- Safer Chemical Analytics LLC, Columbia, MD, United States
As part of the ESG (Environmental, Social, and Governance) dynamics, chemical environmental sustainability, i.e., the impact from chemicals and how to measure it across hundreds of products, is a daunting, but necessary task. Although methods are available to measure the effects and impacts of a single chemical, most enterprises do not focus on a single chemical, let alone produce “pure” chemicals for sale. Nearly all chemicals in commerce are chemical products, i.e., mixtures, while assessment methods for mixtures are few and far between. What is needed is a metric that tracks the potential risk of an enterprise's total product inventory while monitoring its improvements as it greens in coming years. The Chemical Environmental Sustainability Index (ChemESI) metric measures both risk (as the product of exposure and hazard) and hazard across numerous chemicals as a single metric/KPI. The ChemESI's for chemicals, products and facilities are expressed such that they can be summed across facilities to wrap up into a single corporate ChemESI KPI for either hazard or risk. But what about growth—if growth occurs using greener chemical inventory, a company can both grow and improves its ChemESI KPI. Given the lead-time for developing more, true green alternatives to existing chemicals, intermediate substitution of less hazardous “analogs” may drive initial ChemESI reduction. To achieve a representative risk estimate, a primary data need is chemical characterization data for products. SDS's (Safety Data Sheets) unfortunately make poor substitutes for true constituent analyses. A definite need exists for better, more detailed chemical characterization data for both mixtures and individual chemicals, as the latter most often are not 100 % “pure.” However, these SDS's are available universally, across the globe, and provide GHS (UN Globally Harmonized System) classifications for single endpoint chemical hazard assessment. GHS classifications are near universal and here used to derive chemical hazard scores over multiple endpoints for each chemical. A ChemESI Risk metric can be derived by multiplying the hazard score by the exposure, here using inventory as a surrogate. The ChemESI Hazard metric is then derived as an inventory weighted hazard score.
Introduction
Environmental sustainability of chemical inventory is an often-overlooked aspect of ESG, where environmental impacts often reference greenhouse gases or decarbonization (Investopedia, 2021). Enterprise chemical sustainability gets short thrift in nearly all assessments systems, except perhaps TRACI (Tool for Reduction and Assessment of Chemicals and Other Environmental Impacts, e.g., Bare, 2002). Recently systems have been developed to assess sustainability in procurement of health products (UNDP, 2020; SHiPP, 2021). Given that most companies maintain an inventory of chemicals whether it be in large or small quantities, often in an astounding variety, environmental sustainability metrics are needed. Here we propose one ESG metric, the Chemical Environmental Sustainability Index or ChemESI for short. ChemESI aims to measure the inherent risk of chemical inventory across all locations within an enterprise, allowing for identification of locations with increased hazard and risk within the enterprise. Note that it produces both hazard and risk estimates, the latter incorporating the exposure to allow one to distinguish between a test tube and a tanker trailer of a chemical.
Environmental and health effects of chemicals within a value chain are managed through Product Stewardship (Hart, 2018). Product stewardship of location inventory often focuses on the inherent hazard of chemicals classified using the eighth or ninth edition of GHS (Globally Harmonized System) information within SDS's (Safety Data Sheets) (UN, 2021). GHS thus perpetuates the focus on hazard only. Several chemical regulations, including REACH (Registration, Evaluation, Authorization and Restriction of Chemicals, ECHA, 2017), have tried to address this via individual exposure limits based on acceptable risk. The definition of acceptable risk there is fuzzied once again by the use of ballpark uncertainty factors (USEPA, 1993; ECHA, 2017) as compared to data analytics-based factors. These so-called safety/uncertainty factors (better referred to as assessment factors), often of multiple orders of magnitude, are used to account for perceived uncertainties in regulatory risk assessments. Such factors have been shown to be overestimations in those cases where they have been calculated from data analytics (Escher et al., 2020) rather than taken off the proverbial back of an envelope. Health and environmental hazard data also have their own inherent uncertainties based on a comparison of tests for the same chemical (Pham et al., 2019; Kostal et al., 2020; Plugge et al., 2021), often again in the (multiple) order of magnitude range. In-depth, regulatory chemical-specific risk assessments are thus inherently uncertain and available for only a limited number of chemicals. They are also costly: regulatory, single chemical risk assessments without data acquisition often exceed $1,000,000 (Maertens and Plugge, 2018).
While GHS provides a good classification method for chemicals and their associated hazard, it does not account for exposure, especially from a corporate risk inventory/insurance point of view (Chemsec, 2021). Risk is what determines an enterprise's liability/insurance “exposure” from its chemical inventory, hazard is just one of the factors. Risk and exposure are used here as commonly used within the environmental health community, which is incongruent with definitions in other communities. In the insurance community; Risk = Uncertainty arising from the possible occurrence of given events or the actual property/insured (IRMI, 2022a). Similarly: Exposure = The state of being subject to loss because of some hazard or contingency, also used as a measure of the rating units or the premium base of a risk (IRMI, 2022b). The environmental health definition of Risk= hazard * exposure is thus not congruent with insurance usage. Similarly for the financial definition of risk (Investopedia, 2022).
Hazard assessment now nearly universally starts with the (ninth edition of the) GHS (UN, 2021). REACH (Registration, Evaluation, Authorization and Restriction of Chemicals) regulations (ECHA, 2017) offer a similar but not quite congruent system of classification. Not always acknowledged, GHS is also neither completely global nor harmonized. Although negotiated at the UN level, most countries provide their own (small) spin on, or lag in adoption of the latest edition. Nonetheless, these GHS hazard classes are more universal than any other system and chemical specific, single endpoint classifications are widely available commercially (Scivera, 2021; Toxnot, 2021; Verisk 3E, 2021). GHS assessments however still have drawbacks most of which were designed into the system:
• GHS classification is always based on the most sensitive, i.e., hazardous datapoint available, with, in practice, little consideration of data quality and variability; REACH (ECHA, 2017) being a partial exception
• GHS classification bins into rather broad categories, especially for acute toxicity, e.g., a datapoint of 49 vs. 51 can result in classification in different toxicity categories (Kostal et al., 2020)
• GHS only notes absence of data and does not penalize for missing toxicity data
• Classification use/interpolation of data from and/or incorporation of NAMs (New Approach Methodologies, USEPA, 2021) often lags
• GHS compliant SDS's (Safety Data Sheet) often lack a full chemical characterization, i.e., the ingredient percentages do not add up to (or exceed) 100%, resulting in a less detailed GHS classification of a product.
GHS-based SDS's were developed for the purpose of occupational hazard communication for transport of hazardous chemicals, which accounts for some of these limitations. Notwithstanding these weaknesses, GHS is accepted in nearly all countries around the world as “the” hazard assessment/classification system allowing the preparation of globally accepted SDS's with mostly universal classifications, as evidenced by its proliferation in EHS (Environmental Health and Safety) systems (e.g., Verisk 3E, 2021). GHS provides single endpoint scores which are not summable into one score for a chemical, let alone products, e.g., one cannot compare or weight highly acutely toxic via oral route with skin sensitization. ChemESI transforms the GHS classification scores into an all endpoints combined total Chemical Hazard Score (CHS) for each chemical. The hazard portion of the ChemESI is thus based on GHS classifications, while noting its drawbacks, i.e., generally resulting in an overestimation of hazard, although in a non-quantifiable manner.
Risk, as described above, is a more accurate measure of chemical sustainability: after all it accounts for the exposure in addition to the inherent hazard of chemicals. Risk can then be quantified, as always [in (environmental) toxicology/risk assessment], as the product of exposure and hazard: risk = exposure * hazard. GHS classifications act as surrogate hazard quantifiers via the CHS. Here exposure estimates for a facility are approximated as quantities held within a facility, i.e., using inventory as a surrogate for exposure. A similar approach was used to prioritize chemical risk for reproductive effects within the EU: quantity produced times REACH based DNEL (Derived No-Effect Level) estimates, prioritized chemicals for assessment (Risk Policy Analysts, 2019). ChemESI Risk is then calculated as the product of the two, i.e., risk = exposure * hazard summed over all chemicals or products. ChemESI Hazard is calculated as total risk divided by total exposure, i.e., a weighted Hazard Score across a facility, while maintaining individual chemical/product hazard and risk scores.
Exposure models exist for all kinds of media and exposure types. Often chemicals exert effects through a variety of routes, i.e., air, skin, oral, and for biota effects through environmental effects mostly in water. Persistence also plays a large role. Note that all of these routes require their own modeling “software” with specific data requirements and calculations to produce quantitative estimates with some certainty. Although feasible for a few chemicals, this process becomes unmanageable for a large variety of chemicals as well as products. High throughput modeling can overcome this singular exception but also adds high variability (Li et al., 2021). One can model single chemical concentrations reasonably well, but model outputs suffer from fairly high uncertainty intervals which, when modeled in detail for multichemical assessments, exceed 4 orders of magnitude (Li et al., 2021). Mixture interactions are rarely described and then generally only for pair-wise comparisons.
The ChemESI Risk model used here assesses impacts from multiple chemicals in multiple media. As mentioned above exposure modeling for such a scenario would be a nightmare. Other risk assessment methodologies have tried doing this: predict environmental concentrations and calculate risk (e.g., Arnot and Mackay, 2008; Arnot et al., 2012). These are not traditional exposure/risk assessments—they employ high-throughput exposure scenarios to derive risks. Such schemes employ numerous assumptions of toxicity and persistence to derive environmental concentrations coupled with risk estimates. Initially performed for environmental pollutants, risk was used a function of quantity, toxicity, bioaccumulation, and persistence in a model to look at a continuous distribution to prioritize chemicals for regulation instead of the rigid yes/no cutoff model. Exposure estimates include an estimated emission rate based on quantity produced. Here risk needed to be calibrated by actual (rather than estimated) toxicity values and calibrated by actual exposure measurements. Further modeling of environmental risk resulted in a more than 4 orders of magnitude 95% confidence interval, leaving the authors to conclude that quantity may need to be the regulatory driver at this time (Li et al., 2021). Correlating known emission rates with environmental monitoring data demonstrated that existing exposure models could be off by an order of magnitude even when calibrated with actual release factors (Spaniol et al., 2021). Review of risk assessment applications used simplified exposure models in a risk assessment of transformation products in an aquatic environment (Escher and Fenner, 2011). Risk assessment of all transformation products was found to be out of reach. Tiered approaches were recommended based off parent compounds' risk assessments using advanced simulation methods which are now (2022) becoming more available. Specific modes of action would need to be identified for such risk assessments to proceed.
Exposure modeling always starts off with total quantity followed by a mathematical approach using various surrogate/estimated parameters. Given the observed uncertainties in the final exposure estimate, it was acknowledged that in a regulatory approach, quantity may be the best approximation for effect concentration (Li et al., 2021). Regulation of chemicals will thus remain focused on the total quantity, until the modeling becomes more available for multiple chemicals and routes, with a narrower range of uncertainty. Hence, total quantity present can be used as a surrogate for exposure measures.
Some might feel apprehensive at the coarseness of the estimates used in risk estimation here. To put this into perspective: regulatory risk assessment methodology often imputes high levels of data uncertainty (e.g., uncertainty factors), as high as 1-4 orders of magnitude range (e.g., ECHA, 2017; Kostal et al., 2020). GHS ranking is definitively conservative in nature, both by its broad use of binning and more importantly the use of the lowest effect or result, often regardless of data quality and variability, although such data restrictions are sometimes incorporated into REACH based risk estimates and classifications (ECHA, 2017). Risk estimates such as DNEL/PNECs (Derived No-Effect Level/Predicted No-Effect Concentration) often incorporate assessment factors ranging between 100 and 10,000 (ECHA, 2017). Modeled exposure estimates (Li et al., 2021) can give uncertainties in excess of four orders of magnitude for a 95% confidence interval.
ChemESI uses discrete judgment (i.e., classification categories) and transforms them into semicontinuous functions. As our goal is to provide a simple metric allowing for broad chemical coverage, we do not need the same granularity as a regulatory (single-chemical) decision tool which indeed would require much more and detailed data. ChemESI as a metric using hazard derived from GHS classifications and exposure as inventory is indeed coarse but most likely with no worse uncertainty than existing exposure/risk models. ChemESI has also another, great advantage: both hazard and exposure datasets are easily obtainable and most likely are already available within an enterprise.
Most of the chemicals in commerce do not exists as 100% pure chemicals, but as products. Products are defined as mixtures of various chemicals, although the actual composition can be hard to define based on SDS's. As with most other assessment systems, GHS classifications for mixtures are hazard based and classified based on weighted contribution from individual chemicals. No consideration of effect interaction between chemicals, be it negative or positive, is currently incorporated in GHS, nor is the collection of endpoints within GHS comprehensive, e.g., the lack of Endocrine Disruptor considerations. It should be noted here that even most individual chemicals are not 100% pure but, depending on quality grade, can contain up to 5–10% “impurities/contaminants” for, e.g., technical grade chemicals. Risk assessment needs to account for these impurities, which is often problematic due to lack of chemical characterization as well as hazard/effect data. Another, more granular, endpoint-specific, hazard screening program, GreenScreen™, requires (confidential) disclosure of impurities above 100 ppm (Clean Production Action, 2021).
The major advantage of GHS has always been its simplicity—the ease of communication across languages and cultures. A GHS compliant SDS looks the same the world over and has a rather narrow range of possible classifications for the various endpoints (UN, 2021). Product Stewards within the chemical community are very familiar with SDS's and associated data. The ChemESI aims to further condense the amount of available information into a facility/enterprise wide KPI (Key Performance Indicator) metric, easily transmittable to the C-suite. As the concept of ChemESI is developed below, the influence of the robustness of the GHS based Hazard Score as well as the influence of inventory fluctuation, organic growth and introduction of new products on the ChemESI scores will be described.
Methods
Scores were assigned to each of the classification categories for a particular chemical based on classifications following the ninth edition of the GHS (UN, 2021). These scores were then agglomerated for each chemical into a chemical specific score, the actual Chemical Hazard Score, CHS. These scores or weighting factors were derived based on the perception of endpoints: chronic endpoints such as carcinogenicity, reproductive and mutagenic effects are generally given more weight than acute toxicity (e.g., Swanson et al., 1997). These weighting factors are somewhat arbitrary, and users may want to assign their own weighting factors. If done consistently, the rank order of chemical hazards would not be affected, just the magnitude of the hazard score.1 Table 1 describes all the GHS categories for environmental health classifications and their corresponding score. The maximum hazard score for a chemical would be capped at 100 but is unlikely to be achieved by many chemicals. Benzene, for instance, would be scored as a carcinogen but does not have a high acute toxicity, which results, as described in Table 2, in a weighted derivation of the CHS for benzene of 78, based on a publicly available SDS (Airgas, 2020). For very toxic compounds, e.g., chlorinated dioxins such as TCDD, CHS would be capped at 100 but such compounds are unlikely to be present at high concentrations, i.e., more than 0.1 or 1%.
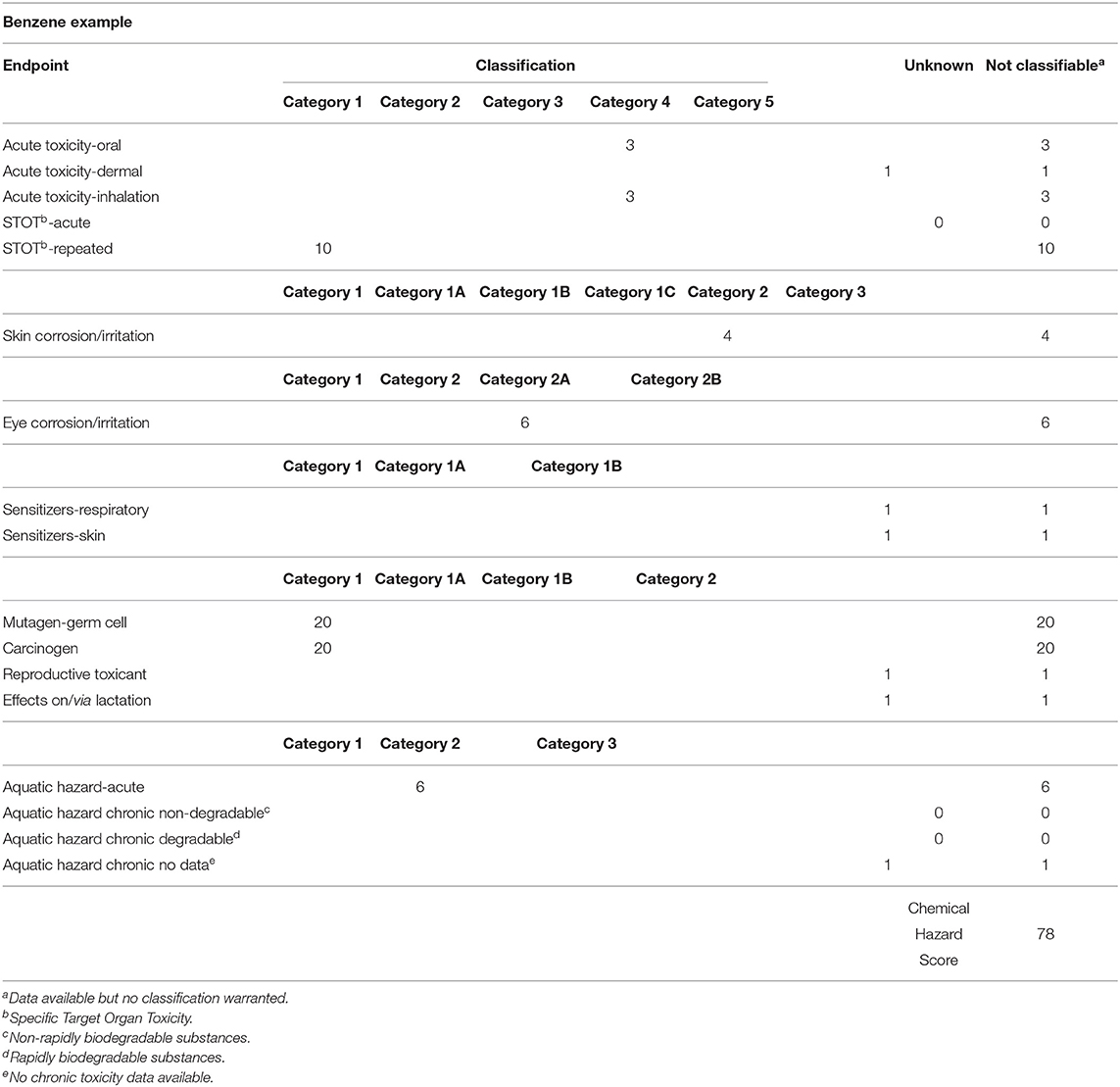
Table 2. Chemical Hazard Score for benzene based on sample SDS (Airgas, 2020) and Table 1.
The lowest score would be 1, e.g., water which is also the default score in the presence of all negative data, i.e., no effects found with supporting data. Missing data are treated differently; unlike in the GHS based SDS, missing data automatically contribute an additional score of 1 for each endpoint with missing data. Most chemicals for instance are missing actual genotoxicity or carcinogenicity information. A score of 1 would thus be hard to achieve without full effect characterization, which may be obtained in future using in silico or in vitro data aka NAMs (New Approach Methodologies, USEPA, 2021). Missing and/or incomplete data are a continuous problem within GHS classifications and especially those reported on SDS's (ECHA, 2019). Classifications may thus need to be checked with “complete” classification databases such as provided commercially (see below).
As shown in Tables 1, 2, individual-endpoint GHS classifications are easily converted into combined Chemical Hazard Scores for each individual chemical. Such conversions are even easier when using some type of online database, e.g., the publicly available CAt databases (CAt, 2021) or commercial equivalents (e.g., Scivera, 2021; Toxnot, 2021; Verisk 3E, 2021). As always classifications are supposed to be “universal,” but small differences based on the regulatory approaches followed, e.g., GHS vs. REACH/CLP may result in different classifications and hence scores. Following the GHS approach, the lowest classification, i.e., highest Hazard Score would be the one used here.
Note the difference between Chemical Hazard Scores (CHS) and GHS: GHS provides classifications for 17 different endpoints, all of which can be blank or have data, with no way of “summing” these effects for each individual chemical. Although theoretically it is possible to calculate weighted GHS scores for each facility, this would still result in 17 parameters that are not comparable. Chemical Hazard Scores on the other hand allow for unified expression of hazard in a single number which is comparable between chemicals. In addition, Chemical Hazard Scores also account to a limited extent for missing data—rare would be the chemical where 3–5 parameters were not identified as no data. Even a major industrial chemical such as benzene (Table 2) only has data for 8 out of 15 classification categories. One thus expects a “minimum” CHS to be in the 4–6 range, except for water which is assigned a hazard score of 1. These minimum scores could drop in the future as more economical and ethical NAMs for, e.g., carcinogenic effects, become available: only then can we, with data demonstrating the absence of effects, truly assign an overall CHS of 1 to a given chemical.
ChemESI Risk and Hazard calculations' workflow is shown in Figure 1. An inventory database provides an inventory of products for which SDS's are obtained. Based on the SDS's and their composition information both a list of products' compositions and an inventory of individual chemicals are derived. The SDS (or external data) provides the GHS classification information for each individual endpoint for each chemical. Using the scoring system from Table 1, a Chemical Hazard Score is derived for each chemical, which can also inform the product hazard score (based on product composition). Using inventory quantities, Risk Scores can then be derived for each chemical and product which when summed result in a ChemESI Risk Score. Using the total weight (kkg) of inventory data, a ChemESI Hazard Score can be derived (risk/exposure = hazard).
Note that Chemical Hazard Scores are for one individual chemical which can then be converted into Chemical Hazard product scores based on composition weighting factors. Conversely one can split each product up into individual chemicals and calculate the total amount of chemical X within an enterprise. There are advantages to both approaches; the product version lets one assess whether products have exceeded a hazard cutoff beyond which they are no longer “acceptable” in this enterprises' value chain. The splitting approach identifies how much a particular chemical is present across all products and, coupled with quantity data, how much these chemicals contribute to the overall risk level within an enterprise. The facility-wide ChemESI Hazard score then provides an indication of how “green” the overall enterprise is. As shown above, a facility-wide ChemESI Risk score identifies the total risk inherent to a facility/enterprise. Thus, ChemESI informs enterprise sustainability through both hazard and risk estimates.
The methodology as described here has the advantage of being relatively easy to implement, at the expense of losing detailed information. Drill-down into the data by experts is a definite possibility, but the ChemESI is meant as a KPI, a management (and investor) metric. Uncertainties vary: product quantities can be rather precise, but chemical characterization data for most products are limited and/or plain sloppy, e.g., the SDS component characterization data exceed 110%. The methodology generally trends conservative, i.e., will produce a higher risk estimate due to GHS rules and the scoring of so-called data gaps. The latter can be diminished by the use of less expensive, more ethical non-animal NAMs (New Approach Methodologies) (USEPA, 2021) to fill in some of the data gaps. Unfortunately, GHS assessment of hazardous chemicals at this time only considers a circumscribed number of endpoints; consideration of, e.g., Endocrine Disruptors is still lacking.
Exposure is defined here as the amount of chemical or product in inventory with chemical characterization defined initially by the SDS, hopefully improved by further (confidential) input data from within the supply chain. Ideally such characterization should occur at 100 ppm for each constituent but given the current data quality, that will not be achieved rapidly. A resolution of 1% would seem to be in reach. Exposure estimates are used expressed here in kkg (metric ton) of inventory. For cross value chain comparisons, we recommend that an inventory unit of kkg (metric ton) be adopted, in order to maintain consistent ChemESI Risk Scores. No units need to be assigned or considered as part of the ChemESI Hazard score.
Results
The scoring system in ChemESI was applied to a fictional facility/enterprise that uses and stores a limited array of three products with known composition of 7 constituent chemicals at various concentrations/percentages (Table 3). Both ChemESI Risk and Hazard scores were calculated for this enterprise. Results are reported without the use of decimals since the original input data do not employ decimals, i.e., a consistent number of significant digits. Sample Chemical Hazard Scores were ascribed to seven constituent chemicals. Here Chemical Hazard Scores were calculated for each mixture/product with low composition granularity (as is common in SDS's), which unfortunately ignores or minimizes the effect of minor, possibly more toxic constituents. Chemical Risk scores were then derived as the product of exposure (i.e., quantity in inventory) and Chemical Hazard Scores. Note that the product B with the highest product Hazard Score is the least risky and vice versa for product A, quantity and hence exposure matters. Summing of the individual product Risk Scores then produces an enterprise ChemESI Risk Score of 2114 which divided by the total inventory results in an inventory weighted enterprise ChemESI Hazard Score of 14.
In Table 4 we use the chemical composition data from Table 3 to derive a facility wide inventory of chemicals 1–7. A chemical-specific risk was then derived using individual chemical inventory estimates (across all products) and the same individual Chemical Hazard Scores as in Table 3. Note that here the highest risk contribution comes from chemical 3 which has an average Chemical Hazard Score. Not surprisingly, the facility ChemESI Hazard and Risk scores are identical between Tables 3, 4—just two different approaches, chemical or product based. For both of these approaches one can make a policy decision to weed out all chemicals/products with a Hazard Score of above, e.g., 50 or focus on the most “risky” chemicals/products. Obviously, a hybrid approach for chemicals/products, e.g., Chemical Hazard Scores over 50 up for elimination followed by a focus on the most risky chemical/product, would work even better. A restricted chemicals list should already be in use within an enterprise, thereby peremptorily eliminating the chemicals with very high hazard scores (e.g., ECHA, 2021).
In the following tables, we focused our assessment on individual chemicals. Given the proliferation of products containing multiple ingredients, an ingredient, i.e., individual chemical-based approach to greening inventory would appear to be more logical as replacement of a single ingredient should result in a greener ingredient and hence multiple greener products. The following analyses thus focus on individual chemical-based ChemESI calculations.
Introduction of a new chemical 8 at the enterprise resulted in a ChemESI Hazard Score of 18 (and ChemESI Risk Score of 3394) as shown in Table 5. Although Product Stewardship should have been involved in new product development throughout the entire process (Hart, 2018), there appears to have been a disconnect. Marketing/R&D introduced a single chemical 8 with a high hazard score of 32 and a 25+% increase in overall Product Quantity, resulting in an undesirable increase in ChemESI Risk by more than 50% to 3,394 and a corresponding increase in ChemESI Hazard to 18 (from 14). This metric was flagged in a C-suite meeting resulting in a directive to green the new product, while maintaining functionality (Principle 4 of Green Chemistry in Anastas and Wagner, 1998). The product was revised in two stages: a temporary direct drop-in replacement with chemical X and a final replacement of chemical 8 with chemical 9 as shown in Table 6. The result is a 25+% increase in product sales while dropping the ChemESI Hazard Score from 14 to 13, with only a 15% increase in ChemESI Risk Score. Note that we have a decrease in ChemESI Hazard Score with a 25% growth in inventory.
Here, two pathways appear for further reduction of ChemESI Risk and Hazard. One approach would be to replace chemicals with a hazard score of 50 and above, here chemicals 6 and 7. The other approach would be to reduce and/or substitute for chemical with a high risk, here chemicals 3 and 6. Since chemical 6 ends up in both categories, it appears to be a good place to start. Introduction of greener chemical substitutes, e.g., replacing chemical 6 with a less hazardous chemical would result in further reduction of both the ChemESI Hazard and Risk, while simultaneously providing greener products. Continuous improvement should obviously be a part of chemical manufacturing/product formulation going forward—this will result in a continual drop in ChemESI Hazard (and often Risk) as greener products are slated to be developed (Golden et al., 2021). The lag from R&D to commercialization of new greener alternative chemicals however approaches a decade, taking into account all processes including market penetration. Intermediate substitution with slightly greener alternatives may thus be important while working toward the ultimate green substitute.
As described here most chemical enterprises use multiple chemicals in a variety of recipes to produce their formulated products aka mixtures. In addition, depending on the (technical) grade used for formulation, more or less hazardous, often ill described, impurities may enter the supply chain. These are often not accounted for due to the lack of detailed composition information on the SDS and/or lack of hazard data. This is an information gap that has many implications and, especially for larger quantities of chemicals (missing information data for 1% of a 100 kkg chemical results in 1 kkg of undefined chemicals), could significantly affect hazard/risk assessment. As mentioned above other, more granular systems require characterization down to 100 ppm (Clean Production Action, 2021). Similarly, one assumes that all chemicals listed on an SDS have a unique identifier as well as at least some hazard information. This assumption is often refuted: chemicals end up being described as complex mixtures without a clear component characterization (think hydrocarbon fractions), and hazard data and information are often lacking, resulting in a non-uniform assignment of GHS classification. These data gaps need to be addressed through expert or automated evaluation via NAMs which adds complexity. Often, however, GHS classifications for these “exceptions” can be found on SDS's, but with varying classifications between manufacturers.
The ideal ChemESI would of course be 1. It is unlikely that any enterprise will get an ChemESI of 1 in the foreseeable future, primarily as the result of a lack of chemical characterization and full hazard data. As mentioned above, even for a commercially important chemical such as benzene only 8 out of 15 classification categories are available (Airgas, 2020). Another incentive here lies in full characterization of effects from each chemical, using for instance New Approach Methodologies, so-called NAMs (USEPA, 2021), without which a ChemESI hazard score of 1 is not attainable.
The ChemESI Hazard/Risk within the supply chain on a facility basis, can be combined into a division/enterprise level ChemESI. Even though Product Stewardship should already be limiting very hazardous chemicals from entering a supply chain, the ChemESI process will also identify remaining very hazardous chemicals with a Hazard Score beyond “acceptable.” Acceptable of course has to be defined on an enterprise-wide basis and may be accompanied by an exposure limit, e.g., no more than X kkg of Y can be within the facility at all times. Such exceptions may prove especially useful when technical grade (i.e., impure) chemicals are in the supply chain; such chemicals often contain more hazardous, contaminant constituents.
Exposure can also vary depending on the amount of inventory, e.g., running up inventory in anticipation of supply chain disruptions or taking advantage of discounted pricing on chemicals in bulk. Such inventory changes will most likely increase the ChemESI Risk. Risk minimization, i.e., fast inventory turnover will limit the risk incurred during daily operations, and in optimized cases even reduce ChemESI Risk while maintaining ChemESI Hazard. One should still perform an annual product inventory quantity survey to determine the total amount of “risk” incurred within the enterprise from an ESG point of view, although a running average would provide a better immediate KPI.
The ChemESI KPI indicates a general level of chemical environmental sustainability, i.e., the progress made in providing greener alternatives to society within the existing supply chain. Such sustainability indices become increasingly important as greenness become a major economic driver within (the chemical) industry. Much progress has been made—a lot of effort (Zimmerman et al., 2020) remains to be expended to truly bring Green Chemistry to the forefront as evidenced by the eventual possibility of an ChemESI near 1. In the meantime, growing industrial output with greener chemicals will slowly reduce the ChemESI KPI, while allowing for substantial growth.
Discussion
ChemESI Risk and Hazard are important KPIs especially for the chemical industry including formulators. These KPIs use exposure and hazard information readily available from multiple sources: in-house, or from free and commercially available databases. Inventory data here is used as a surrogate for exposure. The Chemical Hazard Score system described here in Table 1 is based on the widely accepted and adopted GHS classification approach—one can always dispute the weighting and prioritization of certain endpoints, but such changes would only have a slight impact on the overall ChemESI. Better to adopt a universal metric than to customize each enterprise.
Given the inherent uncertainties and biases built into the GHS classifications [e.g., a direct result of the derivation methodology for hazard classes using lowest effect concentration (ECHA, 2017; UN, 2021)], the ChemESI will tend toward overestimating hazard and hence risk. One of the inherent drawbacks in the GHS is that lack of data simply results in no classification—addressed here by adding additional scores for each hazard endpoint for which no data are available—one would hope that, especially for the higher volume chemicals, such full information would become available, most likely based on NAMs. Another major drawback is the GHS's dependency on the lowest available hazard information, i.e., the most toxic number is always used with little consideration of data quality and variability. Given that where there are repeat data, values often range across more than an order of magnitude (e.g., Plugge et al., 2021), this also adds a severe bias toward a more hazardous classification, especially in the absence of data quality considerations.
The lack of information data for risk assessment from GHS classifications and in SDS's is to some extent not surprising. SDS's were developed as hazard communication tools in occupational health and hazardous material transport. The lack of granularity, i.e., very few components characterized at concentrations below 1%, however is not inherent to the design of SDS's and probably remains the least reliable aspect of an SDS.
Some might question the coarseness of the approach. As addressed above the simplifying assumptions here allow for an uncertainty in the same order(s) of magnitude as the original data. Hazard estimates can have uncertainty/variability well in excess of an order(s) of magnitude. Similarly, modeled exposure often has orders of magnitude of uncertainty/variability. Use of the GHS data does allow for a simpler format, inherently familiar to the Product Steward, thereby enhancing the understanding and hence communication of the ChemESI to the C-suite and investors. Single number KPI's are always attractive while simultaneously allowing drill down into the data to assess which chemicals are contributing most to the overall risk and hazard. Often the chemicals with medium hazard are the biggest drivers of ChemESI risk parameters, simply because they often account for the largest tonnage. The ChemESI data informs both hazard and risk metrics that can be used to address the overall hazard and risk inherent to the inventory. Growth will decrease the ChemESI Hazard Score where growth is derived from greener products. If the Chemical Hazard Scores are low enough even ChemESI Risk Score would drop, coupled with substantial growth in greener products. Supply chain effects on retention/storage of both raw and finished products will most likely increase the ChemESI Risk Score, but have a lesser effect on the ChemESI Hazard Score. Dilution of more hazardous chemicals would decrease the ChemESI Hazard score but not the Chem ESI Risk score. Incorporation of LCA (Life Cycle Analysis) components and additional non-GHS endpoints could be the next logical set of enhancements to the ChemESI, although obtaining (semi-)curated data for all chemicals may prove problematic.
ESG (Environmental, Social, and Governance) discussions often lack (quantitative) data on the environmental impact of the chemicals within an enterprise, beyond greenhouse gases and decarbonization (Investopedia, 2021). The ChemESI described here is a quantitative risk and hazard metric combining chemical exposure and environmental health hazard within the supply chain. It is based on a scoring of readily available GHS hazard/classification data, coupled with proprietary inventory data. The ChemESI will provide a facility/enterprise based KPI metric of risk and hazard, easily communicable to the C-suite, investors, and others within the ESG sphere.
Although achievement of an ChemESI Hazard of 1 is theoretically achievable, it will be most likely a decade before green chemistry-based alternatives will be able to achieve such scores, although penetration of green chemistry derived products is rapidly increasing (Golden et al., 2021). An ChemESI Hazard of 20 or below should be achievable now, with of course continuous improvement expected. Organic growth will not affect a ChemESI per se: most likely a combination of a reduction in ChemESI Hazard Score and increased growth will occur through continued introduction of greener products, especially with the phasing out of less greener products. Adoption of ChemESI will go a long way toward quantifying the environmental sustainability metric in ESG.
Data Availability Statement
The original contributions presented in the study are included in the article, further inquiries can be directed to the corresponding author.
Author Contributions
The author confirms being the sole contributor of this work and has approved it for publication.
Conflict of Interest
HP is employed by Safer Chemical Analytics LLC.
Publisher's Note
All claims expressed in this article are solely those of the authors and do not necessarily represent those of their affiliated organizations, or those of the publisher, the editors and the reviewers. Any product that may be evaluated in this article, or claim that may be made by its manufacturer, is not guaranteed or endorsed by the publisher.
Footnotes
1. ^For Benzene for instance, assigning maximum weighting factors of 10 for each endpoint would result in a hazard score of 58 rather than 78 with a maximum score still capped at 100. The maximum score of 10 would only effect chronic effects such as carcinogenicity and mutagenicity, for which data are only available for select chemicals.
References
Airgas (2020). Safety Data Sheet – Benzene. Available online at: https://www.airgas.com/msds/001062.pdf (accessed December 6, 2021).
Anastas, P., and Wagner, J. C. (1998). Green Chemistry: Theory and Practice. Oxford: Oxford University Press.
Arnot, J. A., Brown, T. N., Wania, F., Breivik, K., and McLachlan, M. S. (2012). Prioritizing chemicals and data requirements for screening-level exposure and risk assessment. Environ. Health Perspect. 120, 1565–1570. doi: 10.1289/ehp.1205355
Arnot, J. A., and Mackay, D. (2008). Policies for chemical hazard and risk priority setting: can persistence, bioaccumulation, toxicity and quantity information be combined? Environ. Sci. Technol. 42, 4648–4654. doi: 10.1021/es800106g
Bare, J. C.. (2002). TRACI: The tool for the reduction and assessment of chemical and other environmental impacts. J. Indus. Ecol. 6, 49–78. doi: 10.1162/108819802766269539
CAt (2021). Available online at: https://www.chemicalawarenesstoolkit.com/home (accessed December 8, 2021).
Chemsec (2021). Hazard vs. Risk – What Is Best Practice When Assessing Chemicals? Available online at: https://chemsec.org/policy-and-positions/hazard-risk/?utm_source=ChemSec+newsletter&utm_campaign=7a51b01ea8-Hazard_risk&utm_medium=email&utm_term=0_03a9107408-7a51b01ea8-483751655 (accessed December 8, 2021).
Clean Production Action (2021). Available online at: https://www.cleanproduction.org/programs/greenscreen (accessed December 8, 2021).
ECHA (2017). Guidance on the Application of the CLP Criteria. Available online at: https://echa.europa.eu/documents/10162/23036412/clp_en.pdf/58b5dc6d-ac2a-4910-9702-e9e1f5051cc5 (accessed December 1, 2021).
ECHA (2019). FORUM Report on Improvement of Quality of SDS WG “Joint initiative ECHA Forum – ECHA ASOs on Improvement of the quality of SDS”. Available online at: https://echa.europa.eu/documents/10162/22749747/echa_sds_report_en.pdf/0ddb021f-bd1a-09e6-29f3-c52ca476e6aa (accessed December 8, 2021).
ECHA (2021). Substances Restricted Under REACH. Available online at: https://echa.europa.eu/substances-restricted-under-reach (accessed December 8, 2021).
Escher, B. I., and Fenner, K. (2011). Recent advances in environmental risk assessment of transformation products. Environ. Sci. Technol. 45, 3835–3847. doi: 10.1021/es1030799
Escher, S. E., Mangelsdorf, I., Hoffmann, D. S., Partosch, F., Karwath, A., Schroeder, K., et al. (2020). Time extrapolation in regulatory risk assessment: the impact of study differences on the extrapolation factors. Regul. Toxicol. Pharmacol. 112, 104584. doi: 10.1016/j.yrtph.2020.104584
Golden, J., Handfield, R., Daystar, J., Kronthal-Sacco, R., and Tickner, J. (2021). Green Chemistry: A Strong Driver of Innovation, Growth, and Business Opportunity. Green Chemistry and Commerce Council. Available online at: https://greenchemistryandcommerce.org/documents/GC3GreenChemReport-ES-Nov2021.pdf?utm_source=BZ (accessed December 1, 2021).
Hart, G. (ed.).., (2018). Professional Practices of Product Stewardship. Falls Church, VA: AIHA Product Stewardship Society.
Investopedia (2021). Available online at: https://www.investopedia.com/terms/e/environmental-social-and-governance-esg-criteria.asp (accessed December 6, 2021).
Investopedia (2022). Available online at: https://www.investopedia.com/terms/r/risk.asp (accessed February 4, 2022).
IRMI (2022a). Available online at: https://www.irmi.com/term/insurance-definitions/risk#:~:text=Risk%20%E2%80%94%20(1)%20Uncertainty%20arising,which%20an%20insurance%20policy%20relates (accessed February 4, 2022).
IRMI (2022b). Available online at: https://www.irmi.com/term/insurance-definitions/exposure (accessed February 4, 2022).
Kostal, J., Plugge, H., and Raderman, W. (2020). Quantifying uncertainty in ecotoxicological risk assessment: MUST, a modular uncertainty scoring tool. Environ. Sci. Technol. 54, 12262–12270. doi: 10.1021/acs.est.0c02224
Li, L., Sangion, A., Wania, F., Armitage, J. A., Toose, L., Hughes, L., and Arnot, J. A. (2021). Development and Evaluation of a Holistic and Mechanistic Modeling Framework for Chemical Emissions, Fate, Exposure, and Risk. Env. Health Persp. 129:127006. doi: 10.1289/EHP9372
Maertens, A., and Plugge, H. (2018). Better metrics for “sustainable by design”: toward an in silico green toxicology for Green(er) Chemistry. ACS Sustain. Chem. Eng. 6, 1999–2003. doi: 10.1021/acssuschemeng.7b03393
Pham, L., Sheffield, T. Y., Pradeep, P., Brown, J., Haggard, D. E., Wambaugh, J., et al. (2019). Estimating uncertainty in the context of new approach methodologies for potential use in chemical safety evaluation. J. Compu Tox. 15, 40–47. doi: 10.1016/j.cotox.2019.04.001
Plugge, H., Das, N., and Kostal, J. (2021). Towards a universal acute fish threshold of toxicological concern (TTC). Environ. Toxicol. Chem. 40, 1740–1749. doi: 10.1002/etc.4991
Risk Policy Analysts (2019). Study to Collect Recent Information Relevant to Modernising EU Occupational Safety and Health Chemicals Legislation With a Particular Emphasis on Reprotoxic Chemicals With the View to Analyse the Health, Socio-Economic and Environmental Impacts in Connection With Possible Amendments of Directive 2004/37/EC and Directive 98/24/EC. Prepared for European Commission, DG Employment, Social Affairs and Inclusion. Available online at: https://op.europa.eu/en/publication-detail/-/publication/094387fb-da9a-11e9-9c4e-01aa75ed71a1; https://www.researchgate.net/publication/334260797_Study_to_collect_recent_information_relevant_to_modernising_EU_Occupational_Safety_and_Health_chemicals_legislation_with_a_particular_emphasis_on_reprotoxic_chemicals_with_the_view_to_analyse_the_heal
Scivera (2021). Available online at: https://www.scivera.com/ (accessed December 13, 2021).
SHiPP (2021). Sustainable Health in Procurement Project. Available online at: https://noharm-global.org/issues/global/sustainable-health-procurement-project (accessed December 8, 2021).
Spaniol, O., Bergheim, M., Dawick, J., Kotter, D., McDonough, K., Schowanek, D., et al. (2021). Comparing the European Union System for the Evaluation of Substances (EUSES) environmental exposuremcalculations with monitoring data for alkyl sulphate surfactants. Environ. Sci. Eur. 33, 3. doi: 10.1186/s12302-020-00435-1
Swanson, M. B., Davis, G. A., Kincaid, L. E., Schultz, T. W., Bartmess, J. E., Jones, S. L., et al. (1997). A screening method for ranking and scoring chemicals by potential human health and environmental impacts. Environ. Toxicol. Chem. 16, 372–383. doi: 10.1002/etc.5620160237
Toxnot (2021). Available online at: https://toxnot.com/ (accessed December 13, 2021).
UNDP (United Nations Development Programme) (2020). Sustainable Health Procurement Guidance Note. Available online at: https://www.undp.org/sites/g/files/zskgke326/files/publications/undp-SPHS-bpps-health_Sustainable_Health_Procurement_Guidance_Note.pdf (accessed December 8, 2021).
United Nations (UN) (2021). Globally Harmonized System of Classification and Labelling of Chemicals (GHS Rev. 9, 2021). Available online at: https://unece.org/transport/standards/transport/dangerous-goods/ghs-rev9-2021 (accessed December 1, 2021).
USEPA (1993). Reference Dose (RfD): Description and Use in Health Risk Assessments. Background Document 1A. Available online at: https://www.epa.gov/iris/reference-dose-rfd-description-and-use-health-risk-assessments (accessed December 8, 2021).
USEPA (2021). Alternative Test Methods and Strategies to Reduce Vertebrate Animal Testing. Available online at: (accessed December 6, 2021).
Verisk 3E (2021). Available online at: https://www.verisk3e.com/ (accessed December 13, 2021).
Keywords: chemical sustainability, sustainability metrics, sustainability index, chemical products, KPI, GHS, chemical inventory, SDS
Citation: Plugge H (2022) ChemESI (Chemical Environmental Sustainability Index)—A KPI (Key Performance Indicator) for Standardizing Environmental Metrics for Chemical Sustainability. Front. Sustain. 3:834849. doi: 10.3389/frsus.2022.834849
Received: 13 December 2021; Accepted: 21 February 2022;
Published: 25 March 2022.
Edited by:
Shutaro Takeda, Kyoto University, JapanReviewed by:
Li Li, University of Nevada, Reno, United StatesDaikichi Seki, Kyoto University, Japan
Copyright © 2022 Plugge. This is an open-access article distributed under the terms of the Creative Commons Attribution License (CC BY). The use, distribution or reproduction in other forums is permitted, provided the original author(s) and the copyright owner(s) are credited and that the original publication in this journal is cited, in accordance with accepted academic practice. No use, distribution or reproduction is permitted which does not comply with these terms.
*Correspondence: Hans Plugge, aGFucy5wbHVnZ2VAc2FmZXJjaGVtaWNhbGFuYWx5dGljc2xsYy5jb20=