- Retired, Koblenz, Germany
The paper shows that in an artificial society lawlike rules emerge “as a result of individual action but without being designed by any individual” agents (Hayek, 1944, p. 288) and discusses earlier literature on the topic. The first example that this paper uses is an artificial society of car drivers moving between their homes and their working places on streets with two lanes crossing each other at right angles. Car drivers start using the left or right lane of the street at random and continue to use the same side of the street until they are stopped by an oncoming car. In this occasion, one of them decides to change to the other side of the street, taking into account which side of the street is used by the locally visible majority. This very simple behavior usually results in a society-wide applied rule: always using the same side of the street. How long it takes for all car drivers to abide by the emerged rule (and how many, if not all, apply the rule) depends on the density of traffic and the range of vision of the car drivers as well as on the distance the cars went. A second example of emerging rule-consistent behavior discussed in the paper and analyzed with different mathematical and computational methods is derived from a model of the emergence of aggression aversion extended to a model of the emergence of a rule against theft and of a rule in favor of almsgiving. In this model, agents receive comments on their theft and alms related actions and form a normative board that controls their propensity to act with respect to theft, prosecuting and punishing theft, asking for and granting alms. This model shows an emerging anti-theft norm whose salience among the agents increases in a rapid transition after a fairly long initial phase during which theft is more or less tolerated.
1. Introduction
The aim of this paper is to show that lawlike rules can emerge without the intervention of a legislator, i.e., the answer to the title question is a cautious yes, as the two examples below will show that at least in these two cases lawlike rules do emerge in an artificial society. This does, of course, not mean that the respective rules in force in real human societies emerged in a similar process, but the literature is full of hints at such processes of emergence although historical sources often point to the contrary, perhaps due to the fact that historical records were written by those political leaders (or on behalf of them) who tried to enforce a 100% obedience of their subjects once the latter had become their subjects.
The rest of this paper is organized as follows. Section 2 discusses earlier literature on the emergence of law and norms. Section 3 presents an example of a lawlike rule—namely the rule “always using the same side of the street,” as this is, for instance, stated in the Geneva Convention on Road Traffic (United Nations, 1949, Art. 6). In the simulation, this rule emerges from the model “as a result of individual action but without being designed by any individual” agents (Hayek, 1944, p. 288). Section 3 analyzes the results of a number of simulation runs of this model in which from the point of view of the isolated individuals it does not really matter which side of the street is used when they are able to maneuver out of the way of each other. Section 4 extends the discussion of simulation models of this type when following a specific rule is of interest for at least one of the isolated individuals in question, for instance, when the rule is forbidding theft. Subsection 4.7 takes a first step at validating this model, and Section 5 concludes.
2. Discussion
2.1. Approaches to the Origin of Law
The origin of law used to be assigned to mythological or religious legislators (νoμo, lawgivers), for instance, in Platos’s Nóμoι (Plato, 1926) where Clinias and Megillos name the gods Zeus and Apollo, respectively, as the primordial lawgivers, the same applies to the Jewish and Islamic tradition (where the laws are also revealed by Jahwe or Allah, respectively) and until the eighteenth century to a “Law of Nature” (Hobbes, 1965, ch. XIV, emphasis in the original) which states “a generall rule of Reason, That every man, ought to endeavor Peace, as farre as he has hope of obtaining it; [… which] containeth the first and Fundamental Law of Nature; which is to seek Peace, and follow it.” For a game-theoretic analysis of this statement of Hobbes’, see Piirimäe (2006). Kant, in his explanations of the categorical imperative “So act as if the maxim of your action were to become through your will a universal law of nature.” (Kant, 1785, p. 38 (Ak4:421), emphasis in the original), gives the example of a person borrowing money from another person thinking to himself “If I believe myself to be in pecuniary distress, then I will borrow money and promise to pay it back, although I know this will never happen.” and then argues that if such a maxim turned into a universal law, this “would make impossible the promise and the end one might have in making it, since no one would believe that anything has been promised him, but rather would laugh about every such utterance as vain pretense.” (Kant, 1785, p. 39 (Ak4:422)) For Kant, the ability to recognize this contradiction lies in “the moral law within me” (Kant, 1785, p. 203 (A289), emphasis in the original). So still here the norm to pay back what one has borrowed is derived from an axiom in the sense of Coleman’s remark “norms may be taken as axiomatic by many sociologists, but for others they constitute an unacceptable deus ex machina—a concept brought in at the macrosocial level to explain social behavior, yet itself left unexplained.” [(Coleman, 1990) p. 242].
But already Kant’s example shows that the origin of law could be the communication between human actors having different opinions on how to behave or taking offense at each other’s actions, a motive which can also be found in (Durkheim, 1895, p. 127) who proposed “sociological phenomena [that] penetrate into us by force or at the very least by bearing down more or less heavily upon us.” In a way, Durkheim anticipated the two arrows—downward and upward—in Coleman’s often-used illustration of the micro-macro link (Coleman, 1990, p. 8, 10, 401, 478, 643, 646) representation of the process by which human actions are determined by and at the same time determine their social environment when he formulates “By aggregating together, by interpenetrating, by fusing together, individuals give birth to a being … of a new kind” (Durkheim, 1895, p. 129). To avoid what fifty years later was called reductionism, Durkheim states that “there is between psychology and sociology the same break in continuiity as there is between biology and the physical and chemical sciences.” (Durkheim, 1895, p. 129) Hence, he defines society and all social facts, such as the law, as emergent phenomena that are not reducible to its interacting components.
2.2. Approaches to the Emergence of Norms
The emergence of norms has been a topic of research in sociology, psychology, and cognitive science for decades, at least back to Coleman’s diagnosis of a “neglect” of the question “why and how norms come into existence.” Coleman discusses “two simple conditions, taken together, … [as] sufficient for the emergence of norms” one of which “is a condition under which a demand for effective norms will arise” whereas the other “is a condition under which that demand will be satisfied” (Coleman, 1990, p. 241). The first condition is given when “persons who initiate or help maintain a norm see themselves as benefiting from its being observed or harmed by its being violated.” (Coleman, 1990, p. 242). To illustrate this in a somewhat anecdotical manner, think of a seminar in a German university of the late 1960s and compare it to a seminar in the late 1980s1: In the late 1960s, no student took offense at other students smoking during classes, i.e., non-smokers did not feel that they benefited from non-smoking or were harmed by smoking, whereas two decades later at least some of the non-smokers in university classes had learnt that passive smoking is harmful for their health, felt that they were harmed by smoking colleagues, and asked them to stop smoking in classes, or, in Coleman’s words, thought they could “claim a right to apply sanctions and recognize the right of others holding the norm to do so” (Coleman, 1990, p. 243) although the norm of non-smoking in university classes was only emerging but not yet effective [in the sense of Coleman’s second condition, “the realization of effective norms” (Coleman, 1990, p. 266)]. Coleman uses a game-theoretic model to explain how a norm emerges (Coleman, 1990, p. 256) and becomes effective (Coleman, 1990, p. 271ff).
Bicchieri and Muldoon (2014), p. 4, suppose that “norms of honesty, loyalty, reciprocity and promise keeping, to name but a few cooperative norms, are crucial to the smooth functioning of social groups. One hypothesis is that they emerge in small, close-knit groups in which people have ongoing interactions with each other” and are in line with Kant’s example cited above [which, by the way, was also used in Axelrod (1986), pp. 1108]. And in line with (Opp, 2002) they assume that norms “are the unintentional and unplanned outcome of human interaction.” (Bicchieri and Muldoon, 2014, p. 7). This is also true for the emergence of descriptive norms (Muldoon et al., 2014) which is exemplified with the scenario of a standing ovation in which people participate partly on their own and partly imitating the actions of others, but in terms of Coleman (1990), p. 242, it is questionable whether in the case of descriptive norms there are persons who “see themselves as benefiting from its being observed or harmed by its being violated”—to stand up or to keep sitting are neither benefits nor disadvantages of the same importance as being allowed to smoke, being prevented from smoking, enjoying fresh air or being exposed to smoke as in the (Opp, 2002) example where a no-smoking norms is certainly a proscripting norm.
Ullmann-Margalit (1977), p. 9, “basic argument is that certain types of norms are solutions to problems posed by certain interaction situations. These problems inhere in the structure—in the game-theoretical sense of structure—of the situations concerned, pertaining to some or all of the interacting participants,” and distinguishes among “three types of paradigmatic interaction situations,” namely Prisoners’ Dilemma, Co-ordination and Inequality [or “norms of partiality” (Coleman, 1990, p. 249, fn. 3) situations, which both Ullmann-Margalit and Coleman describe “using simple payoff matrices from the theory of games” (Coleman, 1990, p. 249)]. Hence, the traditional approach to the emergence of norms adopted by a long list of researchers from Schelling (1966) to Bicchieri (2006) has been “a game-theoretic account of norms and conventions according to which a norm is broadly defined as a Nash equilibrium” (Bicchieri and Muldoon, 2014, p. 31, their emphasis).
But game-theoretic models do not explain what happens within the actors when they play the game. “Modeling an explicit recognition of norms … calls for cognitively more complex agents.” (Neumann, 2014, p. 56, Neumann’s emphasis). An architecture of this kind of agents was designed in Campenní et al. (2014) and implemented in Lotzmann et al. (2014), see also Elsenbroich and Gilbert (2014), chapter 9. A slightly different approach was used (and will be reused in this paper) in Nardin et al. (2016). In terms of Opp (2002), the two examples are about norms emerging by the unintended consequences of human action, and in Bicchieri’s sense (Muldoon et al., 2014, p. 3) the emerging norms are “social or moral norms,” not “descriptive norms.”
3. Always Use the Same Side of the Street!
Article 9 line 1, first sentence, of the Geneva Convention on Road Traffic (United Nations, 1949, Art. 9) reads “All vehicular traffic proceeding in the same direction on any road shall keep to the same side of the road, which shall be uniform in each country for all roads.” This is a rule of law set into force by all states, and it is an interesting question under which conditions the same rule could have emerged without any legislation or international treaty. To find out whether rather simple agents in an artificial society can develop such a rule by which all or a large majority always abide we design a model of a network of streets populated by a number of cars whose driver agents move from their homes to their working places, starting randomly either as right-hand drivers or as left-hand drivers. They continue to use the same side of the street until they are stopped by an oncoming car. In this occasion, one of them decides to change to the other side of the street, taking into account what they know about the behavior of the other car drivers.2 Figure 1 shows a screenshot from such a simulation run.
In this simulation run, 129 out of 200 drivers use the right-hand lane, 71 use the left-hand lane. Due to the low density of traffic (because the distance between home and working place is rather small—30 units, where the distance between two crossroads is only 34—and because the vision range is also low) both kinds of drivers have a chance to arrive at their destinations without conflicts; and in case of conflict, they solve it locally such that in some parts of the network there is majority of left-hand drivers whereas in other parts there is a majority of right-hand drivers, such that there is no need for a network-wide rule. Alternative simulation runs with longer distances between home and working place and/or with a wider vision range show an early emergence of a unified rule by which practically all drivers abide long before they reach their destinations.
To find out what the dependence of the speed and success of the emergence of the rule on density and vision range is, we conduct a high number of simulation runs with varying parameters. The following parameters are varied:
• decision rule: three decision rules are examined, a simple one where the probability to change to the other side of the street depends on the overall majority (which in real life is perhaps difficult to know), on the local majority, or on criticism received from other car drivers in cases of such conflicts in the past, wherever they received or observed such critical remarks.3
• locality: this parameter is not relevant in the global majority case, otherwise car drivers can observe a neighborhood of 12, 24, 36, or 48 patches.
• coupling: this parameter is only relevant in the global majority case, it takes the values κ = 0.6 or κ = 2.5, the probability to change being p = ν exp(κx) where ν = 0.1 and x is the perceived majority, normalized to the interval [–1, 1] such that –1 means “all others use the other side of the street” and 1 means “all others use the same side of the street” (for the background of this formula see Weidlich and Haag (1983)).
• commuting distance: this is a measure for the density of traffic, the values used are 20 and 50.
This leads to 3 × 4 × 2 × 2 parameter combinations, including the parameters that might be irrelevant. The results of this experiment with 240 runs in total (five runs per parameter combination with different random number generator seed) can be seen in Table 1. The output parameter is the time of the last decision of a car driver to change sides; the simulation is stopped 20 time steps after this decision (in most cases, see below, all cars use the same side of the street such that no further conflicts can be expected).
Nearly all simulation runs led to a final situation where all car drivers used the same side of the street, only one run (global majority, low κ, the worst performing parameter combination) ended after 1753 time steps with 152 cars using the right-hand side and 48 using the left-hand side, and four runs ended with minorities of 5, 3 and two times 1, respectively, such that in 235 out of 240 the runs ended with all cars using the same side.
The results show clearly that the variant with a homogeneous population—all individual car drivers decide according to identical global information—also leads to the emergence of coordinated behavior, but this takes longer than with the other two decision rules. It seems that observation alone (local majority decision rule) performs even better than the more complicated rule where car drivers only take into account the norm invocation exchanges on the occasion of conflicts. But this is easily explained by the fact that not all conflicts will necessarily lead to long discussions such that the adaptation may take longer than in the case where at the time of conflict all behavior in the neighborhood is evaluated, not only behavior that led to critical remarks of the opponent.
4. Thou Shalt not Steal!
4.1. Game-Theoretic and Bioeconomic Predecessor Models
Although it seems that the original meaning of the eighth commandment (Exodus 20:15) is against kidnapping, it is nowadays usually understood as a rule against theft (this one is commanded separately in Leviticus 19:11). For the purpose of this example, we construct an artificial society in which agents occupy patches of land containing resources which they use for their living but which can also be given away to others or taken away (stolen) by others. Models of this kind have been published in the past, at least back to Martinez Coll (1986) in his “bioeconomic model of Hobbes” “state of nature” with a population of individuals that are homogeneous with the exception of applying one of three strategies, dove, hawk, and law-abider, the dove giving always way to an intruder, the hawk always trying to steal another individual’s property but fiercely defending its own, and the law-abider never trying to steal another individual’s property (just like a dove) but fiercely defending its own (just like a hawk). In Martinez Coll’s model, there is a kind of evolution of strategies: applying each of these strategies has certain costs and benefits, and the positive or negative difference between these decides which is the evolutionary success of the respective strategy, such that over the generations the optimal strategy wins—which is, of course, the law-abider’s, whose strategy is finally adopted by all individuals, and the end of the story is eternal piece without Hobbes’ Leviathan interfering.
Martinez Coll’s model is an extension of the chicken or hawk–dove game first described by Maynard Smith and Price (1973) and extended in Maynard Smith (1986). Unlike its predecessors it is about three instead of two strategies in the former and the mixture between the two strategies in the latter. And Martinez Coll’s model also goes beyond its predecessors as it develops the game-theoretic model into a system of three nonlinear (cubic) differential equations which has also been used in biology (see Eigen and Schuster (1979) who call this model a model of selection under constrained growth with nonlinear growing rates). Thus, it cannot only define the final equilibrium but also the dynamics leading to this equilibrium and the time needed to reduce the losing strategies to below, say, 1% (as the model is in real numbers of individual instead of integer numbers, the equilibrium is only reached for t → ∞). For a reconstruction, how the eighth commandment could have come into force without Moses dealing with God on Mount Sinai, Martinez Coll’s model is still too simple as it assumes that only the mentioned strategies with certain constant parameters determine the future of the model. Among humans the estimated costs and benefits of giving away, stealing and defending are most heterogeneous and depend on the individual histories of the individuals offending each other and on the reaction of the neighborhood observing their behavior. Even extant agent-based models elaborating on Martinez Coll’s model—(Gilbert and Troitzsch, 2005, pp. 119–122; 132)—do not take the inherent inhomogeneity of human decision making into account.
Unlike the example in section 3, the case of theft does matter to both parties: whereas a conflict between right-hand drivers and left-hand drivers can be solved without any damage for both of them (if they drive carefully, stop and negotiate with one of them swerving they might lose a few seconds of their time but not more), a conflict between thieves and their victims always leaves at least one of them in a deplorable situation: if the theft is success, the victim has lost part of his or her property, and if it is unsuccessful then the thief has not achieved his or her goal, and in the case that the prospective victim was a potential thief (or here: hawk) as well, both will have fought and will have been violated. Whereas in a traffic system with limited density, a central rule is dispensable, in a world with thievery a central rule is desirable. The example is to show whether even under relaxed conditions with respect to homogeneity an anti-theft rule emerges without the interference of a central authority.
4.2. An Event-Oriented Agent-Based Model of Emerging Norms
In this section, we embed the notion of theft into a broader context of property rights, wealth and poverty, endowing agents with the capability to steal and to defend themselves against theft as well as the propensity to ask for alms and to grant (or refuse to grant) alms, integrating two theft-related rules with rules about alms-giving and helping the poor, thus introducing two pairs of possible norms:
• Thou shalt not steal (Exodus 20:15)
• It seems unlawful for a man to appropriate an external thing to himself (Ambrose of Milan, discussed by Thomas Aquinas, Summa Theologica), or shorter and more graphic: Property is robbery (Proudhon, 1876, p. 315)4
• If any would not work, neither should he eat. (2 Thess 3:10)
• Sell that ye have, and give alms (Luke 12:33)
These rules will only be the emergent outcome of a process which starts when initially some individuals take offense at being the victim of theft, at finding that others are richer than themselves, at being asked for alms or at not receiving any alms when they are needy. In any of these cases, they will communicate that they feel offended, not only addressing the immediate neighbor who offended but also others within some distance. With this process we follow earlier research on norm emergence and norm innovation (Lotzmann et al., 2013; Troitzsch, 2015, 2016); details of the model follow, but, in this paper, it will not be possible to discuss all the consequences of the rules implemented in this model.
Hence the simulation model described here foresees the following actions for its agents:
• stealing and robbing,
• defending oneself against theft and robbery,
• getting stolen property back,
• asking for alms,
• granting alms,
• refusing alms,
• offering payment for services instead of granting alms,
• delivering services for offered payment.
The model implementing these ideas is an event-oriented agent-based simulation model in NetLogo (Wilensky, 1999; Railsback et al., 2017).5 Time is structured in days. The simulation starts with spreading agents over the surface of the virtual world and endowing them with an initial wealth. The initial wealth follows a beta distribution of the first kind with parameters p = 2 and q = 6 transformed from the interval (0,1) to the (more or less arbitrary) interval between 200 and 2,000 currency units, such that the mode, median, mean, and SD are m = 500, q0.5 ≈ 609, μ = 650, and .
Every simulated day starts with all agents deciding whether and when they are going to take which action—robbing or asking for alms. The time when agents will perform the selected action is then put into an event-list from which all these events are executed in chronological order. Which of these two actions an agent takes depends on its propensities which in turn depend on two calculations, one of which takes the advantages and disadvantages of an action into account whereas the other depends on the salience of each of the emerging norms it has internalized.
4.3. The Immergence of Norms
If an agent A receives a message which signalizes that the sender (agent B) approved the behavior of another agent (agent A or a third agent C) or took offense at it, this message is stored in one of several slots in the memory of agent A. Following (Nardin et al., 2016; Troitzsch, 2016) these slots are
• C and V which count messages sent by agent A to itself when it complied with the respective (emerging) norm or violated it, respectively,
• Oc and Ov which count messages received by agent A from an agent B directed to another agent C which complied with the respective norm or violated it, respectively,
• P and S which count messages from agent B explicitly reproving agent A, where P counts only those reprovals which contain an explicit punishment,
• Eo and Ev which count messages from agent B to agent A explicitly invocating the respective norm because of an observed compliance or violation, respectively,
In a way, these messages resemble Emile Durkheim’s “sociological phenomena [that] penetrate into us by force or at the very least by bearing down more or less heavily upon us” (Durkheim, 1895, p. 127).
Whenever an agent needs to evaluate its propensity to take an action it takes into account how often it received messages recommending or advising against this action. This is done by applying a formula calculating the salience which the emerging norm has for this agent.
where the capital letters have the meaning explained above and wc, wo, wnpv, wp, ws, and we are the weights for the six factors (“norm cues”) derived from Cialdini et al. (1990) and defined in Andrighetto et al. (2013) (see also Andrighetto and Castelfranchi, (2013)). α and β have to be chosen dependent on the weights wc, wo, wnpv, wp, ws, and we in a way that 0 ≤ σ ≤ 1.
4.4. Individual and Normative Drive
According to Andrighetto et al. (2013) the “normative drive” to take an action is the higher of the two saliences recommending or advising against an action, whereas the “individual drive” is a calculation of the possible advantages and disadvantages of an action (similar to the payoffs in the hawk–dove game).
The individual drive to steal or to beg is quite simply modeled as the rank of the individual agent on the wealth dimension mapped to the interval (0, 1] such that the richest has an individual drive to steal or beg of 0.0, whereas the poorest individual drive is (N − 1)/N for N agents. Inversely, the individual drive to help or to punish is just the other way round: the poorest has an individual drive of 1/N, the richest one of 1.0.
4.5. Consequences of Theft and Alms-Begging
Theft is usually successful in the first place, but when it is detected by the victim, the thief is easily found, sanctioned, and possibly punished. With every theft, a certain percentage of the victim’s wealth is taken away and added to the theft’s wealth; in the case of detection, this amount is restored to the victim, and in the case of punishment, an additional fine (as a percentage of the thief’s wealth) is moved to the victim. Whether the victim punishes depends on this agent’s salience of the respective emerging norms and its individual drive to punish.
Alms begging is not automatically successful, but the potential almsgiver first decides whether it will grant the alms, which again depends on its normative and individual drives. If the alms is granted the respective amount is transferred from the almsgiver’s to the beggar’s wealth account.
Beside the updating of the counters in the memory slots of thief, victim, beggar, almsgiver, and observers in the neighborhood of the other two parties, the wealth accounts are updated, hence the wealth distribution in the population changes over time, usually toward more equality.
4.6. Model Results
In a first experiment, the model was run with a number of different values for several input parameters, namely
• the LISTENERS
(or, abbreviated, ) parameter which decides how many nearest neighbors (3, 6, 12, 24) can observe events and receive messages and to whom an agent compares its wealth when it calculates its individual drive to steal, to beg or to grant alms or to punish theft,
• the RANGE
(or r) parameter which defines within which distance (8, 12, 16) potential thieves and beggars look for victims,
• the NDW
(or n) parameter which defines the weight (0.2, 0.4, 0.6, 0.8) of the normative drive against the individual drive (the latter’s weight is simply 1—NDW
),
• the MDBA
(or m) parameter which determines how often (at most every four or at most every ten days) individual agents plan to steal or to beg (“maximum distance between actions”).
As the model generates probability distributions of saliences and propensities that are mostly far from Gaussian, it is inappropriate to use the parameters usually used such as mean or SD or correlation. Nevertheless they are reported in Table 2, but the main output metrics are the number of modes of the distributions (as more often than not there are two and sometimes more clearly distinguished clusters of agents following one norm quite closely and others which follow a conflicting norm). Although sometimes the SDs of the original agent saliences and propensities are quite large, the graphical representations of the distribution (see Appendix) show that this is misleading, as Figure 2 convincingly demonstrates: most of its diagrams show bimodal and/or skewed and/or peaked (leptokurtic) distributions.
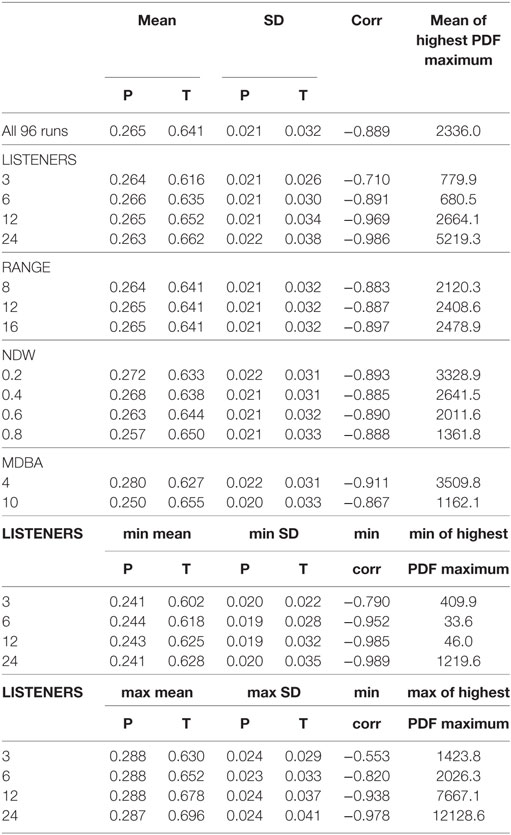
Table 2. Means, SDs, and correlations of the saliences of “Thou shalt not steal!” (T) and “Property is theft!” (P).
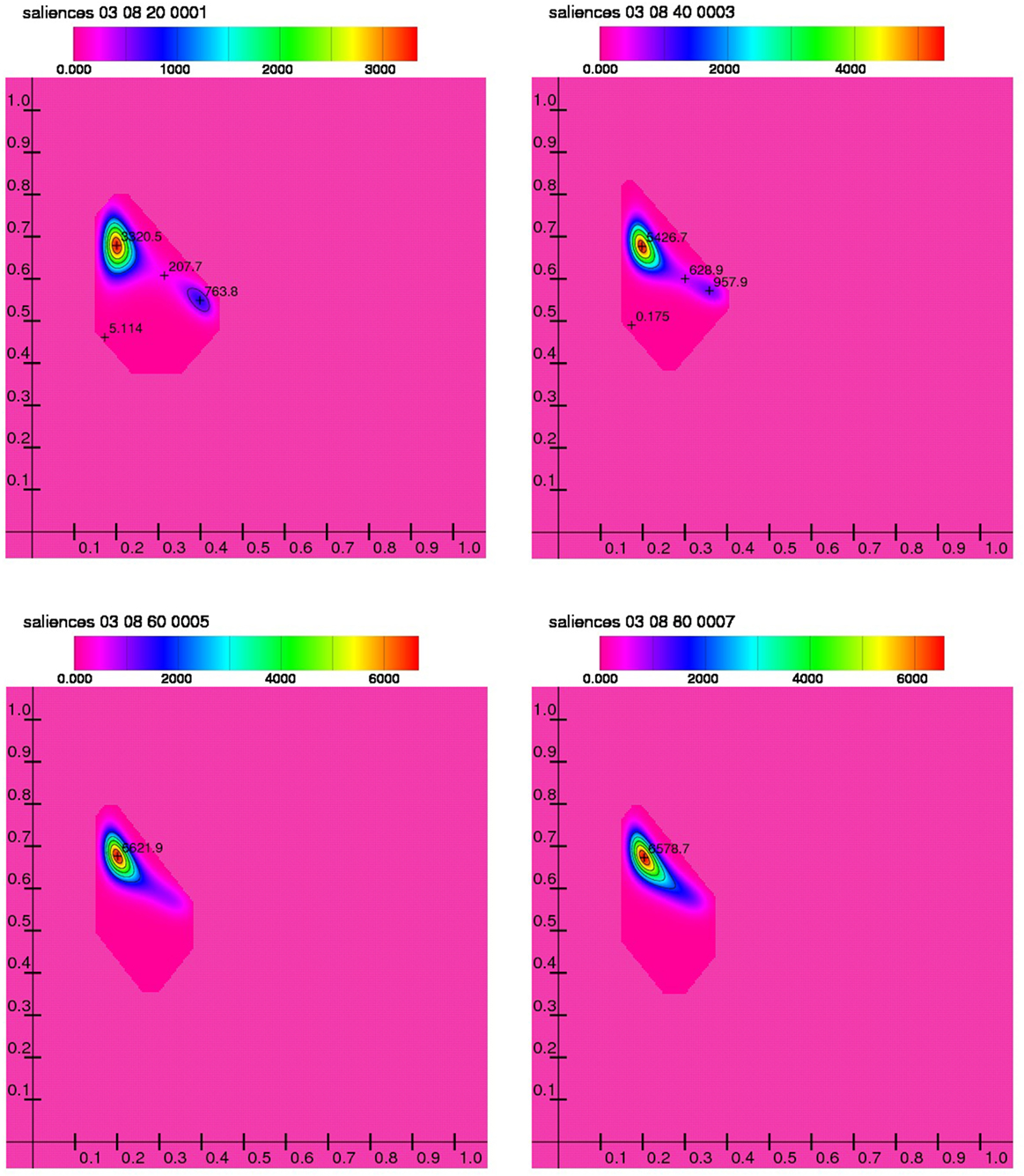
Figure 2. Distributions of the model runs with l = 3 and r = 8 and various n values (from top left to right bottom: 0.2, 0.4, 0.6, 0.8), 10 simulated “days” between successive actions (m = 10).
This becomes also clear when one looks at Table 2 which gives an overview of some of the statistical parameters of the distributions of 96 runs with different combinations of the four parameters. The usual parameters which are sufficient for Gaussian distributions are not sufficient for the distributions emerging during the simulation runs of this model, and this is why an additional feature is mentioned, namely the mean, minimum, and maximum of the maximum of the estimated probability density functions of these distributions.
Table 2 (and more so in combination with Figure 2) reveals that a higher number of LISTENERS
increases the final mean salience of the “Thou shalt not steal!” norm and does not significantly influence the salience of the anti-property norm, but the (negative) correlation between the two saliences grows considerably (from −0.7 to nearly −1.0) with a growing number of LISTENERS
. And perhaps even more strikingly, the distribution becomes more and more leptokurtic with an increasing number of LISTENERS
: in spite of slightly growing SDs the probability density function at its maximum becomes considerably higher or, to put it in another manner, the cluster becomes more and more homogeneous.
The RANGE
parameter has no great effect, as the numbers in the second part of Table 2 do not significantly differ for the three values (see also Table 3 where the η2 is always below 0.025).
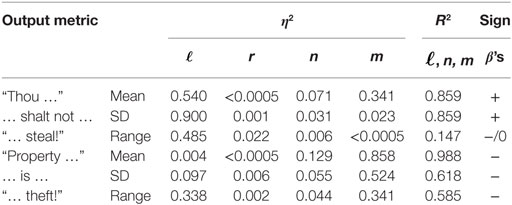
Table 3. Dependence of means, SDs and ranges of the saliences of “Thou shalt not steal!” and “Property is theft!” on the input parameters , r, n, and m.
The higher NDW
, the higher is the anti-theft norm salience and the lower is the anti-property norm salience, but as Table 3 shows these differences are not very important (with the highest η2 < 0.13), but the effect of an increasing importance of the normative drive and the corresponding decrease of the individual drive leads to a decrease of the kurtosis, i.e., the distribution is more widespread with high values of NDW
.
Finally, MDBA
—the speed parameter of the model—also makes a difference: The mean saliences of the anti-property norm differ by 1.5 SDs for MDBA
= 4 and MDBA
= 10, and for the other norm this difference is also about one SD, although, according to Table 3 not very important with an η2 of less than 0.35.
The ranges of the kurtosis indicators for the runs with different LISTENERS
values are quite wide, but the ranges for LISTENERS
= 3 and LISTENERS
= 24 hardly overlap.
So far only the main maximum of the estimated probability density function was discussed. But 18 out of the 96 runs yielded bimodal or multimodal distributions:
• for LISTENERS
= 3, there were five runs where the highest PDF maximum was between 14 and 24 times as high as the second highest maximum—not a clear case for bimodality (and in diagrams as in Figure 2 they would be hardly visible);
• for LISTENERS
= 12, there were two runs where the highest PDF maximum was between 26 and 35 times as high as the second highest maximum—not a clear case for bimodality either;
• for LISTENERS
= 24, there were eleven runs with multiple maxima
– of which five for MDBA
= 10 and NDW
≥ 0.6 where the highest PDF maximum was between only 1.9 and 6.3 times as high as the second highest maximum—a clear case for two separate clusters;
– whereas the other six showed a highest maximum which was between 26 and 870 times as high as the second highest—not a case for multimodality at all.
One would interpret these last findings as an indicator that a high number of LIST−EN−ERS
together with a slow speed of action (MDBA
= 10) and a high weight of the normative drive leads to separate clusters after three simulated months or, as the second experiment will show, to a belated and slow transition from the early indifference with respect to the anti-theft norm to its late high salience.
Hence, this first experiment shows that several interesting output metrics depend on some of the input parameters. We go a little farther taking also the total variance reduction into account in Table 3. The output metrics are the means, SDs and ranges (max–min) of the saliences of the two norms which are in the focus of this paper, “Thou shalt not steal!” and “Property is theft!.”
RANGE
is obviously a negligible input parameter, hence will no longer be used in further experiments, the other three contribute to the reduction of the variance of the statistical parameters mentioned in Table 3 to different degrees: the variance of both mean and SD of the distributions of the saliences of the two norms is reduced considerably by these three input parameters, and this is also true for the range of the distribution of the salience of the norm “Property is theft!.” The regression coefficients for the parameters of the salience of the “Thou shalt not steal!” norm are positive except for the range of this distribution where the variance reduction is modest anyway, whereas the coefficients for the salience of the other norm are all negative.
From this first experiment, we can conclude that the more listeners and observers participate in the communication relations of an individual agent, the higher the salience of the anti-theft norm and the lower the salience of the anti-property norm. Given the correlation between the two saliences, the variance or SD of the two saliences is of lesser importance, as the region in the coordinate system of these two variables which is populated with agents is small and lies on a diagonal in the plane—see Figure 2 which shows that even for a small number of listeners and observers this region is rather narrow (in the direction of the maximum extension, it measures less than 0.3 units; in the orthogonal direction, it is as short as approximately 0.1 for the first graph there; for higher numbers of listeners the length remains the same, but the width shrinks to 0.04 units).
Perhaps more interesting than the final state of the simulation after three simulated “months” is what happens in between: In the beginning of a typical run the joint distribution of the two saliences is concentrated around a state which corresponds to a “property is theft” salience of 0.152 and a “Thou shalt not steal” salience of 0.464 (at this point the density function has the value 761.219), with a second much smaller cluster around (0.153, 0.666, 96.883)—see Figure 3, leftmost diagram. Around the simulated “day” 24, the distribution bifurcates, i.e., is split into two nearly separate clusters, one (which in turn consists of two close clusters) around (0.153, 0.546, 106.162) and one around (0.20, 0.65)—to be more precise: with two maxima at (0.173, 0.690, 72.650) and at (0.214, 0.632, 72.034). Some 20 “days” later only one maximum survives around (0.196, 0.666, 211.254).
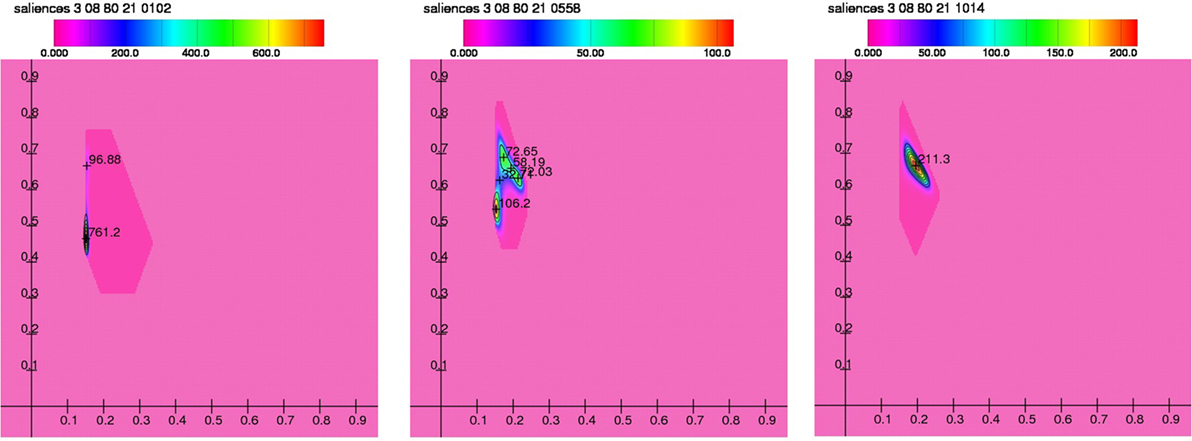
Figure 3. Changed distributions of the model run with l = 3 and r = 8, n = 0.8 and 3 weeks between successive actions, i.e., m = 21 (from left to right on simulated “day” 5, 24, and 43). The area in light magenta is entirely empty, the area in saturated magenta contains very few agents, the vast majority of agents is concentrated inside the contour lines, i.e., in the non-magenta areas.
This leads to the conclusion that at least in this simulation model the phase transition (or the lock-in, as this phenomenon is called elsewhere (Schreyögg and Sydow, 2010)) is rather quick and leads to a stable situation in which the salience of the lawlike rule not to steal is rather high whereas the contrasting, as it were, anarchic anti-property rule is rather weak (and is never very strong in any agent).
4.7. An Attempt at Validating the Model
The World Value Survey (World Values Survey Association, 2015) contains several questions about the justifiability of offenses against property, some of which deal with stealing from individual persons, others with appropriating public services. The former may be taken as empirical correlates of the salience of the “Thou shalt not steal!” norm whereas the latter may be taken as empirical correlates of the “Property is theft” norm: Whoever justifies stealing shows a low salience of the anti-theft norm, and whoever justifies using public services without paying complies with the norm that which is public is nobody’s property. The respective variables in the WVS are on an interval scale with integer values between 1 and 10, hence it seemed appropriate to use a factor analysis to get two correlated scales with zero mean and unit variance. Table 4 shows the correlations between the questionnaire items6 and the factors, making clear that factor 1 is sufficiently similar to the salience of the “Thou shalt not steal” norm of the simulation, whereas factor 2 resembles the “Property is theft” norm of the simulation model.
The comparison between the simulation data and the empirical data suffers from two shortcomings:
• The empirical data were not collected to validate the simulation model and are thus no unequivocal operationalizations of the saliences of the two norms but only substitutes.
• The empirical data had to be standardized as the original items suffer from the fact that a large proportion of the respondents gave extreme answers on the interval scales, declaring the contents of the item sentences as “never justifiable” (for all items more than 50% of the respondents, for some items even 70%; or as definitely justifiable—the percentage of respondents answering “always justifiable” or 10—in the total survey about 3%—is always higher than the percentage of repondents answering 8 or 9). In some countries the pattern of responses is entirely different: in Algeria, for instance about 28% say “never justifiable,” about 10% use the answer “5” and some 11% say “always justifiable.” Mexico is a similar example (one-third say “never justifiable,” 11.5% choose the “5,” and 13.6% say “always justifiable”). Only with the transformation data are generated which allow a comparison to the simulated data. But the problem that the simulated and the empirical scales are differently anchored cannot be overcome.
Notwithstanding the difficulties of this validation one can identify different patterns in the factor distributions between countries as Figure 4 shows.
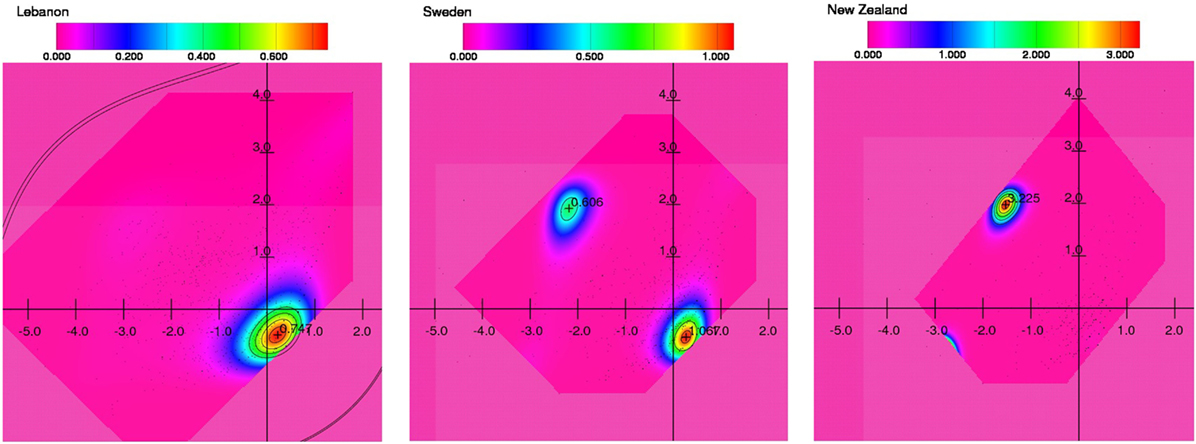
Figure 4. Distribution of factor values for some of the countries represented in the World Values Survey. Horizontal axis: Factor 2 of Table 4, Vertical axis: Factor 1 of Table 4. The orientation, but not the scale of the axes is comparable to those in Figures 2 and 3. The area in light magenta is entirely empty, the area in saturated magenta contains very few respondents, the vast majority of agents is concentrated inside the contour lines, i.e., in the non-magenta areas.
There are obvious similarities between the diagrams in Figures 3 and 4:
• The case of Lebanon resembles the early stage of simulation where there is still a high proportion who do not declare stealing (46.08%) and claiming government benefits (67.33%) as “never justifiable.” Argentina, Cyprus, Colombia, and Ecuador, to name just a few, show a similar distribution.
• Sweden looks like the transition phase of the simulation where 30.43% declare stealing and 43.57% declare claiming government benefits as at least sometimes justifiable, and the transformed data show two separate clusters. Australia, Poland, and Slovenia, to name again just a few, show a similar distribution
• New Zealand, however, corresponds to the final stage of the simulation, with only 16.5% and 30.26% of those who did not respond with “never justifiable.” This kind of distribution is less frequent, only Armenia shares it.
As conceded in the beginning of this section the validation of this simulation suffers from the type of questions the World Value Survey asked its respondents: This type of questions is particularly prone to the well-known “effect of social desirability on answers” [Fowler and Cosenza, 2009, p. 389–390]. Perhaps it might have been better to use an alternative introducing question replacing the “you” in “Please tell me for each of the following actions whether you think it can always be justified, never be justified, or something in between, using this card.” with “people in your country.”7
5. Conclusion
Both models have shown that no central power is necessary to introduce lawlike rules in a society. Making everybody using the same side of the street when they drive cars resulted quite easily even from the most primitive variant of the model in Section 3, and the process was sped up when local information or local norm invocations were introduced into the model. Likewise, the model in Section 4 showed that an anti-theft norm emerges and its salience increases as a consequence of individual interactions between thieves and their victims (or between thieves and third agents observing a theft) even when those who take offense at an action and reproach the offender individually have no formal role such as the role of police or prosecutor.
Hence both models give a positive answer to the question formulated in the title of this paper: Yes, lawlike rules can emerge without the intervention of legislators, no Hobbesian sovereign is necessary to set the “first and Fundamental Law of Nature; which is to seek Peace, and follow it” (Hobbes, 1965, ch. XIV) into force. Whether in the history of humankind the emergence of traffic and anti-theft rules were the same as or similar to the processes modeled in Sections 3 and 4 remains an open question, although not the question of this paper.
Author Contributions
The author confirms being the sole contributor of this work and approved it for publication.
Conflict of Interest Statement
The author declares that the research was conducted in the absence of any commercial or financial relationships that could be construed as a potential conflict of interest.
Footnotes
- ^Opp (2002) analyzed this scenario much more formally and supported it with empirical evidence of the late 1990s, (Bicchieri and Muldoon, 2014) and [(Epstein, 2006), 234], too, use this scenario for illustrating their models.
- ^For a model of a similar target system—conformity to binary norms—see (Epstein, 2006), but unlike the model presented here, Epstein’s agents do not participate in any traffic and do not experience traffic jams but conform to a norm rather thoughtlessly.
- ^Unlike in earlier papers (cf. (Andrighetto and Conte, 2014; Nardin et al., 2016)), we call such an utterance in a conflict only a critical remark, not a norm invocation, as at least in the very beginning no norm has ever emerged that could be invoked.
- ^Proudhon argues that the original meaning of the eighth commandment forbids any kind of appropriation, but then it is the tenth commandment that forbids coveting another person’s property (Exodus 20:17). Hence, we will interpret the eighth commandment in a way that it refers to the appropriation of another person’s private property and distinguish it from the opinion that no private property is justified at all.
- ^Both models used in this paper will be made available at https://ccl.northwestern.edu/netlogo/models/community/TrafficLawEmergence und ../TheftNorm. The interface of the theft model is not shown here as it yields much more output than can be discussed here such that this interface would mislead readers.
- ^The question was “Please tell me for each of the following actions whether you think it can always be justified, never be justified, or something in between, using this card.”, the text of the items used here can be found in Table 4, the answers were coded as 1 for “Never justifiable” and 10 for “Always justifiable”, such that 1 corresponds to a salience of 1.0 whereas 10 corresponds to a salience of 0.0, but see below for the social desirability impaired quality of measurement.
- ^The World Value Survey followed such a strategy when they asked in V56 “Do you think most people would try to take advantage of you if they got a chance, or would they try to be fair? Please show your response on this card, where 1 means that “people would try to take advantage of you,” and 10 means that “people would try to be fair”; applying this technique, too, to the items discussed here might have led to better interpretable results.
References
Andrighetto, G., Brandts, J., Conte, R., Sabater-Mir, J., Solaz, H., and Villatoro, D. (2013). Punish and voice: punishment enhances cooperation when combined with norm-signalling. PLoS ONE 8:e64941. doi: 10.1371/journal.pone.0064941
Andrighetto, G., and Castelfranchi, C. (2013). Norm compliance: the prescriptive power of normative actions. Paradigmi 6, 120–135. doi:10.3280/PARA2013-002011
Andrighetto, G., and Conte, R. (2014). Norms’ Dynamics as a Complex Loop, Vol. 6. Oxford: Oxford University Press, 81–93.
Axelrod, R. (1986). An evolutionary approach to norms. Am. Polit. Sci. Rev. 80, 1095–1111. doi:10.2307/1960858
Bicchieri, C. (2006). The Grammar of Society: The Nature and Dynamics of Social Norms. New York: Cambridge Univerity Press.
Bicchieri, C., and Muldoon, R. (2014). “Social norms,” in The Stanford Encyclopedia of Philosophy, ed. E. N. Zalta (Stanford: Metaphysics Research Lab, Stanford University), 2014.
Campenní, M., Andrighetto, G., Cecconi, F., and Conte, R. (2014). “Hunting for norms in unpredictable societies,” in Minding Norms. Mechanisms and Dynamics OS Social Order in Agent Societies, Vol. 7. eds. R. Conte, G. Andrighetto and M. Campennì. (Oxford, New York: Oxford University Press), 94–117.
Cialdini, R. B., Reno, R. R., and Kallgren, C. A. (1990). A focus theory of normative conduct: recycling the concept of norms to reduce littering in public places. J. Pers. Soc. Psychol. 58, 1015–1026. doi:10.1037/0022-3514.58.6.1015
Cobb, L. (1978). Stochastic catastrophe models and multimodal distributions. Behav. Sci. 23, 360–374. doi:10.1002/bs.3830230407
Cobb, L. (1981). “Stochastic differential equations for the social sciences,” in Mathematical Frontiers of the Social and Policy Sciences, American Association for the Advancement of Science Selected Symposia Series 54, eds L. Cobb and R. M. Thrall (Boulder, CO: Westview), 37–68.
Durkheim, E. (1895). in The Rules of the Sociological Method, eds L. Steven and W. D. Halls, trans. (New York, London, Toronto, Sydney: The Free Press).
Eigen, M., and Schuster, P. (1979). The Hypercycle. A Principle of Natural Self-Organization. Berlin: Springer-Verlag.
Elsenbroich, C., and Gilbert, N. (2014). Modelling Norms. Dordrecht, Heidelberg, New York, London: Springer.
Epstein, J. M. (2006). Learning to Be Thoughtless: Social Norms and Individual Computation, Vol. 10. Princeton, Oxford: Princeton University Press, 228–244.
Fowler, F. J. Jr., and Cosenza, C. (2009). Design and Evaluation of Survey Questions, 2nd Edn, Vol. 12. Los Angeles, London, New Delhi, Singapore, Washington, DC: SAGE, 375–412.
Gilbert, N., and Troitzsch, K. G. (2005). Simulation for the Social Scientist, 2nd Edn. Maidenhead, NY: Open University Press.
Hayek, F. A. V. (1944). Scientism and the study of society. Economica 11, 27–39. doi:10.2307/2549942
Herlitzius, L. (1990). “Schätzung nicht-normaler Wahrscheinlichkeitsdichtefunktionen,” in Computer Aided Sociological Research. Proceedings of the Workshop “Computer Aided Sociological Research” (CASOR’89), Holzhau/DDR, October 2nd–6th, 1989, eds J. Gladitz and K. G. Troitzsch (Berlin: Akademie Verlag), 379–396.
Hobbes, T. (1965). Leviathan, or the Matter, Forme and Power of a Commonwealth, Ecclesiasticall and Civill, Vol. 691. Dutton, London: Everyman’s Library.
Kant, I. (1785). in Groundwork for the Metaphysics of Morals, eds A. W. Wood, J. B. Schneewind, M. Baron, and S. Kagan (New Haven, London: Yale).
Lotzmann, U., Möhring, M., and Troitzsch, K. G. (2013). Simulating the emergence of norms in different scenarios. Artif. Intell. Law 21, 109–138. doi:10.1007/s10506-012-9130-y
Lotzmann, U., Möhring, M., and Troitzsch, K. G. (2014). “The derivation of EMIL-S from EMIL-A: from cognitive architecture to software architecture,” in Minding Norms, eds R. Conte, G. Andrighetto, and M. Campenní (New York: Oxford University Press), 118–126.
Martinez Coll, J. C. (1986). A bioeconomic model of Hobbes’ ’state of nature’. Soc. Sci. Info. 25, 493–505. doi:10.1177/053901886025002010
Maynard Smith, J., and Price, G. (1973). The logic of animal conflict. Nature 246, 15–18. doi:10.1038/246015a0
Muldoon, R., Lisciandra, C., Bicchieri, C., Hartmann, S., and Sprenger, J. (2014). On the emergence of descriptive norms. Polit. Philos. Econ. 13, 3–22. doi:10.1177/1470594X12447791
Nardin, L. G., Andrighetto, G., Conte, R., Székely, A., Anzola, D., Elsenbroich, C., et al. (2016). Simulating protection rackets: a case study of the Sicilian Mafia. J. Auto. Agents Multi Agent Syst. 30, 1117–1147. doi:10.1007/s10458-016-9330-z
Neumann, M. (2014). “How are norms brought about?” in Minding Norms, eds R. Conte, G. Andrighetto, and M. Campenní (New York: Oxford University Press), 50–47.
Opp, K.-D. (2002). When do norms emerge by human design and when by the unintended consequences of human action? The example of the non-smoking norm. Rational. Soc. 14, 131–158. doi:10.1177/1043463102014002001
Piirimäe, P. (1965). The Explanation of Conflict in Hobbes’s Leviathan. TRAMES. Everyman’s Library. (Vol. 691). London: Dent, Dutton 10(60/55) (1):3–21.
Plato. (1926). in Laws, 4th Edn, Vol. IX, ed. R. G. Bury, trans. (Cambridge: Harvard University Press, William Heinbemann).
Proudhon, P. J. (1876). in The Works of P.J. Proudhon. Volume I. What Is Property? An Inquiry into the Principle of Right and Government, ed. B. R. Tucker, trans. (Princeton, MA: Benj. R. Tucker).
Railsback, S., Ayllón, D., Berger, U., Grimm, V., Steven Lytinen, C. S., and Thiele, J. (2017). Improving execution speed of models implemented in netLogo. J. Artif. Soc. Soc. Simul. 20. doi:10.18564/jasss.3282
Schreyögg, G., and Sydow, J. (eds) (2010). The Hidden Dynamics of Path Dependence. London: Palgrave Macmillan.
Troitzsch, K. G. (1990). “Self-organisation in social systems,” in Computer Aided Sociological Research. Proceedings of the Workshop “Computer Aided Sociological Research” (CASOR’89), Holzhau/DDR, October 2nd–6th, 1989, eds J. Gladitz and K. G. Troitzsch (Berlin: Akademie Verlag), 353–377.
Troitzsch, K. G. (2015). Extortion racket systems as targets for agent-based simulation models. Comparing competing simulation models and emprical data. Adv. Complex Syst. 18. doi:10.1142/S0219525915500149
Troitzsch, K. G. (2016). Can agent-based simulation models replicate organised crime? Trends Organ. Crime 20, 100–119. doi:10.1007/s12117-016-9298-8
United Nations. (1949). Geneva Convention on Road Traffic. Available at: http://www.unece.org/fileadmin/DAM/trans/conventn/Convention_on_Road_Traffic_of_1949.pdf
Weidlich, W., and Haag, G. (1983). Concepts and Models of a Quantitative Sociology. The Dynamics of Interacting Populations, Vol. 14. Berlin: Springer-Verlag.
Wilensky, U. (1999). NetLogo. Available at: http://ccl.northwestern.edu/netlogo
World Values Survey Association. (2015). World Values Survey 1981–2014 Longitudinal Aggregate v.20150418. Madrid, Spain: Aggregate File Producer: JDSystems.
Appendix
A. Calculating Estimates for Density Functions of Multimodal Distributions in Two Variables
This appendix gives a short introduction into the technique used to generate the figures of Section 4. This technique goes back to papers written around 1980 (Cobb, 1978, 1981) and was extended in Herlitzius (1990) and used in Troitzsch (1990). It assumes that the density function of the underlying distribution is of the form where F (x1, x2; θ) is a polynomial up to the fourth degree in the variables x1 and x2 which can also be written as a vector x. The background of this technique is the idea that a two-dimensional distribution is generated by a stochastic process whose drift functions
are polynomials up to the third degree. The stationary state of such a process (for t → ∞) is then such a distribution if the covariance function of the process is the identity matrix. In this case equation 2 can also be written as
If this condition is fulfilled, the parameters of f can be calculated using the moments of the empirical (or in our case: the simulated) distribution (of the agent variables, saliences or propensities, two at a time). The stationary probability density functions and the drift functions have the form in equations 4, 5, and 6 (simplified from Herlitzius (1990)).
In two dimensions (where perhaps x and y are more convenient to read than x1 and x2), the probability density function to be estimated is of the type
with n even, i + j ≤ n, i, j ≥ 0, θn0, θn0, θ0n < 0, and by the following procedure.
We first define as many drift functions1 as we have dimensions (in the following only two):
with αij = − iθij and βij = − jθij which implies
for all i, j > 0, i + j ≤ n, and note that
for any polynomial p(x,y) in x and y.
To estimate the θij’s from the empirical moments (or here, the moments of the simulated distributions) , we minimize a quadratic criterion
with constraints on the ’s and ’s as in equation 7, yielding a monstrous yet solvable system of linear equations
where equations 11 and 12 stand for (n2 + n)/2 single equations, and equation 13 stands for (n2 − n)/2 single equations. In the equations of type 11 l runs from 0 to n − 1, and k runs from 1 to n − l; in the equations of type 12 k runs from 0 to n − 1, and l runs from 1 to n − k; in the equations of type 13 k runs from 1 to n − 1, and l runs from 1 to n − k.
Thus, for two dimensions and a polynomial in the exponent of the probability density function{xe "probability density function"} up to order n, a system of (3n2 + n)/2 linear equations has to be solved.
This algorithm will sometimes—especially when the actual distribution in the population is approximately normal—yield θn0 > 0 and/or θ0n > 0 which is contrary to our assumption. In these cases, n will have to be changed appropriately; for n = 2 θ20 > 0 or θ02 > 0 will never occur since the parameters of a normal distribution can always be calculated from the moments up to second order.2
As equation 7 does not contain θ00, finally, it has to be calculated using the constraint : The double integral has to be estimated with an arbitrary , and then θ00 is the negative natural logarithm of the value of the double integral thus calculated.
Footnotes
- ^These drift functions are just a mathematical formalism which in a way represents the changes of the saliences and propensities calculated in the simulation model in an entirely different manner—and in real actors the mechanisms of changing saliences and propensities are once more different and perhaps more or less unknown.
- ^In other cases, the differences between the simulated or, more so, the empirical variables may differ from the assumed form of the postulated distribution. This seems to be partly true for the empirical distributions shown in Figure 4. Higher orders in the polynomial Φ in equation (2) may solve this problem at the expense of an exponential growth of the number of the elements of θ.
Keywords: artificial society, emergence, lawgiver, simulation, road traffic, theft, eigth commandment
Citation: Troitzsch KG (2018) Can Lawlike Rules Emerge without the Intervention of Legislators? Front. Sociol. 3:2. doi: 10.3389/fsoc.2018.00002
Received: 26 October 2017; Accepted: 02 February 2018;
Published: 02 March 2018
Edited by:
Nicola Lettieri, Istituto nazionale per l’analisi delle politiche pubbliche (INAPP), ItalyReviewed by:
Stephen Turner, University of South Florida, United StatesFederico Cecconi, Istituto di Scienze e Tecnologie della Cognizione (ISTC) – CNR, Italy
Copyright: © 2018 Troitzsch. This is an open-access article distributed under the terms of the Creative Commons Attribution License (CC BY). The use, distribution or reproduction in other forums is permitted, provided the original author(s) and the copyright owner are credited and that the original publication in this journal is cited, in accordance with accepted academic practice. No use, distribution or reproduction is permitted which does not comply with these terms.
*Correspondence: Klaus G. Troitzsch, klaus.g.troitzsch@bluewin.ch