- University of North Carolina, Chapel Hill, NC, USA
The mainstream status-achievement model of sociology and economics views individual careers as sequences of causally linked status-related traits and outcomes, such as ability, educational attainment, and occupational status. The variance decomposition models of quantitative genetics interpret variation in these traits and status outcomes as produced by latent factors summarizing genetic, shared environmental, and non-shared environmental sources of influence. This article proposes that the two approaches can be combined and together provide valuable insights into core issues of social stratification research. The argument is illustrated with respect to three issue areas: (1) nature of family environmental influences in educational attainment; (2) sources of associations among successive status-related outcomes; and (3) implications of the combined status-achievement/quantitative genetic model for the relative strengths of opportunities for mobility versus social forces of reproduction facing individuals in different social positions and in different societies.
1. Introduction
The dominant status-achievement model of sociology and economics and the multivariate model of quantitative genetics give differing accounts of the mechanisms of social mobility and career continuity, i.e., the relative consistency of status-related outcomes within individual careers over the life course. In the status-achievement perspective, career continuity reflects direct causal links among status-related outcomes, so that, for example, greater educational attainment “causes” entry into occupations at a higher level of prestige (Blau and Duncan, 1967). For the quantitative genetic model, career continuity reflects similar effects on the outcomes of common latent factors, corresponding to genetic and environmental (shared and non-shared) sources, so that the same combinations of cognitive and non-cognitive skills that enhance educational attainment also favor entry into higher prestige occupations (Rowe, 1994). Associations among status-related outcomes are largely spurious rather than causal. Can these two views be reconciled?
The central argument of this paper is that the status achievement and quantitative genetic models can indeed be reconciled and that the combination of the two perspectives provides novel and valuable insights into core issues of social stratification research. The next section describes and contrasts the status-achievement model and the multivariate quantitative genetic model. The following three sections illustrate the usefulness of a combined model with respect to three issue areas of contemporary stratification research: (1) nature of the substantial family environmental influences in educational attainment; (2) assessing the sources of the interrelationships among successive career outcomes; and (3) conceptualizing and measuring the relative degree of opportunity for mobility versus the impact of ascriptive mechanisms (forces of social reproduction) facing individuals in different social positions and in different types of society. The paper ends with a discussion and a conclusion.
2. Two Perspectives on Social Stratification and Mobility
2.1. The Status-Achievement Model
Research in social stratification and mobility in the first part of the twentieth century had focused on intergenerational mobility tables of class transitions from fathers to sons (Lipset and Bendix, 1959). In the 1960s, the emphasis shifted to the processes by which individuals reach their adult social status. The new paradigm, called the status-achievement model and still dominant in the field, represented various status-related outcomes – completion of formal education, first occupation, current occupation, income – as arrayed in roughly successive stages along the life course (Blau and Duncan, 1967). The status-achievement model is illustrated in simplified form in Figure 1, where later outcomes are shown as caused by earlier ones and ultimately affected by background variables comprising characteristics of the family of origin, such as parental education and occupation. Coefficient estimates in the figure are standardized regression coefficients calculated from 1989 General Social Survey (GSS) data (Table 1), but the empirical pattern for these more recent data is very similar to that originally found by Blau and Duncan (1967).
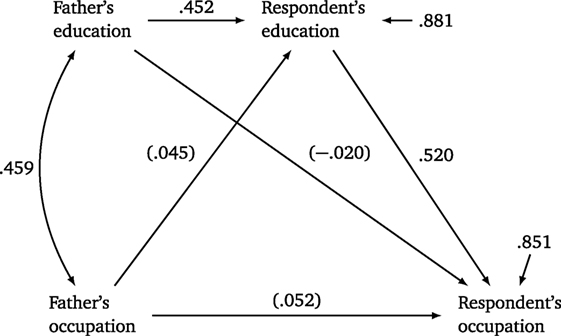
Figure 1. A simple status-achievement model. Numbers along straight arrows are standardized regression coefficients; numbers along curved arrows are correlations; coefficients in parentheses are non-significant (p ≥ 0.05). Source: see Table 1.
The coefficient associated with a straight arrow estimates the direct “effect” of a variable on another, expressed as the change in the response variable (in standardized units) associated with a 1 SD increase in the explanatory variable. The rules of path analysis (Wright, 1934; Li, 1975) can be used to calculate the indirect effect of a variable as the product of the paths linking the two. For example, the indirect effect of Father’s education on Respondent’s occupation was 0.235 = 0.452 × 0.520. The total effect of an explanatory variable is calculated as the sum of the direct and all indirect effects, so the total effect of Father’s education on Respondent’s education was 0.215 = –0.020 + 0.235.
Blau and Duncan (1967) drew three major substantive conclusions from their work.
1. Direct effects of father’s occupation and father’s education on son’s occupation are small or non-significant, and thus there is little evidence of direct reproduction of social status.
2. There is a substantial indirect effect of father’s occupation and father’s education on respondent’s occupation, mostly through respondent’s education, a pattern suggesting that education is a principal mechanism (“a conveyor belt” in the authors’ words) of status reproduction. The central role of education has been well supported in later research (Jencks, 1979; Johnson et al., 2010).
3. A large part of the total association (r = 0.524) between respondent’s education and occupation was driven by the indirect effect (0.458 = 0.881 × 0.520) of residual factors of education, which are (by construction) independent of social origins, a pattern suggesting that achievement is driven in large part by unmeasured personal motivations and abilities not associated with parental status. This combination of traits, inherent to the individual, is often conceptualized as “merit.”
What are these ingredients of achievement independent of social origins? Cognitive ability (intelligence) was an obvious candidate. Blau and Duncan (1967) did not have a measure of ability, but Duncan (1968) was able to assemble estimates of the correlations between achievement model variables and two measures of cognitive ability (in childhood and in adulthood) and thereby estimate an expanded version of the model that included the ability measures. He found that IQ measured in childhood had stronger effects (in terms of standardized regression coefficients) than other background variables (father’s education and occupation, and number of siblings) on all three socioeconomic outcomes analyzed (respondent’s education, occupation, and earnings) [see also Duncan et al. (1972)].
While still recognizing, like Blau and Duncan (1967), that family background affects adult status by affecting access to education, Duncan (1968) noted the relatively weak relationship between background and IQ, concluding with an even stronger emphasis on the central role of unmeasured factors (p. 11).
In view of the loose relationship between IQ and social class in the United States, it seems that one […] function of the ability measured by intelligence tests is that it serves as a kind of springboard, launching many men into achievements removing them considerable distances from the social class of their birth. IQ, in an achievement-oriented society, is the primary leaven preventing the classes from hardening into castes. […] In the event that some appreciable fraction of the variation in IQ itself is ultimately traced to genetic rather than environmental factors, this conclusion will stand a fortiori; but its measure of validity does not depend on any assumption that this is the case.
Duncan (1968), thus, associated a strong effect of IQ on achievement with a high degree of social mobility and socioeconomic opportunity, suggesting that documenting genetic sources of IQ would further strengthen that interpretation. Duncan’s “optimistic” interpretation of the role of IQ and the role of genes in socioeconomic achievement as indicative of greater opportunity and mobility was at variance with the intuition of many in the public and academia (especially outside the fields concerned with intelligence research), as revealed in later controversies (Jensen, 1969; Herrnstein, 1973; Herrnstein and Murray, 1994).
Later research in the status-achievement tradition in sociology and economics has expanded the list of unmeasured, individual-specific traits and habitual behaviors of an individual that may have contributed to residual influences on educational outcomes and labor-market success in the original status-achievement model. These characteristics can be roughly categorized into cognitive and non-cognitive traits. Farkas (2003), 556, summarizes a review of the literature as follows (p. 556).
[T]here is relatively strong evidence that similar cognitive skills and noncognitive behavioral traits determine both school and labor-market success and that these skills and habits together constitute key endogenous variables in the determination of outcomes at all levels of the stratification system.
Non-cognitive behavioral traits associated with achievement outcomes include perseverance, dependability, and consistency (Bowles and Gintis, 1976); leadership, study habits, industriousness, and perseverance (Jencks, 1979); the big five personality traits, especially conscientiousness and emotional stability (Judge et al., 1999; Barrick et al., 2001); and self-esteem and locus of control (Heckman and Rubinstein, 2001; Heckman et al., 2006); see also Farkas (2011).
The possibility that unmeasured cognitive and non-cognitive traits affect several successive stratification outcomes independently in part of social origins, together with Blau and Duncan’s (1967) discussion of the relative importance of these residual factors in the achievement process, points to a fundamental ambiguity in the causal language commonly used in interpreting the status-achievement model. For example, if the same personality trait of conscientiousness affects both school (educational attainment) and occupational success (prestige), then the association between the two measures represented by a straight “causal” arrow in Figure 1 is actually in part spurious as it combines effects of unmeasured common sources of both educational and occupational achievement measures. In other words, an individual scoring high on conscientiousness may achieve higher levels of both educational and occupational success, but occupational success is not the direct consequence of educational success.
2.2. Quantitative Genetics and the ACE Model
The quantitative genetic approach to socioeconomic achievement traces its roots as far back as Galton (1869). The conceptual and statistical apparatus of quantitative genetics was developed in the first half of the twentieth century as part of the modern synthesis of Darwinian evolutionism and Mendelian genetics (Fisher, 1918; Wright, 1920), and further refined in the fields of plant and animal breeding before being applied to human physical and psychological traits (Jinks and Fulker, 1970; Martin and Eaves, 1977). The quantitative genetic approach applied to human traits is commonly known as behavior genetics. Before the advent of molecular genetics, human behavior genetic research relied exclusively on the so-called biometrical methods based on twins, adoptees, and other relatives that vary in their degree of genetic relatedness, in contrast to the samples of unrelated individuals typically used in social science research.
The workhorse of quantitative genetics is a simple model that decomposes the individual value of a trait P into a linear function of three unmeasured (latent) sources corresponding to genetic (A), shared environmental (C), and non-shared environmental (E) influences
Assuming (for simplicity) that the latent variables A, C, and E are standardized with mean = 0 and SD = 1, the three sources are independent, and the sources do not interact in affecting P, the variance in the trait P can be represented as
where a′2, c′2, and e′2 represent the variances in P attributed to each source. When the model is standardized by dividing both sides of the equation by Var(P) the model becomes
where a2, c2, and e2 represent the proportions of variance in P attributed to each source. Assumptions on the independence and absence of interaction among the sources can be relaxed in more elaborate models (Purcell, 2002).
Heritability a2 is the proportion of the variance in a trait that is contributed by all genetic sources of influence.1 The shared environment c2 represents the effects of all characteristics of the family or embedding social environment that tend to make siblings similar on the trait; it includes effects of social class and of other factors, such as ethnicity or the quality of local schools that tend to vary more between than within families. The unshared environment e2 represents the effects of all environmental influences that tend to make siblings different from each other, such as a perinatal event, disease, or inspiring experience affecting one sibling but not the other, and includes (but is not limited to) measurement error. The model is often called the ACE model, after a common notation for the latent factors.
In the context of social mobility, where the phenotype of interest is a status-related outcome, such as years of schooling or earnings, the variance components a2 and c2 of the ACE model have specific substantive interpretations. Consistent with Duncan’s (1968) discussion – and perhaps counter-intuitively – the proportion a2 of the phenotypic variance due to genes indicates greater opportunity for individuals to achieve their native potential, high or low. The shared environment component c2, as the combined effect of (non-genetic) environmental influences on the status-related trait, can be thought of as measuring the strength of social reproduction of status (Conley, 2008). From a policy-oriented point of view, c (the square root of c2) measures the potential effect on the trait (expressed in standard deviation units) of raising the quality of the family environment by 1 SD. c2, thus, reflects the extent of improvement on the trait achievable by an intervention modifying the environment within the existing range of environmental variation (Taubman, 1976a; Behrman and Taubman, 1989; Rowe, 1994).
The highlighted condition of the previous paragraph is essential, as c2 does not measure the potential impact on a trait of environmental interventions that have not yet been discovered, or environmental interventions known to be effective but that do not substantially affect the current variance in the trait because of limited application, perhaps due to their high cost. In sum, greater heritability (proportion of variance in achievement due to genetic variation) in a status-related trait is indicative of greater opportunity for mobility, while a greater proportion of variance due to shared environmental factors is indicative of less opportunity for mobility and stronger social reproduction of advantage (Behrman and Taubman, 1989; Guo and Stearns, 2002; Jencks and Tach, 2006; Nielsen, 2006, 2008; Adkins and Guo, 2008; Adkins and Vaisey, 2009; Saunders, 2010; Marks, 2014).
An important observation concerning the ACE model is that the variance components a2, c2, and e2, just like the mean and variance, are parameters characterizing a population, not a trait. This qualification is often left implicit so that, for example, the statement “the heritability of IQ is 0.6” omits specifying the relevant context, such as “among adolescents in the United States at the beginning of the 21st century.” Parameters of the ACE model can and do differ across societies and time periods. With respect to social stratification and mobility, this point is of considerable substantive as well as methodological importance, as it implies that the parameters of the ACE model can be viewed as properties of the social structure of a society. The relative values of the parameters for a status-related trait then provide meaningful measures of opportunity for mobility (or, conversely, the strength of social ascription) in comparing social structures of societies that differ in level of development, political institutions, or generosity of the welfare system, or the same society at different points in history (Nielsen, 2006; Adkins and Vaisey, 2009).
The role of genes in cognitive ability has been extensively documented (Bouchard and McGue, 2003). Many of the non-cognitive traits associated with achievement outcomes are also determined in part by genes. The quantitative genetic literature on personality factors typically finds moderate heritability but little if any effect of the shared environment on the traits (Eaves et al., 1989; Loehlin, 1992; Rowe, 1994). For example, Bouchard and McGue (2003) state in conclusion of their extensive review of the literature (p. 23): “In all of the studies [of personality factors] shared environmental influence was estimated at zero or near zero.” Thus, to the extent that they behave like personality factors, the non-cognitive traits underlying school and occupational success would contribute to the heritability of later status-related outcomes but would not help explain the strong shared environment component found in quantitative genetic studies of some of these outcomes, notably educational attainment (Branigan et al., 2013), a pattern further discussed later.
One domain that has been relatively neglected in social stratification research is that of vocational interests. The review of Bouchard and McGue (2003) finds that vocational interests are one domain where significant influences of the shared environment have been found. Interestingly, the possibility that vocational interests are substantially affected by the family environment echoes an emerging literature in sociology documenting that part of the intergenerational transmission of status occurs through reproduction of “microclasses” – specific occupations, such as physician, actor, and carpenter – as opposed to more broadly defined “big classes” (Jonsson et al., 2009).
2.3. Combining the Status-Achievement and Behavior Genetic Models
Research on the role of genes in socioeconomic success was slow to emerge in the social sciences. According to historian Degler (1991) the mid-twentieth century reluctance to consider the role of genes in socioeconomic success was the end result of a decades-long trend of declining enthusiasm for Darwinian evolutionism on the part of progressively oriented academics, because of concerns that a substantial role of heredity in socioeconomic achievement would justify social inequalities or falsely imply that they cannot be remedied through social reforms [e.g., Goldberger (1978, 1979) contra Taubman (1976a, 1978, 1981)].
Explicit theoretical and empirical consideration of the role of genes in social mobility emerged gradually in sociology and economics, along with the revival of Darwinism in social thought throughout the social sciences described by Degler (1991). Sociologists and economists began to look at theoretical implications and estimate empirically the role of genes on status-related outcomes using family data, such as twins, and adoptees with their adoptive and biological parents (Eckland, 1967, 1979; Becker, 1975; Behrman and Taubman, 1976; Taubman, 1976a; Behrman et al., 1977; Scarr and Weinberg, 1978; Becker and Tomes, 1979, 1986; Jencks, 1979). A special motivation for economists was a desire to obtain unbiased estimates of the earnings return to investment in human capital, such as education – i.e., the true causal effect of education on earnings – net of the confounding effects of native endowments (Taubman, 1976b; Behrman et al., 1980; Behrman and Rosenzweig, 1999).
The status-achievement and behavior genetic models seem to imply different notions of the causation of status-related outcomes. In the status-achievement perspective, causation takes place through direct and indirect effects of measured traits or prior outcomes. In the behavior genetic model, causation flows from latent factors partitioned according to ultimate sources of influence on the trait (genetic, shared, and non-shared environmental) (Rowe, 1994). A new development is that of a composite view integrating both perspectives (Petrill and Wilkerson, 2000; Kohler et al., 2011).
In the remainder of the paper, I will describe research combining both perspectives that has produced new insights in processes of social mobility as well as some associated issues that are not yet resolved. Three areas are considered: (1) respective roles of genes and the shared environment in educational attainment, (2) role of genes in associations among status-related outcomes, and (3) links of the combined genetic–environmental model with social stratification theories.
3. The Shared Environment in Educational Attainment
Twin and adoption studies of many physical and behavioral traits in humans have found substantial heritability but smaller effects of the shared environment (Freese, 2008). Turkheimer (2000), 160, has listed this pattern as the second of his “three laws” of human behavior genetics: “The effect of being raised in the same family is smaller than the effect of the genes.” The pattern holds specifically for the trait of intelligence in later adolescence and adulthood, for which the role of genes is substantially larger than that of the shared environment. For intelligence, the relative roles of genes and the shared environment change in the course of development: while there is a substantial effect of the family environment in childhood, this component of the variance vanishes for intelligence measured in young adulthood and at older ages (Boomsma et al., 2002; Bouchard, 2009).
Educational attainment, measured as highest degree earned or years of schooling completed, is an apparent exception to Turkheimer’s second law. In his pioneering study of a large sample of World Was II veterans, Taubman (1976a) found a heritability of 44% but also a substantial shared environment component corresponding to 32% of the variance in educational attainment. In a comprehensive meta-analysis of educational attainment (measured as highest degree earned or years of completed schooling) covering studies in many developed countries over several decades Branigan et al. (2013) found the pattern of a substantial shared environment component to be widespread, estimating mean heritability over all studies at 0.40 and the mean shared environment component at 0.36.
The finding that shared family influences contribute to the variance in a status-related trait, such as educational attainment, is of considerable interest for social scientists. The shared environmental component c2 measures the proportion of trait variance due to all environmental influences that are shared by siblings but vary between families – variables, such as social class, ethnic culture, and quality of neighborhood and local schools. Evidence that these family-related factors remain influential in adulthood (when educational attainment is measured) suggests an enduring effect of ascriptive distinctions on the socioeconomic achievement of individuals, and thus a persisting role of social ascription (Taubman, 1976a; Nielsen and Roos, 2015).
In order to further explore the genetic–environmental architecture of educational attainment, Nielsen and Roos (2015) used data on sibling pairs collected as part of the Add Health longitudinal study of adolescents in U.S. schools (Harris et al., 2006; Harris, 2009). The design yielded a total of 1576 pairs of variously related pairs of twins and non-twin siblings living in the same household at the time of the initial survey. The sample was composed of monozygotic (MZ) twins, dizygotic (DZ) twins, full siblings, half siblings, cousins, and non-related siblings. Educational attainment was measured as highest degree earned at the fourth wave of the study, at a time when participants were aged 24–32 years. Correlations in educational attainment for the six sibship types are shown in Table 2. The authors used structural equation models (SEMs) to estimate ACE decompositions of the variance in attainment.
When data on twin pairs only were used, the ACE decomposition yielded a2 = 0.15, c2 = 0.48, and e2 = 0.37, corresponding to relatively weak heritability together with a more substantial shared environment component. When data for all six types of sibling pairs were used the ACE decomposition was a2 = 0.37, c2 = 0.27, and e2 = 0.36, corresponding to larger heritability and a smaller shared environment component compared to twins only. Finally, the authors estimated models in which the effect of genes is constrained to be the same for twin and non-twin siblings, but the effect of the shared environment was allowed to vary. They found that the best-fitting model overall was one with heritability a2 = 0.23; the shared and non-shared environment components were and for twins, and and for non-twin siblings. Thus, the role of the shared environment was greater for twins than it was for non-twin siblings. An alternative way to describe the finding is to say that the family environments of twins (of either kind) were significantly more correlated than the environments of non-twin siblings.
Nielsen and Roos (2015) discussed several implications of these results. First, even for non-twin siblings estimates of c2 were higher than for most psychological traits. The high c2 could not have been the result of environmental family influences on the cognitive and personality traits that are the psychological ingredients of success in the educational and professional realms, including educational attainment, as the latter outcomes tend to exhibit moderate to high heritability but near-zero effects of the shared environment (Bouchard and McGue, 2003). The high shared environment was also unlikely to result from family values encouraging or discouraging all siblings to the same extent toward educational pursuits, as these values would have to be assumed as acting more powerfully on twins than on non-twin siblings. The empirical pattern rather suggested that the shared environment in educational attainment was related to variation in family resources, especially financial ones (Conley, 2001; Jencks and Tach, 2006).2 Second, the finding of a higher shared environmental effect c2 for twins than for non-twin siblings suggested that estimates of the shared environment based on twins in other studies, such as those compiled in the meta-analysis by Branigan et al. (2013), may also have been inflated. The bias would originate in the strong effect of financial resources, and contemporaneity of twins (of both types) in the life course that makes their family environments more similar than for non-twin siblings, who were born on different dates. The possibility that the family environment might be more similar for twins than for other kinds of siblings violates an important assumption for the external validity of twin research (Benjamin et al., 2012). Third, if the strong shared environment component of educational attainment is in fact linked to financial resources, it would imply that there is room for improving educational opportunity through traditional policy means – that is, policies affecting factors involved in extant variation in family environments in the population – such as reducing and equalizing the cost of education for individuals (Taubman, 1976a; Rowe, 1994).3
4. Interrelationships Among Status-Related Outcomes
As much research in the status-attainment tradition has shown, measures of abilities and of educational, occupational, and economic outcomes are intercorrelated (Strenze, 2007). Jensen (1998) has used the term “g nexus” to describe this set of associations, which he views as reflecting the pervasive impact of cognitive ability on socioeconomic outcomes. The standard status-achievement model of Blau and Duncan (1967) explained these associations as resulting from causal links relating these variables. In an early attempt to evaluate the overall impact of the genetic sources of ability on income, Jencks et al. (1972) used a simplified status-achievement model consisting of the causal chain IQ genes → IQ → Years of education → Income and calculated the percentage of variance in income that can be attributed to genes affecting IQ. Jencks et al. (1972) showed that the indirect effect of IQ genes on income is small, as it is the product of a series of path coefficients, each of which is less than unity; the contribution of IQ genes to income variance is smaller still, as it is the square of this indirect effect [see Bowles and Gintis (2002) for a similar argument].
Rowe (1994), 138–140 and his Figure 5.1 criticized this logic, pointing out that the “chain model” is unrealistic and underestimates the effects of genetic heterogeneity on later socioeconomic outcomes (p. 139).
What is seriously wrong with Jencks’s chain model? Its problem is that genes do not produce a test score at age 11, which next directly causes years of education, which next directly causes incomes. Rather, genes produce a phenotype – a person with particular intellectual abilities and weaknesses. Persons’ strengths and weaknesses affect their encounters with the IQ test at age 11, the demands of schooling, the opportunities of the job market, and the rigors of succeeding in a job. Thus the influence of the genes is not mediated through the test score itself, and Jencks’s model as a literal representation of genetic influence becomes misleading.
Rowe (1994) proposed an alternative model that specifies genetic (as well as environmental) influences on each one of the intermediate outcomes, as well as on income. With that model [using the same bivariate correlation estimates as Jencks et al. (1972)], he obtained a much larger estimate of the variance of income attributable to genes (about 50%) in comparison with Jencks et al.’s (7–9%).
The alternative model proposed by Rowe (1994) is a multivariate extension of the ACE model. This quantitative genetic model permits, with suitable family data, to estimate the genetic, shared, and non-shared environmental components of both the variation in the status-related measures and the associations among measures. As such the multivariate ACE model of the type Rowe (1994) proposed is a means of implementing Blau and Duncan’s (1967) intuition that the correlation between education and later occupational status is driven by unmeasured (i.e., latent) individual resources independent of family background.
Nielsen (2006) illustrated the use of the multivariate ACE model in analyzing the interrelationships among three status-related educational outcomes measured when participants in the Add Health study were in high school: (1) Verbal ability (VIQ), was the score on a test comprising 87 items from the Peabody Picture Vocabulary Test, measured on an IQ scale with mean = 100 and SD = 15; (2) High school grade point average (GPA) was based on grades, in each of two survey waves, in the fields of English or language arts, mathematics, history or social studies, and science; and (3) College plans (CPL) was an average of questions on college aspiration and expectation asked in two survey waves. The data were from the siblings subsample of Add Health, with sibling pairs classified into six types as monozygotic twins (MZ, 170 pairs), dizygotic twins (DZ, 290 pairs), full siblings (FS, 702 pairs), half siblings (HS, 242 pairs), cousins (CO, 105 pairs), and non-related siblings (NR, 174 pairs).
The multivariate ACE model was implemented by viewing the three outcomes as functions of (initially) nine latent factors: three genetic factors labeled A1–A3, three shared environmental factors C1–C3, and three unshared environmental factors E1–E3, with the subscript referring to each of the three outcomes. All the latent factors were constrained to have zero mean and unit variance. Just as in the univariate ACE model, within a pair of siblings each A factor was assumed correlated by the degree of genetic relatedness of siblings (proportion of genes shared by common descent): 1 for MZ twins and 0 for non-related siblings; assuming random mating (an assumption that can be relaxed), the correlations were set at 1/2 for DZ twins and full siblings, 1/4 for half siblings, and 1/8 for cousins. Within a pair each C factor was assumed correlated by 1 (each sibling is exposed to the same family environment, irrespective of sibship type), and each E factor by 0. As in the univariate case, within a pair, each Ai was assumed uncorrelated with the corresponding Ci and Ei.
Nielsen (2006) estimated the multivariate ACE model from variances and covariances of the three outcomes for the six types of pairs using the structural equations (SEM) program Mx (Neale et al., 2006). Estimation started, for technical reasons,4 by estimating a special saturated form of the model with triangular coefficient matrices called a Cholesky factorization. In this initial specification, factors of each type (the A factors, C factors, etc.) were assumed orthogonal, and the matrix of coefficients representing effects of the latent factors was assumed triangular. In the illustration, for example, A1 was assumed to affect all three outcomes (VIQ, GPA, CPL), A2 affected only GPA and CPL, and A3 affected only CPL. The initial Cholesky factorization is not in general interpretable substantively (Loehlin, 1996).
To select the final specification the initial model was “pruned” through a nested sequence of chi-squared tests on models simplified by setting effects of some factors on the outcomes to zero. The solution with the minimal set of factors of each type that still fit the data (and made theoretical sense) was retained [see Nielsen (2006), Figure 1 for the initial Cholesky factorization and Table 2 for the sequence of chi-squared tests]. Estimates were then transformed one last time to allow correlations among the latent factors within each of the A, C, and E sets, into a model where each observed variable is affected by at most one factor for a given source of variation.5 The resulting “correlated factors” model was suitable for substantive interpretations (Loehlin, 1996). The entire process is explained in Neale and Maes (2004); empirical examples are found in Emde and Hewitt (2001); a more recent example (albeit embedded in a more complex model) is Johnson et al. (2006).
Results for the VIQ, GPA, and CPL example are shown in Figure 2. The model shows how the latent factors (in circles) affected the manifest outcomes (represented as squares) in the best-fitting model. Estimated standardized coefficients are shown on the left half of the model for the first sibling in a pair. (Coefficients of corresponding paths are the same for the second sibling and are not shown to reduce clutter.) This preferred model represented effects of genes as three separate, albeit correlates genetic factors, each of which affects a specific outcome. The numbers along the straight arrows from each A factor are standardized regression coefficients measuring the impact of genes on the corresponding trait. These estimated coefficients are equal to the square root of heritability for the observed trait. For example, the coefficient of 0.82 relating A2 to GPA means that the heritability of GPA was 0.67 = 0.822, i.e., 67% of the variance in GPA was accounted for by genetic influences. Likewise, percentages of the variance accounted for by the genes was 53% for Verbal IQ (0.533 = 0.732) and 59% for College plans (0.593 = 0.772).
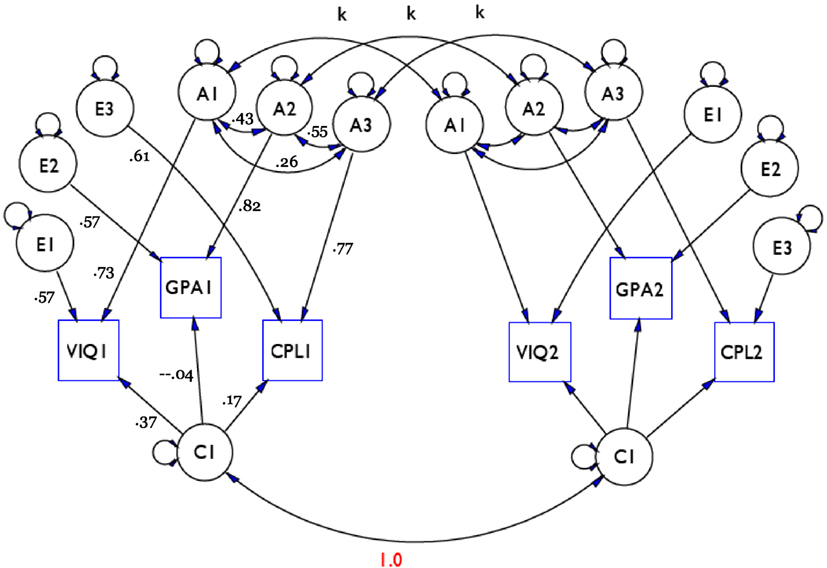
Figure 2. Preferred multivariate ACE model for VIQ (Verbal IQ), GPA (High school grade point average), and CPL (College plans). A1, A2, and A3 are latent factors representing genetic influences on VIQ, GPA, and CPL, respectively; C1 is a single latent factor of shared environmental influences; E1, E2, and E3 are latent factors of unshared environmental influences on the three measures; k is the assumed correlation among genetic factors of siblings: k = 1 for MZ twins and k = 0 for unrelated siblings; assuming random mating k = 1/2 for DZ twins and full siblings, k = 1/4 for half siblings, and k = 1/8 for cousins. Source: Nielsen (2008).
Intercorrelations among the A factors are the result of pleiotropy, or overlap of the sets of genes affecting two traits.6 There were moderate correlations between genes affecting Verbal IQ and GPA (0.43), and GPA and College plans (0.55); the correlation was lower for Verbal IQ and College plans (0.26), suggesting that genes affecting these two traits overlap only partially. Note that the best-fitting model included three separate genetic factors, one for each outcome, rather than one. This is because the hypothesis that there is a single set of “academic success” genes affecting all three measures was rejected in the course of model pruning.
By contrast, the hypothesis that there is a single shared environmental factor C capturing all shared environmental effects affecting all three outcomes could not be rejected. In other words, the same shared environmental influences, represented in the component C, affect each one of the educational outcomes, albeit to varying extents. Social scientists may be tempted to identify this single factor as “privilege.” However, latent factor C acts as a “black box” whose substantive nature remains opaque, so C could well incorporate effects of family culture, such as a tradition of learning, in addition to aspects of social privilege. Effects of the shared environment were relatively small compared to effects of the genes, however. For example, the shared environmental component c2 of the variance was only 0.14 = 0.372 for Verbal IQ and 0.03 = 0.172 for College plans. The shared environment was essentially zero for GPA (and the corresponding path could have been dropped entirely without decreasing model fit).
The particular nature of GPA as a measure of educational success may have been responsible for the small estimated effect of the shared environment component for that variable, because a privileged family environment may affect the GPA of an individual student in two opposite directions. On the one hand, more family resources in the form of better nutrition, a more intellectually stimulating environment, more parental financial support, etc., may improve school performance, resulting in higher grades. On the other hand, family privilege may secure attendance at a better and, therefore, more competitive school, with the same objective level of performance resulting in lower GPA, as the latter reflects lower relative performance in comparison with classmates. This would be an instance of a classic “frog pond” effect (Davis, 1966). It is, thus, possible that the shared environmental component of GPA was near zero because shared family influences acting in opposite directions on that trait canceled out.
Finally, the hypothesis could not be rejected that the unshared environmental factors E1–E3 corresponding to each outcome were uncorrelated within sibling as well as between them (per assumption), as represented by the absence of curved arrows connecting the E factors in Figure 2. The zero correlations were not a necessary assumption of the model but an empirical finding based on the data. This may appear somewhat surprising, since some of the specific events affecting one sibling but not another that contribute to the latent E factors – such as a perinatal injury affecting one sibling, or parental preference for one sibling over another – would be expected to affect all three educational outcomes in the same direction, causing the Es to be correlated. The empirical pattern found suggested that such systematic unshared differences played a relatively small role in the three educational outcomes, so the E factors behaved much like the independent error terms assumed in the classic regression model. The hypothesis that the E factors would be so closely correlated as to be equivalent to a single unshared environmental factor E affecting all three outcomes – in a way similar to the reduction of the shared environmental factors into a single factor C – was even less consistent with the data.
An additional insight provided by the multivariate ACE model is that it was possible to calculate the extent to which a correlation between two outcomes was due to genetic or shared environmental factors. Using Verbal IQ and College plans as an illustration, the computation proceeded as follows. From the tracing rules of path analysis (Wright, 1934) applied to coefficients estimates in Figure 2, the correlation between the traits due to genes was 0.146 = 0.73 × 0.26 × 0.77; the correlation due to the shared environment was 0.063 = 0.37 × 0.17; it followed that the predicted (total) correlation between the two traits was 0.209 = 0.146 + 0.063, so that the proportion of the total correlation due to genes was 0.699 = 0.146/0.209. In other words, 70% of the correlation between verbal ability and college plans was attributed to the overlap of the genes that affect the two traits, and only 30% was due to the shared family environment. This conclusion too may be surprising, given the relatively small degree of overlap between Verbal IQ and College plans genes (corresponding to a correlation of only 0.26). The correlations between Verbal IQ and GPA, and GPA and College plans, derived using similar calculations, turned out to be entirely due to genetic sources, with no contribution of the shared family environment.7 Similarly, strong genetic influences on correlations among status-related outcomes have been found in other studies (Calvin et al., 2012).
The status-achievement model and multivariate quantitative genetic model just illustrated imply different views on the mechanisms of continuity in individual careers, i.e., the mechanisms by which status-related outcomes are related over the life course. In the status-achievement model, career continuity results from direct causal links among status-related outcomes, so that, for example, educational attainment “causes” entry into occupations at a certain level of prestige (Blau and Duncan, 1967). For the quantitative genetic model, career continuity associations among outcomes are due to common factors affecting the traits, corresponding to genetic and environmental (shared and non-shared) sources (Rowe, 1994).
In the context of a quantitative genetic model, it is, however, possible to think of a status-related outcome affecting another in a direct causal fashion. In the illustration, for example, one can envision that the feedback that a student receives from teachers, summarized as the GPA, directly influences College plans.8 A real and substantively important example where distinguishing causal from common factor (including genetic) influences is essential is that of the substantial economics literature on effects of schooling on later economic outcomes, such as earnings, where researchers have used genetically informative data on twins to distinguish the pure causal effect of education on earnings net of the confounding influences of individual endowments (Taubman, 1976b; Ashenfelter and Krueger, 1994; Behrman and Rosenzweig, 1999; Miller et al., 2006).
Incorporating direct causal links in a multivariate ACE model and estimating them is difficult. In the illustration above, for example, one could not estimate a model that adds causal arrows between Verbal IQ and GPA, and between both Verbal IQ and GPA and College plans, as these paths are unidentified in the context of the larger model. In some special cases, genetically informative data might help exclude some types of causal relations. Neale and Maes (2004) discuss the example of the ambiguous relationship between exercise and body weight. If it was found that variation in exercise is purely environmental, and variation in weight partly genetic, then body weight cannot cause exercise since if it did it would induce genetic variation in exercise. Issues of causation are further discussed in the behavior genetic context in Duffy and Martin (1994) and Heath et al. (1993). Limitations on estimating causation are discussed in Johnson et al. (2011a,b,c).
In an important contribution, Kohler et al. (2011) review strategies available for distinguishing causal effects from effects of latent factors in multivariate analyses with twin data. They propose the ACE-β model that incorporates both the ACE decomposition of variance that is central to quantitative genetics and the causal effects (β) that are of primary interest in economics and sociology. They show that identifying both the variance components and direct effects of the traits on each other is possible at the cost of additional assumptions on the model, the most natural being that the unique (non-shared) environmental components (E) corresponding to different traits are uncorrelated within sibling.
5. Genes–Environment Interactions (G × E) in Status Achievement
Scarr-Salapatek (1971) conjectured that the relative roles of genetic and shared environmental sources of intelligence and academic achievement would vary according to socioeconomic status of the family. In advantaged environments, genetic potential can be fully expressed so that heritability of mental ability will be high and the effect of the shared environment low. In deprived environments, expression of genes will be inhibited, so that heritability will be lower and the impact of the shared environment greater. The conjecture has been called the Scarr–Rowe hypothesis of Gene × Socioeconomic Status (SES) interaction (Tucker-Drob and Bates, 2016). In general, interactions between genes and environment of this type are called G × E (Shanahan and Hofer, 2005).
Significant moderation of genetic expression as a function of environment quality for intelligence has been documented in US studies, such as Rowe et al. (1999), Guo and Stearns (2002), and Turkheimer et al. (2003). The latter study, for example, finds that for intelligence in young children in low SES environments heritability is only 10%, with a strong impact of the shared environment (58%). In high SES environments, the pattern is reversed, with heritability at 72% and the shared environment at 15%.
Findings of significant G × E effects for intelligence [such as Rowe et al. (1999) for adolescent verbal IQ and Turkheimer et al. (2003) for childhood IQ, using siblings and twins data] and for other outcomes, such as anti-social behavior [Caspi et al. (2002) using molecular-genetic methods] have captured the imagination of social scientists, as they appear to reaffirm a central role for the social environment as opposed to biological endowment or risk (Nisbett, 2009). In the case of intelligence, however, the systematic review by Tucker-Drob and Bates (2016) finds mixed support for the Scarr–Rowe hypothesis. Low SES was associated with attenuated genetic influences on intelligence in studies conducted in the United States [e.g., Schwartz (2015)], but the interaction was not significantly different from zero in non-US studies [conducted in Western Europe and Australia, e.g., Bates et al. (2016)]. Tucker-Drob and Bates (2016) reckon that G × E may be higher in the United States because of a more inegalitarian access to economic, health, and educational resources in the society. Even in U.S. studies, however, the size of the interaction is typically less than that found by Turkheimer et al. (2003). Bates et al. (2016) further discuss the contrast between US and non-US G × SES patterns, pointing out that the nature of the resources involved is unknown (p. 14): “We place resources in quotes, as it is unclear what it is among US families that creates SES-linked effects on heritability, and what it might be that in Australia decouples this factor or factors from SES.”
The mixed support for G × E in the case of IQ should be interpreted in the context of the high heritability of IQ in adulthood, which insures that there is little room for shared environmental effects, direct or interactive. It does not mean that the G × E mechanism is not at work for status-attainment outcomes other than IQ. Studies of G × E for educational attainment would seem a promising strategy for future research, as this adult outcome is characterized by a substantial shared environment component (Branigan et al., 2013), so there might be more room for environmental modulation of heritability than in the case of IQ.
The Scarr–Rowe hypothesis assumes that gene expression (heritability) increases monotonically as a function of social status. Part of the resonance of the hypothesis is that it is consistent with a broader pattern found throughout the natural world by which a more abundant (i.e., “higher quality”) environment is associated with greater heritability of a trait [e.g., Visscher et al. (2008)]. As Bouchard (2009) bluntly expresses the hypothesis in the context of IQ (p. 539): “It is well known that as the quality of the environment improves morphological traits express themselves more fully.” The simplest model is that heritability increases linearly with SES. A variant model is that starting from the most deprived environment heritability at first increases rapidly as conditions improve and then more slowly once environmental conditions are above a “humane threshold” bounding the normal species range (Scarr, 1992).
These two versions of the Scarr–Rowe hypothesis relating the effect of genes (heritability) to environmental resources are shown schematically in Figure 3B in contrast with the absence of G × E interaction (Figure 3A), which represents heritability as constant irrespective of environmental abundance. The simplest version assumes that heritability increases linearly with resources (Figure 3B, 1); a non-linear version assumes that the impact of genes increases rapidly with resources over the low range, but more slowly above a “humane threshold” of environmental abundance (Figure 3B, 2).
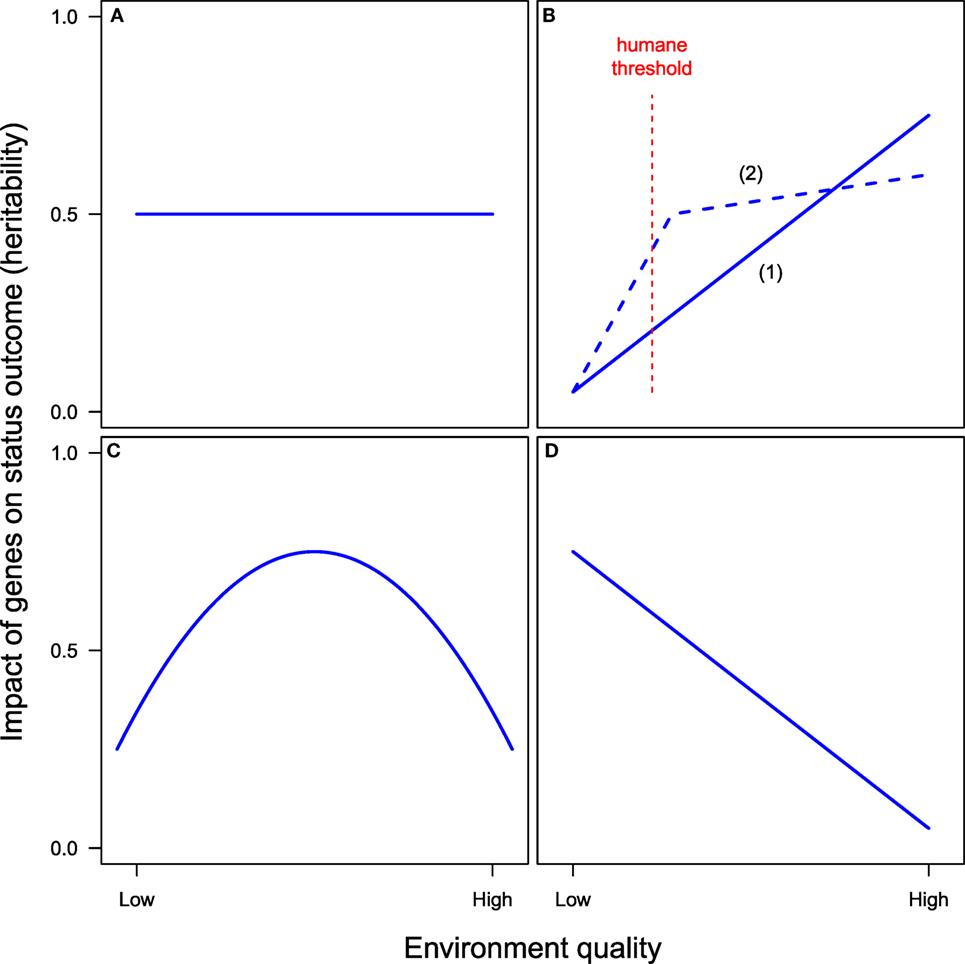
Figure 3. Alternative hypotheses on the interaction of genes and environment quality in affecting a status-related outcome. (A) No G × E interaction. (B) Two versions of the Scarr–Rowe hypothesis: (1) linear and (2) non-linear with heritability increase tapering off above a humane threshold. (C) Pareto hypothesis. (D) Saunders or reverse Scarr–Rowe hypothesis.
An alternative to the Scarr–Rowe hypothesis of monotonic increase in heritability with environmental quality is offered in the discussion of the circulation of elites by economist and sociologist Pareto (1909). Pareto, whose work largely predates modern understanding of genetics, argued that circumstances of rearing affect the potential of individuals to rise or fall in the social hierarchy in non-monotonic fashion. Individuals born to the lower stratum face such detrimental conditions that little upward mobility is possible, irrespective of their innate talents. Individuals born in the middle stratum have maximum opportunity, as environmental resources are not scarce enough to prevent the able from rising but also not sufficiently abundant to prevent downward mobility of those with limited talents. Individuals born in the upper, most privileged stratum, however, are protected by environmental abundance from downward mobility. Pareto’s view, thus, implies a curvilinear relationship between environmental resources and heritability that peaks at an intermediate level of resources (the “middle stratum”). Pareto’s conjecture is shown schematically in Figure 3C [see also Nielsen (2012)].
Still another hypothesis is suggested by the findings of Saunders (2010) that in modern Great Britain the only remaining deviation from perfect meritocratic mobility was a tendency for those born in the upper occupational classes to experience downward mobility at a lower rate than that expected by chance. Saunders’ discussion implies a positive relationship between the effect of social class of origin (i.e., the shared family environment) and achieved status, so that being born a member of the upper stratum has a greater effect on status achievement (in preventing downward mobility) than being born to the working class (in preventing upward mobility), implying a negative relationship between heritability of socioeconomic success and environmental resources, a “reverse Scarr–Rowe” effect, as it were. This possibility is shown in Figure 3D. The Saunders effect could also be viewed as a “degenerate” version of a Pareto conjecture, characterizing a society where equal opportunities have been extended to the lower classes (so that the ascending segment of the Pareto curve has flattened out) and the only remainder of ascription is a greater capacity of higher strata to maintain their offspring in a high status position relative to their abilities.
I am not aware of empirical research systematically comparing these four views of interactions between genes and environmental resources in socioeconomic achievement. Extensions of the SEM model that permit estimation of G × E interactions, including non-linear ones, are available and will permit future research to assess such conjectures (Purcell, 2002; Turkheimer et al., 2003; Bates et al., 2016).
The Scarr–Rowe conjecture was originally developed to predict differences in the relative roles of genes and the shared environment between family environments with different access to resources within a given society at a given point in time (Scarr-Salapatek, 1971; Rowe et al., 1999). It is of considerable interest from the point of view of sociological theory that the parameters of the ACE decomposition for a status-related outcome can be viewed as characterizing the opportunity structure of a society as a whole at a particular point in time, with heritability measuring opportunity to reach one’s potential and, conversely, the shared environment representing the weight of social ascription and closure. Adkins and Guo (2008) and Adkins and Vaisey (2009), for example, conjectured that as inequality in a society increases, heritability of status-related traits decreases. In that view, heritability becomes a macrosocial variable that can be compared across societies and historical periods and related to, and explained by, other structural characteristics of a society, such as its subsistence technology and the resulting degree of inequality in the distribution of resources (Adkins and Guo, 2008). Such theoretical developments are suggestive of how incorporating genetic considerations can invigorate sociological theory.
Heath et al. (1985) illustrates the way the ACE decomposition of the variance in status provides macrosocial indicators of fluidity of the social structure of a society. The authors compared resemblances in educational attainment between DZ and MZ twins in a large Norwegian sample to estimate the components of the ACE model for different birth cohorts, separately by sex. Comparing older (born 1915–1939) and younger (born 1950–1960) cohorts they found that, for males, the effect of genes on educational attainment increased (from 18 to 76%) and the role of the shared environment correspondingly decreased (from 68 to 9%). Heath et al. (1985) interpret these changes in the ACE parameters as resulting from liberal policy reforms in Norway that made access to education more open.
For females over the same period, the role of genes in schooling also increased (from 28 to 46%), and the role of the shared environment decreased (from 61 to 43%), but not as much as for males. The authors concluded that equality of opportunity, measured as the relative size of the genetic component, did not improve to the same extent for females as for males, so that the role of family privilege in educational attainment, measured as the shared environment component, remained correspondingly greater for females [see also Baker et al. (1996), Branigan et al. (2013)]. These interpretations are consistent with the theoretical discussions of Adkins and Guo (2008) and Adkins and Vaisey (2009).
6. Discussion
I have argued in this paper that incorporation of quantitative genetics concepts into Blau and Duncan-type models of status achievement provides new insights into processes governing socioeconomic success. This is because the distinction underlying the quantitative genetic model between the role of genetic sources (summarized as heritability a2) and the role of shared environmental sources (summarized as c2) have important substantive interpretations in the context of social stratification: heritability of a given status-related outcome in a specific social system measures the degree to which individuals achieve their native potential for that outcome, and thus the degree of opportunity in that society; the role of the shared environment c2 measures the strength of social reproduction of status in the society. From a policy point of view, c2 estimates the degree of equalization in the outcome that could be achieved by manipulating those environmental factors underlying the current variation in outcome-relevant environments (excluding from consideration environmental interventions that are potentially effective but do not contribute to current environmental variation).
To develop these points, I have emphasized the simplest version of the quantitative genetic model. As usual in the real world, things are more complicated; some of the complications to the basic ACE model that have implications for the topic at hand are briefly discussed in this section. The two main sources of complication for the ACE model are gene–environment interaction G × E, that was introduced earlier, and gene–environment correlation, denoted rGE (Purcell, 2002). rGE may be decomposed into three parts according to the mechanism involved. Passive correlation is due to an association between parental genes shared with a child and the environment the parents have created for the child; for example, a child (genetically) inheriting a talent for music is likely to have parents who are themselves talented and keep a household full of songs. Evocative rGE occurs if the parents, noting a musical disposition in the child, decide to pay for music lessons. Evocative correlation can be negative, as when parents feel they need to keep a stricter discipline for a rebellious child. Active rGE is generated when the child, growing up and moving away from parental influences, creates his or her own music-rich environment (Plomin et al., 1977).
In a target review article, Johnson et al. (2011a,b,c), with peer commentaries and authors’ rejoinder, have proposed three major critiques of the quantitative genetic model (summarized in the commentary by DeMoor and Boomsma): (1) heritability estimates are affected by serious empirical issues, including measurement error and low item endorsement frequency (e.g., dichotomous outcomes with very low or very high probability of occurrence). (2) Heritability estimates are biased if assumptions of the twin design (or other family-based design) are violated (as they often are); these include absence of assortative mating, and, prominently, absence of G × E and rGE. (3) Because they are affected by so many other factors, heritability estimates do not tell us much about the biology of personality and other behavioral traits. Behavioral scientists should focus instead on mechanisms of G × E and rGE (collectively referred to as the “interplay” of genes and the environment).
The first point is well taken, as it is perhaps too easy to forget that variance component estimates are statistics from a sample, just like a difference in group means or a regression coefficient. However, several of the peer reviews find the more sweeping critique of heritability and other variance components unwarranted. In a peer review entitled “Size Matters! Heritability is not Dichotomous.” Riemann, Kandler, and Bleidorn point out that even though some heritability is ubiquitous among behavioral traits, this finding does not exhaust the informative value of the concept. They write (p. 283, p. 282):
To dismiss differences in heritability estimates between personality measures is – at best – not helpful. Although it is comfortable for a divided scientific community to conclude that genes are about as much important for explaining individual differences as environmental factors are, this position ignores important and theoretically meaningful distinctions. […]
The study of these differences [in heritability estimates] between social settings (environments), age groups, sexes, or (sub)cultures is the means to study gene-environment transactions, a goal we share with Johnson and colleagues.”
The central thread of this paper has been to amplify the message of the second paragraph of the quotation, pointing out its particular relevance in the study of stratification and social mobility. In the latter research context, quantitative genetic estimates for status-related outcomes provide measures of central theoretical concepts, such as equality of opportunity and strength of social ascription, that can be meaningfully compared across the different social, institutional, and historical contexts in which populations are embedded.
Do recent advances in molecular genetics affect this perspective in a fundamental way? The answer seems to be no. The advent of inexpensive genotyping and development of statistical methodologies, such as genomic-relatedness-matrix restricted maximum likelihood (GREML) (also called GCTA, for genome-wide complex trait analysis) and collection of genome-wide DNA data on vast samples of unrelated individuals (GWAS) have allowed estimation of the heritability of human traits (but not the role of the shared environment) on the basis of assumptions quite different from those needed for family data. There is mounting GWAS evidence that status-related traits have substantial heritabilities [e.g., Davies et al. (2011) for IQ; Martin et al. (2011) for education] and that the correlations among these traits are attributable in part to genetic influences (Marioni et al., 2014). These findings are quite similar to those from family-based designs. Few replicable large size effects of specific genes have been found, suggesting that traits are affected by many different common alleles (i.e., alleles with frequencies ≥0.01). Chabris et al. (2015) have called this strong empirical pattern a “fourth law of behavior genetics” [in allusion to Turkheimer’s (2000) “three laws”] (Abstract): “A typical human behavioral trait is associated with very many genetic variants, each of which accounts for a very small percentage of the behavioral variability.” It may have been insufficiently appreciated in the social sciences that the fourth law constitutes a spectacular confirmation of the hypothesis of polygenic inheritance on which Fisher’s (1918) had based the variance decomposition model that serves as the foundation of quantitative genetics as well as the entire edifice of modern evolutionary theory.
As participants in GWAS studies are unrelated, the methodology cannot by principle estimate the effect of the shared family environment on a trait; the estimated environment combines shared and unshared effects. Family-based designs will continue to be needed to estimate the shared environment component c2 that I have argued is central for issues of social stratification and social mobility [see also Burt (2009)].
7. Conclusion
I have argued in this paper that integrating the ACE model of quantitative genetics into the traditional status-achievement model of sociology enhances understanding of the mechanisms of continuity of individual careers over the life course and the process of social mobility.
Notable insights based on the combined perspective, some of which were discussed in this review [items (2), (4), (5), and (6)], and some of which are documents in ongoing research by the author [items (1) and (3)], include the following. (1) Individual variation in most status-related outcomes, measures that either predict (such as Verbal IQ and High school GPA) or reflect adult status (such as Personal earnings or Occupation), included a substantial genetic component, often larger than that associated with the shared family environment (Roos and Nielsen, 2014). (2) In the case of Educational attainment, the role of the shared environment was greater for twins than for non-twin siblings. If this pattern is confirmed, it would indicate that many previous variance component estimates of the role of the shared environment in Educational attainment based on twins may be biased upwards in estimating the role of the family in Educational attainment of (vastly more numerous) ordinary siblings or singletons. (3) There was a general trend of declining heritability of successive status-related outcomes over the life course (Roos and Nielsen, 2014). (4) Correlations among status-related outcomes measured in adolescence (Verbal IQ, High school GPA, College plans) were for the most part or entirely due to partially overlapping genetic factors, while shared environmental effects on all three outcomes corresponded to a single common family environmental factor, perhaps representing “privilege.” (5) The model incorporating latent heterogeneity of genetic and environmental endowments provides a direct and consistent way of measuring equality of opportunities (as heritability) and an upper bound on the extent to which a trait could be affected by environmental manipulation within the range of existing environmental variation (as the shared environment component). The components of trait variance can then be used as measures of the system of stratification in comparisons across social groups and historical periods [e.g., Heath et al. (1985)]. (6) The notion of genes by environment interaction (G × E) allows natural representation within the combined model of substantive social science hypotheses concerning status-achievement over the life course, in particular the way in which resource abundance in the environment affects the heritability of a status-related outcome; the Scarr–Rowe, Pareto, and Saunders hypotheses predict different patterns of empirical estimates and are, therefore, testable in principle.
Author Contributions
The author confirms being the sole contributor of this work and approved it for publication.
Conflict of Interest Statement
The author declared no potential conflicts of interest with respect to the research, authorship, and/or publication of this article.
Funding
The author disclosed receipt of the following financial support for the research, authorship, and/or publication of this article: preparation of this manuscript was supported by Grant SES-1154990 from National Science Foundation USA. This paper summarizes previous research by the author and co-workers (published separately) using data from Add Health, a program project directed by Kathleen Mullan Harris and designed by J. Richard Udry, Peter S. Bearman, and Kathleen Mullan Harris at the University of North Carolina at Chapel Hill, and funded by grant P01-HD31921 from the Eunice Kennedy Shriver National Institute of Child Health and Human Development, with cooperative funding from 23 other federal agencies and foundations. Special acknowledgment is due Ronald R. Rindfuss and Barbara Entwisle for assistance in the original design. Information on how to obtain the Add Health data files is available on the Add Health website (http://www.cpc.unc.edu/addhealth). No direct support was received from grant P01-HD31921 for this analysis.
Footnotes
- ^Another common notation for the proportion of the variance in a trait due to genes is h2. In this discussion, I use the term heritability in a broad sense, as including additive as well as dominance and epistasis effects (Falconer and Mackay, 1996).
- ^Alternatively, Silventoinen et al., 2004 have suggested that the shared environmental component of educational attainment may be large in part because educational decisions are made in childhood when the c2 component of cognitive ability is still substantial. The larger shared environmental component of childhood IQ would then be “inherited” in educational attainment.
- ^As already mentioned, heritability and other variance components have no implication for the potential effectiveness of policies involving environmental interventions that are currently unknown, or that are known to be effective but insufficiently widespread to substantially contribute to population trait variance (Goldberger, 1979; Rowe, 1994).
- ^To identify model parameters, and insure a positive definite implied covariance matrix (Neale and Maes, 2004).
- ^This last transformation is analogous to an “oblique rotation” in factor analytic terms.
- ^An important caveat to interpreting correlations among genetic factors as resulting from pleiotropy is that sets of genes can also be correlated as a result of “population stratification,” or presence of subgroups in the populations with different distributions of genes. On this point, see Carey (2003).
- ^The proportions of the predicted total correlations between these traits due to genes were in fact slightly >1, reflecting the small negative path of C1 on GPA that entailed a slightly negative contribution of the shared environment to the total correlation.
- ^One can see this by imagining a counterfactual experiment (that could not be carried out in reality for ethical reasons) in which teachers covertly raise the GPA of a randomly chosen subset of a class, observing whether the treatment raises College plans in the experimental group.
References
Adkins, D. E., and Guo, G. (2008). Societal development and the shifting influence of the genome on status attainment. Res. Soc. Stratif. Mobil. 26, 235–255. doi: 10.1016/j.rssm.2008.06.001
Adkins, D. E., and Vaisey, S. (2009). Toward a unified stratification theory: structure, genome, and status across human societies. Sociol. Theory 27, 99–121. doi:10.1111/j.1467-9558.2009.01339.x
Ashenfelter, O., and Krueger, A. (1994). Estimates of the economic return to schooling from a new sample of twins. Am. Econ. Rev. 84, 1157–1173.
Baker, L. A., Treloar, S. A., Reynolds, C. A., Heath, A. C., and Martin, N. G. (1996). Genetics of educational attainment in Australian twins: sex differences and secular changes. Behav. Genet. 26, 89–102. doi:10.1007/BF02359887
Barrick, M. R., Mount, M. K., and Judge, T. A. (2001). Personality and performance at the beginning of the new millennium: what do we know and where do we go next? Int. J. Sel. Assess. 9, 9–30. doi:10.1111/1468-2389.00160
Bates, T. C., Hansell, N. K., Martin, N. G., and Wright, M. J. (2016). When does socioeconomic status (SES) moderate the heritability of IQ? No evidence for GxSES interaction for IQ in a representative sample of 1176 Australian adolescent twin pairs. Intelligence 56, 10–15. doi:10.1016/j.intell.2016.02.003
Becker, G. S. (1975). Human Capital, A Theoretical and Empirical Analysis with Special Reference to Education, 2nd Edn. New York: National Bureau of Economic Research.
Becker, G. S., and Tomes, N. (1979). An equilibrium theory of the distribution of income and intergenerational mobility. J. Polit. Econ. 87, 1153–1189. doi:10.1086/260831
Becker, G. S., and Tomes, N. (1986). Human capital and the rise and fall of families. J. Labor Econ. 4, S1–S39. doi:10.1086/298118
Behrman, J. R., Hrubec, Z., Taubman, P., and Wales, T. J. (1980). Socioeconomic Success: A Study of the Effects of Genetic Endowments, Family Environment, and Schooling. Amsterdam: North-Holland Pub. Co.
Behrman, J. R., and Rosenzweig, M. R. (1999). “Ability” biases in schooling returns and twins: a test and new estimates. Econ. Educ. Rev. 18, 159–167. doi:10.1016/S0272-7757(98)00033-8
Behrman, J. R., and Taubman, P. (1976). Intergenerational transmission of income and wealth. Am. Econ. Rev. 66, 436–440.
Behrman, J. R., and Taubman, P. (1989). Is schooling mostly in the genes? Nature-nurture decomposition using data on relatives. J. Polit. Econ. 97, 1425–1446. doi:10.1086/261661
Behrman, J. R., Taubman, P., and Wales, T. J. (1977). “Controlling for and measuring the effects of genetics and family environment in equations for schooling and labor market success,” in Kinometrics: Determinants of Socioeconomic Success within and between Families, ed. P. Taubman (Amsterdam: North-Holland), 35–96.
Benjamin, D. J., Cesarini, D., Chabris, C. F., Glaeser, E. L., Laibson, D. I., Gudnason, V., et al. (2012). The promises and pitfalls of genoeconomics. Ann. Rev. Econ. 4, 627–662. doi:10.1146/annurev-economics-080511-110939
Boomsma, D., Busjahn, A., and Peltonen, L. (2002). Classical twin studies and beyond. Nat. Rev. Genet. 3, 872–882. doi:10.1038/nrg932
Bouchard, T. J. Jr. (2009). Genetic influences on human intelligence (Spearman’s g): how much? Ann. Hum. Biol. 36, 527–544. doi:10.1080/03014460903103939
Bouchard, T. J. Jr., and McGue, M. (2003). Genetic and environmental influences on human psychological differences. J. Neurobiol. 54, 4–45. doi:10.1002/neu.10160
Bowles, S., and Gintis, H. (2002). The inheritance of inequality. J. Econ. Perspect. 16, 3–30. doi:10.1257/089533002760278686
Branigan, A. R., McCallum, K. J., and Freese, J. (2013). Variation in the heritability of educational attainment: an international meta-analysis. Soc. Forces 92, 109–140. doi:10.1093/sf/sot076
Burt, S. A. (2009). Rethinking environmental contributions to child and adolescent psychopathology: a meta-analysis of shared environmental influences. Psychol. Bull. 135, 608–637. doi:10.1037/a0015702
Calvin, C. M., Deary, I. J., Webbink, D., Smith, P., Fernandes, C., Lee, S. H., et al. (2012). Multivariate genetic analyses of cognition and academic achievement from two population samples of 174,000 and 166,000 school children. Behav. Genet. 42, 699–710. doi:10.1007/s10519-012-9549-7
Caspi, A., McClay, J., Moffitt, T. E., Mill, J., Martin, J., Craig, I. W., et al. (2002). Role of genotype in the cycle of violence in maltreated children. Science 297, 851–854. doi:10.1126/science.1072290
Chabris, C. F., Lee, J. J., Cesarini, D., Benjamin, D. J., and Laibson, D. I. (2015). The fourth law of behavior genetics. Curr. Dir. Psychol. Sci. 24, 304–312. doi:10.1177/0963721415580430
Conley, D. (2001). Capital for college: parental assets and postsecondary schooling. Sociol. Educ. 74, 59–72. doi:10.2307/2673145
Conley, D. (2008). “What do low (or high) sibling correlations tell us about social ascription?,” in Social Stratification, 3rd Edn, ed. D. B. Grusky (Boulder, CO: Westview Press), 596–609.
Davies, G., Tenesa, A., Payton, A., Yang, J., Harris, S. E., Liewald, D., et al. (2011). Genome-wide association studies establish that human intelligence is highly heritable and polygenic. Mol. Psychiatry 16, 996–1005. doi:10.1038/mp.2011.85
Davis, J. A. (1966). The campus as a frog pond: an application of the theory of relative deprivation to career decisions of college men. Am. J. Sociol. 71, 17–31. doi:10.1086/224257
Davis, J. A., and Smith, T. W. (1991). General Social Surveys, 1972-1991: Cumulative Codebook, Vol. 12. Chicago, IL: National Opinion Research Center (NORC).
Degler, C. N. (1991). In Search of Human Nature: The Decline and Revival of Darwinism in American Social Thought. Oxford: Oxford University Press.
Duffy, D. L., and Martin, N. G. (1994). Inferring the direction of causation in cross-sectional twin data: theoretical and empirical considerations. Genet. Epidemiol. 11, 483–502. doi:10.1002/gepi.1370110606
Duncan, O. D. (1968). Ability and achievement. Eugen. Q. 15, 1–11. doi:10.1080/19485565.1968.9987747
Duncan, O. D., Featherman, D. L., and Duncan, B. (1972). Socioeconomic Background and Achievement. New York: Seminar Press.
Eaves, L. J., Eysenck, H. J., and Martin, N. G. (1989). Genes, Culture, and Personality: An Empirical Approach. San Diego: Academic Press.
Eckland, B. K. (1967). Genetics and sociology: a reconsideration. Am. Sociol. Rev. 32, 173–194. doi:10.2307/2091810
Eckland, B. K. (1979). Genetic variance in the SES-IQ correlation. Sociol. Educ. 52, 191–196. doi:10.2307/2112324
Emde, R. N., and Hewitt, J. K. (eds) (2001). Infancy to Early Childhood: Genetic and Environmental Influences and Developmental Change. New York: Oxford University Press.
Falconer, D. S., and Mackay, T. F. C. (1996). Introduction to Quantitative Genetics, 4th Edn. Harlow, England: Pearson Education Limited.
Farkas, G. (2003). Cognitive skills and noncognitive traits and behaviors in stratification processes. Annu. Rev. Sociol. 29, 541–562. doi:10.1146/annurev.soc.29.010202.100023
Farkas, G. (2011). “Middle and high school skills, behaviors, attitudes, and curriculum enrollment, and their consequences,” in Whither Opportunity?, eds G. J. Duncan and R. J. Murnane (New York: Russell Sage Foundation), 71–89.
Fisher, R. A. (1918). The correlation between relatives on the supposition of Mendelian inheritance. Trans. R. Soc. Edinb. 52(Part 2), 399–433. doi:10.1017/S0080456800012163
Freese, J. (2008). Genetics and the social science explanation of individual outcomes. Am. J. Sociol. 114(Suppl.), S1–S35. doi:10.1086/592208
Goldberger, A. S. (1978). The Genetic determination of income: comment. Am. Econ. Rev. 68, 960–969; [Comment on Taubman 1976 “Determinants”].
Guo, G., and Stearns, E. (2002). The social influences on the realization of genetic potential for intellectual development. Soc. Forces 80, 881–910. doi:10.1353/sof.2002.0007
Harris, K. M. (2009). The National Longitudinal Study of Adolescent Health (Add Health), Waves I & II, 1994-1996; Wave III, 2001-2002; Wave IV, 2007-2009 [Machine-Readable Data File and Documentation]. Chapel Hill, NC: Carolina Population Center, University of North Carolina at Chapel Hill.
Harris, K. M., Halpern, C. T., Smolen, A., and Haberstick, B. C. (2006). The national longitudinal study of adolescent health (add health) twin data. Twin Res. Hum. Genet. 9, 988–997. doi:10.1375/twin.9.6.988
Heath, A. C., Berg, K., Eaves, L. J., Solaas, M. H., Corey, L. A., Sundet, J., et al. (1985). Education policy and the heritability of educational attainment. Nature 314, 734–736. doi:10.1038/314734a0
Heath, A. C., Kessler, R. C., Neale, M. C., Hewitt, J. K., Eaves, L. J., and Kendler, K. S. (1993). Testing hypotheses about direction of causation using cross-sectional family data. Behav. Genet. 23, 29–50. doi:10.1007/BF01067552
Heckman, J. J., and Rubinstein, Y. (2001). The importance of noncognitive skills: lessons from the GED testing program. Am. Econ. Rev. 91, 145–149. doi:10.1257/aer.91.2.145
Heckman, J. J., Stixrud, J., and Urzua, S. (2006). The effects of cognitive and noncognitive abilities on labor market outcomes and social behavior. J. Labor Econ. 24, 411–482. doi:10.1086/504455
Herrnstein, R. J., and Murray, C. (1994). The Bell Curve: Intelligence and Class Structure in American Life. New York: Free Press.
Jencks, C. (1979). Who Gets Ahead? The Determinants of Economic Success in America. New York: Basic Books.
Jencks, C., Smith, M., Acland, H., Bane, M. J., Cohen, D., Gintis, H., et al. (1972). Inequality: A Reassessment of the Effect of Family and Schooling in America. New York: Basic Books.
Jencks, C., and Tach, L. (2006). “Would equal opportunity mean more mobility?,” in Mobility and Inequality: Frontiers of Research from Sociology and Economics, eds M. Stephen, G. David, and F. Gary (Stanford, CA: Stanford University Press), 23–58.
Jensen, A. R. (1969). How much can we boost IQ and scholastic achievement. Harv. Educ. Rev. 39, 1–123. doi:10.17763/haer.39.1.l3u15956627424k7
Jinks, J. L., and Fulker, D. W. (1970). Comparison of the biometrical genetical, MAVA, and classical approaches to the analysis of human behavior. Psychol. Bull. 73, 311–349. doi:10.1037/h0029135
Johnson, W., Brett, C. E., and Deary, I. J. (2010). The pivotal role of education in the association between ability and social class attainment: a look across three generations. Intelligence 38, 55. doi:10.1016/j.intell.2009.11.008
Johnson, W., McGue, M., and Iacono, W. G. (2006). Genetic and environmental influences on academic achievement trajectories during adolescence. Dev. Psychol. 42, 514–532. doi:10.1037/0012-1649.42.3.514
Johnson, W., Penke, L., and Spinath, F. M. (2011a). Heritability in the era of molecular genetics: some thoughts for understanding genetic influences on behavioral traits. Eur. J. Pers. 25, 254–266. doi:10.1002/per.836. [Target article]
Johnson, W., Penke, L., and Spinath, F. M. (2011b). [Peer reviews]. Eur. J. Pers. 25, 267–286. doi:10.1002/per.834
Johnson, W., Penke, L., and Spinath, F. M. (2011c). Understanding heritability: what it is and what it is not. Eur. J. Pers. 25, 287–294. doi:10.1002/per.835
Jonsson, J. O., Grusky, D. B., Di Carlo, M., Pollak, R., and Brinton, M. C. (2009). Microclass mobility: social reproduction in four countries. Am. J. Sociol. 114, 977–1036. doi:10.1086/596566
Judge, T. A., Higgins, C. A., Thoresen, C. J., and Barrick, M. R. (1999). The big five personality traits, general mental ability, and career success across the life span. Pers. Psychol. 52, 621–652. doi:10.1111/j.1744-6570.1999.tb00174.x
Kohler, H. P., Behrman, J. R., and Schnittker, J. (2011). Social science methods for twins data: integrating causality, endowments, and heritability. Biodemography Soc. Biol. 57, 88–141. doi:10.1080/19485565.2011.580619
Lipset, S. M., and Bendix, R. (1959). Social Mobility in Industrial Society. New Brunswick, NJ: Transaction Publishers.
Loehlin, J. (1996). The Cholesky approach: a cautionary note. Behav. Genet. 26, 65–69. doi:10.1007/BF02361160
Marioni, R. E., Davies, G., Hayward, C., Liewald, D., Kerr, S. M., Campbell, A., et al. (2014). Molecular genetic contributions to socioeconomic status and intelligence. Intelligence 44, 26–32. doi:10.1016/j.intell.2014.02.006
Marks, G. N. (2014). Education, Social Background and Cognitive Ability: The Decline of the Social. London, New York: Routledge, Taylor & Francis Group.
Martin, N. G., and Eaves, L. J. (1977). The genetical analysis of covariance structure. Heredity 38, 79–95. doi:10.1038/hdy.1977.9
Martin, N. W., Medland, S. E., Verweij, K. J., Lee, S. H., Nyholt, D. R., Madden, P. A., et al. (2011). Educational attainment: a genome wide association study in 9538 Australians. PLoS ONE 6:e20128. doi:10.1371/journal.pone.0020128
Miller, P., Mulvey, C., and Martin, N. (2006). The return to schooling: estimates from a sample of young Australian twins. Lab. Econ. 13, 571–587. doi:10.1016/j.labeco.2004.10.008
Neale, M. C., Boker, S. M., Xie, G., and Maes, H. (2006). Mx: Statistical Modeling, 7th Edn. Richmond: Department of Psychiatry, Virginia Commonwealth University.
Neale, M. C., and Maes, H. M. (2004). Methodology for Genetic Studies of Twins and Families, 2nd Edn. Dordrecht, Netherlands: Kluwer Academic Press.
Nielsen, F. (2006). Achievement and ascription in educational attainment: genetic and environmental influences on adolescent schooling. Soc. Forces 85, 193–216. doi:10.1353/sof.2006.0135
Nielsen, F. (2008). The nature of social reproduction: two paradigms of social mobility. Sociologica 2, 1–35. doi:10.2383/28771. Symposium/Social Sciences and Natural Sciences – What Connection?, edited by Mario Lucchini and Maurizio Pisati.
Nielsen, F. (2012). “Talents and obstacles: Pareto’s morphological schema and contemporary social stratification research,” in Beyond Disciplinary Boundaries: Essays on Pareto, eds J. V. Femia and A. J. Marshall (Burlington, VT: Ashgate), 21–46.
Nielsen, F., and Roos, J. M. (2015). Genetics of educational attainment and the persistence of privilege at the turn of the 21st century. Soc. Forces 94, 535–561. doi:10.1093/sf/sov080
Nisbett, R. E. (2009). Intelligence and How To Get It: Why Schools and Cultures Count. New York: Norton.
Petrill, S. A., and Wilkerson, B. (2000). Intelligence and achievement: a behavioral genetic perspective. Educ. Psychol. Rev. 12, 185–199. doi:10.1023/A:1009023415516
Plomin, R., DeFries, J. C., and Loehlin, J. C. (1977). Genotype-environment interaction and correlation in the analysis of human behavior. Psychol. Bull. 1977, 309–322. doi:10.1037/0033-2909.84.2.309
Purcell, S. (2002). Variance components models for gene-environment interaction in twin analysis. Twin Res. 5, 554–571. doi:10.1375/136905202762342026
Roos, J. M., and Nielsen, F. (2014). Genetic and environmental influences on status-related outcomes in the early lifecourse Presented at the Integrating Genetics and the Social Sciences Conference, Boulder, CO.
Rowe, D. C. (1994). The Limits of Family Influence: Genes, Experience, and Behavior. New York: Guilford.
Rowe, D. C., Jacobson, K. C., and Van den Oord, E. J. (1999). Genetic and environmental influences on vocabulary IQ: parental education level as moderator. Child Dev. 70, 1151–1162. doi:10.1111/1467-8624.00084
Scarr, S. (1992). Developmental theories for the 1990s: Development and individual differences. Child Development 63, 1–19.
Scarr, S., and Weinberg, R. A. (1978). The influence of ‘family background’ on intellectual attainment. Am. Sociol. Rev. 43, 674–692. doi:10.2307/2094543
Schwartz, J. A. (2015). Socioeconomic status as a moderator of the genetic and shared environmental influence on verbal IQ: a multilevel behavioral genetic approach. Intelligence 52, 80–89. doi:10.1016/j.intell.2015.07.004
Shanahan, M. J., Scott M., and Hoffer, S. M. (2005). Social Context in Gene-Environment Interactions: Retrospect and Prospect. J. Gerontol. B. Psychol. Sci. Soc. Sci. 60, 65–76. doi:10.1093/geronb/60.Special_Issue_1.65
Silventoinen, K., Krueger, R. F., Bouchard, T. J. Jr., Kaprio, J., and McGue, M. (2004). Heritability of body height and educational attainment in an international context: comparison of adult twins in Minnesota and Finland. Am. J. Hum. Biol. 16, 544–555. doi:10.1002/ajhb.20060
Strenze, T. (2007). Intelligence and socioeconomic success: a meta-analytic review of longitudinal research. Intelligence 35, 401–426. doi:10.1016/j.intell.2006.09.004
Taubman, P. (1976a). The determinants of earnings: genetics, family and other environments: a study of white male twins. Am. Econ. Rev. 66, 858–870.
Taubman, P. (1976b). Earnings, education, genetics, and environment. J. Hum. Resour. 11, 447–461. doi:10.2307/145426
Taubman, P. (1978). What we learn from estimating the genetic contribution to inequality in earnings: reply. Am. Econ. Rev. 68, 970–976.
Tucker-Drob, E., and Bates, T. C. (2016). Large cross-national differences in gene x socioeconomic status interaction on intelligence. Psychol. Sci. 27, 138–149. doi:10.1177/0956797615612727
Turkheimer, E. (2000). Three laws of behavior genetics and what they mean. Curr. Dir. Psychol. Sci. 9, 160–164. doi:10.1111/1467-8721.00084
Turkheimer, E., Haley, A., Waldron, M., Onofrio, B. D., and Gottesman, I. I. (2003). Socioeconomic status modifies heritability of IQ in young children. Psychol. Sci. 14, 623–628. doi:10.1046/j.0956-7976.2003.psci_1475.x
Visscher, P. M., Hill, W. G., and Wray, N. R. (2008). Heritability in the genomics era: concepts and misconceptions. Nat. Rev. Genet. 9, 255–266. doi:10.1038/nrg2322
Wright, S. (1920). The relative importance of heredity and environment in determining the piebald pattern of guinea-pigs. Proc. Natl. Acad. Sci. U.S.A. 6, 320–332. doi:10.1073/pnas.6.6.320
Keywords: status achievement, social mobility, social stratification, nature and nurture, behavior genetics
Citation: Nielsen F (2016) The Status-Achievement Process: Insights from Genetics. Front. Sociol. 1:9. doi: 10.3389/fsoc.2016.00009
Received: 15 April 2016; Accepted: 27 June 2016;
Published: 14 July 2016
Edited by:
Susanne Huber, University of Vienna, AustriaReviewed by:
Joanna Schug, College of William and Mary, USADaniel E. Adkins, Virginia Commonwealth University, USA
Copyright: © 2016 Nielsen. This is an open-access article distributed under the terms of the Creative Commons Attribution License (CC BY). The use, distribution or reproduction in other forums is permitted, provided the original author(s) or licensor are credited and that the original publication in this journal is cited, in accordance with accepted academic practice. No use, distribution or reproduction is permitted which does not comply with these terms.
*Correspondence: François Nielsen, ZnJhbmNvaXNfbmllbHNlbkB1bmMuZWR1