- Faculty of Science, University of Nottingham, Nottingham, United Kingdom
Improvements in production efficiencies and profitability of products from cattle are of great interest to farmers. Furthermore, improvements in production efficiencies associated with feed utilization and fitness traits have also been shown to reduce the environmental impact of cattle systems, which is of great importance to society. The aim of this paper was to discuss selected novel monitoring systems to measure dairy cattle phenotypic traits that are considered to bring more sustainable production with increased productivity and reduced environmental impact through reduced greenhouse gas emissions. With resource constraints and high or fluctuating commodity prices the agricultural industry has seen a growing need by producers for efficiency savings (and innovation) to reduce waste and costs associated with production. New data obtained using fast, in some cases real-time, and affordable objective measures are becoming more readily available to aid farm level monitoring, awareness, and decision making. These objective measures may additionally provide an accurate and repeatable method for improving animal health and welfare, and phenotypes for selecting animals. Such new data sources include image analysis and further data-driven technologies (e.g., infrared spectra, gas analysis), which bring non-invasive methods to obtain animal phenotypes (e.g., enteric methane, feed utilization, health, fertility, and behavioral traits) on commercial farms; this information may have been costly or not possible to obtain previously. Productivity and efficiency gains often move largely in parallel and thus bringing more sustainable systems.
Introduction
New systems that provide automated and real-time information to monitor cattle are being adopted to make meat and milk production more sustainable due to economic, social, and environmental pressures. Changes that improve production efficiencies and profitability of products from cattle are of great interest to farmers, with the added benefit of efficiency savings helping to reduce the environmental impact of production (Bell et al., 2011), which has social importance e.g., air and water quality (Gunton et al., 2016). Increasing animal welfare standards, better quality of life for farm workers, enhanced traceability, and consumer confidence in livestock production are all important social considerations that new technologies can help address for high and low input systems. New tools, technology, and information can provide continuous and repeatable methods for monitoring individual animals, rather than just groups of animals, which may also improve farmer awareness, be used for farm assurance schemes and provide a reliable phenotype measurement for selecting animals. Early detection and awareness of poor health, fertility, and animal welfare will allow farmers to make informed decisions and changes.
The livestock industry has made large improvements to efficiencies over the past 60 years because of changes in breeding, nutrition, and management. However, inefficiencies still exist, for example in dairy cows genetic selection has historically favored production (e.g., milk) rather than fitness traits (e.g., fertility, lameness, mastitis) and ultimately impacting on survival (Pryce et al., 1999; Dillon et al., 2006). Cows bred for high yields are known to mobilize body fat for production in early lactation as they cannot consume enough food to meet the rapid increase in energy demands caused by the onset of lactation, resulting in negative energy balance. While a dilution in animal maintenance requirements with increased average milk yields per cow has reduced greenhouse gas emissions per unit milk (Bell et al., 2011), there is little evidence that improvements in health (e.g., lameness and mastitis) and fertility have been made during the same period (FAWC, 2009); therefore, there is potential to enhance health, fertility, and welfare leading to reduced resource use, input costs, and emissions intensity of production.
Increasing standards for health and welfare of livestock has led to considerable research activity into ways to monitor and measure a wide range of traits (e.g., associated with fertility, legs/feet, metabolism, udder, birth, feeding, behavior, milk composition, body composition) that can be used for management and genetic selection purposes, as well as parameters of public interest (Eggar-Danner et al., 2015). Bell and Wilson (2018) found that regional differences in longevity of cows exists within UK dairy herds, with cows having a shorter life (averaging 2.6 lactations) in the region with the highest milk yields and longest interval between calvings (associated with poor fertility), compared to other regions studied (about 2.8 lactations on average) with lower milk yields and calving intervals; the average number of lactations across the UK was still below three lactations when cows are expected to reach their mature and optimum level of productivity. Ultimately maintaining healthy animals will enhance production, particularly later in life from increased lifetime performance (Bell et al., 2015). Therefore, management and breeding policies should be directed toward not only increasing production but decreasing the causes of involuntary culling (fertility, lameness, and udder health) (Bell et al., 2010). Survival within a herd influences the number of replacement animals needed, which in turn influences the productivity and profitability of the herd, as at a high replacement rate the costs are high but at too low a rate the production, reproduction, or genetic improvement of the herd may be impaired (Hadley et al., 2006). In dairy cows, several countries around the world (France, Italy, Germany, Switzerland, Belgium, Australia, United States, UK, Nordic countries, Ireland, The Netherlands) now give fitness traits more emphasis and weighting in their total economic merit index for ranking cattle for genetic selection purposes (Eggar-Danner et al., 2015) and less weighting than other countries toward milk production traits (milk, fat, and protein yield) at <50% weighting in the index with The Netherlands being the lowest at about 25% weighting on production traits. Therefore, with more weighting given to fitness traits rather than production traits, the health, and fertility of animals is expected to improve in the future. Although heritabilities of fitness traits in cattle can be low compared to production traits, the large coefficient of genetic variation for traits such as mastitis (33%) and lameness (45%) suggests there is considerable potential for breeding (Pritchard et al., 2012) with the effect being permanent and cumulative. Pritchard et al. (2012) found the coefficient of genetic variation to range from 11 to 13% for moderately heritable milk production traits, but to be as little as 3% for calving interval (an indicator of fertility).
As financial pressures on farmers increases (Defra, 2018), each stockperson will be expected to look after more animals. Tools that can assist farmers in monitoring individual animals or groups will be beneficial to the animal and farmer. Enhanced monitoring tools will enable available farm labor to be targeted toward those animals that need it. For example, management at calving plays an important role in the subsequent health and reproductive performance of cattle during their lifetime (Bell and Roberts, 2007). A difficult birth can lead to tissue damage and introduce infectious microorganisms into the uterus leading to a uterine infection (Lewis, 1997; Kim and Kang, 2003). The development of precision monitoring of individual animals that are non-invasive, automated, and produce results in real-time, such as digital image applications and online measurements, are becoming more available as “machine learning” technologies develop and the cost of implementation on farms reduces. Such technologies have the potential to allow welfare and health issues to be detected quickly for more animals compared to more manual methods currently used, thus improving animal health and welfare outcomes. More intensively monitored production systems can provide data to capture a large number of phenotypic measures to manage animals and their environment (e.g., climate, plant, soil) (Figure 1). The data can potentially be combined to create monitoring systems that describe animal “wellbeing” or identify abnormal patterns by linking production (e.g., live weight, body composition change, growth rate, milk yield, and composition), fitness or functional (e.g., fertility, lameness, survival, conformation), and behavior (e.g., activity) data. The challenge to society, scientists, and farmers is to improve efficiency of food production by better matching available and appropriate resources to requirements, to optimize profit, production, and minimize pollution (from waste).
The objective of this paper was to discuss selected novel monitoring systems to measure phenotypic traits associated with dairy cows that are considered to bring more sustainable production with increased productivity and reduced environmental impact through reduced greenhouse gas emissions. Bell et al. (2018) identified the phenotypic traits of feed utilization, enteric methane emissions, body condition, health, fertility, and overall survival of dairy cows as important traits for more sustainable production on commercial farms. Novel objective ways to monitor these traits was the focus of this review.
Production Traits
Feed Utilization
With resource constraints and high or fluctuating commodity prices the agricultural industry has seen a growing need by producers to make savings in inputs costs (i.e., feed, health, and fertility). Feed inputs can account for 70% of variable input costs associated with cattle enterprises (Redman, 2015), and with feed intake being high and positively correlated with animal enteric methane emissions (Bell and Eckard, 2012), there has been considerable interest in phenotypic measurements of feed intake (Berry and Crowley, 2013; Pryce et al., 2014) and enteric methane emissions on commercial farms. Improvement in feed efficiency in non-ruminant livestock systems has been remarkable, for example, in broiler chickens the meat produced per ton of feed has nearly doubled from 85 kg/t in the 1960 s to 170 kg/t in 2005 (van der Steen et al., 2005). Optimizing the utilization of available food and its quality is important to the profitability of any production system, as well as helping to minimize the proportion of nutrients consumed by the animal that are lost to the environment. In cattle, about 35% of energy consumed in the diet can be lost in the form of enteric methane, feces, or urine and 77% of nitrogen consumed can be excreted in feces or urine (Bell et al., 2015). Measuring feed intake or feed utilization efficiency (such as residual feed intake, which is the difference between an animal's actual feed intake and its expected feed intake based on its size and growth over a defined period) for a large number of cattle is more costly than for pigs or poultry, due to the equipment needed to measure intakes of a mixed ration. Nieuwhof et al. (1992) found that feed efficiency in growing animals was correlated with feed efficiency in mature breeding and lactating animals, which is important when measuring feed efficiency as younger animals have lower feed intakes and feed consumed is largely used for maintenance and growth.
When formulating a diet to be fed to livestock, the conventional approach is to determine the least-cost ration depending on the estimated nutrient requirement of the average animal in the group based on infrequent determination of diet nutrient concentrations. This means that some animals will be underfed, and others overfed. Typically nutrient concentrations, delivered via concentrate feeds, in the diet are held constant and dependent on how often the feed is analyzed, for example frequency of forage analysis. In reality, considerable temporal variation can exist in quality of feed ingredients and diets, and among animals, and more precise determination of nutrient availability delivered at the level of the individual animal offers considerable productive, financial, and environmental benefits. Specifically, the overall benefits of more precise allocation of nutrients to animals would be to (1) improve production system sustainability by increasing feed utilization efficiency, (2) improve performance of individual animals and the herd, and (3) reduce the environmental impact of food production through less nutrient waste. Near-infrared reflectance (NIR) spectroscopy has been shown to provide a fast and reliable analytical method for analyzing feed and products of digestion (Decruyenaere et al., 2009). Such an approach could provide not only real-time nutrient concentrations in feed and excreta but a prediction of feed intake for housed and grazing animals. Furthermore, poor quality food can impair the production and wellbeing of the animal which leads to an inability to achieve desired intakes of food, therefore resulting in increased land required and reduced nutrient efficiency. Improved utilization of feed by one kilogram per year over a dairy cow's lifetime would amount to about £324,000 in increased profit to the dairy industry per year (assuming a population of 1.8 million cows in the UK), together with a potential reduction of 1.3 kg carbon dioxide equivalent emissions produced per cow per year (Bell et al., 2015).
Enteric Methane Emissions
The emissions of enteric methane from ruminant animals follow a diurnal pattern (Crompton et al., 2011; Manafiazar et al., 2017; Bell et al., 2018), with a peak in emissions after feeding followed by a decline until the next consumption of feed. The diurnal pattern is affected by feed allowance and feeding frequency (Crompton et al., 2011), and does not appear to change over time or with a change in diet (Bell et al., 2018). Historically most studies assessing methane emissions from cattle have been done using respiration chambers (Ellis et al., 2007; Yan et al., 2009, 2010), which is impractical for large-scale estimation of methane emissions by individual animals on commercial farms. Approaches to measure enteric methane emissions from individual dairy and beef cattle on commercial farms are being developed (Garnsworthy et al., 2012a,b; Lassen et al., 2012; Manafiazar et al., 2017) due to the availability of more portable gas analysis equipment and the considerable interest in the possibility of identifying high and low methane emitters for benchmarking farms, improving national emissions inventories and/or genetic selection. The frequent “spot” sampling of breath methane emissions when an animal is at a feed bin can provide repeated measurements to allow assessment of between-cow, within-cow, diet, and temporal effects on emissions when sampled over several days. The duration of sampling needed to assess variation among individual animals is dependent on the frequency of spot measurements and visits to the sampling location (Cottle et al., 2015). Garnsworthy et al. (2012a), showed that estimates of methane made during milking were correlated with total daily methane emissions by the same cows when housed subsequently in respiration chambers. Quantifying enteric methane emissions from peaks in concentration whilst feeding (Figure 2) has been demonstrated to provide repeatable phenotypic estimates of emissions (Garnsworthy et al., 2012a,b; Lassen et al., 2012).
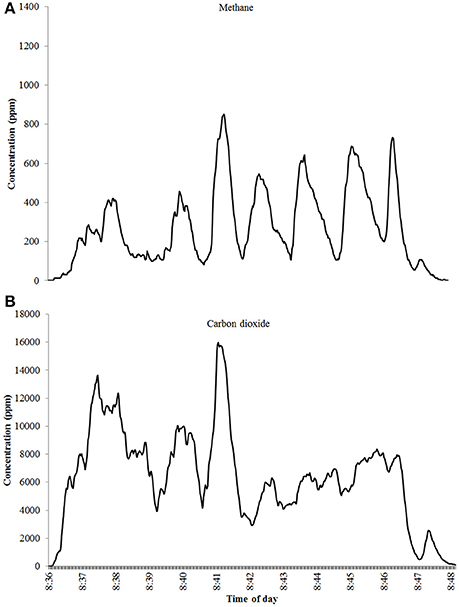
Figure 2. Concentration in parts per million of (A) methane and (B) carbon dioxide during a single milking showing peaks in breath (Bell et al., 2014).
As with NIR spectra for feed analysis, mid-infrared reflectance (MIR) spectra have gained considerable interest for identifying biomarkers in milk. Standard milk components such as fat, protein, urea, and lactose contents are routinely obtained using MIR spectroscopy. However, the potential exists for a wide range of biomarkers to be monitored using the technique (e.g., fatty acids, lactoferrin, minerals, acetone, and β-hydroxybutyrate) (Gengler et al., 2016). The calibration process for MIR spectra estimates the amount of biomarker based on specific data points within the spectra (Figure 3) (Vanlierde et al., 2016). The use of MIR spectra to estimate methane emissions is based on the relationship between changes in rumen fermentation and milk composition. As methane synthesis increases with an increase in the ratio of butyrate to propionate in the rumen, such as with increased forage intake in the diet, this causes a decrease in milk lactose content and an increase in fat content (Miettinen and Huhtanen, 1996). Machine learning on large datasets such as spectral data, accelerometer, or breath sampling can process, refine, or classify, and generate predictions from raw analytical data based on predetermined algorithms to create meaningful outputs for real-time decision making.
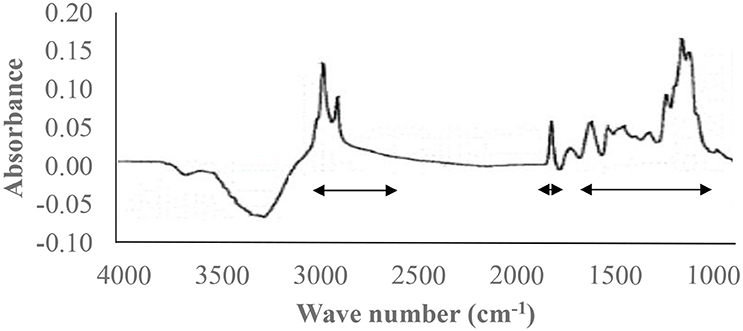
Figure 3. Mid-infrared spectra for milk (Sivakesava and Irudayaraj, 2002) with arrows indicating the three regions of the spectra for estimating enteric methane emissions.
Body Condition
Body condition scoring has traditionally been done by manual scoring of the amount of body fat reserves associated with a live animal at a given time. The scoring method provided a simiple means for farmers to manually assess the body fat of animals rather than rely on more specialized ultrasound equipment to more accurately measure body fat. This is a subjective scoring measure with potential differences in human interpretation leading to reduced reliability and repeatability. Body condition is scored using a variety of scales and approaches (Bewley et al., 2008a), but typically on a scale of extremely thin (1) to very fat (5 or 9 depending on scale adopted) in quarter intervals. The measure gained prominance as a means of monitoring changes in body fat reserves, which can alter depending on the animal's stage of production (e.g., at calving, conception, and when dried off). Also, in dairy cows, low fat levels and the mobilizing of body fat reserves for milk production has been found to have a deleterious effect on the health and fertility of the cow (Pryce et al., 1999) and lifespan. Modern high milk yielding dairy cows have a high genetic potential for mobilizing body energy reserves for milk. Automated image analysis can be used to objectively assess the body condition (e.g., fat depth) of individual animals in real-time (Bewley et al., 2008b; Halachmi et al., 2008; Azzaro et al., 2011).
Fitness Traits
New technologies are developing that provide new ways to measure fitness traits associated with farmed animals (Day, 2005; Berckmans, 2008; Wathes et al., 2008). A number of sensor technologies (Wathes et al., 2008; Neethirajan, 2017) that can be used on animals exist such as accelerometers, GPS, rumen boluses, and temperature sensors. Other technologies are emerging such as image analysis and online data sources such as spectral data. These technologies benefit from not relying on human intervention, transponder attachments, or invasive equipment (e.g., boluses, collars), and may provide more information compared to other monitoring systems at a relatively low cost. Also, some existing movement or activity sensors, such as accelerometers, are calibrated using video image material. Accelerometers provide information on both body posture (standing, lying, walking) and activity, which are used as descriptors to define behaviors, which can now also be done using live video footage. Accelerometers have provided a useful tool to help farmers to identify estrus activity in cows (Wathes et al., 2008). Data can be acquired from animals when they visit a common location such as milking station, feed, and/or water trough. A disadvantage of video image monitoring is that it is more suited to housed animal environments. Such phenotypes of interest include breath concentrations of biomarkers such as methane (energy lost from rumen fermentation) and carbon dioxide (energy lost by respiration) gas mentioned above (Bell et al., 2014), milk (Gengler et al., 2016), conformation or locomotion (Stock et al., 2017), and behavior recognition (Cangar et al., 2008) systems which filter large amounts of data to produce real-time results. Not only is milk composition affected by the genetic background of cows (e.g., breed), but also the diet they are fed, their health, and environment—therefore providing a means to monitor the status of the animal and potentially subclinical cases such as udder health.
Animal Health and Welfare
The annual cost of common health and welfare challenges in the dairy industry is considerable. With rapid developments in camera surveillance technology, machine learning and processing, and computer vision techniques, new objective methods to monitor animals are possible that can help improve early detection of health, fertility, and welfare problems. The combination of sensors i.e., images with transponder technologies, may ultimately provide a more “complete” approach to monitoring animal wellbeing but further research is needed to determine this. Using camera images to monitor animal behavior manually has been used for decades and automated monitoring of group housed pig and poultry systems is available (Wathes et al., 2008). While still developing, the automatic prediction of individual animal behavior and welfare of animals may be useful for farm assurance schemes as a repeatable, reliable and objective measure across different farm environments. As a management tool, the monitoring of cows at calving is essential to determine if there is a need for intervention, which can be hazardous for the cow, calf and stockperson. Alterations in behavior, such as standing, lying, head, and tail movements, can give an indication of the need for assistance (Hyslop et al., 2008).
Recent technological advances in the field of computer vision based on the technique of deep learning (Krizhevsky et al., 2012; Girshick et al., 2014) have emerged which now makes automated monitoring of video feeds feasible. Deep neural networks can be used for a number of animal monitoring tasks such as recognizing the type of animals (recognition), detecting where the animals (and any other objects of interest) are located in the image (detection), localizing their body parts, and even segmenting their exact shape (silhouette) from the image. See Figure 4 for an example. Furthermore, adaptations of neural networks for analyzing video can be used for a number of high level analysis tasks such as recognition of specific animal behaviors (Gkioxari et al., 2015).
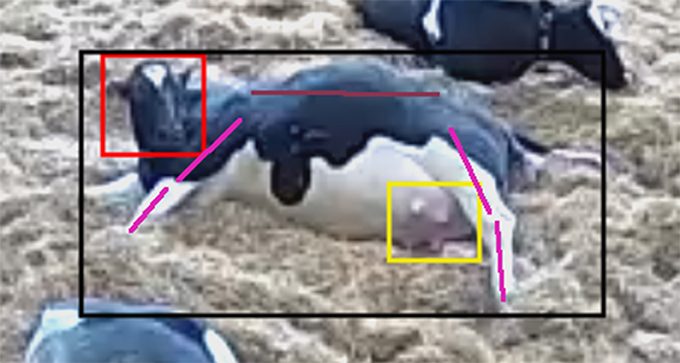
Figure 4. Cow whilst calving with location of the cow, its body parts and the configuration of the cow body (shown in terms of bounding boxes and lines) identified by computer vision from video surveillance.
A major benefit of automated image analysis is that it allows continuous monitoring for long-periods of time which is not possible for a stockperson, and can complement existing surveillance video footage accessed remotely. Image analysis can not only detect and track individuals but also groups of animals (i.e., herd, flock, or mother with offspring), which is not possible using other monitoring methods.
Combining Data Sources
Precision management systems that recognize the needs of individual animals could potentially contribute to significant reductions in feed costs and nutrients wasted, but techniques to do this require development. This approach offers increased efficiency in the use of input resources such as feed, by improved predictive capabilities and tools that allow variability among animals to be managed. Farm data, modeling, and computer programs can be integrated (Figure 5) to create a real-time system for precise allocation of food (Pomar et al., 2010). The need for testing and practical application of such an approach has been identified by others (Wathes et al., 2008; Pomar et al., 2011), before being implemented on farms. Precision feeding aims to provide a diet tailored to the requirements of an individual animal to enhance overall performance and nutrient utilization. In theory, collated real-time farm information should allow the quantity and composition of the diet to be adjusted daily to the needs of each animal on the farm. Computer-based methods of processing these data will aid the automation of feeding.
In the short-term, recording systems that obtain new information and phenotypes may provide a benchmarking or decision support system for the farmer to improve awareness and management. In the medium to long-term, recording systems may provide customized animal selection indices (Bell et al., 2013, 2015) for herd management or breeding. customized selection indices are appropriate for fitness traits with low heritability (Cottle and Coffey, 2013) or largely influenced by farm environment. A reduction in greenhouse gas emissions per unit product from dairy cows of about 1% per annum has been estimated over the last few decades because of genetic selection alone (Bell et al., 2015), with no change found in the emission intensity of beef cattle (Jones et al., 2008). Due to increasing production per animal over this same period, the emissions per cow are estimated to increase by 1.0% (Bell et al., 2015). Selection on body maintenance requirements (or live weight as an approximation for maintenance) or feed efficiency/methane could help reduce the increase in emissions per cow and per unit product.
Furthermore, automated and objective farm level recording systems may capture the effect of environment and its interaction with the genetic background of the animal. Evaluating progeny for production and fitness traits across breeds and environments fails to fully account for the effect of environment on different genotypes, and therefore there is potential for better genetic progress to be made within different production systems using customized indices. Strandberg et al. (2009) found a genotype by environment interaction for fertility traits, with days to first insemination and calving interval explaining the majority of the genotype by environment variation observed. It could be that these objective fertility traits are more accurately acquired than traits that rely on a subjective pregnancy diagnosis. Haskell et al. (2007) studied Holstein-Friesian herds and found production intensity (age at first calving, kilograms milk, milk fat, and protein production) and climate (temperature and rainfall) were the factors explaining the majority of the variation seen in production systems across the UK. Several of these variables were also common variables identified in a study on Holstein-Friesian cows across countries by Zwald et al. (2003). Zwald et al. (2003) found climatic temperature, herd size, sire for milk, percentage of North American Holstein genes, peak milk yield, fat to protein ratio in milk, and standard deviation of milk yield to be the main variables explaining the majority of variation between a genotype and its environment. Sires vary in the sensitivity of their daughters to different farm environments, with a small proportion of sires producing daughters that are less affected by their farm environment (Haskell et al., 2007) i.e., more robust animals. Therefore, identifying progeny that are more robust to a certain production system or farm environment would be beneficial to the efficiency of the system.
Concluding Remarks
This study discussed selected novel monitoring systems that have the potential to increase productivity and reduce the environmental impact of commercial cattle systems. Improvements in the production efficiency and utilization of resources needed to produce meat and milk from cattle is of great interest to farmers, policy makers, and society. New technologies are providing opportunities to objectively monitor and measure phenotypes using non-invasive methods associated with cattle that were previously seen as difficult or costly to obtain (e.g., enteric methane, feed utilization, and behavioral traits). This potentially brings new information or data sources for enhanced farm level monitoring, awareness, and decision making. For any new monitoring system it needs to easily integrate into the farm system, as well as be accurate and reliable for longevity of use. Adoption by the farmer is reliant on the perceived benefits and investment needed, which may be influenced by the production system i.e., high versus low input system. Whatever the farmers' needs might be depending on their production system, new ways of monitoring performance can complement the existing work of the farmer, especially with regard to traits that are difficult to continually monitor (e.g., feed utilization, methane emissions, body condition, animal behavior, health, and welfare).
Author Contributions
MB conducted the literature survey, collated the relevant information, and wrote the paper with GT.
Conflict of Interest Statement
The authors declare that the research was conducted in the absence of any commercial or financial relationships that could be construed as a potential conflict of interest.
Acknowledgments
The authors are grateful for the assistance by John McDonagh in creating Figure 4 from video surveillance. The video surveillance work is funded by The Douglas Bomford Trust and EPSRC. The greenhouse gas work was part of the UK Agricultural Greenhouse Gas (GHG) Research Platform project (http://www.ghgplatform.org.uk).
References
Azzaro, G., Caccamo, M., Ferguson, J. D., Battiato, S., Farinella, G. M., Guarnera, G. C., et al. (2011). Objective estimation of body condition score by modelling cow body shape from digital images. J. Dairy Sci. 94, 2126–2137. doi: 10.3168/jds.2010-3467
Bell, M. J., Craigon, J., Saunders, N., Goodman, J. R., and Garnsworthy, P. C. (2018). Does the diurnal pattern of enteric methane emissions from dairy cows change over time? Animal doi: 10.1017/S1751731118000228 [Epub ahead of print].
Bell, M. J., and Eckard, R. J. (2012). “Reducing enteric methane losses from ruminant livestock – its measurement, prediction and the influence of Diet,” in Livestock Production, ed K. Javed (Rijeka: InTech), 135–150.
Bell, M. J., Eckard, R. J., Haile-Mariam, M., and Pryce, J. E. (2013). The effect of changing cow production and fitness traits on net income and greenhouse gas emissions from Australian Dairy systems. J. Dairy Sci. 96, 7918–7931. doi: 10.3168/jds.2012-6289
Bell, M. J., Garnsworthy, P. C., Stott, A. W., and Pryce, J. E. (2015). The effect of changing cow production and fitness traits on profit and greenhouse gas emissions from UK Dairy systems. J. Agric. Sci. 153, 138–151. doi: 10.1017/S0021859614000847
Bell, M. J., and Roberts, D. J. (2007). The impact of uterine infection on a dairy cow's performance. Theriogenology 68, 1074–1079. doi: 10.1016/j.theriogenology.2007.08.010
Bell, M. J., Saunders, N., Wilcox, R., Homer, E., Goodman, J. R., Craigon, J., et al. (2014). Methane emissions among individual dairy cows during milking quantified by eructation peaks or ratio with carbon dioxide. J. Dairy Sci. 97, 6536–6546. doi: 10.3168/jds.2013-7889
Bell, M. J., Wall, E., Russell, G., Roberts, D. J., and Simm, G. (2010). Risk factors for culling in Holstein-Friesian dairy cows. Vet. Record 167, 238–240. doi: 10.1136/vr.c4267
Bell, M. J., Wall, E., Russell, G., Simm, G., and Stott, A. (2011). The effect of improving cow productivity, fertility, and longevity on the global warming potential of dairy systems. J. Dairy Sci. 94, 3662–3678. doi: 10.3168/jds.2010-4023
Bell, M. J., and Wilson, P. (2018). Estimated differences in economic and environmental performance of forage-based dairy herds across the UK. Food Energy Secur. 7:e00127. doi: 10.1002/fes3.127
Berckmans, D. (2008). Precision livestock farming. Comput. Electron. Agric. 62:1. doi: 10.1016/j.compag.2007.09.002
Berry, D. P., and Crowley, J. J. (2013). Genetics of feed efficiency in dairy and beef cattle. J. Anim. Sci. 91, 1594–1613. doi: 10.2527/jas.2012-5862
Bewley, J. M., and Schutz, M. M. (2008a). Review: an interdisciplinary review of body condition scoring for dairy cattle. Profess. Anim. Sci. 24, 507–529. doi: 10.15232/S1080-7446(15)30901-3
Bewley, J. M., Peacock, A. M., Lewis, O., Boyce, R. E., Roberts, D. J., Coffey, M. P., et al. (2008b). Potential for estimation of body condition scores in dairy cattle from digital images. J. Dairy Sci. 91, 3439–3453. doi: 10.3168/jds.2007-0836
Cangar, Ö., Leroy, T., Guarino, M., Vranken, E., Fallon, R., Lenehan, J., et al. (2008). Automatic real-time monitoring of locomotion and posture behaviour of pregnant cows to calving using online image analysis. Comput. Electron. Agric. 64, 53–60. doi: 10.1016/j.compag.2008.05.014
Cottle, D. J., and Coffey, M. P. (2013). The sensitivity of predicted financial and genetic gains in Holsteins to changes in the economic value of traits. J. Anim. Breed. Genet. 130, 41–54. doi: 10.1111/j.1439-0388.2012.01002.x
Cottle, D. J., Velazco, J., Hegarty, R. S., and Mayer, D. G. (2015). Estimating daily methane production in individual cattle with irregular feed intake patterns from short-term methane emission measurements. Animal 9, 1949–1957. doi: 10.1017/S1751731115001676
Crompton, L. A., Mills, J. A. N., Reynolds, C. K., and France, J. (2011). “Fluctuations in methane emission in response to feeding pattern in lactating dairy cows,” in Modelling Nutrient Digestion and Utilization in Farm Animals, eds D. Sauvant, J. Van Milgen, P. Faverdin, and N. Friggens (Wageningen: Wageningen Academic Publishers), 76–180.
Day, W. (2005). Engineering precision into variable biological systems. Ann. Appl. Biol. 146, 155–162. doi: 10.1111/j.1744-7348.2005.040064.x
Decruyenaere, V., Lecomte, P. H., Demarquilly, C., Aufrere, J., Dardenne, P., Stilmant, D., et al. (2009). Evaluation of green forage intake and digestibility in ruminants using near infrared reflectance spectroscopy (NIRS): developing a global calibration. Anim. Feed Sci. Technol. 148, 138–156. doi: 10.1016/j.anifeedsci.2008.03.007
Defra (2018). The Future Farming and Environment Evidence Compendium. Available online at: https://assets.publishing.service.gov.uk/government/uploads/system/uploads/attachment_data/file/683972/future-farming-environment-evidence.pdf (Accessed April 13, 2018).
Dillon, P., Berry, D. P., Evans, R. D., Buckley, F., and Horan, B. (2006). Consequences of genetic selection for increased milk production in European seasonal pasture based systems of milk production. Livest. Sci. 99, 141–158. doi: 10.1016/j.livprodsci.2005.06.011
Eggar-Danner, C., Cole, J. B., Pryce, J. E., Gengler, N., Heringstad, B., Bradley, A., et al. (2015). Invited review: overview of new traits and phenotyping strategies in dairy cattle with a focus on functional traits. Animal 9, 191–207. doi: 10.1017/S1751731114002614
Ellis, J. L., Kebreab, E., Odongo, N. E., McBride, B. W., Okine, E. K., and France, J. (2007). Prediction of methane production from dairy and beef cattle. J. Dairy Sci. 90, 3456–3467. doi: 10.3168/jds.2006-675
Garnsworthy, P. C., Craigon, J., Hernandez-Medrano, J. H., and Saunders, N. (2012a). Variation among individual dairy cows in methane measurements made on farm during milking. J. Dairy Sci. 95, 3181–3189. doi: 10.3168/jds.2011-4606
Garnsworthy, P. C., Craigon, J., Hernandez-Medrano, J. H., and Saunders, N. (2012b). On-farm methane measurements during milking correlate with total methane production by individual dairy cows. J. Dairy Sci. 95, 3166–3180. doi: 10.3168/jds.2011-4605
Gengler, N., Soyeurt, H., Dehareng, F., Bastin, C., Colinet, F., Hammami, H., et al. (2016). Capitalizing on fine milk composition for breeding and management of dairy cows. J. Dairy Sci. 99, 4071–4079. doi: 10.3168/jds.2015-10140
Girshick, R., Donahue, J., Darrell, T., and Malik, J. (2014). “Rich feature hierarchies for accurate object detection and semantic segmentation,” in IEEE Conference on Computer Vision and Pattern Recognition (Columbus, OH).
Gkioxari, G., Girshick, R., and Malik, J. (2015). “Actions and attributes from wholes and parts,” IEEE International Conference on Computer Vision. arXiv:1412.2604v2. Available online at: https://arxiv.org/abs/1412.2604v2
Gunton, R. M., Firbank, L. G., Inman, A., and Winter, D. M. (2016). 0How scalable is sustainable intensification? Nat. Plants 2, 1–4. doi: 10.1038/nplants.2016.65
Hadley, G. L., Wolf, C. A., and Harsh, S. B. (2006). Dairy cattle culling patterns, explanations, and implications. J. Dairy Sci. 89, 2286–2296. doi: 10.3168/jds.S0022-0302(06)72300-1
Halachmi, I., Polak, P., Roberts, D. J., and Klopcic, M. (2008). Cow body shape and automation of condition scoring. J. Dairy Sci. 91, 4444–4451. doi: 10.3168/jds.2007-0785
Haskell, M. J., Brotherstone, S., Lawrence, A. B., and White, I. M. S. (2007). Characterization of the dairy farm environment in Great Britain and the effect of the farm environment on cow life span. J. Dairy Sci. 90, 5316–5323. doi: 10.3168/jds.2006-865
Hyslop, J., Ross, D., Bell, M., and Dwyer, C. (2008). “Observations on the time course of calving events in unassisted multiparous spring calving suckler cows housed in a straw bedded yard,” in BSAS proceedings (Scarborough), 158.
Jones, H. E., Warkup, C. C., Williams, A., and Audsley, E. (2008). “The effect of genetic improvement on emission from livestock systems,” in Proceedings of the European Association of Animal Production (Vilnius), 24–27.
Kim, I. H., and Kang, H. G. (2003). Risk factors for endometritis and the effect of endometritis on reproductive performance in dairy cows in Korea. J. Reprod. Develop. 49, 485–491. doi: 10.1262/jrd.49.485
Krizhevsky, A., Sutskever, I., and Hinton, G. (2012). “Imagenet classification with deep convolutional neural networks,” in Proceedings 25th International Conference on Neural Information Processing Systems (Lake Tahoe, CA), 1097–1105. Available online at: https://dl.acm.org/citation.cfm?id=2999257
Lassen, J., Løvendahl, P., and Madsen, J. (2012). Accuracy of noninvasive breath methane measurements using fourier transform infrared methods on individual cows. J. Dairy Sci. 95, 890–898. doi: 10.3168/jds.2011-4544
Lewis, G. S. (1997). Uterine health and disorders. J. Dairy Sci. 80, 984–994. doi: 10.3168/jds.S0022-0302(97)76024-7
Manafiazar, G., Zimmerman, S., and Basarab, J. A. (2017). Repeatability and variability of short-term spot measurement of methane and carbon dioxide emissions from beef cattle using GreenFeed emissions monitoring system. Can. J. Anim. Sci. 97, 118–126. doi: 10.1139/cjas-2015-0190
Miettinen, H., and Huhtanen, P. (1996). Effects of the ration of ruminal propionate to butyrate on milk yield and blood metabolites in dairy cows. J. Dairy Sci. 79, 851–861.
Neethirajan, S. (2017). Recent advances in wearable sensors for animal health management. Sens. Bio-Sensing Res. 12, 15–29. doi: 10.1016/j.sbsr.2016.11.004
Nieuwhof, G. J., van Arendonk, J. A. M., Vos, H., and Korver, S. (1992). Genetic relationships between feed intake, efficiency and production traits in growing bulls, growing heifers and lactating heifers. Livest. Prod. Sci. 32, 189–202.
Pomar, C., Hauschild, L., Zhang, G. H., Pomar, J., and Lovatto, P. A. (2010). “Precision feeding can significantly reduce feeding cost and nutrient excretion in growing animals,” in Modelling Nutrition Digestion and Utilization in Farm Animals, eds D. Sauvant, J. van Milgen, P. Faverdin, and N. Friggens (Wageningen: Wageningen Academic Publishers), 327–334.
Pomar, J., Lopez, V., and Pomar, C. (2011). Agent-based simulation framework for virtual prototyping of advanced livestock precision feeding systems. Comput. Electron. Agric. 78, 88–97. doi: 10.1016/j.compag.2011.06.004
Pritchard, T., Coffey, M., Mrode, R., and Wall, E. (2012). Genetic parameters for production, health, fertility and longevity traits in dairy cows. Animal 7, 34–46. doi: 10.1017/S1751731112001401
Pryce, J. E., Nielson, B. L., Veerkamp, R. F., and Simm, G. (1999). Genotype and feeding system effects and interactions for health and fertility traits in dairy cattle. Livest. Prod. Sci. 57, 193–201. doi: 10.1016/S0301-6226(98)00180-8
Pryce, J. E., Wales, W. J., de Haas, Y., Veerkamp, R. F., and Hayes, B. J. (2014). Genomic selection for feed efficiency in dairy cattle. Animal, 8, 1–10. doi: 10.1017/S1751731113001687
Redman, G. (2015). The John Nix Farm Management Pocketbook 2016. Melton Mowbray: Agro Business Consultants Ltd.
Sivakesava, S., and Irudayaraj, J. (2002). Rapid determination of tetracycline in milk by FT-MIR and FT-NIR spectroscopy. J. Dairy Sci. 85, 487–493. doi: 10.3168/jds.S0022-0302(02)74099-X
Stock, J. D., Calderón Díaz, J. A., Abell, C. E., Baas, T. J., Rothschild, M. F., Mote, B. E.J, et al. (2017). Development of an objective feet and leg conformation evaluation method using digital imagery in swine. J. Anim. Sci. Livestock. Prod. 1, 1–7. Available online at: http://www.imedpub.com/articles/development-of-an-objective-feet-and-leg-conformation-evaluation-method-using-digital-imagery-in-swine.php?aid=19291
Strandberg, E., Brotherstone, S., Wall, E., and Coffey, M. P. (2009). Genotype by environment interaction for first-lactation female fertility traits in UK dairy cattle. J. Dairy Sci. 92, 3437–3446. doi: 10.3168/jds.2008-1844
van der Steen, H. A. M., Prall, G. F. W., and Plastow, G. S. (2005). Applications of genomics to the pork industry. J. Anim. Sci. 83, E1–E8. doi: 10.2527/2005.8313_supplE1x
Vanlierde, A., Vanrobays, M.-L., Gengler, N., Dardenne, P., Froidmont, E., Soyeurt, H., et al. (2016). Milk mid-infrared spectra enable prediction of lactation-stage-dependent methane emissions of dairy cattle within routine population-scale milk recording schemes. Anim. Prod. Sci. 56, 258–264. doi: 10.1071/AN15590
Wathes, C. M., Kristensen, H. H., Aerts, J.-M., and Berckmans, D. (2008). Is precision livestock farming an engineer's daydream or nightmare, an animal's friend or foe, and a farmer's panacea or pitfall? Comput. Electron. Agric. 64, 2–10. doi: 10.1016/j.compag.2008.05.005
Yan, T., Mayne, C. S., Gordon, F. G., Porter, M. G., Agnew, R. E., Patterson, D. C., et al. (2010). Mitigation of enteric methane emissions through improving efficiency of energy utilization and productivity in lactating dairy cows. J. Dairy Sci. 93, 2630–2638. doi: 10.3168/jds.2009-2929
Yan, T., Porter, M. G., and Mayne, C. S. (2009). Prediction of methane emission from beef cattle using data measured in indirect open-circuit respiration calorimeters. Animal 3, 1455–1462. doi: 10.1017/S175173110900473X
Keywords: cattle, phenotypes, technology, objective assessment, sustainability
Citation: Bell MJ and Tzimiropoulos G (2018) Novel Monitoring Systems to Obtain Dairy Cattle Phenotypes Associated With Sustainable Production. Front. Sustain. Food Syst. 2:31. doi: 10.3389/fsufs.2018.00031
Received: 21 February 2018; Accepted: 06 June 2018;
Published: 26 June 2018.
Edited by:
Leslie George Firbank, University of Leeds, United KingdomReviewed by:
Taro Takahashi, University of Bristol, United KingdomJohn Michael Lynch, University of Oxford, United Kingdom
Copyright © 2018 Bell and Tzimiropoulos. This is an open-access article distributed under the terms of the Creative Commons Attribution License (CC BY). The use, distribution or reproduction in other forums is permitted, provided the original author(s) and the copyright owner are credited and that the original publication in this journal is cited, in accordance with accepted academic practice. No use, distribution or reproduction is permitted which does not comply with these terms.
*Correspondence: Matt J. Bell, bWF0dC5iZWxsQG5vdHRpbmdoYW0uYWMudWs=