- 1University of Illinois at Chicago, Chicago, IL, United States
- 2Illinois Department of Public Health, Springfield, IL, United States
- 3University of Chicago, Chicago, IL, United States
The current opioid crisis and the increase in injection drug use (IDU) have led to outbreaks of HIV in communities across the country. These outbreaks have prompted country and statewide examination into identifying factors to determine areas at risk of a future HIV outbreak. Based on methodology used in a prior nationwide county-level analysis by the US Centers for Disease Control and Prevention (CDC), we examined Illinois at the ZIP code level (n = 1,383). Combined acute and chronic hepatitis C virus (HCV) infection among persons <40 years of age was used as an outcome proxy measure for IDU. Local and statewide data sources were used to identify variables that are potentially predictive of high risk for HIV/HCV transmission that fell within three main groups: health outcomes, access/resources, and the social/economic/physical environment. A multivariable negative binomial regression was performed with population as an offset. The vulnerability score for each ZIP code was created using the final regression model that consisted of 11 factors, six risk factors, and five protective factors. ZIP codes identified with the highest vulnerability ranking (top 10%) were distributed across the state yet focused in the rural southern region. The most populous county, Cook County, had only one vulnerable ZIP code. This analysis reveals more areas vulnerable to future outbreaks compared to past national analyses and provides more precise indications of vulnerability at the ZIP code level. The ability to assess the risk at sub-county level allows local jurisdictions to more finely tune surveillance and preventive measures and target activities in these high-risk areas. The final model contained a mix of protective and risk factors revealing a heightened level of complexity underlying the relationship between characteristics that impact HCV risk. Following this analysis, Illinois prioritized recommendations to include increasing access to harm reduction services, specifically sterile syringe services, naloxone access, infectious disease screening and increased linkage to care for HCV and opioid use disorder.
Introduction
HIV outbreaks related to injection drug use (IDU) in the setting of the current opioid crisis have been reported in communities across the U.S. In 2014, Scott County, Indiana identified more than 200 cases of HIV linked to the injection of prescription opioids. In subsequent years Massachusetts identified an outbreak of 116 cases with HIV that occurred among people who inject drugs (PWID)1 (Alpren et al., 2020), and more recently, West Virginia has experienced HIV clusters amongst the PWID communities in multiple counties2,3. Outbreaks of HIV have also been associated with underlying and preceding networks of hepatitis C virus (HCV) infection, which may portend future risk of HIV given the relationship with drug injection (Shepard et al., 2005; Mumtaz et al., 2015; Ramachandran et al., 2018).
The opioid crisis, which has disproportionately affected rural communities has laid bare the multiple population and environmental factors that underlay vulnerability to these infectious diseases (Keyes et al., 2014; Van Handel et al., 2016). Individual-level characteristics, such as demographic (e.g., age, race, and disability status) and socioeconomic factors (i.e., poverty status, employment, homelessness, and education) are associated with risk of HIV (Des Jarlais et al., 2020; Schalkoff et al., 2020). Low healthcare access, including infectious disease screening, prevention measures, such as syringe service programs and substance use disorder treatment can affect the spread of a disease related to injection drug use (Havens et al., 2018; Lerner and Fauci, 2019; McLuckie et al., 2019; Siegler et al., 2019). At the level of the physical environment, characteristics, such as transportation and types of housing can impact engagement in and utilization of HIV services4 (Aidala et al., 2016). In Illinois, fatal and nonfatal overdoses related to opioids have increased by 3% between 2018 and 20195. While the major metropolitan areas have represented the majority of absolute cases, rural areas have experienced some of the highest per population rates of both overdose as well as other consequences of the opioid epidemic, such as neonatal abstinence syndrome6,7. HCV infection in the state has increased by 43% from 6,887 in 2006 to 9,838 in 2017, with many of the cases in individuals younger than 35 years of age and linked to injection drug use8.
Prior studies, including analyses performed by the Centers for Disease Control and Prevention (CDC) have examined multiple factors at the national level to identify areas at high risk of rapid HIV transmission (Rickles et al., 2018; McLuckie et al., 2019). Such work has outlined a methodological model for vulnerability analysis that has inspired states to take a finer approach in examining factors based on local data.
We offer further insight at the subcounty, ZIP code level in Illinois to what local areas are vulnerable to an HIV outbreak. Recent work in southern Illinois showed more variation at the ZIP-scale than the county-level, necessitating further investigation at the full state scale (McLuckie et al., 2019). ZIP code-level analysis provides a more granular understanding of risk within large and diverse counties in Illinois, but is also generally large enough to protect individual privacy when summarizing health records. This may allow local jurisdictions to more narrowly target specific public health interventions within these vulnerable areas in an effort to prevent future outbreaks. Additional inclusion of environmental factors will provide further contextual information to identify resource gaps that may impact outbreak risk.
Materials and Methods
Study Design
An ecologic study design was used to evaluate associations of HCV health outcomes and various related health outcomes, treatments, intervention, and risk factors at an environmental scale. ZIP code tabulation (ZCTA) level indicators were collected from the 2013–2017 five-year average US Census American Community Survey, Illinois state-specific indicators from the CDC, and surveillance data related to HCV, sexually transmitted diseases and fatal and non-fatal opioid-related overdose from the Illinois Department of Public Health. Data were collected for 2017 and 2018 to mirror the methodology used by CDC (Van Handel et al., 2016). Illinois' 1,383 ZCTA codes comprised the study sample. Covariates were collected and analyses conducted at the ZIP code level.
Data
ZIP code level vulnerability to an HIV or HCV outbreak related to injection drug use was indicated by the detection incidence of HCV cases from 2017 to 2018. All confirmed and probable cases of HCV infection (chronic and acute) in individuals <40 years of age were included (Table 1). This designation was used as a proxy for acute HCV infections which are known to be underreported due to the asymptomatic and minimally symptomatic nature of most acute infections (Onofrey et al., 2015). HCV detection incidence was defined as diagnosed cases meeting a confirmed case definition for HCV infection, indicated by a positive HCV nucleic acid test or HCV antigen test. Rates were obtained from the Illinois Department of Public Health (IDPH) program and the Illinois- National Electronic Disease Surveillance System (I-NEDSS), which collects mandated infectious disease reporting from laboratories, health care providers, and other mandatory reporters.
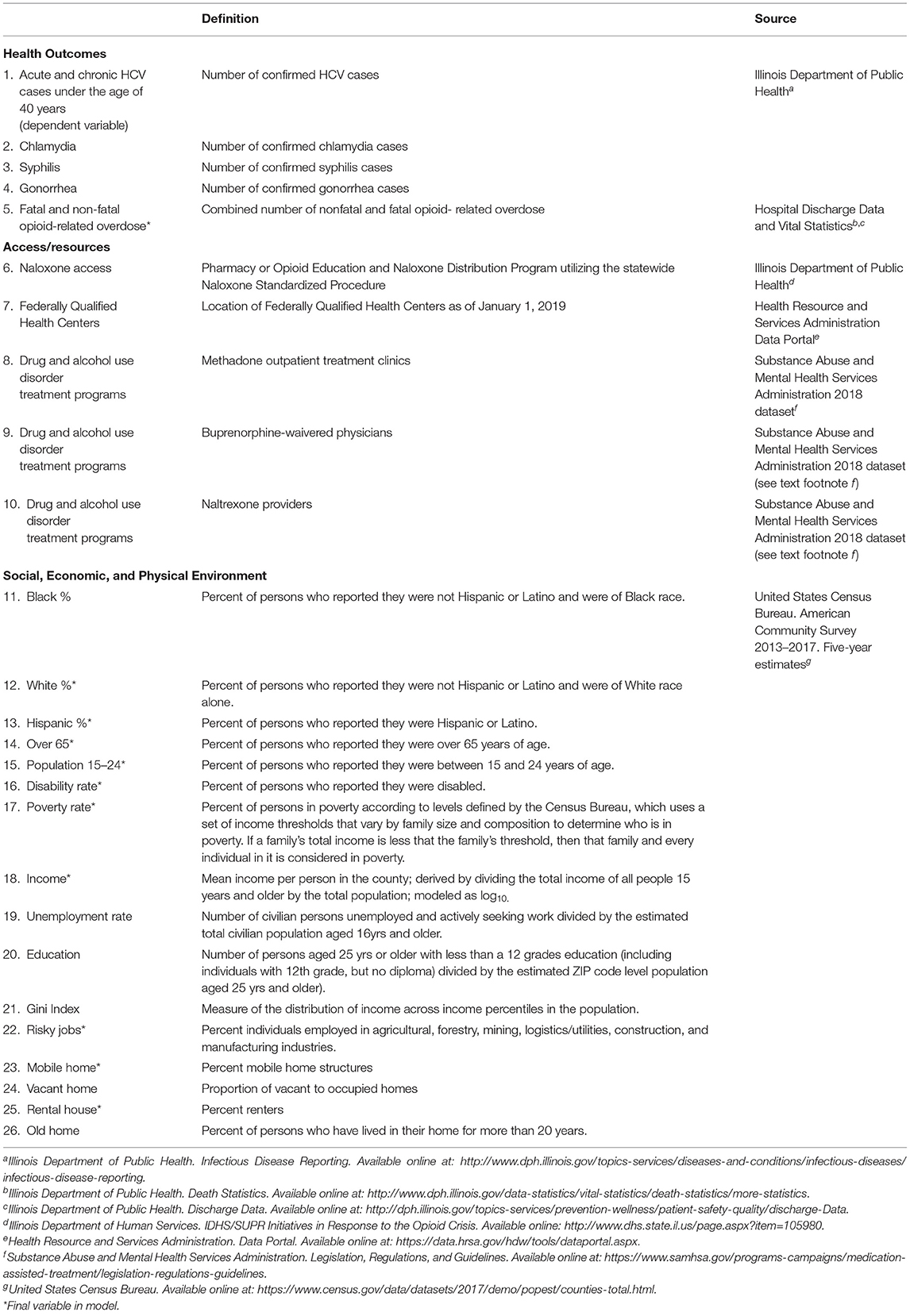
Table 1. Dependent variable and indicators originally identified to be used in regression model at the ZIP code level, Illinois, 2017–2018.
ZIP code level predictors were identified through author consensus as potentially predictive of high risk for HIV/HCV transmission and summarized across all Illinois ZCTA areas (n = 1383). Some indicators were collected as counts and subsequently calculated as rates, per total population between ages 15–40 years (Table 1).
Chlamydia, gonorrhea and syphilis cases were collected via the same mechanism as HCV. Fatal overdose rates were extracted from Illinois Vital Records: heroin deaths were assigned as any drug overdose death in which heroin (ICD-10 code T40.1) was reported as a cause of death; analgesic deaths were assigned as any drug overdose death in which prescription analgesics (methadone, synthetic narcotics, or other prescription opioids, ICD-10 codes T40.2, T40.3, T40.4) were reported as a cause of death; opioid deaths as any drug overdose death in which any opioid drug was a contributing cause of death—includes the above four ICD-10 codes as well as T40.0 (opium) and T40.6 (other/unspecific narcotics). Non-fatal opioid-related overdose rates were collected from IDPH hospital and emergency department (ED) discharge data.
To better understand access to resources throughout the state, we included several treatment and intervention variables: 1) access to a pharmacy utilizing a naloxone standing order; 2) access to clinicians or clinics that can prescribe/dispense buprenorphine, methadone, or naltrexone; 3) and access to federally qualified health centers (FQHC). These systems were included within access to resources due to their ability to serve and engage the community (Joudrey et al., 2019). Specifically, FQHC's serve medically underserved areas, provide a wide range of services, including counseling and medication-assisted treatment, and have been shown to be associated with opioid-related mortality (Haley et al., 2019; Flores et al., 2020). Access to pharmacies with a standing order for naloxone (from the IDPH registry), “drug use disorder treatment programs” (as defined by methadone outpatient treatment clinics), buprenorphine-waivered physicians with records of prescribing in the Illinois Prescription Monitoring Program, and naltrexone providers (sourced from the Substance Abuse and Mental Health Services Administration 2018 dataset), and federally qualified health centers (from study collection) were available as locations at the address level, and subsequently geocoded and converted to spatial data points. Distance from the ZIP code center to nearest facility was then calculated in QGIS software.
ZIP code level demographics were collected from the American Community Survey 2013–2017, five-year estimate (American Community Survey 2017). Covariates reflect place-based features of the Risk Environment Model that guide studies of the social determinants of HIV-related outcomes among PWID, recently extended and adapted to southern Illinois at the ZCTA scale (Rhodes, 2009; Kolak et al., 2020). Covariates were extracted to approximate varying dimensions of risk across social, economic, and physical environments as guided by review of the Risk Environment Model literature, as well as input from local and state-level taskforce meetings involving stakeholders from local health departments, emergency medical services and other first responders, community-based service organizations. and advocacy groups. Percent Whites, Blacks, and Hispanic persons were included at the ZIP code level (Keyes et al., 2014). Seniors were designated by percent of population over 64 years of age, young adults by percent of population aged 15–24 years, and percentage of persons with a disability (Keyes et al., 2014). Areas with greater proportions of seniors, young adults, and/ or persons with a disability may reflect different dimensions of neighborhood structures that can influence, interact with, and impact opioid use disorder (OUD) risk environments (Brady et al., 2017). Also included were indicators of socioeconomic status including percent of households in poverty, per capita income; percent unemployment; percent of working aged individuals without a high school diploma; and an income inequality Gini coefficient. Income was normalized for interpretability through a log transformation. “High risk” employment was proxied as the percent of individuals employed in agricultural, forestry, mining, logistics/utilities, construction, and manufacturing industries; these jobs were identified as at a greater risk of injury according to the CDC. Furthermore, variables were included that may act as physical environment indicators such as proportion of mobile homes, percent of vacant homes, percent persons who have lived in their home for more than 20 years, and percent renters (Kolak et al., 2020).
Regression
Given the large sample size (n = 1383) due to using the ZIP code level approach, the number of indicators did not need to be reduced for analysis. Each variable was independently assessed for association with the outcome. To assess correlation between indicators, we developed a Spearman correlation matrix. The correlation matrix was calculated for pairwise complete observations and correlation plot was implemented with the proc corr package in SAS version 9.4 software (SAS Institute, Cary, North Carolina).
A multivariable negative binomial regression was performed for all five-digit ZIP code tabulation areas in Illinois, with ZCTA code level population as an offset. Negative binomial regression allowed for adjusting of the model variance independently of the mean compared to Poisson. Social, economic, and physical environment variables were included in the model based on a-priori hypotheses (Kolak et al., 2020). The goal was to create a parsimonious model, retaining variables only at the p ≤ 0.05 level. Backwards stepwise deletion was performed. Following each regression, the most non-significant variable was removed individually. This step was then repeated until all predictors were significant at p ≤ 0.05.
Vulnerability Score and Ranking
The vulnerability score was developed using the final regression model. The coefficient of each significant indicator was used to compute each ZIP code's index score. This score was the predicted count value at the ZIP code level. This score was then converted to a rate by dividing twice the 2017 population since counts were from 2017 and 2018. This predicted rate value was then used to rank ZIP codes from highest to lowest, where higher scores indicated increased vulnerability. The top 10% of ZIP codes were designated as “most vulnerable” and the next 10% of ZIP codes were designated as “very vulnerable.”
Results
All covariates were assessed for correlation. Individual rates of chlamydia, syphilis and gonorrhea were strongly correlated (>0.90). One variable was created as an overall sexually transmitted infection rate by summing values for the number of cases of chlamydia, syphilis and gonorrhea. All other predictors were not significantly correlated (<0.65) and therefore included in the model. The remaining predictors were used to model HCV infection in those under 40 years of age as a proxy for an HIV/ HCV outbreak. Vacant housing was excluded due to not being associated with HCV infection.
An association was observed between 11 covariates and HCV detection incidence [χ2 (1358, N = 1370) = 1238.71, p ≤ 0.001]. Of these, one was health related: (1) overdose risk (fatal and nonfatal) (estimate, 0.024; P ≤ 0.0001) (Table 2). Five variables reflected social characteristics across ZCTAs: (1) percentage White (estimate, 0.015; P ≤ 0.0001); (2) percentage Hispanic (estimate, −0.009; P ≤ 0.0001); (3) percentage over 65 years of age (estimate, −0.018; P = 0.018); (4) percentage disabled (estimate, 0.038; P ≤ 0.0001) and (5) percentage 15 to 24 years of age (estimate, −0.014; P = 0.032). Three of the indicators were economic: (1) poverty rate (estimate, 0.016; P = 0.008); (2) log of per capita income (estimate, −1.059; P = 0.002); and (3) risky jobs (estimate, −0.009, P = 0.031). Two variables were physical environment indicators: (1) mobile home (estimate, 0.028; P < 0.0001) and (2) rental housing (estimate, 0.015; P < 0.0001).
When using our vulnerability ranking, the ZIP codes with highest vulnerability were found to be distributed across the state yet focused in the rural southern region (Figure 1). Ten counties in the more populated northern region of the state had at least one vulnerable ZIP code. Only one vulnerable ZIP code was in highly urbanized Cook County. Among the central region of the state, a group of vulnerable ZIP codes appeared around the third largest urban area in the state, the city of Peoria, in Peoria and Tazewell counties. Two rural counties in the western/ central region of the state, Mason and Greene counties, also had groupings of vulnerable ZIP codes. Two rural counties in the eastern region of the state, Iroquois and Ford counties, had a few vulnerable ZIP codes. Two counties in the southern, central region of the state were almost fully identified as vulnerable through their ZIP codes (Franklin and Saline County). Hardin counties, a southeastern rural county and the only Illinois, county identified by in the CDC analysis had two vulnerable ZIP codes.
Discussion
We identified communities at the ZIP code level across Illinois vulnerable to an injection drug use-related HIV outbreak using statewide data sources from 2017–2018. Our finer-scaled analysis illustrated more vulnerable regions compared to the national view, providing more precise indications of vulnerability below county scale. The CDC identified one county, Hardin County, as highest risk through their assessment (Van Handel et al., 2016). This county is the least populous county in rural, southern Illinois and shares a border with Kentucky. Out of the four ZIP codes within this county, our study found that two were of the most vulnerable ZIP codes in the entire state of Illinois. We furthermore refined our understanding of HCV detection incidence in Illinois with an extended review of multiple risk factors. Areas with more Hispanic residents, seniors, college-aged students, persons employed in “high risk jobs,” and/or higher incomes were associated with decreased HCV detection incidence, whereas the remaining covariates (opioid-related overdose, areas with more White residents, persons with a disability, mobile home structures, and/or greater proportion of rental housing) were associated with increased incidence.
Illinois has often been referred to as a microcosm of the country, being representative of the national demographics related to age, race, education, and household income. While Chicago is the third most populous city in the US, nearly two-thirds of the state's 102 counties are rural. Disparities between large metropolitan and rural areas exist for a broad swath of health outcomes much as they do nationwide. While absolute cases of chronic HCV are correlated with higher population density, acute HCV incidence in the US has been increasing with a greater predominance in rural counties in association with the opioid epidemic (Zibbell et al., 2018). Consistent with these national trends and other published vulnerability analyses, ZIP codes of high risk were clustered in rural Illinois (Wesner et al., 2020). At the same time, regions of heightened vulnerability in southern Illinois identified in previous work persist in this analysis, but are put into context within the wider state's landscape (McLuckie et al., 2019). While the spread of HIV in the country is primarily driven by sexual transmission, in non-metropolitan settings injection drug use contributes to higher rates of new diagnoses as compared to urban areas (Schranz et al., 2018). These risks are also reflected in Illinois where the rate of opioid overdose increase disproportionately burdens rural counties and were identified as the significant health-related predictor in this regression model. Factors such as limited access to OUD, HIV and HCV treatment in rural settings may contribute to worse health outcomes and increased risk9 (Illinois Department of Public Health, 2017; McLuckie et al., 2019).
Prior analyses of Illinois public health surveillance data have demonstrated other demographic variables associated with increased risk of HCV, including white race, 25–64 age group and residence in rural counties (Jones et al., 2017). In contrast to a jurisdictional vulnerability analysis performed for South Dakota where disability was noted to be protective, in our analysis self-reported disability was associated with increased vulnerability to HCV. Given the complex association of disability with substance use disorder and that both overdose-related hospitalizations and mortality have been shown to be disproportionately represented in Medicare-disability beneficiaries, our findings are plausible and suggest that this population may benefit from focused preventive interventions (Compton et al., 2007; Glazier and Kling, 2013; Peters et al., 2018; Kuo et al., 2019).
Higher income and lower percentage in poverty were protective factors, consistent with prior vulnerability analyses (Van Handel et al., 2016; Rickles et al., 2018; Sharareh et al., 2020). Risky jobs, characterized as employment in the agricultural, forestry, mining, logistics/utilities, construction, and manufacturing industries are more prevalent in non-urban Illinois10. While high risk employment may be prone to work-related injury and potentially increased utilization of prescription opioids, we found the proportion of such jobs to be protective at a ZIP code level. This may be due to the higher income and employment benefits. However, in a regional analysis we previously identified southern regions of the state with a high proportion of high-risk jobs that correlated with increased opioid-related overdose prevalence, suggesting a spatially heterogeneous effect (Kolak et al., 2020). Additionally, during the timeframe of the analysis employment in the agricultural, mining and logging, manufacturing, and construction industries were stable or increasing in the state11. It is possible that factors, such as job stability/growth and non-payroll incentives or benefits may be mediating factors. Further research is warranted to better understand the dynamics around degrees of rurality, high risk employment, and other structural benefits not reflected in per capita income in relation to disease risk.
The percentage of mobile homes and rental housing were independently associated with HCV risk suggesting that some aspects of the physical environment may impact disease vulnerability. These factors were not included in the nationwide CDC analysis, and were not significant in the county level analysis performed in Tennessee. Of note, in the South Dakota vulnerability analysis, the percent of mobile homes was associated with lower HCV infection rate in minority dominant counties, whereas in white dominant counties, this association was not significant. Given the known relationship between housing and health, this protective effect of race would be important to explore. In studies adjusted by race, homelessness and unstable housing has been shown to be associated with sharing used syringes among people who inject drugs, and higher physical health and mental health morbidity and mortality overall (Maness and Khan, 2014; Auerswald et al., 2016; Rezaei et al., 2020). Unstable housing for renting families can result in poorer health outcomes for caregivers and their children, including increased adult depression and childhood hospitalizations, and in one study the percent of mobile homes has been inversely associated with life expectancy at the census tract level (Sandel et al., 2018; Melix et al., 2020).
The findings above reveal a greater complexity underlying the interplay between demographic, socioeconomic and environmental characteristics that impact HCV risk. In our previous work focused on a predominantly rural southern areas of the state, complex heterogeneities emerged as smaller spatial units were examined and aggregated based on common environmental typologies. In short, rural areas are not monolithic, and the ability to assess risk at the ZIP code level affords jurisdictions, the capacity to finely target relatively high-risk regions regardless of county lines and of state rankings overall.
Additionally, broadening geospatial focus beyond areas of prevalent risk to those of increased vulnerability allows for more diffuse provision of preventive public health activities. State and local health departments may engage stakeholders such as community-based organizations, first responders and other health providers, to review high-risk ZIP codes that may or may not have been areas of concern based on previous service provision. Resources may be directed accordingly to build capacity and/or engage in field activities, such as disease screening as well as harm reduction services including sterile syringe provision, sexual risk reduction, and overdose education and naloxone distribution. The attention to prevention activities on vulnerable and potentially overlooked areas provides an important opportunity to expand investigation beyond active outbreaks as detected through traditional public health data, such as passive HCV and HIV surveillance, syndromic surveillance and overdose surveillance using emergency medical services and law enforcement sources.
Our analysis has several limitations. Associations between the variables and HIV/HCV vulnerability should not be considered causal. HCV infection is known to be under reported, although it is unclear how this may vary across demographic and risk-related variables (Klevens et al., 2014). We did not have access to opioid prescribing data at the time of this analysis. Variables including opioid analgesic dosing and prescriptions per person were significant in the prior vulnerability analyses (Van Handel et al., 2016; Rickles et al., 2018; Wesner et al., 2020). Fatal overdoses in Illinois have been shown to be heterogeneous in type of opioid (prescription vs. illicit) involved, history of antecedent opioid prescribing and race (Abbasi et al., 2020). In prior analyses of the Illinois Prescription Drug Monitoring Program, rural counties in southern areas had disproportionately higher opioid prescription rates, supporting our findings of vulnerability in these ZIP codes12. Future analysis including this data would help elucidate the impact of opioid prescribing on HCV risk. Finally, we did not have access to HCV nor HIV treatment data. These data are incompletely available in public health surveillance datasets and are resource intensive to collect (Ly et al., 2015). Given the history of highly restrictive prior authorization criteria for direct acting antiviral medications used for HCV required by the Illinois Medicaid program, and the well-accepted strategy of treatment as prevention for HIV, incorporating this information into future models could present a more complete assessment of risk (Dieffenbach and Fauci, 2009; Granich et al., 2009; Montaner, 2011; Barua et al., 2015). Identifying vulnerable ZIP codes through our analytical approach may allow local jurisdictions to focus their limited resources on collecting treatment data at a hyper local level.
Our study updates prior, US-wide county level analysis of geospatial risk for HCV and HIV outbreak related to injection drug use with a fine-scale approach. We validated and extended previous findings to identify additional areas of vulnerability. Priority recommendations defined by the state as a result of the analysis include increasing access to harm reduction services, specifically sterile syringe services, naloxone access, infectious disease screening and increased linkage to care for HCV and opioid use disorder13. ZIP code level rankings allow local public health jurisdictions to more finely tune surveillance and preventive measures and target activities at a sub-county level.
Data Availability Statement
The data analyzed in this study is subject to the following licenses/restrictions: confidential infectious disease reporting data. Requests to access these datasets should be directed to Cara Jane Bergo, Y2FyYS5iZXJnb0BpbGxpbm9pcy5nb3Y=.
Author Contributions
MP and MK helped form this paper and the overall project. SH and JE helped allow data access and explore ways to implementation, also were consistent editors of the paper. CB worked on the analyses of this paper. All authors contributed to the article and approved the submitted version.
Funding
This study was funded by U.S. Centers for Disease Control and Prevention Cooperative Agreement for Emergency Response: Public Health Crisis Response and the National Institute on Drug Abuse (UG3DA044829, PI: MP).
Conflict of Interest
The authors declare that the research was conducted in the absence of any commercial or financial relationships that could be construed as a potential conflict of interest.
Footnotes
1. ^Centers for Disease Control and Prevention (2019). Available online at: https://www.cdc.gov/mmwr/volumes/67/wr/mm6708a6.htm (accessed October, 2020).
2. ^Centers for Disease Control and Prevention (2019). Available online at: https://www.cdc.gov/mmwr/volumes/68/wr/mm6810a6.htm?s_cid=mm6810a6_w (accessed November, 2020).
3. ^West Virginia Department of Health and Human Resources (2019). Available online at: https://oeps.wv.gov/hiv-aids/pages/default.aspx (accessed November, 2020).
4. ^Understanding Local Spatial Variation Along the Care Continuum: The Potential Impact of Transportation Vulnerability on HIV Linkage to Care and Viral Suppression in High-Poverty Areas, Atlanta, Georgia.
5. ^Illinois Department of Public Health (2020). Available online at: www.dph.illinois.gov/sites/default/files/publications/semiannual-opioid-overdose-report9292020final.pdf
6. ^Illinois Department of Public Health (2017). Available online at: https://www.dph.illinois.gov/sites/default/files/publications/publicationsdoil-opioid-data-report.pdf (accessed November, 2020).
7. ^Illinois Department of Public Health (2019). Available online at: https://dph.illinois.gov/sites/default/files/publications/nas-annual-report-march-2019.pdf (accessed November, 2020).
8. ^Illinois Department of Public Health (2018). Available online at: https://dph.illinois.gov/news/hepatitis-cases-are-increasing (accessed November 2020).
9. ^Neonatal Abstinence Syndrome Advisory Committee and Illinois Department of Public Health (2019). Available online at: https://dph.illinois.gov/sites/default/files/publications/nas-annual-report-march-2019.pdf
10. ^Illinois Institute for Rural Affairs. A Snapshot of Rural Illinois. Investing in Healthy Rural Communities: Harvesting the Power of People, Place, and Partnerships. Available online at: http://www.rwhc.com/mediasite/6-App-Chris%20Merrett_Plenary%20am.pdf
11. ^Bureau of Labor Statistics. BLS Data Finder. Available online at: https://beta.bls.gov/dataQuery/find?fq=survey:%5Bsm%5Dands=popularity:D
12. ^Opioid Prescribing in Ilinois: Examining Prescription Drug Monitoring Program Data. (2018). Available online at: https://jrsa.org/pubs/sac-digest/vol-28/il-opioidprescribing.pdf
13. ^IDPH JVA Report.
References
Abbasi, A. B., Salisbury-Afshar, E., Berberet, C. E., Layden, J. E., and Pho, M. T. (2020). Opioid prescribing patterns before fatal opioid overdose. Am. J. Prev. Med. 58, 250–253. doi: 10.1016/j.amepre.2019.09.022
Aidala, A. A., Wilson, M. G., Shubert, V., Gogolishvili, D., Globerman, J., Rueda, S., et al. (2016). Housing status, medical care, and health outcomes among people living with HIV/AIDS: a systematic review. Am. J. Public Health. 106, e1–e23. doi: 10.2105/AJPH.2015.302905
Alpren, C, Dawson, E. L., John, B., Cranston, K., Panneer, N., Dawn Fukuda, H., et al. (2020). Opioid use fueling HIV transmission in an urban setting: an outbreak of HIV infection among people who inject drugs—Massachusetts, 2015–2018. Am. J. Public Health 110, 37–44. doi: 10.2105/AJPH.2019.305366
Auerswald, C. L., Lin, J. S., and Parriott, A. (2016). Six-year mortality in a street-recruited cohort of homeless youth in San Francisco, California. PeerJ 4:e1909. doi: 10.7717/peerj.1909
Barua, S., Greenwald, R., Grebely, J., Dore, G. J., Swan, T., and Taylor, L. E. (2015). Restrictions for medicaid reimbursement of sofosbuvir for the treatment of hepatitis C virus infection in the United States. Ann. Intern. Med. 163, 215–23. doi: 10.7326/M15-0406
Brady, J. E., Giglio, R., Keyes, K. M., DiMaggio, C., and Li, G. (2017). Risk markers for fatal and non-fatal prescription drug overdose: a meta-analysis injury. Epidemiology 4:24. doi: 10.1186/s40621-017-0118-7
Compton, W. M., Thomas, Y. F., Stinson, F. S., and Grant, B. F. (2007). Prevalence, correlates, disability, and comorbidity of DSM-IV drug abuse and dependence in the United States: results from the national epidemiologic survey on alcohol and related conditions. Arch. Gen. Psychiatry 64, 566–576. doi: 10.1001/archpsyc.64.5.566
Des Jarlais, D. C., Sypsa, V., Feelemyer, J., Abagiu, A. O., Arendt, V., Broz, D., et al. (2020). HIV outbreaks among people who inject drugs in Europe, North America, and Israel. Lancet 7, e434–e442. doi: 10.1016/S2352-3018(20)30082-5
Dieffenbach, C. W., and Fauci, A. S. (2009). Universal voluntary testing and treatment for prevention of HIV transmission. JAMA 301, 2380–2382. doi: 10.1001/jama.2009.828
Flores, M. W., Lê Cook, B., Mullin, B., Halperin-Goldstein, G., Nathan, A., Tenso, K., et al. (2020). Associations between neighborhood-level factors and opioid-related mortality: a multi-level analysis using death certificate data. Addiction 115, 1878–1889. doi: 10.1111/add.15009
Glazier, R., and Kling, R. (2013). Recent trends in substance abuse among persons with disabilities compared to that of persons without disabilities. Disabil. Health J. 6, 107–115. doi: 10.1016/j.dhjo.2013.01.007
Granich, R. M., Gilks, C. F., Dye, C., De Cock, K. M., and Williams, B. G. (2009). Universal voluntary HIV testing with immediate antiretroviral therapy as a strategy for elimination of HIV transmission: a mathematical model. Lancet 373, 48–57. doi: 10.1016/S0140-6736(08)61697-9
Haley, S. J., Maroko, A. R., Wyka, K., and Baker, M. R. (2019). The association between county-level safety net treatment access and opioid hospitalizations and mortality in New York. J. Substance Abuse Treat. 100, 52–58. doi: 10.1016/j.jsat.2019.02.004
Havens, J. R., Walsh, S. L., Korthuis, P. T., and Fiellin, D. A. (2018). Implementing treatment of opioid-use disorder in rural settings: a focus on HIV and hepatitis C prevention and treatment. Curr. HIV/AIDS Rep. 15, 315–323. doi: 10.1007/s11904-018-0402-3
Illinois Department of Public Health (2017). State of Illinois Comprehensive Opioid Data Report. Illinois Department of Public Health. Available online at: https://www.dph.illinois.gov/sites/default/files/publications/publicationsdoil-opioid-data-report.pdf (accessed November 20, 2020).
Jones, T., Hoferka, S., Kauerauf, J., Layden, J., and Pho, M. T. (2017). “Changing epidemiology of Hepatitis C in Illinois. Late-Breaker abstract #7,” in Annual Meeting of the American Public Health Association. Atlanta, GA.
Joudrey, P. J., Edelman, E. J., and Wang, E. A. (2019). Drive times to opioid treatment programs in urban and rural counties in 5 US states. JAMA 322, 1310–1312. doi: 10.1001/jama.2019.12562
Keyes, K., Cerdá, M., Brady, J., Havens, J., and Galea, S. (2014). Understanding the rural–urban differences in nonmedical prescription opioid use and abuse in the United States. Am. J. Public Health 104, e52–e59. doi: 10.2105/AJPH.2013.301709
Klevens, R. M., Liu, S., Roberts, H., Jiles, R. B., and Holmberg, S. D. (2014). Estimating acute viral hepatitis infections from nationally reported cases. Am. J. Public Health 104, 482–7. doi: 10.2105/AJPH.2013.301601
Kolak, M. A., Chen, Y. T., Joyce, S., Ellis, K., Defever, K., McLuckie, C., et al. (2020). Rural risk environments, opioid-related overdose, and infectious diseases: a multidimensional, spatial perspective. Int. J. Drug Policy 5:102727. doi: 10.1016/j.drugpo.2020.102727
Kuo, Y. F., Raji, M. A., and Goodwin, J. S. (2019). Association of disability with mortality from opioid overdose among US medicare adults. JAMA Netw Open 2:e1915638. doi: 10.1001/jamanetworkopen.2019.15638
Lerner, A. M., and Fauci, A. S. (2019). Opioid injection in rural areas of the United States: a potential obstacle to ending the HIV epidemic. JAMA 322, 1041–1042. doi: 10.1001/jama.2019.10657
Ly, K. N., Hughes, E. M., Jiles, R. B., Klevens, R. M., Roberts, H., and Teshale, E. (2015). Surveillance for Hepatitis C Global Virology I - Identifying and Investigating Viral Diseases. Internet Interventions, 93–124. doi: 10.1007/978-1-4939-2410-3_6
Maness, D. L., and Khan, M. (2014). Care of the homeless: an overview. Am. Fam. Physician 89, 634–640.
McLuckie, C., Pho, M. T., Ellis, K., Navon, L., Walblay, K., Jenkins, W. D., et al. (2019). Identifying areas with disproportionate local health department services relative to opioid overdose, HIV and hepatitis C diagnosis rates: a study of rural Illinois. Int. J. Environ. Res. Public Health 16:989. doi: 10.3390/ijerph16060989
Melix, B. L., Uejio, C. K., Kintziger, K. W., Reid, K., Duclos, C., Jordan, M. M., et al. (2020). Florida neighborhood analysis of social determinants and their relationship to life expectancy. BMC Public Health. 20:632. doi: 10.1186/s12889-020-08754-x
Montaner, J. S. G. (2011). Treatment as prevention: a double hat-trick. Lancet. 378, 208–209. doi: 10.1016/S0140-6736(11)60821-0
Mumtaz, G. R., Weiss, H. A., Vickerman, P., Larke, N., and Abu-Raddad, L. J. (2015). Using hepatitis C prevalence to estimate HIV epidemic potential among people who inject drugs in the Middle East and North Africa. AIDS 29, 1701–1710. doi: 10.1097/QAD.0000000000000761
Onofrey, S., Aneja, J., and Haney, G. A. (2015). Underascertainment of acute hepatitis C virus infections in the U.S. surveillance system: a case series and chart review. Ann. Intern. Med. 163, 254–261. doi: 10.7326/M14-2939
Peters, J. L., Durand, W. M., Monteiro, K. A., Dumenco, L., and George, P. (2018). Opioid overdose hospitalizations among Medicare-disability beneficiaries. J. Am. Board Fam. Med. 31, 881–896. doi: 10.3122/jabfm.2018.06.180152
Ramachandran, S., Thai, H., Forbi, J. C., Galang, R. R., Dimitrova, Z., Xia, G. L., et al. (2018). A large HCV transmission network enabled a fast-growing HIV outbreak in rural Indiana, 2015. EBioMedicine 37, 374–381. doi: 10.1016/j.ebiom.2018.10.007
Rezaei, O., Ghiasvand, H., Higgs, P., Noroozi, A., Noroozi, M., Rezaei, F., et al. (2020). Factors associated with injecting-related risk behaviors among people who inject drugs: a systematic review and meta-analysis study. J. Addict. Dis. 38, 420–437. doi: 10.1080/10550887.2020.1781346
Rhodes, T. (2009). Risk environments and drug harms: a social science for harm reduction approach. Int. J. Drug Policy 20, 193–201. doi: 10.1016/j.drugpo.2008.10.003
Rickles, M., Rebeiro, P. F., Sizemore, L., Juarez, P., Mutter, M., Wester, C., et al. (2018). Tennessee's in-state vulnerability assessment for a “rapid dissemination of human immunodeficiency virus or hepatitis C virus infection” event utilizing data about the opioid epidemic. Clin. Infect. Dis. 66, 1722–1732. doi: 10.1093/cid/cix1079
Sandel, M., Sheward, R., Cuba, S. E., Coleman, S. M., Frank, D. A., Chilton, M., et al. (2018). Unstable housing and caregiver and child health in renter families. Pediatrics 141:2199. doi: 10.1542/peds.2017-2199
Schalkoff, C. A., Lancaster, K. E., Gaynes, B. N., Wang, V., Pence, B. W., Miller, W. C., et al. (2020). The opioid and related drug epidemics in rural Appalachia: a systematic review of populations affected, risk factors, and infectious diseases. Substance Abuse 41, 35–69. doi: 10.1080/08897077.2019.1635555
Schranz, A. J., Barrett, J., Hurt, C. B., Malvestutto, C., and Miller, W. C. (2018). Challenges facing a rural opioid epidemic: treatment and prevention of HIV and hepatitis C. Curr. HIV/AIDS Rep. 15, 245–254. doi: 10.1007/s11904-018-0393-0
Sharareh, N., Hess, R., White, S., Dunn, A., Singer, P. M., and Cochran, J. (2020). A vulnerability assessment for the HCV infections associated with injection drug use. Prev. Med. 134:106040. doi: 10.1016/j.ypmed.2020.106040
Shepard, C. W., Finelli, L., and Alter, M. J. (2005). Global epidemiology of hepatitis C virus infection. Lancet Infect. Dis. 5, 558–567. doi: 10.1016/S1473-3099(05)70216-4
Siegler, A. J., Bratcher, A., and Weiss, K. M. (2019). Geographic access to preexposure prophylaxis clinics among men who have sex with men in the United States. Am. J. Public Health 109, 1216–1223. doi: 10.2105/AJPH.2019.305172
Van Handel, M. M., Rose, C. E., Hallisey, E. J., Kolling, J. L., Zibbell, J. E., Lewis, B., et al. (2016). County-level vulnerability assessment for rapid dissemination of HIV or HCV infections among persons who inject drugs, United States. J. Acquir. Immune Defic. Syndr. 73, 323–331. doi: 10.1097/QAI.0000000000001098
Wesner, C. A., Zhang, W., Melstad, S., Ruen, E., Deffenbaugh, C., Gu, W., et al. (2020). Assessing county-level vulnerability for opioid overdose and rapid spread of human immunodeficiency virus and hepatitis C infection in South Dakota. J. Infect. Dis. 222(Suppl.5), S312–S321. doi: 10.1093/infdis/jiaa231
Zibbell, J. E., Asher, A. K., Patel, R. C., Kupronis, B., Iqbal, K., Ward, J. W., et al. (2018). Increases in acute hepatitis C virus infection related to a growing opioid epidemic and associated injection drug use, United States, 2004 to 2014. Am J Public Health. 108, 175–181. doi: 10.2105/AJPH.2017.304132
Keywords: injection drug abuse, HIV, vulnerability analysis, outbreak, infectious disease, hepatitis C (HCV) infection
Citation: Bergo CJ, Epstein JR, Hoferka S, Kolak MA and Pho MT (2021) A Vulnerability Assessment for a Future HIV Outbreak Associated With Injection Drug Use in Illinois, 2017–2018. Front. Sociol. 6:652672. doi: 10.3389/fsoc.2021.652672
Received: 12 January 2021; Accepted: 07 April 2021;
Published: 19 May 2021.
Edited by:
David Charles Perlman, Mount Sinai Medical Center, United StatesReviewed by:
Kimberly Page, University of New Mexico Health Sciences Center, United StatesJonathan Feelemyer, New York University, United States
Copyright © 2021 Bergo, Epstein, Hoferka, Kolak and Pho. This is an open-access article distributed under the terms of the Creative Commons Attribution License (CC BY). The use, distribution or reproduction in other forums is permitted, provided the original author(s) and the copyright owner(s) are credited and that the original publication in this journal is cited, in accordance with accepted academic practice. No use, distribution or reproduction is permitted which does not comply with these terms.
*Correspondence: Cara Jane Bergo, Y2JlcmdvMkBnbWFpbC5jb20=