., Boucher et al., 2012). Specifically, Chaminade and Okka (2013) found that both human faces and those of a humanoid robot (Nao) led to automatic attentional shifts, Wiese et al. (2018) showed that eye movements of a social robot (Meka) triggered automatic attention-directing effects, and Pérez-Osorio et al. (2018) successfully replicated the gaze cueing effect using a humanoid robot (iCub). However, it is noteworthy that none of these studies explored an isolated use of eye movements, but a more ecologically typical integration of eye movements, head movements and/or pointing gestures. Some of them (Admoni et al., 2011; Chamindae and Okka, 2013) did not seem to use robots with moving or animated eye parts at all. The specific variance-explaining proportions of gaze thus cannot be determined. Mutlu et al. (2009) investigated the communication of behavioral intentions through robotic eyes without any head movements and found a positive effect of anthropomorphic eyes, but did not include a non-anthropomorphic control condition. Accordingly, it remains unclear whether abstract anthropomorphic robot eyes actually triggered automatic attentional shifts or whether positive effects were only due to the additional information compared to an interaction without any cues.
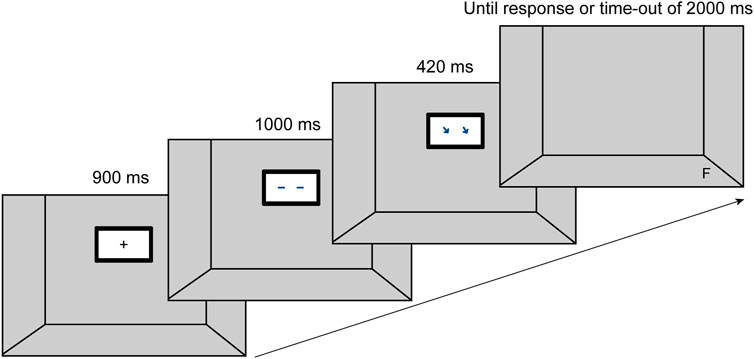
FIGURE 1. Set-up and sequence of events on a given valid trial. (Figure adapted from Onnasch et al., 2022).
In summary, empirical evidence seems to favor the assumption of a beneficial effect of abstract anthropomorphic gaze cues. However, given the methodological characteristics of the existing research it remains unclear whether, in line with the cooperative eye hypothesis (Tomasello et al., 2007), eye movements of a robot are sufficient directional cues without head movements or point gestures. Further systematic research comparing anthropomorphic eye stimuli with non-anthropomorphic cue stimuli is therefore needed. In addition, there is a lack of studies specifically for the industrial application area and the associated special requirements mentioned above (functionalist design, straight forward implementation).
According to these requirements, we investigated in a previous study directional stimuli differing in their degree of anthropomorphism to facilitate attentional shifts for the potential use as robot eyes on an industrial robot (Onnasch et al., 2022). The online study used a modified version of the spatial cueing paradigm (Posner, 1980), using either arrows, abstract anthropomorphic eyes or photographed human eyes as directional stimuli. Attentional shifts were measured indirectly as the time from the target onset (in that case the presentation of a single letter) until the according key press. Results supported the assumption that abstract anthropomorphic eyes have the potential to facilitate HRI, as they led to the fastest responses which is indicative for reflexive gaze cueing. Surprisingly and in contrast to hypotheses, the human eyes did not evoke reflexive attentional shifts as evidenced by longer response times. We suspected that the abstract anthropomorphic eyes elicited the desired effects because they were sufficiently human-like and at the same time much easier to perceive than human eyes, with the latter being due to the abstract anthropomorphic eyes’ design featuring strong contrasts and clean lines. This is an interesting finding and may prove helpful for designing better HRI. However, to see whether this is in fact a solid basis for further conclusions and actions, those unexpected findings with regard to the superiority of anthropomorphic, non-human eyes, even in comparison to human eyes, call for a validation. Especially, because the implementation as an online-study comes with a lack of control in terms of standardized situational circumstances and hardware (light conditions, distraction, screen resolution, …). Moreover, the measurement of attention was only realized via covert measures in terms of reaction times. Thus, to further strengthen results and the interpretation that abstract anthropomorphic eyes induce reflexive gaze cueing, the aim of the current study was therefore to validate findings of the previous online study (Onnasch et al., 2022) in a highly controlled laboratory environment and to further deepen insights by introducing direct attentional measures via eye-tracking. We investigated how the design of highly abstract anthropomorphic eyes for a potential use on a collaborative robot should look like in order to reflexively trigger attention reallocation to improve the prediction of robot motion.
2 Materials and methods
The experiment was performed with ethical committee approval by the Institute of Psychology, Humboldt-Universität zu Berlin, and in accordance with the Declaration of Helsinki. Informed consent was obtained from each participant. We preregistered the study at the Open Science Framework (osf.io/wue6d).
2.1 Participants
A sample size of N = 80 was defined based on an a priori power analysis using GPower (Faul et al., 2007; Faul et al., 2009). Due to COVID-19 induced restrictions we had to halve the sample size and recruited 40 participants via the local online recruiting system of the Institute of Psychology, Humboldt-Universität zu Berlin. Participants either received course credit or a €10 compensation at the end of the experiment. One participant had to be excluded because of technical issues. We therefore conducted data analysis with a sample of N = 39 participants with German as native language or equal language abilities (M = 32.26 years, SD = 10.78 years, 27 females).
2.2 Apparatus and task
The experiment was conducted on a 27″ HD Dell Monitor (1,920 × 1,080 px) which was positioned at a distance of 67 cm to a chin rest. The latter was used to minimize artefacts of head movements for eye-tracking data. The setup was a modified version of a traditional spatial cueing paradigm (Posner, 1980; Figure 1) and corresponded to the setup of the previous online study (compare Onnasch et al., 2022). Each trial began with the presentation of a fixation cross in the center of a depicted display on the computer screen (see Figure 1). After 900 ms, a display appeared with a “gaze” facing to the front. 1,000 ms later the gaze averted to a position where the target appeared after a stimulus onset asynchrony (SOA) of 420 ms,. The target disappeared upon participants’ reaction or a time-out of 2000 ms (description taken from Onnasch et al., 2022).
Figure 1 All central cue stimuli as well as the fixation cross were displayed at the subjects’ eye level on the screen. The target stimuli appeared in a 3D-like image of a room. It seems noteworthy at this point that we intentionally designed a screen-based experiment instead of one using a real human-robot interaction. This has been done not only to exclude any confounding effects that the HRI might induce, but also to avoid parallax effects by making the angle between robot eyes and target positions, i.e., the stimulus geometry, absolutely invariant. Nevertheless, to increase ecological validity, we modeled the three-dimensional space with target positions in reference to a physical setup of a shared workspace with an industrial robot (Sawyer by Rethink Robotics). We measured the distances between actual target positions, i.e., positions that the robot could reach with its gripper, the robot’s display, and the human co-worker. These distances were then scaled down and transferred as parameters into our model, that used HTML, JavaScript and raster graphics to render the virtual set-up. Eight different positions were determined for the target stimuli to appear in the experiment. Six target positions were located below the display on what appeared to be a floor (three positions in a front row, three positions in a back row), two target positions were on the side walls, one left and one right, each in a centered position. Implementing eight different target positions represents a significant change from the experimental gaze cueing setup which is conventionally distributed between two positions or a maximum of four positions (e.g., Admoni et al., 2011). This change was deemed necessary to approximate a real industrial HRI situation, thus further increasing ecological validity. The size of the frame in which the fixation cross and cue stimuli were presented centrally covered 7.91° × 4.81° in angle of view (AOV), which corresponds in its relative dimensions to the display of a Sawyer robot. The size of the display of the stimuli in angular degrees was determined approximately oriented to the mean value of previous studies. The cueing stimuli were either images of human eyes, arrows, or two different versions of abstract anthropomorphic eyes (pixel, cross). Following classical gaze cueing tasks, two black sans-serif letters F and T were presented as target stimuli (e.g., Friesen and Kingstone, 1998). These corresponded to 0.50° AOV in their presented size and were presented at a distance of 13.40°–22.75° AOV from the center of the fixation cross, depending on their position in space. The small size of the target stimuli in combination with the high degree of similarity in the typeface of the two letters was to ensure that no discrimination of the target stimuli was possible in the peripheral field of view. It should be necessary to shift the foveal field of view for task performance in order to trigger eye movements of the subjects. Table 1 summarizes the information on the AOV of the respective elements in the experimental set up.
For recording participants’ manual responses to the cue stimuli, the Microsoft Xbox Wireless 1708 controller was used. For the recording of oculomotor movements, the screen-based remote eye tracker model RED500 from iMotion (Senso-Motoric Instruments GmbH, SMI) with a sampling rate of 500 Hz was used. The spatial accuracy of the device amounts to 0.40° for binocular recording, which was also chosen in this study.
2.3 Design
Two variables were systematically varied in the experiment. First, the cues were varied between-subject, representing either human eyes, abstract anthropomorphic eyes, or arrow stimuli. For the previous online study, the anthropomorphic eyes were designed striving for a maximum level of abstraction while retaining the essential features of the human eye (e.g., visible pupil-sclera size ratio). This resulted in two different anthropomorphic eye designs, that were both exploratively compared in the online study and therefore also implemented in the current laboratory experiment (cross and pixel design, Figure 2). Second, the trial congruency was manipulated as a within-subject factor. From a total of 304 trials, the target stimuli appeared at cued locations in 80% of the trials (240 trials congruent), while in the remaining 20% of trials the target appeared at uncued locations (64 trials incongruent). The distribution of congruent and incongruent trials was defined with a random number generator and was the same in all four conditions. Overall, this resulted in a 4 (stimulus type) × 2 (trial congruency) mixed design. In the previous online study, a third factor was implemented which investigated the impact of paired vs. single stimulus representations (Onnasch et al., 2022). However, as this variation did not have an impact on reaction times, we decided to discard this factor for the follow up study.
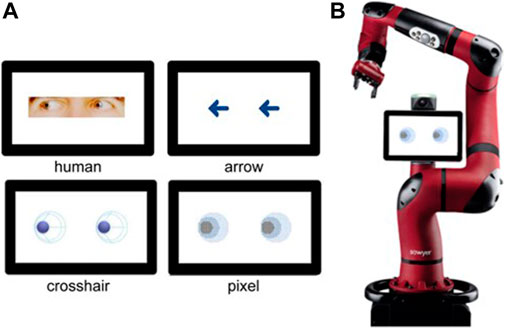
FIGURE 2. (A) The four stimulus types, labelled respectively. The top row includes the human (left) and arrow (right) stimuli. The abstract anthropomorphic robot eyes are presented in the second row. (B) Image of the collaborative robot Sawyer used in the questionnaire. In this case, presented featuring the pixel eye design. (Figure adapted from Onnasch et al., 2022).
2.4 Dependent measures
2.4.1 Reaction time
We assessed the reaction times as a covert measure of attention and to evaluate the potential for reflexive cueing of the different stimuli. Reaction times were measured from the target onset to a key press (F or T) on the controller. We only included trials with correct answers (e.g., target F, key press F) as incorrect answers could have biased the results.
2.4.2 Gaze-cueing effect
We calculated the gaze cueing effect (GCE) by subtracting mean reaction times of congruent trials from the mean reaction times of incongruent trials.
2.4.3 Saccadic latency
As an overt attentional measure, saccadic latency was measured. This describes the time elapsing between the appearance of the target letter and the initiation of the orienting saccade away from the cue stimulus. It serves as an indicator of attention directing properties of the cue stimulus and describes how long a disengagement of attention from the cue stimulus took (e.g., Admoni and Scassellati, 2017). Fixations were detected using a dispersion based algorithm with 0.5° and 120 ms as spatial and temporal thresholds. Saccade initiation was defined as the first sample captured outside the fixation area (Nyström and Holmqvist, 2010).
2.4.4 Social attributes
On an explorative basis, we were further interested in how a robot having incorporated the stimulus designs would be perceived. A positive perception of the overall robot design is a crucial precondition for an implementation of such designs in terms of user acceptance. Accordingly, we presented the different stimulus designs as part of an image of an industrial collaborative robot (Sawyer, Rethink Robotics, Figure 2) and asked participants to fill in the Robotic Social Attributes Scale (RoSAS; Carpinella et al., 2017). The RoSAS consists of a total of 18 adjectives and three subscales: warmth, competence and discomfort. Participants have to indicate how closely each adjective is associated with the robot image on a 7-point Likert scale from 1 (definitely not associated) to 7 (definitely associated).
2.5 Procedure
Participants were randomly assigned to one of the four between-subject conditions. Upon arrival at the lab, participants received detailed information about the study and data handling. After giving their informed consent, they received instructions for the experiment and started with two training sessions that familiarized them with the task. The first training comprised 12 trials during which a letter (T or F) appeared centrally on the screen. Participants were instructed to place their index fingers on the directional pads of the controller (left shoulder key for F, right shoulder key for T) and to react upon seeing the letters, using the respective keys. The letter changed its color from white to green upon correct response and from white to red, indicating an incorrect reaction.The aim of this training was to get participants used to the key presses without having to shift their gaze to the controller. During the 40 trials of the second training, participants practiced the experimental task. They were told they would look into a room in which a display was hanging at the back wall (see Figure 1). The appearance of a fixation cross started a trial. After each trial an inter-trial interval of 200 ms elapsed before the next trial began. After completing the second training, the main test procedure started, consisting of 304 trials. The training did not include incongruent trials and participants were not told that there would be incongruent trails during the experiment. The time course followed in each trial of the second training and the test procedure is shown in Figure 1. Upon successful completion of the actual experiment, in a last step, participants were asked to fill in remaining questionnaires (sociodemographics & RoSAS). Only for the RoSAS, we presented a contextualized version of the stimulus design as part of the industrial robot Sawyer (Figure 2). The main test (spatial cueing paradigm) was done without depicting a robot but only a screen featuring the stimulus (Figure 1). The entire procedure took approximately 45 min.
3 Results
The descriptive data for all three dependent variables are reported for each stimulus condition in Table 2.
3.1 Reaction time
Results are depicted in Figure 3. Reaction times were longer in incongruent trials (M = 829.89 ms; SD = 97.95 ms) compared to congruent trials (M = 687.82 ms; SD = 70.36 ms). This was supported by a main effect of trial congruency, F (1,70) = 61.91, p < 0.001, ηp2 = 0.469.
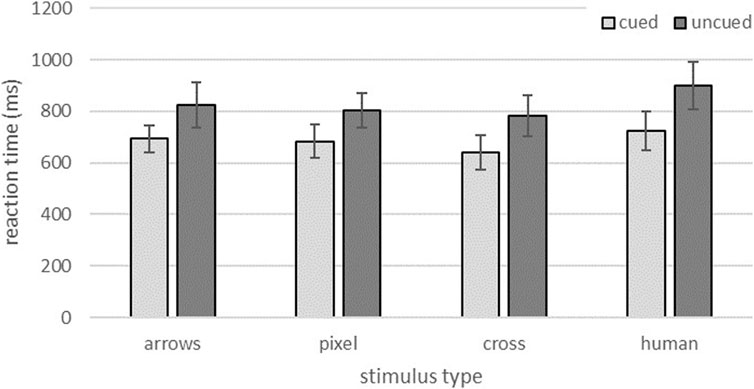
FIGURE 3. Reaction times for cued and uncued trials for the different stimulus type conditions. Error bars represent standard deviations.
The data also revealed a significant main effect of stimulus type, F (3,70) = 11.78, p = 0.001, ηp2 = 0.200. In congruent as well as incongruent trials, the human eyes led on average to the longest reaction times (M = 812.47 ms; SD = 122.00 ms). The anthropomorphic cross condition elicited the fastest reactions (M = 711.52 ms; SD = 101.27 ms). No interaction effect was found (F < 1).
Bonferroni corrected post hoc comparisons showed that only the anthropomorphic cross design (mean difference −100.95 ms, p = 0.001) and the pixel design differed significantly from the human eye stimuli (mean difference −53.85 ms, p = 0.033) whereas no significant difference emerged between human eyes and arrow stimuli.
3.2 Gaze-cueing effect
The mean values for the GCE differed gradually, descriptively decreasing from human stimuli (M = 181.54, SD = 61.14) over anthropomorphic cross design (M = 143.83, SD = 46.84) and arrows (M = 133.95, SD = 45.55) to the anthropomorphic pixel condition (M = 124.86, SD = 45.55). The univariate ANOVA however did not support this descriptive pattern as no significant main effect of stimulus type was found for GCE, F (3,35) = 2.16, p = 0.110.
3.3 Saccadic latency
Similar to the manual reaction times via key press, human eyes appeared to produce the longest (visual) reaction times in both congruency conditions (Figure 4; Mcongruent = 293.44 ms; SDcongruent = 17.99 ms; Mincongruent = 293.71 ms; SDincongruent = 18.05 ms). The descriptive data of the congruent trials also indicated the second longest times for the arrow eyes (M = 287.38 ms; SD = 18.44 ms) and, at some distance, the anthropomorphic stimuli both followed at about the same level with the lowest values (Mcross = 273.37 ms; SDcross = 11.17 ms; Mpixel = 267.63 ms; SDpixel = 21.53 ms). For incongruent trials, the difference between the arrow condition (M = 282.45 ms; SD = 21.56 ms) and the abstract anthropomorphic eyes (M = 293.71 ms; SD = 18.05 ms) appeared less evident. Overall, saccadic latency plausibly appeared to be independent of trial congruency.
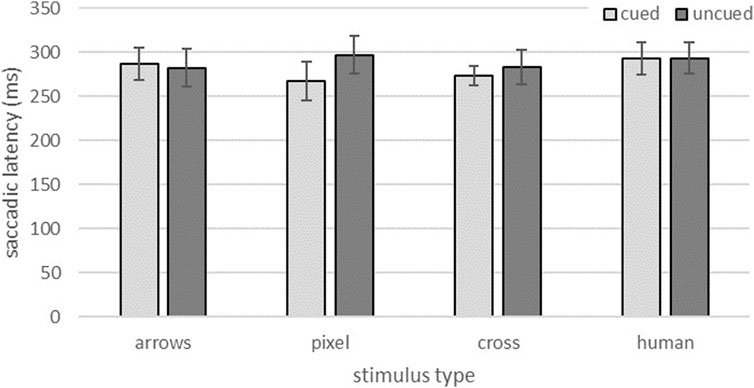
FIGURE 4. Saccadic latencies for congruent and incongruent trials for the different stimulus type conditions. Error bars represent standard errors.
The two-factorial ANOVA did not show a significant impact of trial congruency on saccadic latencies, F (1,70) = 0.94, p = 0.337, but a significant effect of stimulus type, F (3,70) = 4.40, p = 0.007, ηp2 = 0.159. Bonferroni corrected post hoc pairwise comparisons detailed this effect and revealed significant differences only between the human stimuli and the anthropomorphic pixel design (p = 0.006). All other comparisons did not reach significance.
3.4 Social attributes
Results of the RoSAS are displayed in Table 3. On the warmth dimension, participants rated the two anthropomorphic stimulus designs highest while arrows received the overall lowest ratings. The ANOVA, however, did not reveal significant differences between the conditions, F (3,35) = 2.33, p = 0.091.
The perceived competence subscale showed substantial differences for the stimulus designs, F (3,35) = 3.72, p = 0.020, ηp2 = 0.242. This was due to the high competence ratings of the cross design. Post hoc tests with Bonferroni correction further showed a significant difference of this design compared to the pixel design that was perceived least competent (p = 0.010).
With regard to the perceived discomfort of the overall robot’s design, the different stimulus types did not significantly change participants’ perception, F (3,35) = 0.93, p = 0.436.
4 Discussion
This study aimed to validate findings of a previous online study on the effectiveness of different directional stimuli regarding reflexive attention allocation (Onnasch et al., 2022) in a highly controlled laboratory environment and to further deepen insights by introducing direct attentional measures via eye-tracking. Both studies investigated how directional stimuli should be designed for a potential use on a collaborative industrial robot to enable human interaction partners to predict the robot’s movements in a cognitively efficient way.
As expected, and in line with the previous online study, a congruency effect could be demonstrated for all four stimulus types. Subjects reacted faster to targets that were correctly indicated by the gaze direction of the stimuli (cued trials) than to those indicated in the opposite direction (uncued trials). This means that all stimulus types were essentially able to support the subjects’ attentional orientation. Such a congruency effect has been demonstrated several times before for different directional cueing stimuli (e.g., Admoni et al., 2011; Chaminade and Okka, 2013; Wiese et al., 2018).
However, a closer look at the reaction times revealed surprising differences in how efficiently the guidance of the subjects’ attention could be supported. Whereas no differences emerged for the GCE, the two abstract anthropomorphic eye designs each resulted in the shortest reaction times in cued trials. The current findings therefore support results from the previous online study, which also revealed the fastest reaction times for the abstract anthropomorphic eyes. In the current study, these findings were further underlined by the subjects’ eye movements. For both anthropomorphic stimulus designs saccadic latencies were descriptively shorter compared to the arrows and the human eyes. A significant difference to the human eye design emerged however, only for the pixel design. Results of the current and the online study therefore conflict with studies that consider human eyes to be the strongest stimulus to reflexively direct the visual attention of an interaction partner due to their biological and social relevance (Tipper et al., 2008). The results also contradict studies that observed slower responses in direct comparisons of human and robotic eyes (Bonmassar et al., 2019).
Also, for the uncued trials, either of the anthropomorphic eye designs led to shorter reaction times compared to human eyes, and one of the anthropomorphic designs (cross) produced shorter saccadic latencies. Hereby results differ from the online study. As we did not change the stimuli it is hard to explain why the abstract anthropomorphic eyes supported attentional shifts in both, cued and uncued trials. This pattern of results is in contrast to the key mechanism of reflexive gaze cueing, which should always reveal shorter reaction times in cued trials compared to non-reflexive gaze cueing, but longer reaction times in invalid trials because of the higher effort to disengage attention (Ricciardelli et al., 2002; Friesen et al., 2004; Ristic and Kingstone, 2005). Thus, results still have to be further validated by future research to see whether abstract anthropomorphic eyes are the silver bullet in gaze cueing inducing only beneficial effects or whether the current results for the uncued trials do not represent a valid finding.
As was already discussed in more detail for the online study (Onnasch et al., 2022), the overall slower reactions to the human stimuli might have been due to a lack of saliency compared to the other stimuli because they were smaller (although the overall image size was the same) and less rich in contrast compared to the other cues. But this seems to be only half of the story, because if this was the exclusive driving force for the superior processing of the abstract anthropomorphic eyes then this should have also applied for the chunky, but purely symbolic arrows. Since this was not the case, it seems reasonable that the abstract anthropomorphic eyes combined best of both worlds. The anthropomorphic eye design triggered a social and therefore reflexive processing of the stimuli (Tomasello et al., 2007) while at the same time being easier to perceive than human eyes due the high contrast imagery.
To summarize results on reaction times and saccadic latencies, the findings are in favor of the abstract anthropomorphic eye designs as these eye gaze prototypes performed best in the cueing of attention.
The explorative analyses on the robot’s overall perception with the according stimulus prototypes favor an anthropomorphic eye design, too. Whereas no stimulus design discomforted participants, they attributed more competence to a robot with an anthropomorphic cross eye design. The perceived warmth of the robot was not significantly different but again descriptively higher for the anthropomorphic designs.
A clear limitation of this study is the small sample size. We aimed at 80 participants for a sufficient statistical power but had to halve the sample size because of an ongoing lockdown due to the COVID-19 pandemic. Some of the reported results just missed the conventional level of significance, which could have been a consequence of the small sample. Further studies are needed to replicate the current design with sufficient power. Another drawback with regard to transferability of results is that we used a highly controlled computer-based paradigm instead of engaging participants in an interaction with an actual robot. Our results therefore have to be interpreted as a first step to identify directional stimuli for robot design that support humans’ smooth attention reallocation in order to improve coordination in HRI. The current study did not represent a real human-robot collaboration. Naturalistic follow-up studies will have to validate the results in a real-world interaction and investigate whether the benefits of abstract anthropomorphic eyes persist and effectively ease the prediction of robot movements. In an actual working situation where people have to focus on other elements (such as assembly tasks), results may differ significantly which underlines the importance of more research. Another point to be considered in future studies is to parametrize and empirically explore the differences between the stimulus designs to better understand the underlying mechanisms leading to the observed effects. Lastly, since the stimulus condition was varied between subjects, no statement can be made about possible interindividual differences.
In sum, the current study supported previous findings of the online study, showing a clear tendency for superior processing of abstract anthropomorphic eyes. Both of the abstract eye gaze prototypes performed well in attentional cueing, yet, as the results were not consistent across all measures, neither of the prototypes stands out in particular. However, one of the designs received higher competence ratings, which makes it seem appropriate for the implementation in work-related settings. These insights on predictive visual stimuli are a first step to translate basic social mechanisms into useful design recommendations to ease the coordination in HRI.
Data availability statement
The raw data supporting the conclusions of this article are available at the OSF (osf.io/wue6d).
Ethics statement
The studies involving human participants were reviewed and approved by The Ethical Committee of the Institute of Psychology, Humboldt-Universität zu Berlin. The patients/participants provided their written informed consent to participate in this study.
Author contributions
LO, PS, and HS contributed to the conception and design of the study. HS collected the data. PS checked the data quality. Data analyses were conducted by LO and HS. All authors LO, PS, and HS contributed to the interpretation of findings. LO drafted the manuscript and LO and PS revised it. All authors contributed to the article and approved the submitted version.
Funding
This research project has received funding from the European Union’s Horizon 2020 research and innovation programme under grant agreement No. 779966. We acknowledge support by the German Research Foundation and the Open Access Publication Fund of TU Berlin.
Acknowledgments
We would like to thank Silvio Tristram for the professional programming of the experimental environment in combination with the eye-tracking set-up.
Conflict of interest
Author PS was employed by HFC Human-Factors-Consult GmbH. HS was currently employed by Land in Sicht—PROWO gGmbH, but was a master’s student at Humboldt-Universität zu Berlin during the study implementation. The study was her master thesis which was conducted under the supervision of LO.
The remaining author declares that the research was conducted in the absence of any commercial or financial relationships that could be construed as a potential conflict of interest.
Publisher’s note
All claims expressed in this article are solely those of the authors and do not necessarily represent those of their affiliated organizations, or those of the publisher, the editors and the reviewers. Any product that may be evaluated in this article, or claim that may be made by its manufacturer, is not guaranteed or endorsed by the publisher.
References
Admoni, H., Bank, C., Tan, J., Toneva, M., and Scassellati, B. (2011). Robot gaze does not reflexively cue human attention. Proc. Annu. Meet. Cognitive Sci. Soc. 33, 1983–1988.
Admoni, H., and Scassellati, B. (2017). Social eye gaze in human-robot interaction: A review. J. Human-Robot Interact. 6 (1), 25. doi:10.5898/jhri.6.1.admoni
Bacula, A., Mercer, J., and Knight, H. (2020). “Legible light communications for factory robots,” in Companion of the 2020 ACM/IEEE International Conference on Human-Robot Interaction, Stockholm, March 13-16, 2023, 119–121.
Bonmassar, C., Pavani, F., and van Zoest, W. (2019). The role of eye movements in manual responses to social and nonsocial cues. Atten. Percept. Psychophys. 81 (5), 1236–1252. doi:10.3758/s13414-019-01669-9
Boucher, J. D., Pattacini, U., Lelong, A., Bailly, G., Elisei, F., Fagel, S., et al. (2012). I reach faster when i see you look: Gaze effects in human-human and human-robot face-to-face cooperation. Front. Neurorobotics 6, 3–11. doi:10.3389/fnbot.2012.00003
Carpinella, C. M., Wyman, A. B., Perez, M. A., and Stroessner, S. J. (2017). “The robotic social attributes scale (RoSAS) development and validation,” in Proceedings of the 2017ACM/IEEE International Conference on human-robot interaction, Vienna, Austria, March 6–9, 2017, 254–262.
Chaminade, T., and Okka, M. M. (2013). Comparing the effect of humanoid and human face for the spatial orientation of attention. Front. Neurorobotics 7, 12–17. doi:10.3389/fnbot.2013.00012
Faria, M., Melo, F. S., and Paiva, A. (2021). Understanding robots: Making robots more legible in multi-party interactions. In 2021 30th IEEE International Conference on Robot & Human Interactive Communication (RO-MAN). IEEE, 1031–1036.
Faul, F., Erdfelder, E., Buchner, A., and Lang, A. G. (2009). Statistical power analyses using G*Power 3.1: Tests for correlation and regression analyses. Behav. Res. Methods 41 (4), 1149–1160. doi:10.3758/brm.41.4.1149
Faul, F., Erdfelder, E., Lang, A.-G., and Buchner, A. (2007). G*Power 3: A flexible statistical power analysis program for the social, behavioral, and biomedical sciences. Behav. Res. Methods 39 (2), 175–191. doi:10.3758/bf03193146
Friesen, C. K., and Kingstone, A. (1998). The eyes have it! Reflexive orienting is triggered by nonpredictive gaze. Psychonomic Bull. Rev. 5 (3), 490–495. doi:10.3758/bf03208827
Friesen, C. K., Ristic, J., and Kingstone, A. (2004). Attentional effects of counterpredictive gaze and arrow cues. J. Exp. Psychol. Hum. Percept. Perform. 30 (2), 319–329. doi:10.1037/0096-1523.30.2.319
Hetherington, N. J., Croft, E. A., and Van der Loos, H. M. (2021). Hey robot, which way are you going? Nonverbal motion legibility cues for human-robot spatial interaction. IEEE Robotics Automation Lett. 6 (3), 5010–5015. doi:10.1109/lra.2021.3068708
Khoramshahi, M., Shukla, A., Raffard, S., Bardy, B. G., and Billard, A. (2016). Role of gaze cues in interpersonal motor coordination: Towards higher affiliation in human-robot interaction. PLOS ONE 11 (6), 01568744–e156921. doi:10.1371/journal.pone.0156874
Moon, A., Zheng, M., Troniak, D. M., Blumer, B. A., Gleeson, B., MacLean, K., et al. (2014). “Meet me where I’m gazing: How shared attention gaze affects human-robot handover timing,” in Proceedings of the 2014 ACM/IEEE International Conference on Human-Robot Interaction, Bielefeld, Germany, March 3-6, 2014, 334–341.
Mutlu, B., Yamaoka, F., Kanda, T., Ishiguro, H., and Hagita, N. (2009). “Nonverbal leakage in robots: Communication of intentions through seemingly unintentional behavior,” in Proceedings of the 4th ACM/IEEE International Conference on Human Robot Interaction, La Jolla, CA, March 11-13, 2009, 69–76.
Neider, M. B., Chen, X., Dickinson, C. A., Brennan, S. E., and Zelinsky, G. J. (2010). Coordinating spatial referencing using shared gaze. Psychonomic Bull. Rev. 17 (5), 718–724. doi:10.3758/pbr.17.5.718
Nyström, M., and Holmqvist, K. (2010). An adaptive algorithm for fixation, saccade, and glissade detection in eyetracking data. Behav. Res. methods 42 (1), 188–204. doi:10.3758/brm.42.1.188
Onnasch, L., and Hildebrandt, C. L. (2021). Impact of anthropomorphic robot design on trust and attention in industrial human-robot interaction. ACM Trans. Human-Robot Interact. (THRI) 11 (1), 1–24. doi:10.1145/3472224
Onnasch, L., Kostadinova, E., and Schweidler, P. (2022). Humans can’t resist robot eyes – reflexive cueing with pseudo-social stimuli. Front. Robotics AI 9, 848295. doi:10.3389/frobt.2022.848295
Onnasch, L., and Roesler, E. (2021). A taxonomy to structure and analyze human–robot interaction. Int. J. Soc. Robotics 13 (4), 833–849. doi:10.1007/s12369-020-00666-5
Onuki, T., Ishinoda, T., Kobayashi, Y., and Kuno, Y. (2013). “Design of robot eyes suitable for gaze communication,” in 2013 8th ACM/IEEE International Conference on Human-Robot Interaction (HRI), Tokyo, Japan, March 3-6, 2013 (IEEE), 203–204.
Pérez-Osorio, J., De Tommaso, D., Baykara, E., and Wykowska, A. (2018). “Joint action with icub: A successful adaptation of a paradigm of cognitive neuroscience in hri,” in 2018 27th IEEE International Symposium on Robot and Human Interactive Communication (RO-MAN), Nanjing, China, August 27-31, 2018 (IEEE), 152–157.
Posner, M. I. (1980). Orienting of attention. Q. J. Exp. Psychol. 32 (1), 3–25. doi:10.1080/00335558008248231
Restrepo, S. S., Raiola, G., Chevalier, P., Lamy, X., and Sidobre, D. (2017). “Iterative virtual guides programming for human-robot comanipulation,” in . IEEE/ASME International Conference on Advanced Intelligent Mechatronics, AIM, Munich, Germany, July 3-7, 2017, 219–226.
Ricciardelli, P., Bricolo, E., Aglioti, S. M., and Chelazzi, L. (2002). My eyes want to look where your eyes are looking: Exploring the tendency to imitate another individual’s gaze. NeuroReport 13 (17), 2259–2264. doi:10.1097/00001756-200212030-00018
Ristic, J., and Kingstone, A. (2005). Taking control of reflexive social attention. Cognition 94 (3), B55–B65. doi:10.1016/j.cognition.2004.04.005
Shepherd, S. V. (2010). Following gaze: Gaze-following behavior as a window into social cognition. Front. Integr. Neurosci. 4, 5. doi:10.3389/fnint.2010.00005
Shrestha, M. C., Kobayashi, A., Onishi, T., Yanagawa, H., Yokoyama, Y., Uno, E., et al. (2016). “Exploring the use of light and display indicators for communicating directional intent,” in 2016 IEEE International Conference on Advanced Intelligent Mechatronics (AIM), Banff, AB, July 12-15, 2016 (IEEE), 1651–1656.
Skantze, G., Hjalmarsson, A., and Oertel, C. (2013). “Exploring the effects of gaze and pauses in situated human-robot interaction,” in SIGDIAL 2013 - 14th Annual Meeting of the Special, Metz, France, August 22-24, 2013.
Staudte, M., and Crocker, M. W. (2008). “Visual attention in spoken human-robot interaction,” in Proceedings of the 4th ACM/IEEE International Conference on Human-Robot Interaction, HRI’09, La Jolla, CA, March 11-13, 2009, 77–84.
Tipper, C. M., Handy, T. C., Giesbrecht, B., and Kingstone, A. (2008). Brain responses to biological relevance. J. Cognitive Neurosci. 20 (5), 879–891. doi:10.1162/jocn.2008.20510
Tomasello, M., Hare, B., Lehmann, H., and Call, J. (2007). Reliance on head versus eyes in the gaze following of great apes and human infants: The cooperative eye hypothesis. J. Hum. Evol. 52 (3), 314–320. doi:10.1016/j.jhevol.2006.10.001
Keywords: human-robot interaction (HRI), attentional processes, joint attention, anthropomorphism, robot design
Citation: Onnasch L, Schweidler P and Schmidt H (2023) The potential of robot eyes as predictive cues in HRI—an eye-tracking study. Front. Robot. AI 10:1178433. doi: 10.3389/frobt.2023.1178433
Received: 02 March 2023; Accepted: 03 July 2023;
Published: 28 July 2023.
Edited by:
Erik A. Billing, University of Skövde, SwedenReviewed by:
Christian Balkenius, Lund University, SwedenFederico Fraboni, University of Bologna, Italy
Copyright © 2023 Onnasch, Schweidler and Schmidt. This is an open-access article distributed under the terms of the Creative Commons Attribution License (CC BY). The use, distribution or reproduction in other forums is permitted, provided the original author(s) and the copyright owner(s) are credited and that the original publication in this journal is cited, in accordance with accepted academic practice. No use, distribution or reproduction is permitted which does not comply with these terms.
*Correspondence: Linda Onnasch, linda.onnasch@tu-berlin.de