- 1Department of Chemistry, Biology, and Biotechnology, Università degli Studi di Perugia, Perugia, Italy
- 2Department of Biological and Environmental Sciences and Technologies (DiSTeBA), University of Salento, Lecce, Italy
Abstract
Chemical Artificial Intelligence (CAI) is a brand-new research line that exploits molecular, supramolecular, and systems chemistry in wetware (i.e., in fluid solutions) to imitate some performances of human intelligence and promote unconventional robotics based on molecular assemblies, which act in the microscopic world, otherwise tough to be accessed by humans. It is undoubtedly worth spreading the news that AI researchers can rely on the help of chemists and biotechnologists to reach the ambitious goals of building intelligent systems from scratch. This article reports the first attempt at building a Chemical Artificial Intelligence knowledge map and describes the basic intelligent functions that can be implemented through molecular and supramolecular chemistry. Chemical Artificial Intelligence provides new tools and concepts to mimic human intelligence because it shares, with biological intelligence, the same principles and materials. It enables peculiar dynamics, possibly not accessible in software and hardware domains. Moreover, the development of Chemical Artificial Intelligence will contribute to a deeper understanding of the strict link between intelligence and life, which are two of the most remarkable emergent properties shown by the Complex Systems we call biological organisms.
1 Introduction
Humanity is experiencing a fourth technological revolution, altering how we live, work, and relate to one another (Schwab, 2016). Cutting-edge biotechnologies, nanotechnologies, Artificial Intelligence (AI), and Robotics are blurring the boundaries between biological, physical, and digital-virtual spaces. Specifically, AI and robotics assist us in our daily mental and manual efforts; they augment our intelligence through powerful computing machines (Wooldridge, 2022); they can even replace us in accomplishing specific tasks, sometimes going beyond human performances (Kurzweil, 2014). However, their functionalities are restricted to what they are programmed to do. In other words, AI and robots are still “weak” or “narrow” because they are capable of accomplishing specific tasks, but they cannot perform “general” intelligent actions as humans do (Goertzel, 2007; Russell and Norvig,2010). “General” AI will probably become a reality soon due to the hectic interdisciplinary research activities devoted to designing and constructing machines thinking humanely (Müller and Bostrom, 2016). The plethora of methods proposed to abstract human intelligence (Lehman et al., 2014) and develop AI might be grouped into two principal approaches. 1) The first approach relies on current general-purpose electronic computers or other special-purpose hardware and consists in writing software that can think rationally and humanly. Such software can reproduce the thinking process when it is a flow of rigorous logical operations (this strategy is known as the symbolic paradigm). Alternatively, software mimics some structural and/or functional features of neural networks to learn how to perform tasks from data (this latter strategy encompasses the subsymbolic and statistical paradigms) (Corea, 2019; Mitchell, 2019). 2) The second methodological approach to developing AI is reverse engineering of the brain in hardware, also known as neuromorphic engineering, which implements neural surrogates through non-biological systems. Such neural surrogates are used as neuroprostheses or to design brain-like computing machines that revolutionise von Neumann’s architectures of current electronic computers. Neural surrogates are implemented in hardware through specific solid materials. Such hardware is rigid if implemented through silicon-based circuits or inorganic memristors. It is flexible if based on organic semiconductor films (Nawrocki et al., 2016; Lee and Lee, 2019; Zhu et al., 2020; Christensen et al., 2022).
A brand-new idea for mimicking intelligence (intended in the broadest sense) has been recently sparked by three factors. Firstly, the relentless miniaturisation of the transistors (i.e., the basic computing elements of electronic computers), has been pushed to the limit, whereby a few atoms or single molecules become the basic switching elements (Fu et al., 2022). Secondly, it is clear that all living beings have the capacity to process physicochemical information through their bodies, which ultimately are fluid solutions. Thirdly, every living being, both pluricellular and unicellular, is provided with a sort of nervous system, consisting of sensors, effectors, and a sort of brain (Roederer, 2005), which are implemented through molecules and their networks. These three observations triggered the dawn and the initial development of the so-called Chemical Artificial Intelligence (CAI) (Gentili, 2013): a research line that exploits molecular, supramolecular, and systems chemistry in wetware (i.e., in fluid solutions) to imitate some performances of biological intelligence—at a minimal but organizationally significant level—and promote unconventional robotics, based on molecular assemblies.
This article is the first of two papers series, which aim to present our viewpoints on the current strategies for developing CAI, its paradigms, and its scope, also called the problems domain. Our opinions about its perspectives are also expressed. This first part focuses on Molecular and Supramolecular Chemistry. The second contribution will focus on Systems Chemistry.
It is undoubtedly worth spreading the news that AI researchers can rely on the help of chemists and biotechnologists to reach the ambitious goals of building intelligent systems from scratch. We expect that, in the following years, the collaboration among chemists, biotechnologists, computer scientists, mathematicians, engineers, and physicists, will boost the development of chemical AI and chemical robotics. From a technological viewpoint, CAI will contribute by providing new tools and concepts to the sciences of the artificial because it shares, with the intelligence of biological systems, the same principles and materials, enabling peculiar dynamics not accessible in software and hardware domains (or accessible via simulations, not by embodiment). Moreover, we suggest that CAI will also contribute to generating basic scientific knowledge by allowing a deeper understanding of the strict link between intelligence and life, which are two of the most astounding emergent properties shown by the Complex Systems we call biological organisms (Gentili P. L., 2021).
2 The field of CAI
Before presenting the strategies proposed so far to develop CAI and its domains of application, it is paramount to neatly outline its scope. First of all, the burgeoning research field of CAI must be distinguished from the already impactful and still tremendously soaring field of AI in chemistry (Baum et al., 2021), in which the usual chemists’ actions of design, synthesis, characterisation and application of molecules and materials are assisted and accelerated by the AI tools and methods (Butler et al., 2018) such as machine learning, quantitative structure-activity relationship (QSAR) models, molecular docking, chemical reaction prediction, generative models, chemoinformatics and data mining. Secondly, CAI refers to designing and implementing intelligent complex molecular systems, which—as it happened with the conventional AI path—have the human nervous systems and its components, i.e., the Sensory System (SS), the Central Nervous System (CNS), and the Effector System (ES) (ultimately made of single neural cells) as epitomes.
There is more, however. The features of CAI allow starting new investigation directions, in particular those inspired to lower and more basic, but nevertheless relevant, forms of intelligence, e.g., the one referred to uni- or pauci-cellular organisms. The biologically-inspired molecular systems of CAI are complex fluid mixtures, usually made of soft matter, likewise living cells. As it happens in living cells, the molecular elements literally transform into each other, blurring the difference between the “computer” and “computed” elements of the circuit. The result of these dynamics is a sort of computation that can be interpreted in the classical manner (input/output) or according to systemic perspectives: Indeed, these systems can display linear and circular causalities, and all perform parallel computations.
Figure 1 shows the first attempt at building a CAI knowledge map in analogy to that built for AI (Corea, 2019). It is a graph whose x-axis reports the CAI domains that are split, for convenience, into two parts: Molecular and Supramolecular Chemistry and Systems Chemistry, respectively. The y-axis reports the CAI paradigms, which are the main components of the Human Nervous System (HNS), i.e., the Sensory System (SS), the Central Nervous System (CNS), and the Effector System (ES). We use these terms here because of their importance in human-centered descriptions of intelligence, but it is clear that functionally similar parts or modules can also be found in other organisms, down to unicellular ones. The problems CAI faces are properly distributed between the two coordinates of the graph. The SS, which includes the sensory cells catching physical and chemical stimuli, allows for collecting data. The ability to transform raw sensorial inputs into usable information is perception and requires the brain (included in the CNS). The brain also allows computing and reasoning, i.e., solving problems. Knowledge is generated by the ability to understand and represent the world. Planning is the capability of fixing goals. The ES, made of muscles and glands, allows working, communicating, and acting, which means pursuing and achieving the planned goals to maximize the expected utility. The principal results achieved in mimicking human intelligence through Molecular and Supramolecular chemistry are highlighted in this manuscript, along with their perspectives. The results and perspectives related to Systems Chemistry will be presented in the second part of this series.
3 Molecular and supramolecular chemistry
The behavior of molecules and how they can be employed to develop CAI depend primarily on their structure. The molecular structure is defined by specifying i) the type and number of the atomic elements that are present (through the so-called “Molecular Formula”) ii) how the constitutive atoms are reciprocally bound (through the so-called “Molecular Structure”), and iii) how the groups of atoms are arranged in the three-dimensional space. Figure 2A shows an example of a molecule that can exist under two structures (labelled as SpO and MC) that have the same Molecular Formula (
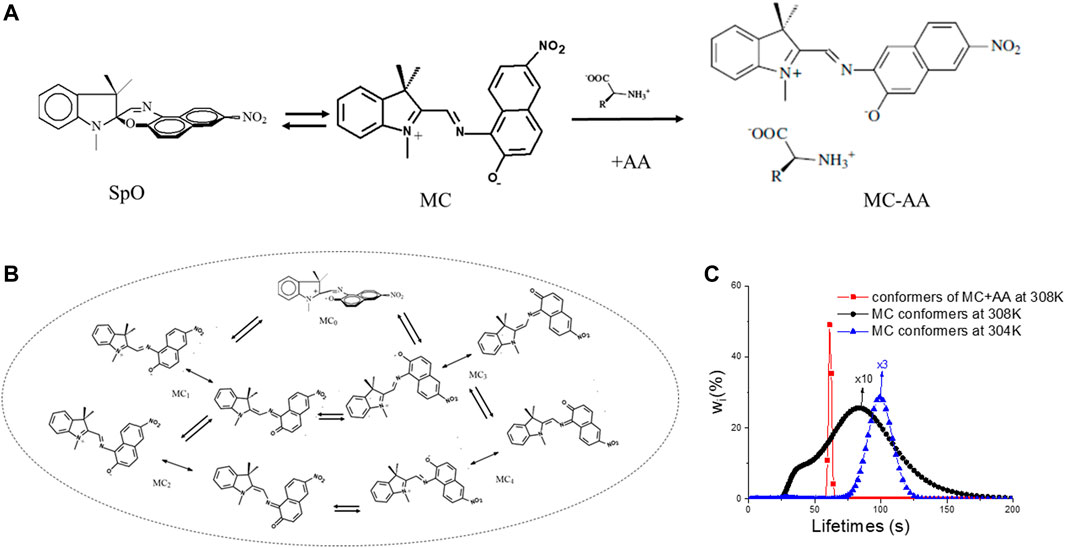
FIGURE 2. Two molecules (labelled as SpO and MC, respectively) with the same Molecular Formula but different structures are shown, along with a supramolecule generated by the interplay between an amino acid (AA) and MC in (A). (B) Graph B displays a collection of conformations for MC. (C) Plot C depicts the lifetimes’ distribution for the MC conformers at 304 K (blue trace) and 308 K (black trace), and in the presence of AA at 308 K (red trace): the conformational distribution for MC gives rise to a molecular fuzzy set whose shape and position are clearly context-dependent.
3.1 Sensing
Most of the molecules and supramolecules respond to chemical and physical inputs by changing their structures. For any solution containing a number of responsive molecules of the order of Avogadro’s number (i.e., 1023), the outputs are macroscopic variables that can be monitored through proper instruments, which often are spectroscopic. Every output is a function of the inputs, the molecular structure, and the features of the surrounding microscopic environment. Such chemical compounds are sensors of the molecular world (De Silva, 2013). Since the molecular structures and properties can change in combinational (devoid of memory effects) or sequential (including memory) manners, the behaviour of these sensors can be exploited to process information (Credi, 2007; Szaciłowski, 2008).
3.2 Computing and communicating
When the molecules are maintained in coherent quantum states (through demanding procedures of isolations), they are employed to implement quantum computing, which is alluring for its parallelism (Bennett and Di Vincenzo, 2000; Nielsen and Chuang, 2004). When the random Brownian motion, sustained by the available thermal energy, induces the collapse of quantum states through molecular collisions, it is still possible to use molecules to compute (Gentili, 2011). When the molecular states available are two or a few more, the system can be used to process binary or multi-valued discrete logics. Since the early 1990s, molecules and supramolecules have been proposed as alternatives to solid inorganic semiconductors for implementing Boolean logic gates and functions (De Silva, 2013). Since it is possible to exploit several (spectroscopic) techniques to probe the input-output relationships, molecular logic gates are reconfigurable: the logic function depends on which output is monitored. It is challenging to connect different molecular logic gates to obtain extended circuits, as in electronics, due to the variety of physicochemical inputs and outputs that are usually involved. Therefore, molecular logic gates are inappropriate for implementing the “wet” counterparts of modern electronic computers, i.e., the so-called chemical or molecular computers. However, molecular and supramolecular logic gates remain valuable probes of the microscopic world (Pischel et al., 2013). They can reciprocally communicate through collisions, after diffusing closely, or through much swifter optical signals.
When the accessible molecular states are almost infinite, it is reasonable to exploit them for processing infinite-valued logic, such as fuzzy logic. Any chemical compound that exists under a plethora of conformers (i.e., potentially an infinite number of structures that differ in the 3D arrangement of the atoms) or is embedded in micro-heterogeneous environments (Gentili and Perez-Mercader, 2022) can be used to implement a fuzzy set. Figure 2B shows some conformers of MC: they are labelled as MC0, MC1, MC2, MC3, and MC4. MC0 is obtained as soon as the C-O bond of the spiro-oxazine (SpO) is broken. It is a sort of matrix of the other conformers that are in chemical equilibrium (represented by two opposite arrows) and are obtained by mutually rotating the two-halves of the molecule. Each conformer exists as a superposition (represented by the dual arrow) of two or more structures that differ in how the electrons are distributed across the molecular skeleton. The features of MC conformational distribution, which are the number of conformers and their relative weights (i.e., the
3.3 Working
Many of the molecules employed to compute respond to the stimuli by changing their skeleton through relative movements of some of its molecular fragments, which can be assimilated to the movement of parts of a macroscopic machine. In this case, the molecules are also called Molecular Machines. Some of them carry out useful work within a cycle, and they are named Motors. Some others are simple switches because they go back and forth between two states (Aprahamian, 2020). Molecular Machines are forced to work in that peculiar environment, which is the molecular world. It is dominated by the Brownian motion, fueled by thermal energy, and it is characterised by low Reynolds numbers. Any useful work must fight against the random motion of the particles and the viscous force exerted by the surrounding micro-environment (Bustamante et al., 2001). Molecular Machines perform work at the Ångstrom and nano-levels. Their work can be transferred to higher spatial scales (from the micro-to the macro-level) if the molecules are aligned in space and act synchronously. Alternatively, work can be hierarchically transferred from the nano-to the macro-level through Systems Chemistry, as explained in the mentioned second part of this series.
4 Conclusive remarks
Molecular and supramolecular chemistry is clearly contributing to the development of CAI. Responsive molecules and supramolecules are valuable for mimicking basic functions of the human nervous system and any other biological system, such as sensing, computing, communicating, and working. The chemical compounds that play these roles assist and augment humans because they establish a direct link between our macroscopic world and the microscopic molecular world. Among all the possible physicochemical variables that can help to link the two worlds, the optical ones are particularly appealing (Gentili, 2011). As inputs, they can be focused on tiny areas, selecting the number of molecules to communicate with. As outputs, they can be easily caught even by human eyes (if they have frequencies belonging to the visible region) or conveyed over long distances through optical fibres, which work as communication channels. Each chemical compound employed in CAI can accomplish just one or a limited range of tasks. In other words, molecules and supramolecules can promote “weak” forms of CAI. We might think of approaching “general” forms of CAI by assembling many types of molecules, each playing a peculiar function and giving rise to an autonomous chemical system capable of perceiving, planning, and acting (see Figure 1). Reaching “general” CAI will probably mean having the capability of controlling the transition from non-living to living matter, as will be commented on in our next manuscript.
Data availability statement
The original contributions presented in the study are included in the article/supplementary material, further inquiries can be directed to the corresponding author.
Author contributions
Both authors extensively discussed the main topic covered in this study. PG identified the main subjects and the chemical implementations and wrote the manuscript draft. All authors contributed to the article and approved the submitted version.
Funding
This work has been funded by the European Union-NextGenerationEU under the Italian Ministry of University and Research (MUR) National Innovation Ecosystem grant ECS00000041—VITALITY—CUP J97G22000170005.
Acknowledgments
PG acknowledges the Università degli Studi di Perugia for the “Ricerca di Base” fund. We acknowledge Università degli Studi di Perugia and MUR for support within the project Vitality.
Conflict of interest
The authors declare that the research was conducted in the absence of any commercial or financial relationships that could be construed as a potential conflict of interest.
Publisher’s note
All claims expressed in this article are solely those of the authors and do not necessarily represent those of their affiliated organizations, or those of the publisher, the editors and the reviewers. Any product that may be evaluated in this article, or claim that may be made by its manufacturer, is not guaranteed or endorsed by the publisher.
References
Aprahamian, I. (2020). The future of molecular machines. ACS central Sci. 6 (3), 347–358. doi:10.1021/acscentsci.0c00064
Baum, Z. J., Yu, X., Ayala, P. Y., Zhao, Y., Watkins, S. P., and Zhou, Q. (2021). Artificial intelligence in chemistry: current trends and future directions. J. Chem. Inf. Model. 61 (7), 3197–3212. doi:10.1021/acs.jcim.1c00619
Bennett, C. H., and Di Vincenzo, D. P. (2000). Quantum information and computation. Nature 404, 247–255. doi:10.1038/35005001
Bustamante, C., Keller, D., and Oster, G. (2001). The physics of molecular motors. Acc. Chem. Res. 34 (6), 412–420. doi:10.1021/ar0001719
Butler, K. T., Davies, D. W., Cartwright, H., Isayev, O., and Walsh, A. (2018). Machine learning for molecular and materials science. Nature 559 (7715), 547–555. doi:10.1038/s41586-018-0337-2
Christensen, D. V., Dittmann, R., Linares-Barranco, B., Sebastian, A., Le Gallo, M., Redaelli, A., et al. (2022). 2022 Roadmap on neuromorphic computing and engineering. Neuromorphic Comput. Eng. 2 (2), 022501. doi:10.1088/2634-4386/ac4a83
Corea, F. (2019). Introduction to data. Everything you need to know about AI, big data, and data science. Switzerland: Springer.
Credi, A. (2007). Molecules that make decisions. Angew. Chem. Int. Ed. 46 (29), 5472–5475. doi:10.1002/anie.200700879
De Silva, A. P. (2013). Molecular logic-based computation. Cambridge (UK): Royal Society of Chemistry.
Fu, H., Zhu, X., Li, P., Li, M., Yang, L., Jia, C., et al. (2022). Recent progress in single-molecule transistors: their designs, mechanisms and applications. J. Mater. Chem. C 10 (7), 2375–2389. doi:10.1039/D1TC04079K
Gentili, P. L. (2021b). Establishing a new link between fuzzy logic, neuroscience, and quantum mechanics through bayesian probability: perspectives in artificial intelligence and unconventional computing. Molecules 26, 5987. doi:10.3390/molecules26195987
Gentili, P. L. (2011). Molecular processors: from qubits to fuzzy logic. ChemPhysChem 12, 739–745. doi:10.1002/cphc.201000844
Gentili, P. L., and Perez-Mercader, J. (2022). Quantitative estimation of chemical microheterogeneity through the determination of fuzzy entropy. Front. Chem. 10, 950769. doi:10.3389/fchem.2022.950769
Gentili, P. L. (2013). Small steps towards the development of chemical artificial intelligent systems. RSC Adv. 3 (48), 25523–25549. doi:10.1039/C3RA44657C
Gentili, P. L. (2014). The fuzziness of a chromogenic spirooxazine. Dyes Pigments 110, 235–248. doi:10.1016/j.dyepig.2014.03.024
Gentili, P. L. (2021a). Why is Complexity Science valuable for reaching the goals of the UN 2030 Agenda? Rend. Fis. Acc. Lincei 32, 117–134. doi:10.1007/s12210-020-00972-0
Goertzel, B. (2007). Human-level artificial general intelligence and the possibility of a technological singularity: A reaction to Ray Kurzweil's the singularity is near, and McDermott's critique of Kurzweil. Artif. Intell. 171 (18), 1161–1173. doi:10.1016/j.artint.2007.10.011
Lee, Y., and Lee, T. W. (2019). Organic synapses for neuromorphic electronics: from brain-inspired computing to sensorimotor nervetronics. Acc. Chem. Res. 52 (4), 964–974. doi:10.1021/acs.accounts.8b00553
Lehman, J., Clune, J., and Risi, S. (2014). An anarchy of methods: current trends in how intelligence is abstracted in AI. IEEE Intell. Syst. 29, 56–62. doi:10.1109/MIS.2014.92
Mitchell, M. (2019). Artificial Intelligence. A guide for thinking humans. New York (USA): Farrar, Strauss and Giroux.
Müller, V. C., and Bostrom, N. (2016). “Future progress in artificial intelligence: A survey of expert opinion,” in Fundamental issues of artificial intelligence. Editor V. C. Müller (Cham, Switzerland: Springer International), 555–572.
Nawrocki, R. A., Voyles, R. M., and Shaheen, S. E. (2016). A mini review of neuromorphic architectures and implementations. IEEE Trans. Electron Devices 63 (10), 3819–3829. doi:10.1109/ted.2016.2598413
Nielsen, M. A., and Chuang, I. L. (2004). Quantum computation and quantum information. Cambridge University Press.
Pischel, U., Andréasson, J., Gust, D., and Pais, V. F. (2013). Information processing with molecules—quo vadis? ChemPhysChem 14 (1), 28–46. doi:10.1002/cphc.201200157
Russell, S., and Norvig, P. (2010). Artificial intelligence. A modern approach. New Jersey (USA): Prentice Hall.
Szaciłowski, K. (2008). Digital information processing in molecular systems. Chem. Rev. 108 (9), 3481–3548. doi:10.1021/cr068403q
Wooldridge, M. (2022). What is missing from contemporary AI? The world. Intell. Comput. 2022, 9847630. doi:10.34133/2022/9847630
Keywords: chemical artificial intelligence, molecular probes, molecular motors, molecular machines, fuzzy molecules, molecular logic gates
Citation: Gentili PL and Stano P (2023) Tracing a new path in the field of AI and robotics: mimicking human intelligence through chemistry. Part I: molecular and supramolecular chemistry. Front. Robot. AI 10:1238492. doi: 10.3389/frobt.2023.1238492
Received: 11 June 2023; Accepted: 30 August 2023;
Published: 08 September 2023.
Edited by:
Vassilis George Kaburlasos, International Hellenic University, GreeceReviewed by:
Sujoy Baitalik, Jadavpur University, IndiaJerzy Gorecki, Polish Academy of Sciences, Poland
Copyright © 2023 Gentili and Stano. This is an open-access article distributed under the terms of the Creative Commons Attribution License (CC BY). The use, distribution or reproduction in other forums is permitted, provided the original author(s) and the copyright owner(s) are credited and that the original publication in this journal is cited, in accordance with accepted academic practice. No use, distribution or reproduction is permitted which does not comply with these terms.
*Correspondence: Pier Luigi Gentili, cGllcmx1aWdpLmdlbnRpbGlAdW5pcGcuaXQ=