- 1Centre of Expertise Urban Technology, Amsterdam University of Applied Sciences, Amsterdam, Netherlands
- 2Faculty of Technology, Policy and Management, Delft University of Technology, Delft, Netherlands
- 3Research Centre for Sustainable Port Cities, Rotterdam University of Applied Sciences, Rotterdam, Netherlands
- 4Faculty of Applied Sciences, Delft University of Technology, Delft, Netherlands
This study furthers game-based learning for circular business model innovation (CBMI), the complex, dynamic process of designing business models according to the circular economy principles. The study explores how game-play in an educational setting affects learning progress on the level of business model elements and from the perspective of six learning categories. We experimented with two student groups using our game education package Re-Organise. All students first studied a reader and a game role description and then filled out a circular business model canvas and a learning reflection. The first group, i.e., the game group, updated the canvas and the reflection in an interactive tutorial after gameplay. The control group submitted their updated canvas and reflection directly after the interactive tutorial without playing the game. The results were analyzed using text-mining and qualitative methods such as word co-occurrence and sentiment polarity. The game group created richer business models (using more waste processing technologies) and reflections with stronger sentiments toward the learning experience. Our detailed study results (i.e., per business model element and learning category) enhance understanding of game-based learning for circular business model innovation while providing directions for improving serious games and accompanying educational packages.
Highlights
- Game-based learning is effective for teaching circular business model innovation.
- Explorative business games for practitioners can be redesigned for higher education purposes by aligning the game narrative with the learning objectives and assignments of the course.
- Text mining methods provide opportunities to gain detailed insights in learning about circular business model innovation and in the student's perception of their learning experiences.
Introduction
The transition to a circular economy (CE) has increasingly gained attention among scholars and practitioners over the past 15 years (European Commission, 2019). CE is an alternative to the dominant linear economic model (Ness, 2008; Ghisellini et al., 2015; Geissdoerfer et al., 2017b). CE aims to close, slow and narrow resource loops, e.g., products, materials, energy (Bocken et al., 2016), and create economic, environmental and social benefits (EMF and McKinsey, 2014). Therefore, CE provides a promising way to decrease resource demands and environmental footprints while maintaining (or even increasing) social welfare and wellbeing.
Businesses developing and implementing novel circular business models (CBMs) play a crucial role in the circular economy transition (Bocken et al., 2016; De Angelis, 2016, p. 38; Geissdoerfer et al., 2017a). Business model innovation is the process of devising or improving value creation, delivery and capture by designing new business models or reshaping existing business models (Osterwalder and Pigneur, 2010; Zott and Amit, 2010). In line with the work of Guldmann (2018), our definition of Circular Business Model Innovation (CBMI) is the process of redesigning linear business models into circular business models to create, deliver and capture value through slowing down, closing and narrowing cycles.
Students in higher education are increasingly drawn to CE knowledge and practices. Many of them will become future CBM innovators or—at least—stakeholders affected by novel CBMs. This paper aims to contribute to teaching business model innovation toward circularity by evaluating an education package around a serious board game called “Re-Organise—The Game”, abbreviated as Re-Organise, developed in earlier work (Lange, 2019b). We hypothesize that explorative and design-driven serious gaming is a powerful method to simulate and teach the intricate dynamics of shaping and implementing CBMs. In addition, we study the extent to which students learn and perceive their learning experience in detail. The findings of this study contribute to the further development of circular economy business modeling in higher education.
In the following sections, we first elaborate on the challenges regarding circular business model innovation in higher education and explore using game-based learning in circular business model education. Then, we introduce the game and the education package. Next, we explain how we studied the learning effects of the Re-Organise education package. The last sections discuss the learning effects and what this means for future research and education concerning circular business model innovation.
Literature Review
Learning and Teaching in Higher Education
Literature on game-based learning in higher education lacks shared definitions of learning (Vlachopoulos and Makri, 2017). However, there is abundant general literature on learning taxonomies. Scholars generally accept three learning taxonomies (Dee Fink, 2013): (1) cognitive, involving understanding, thinking and solving problems; (2) affective, comprising interaction with others and self-reflection; and (3) psychomotor, entailing experiential learning, reflection and action. The most influential cognitive taxonomy is the one created by Bloom (1956) and revised by Krathwohl et al. (2002). The taxonomy describes six types of learning, from remembering knowledge to creating solutions. Teachers have widely used it to design teaching activities. However, this taxonomy has been criticized for lacking affective and psychomotor aspects of learning. Several scholars have created taxonomies to structure those learning perspectives (e.g., Krathwohl, 1964; Cooper and Harrow, 1973). Dee Fink's (2013) taxonomy is a widely used and practical framework that integrates cognitive, affective and psychomotor learning into six learning categories: foundational knowledge, application, integration, human dimension, caring, and learning how to learn. This study uses Dee Fink's taxonomy to provide structure in analysing the learning impact.
Circular Business Model Innovation
Circular business models are rooted in multiple business and sustainability-related fields (Geissdoerfer et al., 2018). Some of these fields are Industrial Ecology (Graedel and Allenby, 2010), Cradle-to-Cradle (Braungart et al., 2007) and the Performance Economy (Stahel, 2008). Based on the work of Richardson (2008), Geissdoerfer et al. (2018) state that CBMs articulate the logic of how organizations create, deliver, capture and offer economic, environmental and social value to multiple stakeholders by closing materials cycles. Circular business model innovation—more than regular business model innovation—involves developing a comprehensive set of novel collaborations among previously unacquainted stakeholders (Geissdoerfer et al., 2018). While traditional business model innovation is already challenging and time-consuming (Chesbrough, 2010), sustainable business modeling—such as CBMI—is even more complex (Roome and Louche, 2016). Understanding how business model designs affect key activities, processes, and economic, environmental and social impact across multiple stakeholders is crucial to achieving circularity. To do so, students need to understand, apply and integrate all dimensions of circular business models, including value proposition, value creation and delivery and value capture for creating and maintaining successful circular practices. EMF and IDEO (2016) provided a business model canvas to support this broad integration of CBM dimensions. It is built upon the traditional canvas elements by Osterwalder and Pigneur (2010), addressing circularity and multiple sustainability values.
Challenges in Teaching Circular Business Model Innovation
Innovating circular business models requires a variety of competencies because of the broad set of stakeholders involved, each with different economic, environmental and social values. Professionals designing new circular business models are expected to have combinations of applied knowledge and skills regarding operations and organization and develop attitudes that enhance coordination collaboration (Herczeg et al., 2018). We now elaborate on the different types of learning required for circular business model innovation.
Content-Related Competencies
Circular practices involve connecting output flows to production inputs, often first converting the waste streams into usable resources, requiring technological and organizational knowledge and skills. Furthermore, students must consider multiple CE strategies (PBL Netherlands Environmental Assessment Agency, 2019) for economic, environmental and social value creation and capture (EMF and IDEO, 2016). Thus, students need to understand, create and apply business models that describe the implementation of new technologies and organizational arrangements (Guldmann and Huulgaard, 2020). They also need to understand and apply foundational concepts of the circular economy and sustainable impact (Centobelli et al., 2020). In this study, we refer to these as content-related competencies.
Personal and Interpersonal Competencies
CBMI requires more than connecting residual materials to production processes.
It entails the creation of shared and sustainable value among partners and customers, spanning across organizational boundaries (Geissdoerfer et al., 2018). Creating collaborations across stakeholders requires personal and interpersonal skills, behaviors and attitudes. The viewpoints regarding normative sustainability goals and affective competencies to reach collaborations are crucial to creating and maintaining a circular business (Breuer et al., 2018). Thus, students need to reflect upon their learning experiences and develop values, interests, and feelings related to the topic, their peers and the learning process.
Iterative Design Thinking Competencies
CBMI is characterized by highly uncertain and changing conditions (Guldmann, 2018, p. 78). Companies may hesitate because alternative options are automatically discarded by cooperating and investing in certain resource conversion technologies. A competition among technical, organizational and network solutions may thus emerge (Korhonen et al., 2018). As circumstances change and stakeholders learn and adapt, CBMs support conversations among stakeholders to improve the CBM design. Thus, learning outcomes in CBMI education must include the corrective iterative nature of CBMI (Wynn and Eckert, 2017). Improving and learning are basic elements of CBMI (Baldassarre et al., 2019) since iterative design thinking processes support integrating theory with practice. Therefore, design thinking must be included in teaching CBMI (Geissdoerfer et al., 2016).
To summarize, teaching CBMI should include technical and social aspects, facilitate stakeholder integration, follow collaborative and inclusive processes (Breuer et al., 2018), and incorporate iterative design thinking to develop new collaborations and organizational arrangements in a dynamic environment. It needs personal and interpersonal reciprocity and integration of technical and social perspectives. Iterative thinking is a key element in CBMI (Geissdoerfer et al., 2016), requiring reflective action, adaptation or even rethinking the CBM design. The students learning about CBMI need to be engaged in all learning domains. Teaching students about these complex issues and processes strictly theoretically is therefore insufficient.
Game-Based Learning for Circular Business Model Innovation
Higher education institutes increasingly use serious games as rich, safe and interactive learning tools (Caponetto et al., 2014). Serious games enable players to experience situations in a virtual world, aiming to have a positive and meaningful impact on skills development (Susi and Johannesson, 2007). Fraccascia et al. (2021) show that digital games are suitable for teaching business dynamics of circular practices but are limited in direct communication. Board games have not yet been studied as extensively as digital games, but they are considered suitable for collaborative games due to their transparency and immediate interactive nature (William et al., 2018). Serious games are explicitly suitable for solving complex problems in collaborative settings (Geurts et al., 2007). They provide a safe virtual learning environment, allowing students to make mistakes without risk. Game-based learning—learning during and from playing serious games—fosters learning engagement through role-playing (Tobias et al., 2014).
Numerous studies have shown that teaching sustainability through game-based learning is effective (e.g., Katsaliaki and Mustafee, 2012; Bevilacqua et al., 2015; Chappin et al., 2017). Serious games have already been developed to teach sustainable design decisions (e.g., Clarke, 2020), circular economy design and business opportunities (e.g., Inchainge, 2019), finance-focused circular economy (Whalen, 2017) and circular product design (Whalen and Peck, 2014; Whalen et al., 2018). Multiple games have been developed to teach business modeling (e.g., Innovative Dutch, 2021) and business model innovation (e.g., Davidovici-Nora, 2013). Fraccascia et al. (2021) conclude that game-based learning is necessary to teach innovations in circular business modeling. Manshoven and Gillabel (2021) recently published an article presenting a game for educating business model innovation. They tested the learning effects using game observations and post-test surveys.
Literature suggests increasing rigor, detail and structure to enhance understanding of game-based learning for CBMI. Rigor is obtained through utilizing pre- and post-test data from randomized experiment and control groups (Connolly et al., 2012). Boyle et al. (2016) suggest studying the interplay between game design and mechanics, other educational activities and learning on a detailed level. By connecting game mechanics to learning taxonomies, a structured foundation for understanding and communicating the effects of game-based learning is provided (Arnab et al., 2015).
Study Objectives
CBMI is already challenging for practitioners, let alone for students without working experience. Therefore, teaching must take place in a safe but realistic learning environment to facilitate students becoming the circular professionals of the future. Most existing studies on game-based learning of CBMI use data from game observations and post-test surveys. By experimenting using our education package Re-Organise, this study furthers understanding game-based learning for CBMI. We collected data from a pre- and post-test experimental and control randomized group study. We analyzed this data on a detailed level: per business model element (EMF and IDEO, 2016) and learning category (Dee Fink, 2013). By doing so, this study aims to provide rigorous, detailed and structured recommendations for enhancing research on game-based learning for circular business model innovation while improving the game and accompanying educational package.
Methods
We start this section by presenting the origin of the game, the game characteristics, and the game education package, including student assignments. Then, we explain how we conducted the experiment and collected data from the submitted student assignments. Last, we explain how we analyzed the data to explore any learning effect.
Re-organise—A Game Originating From Case Study Research
Re-Organise is an explorative and design-oriented serious game. It was based on practice-oriented research in two case studies of agro-industrial symbiosis networks in Amsterdam, the Netherlands (Lange et al., 2017; Mulder et al., 2018). These networks consist of industrial and commercial actors that exchange (mainly biotic) materials, energy and information to increase resource efficiency (Lombardi and Laybourn, 2012). The game has been developed in two versions: version 1 for the research project and version 2 for the education package.
In the research project, practitioners in the two cases aimed to find ways to exchange, process and re-use their waste streams locally. By doing so, they intended to increase the creation—and avoid the loss—of economic, environmental and social value (Mulder et al., 2018). The board game was developed by a consortium of project researchers, circular economy consultants and a game developer. Re-Organise is a typical simulation game, creating a scenario-based environment. It consists of various real-life activities, phenomena or mechanisms and has rules to simulate reality for training and learning (Vlachopoulos and Makri, 2017). We used the game for two purposes. Firstly, it was used to validate collected data regarding waste streams and necessities with the stakeholders. Secondly, it was used for the ideation of new closed-loop value networks. The game resulted in the co-creation of several collaborative and circular business model concepts. After ending the research project, one of the concepts created during gameplay, was implemented in the form of a composting cooperative (Tuinen van West, 2019).
We anonymised the game and added an education package to teach circular business model innovation to bachelor and master's degree students. The game and education package were iteratively redesigned to improve the learning experience during two pilot studies with undergraduate engineering students and lecturers at the Amsterdam University of Applied Sciences.
Game Characteristics
Re-Organise—The Game is a collaborative multiplayer board game consisting of 8–16 roles (Figure 1).
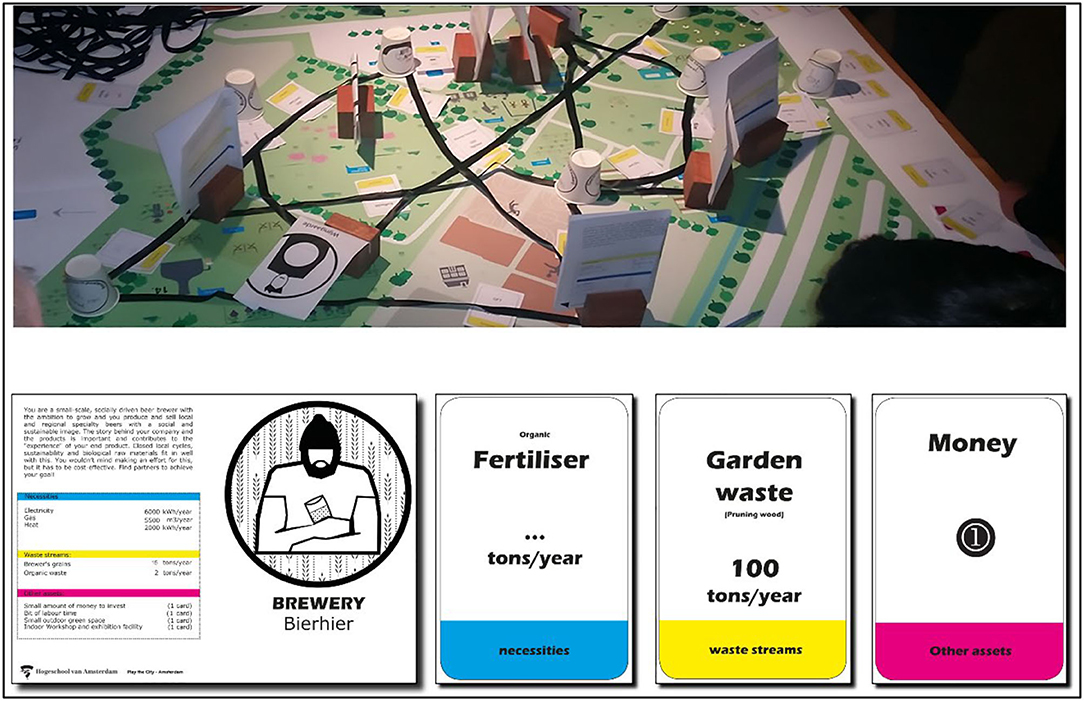
Figure 1. An impression of Re-organise—The Game. The game consists of a board, technology and role pawns, connection ribbons and play cards (representing necessities, waste streams and other assets).
In the game, each player (or duo) takes on the role of an organization within an agro-industrial park. A role description is given on a card placed as a pawn on the board. Examples of game roles are a cattle farmer, restaurant owner and recreational area manager (public institution). The players' goal is to find as much local use for their waste streams (represented by “Waste” cards) as possible while maximizing the fulfillment of their own needs (“Necessities” cards). To achieve this, they can use several assets (“Other assets” cards) to invest in processing technologies. The optimal outcome for a player is to play all waste and necessities cards on the board without using other assets. The players are free to do whatever it takes to close their loops, provided that the game is played in a respectful, safe manner, and the rules as described on the cards are being followed. Despite the freedom given to the players, the gameplay unfolds more or less according to the process in Figure 2. The game ends after a given playing time of 1 h.
Table 1 shows how real-life elements were reflected in the first version (business game) and the last version (education game).
We aimed to explore solutions in the original game for the research project. However, in education, we aimed to teach the broader concept of CBMI. Therefore, we iteratively redesigned the game during the pilot to address this change of purpose. Compared to the original game, some complexities were simplified or added to improve the game experience for our teaching purposes.
One of the improvements entailed reducing the variety of waste cards by rounding off quantities to multiple units of 1, 2, 5, 10, etcetera. To avoid players losing interest or wasting time making exact input-output calculations, we deleted the quantities from the necessities cards and allowed gameplayers to estimate the quantities roughly. The third difference with the original game was the addition of the “other assets” cards. We intentionally left out any obstacles to creating novel business concepts during the research project to increase creativity among case study participants. However, for educational purposes, we decided it was necessary to enrich the game experience with the notion that assets such as money, time, space, network connections, and knowledge are required to close the loop. The “other assets” cards created this extra dimension. For example, the players had to collect money, time, and space cards to invest in technology. The instructions were provided on the technology pawn.
Game Education Package
According to the principles of constructive alignment (Biggs and Tang, 2010), the education package was created to align learning objectives (study and gameplay), activities and assessment.
Based on the literature study, the following learning objectives were determined:
Content-related competencies, i.e.:
1. Applying biotic waste streams, resource needs, processing technologies and other assets to close loops in an agro-industrial symbiosis network;
2. Applying the Circular Business Model Canvas by EMF and IDEO (2016).
Personal and interpersonal competencies, i.e.:
3. Understanding different perspectives and goals of the various actors, including their own;
4. Reflective skills on the learning experience.
A design thinking competency, i.e.:
5. Understanding and experiencing that CBMI is a dynamic, iterative design process.
To achieve the learning goals, we provided the student with the education package consisting of the following items:
• the game, and a flip-over to summarize the game outcomes.
• digital supplementary teaching materials:
• an introductory presentation (presented during a lecture);
• a digital reader with a brief explanation of the assignment, a case description, and descriptions of the roles and technologies;
• an assigned role (ticket).
• digital assessment materials:
• a circular business model canvas (CBMC) questionnaire; and
• a reflection questionnaire.
We assessed the learning experience utilizing two existing methods. Students filled out a Circular Business Model Canvas (CBMC) to prove that they achieved the required learning goals. In addition, a reflection assignment based upon Dee Fink's learning taxonomy was used to demonstrate the students' perception of learning. The CBMC assignment consisted of the canvas provided by EMF and IDEO (2016); (see Appendix A). The CBMC was assumed to be easy to comprehend by our students because the traditional canvas is widely known by many students that obtained a bachelor's degree in business and technology innovation. The canvas has widely been in use since 2017. Furthermore, we assumed the questions in the CBMC to be quite straightforward. The reflection assignment consisted of a questionnaire based on the taxonomy by Dee Fink (2013) to address knowledge construction, problem-solving skills and affective attitudes and behavior. The questions in the reflection assignments can be found in Appendix A.
The following sections show how we conducted an experiment using the submitted CBMs and personal reflections to measure the actual and perceived learning experience. Furthermore, we show how a mix of qualitative and quantitative data analysis methods was used to provide insights concerning the required competencies.
Experimental Design to Measure Learning Effects of Gameplay
We conducted an experiment with 85 first-year students at the Master of Science Program Industrial Ecology (joint degree of Delft University of Technology and Leiden University, the Netherlands), and furthermore, 11 undergraduate students and PhD students from the Faculty of Technology, Policy, and Management (Delft University of Technology, The Netherlands), (see Table 2).
Many Industrial Ecology students were new to the field, as the experiment was performed during their introduction week. About half of the participants were international, and the other half were Dutch. English was the language used, but this was not the primary language for most students. The students attended a lecture 2 days before gameplay in which they were introduced to the game. During the lecture, they received the reader and the assignments. They were randomly given a role ticket. The students filled out and submitted the CBMC and reflection assignment in the following days. Then, we split the group into a game group and a control group, ensuring that the roles were equally distributed. The game group first played the game, then discussed the outcomes during an interactive tutorial in the classroom and updated both CBMC and reflection assignments. The control group students first discussed their CBMCs and reflections during the interactive tutorial. They updated their work and then played the game afterwards. Three weeks after playing the game, the students were asked to reflect and discuss their opinions on their learning experience during a lecture. Furthermore, they provided written feedback during the course evaluation.
Data Analysis Methods
Table 3 provides an overview of how various data collection and analysis methods contribute to multiple perspectives on this study's learning objectives. Text mining is a quantitative manner of automatically extracting and processing written information by computer. It supports gaining new insights on that information (Hearst, 2003). We generally followed the generic text mining steps from Kwartler (2017). Table 3 shows that three simple text mining analysis methods were used for the comparative analysis. These are: (1) comparison of pre-test and post-test CBMC and reflection answers per student, (2) a comparison of the use of technology and role card terms in the CBMCs, and (3) a sentiment analysis of the answers in the submitted reflection. After text organization and data clean-up, the work of 79 students (44 in the game group, 35 in the control group) remained suitable for data analysis.
Difference Between Pre-test and Post-test CBMC Answers
We compared the word-by-word difference between pre-test and post-test CBMCs of each student. The results show us whether the CBMCs were filled out multiple times. Since scholars widely consider repetition as an important premise to the improvement of knowledge and skills (Brabeck and Jeffrey, 2014), this measurement tells us which group experienced a better application of the CBMC (learning objective 2) and a more thorough learning reflection (learning objective 4). Furthermore, the change of answers shows which of the groups experienced the dynamic and iterative nature of the design process (learning objective 5).
We performed multiple text organization, data cleaning and feature extraction steps. First, the pre- and post-test answers were extracted from the submitted work, anonymised and ordered per student, group, and question. The data was cleaned, and unanswered questions were tagged as such. In addition, the complete answers of the pre-test and post-test were ordered per question. Then, the absence of answers in both pre-test and post-test and the presence of similar patterns were detected.
For visualization and analysis purposes, the results were ordered in four categories of answers, see Results:
• “Both no answers”, in which no answer was given in both the pre-test and the post-test,
• “Same answer”, in which the pre-test and post-test consisted of precisely the same answer,
• “Addition to the first answer”, containing a similar pattern between pre-test and post-test, and an addition, and
• “New answer”, in which the pre-test answer was completely removed and exchanged for a new answer.
To gain insights into the words most used, we also created word clouds of the control and game group (see Appendix B).
Use of Information From the Technology and Role Cards in the CBMCs
We measured the extent to which words used in each student's complete work (pre-test + post-test) related to the game cards (i.e., technologies, waste streams, needs and other assets). By doing so, we compared the effect of gameplay on students' learning objectives 1–3.
This measurement's text organization and feature extraction steps were conducted as follows. First, a document-term matrix was constructed from the anonymised data by extracting the answers from the CBMC questions. Another document-term matrix was constructed from the technology cards and the role cards of the game. The data was ordered into four categories. The categories included words related to: (1) technology names on the technology cards and terms related to the (2) waste streams, (3) needs, and (4) other assets on the player's role cards. Then, both document-term matrices were cleaned by:
• correcting misspellings;
• removing punctuation, numbers, capitals and whitespace;
• removing stop words and irrelevant words, e.g., words related to the university name or game material;
• replacing synonyms through lemmatization;
• word stemming (reducing inflected words) to avoid a mismatch between words with the same meaning but a different suffix.
The card words used at least once in both pre-test and post-test CBMCs were then identified by comparing the cleaned document-term matrices. We visualized the percentage of words used in the CBMCs compared to the total number of relevant words in the game cards (see Results). The word clouds in Appendix B show the words most used.
Difference Between Pre-test and Post-test Reflection Answers
The answers of the two measurements of the submitted reflections were examined. These questions are about the perception of learning, according to the learning categories of Dee Fink (2013). In the same way as the CBMCs, we compared the differences between the answers per learning category, see Section Results.
Sentiment Analysis of the Answers to the Reflection Assignments
Sentiment analysis is intended to measure the emotional intent of persons based on the text these persons produced (Kwartler, 2017). In this study, we used the sentiment dictionary by Hu and Liu (2004) to tag polarized words (RDocumentation, 2019) and measure the emotional intent of students toward their learning experience. The polarity of the student's answers indicates how positive or negative the students think about each of these categories. A polarity of 0 represents a neutral sentiment; 1 is positive; −1 is negative. A change in polarity score between the pre-test and post-test shows whether the students felt positive or negative sentiments toward the game-based learning experience. We set the number of words considered valence shifters at 6 before and 3 after the polarized word to optimize the polarity differences. The sentiment clouds in Appendix B provide insights into the relationship between words and sentiment.
Qualitative Data From Student Feedback and Observations
Through observations during the game, classroom feedback, and course evaluation, we gained more insights into students' learning experiences and perceptions.
Results
Students from the minor Data Science largely prepared data extraction from the submitted work and visualization code at AUAS (Mooij et al., 2020; Zweep et al., 2020). The anonymised data is available in a public data repository (Lange, 2019a). In addition to the quantitative results presented in this section, Appendix B provides several word clouds to get a feel for the data.
Difference Between Pre-test and Post-test CBMC Answers
The measured differences between CBMC answers are shown in Figure 3.
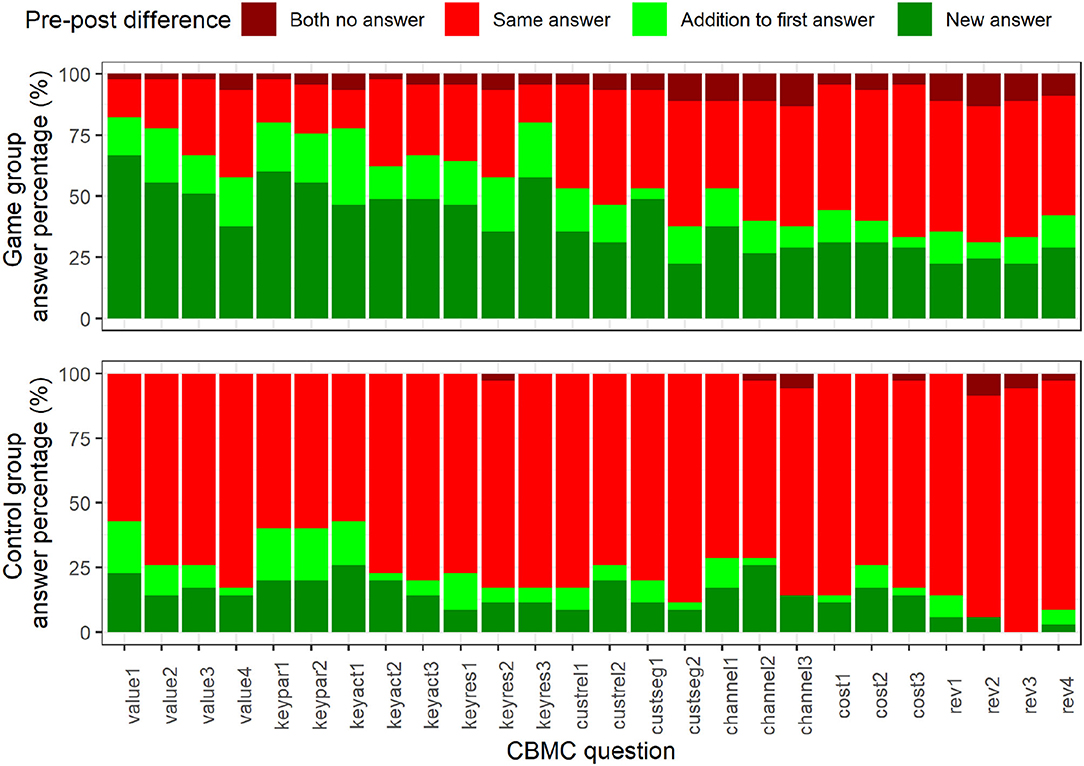
Figure 3. Difference between pre-test and post-test CBMC answers. The questions used in the CBMC assignments can be found in Appendix A. Visualization prepared by our students (Mooij et al., 2020).
These results show that the game group made more changes in all questions of the post-test CBMC assignment. Questions related to the value propositions, key partners and key resources changed more than 50% after gameplay. However, the game group also contained more unanswered questions, particularly channels and revenues. The game group participants were more actively involved in applying the CBMC for the second time, indicating a positive learning objective 2. Furthermore, the game group students have experienced a more dynamic and iterative design process, positively affecting learning objective 5.
Use of Information From the Technology and Role Cards in the CBMCs
The use of information from the cards in the submitted CBMCs is shown in Figure 4.
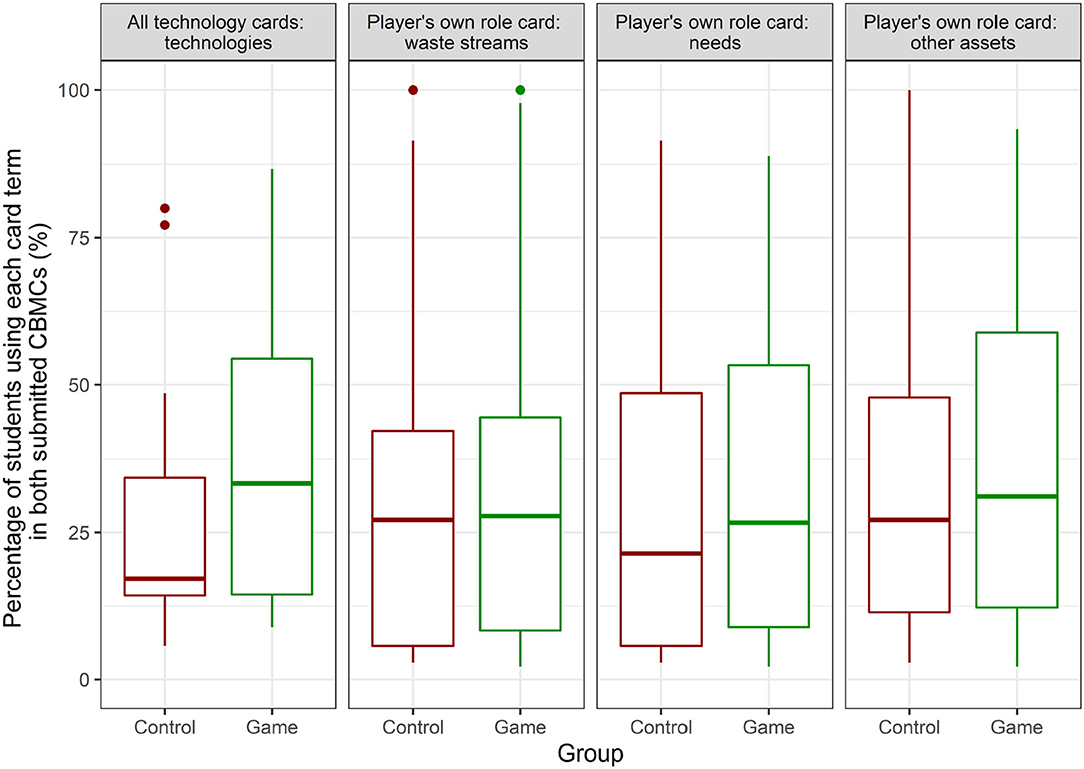
Figure 4. Percentage of students using each card term in both submitted CBMCs. Standard Tukey boxplot representation.
The small sample size weakens the results, and therefore, we found no statistically significant differences between the groups. Nevertheless, these boxplots indicate that the game group used more terms related to technologies and needs and other assets to a lesser extent. These results suggest that there is a positive impact of the game on learning objective 1 (application of technologies) and 3 (understanding the roles) and more thorough use of the CBMC (learning objective 2).
Difference Between Pre-test and Post-test Reflection Answers
In the same way as the CBMCs, the answers between the pre- and post-test reflections were examined. This time, each of the answers to questions regarding the learning categories of Dee Fink (2013) were compared, (see Figure 5).
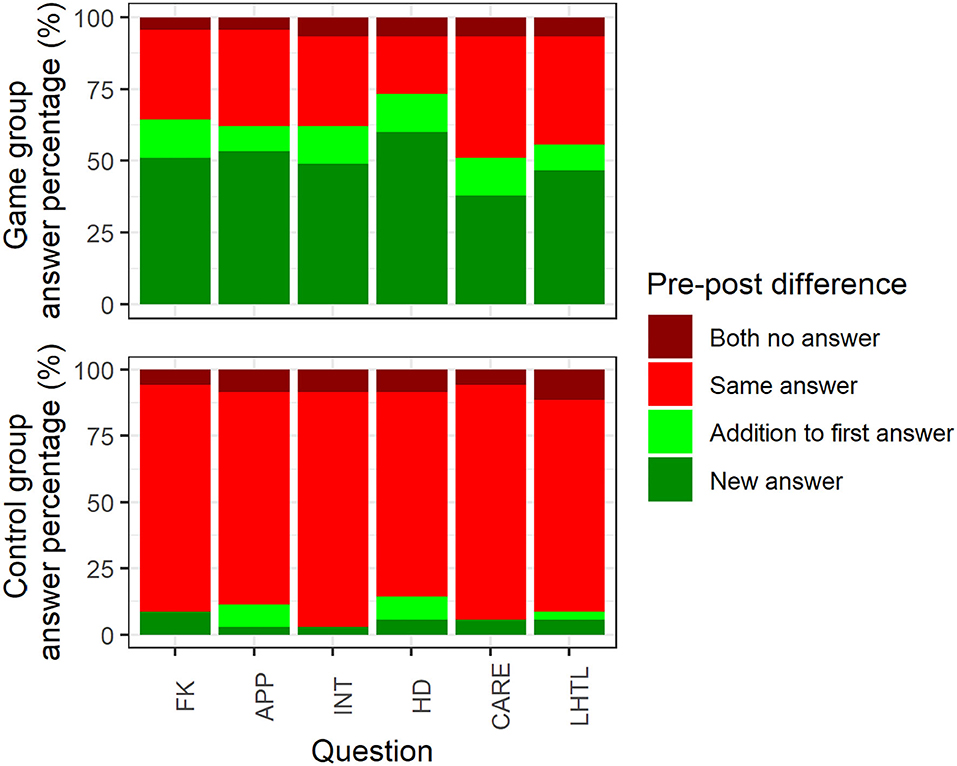
Figure 5. Difference between pre-test and post-test reflection answers. The questions can be found in Appendix A; each involves one question per learning category (Dee Fink, 2013). Students prepared this visualization (Mooij et al., 2020).
The results provide insights into the extent to which the perceived learning experience of students evolved. The game group participants made much more changes to the reflection than the control group. In line with Dee Fink (2013, p. 7), changes in thinking affect learning positively. Game group students have thus practiced more reflecting on the learning experience (learning objective 4). Since the game group reflections show more answer differences, we can conclude that the game positively affects learning about iterative design thinking (objective 5).
Sentiment Analysis of the Answers to the Reflection Assignments
Figure 6 shows that the average polarity did not change. However, the boxplots' variance (i.e., interquartile range) reveals that many answers from the game group resulted in a more significant polarity change than the control group students. These results indicate that gameplay leads to practicing a more thorough and outspoken reflection in the post-test (learning objective 4). The game group experienced more positive or negative feelings concerning application and integration of knowledge, for example, excitement or disappointment by (not) realizing their plan. Moreover, we observed a broader sentiment variance concerning learning how to learn (LHTL). This variance suggests that the game invokes a stronger sense of contextual dynamics and the need for iterative design (learning objective 5). The question regarding the category Human Dimension (HD) reflects that game group students were clearly either more positive or negative about learning from themselves and other participants, referring to perspectives and goals of the various participants (learning objective 3). The sentiment cloud in Figure 7 gives an impression of the words used by the game group in this learning category.
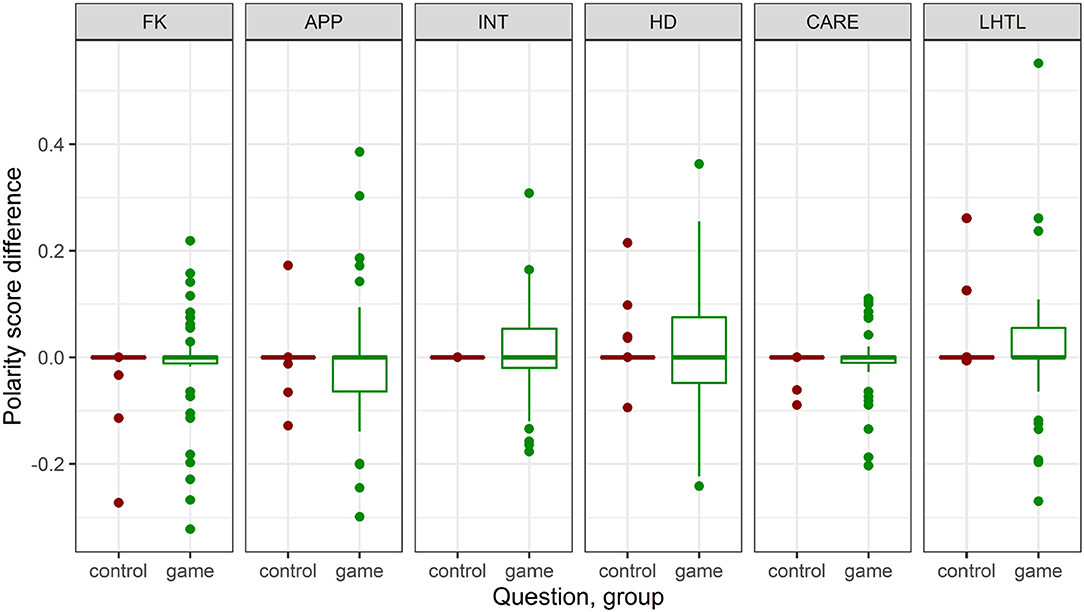
Figure 6. Polarity score difference of student answers to the reflection questions. Standard Tukey boxplot representation. The questions can be found in Appendix A.
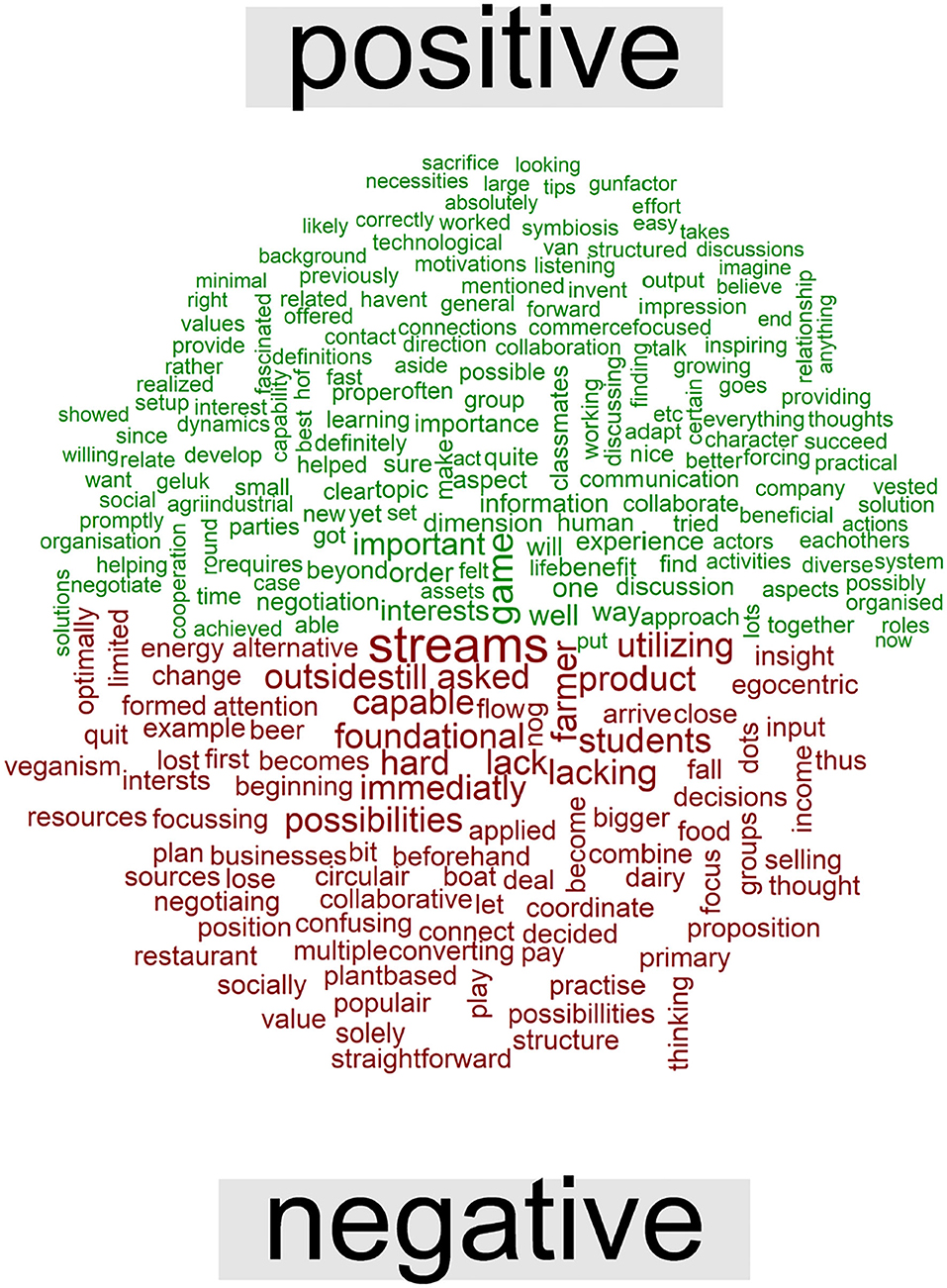
Figure 7. Sentiment cloud of the terms used in the learning category “Human Dimension”. Source: submitted reflections of the game group.
Student Feedback and Other Observations
A qualitative analysis of the participant's feedback was performed to give more meaning to the results.
In the course evaluation, students from the Industrial Ecology program generally found it “a nice game to get the feeling of complexity and how processes can go unstructured”. However, few students did not quite understand why the game was not structured: they felt it would be “more professional and better prepared” if it were more bound by rules. Some students came up with ideas to improve the game in that direction. We explained to these students that coincidence and the unstructured nature of the game were deliberate elements to simulate real-world complexity and dynamics. Some students would have liked to have more background to apply the knowledge better, especially on applying the business model canvas. The business model canvas was more thoroughly explained in the discussion sessions (control group step 2 and game group step 3). The students declared that this made them feel more familiar with the canvas and comfortable using it. Their comments implied that more time and effort should be invested in explaining the CBMC and building up experience with its various elements. A few students declared that they disliked playing board games and did not like the assignment. Furthermore, a student suggested combining the game with other games to extend the learning scope on CBMI, industrial symbiosis and network development.
Discussions with participants of the mixed group of undergraduate and PhD students showed that they enjoyed the gameplay and found it instructive. However, these students were volunteering in their own time, so we can assume that they already had an intrinsic motivation to play a game about the topic. In addition, these students all have some degree of knowledge about the business model canvas and the circular economy.
Discussion
Reflection on the Experiment Results
The experiment results show that gameplay invoked the students to put more effort into adapting their work. It supported creating richer business models. Game participants used a richer palette of technologies, fulfilled needs and used other assets. However, the game did not result in more waste streams being re-used. The game imposes some boundaries on the maximum amount of waste to be recovered. It thereby simulates competition between the various solutions due to limited resources. However, we also conjecture that students' limited game time may have resulted in unoptimized resource recovery.
The results show the extent to which business model elements were affected by gameplay. For example, in “customer segments” and “revenues”, the questions related to who else might benefit from the business model were the least adapted by both groups (although still more than the control group, which almost left these topics unchanged). Since these questions require more background knowledge of the case, the limited modifications may be caused by the limitations of the case description and the simplified world the game represents. Thus, educators must be aware that the learning goals in mind are properly addressed by the game and accompanying educational materials. Another option is to iteratively adapt the game when certain questions are not addressed satisfactorily.
Furthermore, sentiment analysis results offer detailed insights into the students' perceived progress per learning category. The game resulted in stronger sentiments regarding the learning experience. The change of sentiment was the highest in learning categories application, integration, human dimension and learning how to learn. In the application and integration categories, negative sentiments were related to some students' frustrations when their pre-test CBM was not implemented during gameplay. These negative sentiments imply that students learned about competition in CBMI. Positive sentiments were more related to a sense of victory when loops were closed. Students in the game group had stronger opinions on integrating knowledge, whereas the control group students remained neutral on this topic. Thus, the game engaged students to integrate and connect ideas, technologies or people/organizations. The game also invoked stronger feelings on learning from themselves and others and the learning effectivity of the gameplay, implying that the perception of affective learning varies more as a result of the game.
The sentiment analysis and the qualitative evaluation strongly suggest that the game invokes a stronger sense of contextual dynamics. Some students only discovered during the evaluation lecture afterwards that the unstructured nature of the game was a deliberate element to teach complexity and dynamics. Thus, the game often makes students experience a rollercoaster of frustrations, passion and emotions, which provides a (perhaps even critical) positive influence on learning (Tyng et al., 2017).
Key Findings and Implications
Effect of Gameplay on Learning and Implications for Research and Education
The effect of gameplay for each learning objective, as stated in Section Game Education Package, is discussed below.
Content-related competencies:
1. The game encourages the application of a more diverse set of resource needs, processing technologies and other assets to close loops in an agro-industrial symbiosis network.
2. The game motivates students to rethink their business model designs. Although students in both groups found the discussions on business model canvasses enlightening, the control group did not change their canvasses based on these discussions. These findings imply that the game positively impacts learning to apply the canvas. This evidence supports the conclusion of Fraccascia et al. (2021) that games are suitable learning tools for teaching circular business model innovation.
Personal and interpersonal competencies:
3. The game helps students understand the different perspectives and goals of the various actors, including their own. Evidence can be found in modifications made in canvas elements value proposition, key partnerships and customer relationships. In the reflection assignment, the human dimension learning category was mostly affected by gameplay. In this category, a change of sentiment was strongly present.
4. Gameplay motivates students to reflect on their learning experience, as seen in the differences in modifications of the game and control group reflections.
Design thinking:
5. The answers to the CBMC and reflection assignments and the student feedback show that the game increases an understanding of the dynamic, unstructured and iterative process of CBMI. It also shows the need for an evaluative discussion after finishing the assignment to help some students understand the unstructured and competitive nature of the game. The richness of the submitted game group CBMCs shows that these students got to fulfill more needs and use more technologies and other assets.
To summarize, the results show that the aimed content-related, personal and interpersonal and iterative design thinking competencies are better developed after gameplay. Furthermore, the students' perceptions of their learning were also demonstrably higher after gameplay.
Experiment and Analysis Methods and Implications for Research
Using pre-test and post-test experiments with control and game groups provides strong evidence of how the circular business model canvas by EMF and IDEO (2016) and students' reflections evolved from game-play. We have shown that this can be done on a detailed level, i.e., results are visible per business model canvas element and learning category.
To our knowledge, measuring and analyzing the student's perception of learning using Dee Fink's taxonomy is new in both the context of game-based learning and text mining. The taxonomy assumes that learning goes beyond the cognitive domain and that change is one of the most important learning indicators (Dee Fink, 2013, p. 7). Applying the sentiment polarity method on Dee Fink's six learning categories enabled us to measure each student's change of perception per learning category. This study thus demonstrated that change is measurable and can be analyzed employing digital text collection and mining methods.
The use of text mining for analyzing written information from submitted student assignments is gaining attention among game researchers and educators but is still in full development (Ferreira-Mello et al., 2019). Some text mining methods, such as sentiment analysis, have been used to measure learning in serious board games, e.g., William et al. (2018). The novelty of this study lies in proposing a method to match data from an existing CBMI tool and learning taxonomy with learning objectives (Table 3). By using mixed quantitative and qualitative data analysis methods, this study contributes to a broad and deep understanding of game-based learning.
To conclude, the amount of detail our experimental method provides can guide researchers and educators in shaping games so that the desired business model elements and learning competencies are addressed according to the needs of the course program.
Game and Education Package Design and Implications for Education
Although the original purpose of the game was to help practitioners explore solutions for closing the loop, its purpose could be extended to the broader scope of circular business model innovation after modifying the game. During redevelopment, the game was used with various players from different backgrounds. Undergraduate, graduate and PhD students from different programs, part-time students with professional backgrounds, and research participants played the game for different purposes. The students in our pilots merely learned about the concept of closing loops; students participating in our experiment learned about CBMI.
The business model canvas' format provides formulated open questions, making the assignment comprehensible to students. The used business model canvas is only one of many (circular) business modeling tools. The game can thus be combined with other tools if it has a certain common ground with the game elements (i.e., technologies, roles, and waste streams). The game is versatile, and it can be tailored for different learning objectives. Still, it must not be used standalone: it always needs additional materials and activities to help the participants understand and interpret the meaning of gameplay.
Limitations and Avenues for Future Research
Some limitations concern our sample group. The groups of students consisted of a diverse mixture of Dutch and international students with different educational backgrounds. As a result, we observed a dichotomy between experienced and inexperienced students regarding the circular economy, business model innovation, and reflective skills or customs. English was not the native language for most students of our experiment, and specific circular business modeling jargon was even more challenging for many students. We have attempted to deal with these differences by randomizing the division of students into groups, using pre- and post-test results to measure the development of each student, and using text mining methods to support the software in finding the right co-occurrences with the reader. Still, it would be of value to experiment with other types of students and groups, e.g., native English-speaking students or professionals in further education and compare the differences in outcomes. Furthermore, we expect that the game facilitator may also affect the results. Therefore, we recommend providing both lecturers and students with a minimum level of knowledge regarding the business model canvas and the taxonomy of Dee Fink.
The board game was intended to be played in a setting of multiple interacting participants to encourage free communication as if it were happening in real-world conversations. However, negotiations are increasingly being held through digital communication (Harvard Law School, 2020), especially during the COVID-19 pandemic. Therefore, we recommend converting this game into a digital variant, in which participants can communicate through chats or other social media services. It could provide interesting insights into the similarities and differences between physical and digital negotiation and its effect on CBMI. This game is created in the small context of agro-industrial symbiosis networks. It thus only simulates local and small-scale exchange and processing of organic materials, which may not appeal to all target groups. Future work may therefore encompass the simulation of technical material loops on multiple scales of collection and re-use. It would also be interesting to develop a less unstructured game version that includes the role of a facilitator to demonstrate how this will affect the course of events and the outcomes.
Although the used circular business model canvas proved to be suitable for our educational purposes, it is certainly not the only relevant circular business modeling tool, especially since the circular economy is currently under rapid development. It would be of value to experiment with other tools, for example, the value mapping tool by Nancy Bocken et al. (2015). The same applies to the used taxonomy in the reflection assignment. Since CBMI processes touch upon affective behavior, skills and attitudes, the traditional taxonomy of Bloom (Krathwohl, 2002) would not have been sufficient, whilst Dee Fink's taxonomy does include these “softer” sides of learning. Nevertheless, studying the effect of gameplay using other taxonomies that incorporate cognitive, affective and psychomotor learning is still warranted to enhance our understanding of teaching CBMI.
Quantitative text analysis methods are still most suitable for English texts. Therefore, we recommend conducting experiments with games in English until the next generation of text mining tools has been developed to an equal level. Another caveat is that the measurement groups were not large enough to make statements about statistical significance. Repetition of the experiment with more groups of students is needed to provide a conclusive answer to this.
This study provides opportunities to develop new ideas for both education and research. The education package could be tailored in detail. It can also be combined with other games and simulations of industrial symbiosis networks and circular business modeling contexts, which may provide students with other perspectives on the circular transition, such as material criticality or financial, environmental and social impact. In line with suggestions made by Tseng et al. (2021), the game could be used to create a digital twin, e.g., an agent-based model, to study the different decision-making processes and other activities during the development and implementation of circular business models. Doing so may eventually provide more insights into the role of business model innovation in the transition toward the circular economy.
Concluding Remarks
Our study findings provide detailed information per business model element and learning category. It furthers understanding of game-based learning for circular business model innovation while improving serious games and accompanying educational packages. Therefore, this section presents this study's conclusions and contributions from three perspectives: the game, the education package, and the experiment.
This study demonstrates how Re-Organise evolved from a product of practice-oriented research—a business game—into an educational tool for game-based learning—an educational game. During this process, the game objectives changed from exploring solutions for closing loops into teaching about circular business model innovation. It was necessary to enhance the gameplay experience by simplifying the game cards. Adding the assets cards to the game was necessary to ensure that the students understood that innovation also involves making investments. The game is versatile, and it would be interesting to extend or redevelop it for other contexts and sectors. However, additional materials need to be developed to ensure an optimal learning experience if this is done. In general, this study thus shows how originally explorative business games for practitioners can be redesigned for higher education purposes by aligning the game narrative with course learning objectives and assignments.
The Re-Organise game—education package teaches the iterative process of circular business model innovation. The game is a realistic simulation, as it was based on real-world case evidence. It encourages the application of technologies to fulfill needs, which helps in a better understanding of the role of technologies in the real world. Important dynamics and path dependencies that affect circular business model innovation are revealed during gameplay; the players' decisions can undercut planned solutions in earlier game stages. The gameplay also increases the student's reflective competencies on the learning experience. It gives insights into the importance of social interactions and learning from oneself and others. Furthermore, it motivates students to develop interests and feelings about circular business model innovation, entrepreneurship and the concept of industrial symbiosis. Based on these findings, we argue that game-based learning is of important value to help students understand, synthesize, and iteratively improve circular business models. We recommend including this education package and other game-based learning tools in curricula that teach circular business model innovation and industrial symbiosis.
In the context of game-based learning for circular business model innovation, this article is the first to combine mixed quantitative and qualitative analysis methods on data from pre- and post-test experimental and control groups. Echoing Dee Fink (2013, p. 34), learning is a process of change: “No change, no learning”. The methods used offer insights into this change process on a highly detailed level: per business model element and Dee Fink's learning category. Text mining is an exceptionally powerful method to study the changes in large text corpora of many sources. We applied it to CBMCs and reflections of large student groups in this study. Since emotional change is a strong indicator of learning, this study's sentiment analysis on Dee Fink's learning categories demonstrates the broadness of the game-based learning experience. Text mining methods thus provide opportunities to gain insights in learning about circular business model innovation and in the student's perception of their learning experiences. The current developments in text mining uncover a world of new insights for game-based learning and sustainability in higher education. This study advances a deeper understanding of game-based learning by utilizing these methods. Moreover, it provides detailed directions for shaping serious games and accompanying educational packages to meet educational and professional requirements.
Data Availability Statement
The datasets presented in this study can be found in online repositories. The names of the repository/repositories and accession number(s) can be found below: https://research.hva.nl/en/datasets/dataset-gameplay-re-organise-game.
Ethics Statement
The studies involving human participants were reviewed and approved by Maurice Pelt, Data Steward of the Faculty of Technology, Amsterdam University of Applied Sciences. Written informed consent for participation was not required for this study in accordance with the national legislation and the institutional requirements.
Author Contributions
KL contributed to the conception of the work, data collection, analysis and interpretation, and drafting and writing the article. GK contributed to data collection and interpretation, critical revisions of the article, and final approval. IO contributed to data interpretation, critical revisions of the article, and final approval. PH contributed to data interpretation, critical revisions, and final approval. All authors contributed to the article and approved the submitted version.
Funding
The case studies in the project Re-Organise were funded by the RAAK-mkb Subsidy of Stichting Innovatie Alliantie (SIA, Project Number 2015-03-03M), a Dutch National Subsidy Organization for Applied Research. This open-access publication was financed by the Netherlands Organization for Scientific Research (NWO, Project Number 023.009.037) and the Amsterdam University of Applied Sciences (AUAS).
Conflict of Interest
The authors declare that the research was conducted in the absence of any commercial or financial relationships that could be construed as a potential conflict of interest.
Publisher's Note
All claims expressed in this article are solely those of the authors and do not necessarily represent those of their affiliated organizations, or those of the publisher, the editors and the reviewers. Any product that may be evaluated in this article, or claim that may be made by its manufacturer, is not guaranteed or endorsed by the publisher.
Acknowledgments
Many people contributed to the realization of this study. The authors would like to thank the editor and reviewers for their highly valuable comments on the previous versions of this article. In addition, we thank Txell Blanco, Simone Gager, Ben Kubbinga, Janne van den Akker, Maarten Mulder, Yannick Schrik, Marco van Hees, and Jan Willem Verloop for their collaboration and discussions during the iterative development of the first version of the game. We also thank Britt Broekhaus, Tessa Baart, and Quirien Reytenbagh, who supported developing the education package and preparing data collection. Thanks to Tristan de Wildt, Özge Okur, Molood Aleebrahimdehkordi, and Hanxin Zhao for helping us during gameplay preparations. We sincerely thank Kiki Boomgaard for supporting data collection in the GIEEE program. We thank the students of the AUAS minor Data Science, and lecturers Lyske Gais de Bildt, Charaf Batou and Pieter Bons in particular, for their contribution and support on the process of text mining. Finally, we cordially thank all the contributors in our case studies and game participants.
Supplementary Material
The Supplementary Material for this article can be found online at: https://www.frontiersin.org/articles/10.3389/frsus.2022.809700/full#supplementary-material
References
Arnab, S., Lim, T., Carvalho, M. B., Bellotti, F., De Freitas, S., Louchart, S., et al. (2015). Mapping learning and game mechanics for serious games analysis. Br. J. Educ. Technol. 46, 391–411. doi: 10.1111/bjet.12113
Baldassarre, B., Schepers, M., Bocken, N., Cuppen, E., Korevaar, G., and Calabretta, G. (2019). Industrial Symbiosis: towards a design process for eco-industrial clusters by integrating Circular Economy and Industrial Ecology perspectives. J. Clean. Prod. 216, 446–460. doi: 10.1016/j.jclepro.2019.01.091
Bevilacqua, M., Ciarapica, F. E., Mazzuto, G., and Paciarotti, C. (2015). ‘cook andteach': learning by playing. J. Clean. Prod. 106, 259–271. doi: 10.1016/j.jclepro.2014.11.085
Biggs, J., and Tang, C. (2010). “Applying constructive alignment to outcomes-based teaching and learning,” in Training Material for “Quality Teaching for Learning in Higher Education” Workshop for Master Trainers (Kuala Lumpur: Ministry of Higher Education), 2325.
Bloom, B. S. (1956). Taxonomy of Educational Objectives. Vol. 1: Cognitive Domain. New York, NY: McKay.
Bocken, N., de Pauw, I., Bakker, C., and van der Grinten, B. (2016). Product design and business model strategies for a circular economy. J. Indust. Prod. Eng. 33, 308–320. doi: 10.1080/21681015.2016.1172124
Bocken, N., Rana, P., and Short, S. W. (2015). Value mapping for sustainable business thinking. J. Indust. Prod. Eng. 32, 67–81. doi: 10.1080/21681015.2014.1000399
Boyle, E. A., Hainey, T., Connolly, T. M., Gray, G., Earp, J., Ott, M., et al. (2016). An update to the systematic literature review of empirical evidence of the impacts and outcomes of computer games and serious games. Comput. Educ. 94, 178–92. doi: 10.1016/j.compedu.2015.11.003
Brabeck, M., and Jeffrey, J. (2014). Practice for Knowledge Acquisition (Not Drill and Kill). APA, p. 1–14. Available online at: https://www.apa.org/education/k12/practice-acquisition (accessed may 1, 2022).
Braungart, M., McDonough, W., and Bollinger, A. (2007). Cradle-to-cradle design: creating healthy emissions - a strategy for eco-effective product and system design. J. Clean. Prod. 15, 1337–1348. doi: 10.1016/j.jclepro.2006.08.003
Breuer, H., Fichter, K., Lüdeke-Freund, F., and Tiemann, I. (2018). Sustainability-oriented business model development: principles, criteria and tools. Int. J. Entrepren. Vent. 10, 256–286. doi: 10.1504/IJEV.2018.092715
Caponetto, I., Earp, J., and Ott, M. (2014). “Gamification and education: a literature review,” in European Conference on Games Based Learning, Vol. 1 (Academic Conferences International Limited), 50.
Centobelli, P., Cerchione, R., Chiaroni, D., Vecchio, P., and Del, Urbinati, A. (2020). Designing business models in circular economy: a systematic literature review and research agenda. Bus. Strat. Environ. 29, 1734–1749. doi: 10.1002/bse.2466
Chappin, E. J. L., Bijvoet, X., and Oei, A. (2017). Teaching sustainability to a broad audience through an entertainment game – the effect of Catan: oil springs. J. Clean. Prod. 156, 556–568. doi: 10.1016/j.jclepro.2017.04.069
Chesbrough, H. (2010). Business model innovation: opportunities and barriers. Long Range Plan. 43, 354–363. doi: 10.1016/j.lrp.2009.07.010
Clarke, J. L. (2020). “Serious games for the built environment: eco material trumps,” in Sustainable Ecological Engineering Design, eds L. Scott, M. Dastbaz, and C. Gorse (Cham: Springer), 425435. doi: 10.1007/978-3-030-44381-8_32
Connolly, T. M., Boyle, E. A., MacArthur, E., Hainey, T., and Boyle, J. M. (2012). A systematic literature review of empirical evidence on computer games and serious games. Comput. Educ. 59, 661–686. doi: 10.1016/j.compedu.2012.03.004
Cooper, W. F., and Harrow, A. J. (1973). A taxonomy of the psychomotor domain: a guide for developing behavioral objectives. Am. Educ. Res. J. 10, 325. doi: 10.2307/1161665
Davidovici-Nora, M. (2013). Innovation in business models in the video game industry: free-to-play or the gaming experience as a service. Comput. Games J. 2, 22–51. doi: 10.1007/BF03392349
De Angelis, R. (2016). A Conceptualisation of Circular Business Models and Explanation of Their Adoption: Evidence From Four In-Depth Case Studies Submitted. In University of Exeter.
Dee Fink, L. (2013). “A taxonomy of significant learning,” in Creating Significant Learning Experiences: An Integrated Approach to Designing College Courses (San Francisco, CA: Jossey Bass A Wiley Brand).
EMF IDEO (2016). Worksheet Business Model Canvas. In The Circular Design Guide. Ellen MacArthur Foundation. Available online at: https://www.circulardesignguide.com/post/circular-business-model-canvas (accessed May 1, 2022).
EMF McKinsey (2014). Towards the Circular Economy: Accelerating the scale-up across global supply chain. Report World Economic Forum. Available online at: http://www3.weforum.org/docs/WEF_ENV_TowardsCircularEconomy_Report_2014.pdf (accessed May 1, 2022).
Ferreira-Mello, R., Andr?, M., Pinheiro, A., Costa, E., and Romero, C. (2019). Text mining in education. WIREs Data Mining Knowl. Discov. 9, e1332. doi: 10.1002/widm.1332
Fraccascia, L., Sabato, A., and Yazan, D. M. (2021). An industrial symbiosis simulation game: evidence from the circular sustainable business development class. J. Ind. Ecol. 25, 1688–1706. doi: 10.1111/jiec.13183
Geissdoerfer, M., Bocken, N., and Hultink, E. J. (2016). Design thinking to enhance the sustainable business modelling process – a workshop based on a value mapping process. J. Clean. Prod. 135, 1218–1232. doi: 10.1016/j.jclepro.2016.07.020
Geissdoerfer, M., Morioka, S. N., de Carvalho, M. M., and Evans, S. (2018). Business models and supply chains for the circular economy. J. Clean. Prod. 190, 712–721. doi: 10.1016/j.jclepro.2018.04.159
Geissdoerfer, M., Savaget, P., Bocken, N., and Hultink, E. J. (2017b). The circular economy – a new sustainability paradigm? J. Clean. Prod. 143, 757–768. doi: 10.1016/j.jclepro.2016.12.048
Geissdoerfer, M., Savaget, P., and Evans, S. (2017a). The cambridge business model innovation process. Proc. Manufact. 8, 262–269. doi: 10.1016/j.promfg.2017.02.033
Geurts, J. L. A., Duke, R. D., and Vermeulen, P. A. M. (2007). Policy gaming for strategy and change. Long Range Plann. 40, 535–558. doi: 10.1016/j.lrp.2007.07.004
Ghisellini, P., Cialani, C., and Ulgiati, S. (2015). A review on circular economy: the expected transition to a balanced interplay of environmental and economic systems. J. Clean. Prod. 114, 11–32. doi: 10.1016/j.jclepro.2015.09.007
Graedel, T. E., and Allenby, B. R. (2010). Industrial Ecology and Sustainable Engineering. Pearson Education, Inc.
Guldmann, E. (2018). Circular Business Models: Innovation Journeys Towards a Circular Economy. Aalborg Universitetsforlag. Ph.d.-serien for Det Tekniske Fakultet for IT og Design, Aalborg Universitet.
Guldmann, E., and Huulgaard, R. D. (2020). Barriers to circular business model innovation: a multiple-case study. J. Clean. Prod. 243, 118160. doi: 10.1016/j.jclepro.2019.118160
Harvard Law School (2020). Does Using Technology in Negotiation Change Our Behavior? Available online at: https://www.pon.harvard.edu/daily/negotiation-skills-daily/technology-changing-us-way-negotiate-nb/ (accessed May 1, 2022).
Hearst, M. (2003). What Is Text Mining? Available online at: https://people.ischool.berkeley.edu/~hearst/text-mining.html (accessed September 1, 2021).
Herczeg, G., Akkerman, R., and Hauschild, M. Z. (2018). Supply chain collaboration in industrial symbiosis networks. J. Clean. Prod. 171, 1058–1067. doi: 10.1016/j.jclepro.2017.10.046
Hu, M., and Liu, B. (2004). “Mining and summarizing customer reviews,” in KDD-2004 - Proceedings of the Tenth ACM SIGKDD International Conference on Knowledge Discovery and Data Mining, 168–177.
Inchainge (2019). The Blue Connection - Circular Economy Business Game. Available online at: https://inchainge.com/business-games/tbc/ (accessed May 1, 2022).
Innovative Dutch (2021). Business Model Game. Available online at: https://www.innovativedutch.com/business-model-game/ (accessed May 1, 2022).
Katsaliaki, K., and Mustafee, N. (2012). “A survey of serious games on sustainable development,” in Proceedings of the 2012 Winter Simulation Conference (WSC), 113. doi: 10.1109/WSC.2012.6465182
Korhonen, J., Honkasalo, A., and Seppälä, J. (2018). Circular economy: the concept and its limitations. Ecol. Econ. 143, 37–46. doi: 10.1016/j.ecolecon.2017.06.041
Krathwohl, D. (1964). “Taxonomy of educational objectives: the classification of educational goals,” in Handbook II: Affective Domain, eds D. R. Krathwohl, B. S. Bloom, B. B. Masia, and D. McKay (New York, NY).
Krathwohl, D. R., Anderson, L. W., Airasian, P. W., Cruikshank, K. A., Mayer, R. E., Pintrich, P. R., et al. (2002). A revision of bloom's taxonomy of educational objectives. Theory into Pract. 41, 212–218. doi: 10.1207/s15430421tip4104_2
Kwartler, T. (2017). Text Mining in Practice With R. Chichester; West Sussex:Wiley & Sons Ltd; The Atrium; Southern Gate.
Lange, K. P. H. (2019b). Re-Organise - The Game: English - V2.1 — HvA Research Database. Available online at: https://research.hva.nl/en/publications/re-organise-the-game-english-v21 (accessed May 1, 2022).
Lange, K. P. H., Korevaar, G., Oskam, I. F., and Herder, P. M. (2017). Developing and understanding design interventions in relation to industrial symbiosis dynamics. Sustainability. 9, 826. doi: 10.3390/su9050826
Lange, K. P. H. (2019a). Dataset Gameplay Re-Organise Game — HvA Research Database. Amsterdam: Amsterdam University of Applied Sciences.
Lombardi, D. R., and Laybourn, P. (2012). Redefining industrial symbiosis: crossing academic-practitioner boundaries. J. Indust. Ecol. 16, 28–37. doi: 10.1111/j.1530-9290.2011.00444.x
Manshoven, S., and Gillabel, J. (2021). Learning through play: a serious game as a tool to support circular economy education and business model innovation. Sustainability 13, 13277. doi: 10.3390/su132313277
Mooij, M., Terra, J., Welvaars, M., and Biçer, L. (2020). Data Analysis - Re-Organise - Minor Data Science (1). Amsterdam University of Applied Sciences.
Mulder, M., Akker, J., van den Lange, K. P. H., Hees, M., van Verloop, J. W., Schrik, Y., et al. (2018). Re-organise - Sluiten van stedelijke kringlopen door decentrale verwerking van organisch bedrijfsafval. Available online at: http://www.hva.nl/kc-techniek/gedeelde-content/projecten/projecten-algemeen/re-organise.html (accessed May 1, 2022).
Ness D. (2008). Sustainable urban infrastructure in China: towards a Factor 10 improvement in resource productivity through integrated infrastructure systems. Int. J. Sustain. Dev. World Ecol. 15, 288301. doi: 10.3843/SusDev.15.4:2a.
Osterwalder, A., and Pigneur, Y. (2010). Business Model Generation: A Handbook for Visionaries, Game Changers, and Challengers, Vol. 1. John Wiley & Sons.
PBL Netherlands Environmental Assessment Agency (2019). Outline of the Circular Economy. In PBL publication number (Vol. 3633). Available online at: https://circulareconomy.europa.eu/platform/sites/default/files/pbl-2019-outline-of-the-circular-economy-3633.pdf (accessed May 1, 2022).
RDocumentation (2019). Polarity Function - qdap (2.4.3). RDocumentation. Available online at: https://www.rdocumentation.org/packages/qdap/versions/2.4.3/topics/polarity (accessed May 1, 2022).
Richardson, J.. (2008). The business model: an integrative framework for strategy execution. Strat. Change 17, 133–144. doi: 10.1002/jsc.821
Roome, N., and Louche, C. (2016). Journeying toward business models for sustainability: a conceptual model found inside the black box of organisational transformation. Org. Environ. 29, 11–35. doi: 10.1177/1086026615595084
Stahel, W. R. (2008). “The performance economy: business models for the functional service economy,” in Handbook of Performability Engineering, ed K. B. Misra (London: Springer). doi: 10.1007/978-1-84800-131-2_10
Susi, T., and Johannesson, M. (2007). Serious Games-An Overview. Institutionen för kommunikation och information. Available online at: https://www.diva-portal.org/smash/get/diva2:2416/fulltext01.pdf (accessed May 1, 2022).
Tobias, S., Fletcher, J., and Wind, A. (2014). “Game-based learning,” in Handbook of Research on Educational Communications and Technology (Springer), 485–503. Available online at: https://www.academia.edu/23408565/Game_Based_Learning (accessed May 1, 2022).
Tseng, M. L., Tran, T. P. T., Ha, H. M., Bui, T. D., and Lim, M. K. (2021). Sustainable industrial and operation engineering trends and challenges Toward Industry 4.0: a data driven analysis. J Indust Prod Eng. 38, 581–598. doi: 10.1080/21681015.2021.1950227
Tuinen van West (2019). Tuinen van West - Collectief composteren. Available online at: https://tuinenvanwest.info/ (accessed May 1, 2022).
Tyng, C. M., Amin, H. U., Saad, M. N. M., and Malik, A. S. (2017). The influences of emotion on learning and memory. Front. Psychol. 8, 1454. doi: 10.3389/fpsyg.2017.01454
Vlachopoulos, D., and Makri, A. (2017). The effect of games and simulations on higher education: a systematic literature review. Int. J. Educ. Technol. High. Educ. 14:22. doi: 10.1186/s41239-017-0062-1
Whalen, K. (2017). “Risk and race: creation of a finance-focused circular economy serious game,” Product Lifetimes and The Environment 2017 - Conference Proceeding, 422–425.
Whalen, K., and Peck, D. (2014). In the loop – sustainable, circular product design and critical materials. Int. J. Autom. Technol. 8, 664–676. doi: 10.20965/ijat.2014.p0664
Whalen, K. A., Berlin, C., Ekberg, J., Barletta, I., and Hammersberg, P. (2018). ‘All they do is win': lessons learned from use of a serious game for Circular Economy education. Resour. Conserv. Recycl. 135, 335–345. doi: 10.1016/j.resconrec.2017.06.021
William, L., Rahim, Z. B. A., De Souza, R., Nugroho, E., and Fredericco, R. (2018). Extendable board game to facilitate learning in supply chain management. Adv. Sci. Technol. Eng. Syst. 3, 99–111. doi: 10.25046/aj030411
Wynn, D. C., and Eckert, C. M. (2017). Perspectives on iteration in design and development. Res. Eng. Design 28, 153–184. doi: 10.1007/s00163-016-0226-3
Zott, C., and Amit, R. (2010). Business model design: an activity system perspective. Long Range Planning, 43, 216–226. doi: 10.1016/j.lrp.2009.07.004
Keywords: circular economy (CE), business model innovation (BMI), serious games (SG), game-based learning (GBL), text mining (TM), industrial symbiosis (IS), learning taxonomies
Citation: Lange KPH, Korevaar G, Oskam IF and Herder PM (2022) Re-organise: Game-Based Learning of Circular Business Model Innovation. Front. Sustain. 3:809700. doi: 10.3389/frsus.2022.809700
Received: 05 November 2021; Accepted: 21 April 2022;
Published: 30 May 2022.
Edited by:
Anna Bogush, Coventry University, United KingdomReviewed by:
Ming Lang Tseng, Asia University, TaiwanUsama Awan, Lappeenranta University of Technology, Finland
Copyright © 2022 Lange, Korevaar, Oskam and Herder. This is an open-access article distributed under the terms of the Creative Commons Attribution License (CC BY). The use, distribution or reproduction in other forums is permitted, provided the original author(s) and the copyright owner(s) are credited and that the original publication in this journal is cited, in accordance with accepted academic practice. No use, distribution or reproduction is permitted which does not comply with these terms.
*Correspondence: Kasper P. H. Lange, ay5wLmgubGFuZ2VAaHZhLm5s