- 1Space Weather Laboratory, NASA Goddard Space Flight Center, Greenbelt, MD, United States
- 2Department of Physics, The Catholic University of America, Washington DC, WA, United States
Freedom to explore the unknown is key to scientific discovery. Maximizing modern individualistic measures of scientific productivity like the number of citations and publications may impede the progress of science as a whole.
1 Scientific discovery
Perhaps it is reasonable to categorize scientific endeavors into two essential elements: discovery and assimilation. Discoveries entail breakthroughs, e.g., finding novel truths about our Universe, constructing new theories that explain phenomena, finding new materials, identifying new pathogens, and uncovering new cures for old diseases. While assimilation involves putting to use this newly found knowledge: e.g., building a global positioning system, advancing a theory to new regimes, or making a medical cure cheaper and more effective. With this framework in mind, we can ask whether the requirements for making discoveries are the same as for assimilating new knowledge.
A perspective I present in this paper is that the requirements for making discoveries are qualitatively different from the assimilation phase of scientific progress. Continuous conceptual breakthroughs of the kind we saw a century ago, like quantum mechanics, relativity, genetic inheritance, psychoanalysis, or statistical mechanics, are not common in the present day (Graeber, 2012). Scientific discoveries appear to happen in bursts with even a certain quasi-periodicity (Jaynes, 1967), and many are accidental (Humberstone, 1943; Gambardella et al., 2005), unlike the directed efforts of implementing existing knowledge in the assimilation stage. Hence, scientific discoveries may benefit from many scientists wandering and exploring freely, driven by curiosity, in the vast n-dimensional space of knowledge. By following this line of thought, we notice two surprising possibilities: 1) The central aspects of the current practice of modern science, like ‘publish or perish’ or ‘citation maximization’, might be holding back discoveries and breakthroughs, 2) What appears to be “productive” for scientists, based on our current individualistic metrics of productivity, may be unproductive for scientific discovery as a whole.
2 A thought experiment: Discovery through random walks
I explain the logical basis for these possibilities by constructing a simple thought experiment that captures an aspect of scientific discoveries: its accidental nature. In our toy model, scientists (point particles) carry out random walks to explore an abstract knowledge space subject to non-random pressures associated with the need to maximize citations. The thought experiment’s only purpose is to provide an analogy that helps us understand how the pressure to maximize specific individual metrics of agents (in our case, scientists) need not necessarily maximize the goals of the system as a whole.
Consider an abstract space of knowledge that remains unexplored in the beginning. Such spaces are perhaps n-dimensional, with many degrees of freedom for scientists to traverse along some continuous paths. For simplicity, let us assume scientists are restricted to traveling only along a 2D plane in this abstract space.
Figure 1A depicts such a space of knowledge, with some beneficial knowledge considered by scientists as important and ground-breaking and others considered not-so-useful and not to be of much immediate or apparent value. Regions within black circles represent discoveries of significant magnitude and the scientific field or sub-field that develops around them. These knowledge circles are separated by distance, representing a barrier of knowledge considered “not-so-useful” to be gained before reaching one. In other words, one needs to traverse through knowledge that might not be considered breakthroughs to go from knowing thermodynamics to discovering quantum mechanics. In fact, this construction implies that knowledge considered “not-so-useful” might indeed be actually useful and essential to the making of a discovery. Note that in the knowledge space, knowledge can be uncovered that is not true, and what is considered true might change within the scientific community over time. The model does not discriminate between truth and false knowledge.
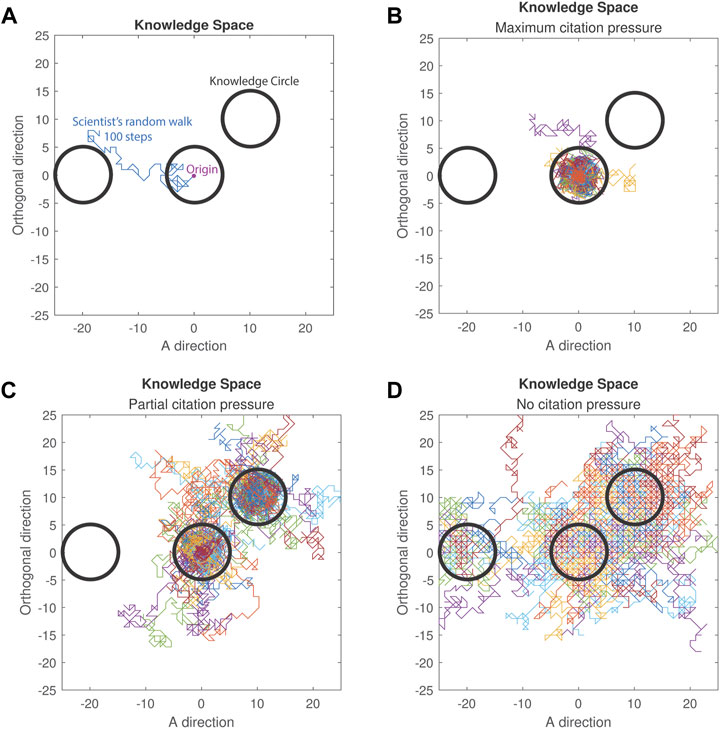
FIGURE 1. Sample runs of the thought experiment showing scientists discover more when citation pressure reduces. (A) Shows the abstract knowledge space, with knowledge circles where important breakthroughs reside, and a scientist’s random path within the space for a duration of 100 steps. (B) Simulation of 100 scientists paths with maximum citation pressure, forcing most scientists to keep exploring within their starting knowledge circle. (C) Simulation with partial citation pressure, with scientists discovering a new knowledge circle within the same simulation time frame. (D) When there is no citation pressure, scientists discover all knowledge circles within the simulation space during the same simulation time.
In this toy model, all scientists with similar education and basic knowledge start at the center of the coordinate system. The horizontal axis of the plot is simply an arbitrary direction in the knowledge space, with the vertical direction being orthogonal to it. After a scientist appears at the center, they traverse this space through a random walk, with a step in the horizontal or vertical direction that can be either [+1,0,−1] with equal probability. In a random walk, the previous step influences the next step. Though it need not be what happens in reality, in this thought experiment, this is the default motion of a scientist in the knowledge space. It does not imply that the thinking of a scientist is random; instead, it represents scientists’ trajectories that are affected by their particular conscious decisions and factors unknown to us.
If a scientist encounters a new knowledge circle, that event is a “discovery”. The time taken for the first such event since the start of the simulation is the “time to first discovery”. A scientist takes 100 steps in the simulation. After that, it is equally likely that a new scientist will appear at the center of any discovered knowledge circle.
Now, we add some directionality to scientists, perhaps analogous to the real world. For each step a scientist takes, we assume they find something and publish it. If inside a knowledge circle, this publication can gather citations probabilistically from every publication or step by future scientists within the circle. In the toy model, there is a 50–50 chance that a future scientist’s publication might cite a scientist’s current publication or step.
To model the pressure to maximize one’s performance metric in terms of publications and citations, each scientist feels a constant pull towards the center of the knowledge circle they currently exist within. Therefore, scientists in a circle carry out a random walk with a bias toward the center of the circle. I specify the degree of this pull using a parameter called citation pressure, which can vary from no citation pressure to maximum citation pressure. This pressure is analogous to the incentives scientists have towards conducting research that can garner publications and citations from other scientists. Hence it becomes vital to carry out research close to or considered important by other scientists. There are similar pressures scientists feel to propose scientific projects that are close to what other scientists work on and think is essential so that peer reviewers assigned by funding agencies to review your proposals are likely to consider the proposal important.
Assumptions and limitations of the thought experiment: A primary assumption of the thought experiment is that citation pressure forces scientists to remain close to existing fields or breakthroughs. This is described in the toy model as a bias in the random walk towards the center of the knowledge circle. The intuition for this assumption stems from my personal experience. As one attempts to pursue research topics farther away from established areas of research where most scientists spend their time, there are far fewer scientists who may cite your work. But if one does work related to and supportive of most scientists’ projects, your paper is more likely to receive citations by them. Pressures to be awarded grants or even to form successful collaborations also appear to incentivize one to remain close to the established research areas, as most of your collaborators and peer reviewers are also likely to work within the established areas of the field. Our toy model allows us to explore what might happen to scientific discovery when these pressures exist in the manner described here or do not exist. A major limitation in the scope of the toy model is that it is not a good model of science, and it is not intended to be. Instead, it is an abstract thought experiment meant to demonstrate certain specific mechanisms of the workings of science. Significant work is needed before one can upgrade this toy model into a realistic model of scientific progress, which is outside the scope of this perspective paper (See Supplementary Material for details). However, there are studies in the field of the Science of Science (SciSci) that provide results that support the mechanism demonstrated by the toy model Fortunato et al. (2018).
3 Result: Citation pressure delays discovery
We run the above toy model for different citation pressures, with 100 scientists taking 100 steps each. Figure 1B shows what happens when citation pressure is set to maximum, i.e., the highest pull towards the center of the knowledge circle. Almost all scientists remain within the starting knowledge circle. In Figure 1C, more scientists wander out of the first knowledge circle as citation pressure is lower. One scientist discovers a new knowledge circle at some point, and new scientists appear within the newly discovered knowledge circle. Figure 1D shows what happens when there is no citation pressure: scientists will most likely discover all the knowledge circles within the simulation space. These sample runs suggest that discovery is least likely under maximum citation pressure. These figures demonstrate the intuition that if citation pressure makes scientists spend more time close to established research areas, the likelihood of making breakthroughs might decrease. Studies in the field of SciSci appears to agree with this insight as well. A comprehensive review of the field by Fortunato et al. (2018) states “…measurements indicate that scholars are risk-averse, preferring to study topics related to their current expertise, which constrains the potential of future discoveries.”
To further investigate this qualitative notion, we delve deeper by running the toy model several times to gather statistics of three relevant and interesting parameters:
1. Time to the first discovery
2. Average citations per scientist
3. Share of time “wasted” by all scientists
The time to first discovery is the simulation time it takes for any scientist to first chance upon a new knowledge circle. The smaller this value is, the more productive science is in making discoveries as a whole. The average citations per scientist is the total citations gathered by all scientists divided by the total number of scientists in that model run. This metric measures how well individual scientists are doing on average, based on the belief that citations are a good measure of a scientist’s productivity. The share of time “wasted” by all scientists is the percent of simulation time where scientists’ work does not gather any citation. It is the time spent by scientists outside the knowledge circles. The complement of “wasted” time is “productive” time, which is the share of time scientists spend within knowledge circles. The term productive and wasted are placed in quotations to emphasize the subjectivity of this term, i.e., they are perceived to be productive or wasted time, but they need not be in reality. Not many scientists will deny that in the modern academic world, the number of papers and citations is considered a proxy for the “productivity” of scientists, even though it does not necessarily mean more progress toward scientific discoveries.
We run the simulation 30 times, each with different citation pressures to generate the statistics displayed in Figure 2. Figure 2A shows that the time to first discovery increases with citation pressure. In fact, it increases exponentially when citation pressure is greater than 1. Each dot represents data from a simulation run. For high citation pressures, no discovery is made during the entire simulation time, like in Figure 1B. Therefore, there are many data points with a time to first discovery greater than or equal to the simulation time of 100 × 100 = 104 units.
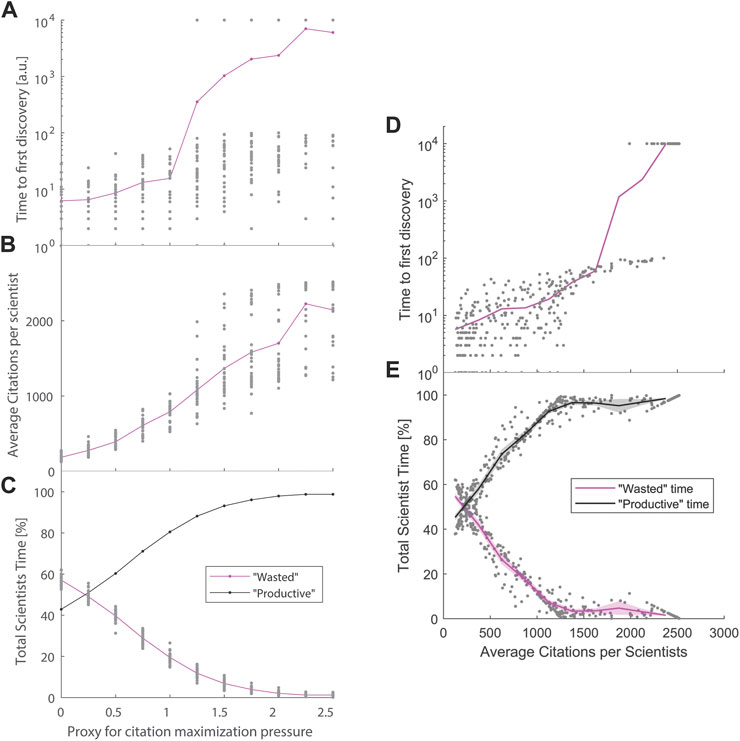
FIGURE 2. Statistics from an ensemble of model runs for different citation pressures. (A) The time to the first discovery increases with increasing citation pressure. (B) Average citation per scientist tracks the citation pressure parameter in the toy model. (C) Fraction of total “productive” time or share of total time spent gathering citations increases with increasing citation pressure. (D) Time to first discovery is longer with increasing average citations per scientist. (E) Fraction of total “productive” time increases with average citations per scientist.
Figure 2B shows that average citations per scientist increase linearly with citation pressure. This confirms that the proxy for citation pressure: the pull towards the center of the knowledge circle, is consistent with an increase in the average number of citations for scientists. Figure 2C conveys that the share of time “wasted” decreases with increasing citation pressure, which is consistent with the modern definitions of scientific productivity. As the notion of “productive” time also increases with citation pressure.
By combining Figures 2A–C, we can conclude that when most scientists in this toy model appear productive, it takes longer for the society of scientists to discover an entirely new knowledge circle. A more direct description of this result is shown in Figures 2D, E. They show that the time to first discovery is indeed delayed as average citations per scientist are higher in the simulation. Similarly, as average citations per scientist are higher, the total share of time scientists are individually productive based on their publications gathering citations is also higher. By combining the results of Figures 2D, E, we can conclude that when a large share of scientists’ time appears to be “productive”, there is a large delay in the time to first discovery. Or when science is most productive, i.e., when breakthroughs are being made rapidly, a large share of scientists’ time might appear “wasted” as they are wandering outside knowledge circles.
4 The wanderer and the climber
All scientists in the toy model, and perhaps in the modern academic world, spend time wandering and climbing. As wanderers, scientists venture outside knowledge circles, but as climbers, scientists spend time within knowledge circles publishing papers that gather citations. Consider the example of Isaac Newton, who made revolutionary scientific discoveries. Though it is common to ascribe his genius to certain individual characteristics and less to chance, we must consider the possibility that his genius might be attributed to his interest and commitment to wandering (Grassmann, 2022). In fact, a larger fraction of senior physicists are likely to think that “you need to have an innate gift or talent” to “succeed in physics” than students or post-docs (See Supplementary Material for details of a survey by Leslie et al. (2015)). Newton spent considerable effort exploring alchemy (Schettino, 2017), and the biblical apocalypse (Snobelen, 2016). Are we to consider these endeavors to be outside his genius? Or perhaps, was Newton doing what he was good at doing - wandering? Newton most certainly also spent time climbing, aided by his successful discoveries. I choose the word ‘climb’ here to illuminate other pressures that mimic the citation pressure that biases scientists to be within knowledge circles.
4.1 The ladder
In modern societies, social power hierarchies are pervasive. In one’s workplace, for example, one might find “higher ups” who receive more pay, who has more say in decision-making, and perhaps even decides whether you keep your job or not. Rules are in place that grants more power to some people over others. In academia, similar power inequalities exist without question. The review by Fortunato et al. (2018) also mentions the phenomena: “Science often behaves like an economic system with a one-dimensional “currency” of citation counts. This creates a hierarchical system, in which the “rich-get-richer” dynamics suppress the spread of new ideas, particularly those from junior scientists and those who do not fit within the paradigms supported by specific fields.” Academic ranks and titles like Dr. or Prof. announce such power differentials. The higher up you are in this power ladder, the more pay you are likely to receive and the more influence you have over the direction of science and even the direction of scientists below the ladder. In such systems, there is a clear incentive to climb the ladder. So how does a scientist climb the ladder fairly? Via widely accepted mechanisms of competition.
4.2 The climbing
The capitalist world widely considers competition necessary because we are told it is for the greater good, i.e., good of the society and the system at large. The promise is that the competition will select better scientists. And the better scientists are rewarded with a promotion up the ladder: from a graduate student to a postdoc, to assistant professor, to professor, to Director, and so on. What are the mechanisms of competition? This is, of course, complex, but the primary metric considered during competition within academia is the number of published papers and the number of citations gathered. Other metrics include successful grants, prestigious awards, your network of collaborators, institutional affiliations, your reputation, and the reputation of your mentors. I’d argue that the pressure to maximize the above parameters is roughly aligned with the direction of citation pressure in the toy model, i.e. towards the center of the knowledge circle. For example, one is more likely to develop a network of reputed collaborators or be affiliated to a reputed institution, if one is within established areas of research that garner lot of interest from the scientific community.
Whatever one might think of the utility of competition within science, we might all agree that competition is good, at least in selecting people who are good at the competition. And as I discussed previously, the best way to climb the ladder is to win competitions, so competitions incentivize scientists to spend their time climbing. If climbing is incentivized, then wandering is disincentivized. Wanderers may quickly find themselves at a disadvantage depending on the degree of competitiveness in the system.
If the insight from the toy model is true, then it would imply that it is better for science and scientific discovery for more scientists to spend their time wandering than climbing. But if competition forces and incentivizes scientists to climb rather than wander, we are compelled to ask the question: is competition good for science, or is it just good at producing competitors?
One interesting thing to note here is that no matter the citation pressure, only a maximum of two scientists will discover new knowledge circles in the toy model. The overwhelming majority of wanderers will not chance upon a revolutionary discovery. However, with more wandering scientists, the rate of discovery increases for science as a whole.
5 Conclusion
Science is a collective enterprise. If not, it ought to be. We will all be better off for it. Competition and the pressures it places on scientists beyond a threshold may be detrimental to scientific progress. The more pressure there is in our academic system to maximize citations, the more we might dampen the pace of scientific discovery and breakthroughs. What appears “productive” for individual scientists may not necessarily be productive for science.
To foster discovery, we ought to move towards a more egalitarian social system where free exploration is not discouraged by the rules of interactions that make up the system. The reader might expect me to suggest ways to achieve this, e.g., that we should consider better ways to quantify (or even not quantify) the degree of novelty of a given research or set up a grant solicitation that randomly distributes awards to high-risk proposals. But I refrain from making such recommendations, as they may not lead to what we intend. Before one examines how we can modify the rules to foster scientific discovery and free scientists to explore the unknown, we should investigate the systems-level effects of the rules we set for conducting science in greater detail.
Data availability statement
The original contributions presented in the study are included in the article/Supplementary Material; further inquiries can be directed to the corresponding author.
Author contributions
NS conceptualized, and wrote this manuscript.
Funding
NASA Cooperative Agreement 80NSSC21M0180G: Partnership for Heliophysics and Space Environment Research.
Acknowledgments
This paper was inspired by an online lecture given by Professor David Graeber. I acknowledge useful discussions with David Sibeck, Varsha Subramanyan, Lauri Holappa, Pranay Patil, Dogacan Su Ozturk, Gonzalo Cucho-Padin, Poorya Hosseini, Dolon Bhattacharyya, Maria-Theresia Walach, Gang Kai Poh, Katrina Bossert, and Nitin Prasad.
Conflict of interest
The author declares that the research was conducted in the absence of any commercial or financial relationships that could be construed as a potential conflict of interest.
Publisher’s note
All claims expressed in this article are solely those of the authors and do not necessarily represent those of their affiliated organizations, or those of the publisher, the editors and the reviewers. Any product that may be evaluated in this article, or claim that may be made by its manufacturer, is not guaranteed or endorsed by the publisher.
Supplementary material
The Supplementary Material for this article can be found online at: https://www.frontiersin.org/articles/10.3389/fspas.2023.1071809/full#supplementary-material
References
Fortunato, S., Bergstrom, C. T., Börner, K., Evans, J. A., Helbing, D., Milojević, S., et al. (2018). Science of science. Science 359, eaao0185. doi:10.1126/science.aao0185
Gambardella, B. V. A., Giuri, P., and Mariani, M. (2005). The value of european patents: Evidence from a survey of european inventors.
Graeber, D. (2012). Of flying cars and the declining rate of profit. Baffler 19, 66–84. doi:10.1162/bflr_a_00003
Humberstone, T. L. (1943). Scientific discoveries by accident. Nature 151, 215–216. doi:10.1038/151215A0
Jaynes, E. T. (1967). Foundations of probability theory and statistical mechanics, 77–101. doi:10.1007/978-3-642-86102-4_6
Leslie, S. J., Cimpian, A., Meyer, M., and Freeland, E. (2015). Expectations of brilliance underlie gender distributions across academic disciplines. Science 347, 262–265. doi:10.1126/science.1261375
Keywords: discovery, scientific progress, citation, random walk, diffusion model
Citation: Sivadas N (2023) Does the way we do science foster discovery?. Front. Astron. Space Sci. 10:1071809. doi: 10.3389/fspas.2023.1071809
Received: 16 October 2022; Accepted: 02 January 2023;
Published: 18 January 2023.
Edited by:
Joseph E. Borovsky, Space Science Institute, United StatesReviewed by:
Juan Alejandro Valdivia, University of Chile, ChileCopyright © 2023 Sivadas. This is an open-access article distributed under the terms of the Creative Commons Attribution License (CC BY). The use, distribution or reproduction in other forums is permitted, provided the original author(s) and the copyright owner(s) are credited and that the original publication in this journal is cited, in accordance with accepted academic practice. No use, distribution or reproduction is permitted which does not comply with these terms.
*Correspondence: Nithin Sivadas, sivadas@cua.edu