- 1Soil Management and Sugar Beet Research Unit, Agricultural Research Service, United States Department of Agriculture (USDA-ARS), Fort Collins, CO, United States
- 2Environmental Systems Research Institute, National Government Unit, Redlands, CA, United States
- 3Sustainable Agricultural Systems Laboratory, Agricultural Research Service, United States Department of Agriculture (USDA-ARS), Beltsville, MD, United States
- 4Center for Agricultural Resources Research, Agricultural Research Service, United States Department of Agriculture (USDA-ARS), Fort Collins, CO, United States
Humanity is confronted with the grand challenge of how to increase agricultural production to achieve food security during the 21st century and feed a population that is expected to grow to 10 billion people. This needs to be done while maintaining sustainable agricultural systems and simultaneously facing challenges such as a changing climate, depletion of water resources, and the potential for increased erosion and loss of productivity due to the occurrence of extreme weather events. Precision Agriculture emerged out of the advances in the 1980s because of the development of several key technologies like GPS and satellite imagery. This paper argues that with the increasing impact of climate change, the next revolution in precision agriculture and agriculture in general will be driven by Sustainable Precision Agriculture and Environment (SPAE, similar to the 7 Rs), which could leverage past technologies combined with Big Data analysis. This new, technology-focused SPAE transitions from a site-specific management focus to the notion of global sustainability. To accomplish this transition, we introduced the WebGIS framework as an organizing principle that connects local, site-specific data generators called smart farms to a regional and global view of agriculture that can support both the agricultural industry and policymakers in government. This will help integrate databases located in networks of networks into a system of systems to achieve the needed SPAE management and connect field, watershed, national, and worldwide sustainability. Automation and the use of artificial intelligence (AI), internet of things (IoT), drones, robots, and Big Data serve as a basis for a global “Digital Twin,” which will contribute to the development of site-specific conservation and management practices that will increase incomes and global sustainability of agricultural systems.
Introduction
The 21st century presents formidable challenges to sustainability that humanity will have to confront. The need to increase agricultural production to ensure food security for a global population estimated to grow to 9–10 billion people in the coming decades while confronting a changing climate that threatens sustainability will put pressure on agricultural systems. The United Nations Secretary-General recently warned the global community that climatic changes are occurring at a faster rate than humanity is addressing them and that humanity will be impacted by sea level rise and more extreme weather (United Nations, 2018). Recent reports released by the UN Intergovernmental Panel on Climate Change support these statements (United Nations, 2018). The increased occurrence of extreme weather events will increase the potential for erosion in agricultural systems (Pruski and Nearing, 2002; SWCS, 2003). Pruski and Nearing (2002) reported that erosion rates could increase by 1.7% for every 1% increase in total rainfall due to climate change. Without conservation practices humanity will not be able to adapt to a changing climate, as conservation practices will be key tools to maintain and increase the productivity and sustainability of agricultural systems (Delgado et al., 2011; Walthall et al., 2012; Spiegal et al., 2018). Big data analysis will also be one of the key tools that will contribute to development of sustainable systems.
It is thought that crop production must be increased by 60–100% by the year 2050 to meet the nutritional needs of a future human population of 9–10 billion. Crop production systems that yield more food of higher nutritional content are needed, yet at the same time, they must have a diminished impact on the environment. Agricultural intensification during the 20th century was through the substantial use of fertilizer, pesticides, and irrigation, all at a significant environmental cost. These technologies were part of the Green Revolution that helped achieve food security for billions of people. However, the challenges of the 21st century are different, and soil and water conservation will be key to achieve food security, and sustainable precision agriculture and environment (SPAE) will be needed so that intensive agriculture and a changing climate will not generate additional impacts that could contribute to accelerating the pace of a changing climate. As a part of sustainable agriculture, next-generation cropping systems that couple biologically-based technologies (plant-beneficial microbes, cover crops) and precision agriculture (PA) and precision conservation (PC) need to be developed to decrease fertilizer, pesticide, and water inputs while increasing conservation effectiveness to maintain sustainable agriculture at a field level and sustainability across a watershed. Crop cultivars with enhanced nutritional content and enhanced tolerance to abiotic (drought, salinity, heat, etc.) and/or biotic (disease) stresses need to be developed using advanced breeding and biotechnology approaches. These enhanced cultivars will no doubt disrupt the status quo of agricultural business.
Central to SPAE and the rapid development of these cropping systems is the use of PA and PC in the development and use of technology with the capacity to manage and disseminate accurate data and information at all levels of the agricultural ecosystem. PA (Pierce and Nowak, 1999), PC (Berry et al., 2003, 2005; Delgado and Berry, 2008; Sassenrath and Delgado, 2018) (Figure 1), and sustainable agriculture “are inextricably linked” (Berry et al., 2003, 2005; Bongiovanni and Lowenberg-DeBoer, 2004). Sustainable agriculture and PC focus on increasing conservation effectiveness and stress environmental impact and sustainability, while PA is often about immediate cost savings at a specific location by optimizing returns on inputs. This paper focuses on sustainability at both a site-specific management scale and a global scale. For that reason, the emphasis is on information systems and their ability to support a variety of characteristics of PA, PC, and global sustainability.
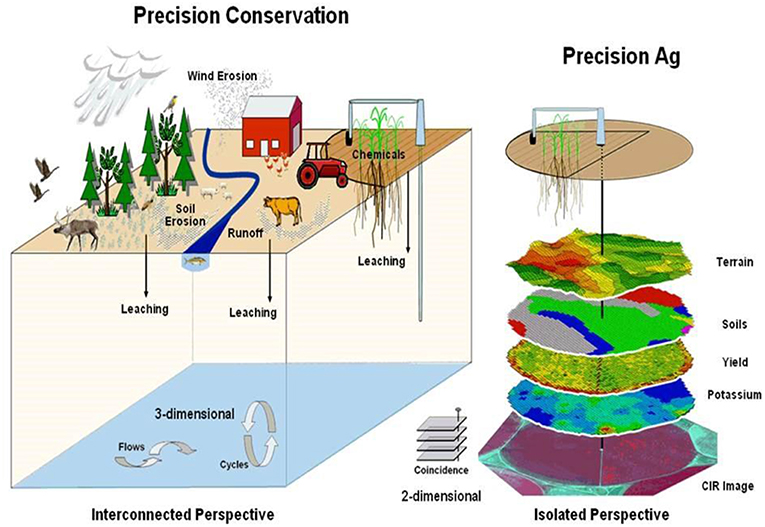
Figure 1. The site-specific approach can be expanded to a three- dimensional scale approach that assesses inflows and outflows from fields to watershed and regional scales [Permission granted by Soil Water Conservation Society for reprint from source: (Berry et al., 2003)].
Inherent in both the complexity and accuracy of SPAE is the need to manage data spatially, which has traditionally been the realm of Geographic Information Systems (GIS)1 For example, PA and PC use geospatial data and sensors for crop yield and any other measurable variable to apply the correct rate of fertilizer, water, and pesticide; manage drainage and water runoff; reduce movement of agrochemicals; and use the right management practices at the field and off-site (Delgado et al., 2018a). Both PA and PC allow the farmer to treat the production field as the heterogeneous surface it is (fertility, water, plant pathogens, slope, surface runoff, drainage, etc., which are all highly variable throughout the field) instead of as a homogeneous surface as it was treated in the past. SPAE also manages geospatial data, but its spatial relationships can be more abstract from the soil level to the molecular level in order to model more complex biological systems. In fact, Esri refers to this concept as the “science of where,” implying that GIS is evolving beyond the traditional geospatial realm of maps, images, etc. to modeling more complex relationships (Dolan et al., 2006).
With increased adoption of PA and PC by farmers will come increased development and marketing of tools for PA and PC to speed up the adoption of technology. In the developed world primarily, advanced sensors and systems that deliver decision support tools directly to the farmer will be developed, allowing real-time decisions on the delivery of appropriate rate of inputs (water, fertilizer, pesticide) (Fulton and Darr, 2018) as the farmer drives the tractor through the field, implements drainage systems (Shedekar and Brown, 2018) and/or tries to simultaneously conserve wildlife (McConnell and Burger, 2018).
The need for PA and PC will only heighten, as, for example, nutrient losses to the environment impact groundwater and surface water resources, such as has occurred with the hypoxic zone in the Gulf of Mexico (Goolsby et al., 2001; Rabalais et al., 2002a,b) and the hypoxic zone and microcystin levels in Lake Erie (Smith et al., 2018). The World Health Organization (2011) has reported that microcystin concentrations above 1.0 μg L−1 in treated drinking water is not safe and is unhealthy for human consumption and in the USA, the EPA reports that for children less than 6 years of age the safe level is 0.3 μg L−1 (United States Environmental Protection Agency, 2015). Water issues will continue to increase in many parts of the world in the near term, especially if there are legacy effects. New cropping systems with improved management practices must be developed that contribute to environmental sustainability to minimize negative impacts on air, soil and water. In addition, advanced crop cultivars must be developed that better use soil nutrient and water resources, are more resistant to environmental stress (temperature extremes, drought, flood, plant pathogens), and are packed with nutrients that are found deficient in populations in the developing world (Bouis and Saltzman, 2017). This all must be done very rapidly as 30 years is not a long time when dealing with science and adoption of scientific technology. In other words, SPAE, which aims to preserve ecosystem services, must work with modern technologies and practices quickly through the rapid transfer of knowledge from the agricultural lab to the producer.
Over the past several decades, Information Technology (IT) has been the disruptive force in industries by driving out market inefficiencies through automation and better decision support tools that require the inclusion of both the citizens and consumers in the process. Like all industries, agriculture has not been immune to the constant disruptions over the past century (e.g., introduction of the tractor, PA, PC, and new governance models for dealing with inelasticity of demand, etc.). However, recent advances in computing infrastructure, sensor technology, big data, and advanced algorithms (e.g., Deep Learning) suggest that a major disruption or paradigm shift is on the horizon, leading to opportunities for SPAE entering the mainstream in a smart, advanced system for SPAE. IT also holds the key for accelerating knowledge transfer from the lab to the producer.
Eventually, a system based on these new technologies will be needed for mass transfer of genomic and other genetic data for the development of these advanced crop cultivars, for the management of agronomic data, and for the development of these next-generation production systems. Data generated from these cropping systems are inherently geospatial in that crop types and their environmental hardiness obviously vary regionally by latitude. Agricultural field crop production inputs and conservation management to achieve sustainable systems can vary considerably over space and time (Pierce and Nowak, 1999; Berry et al., 2003, 2005; Delgado and Berry, 2008; Gebbers and Adamchuk, 2010). Therefore, monitoring crop and environmental performance will highly depend not only on traditional methods of Earth Observation (EO), but also data generated in situ (i.e., ground truth).
Hence, geospatial solutions based on imagery from EO at all scales integrated with sensor networks will increasingly become critical for the operation of PA systems where resource inputs are applied at precise geo-specific field locations based on crop need. Solutions will eventually be needed to allow immediate feedback from digital farm communities regarding the performance of these new cropping systems; speeding their development based on immediate feedback to the labs and other interested parties. To accomplish this, we describe a “system of systems” approach to building a scientific network that integrates the scientific and farming communities, based on a common, global IT platform (i.e., the cloud).
Historical Challenges in Agricultural Technology Adoption for PA
Although PA has been around since the 1980s, the adoption of the technology has been slow. Schimmelphfenning (2016) reports that PA technology, for example, was used on about 30–50% of U.S. corn and soybean acres in 2012. Van der Wal (2019) suggests that the reason for poor adoption is due to the “growing complexity of adoption in the use of information technology” and the fact that “incomes in agriculture are generally low and young generations seek their prosperity in cities,” implying that those with technical prowess are heading to the cities for hi-tech jobs that pay better. PA also tends to be expensive (Shama, 2017), which is reflected in the fact that it is mostly implemented on larger farms (Schimmelphfenning, 2016) which can afford the complex and changing technology. However, low-tech precision agriculture/precision conservation approaches have been implemented by farmers in Sub-Saharan Africa, contributing to improved yields, incomes and conservation (Jenrich, 2011). There are numerous reasons for the low adoption rates and the next few sections offer several common explanations. However, there are opportunities for leveraging existing technological trends.
Poor Adoption of Decision Support Tools
While the agricultural sector has a long tradition of relying on best practices rooted in science, the industry hasn't always been an early adopter of decision support tools or extension services that resulted in speeding up the adoption of new precision agricultural practices (Rose et al., 2016). Ribaudo et al. (2011a) reported in a national study that 65 percent of the cropland studied (109 million acres) needed best practices for nitrogen management. There are several reasons for limited adoption including culture in the producer community, skills, current information management processes, etc. Perhaps the biggest reason, until recently, has been the limitations of both the technologies and agricultural systems models used to support PA, much less PC and SPAE (Antle et al., 2017). Specifically, Antle et al. (2017) states that “many advances in data, information and communication technology of the past decade have not been fully exploited… [because of the] underinvestment in agricultural research, particularly in non-proprietary public good research, and in research aiming to improve the well-being of poor, smallholder farm households in the developing world.” While user-centered design techniques in the IT industry will improve adoption, an active area of research exists to more quantitatively understand positive adoption of new practices (Kuehne et al., 2017).
That being said, technology adoption challenges in the IT industry are well understood and noted by Moore (1991) who discusses the diffusion of innovation through groups of technologists, early adopters who see the possibilities of innovation, pragmatists who resist change until an economic benefit is defined, the late majority adopters who require low risk and high reliability, and the laggards. By utilizing targeted messaging, Silicon Valley and other tech centers' success over the past few decades in disrupting markets is often attributed to the model cited in Moore (1991), which ironically had its roots in the study of farming practice adoption (Beal and Bohlen, 1957).
Limitations of Earth Observation Data in Agriculture
From a practical point of view, these historical technical limitations in the past have ranged from lack of standards, non-scalable systems, cost of sensors, and limited support from governments around the use of EO in agriculture or remotely sensed data that focused on climate change observations as opposed to agriculture. Although there was early adoption in agriculture in the US through programs like AgRISTARS and LACIE in the 1970s/80s (Pinter et al., 2003), the Landsat series, for example, primarily focused on moderate spatial resolution (i.e., 30 × 30 m), which while good for crop monitoring at the macro level, was too course for PA. Luccio (2014) suggests that “…farm management decisions, such as weed detection and management, require imagery with a spatial resolution in the order of centimeters and, for emergent situations (such as to monitor nutrient stress and disease), a temporal resolution of <24 h.”
Likewise, these moderate resolution satellites, which were primarily designed for answering scientific questions, haven't always focused on the appropriate spectral bands (e.g., red edge band) for agriculture or frequency of data acquisition to make it easy to monitor crop growth during the growing season. Yet, because of the global coverage, these satellite networks have been recognized as the original generators of big data and represented a compromise or trade-off between spatial resolution and storage capacity. In recent years, commercial satellites, such as those from DigitalGlobe, which serve a variety of markets beyond agriculture have promised to fill the gaps made from the public-sponsored platforms. However, the cost and complexity of using these data have been limiting factors at the producer level for all but the large farm operations who can justify data costs. As a result much of the use of remote sensing data has come in the past from aerial platforms and now from drones carrying small sensors focused on frequent observations over the growing season. Although at a limited coverage area, the cost of entry can be considered high for smaller farms operations in the US and, especially in the developing world creating a logistics problem of sensor and data exchange.
Poor Communication Infrastructure
In addition to the difficulty arising from a variety of remote sensing data from multiple sensors, the lack of a comprehensive backbone for high-bandwidth transmission of data to remote farm areas has limited the ability of the exchange of data between the farm and value-added services. In the US broadband adoption promises to solve this problem with bandwidth at the megabyte level, but small farm operations in low-income countries will have to rely on alternative architectures that utilize local sensor networks. Tyler and Griffin (2016) argues that “The realization of ‘Big Data's’ value will not happen until [the data transfer bandwidth] barrier is overcome.” On the surface, PA looks to not be a big generator of data for upload. Tyler and Griffin (2016) suggests otherwise for just corn alone, where for each plant generating 0.5 k bytes, the 88.9 million acres in 2015 would generate 1.3 petabytes of data, not including the notorious big data generator from aerial drones. Upload bandwidth will clearly be needed.
Siloed Data Management
Given the variety of data and limitations of bandwidth in many farming communities, it's not surprising that data management has also been an issue. First, the public remote sensing platforms, whose data generation capabilities have led to data archives at the exabyte (i.e., 1018 bytes) and above levels, have resulted in driving the public sector to invest into large data systems in order to serve a wide scientific community. These systems, while good at disseminating data, still require extensive and complex knowledge of a variety of satellites and sensors, file formats, meta data standards, physics, etc. (Blumenfeld, 2019). In short, it still does require considerable expertise to gain any significant agricultural benefits from these systems.
Second, commercial satellite, aerial, and drone-based data systems, while somewhat easier to use, are still fragmented due to competing interests. In the end, the variety of data formats, velocity of data coming off of a variety of platforms, and volume of data generated have led to a fragmented and siloed data management infrastructure for agriculture. In other words, this is a Big Data problem, which is formally defined as a combination of a variety of data, the velocity of data and/or the volume of data.
Given the inherently spatial nature of agriculture and remotely sensed data, GIS offered the opportunity to minimize data siloes by providing spatial context (i.e., maps) around data. This has led to a proliferation of GIS systems that span topic areas well beyond remotely sensed data, yet has led to additional silos of geospatial information.
Immature Applications of Analytics and AI
While data silos are not inherently a problem per se, techniques including those from analytics, AI and Machine Learning, require that the data be readily accessible, coherent, and consistent before these algorithms can provide any value. Certainly, first-generation solutions like the world wide web and data warehouses have gone a long way in connecting these data siloes, but they fell short in addressing the computational capacity necessary for analytic techniques. These challenges made it difficult to apply analytic techniques comprehensively such as yield forecasting and all the other advanced techniques tried in the scientific literature.
The problem is further exacerbated when considering SPAE and modeling. In PA, applications focus on connection of inputs to needs in order to determine the action based on a spatial area. That is, while there is some modeling going on, it's relatively simple in that a precision map shows exactly where to apply the fertilizer, water, etc. SA, which places emphasis on complex interactions between biological systems, tends to have more complex models that are crafted carefully by the scientific field. Consider the Denitrification and Decomposition (DNDC) model used in the simulation of carbon and nitrogen biogeochemistry through complex interactions of soil, crop growth/decomposition, etc. to predict, among other things, nitrate leaching and C/N greenhouse gas emissions (Salas, 2010; University of New Hampshire, 2012). Whereas initial PA efforts may not be as focused on assessing greenhouse emissions from agriculture, this more complicated model or analytic technique is an example of one that could contribute to achieving the objectives of SPAE and, as such, requires a strong source of “regional databases…for mapping and potentially monitoring management practices…for compliance, verification, or tracking sustainability” (Salas, 2010).
Another recent example is the COMET-Farm system web-based tool developed by Paustian et al. (2018) to do full greenhouse gas assessments for CO2, CH4, and N2O from all major on-farm emission sources as well as assessments of soil carbon sequestration. This web-based tool can assess GHG emissions from perennial crops, pasture, range and agroforestry systems, as well as emissions from livestock and on-farm energy use (Paustian et al., 2018). This tool can be used to assess PC practices. The user could upload a custom soil map that can specify sub-field map units, allowing the user to define spatially explicit management zones (Paustian et al., 2018). An application could be added in the future to assess the effects of PA and PC on GHG emissions (Paustian et al., 2018). Saleh and Osei (2018) reported that the Comprehensive Economic Environmental Optimization Tool (CEEOT) and the more user-friendly Nutrient Tracking Tool (NTT) can be used at a watershed level to assess the optimal conservation practices, using a PC approach to maximize the benefit from each dollar of conservation practice investment. They reported that by using spatial distributions of field attributes such as soil type, topography, and soil chemical and physical properties, PC can be applied to reduce the uncertainty of where to apply a given practice to increase conservation effectiveness and thus sustainability (Saleh and Osei, 2018).
Regional databases are equally as critical for building accurate machine-learning algorithms. In the geospatial world, early applications of AI focused on classification of imagery such as Landsat (Campbell et al., 1989), which led to applications mostly around macroeconomic crop forecasting, as is done by the United States Department of Agriculture's (USDA) National Agricultural Statistics Service. Figure 2 illustrates the problem through the classifications of cropland (yellow), forest (green), and roadways (gray) in the left picture, where even a casual inspection shows misclassification of the entire farm in the polygon. When taken at a national or global level, these classification error rates may well be within statistical tolerances for macroeconomic problems, but clearly fertilizing the road or farm buildings would not be acceptable in the left picture. The picture to the right on higher resolution data (NAIP) from a recent use of machine learning in the cloud shows drastically improved classification (Tayyebi, 2019) due to not only higher spatial resolution data but also significant computational capacity in today's cloud environment.
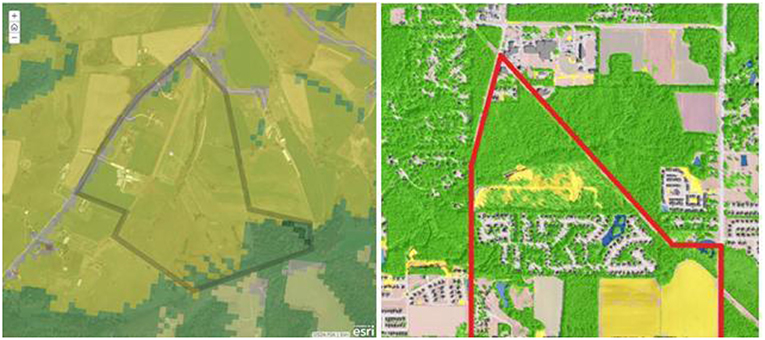
Figure 2. Coarse classification (left) vs. recent AI classification methods (right) (Tayyebi, 2019).
In either case, Campbell et al. (1994) and Short et al. (1995) recognized early that regional databases needed to be developed as a mechanism for providing training data to machine-learning algorithms, which capture local knowledge from different individuals or organizations (e.g., farmers). In other words, these classification techniques rely heavily on supervised learning where training datasets consisting of known classification labels associated with spectral properties (i.e., spectral signature) are presented to the algorithms, which generalize or learn.
In the 1990s, NASA recognized the need to develop a network of distributed, end-to-end satellite processing centers, called Regional Application Centers, in order to leverage local knowledge in the building of training datasets for localized machine-learning implementations. Implemented around the world in countries with no interconnectivity at the time (i.e., the internet), NASA deployed low-cost, high-performance technology to acquire data directly from the satellites with direct broadcast capability and perform all the necessary processing routines including machine learning, in order to produce spatial information products for not only NASA but also the local and international governments (Campbell et al., 1994; Davis et al., 1994; Short and Dickens, 1995). Because the mission areas ranged from hurricane forecasting to early applications of PA, the network of regional data centers demonstrated a coexistence between science goals and operation mission effectiveness that resulted in, for example, an “early warning [that] allowed the movement of over 200,000 people [in Bangladesh] to higher elevation thereby avoiding certain drowning due to flooding” (Campbell et al., 1994).
The Paradigm Shift: Digital Agriculture
Research and industry could solve many of these problems given the inherent nature of PA as a simple business solution of minimizing input costs through big data management in order to maximize yield and profits in a commodity-based industry. In a recent Bloomberg article (Noel, 2019) argues that companies like Bayer can acquire data from the farm, process it with analytics, and sell it back to the producers. With advances in the cloud where computational power and storage are relatively inexpensive, companies will probably move into a new era of selling information products by coalescing many of the aforementioned data sources, thereby reducing siloes and the knowledge complexity of operating the variety of data generators.
However, SPAE is different due to its focus on more environmentally friendly techniques and reliance on natural farming practices that require a level of complexity beyond simple input/outcome optimizations. Not only are the techniques less scientifically understood, but the potential improvements in yield may not generate the economic benefits required to justify the increased complexity and costs in the short run, such as the aforementioned analytic complexity for SPAE alone. With that said, recent advances in PC to increase SPAE has shown that there is potential for some quick profits when conservation practices use these new technologies. For example, Thompson and Sudduth (2018) reported that using PA tools offers an advantage in both designing and utilizing terracing and contour farming approaches to conservation management by reducing terrace layout time by 50%, contributing to savings in time and money. Thompson and Sudduth (2018) also reported that using PA will contribute to economic sustainability of these systems by using field machines that utilize GPS guidance and automatic row or section control.
New opportunities may also be possible if ecosystem markets are developed where nitrogen, carbon, or even reduction of GHG can be traded in market systems, providing additional income to farmers due to implementation of conservation practices (Ribaudo et al., 2005, 2011a,b; Delgado et al., 2008, 2010; Paustian et al., 2018; Saleh and Osei, 2018). Paustian et al. (2018) reported that GHG mitigation, carbon storage, and water filtration, for example, could potentially be appreciated and monetized, but it will then bring greater complexity to the decision-making and management choices of farmers and ranchers; it will also require tools and technologies that have not previously been available to agricultural producers. Delgado et al. (2008, 2010) reported that a GIS nitrogen trading tool could potentially assess the spatial effects of implementation of conservation practices on reductions in nitrate leaching and direct and indirect GHG emissions that could be traded in air and water quality markets. Saleh and Osei (2018) reported that we could use spatial tools to assess the effects of conservation practices and how PC could be used to generate credits for water quality markets and to specify, for example, if the credits will be for sediment load reduction or nitrogen or phosphorus reduction. Saleh and Osei (2018) reported that by using PC there will be a reduction in the “margin of safety” credit adjustments necessary for water quality trades, improving the water quality trading options for farmers. As suggested in Campbell et al. (1994) around Distributed AI techniques for automated cooperative systems, we propose that by using AI and the new SPAE approach and technology there will be greater opportunities to make SPAE generate income in these trading systems for farmers and ranchers, and increase field, watershed, national, and global conservation.
The rest of this paper argues that big data analytics is at the core of combining precision and sustainability into an earlier notion of Sustainable Agriculture (Berry et al., 2003, 2005; Bongiovanni and Lowenberg-DeBoer, 2004; Delgado and Berry, 2008). Berry et al. (2003) reported that precision conservation will be needed to maintain the productivity of intensive agricultural systems and global sustainability. Berry et al. (2003) also reported that precision conservation has the potential to integrate conservation practices at a site-specific field level with off-site conservation practices, which would contribute to watershed sustainability. To support this argument, we will focus on both farm management and a geoinformatics cloud framework as a step toward global agricultural sustainability.
Part of the PA concept to increase the efficiency of fertilizers has been the use of a 4 Rs approach to reduce nutrient losses from farming systems (the right product, at the right rate, at the right time, and at the right place (Roberts, 2007). However, Delgado (2016) reported that the 4 Rs are not enough and gave examples where significant losses of nutrients and soil could occur without precision conservation (Figure 3). The concept of agricultural sustainability using a precision conservation approach in agriculture presented by Berry et al. (2003) will contribute to reduced off-site impacts across a watershed by using these new technologies to improve the design of conservation practices and increase the efficiencies of conservation practices such as field buffers, sediment traps, denitrification traps, and riparian buffers to minimize the losses of nutrients from the field and across a watershed. Cox (2005) reported that the Berry et al. (2003) PC concept could also be described using a 4 Rs approach, by applying the right conservation practice, at the right place, at the right time, and at the right scale (the 4 Rs of conservation). Delgado (2016) combined these 4 Rs of precision farming with the 4 Rs of precision conservation to create the 7 Rs for nutrient management and conservation, which are applying the right product (1), the right rate (2) and the right method of fertilizer application (3), the right conservation practice (4) placed at the right place (5) and right scale (6), with the right time of application of fertilizer and establishment of the conservation practice (7). We propose that we could use new Big Data analytics to combine precision agriculture and precision conservation (Berry et al., 2003, 2005; Bongiovanni and Lowenberg-DeBoer, 2004; Delgado and Berry, 2008) to achieve SPAE. This is similar to the 7 Rs approach described by Delgado (2016) and Sassenrath and Delgado (2018).
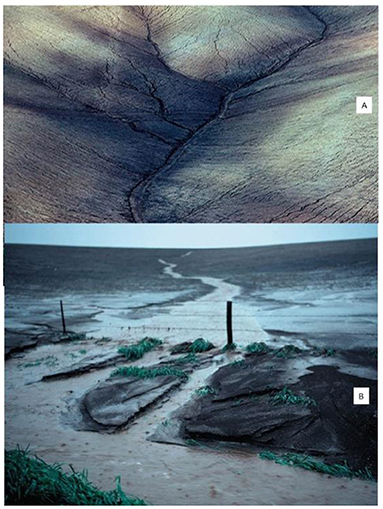
Figure 3. Locations (A,B) where the 4 Rs alone would not reduce the off-site transport of nutrients and a 7 Rs approach would contribute to reducing off-site transport of nutrients (Images from NRCS showing development of ephemeral gullies).
Modern Farm Management
With the emergence of commercial viability of PA over the past few decades, today's developed world farmer is moving from a grower and distributor of produce to a modern day data scientist who must utilize analytic techniques to both collect the right data at the right time, but also apply advanced information products to increase yield. Acting in the role of an early adopter of technology (Moore, 1991), today's farmer will have to quickly learn how these new technologies can be used to help make decisions about how to increase profits by increasing yields or implementing precision management and conservation practices that could produce sustainability benefits that could potentially be traded in ecosystem service markets. The use of this new technology for SPAE will be dominated in the future by analytic techniques and AI to help provide solutions to complex problems and decisions.
Today's “early majority” farmer is increasingly aware of the role of cost externalities as they relate to input costs and will act as the bridge from the early adopter technology community. For example, whether it is organic farms that must deal with cross-species contamination from neighboring farms or the increased externalities from management practices that contribute to emissions of GHG or losses of agrochemicals that could impact water quality, or weather impacts on erosion and loss of productivity, farming must increasingly deal with long-term factors that may not show up immediately in increased yield profits but that will contribute to reduced yields if they remain unchecked. Using precision conservation to increase the sustainability of agricultural systems will contribute to adaptation to a changing climate and maintaining long-term productivity. In other words, sustainability in agriculture is increasingly becoming a necessary component of today's agricultural practices. Organizations like USDA's Natural Resources Conservation Service (NRCS) have long known that conservation programs are methods for removing the cost burden of externalities, but with decreased public sector budgets, this is becoming more difficult as external costs increase.
Managing nutrients at a farm level is very important since losses of reactive nitrogen at this level significantly impact the environment via emissions of nitrous oxide, ammonia emissions, nitrate leaching losses, and off-site transport of surface losses of nitrogen (Smith et al., 1997; U.S. Environmental Protection Agency, 2010). Nitrogen losses significantly impact terrestrial resources, water bodies, and the atmosphere (Hutchinson et al., 1982; Legg and Meisinger, 1982; Cowling et al., 2002; Rabalais et al., 2002a,b; Galloway et al., 2008; Dubrovsky et al., 2010). It has been documented that these losses contribute to significant impacts such as impacts on species composition and the functioning of terrestrial, freshwater, and marine ecosystems, among others (Matson et al., 1997; Vitousek et al., 1997). Reactive nitrogen could also negatively impact human health (Follett et al., 2010). In addition to significant environmental impacts, these losses can also have negative economic impacts. Ribaudo et al. (2011a) reported that the cost of removing agriculture's contribution to nitrate loadings in drinking water in the USA is about $1.7 billion per year.
Fortunately, organizations like USDA's NRCS and private consultants have been increasing the use of these new technologies that contribute to implementation of conservation practices on the ground to achieve precision conservation. Precision conservation is increasingly being embraced by agencies such as NRCS and the private sector in a new revolutionary approach that is increasing conservation effectiveness (USDA-NRCS, 2017). Some of the USDA ARS' voluntary conservation programs such as the Environmental Quality Incentives Program (EQIP) can be used to implement precision conservation (USDA-NRCS, USDA-NRCS). There are several examples of private sector and non-profit organization implementation of precision conservation to increase sustainability (Buman, 2016a,b; Hammes, 2016; Heartland Science Technology Group, 2017; Chesapeake Conservancy, 2018; Illinois Sustainable Ag Partnership, 2018).
There is a revolution going on in the agricultural landscape, making it possible to move agricultural science from on-site research facilities directly to the farm. Farmers are no longer passive recipients of information but are rather actively involved in the science and development of new crop production systems that can either be yield neutral or possibly improve yield. Over the long run, this paradigm shift could reduce the need for public subsidies for external costs, as they would be an automatic byproduct of agricultural best practices.
As suggested in Wolfert et al. (2017) (Figure 4), the key to the modern farm is the application of big data to the development of a smart farm and smart soil and water conservation (Sassenrath and Delgado, 2018). The smart farm and smart soil and water conservation conceptual frameworks focus on the cyber-physical management cycle built around a cloud-based infrastructure that manages all farm operations. Where bandwidth is plenty (i.e., due to rural broadband), then the farmer operates as a data collector transmitting to the cloud for spatially-based analytic techniques that tell the farmer what to do and when based on looking at the macro variables across the farm landscape (e.g., weather input prediction).
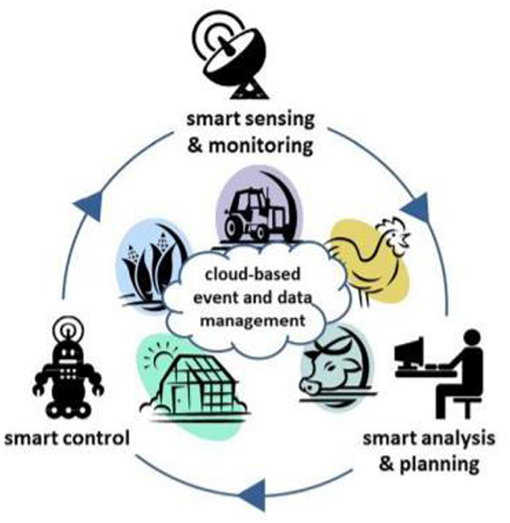
Figure 4. The smart farm conceptual framework (Wolfert et al., 2017). ©The Authors. Published by Elsevier Ltd. This is an open access article under the CC BY-NC-ND license (http://creativecommons.org/licenses/by-nc-nd/4.0/).
For agriculture in the developed world, Unmanned Aerial Vehicles (UAV) and Unmanned Ground Vehicles (UGV) armed with hyper-spectral and other sensor types generate significant amounts of data that can be processed remotely enabling a variety of advanced analytic techniques that can drive the application of inputs automatically. Computer visions techniques are increasingly being used on applications like weed vs. crop detection (Lin et al., 2017), planting, irrigation, pruning, harvesting, and plant disease detection/identification (Ampatzidis et al., 2017), leading to the potential for further automation and minimizing of labor input costs.
Where bandwidth is limited, especially in the developing world, computation must be pushed to the edge where information products are produced in situ and only small data volume information products are exchanged between the cloud and farmer if at all. Referred to as edge computing, this “distributed computing paradigm….brings computer data storage closer to the location where it is needed” (Edge Wiki)2 and is possible to the drastic drops in price/performance for hardware. In the context of agriculture, this means that machine learning algorithms that are developed in the cloud can be pushed to the edge for farm operations.
A powerful example of this model can be found in Microsoft's FarmBeats program (Vasisht et al., 2017). Rather than wait for governments or private industry to invest in connectivity programs like rural broadband, FarmBeats “piggy backs” off of T.V. whitespaces3, which are frequencies allocated to broadcasting service but not locally used, especially in rural areas. FarmBeats deploys a field sensor array to collect variables (e.g., soil moisture) where the machine learning is used to impute or predict data points from sensor nodes and brief fly overs from UAVs. By building on inexpensive and reliable hardware, FarmBeats addresses the “late majority's” market need for simplicity and low risk, thereby facilitating technology adoption (Moore, 1991). As a result, FarmBeats has already been deployed internationally in countries like India, New Zealand, and Kenya, resulting in a reported 30% reduction in water consumption (Sims, 2019).
Based on trends in farm operations and management, farms are becoming the new Big Data generator that complements much of the EO data that has been gathered to date. With these advances architecturally in farm management, the modern farm can resemble the notion of “Digital Twins”, which is the confluence of IoT, AI, and big data. [Digital Twin Wiki]4 defines a digital twin as “a digital replica of a living or non-living physical entity” that is used “to create living digital simulation models that update and change as their physical counterparts change.” In terms of agriculture and farm management, digital twins means that “farm operations would no longer require physical proximity, which allows for remote monitoring, control and coordination of farm operations” (Verdouw and Kruize, 2015). From a SPAE perspective, simulation models like the aforementioned DNDC, COMET-Farm, CEEOT, and/or the NTT models are among some of the available tools that could be used to form the basis of ensuring the least impact on environment without driving up costs.
A Geoinformatics Sustainable Agriculture Framework
Regardless of the approaches, clearly today's farms are quickly becoming data generators that when stitched together spatially provide a higher resolution view into the agricultural industry, which ultimately will provide a more precise view of not only global food security but overall environmental sustainability. Governments currently have to rely heavily on EO data to estimate crop yield as it relates to food security and market predictions, which is an indirect indicator of sustainability more from an economic than environmental perspective. This has certainly been the goal of USDA's Global Agricultural & Disaster Assessment System (GADAS), which uses satellite imagery “to assist in…agricultural estimates of global crop conditions” (Frantz, n.d.).
GADAS represents a first-generation system that potentially forms the foundation for a broader public/private partnership of connecting farm data generators to an interconnected framework for sustainability. Or namely, it brings together a variety of spatial data via a GIS to illustrate impacts of weather, water, crop conditions, land use, etc. to give a global assessment of agriculture (Figure 5). In fact, the US Federal government is starting to recognize the need for governance of geospatial data from a comprehensive point-of-view. Through the Geospatial Data Act (GDA), the intent of the act is to “coordinate and work in partnership with other Federal agencies, agencies of State, tribal, and local governments, institutions of higher education, and the private sector to efficiently and cost-effectively collect, integrate, maintain, disseminate, and preserve geospatial data, building upon existing non-Federal geospatial data to the extent possible” (URISA, 2018). In other words, the GDA provides a governance framework for bringing together billions of dollars of investment in geospatial data from a variety of environmental and military mission areas.
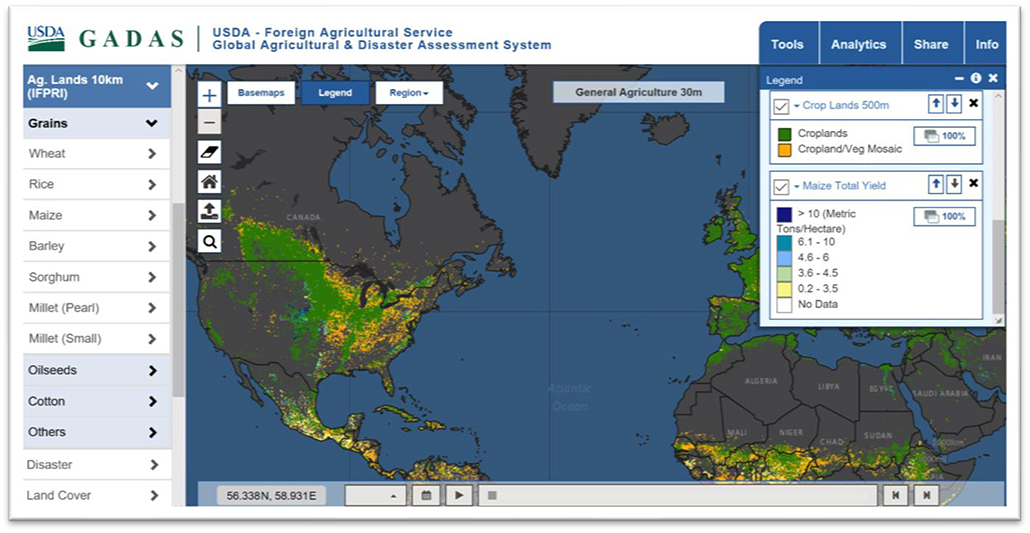
Figure 5. The Global Agricultural and Disaster Assessment System (GADAS) is a GIS system that provides data about global crop conditions based on satellite imagery and remotely sensed data [USDA-FAS].
Jack Dangermond from Esri established the vision for a geospatial infrastructure so that “users can easily and inexpensively access an immense wealth of geographic information on almost any subject…[leveraging] cloud computing resources to perform analysis and mapping” (Dangermond, 2018). Technologically, this means providing a platform for connecting existing GIS systems together into a new architectural pattern referred to as WebGIS. The WebGIS pattern supports multiple implementation patterns from the on Farm, edge-oriented architecture presented in the previous section to a “system of systems” spread across the private and public sector.
From an agricultural perspective, WebGIS provides a framework for reducing past siloes not only across the public sector agencies, but also between the public sector and agricultural industry. USDA's Agricultural Research Service (ARS) has taken a lead in breaking down these silos by leveraging WebGIS. Although in its early stages, the Agricultural Collaborative Research Outcome System (AgCROS) illustrates the vision of collaboration by providing a single platform for the dissemination of new agricultural scientific discoveries and techniques (Delgado et al., 2018b). AgCROS was built based on individual ARS national research projects. These projects studied areas such as greenhouse gas emissions, soil health, genomics, cover crops, renewable energy, antibiotic resistance, nutrient use and nutrition (Del Grosso et al., 2013; Delgado et al., 2016; Delgado et al., 2018b).
Sustaining the Earth's Watersheds, Agricultural Research Data System (STEWARDS) started the ARS multilocation national natural resource projects. STEWARDS used what is now called WebGIS, but each location had its own measurement vocabulary, so location cross comparison was not possible (Steiner et al., 2009). STEWARDS did introduce a measurement methods catalog which is a very important component in any of these systems. The Greenhouse gas Reduction through Agricultural Carbon Enhancement network (GRACEnet) was the first project to show true collaboration among scientists (Del Grosso et al., 2013). GRACEnet, also used a WebGIS. GRACEnet showed that through collaboration among the scientists, a way to build up a common vocabulary of measurements for greenhouse gas emissions. This has been key in building systems around each of these individual projects since for ARS. GIS provided a way to relate all this data based on location. The addition of metadata for public discovery and measurement methods now allow the individual location data to be combined to look at trends across ARS research locations. The next steps for AgCROS will be adding imagery, real time sensors, and electronic field collection to allow more data to be added with the goal of allowing the machine learning techniques discussed above to be realized. More importantly, it promotes the integration of agricultural knowledge using the WebGIS pattern in order to promote collaboration across government, industry and academia. In other words, it is the mechanism for speeding up the adoption of new crop production techniques to the smart farm, which can in turn deliver higher quality data back to the scientific community for enabling sound science. In effect, these aforementioned systems along with a smart farm network suggest that WebGIS could, in fact, become the Digital Twin for the Globe. In this way, such a system would facilitate the transparency of environmental costs and reduce cost externalities based on real-data from monitoring systems down to the farm/producer, thereby enabling more data-driven policy making.
Conclusion
Precision Agriculture emerged out of the 1980s because of the development of several key technologies as a way to improve margin through cost management of inputs while improving yield. Development of precision-conservation practices started in the early 2000s. New technologies like GPS, satellite imagery, and new methods of genetic modification in the green revolution have represented a disruption in agriculture not seen since the introduction of the first successful commercial tractor in the early 1900s and the green revolution that occurred between 1950 and the late 1960s. With the increasing impact of climate change, this paper has argued that the next revolution in precision agriculture will be driven by SPAE which could leverage past technologies combined with Big Data analysis. Among other positive impacts, SPAE will contribute to increased yields and profits, increased adaptation to a changing climate, increased sustainability of agricultural systems, and increased sustainability outside of the field and across watersheds, reducing nutrient transport across watersheds and contributing to global sustainability.
While the traditional definition of sustainable agriculture focused on incorporating new practices that deal with ecosystem services, this new, technology-focused sustainable agriculture transitions from a site-specific management focus to the notion of global sustainability. To accomplish this transition, we introduced the WebGIS framework as an organizing principle that connects local, site-specific data generators called smart farms to a regional and global view of agriculture that can support both the agriculture industry and policy makers in government.
Automation and the use of AI, IoT, drones, robots, and Big Data serve as a basis for “Digital Twins,” which could allow for simulations of new ideas that can be tested virtually to determine environmental impact before implementation in the real world. In other words, constructing new practices in the virtual world will reduce the time to deploy new practices that lead to better environmental outcomes. If we are to feed 10 billion people by 2100 while preserving our environment, the next green revolution must incorporate the virtual world.
Author Contributions
All authors listed have made a substantial, direct and intellectual contribution to the work, and approved it for publication.
Disclaimer
Trade and manufacturer's names are necessary to report factually on available data; however, the USDA neither guarantees nor warrants the standard of the product, and the use of the name by USDA implies no approval of the product to the exclusion of others that may also be suitable. USDA is an equal opportunity provider and employer.
Conflict of Interest Statement
The authors declare that the research was conducted in the absence of any commercial or financial relationships that could be construed as a potential conflict of interest.
Acknowledgments
The authors would like to extend a special thanks to Dr. Charlie Walthall for inspiring this work and improving our knowledge of airplanes. We would also like to thank Jack Dangermond and Nicholas Short, Sr. for long ago inspiring us to go into this field.
Footnotes
1. ^Applying GIS and remote sensing to landscape genetics and genome size research. Available online at: https://pdfs.semanticscholar.org/3054/4c8c85b5bfc7e3f07725329376120473178a.pdf
2. ^Edge computing Wiki. https://en.wikipedia.org/wiki/Edge_computing
3. ^Adoption of computer based information systems: The case of dairy farmers in Canterbury, NZ, and Florida, Uruguay. Available online at: https://mentor.ieee.org/802.22/dcn/19/22-19-0013-00-0000-tutorial-on-whitespaces.pdf
4. ^Digital Twin Wiki. Available online at: https://en.wikipedia.org/wiki/Digital_twin
References
Ampatzidis, Y., De Bellis, L., and Luvisi, A. (2017). iPathology: robotic applications and management of plants and plant diseases. Sustainability 9:1010. doi: 10.3390/su9061010
Antle, J., Jones, J., and Rosenzweig, C. (2017). Next Generation agricultural system data, models and knowledge products: introduction. Agric. Sys. 155, 186–190. doi: 10.1016/j.agsy.2016.09.003
Beal, G., and Bohlen, J. (1957). The Diffusion Process. Special Report No. 18. Iowa Agricultural and Home Economics Station Publications, Iowa State University.
Berry, J. K., Delgado, J. A., Khosla, R., and Pierce, F. J. (2003). Precision conservation for environmental sustainability. J. Soil Water Conserv. 58, 332–339.
Berry, J. K., Delgado, J. A., Pierce, F. J., and Khosla, R. (2005). Applying spatial analysis for precision conservation across the landscape. J. Soil Water Conserv. 60, 363–370.
Blumenfeld, J. (2019). Meeting Data User Needs: A Look Behind the Curtain. Available online at: https://earthdata.nasa.gov/meeting-data-user-needs
Bongiovanni, R., and Lowenberg-DeBoer, J. (2004). Precision agriculture and sustainability. Prec. Agric. 5, 359–387. doi: 10.1023/B:PRAG.0000040806.39604.aa
Bouis, H., and Saltzman, A. (2017). Improving nutrition through biofortification: a review of evidence from HarvestPlus, 2003 through 2016. Global Food Sec. 12, 49–58. doi: 10.1016/j.gfs.2017.01.009
Buman, T. (2016a). Testimony of Tom Buman, CEO of Agren. For the House Agriculture Sub-Committee on Conservation Forestry. HearingTopic: Solutions Through Voluntary and/or Locally Led Conservation Efforts. Washington, DC: House Committee on Agriculture. Available online at: https://agriculture.house.gov/uploadedfiles/buman_testimony.pdf (accessed August 31, 2018).
Buman, T. (2016b). Voluntary Conservation Solutions. Carroll, IA: Agren Tools. Available online at: https://precisionconservation.com/voluntary-conservation-solutions/ (accessed August 30, 2018).
Campbell, W., Hill, S., and Cromp, R. (1989). Automatic labeling and characterization of objects using artificial neural networks. Telemat. Inform. 6, 259–271. doi: 10.1016/S0736-5853(89)80021-8
Campbell, W., Short, N., Coronado, P., and Cromp, R. (1994). “Distributed earth science validation centers for mission to planet earth,” in Methodologies for Intelligent Systems. ISMIS 1994. Lecture Notes in Computer Science (Lecture Notes in Artificial Intelligence), Vol. 869, eds Z. W. Raś and M. Zemankova (Berlin; Heidelberg: Springer), 1–12.
Chesapeake Conservancy (2018). Precision Conservation. Annapolis, MD: Chesapeake Conservancy. Available online at: http://chesapeakeconservancy.org/conservation-innovation-center/precision-conservation/ (accessed August 30, 2018).
Cowling, E., Galloway, J., Furiness, C., Erisman, J. W., et al. (2002). “Optimizing nitrogen management and energy production and environmental protection,” in Presented at the Second International Nitrogen Conference (Potomac, MD: Bolger Center). Available at www.initrogen.org/fileadmin/user_upload/Second_N_Conf_Report.~pdf
Dangermond, J. (2018). “The Emergence of a Geospatial Cloud and the Continuing Evolution of Cloud Computing”, ArcNews, Spring 2019, Vol. 41, No. 2.
Davis, D., Bennett, T., and Short, N. Jr. (1994). “A low-cost transportable ground station for capture and processing of direct broadcast EOS satellite data,” in Proceedings from the 3rd International Symposium on Space Mission Operations and Ground Data Systems, Space Ops '94, Part 1 (Greenbelt, MD), 187–195.
Del Grosso, S. J., White, J. W., Wilson, G., Vandenberg, B., Karlen, D. L., Follett, R. F., et al. (2013). Introducing the GRACEnet/ REAP data contribution, discovery, and retrieval system. J. Env. Qual. 42, 1274–1280. doi: 10.2134/jeq2013.03.0097
Delgado, J. A. (2016). “4 Rs are not enough. We need 7 Rs for nutrient management and conservation to increase nutrient use efficiency and reduce off-site transport of nutrients,” in Soil Specific Farming: Precision Agriculture. Advances in Soil Science Series, eds R. Lal and B. A. Stewart (Boca Raton, FL: CRC Press, 89–126.
Delgado, J. A., and Berry, J. K. (2008). Advances in precision conservation. Adv. Agron. 98, 1–44. doi: 10.1016/S0065-2113(08)00201-0
Delgado, J. A., Groffman, P. M., Nearing, M. A., Goddard, T., Reicosky, D., Lal, R., et al. (2011). Conservation practices to mitigate and adapt to climate change. J. Soil Water Conserv. 66, 118A−129A. doi: 10.2489/jswc.66.4.118A
Delgado, J. A., Gross, C. M., Lal, H., Cover, H., Gagliardi, P., McKinney, S. P., et al. (2010). A new GIS nitrogen trading tool concept for conservation and reduction of reactive nitrogen losses to the environment. Adv. Agron. 105, 117–171. doi: 10.1016/S0065-2113(10)05004-2
Delgado, J. A., Sassenrath, G., and Mueller, T. (ed.) (2018a). Precision Conservation: Geospatial Techniques for Agricultural and Natural Resources Conservation. Agronomy Monograph 59. Madison, WI: ASA, CSSA, and SSSA.
Delgado, J. A., Shaffer, M. J., Lal, H., McKinney, S. P., Gross, C. M., and Cover, H. (2008). Assessment of nitrogen losses to the environment with a nitrogen trading tool (NTT). Comput. Electron. Agric. 63, 193–206. doi: 10.1016/j.compag.2008.02.009
Delgado, J. A., Vandenberg, B. C., Kaplan, N. E., Neer, D. L., Wilson, G. J. D., et al. (2018b). Agricultural collaborative research outcomes system: AgCROS - An emerging network of networks for national food and environmental security and human health. J. Soil Water Conserv. 73, 158A−164A. doi: 10.2489/jswc.73.6.158A
Delgado, J. A., Weyers, S., Dell, C., Harmel, D., Kleinman, P., Sistani, K., et al. (2016). USDA agricultural research service creates Nutrient Uptake and Outcome Network (NUOnet). J. Soil Water Conserv. 71, 147A−148A. doi: 10.2489/jswc.71.6.147A
Dolan, M., Holden, C., Beard, M., and Bult, C. J. (2006). Genomes as geography: using GIS technology to build interactive genome feature maps. BMC Bioinform. 7:416. doi: 10.1186/1471-2105-7-416
Dubrovsky, N. M., Burow, K. R., Clark, G. M., Gronberg, J. A. M., Hamilton, P. A., Hitt, K. J., et al. (2010). The Quality of Our Nation's Waters - Nutrients in the Nation's Streams and Groundwater, 1992-2004. Circular-1350. U.S. Geological Survey, Sacramento, CA.
Follett, J. R., Follett, R. F., and Herz, W. C. (2010). “Environmental and human impacts of reactive nitrogen,” in Advances in Nitrogen Management for Water Quality, eds J. A. Delgado and R. F. Follett (Ankeny, IA: Soil and Water Conservation Society) 1–37.
Frantz, R. (n.d.). About Global Agriculture and Disaster Assessment System. Available online at: https://geo.fas.usda.gov/GADAS/pageDirectories/about/about.html
Fulton, J., and Darr, M. (2018). “GPS, GIS, guidance, and variable rate technologies for conservation management,” in Precision Conservation: Geospatial Techniques for Agricultural and Natural Resources Conservation. Agronomy Monograph 59, eds J. Delgado, G. Sassenrath, and T. Mueller (Madison, WI: ASA, CSSA, and SSSA, 65–82.
Galloway, J. N., Townsend, A. R., Erisman, J. W., Bekunda, M., Cai, Z., Freney, J. R., et al. (2008). Transformation of the nitrogen cycle: recent trends, questions, and potential solutions. Science 320, 889–892. doi: 10.1126/science.1136674
Gebbers, R., and Adamchuk, V. (2010). Precision agriculture and food security. Science 327, 828–831. doi: 10.1126/science.1183899
Goolsby, D. A., Battaglin, W. A., Aulenbach, B. T., and Hooper, R. P. (2001). Nitrogen input to the Gulf of Mexico. J. Environ. Qual. 30, 329–336. doi: 10.2134/jeq2001.302329x
Hammes, A. (2016). Precision Conservation Management Program Receives National Grant. Ames, IA: AgSolver. Available online at: https://medium.com/@amber_hammes/precision-conservation-management-program-receives-national-grant-97404c374ca2
Heartland Science Technology Group (2017). Precision Conservation Management. Champaign, IL: Heartland Science and Technology Group. Available online at: https://farmerportal.precisionconservation.org/authentication/signup
Hutchinson, G. L., Mosier, A. R., and Andre, C. E. (1982). Ammonia and amine emissions from a large cattle feedlot. J. Env. Qual. 11, 288–293. doi: 10.2134/jeq1982.00472425001100020028x
Illinois Sustainable Ag Partnership (2018). Precision Conservation Management. East Peoria, IL. Available online at: http://ilsustainableag.org/programs/precision-conservation-management/ (accessed August 30, 2018).
Jenrich, M. (2011). Potential of precision conservation agriculture as a means of increasing productivity and incomes for smallholder farmers. J. Soil Water Conserv. 66, 171–174. doi: 10.2489/jswc.66.6.171A
Kuehne, G., Llewellyn, R., Pannell, D., Wilkinson, R., Dolling, P., Ouzman, J., et al. (2017). Predicting farmer uptake of new agricultural practices: a tool for research extension and policy. Agricult. Sys. 156, 115–125. doi: 10.1016/j.agsy.2017.06.007
Legg, J. O., and Meisinger, J. J. (1982). “Soil nitrogen budgets,” in Nitrogen in Agricultural Soils, Agronomic Monograph 22, ed F. J. Stevenson (Madison, WI: American Society of Agronomy, 503–557.
Lin, F., Zhang, D., Huang, Y., Wang, X., and Chen, X. (2017). Detection of corn and weed species by the combination of spectral, shape, and textural features. Sustainability 9:1335; doi: 10.3390/su9081335
Luccio, M. (2014). Satellite Imagery for Precision Agriculture. Available online at: https://www.xyht.com/enviroag/satellite-imagery-precision-agriculture/
Matson, P. A., Parton, W. J., Power, and Swift, M. J. (1997). Agricultural intensification and ecosystem properties. Science 277, 504–509. doi: 10.1126/science.277.5325.504
McConnell, M. D., and Burger, L. W. Jr. (2018). “Precision conservation to enhance wildlife benefits in agricultural landscapes,” in Precision Conservation: Geospatial Techniques for Agricultural and Natural Resources Conservation. Agronomy Monograph 59, eds J. Delgado, G. Sassenrath, and T. Mueller (Madison, WI: ASA, CSSA, and SSSA, 285–312.
Moore, G. A. (1991). Crossing The Chasm: Marketing and Selling Technology Products to Mainstream Customers. New York, NY: Harper Business.
Noel, A. (2019). Data Becomes Cash Crop in Big Agriculture. Available online at: https://www.bloomberg.com/news/articles/2019-03-13/data-becomes-cash-crop-for-big-agriculture agriculture (accessed March 13, 2010).
Paustian, K., Easter, M., Brown, K., Chambers, A., Eve, M., Huber, A., et al. (2018). “Field- and farm-scale assessment of soil greenhouse gas mitigation using COMET-farm,” in Precision Conservation: Geospatial Techniques for Agricultural and Natural Resources Conservation. Agronomy Monograph 59, eds J. Delgado, G. Sassenrath, and T. Mueller (Madison, WI: ASA, CSSA, and SSSA, 341–360.
Pierce, F. J., and Nowak, P. (1999). Aspects of precision agriculture. Adv. Agron. 67:1–85. doi: 10.1016/S0065-2113(08)60513-1
Pinter, P., Ritchie, J., Hatfield, J., and Hart, G. (2003). The Agricultural Research Service's remote sensing program: an example of interagency collaboration. Photogram. Engin. Remote Sensing. 69, 615–618. doi: 10.14358/PERS.69.6.615
Pruski, F. F., and Nearing, M. A. (2002). Runoff and soil loss responses to changes in precipitation: a computer simulation study. J. Soil Water Conserv. 57, 7–16.
Rabalais, N. N., Turner, N. E., and Scavia, D. (2002b). Beyond science into policy: Gulf of Mexico hypoxia and the Mississippi River. Bioscience 52, 129–142. doi: 10.1641/0006-3568(2002)052[0129:BSIPGO]2.0.CO;2
Rabalais, N. N., Turner, R. E., and Wiseman, W. J. Jr. (2002a). Gulf of Mexico hypoxia, a.k.a. The dead zone. Annu. Rev. Ecol. Evol. Syst. 33, 235–263. doi: 10.1146/annurev.ecolsys.33.010802.150513
Ribaudo, M., Delgado, J. A., Hansen, L., Livingston, M., Mosheim, R., and Williamson, J. (2011a). Nitrogen in Agricultural Systems: Implications for Conservation Policy. ERS: Economic Research Report Number 127. Washington, DC, 1–82.
Ribaudo, M., Delgado, J. A., and Livingston, M. (2011b). Preliminary assessment of the potential for nitrous oxide offsets in a cap and trade program. Agric. Resour. Econ. Rev. 40, 1–16. doi: 10.1017/S1068280500008054
Ribaudo, M. O., Heimlich, R., and Peters, M. (2005). Nitrogen sources and Gulf hypoxia: potential for environmental credit trading. Ecol. Econ. 52, 159–168. doi: 10.1016/j.ecolecon.2004.07.021
Roberts, T. L. (2007). Right product, right rate, right time, and right place…the foundation of best management practices for fertilizer, in Fertilizer Best Management Practices: General Principles, Strategies for Their Adoption, and Voluntary Initiatives vs Regulations. Paper presented at the IFA International Workshop on Fertilizer Best Management Practices, Brussels, Belgium, March 7-9, 2007 (Paris: International Fertilizer Industry Association), 29–32.
Rose, D., Sutherland, W., Parker, C., Lobbly, M., Winter, M., Morris, C., et al. (2016). Decision support tools for agriculture: towards effective design and delivery. Agric. Sys. 149, 165–174. doi: 10.1016/j.agsy.2016.09.009
Salas, W. (2010). Agricultural Strategies for Mitigating GHG Emission: DNDC Model and Case Studies. Available online at: https://ww3.arb.ca.gov/research/seminars/salas/salas.pdf
Saleh, A., and Osei, E. (2018). “Precision conservation and water quality markets,” in Precision Conservation: Geospatial Techniques for Agricultural and Natural Resources Conservation. Agronomy Monograph 59, eds J. Delgado, G. Sassenrath, and T. Mueller (Madison, WI: ASA, CSSA, and SSSA, 313–340.
Sassenrath, G. F., and Delgado, J. A. (2018). “Precision conservation and precision regulation,” in Precision Conservation: Geospatial Techniques for Agricultural and Natural Resources Conservation, eds J. A. Delgado, G. F. Sassenrath, and T. Mueller (Madison, WI: ASA, CSSA, and SSSA).
Schimmelphfenning, D. (2016). Farm Profits and Adoption of Precision Agriculture. Available online at: https://www.ers.usda.gov/webdocs/publications/80326/err-217.pdf?v=0, USDA ERS
Shama, J. (2017). Immediate Conditions Could Slow Adoption of Precision Agriculture, Expert Says. Available online at: https://www.agweek.com/news/4219103-immediate-conditions-could-slow-adoption-~precision-agriculture-expert-says
Shedekar, V. S., and Brown, L. C. (2018). “GIS and GPS applications for planning, design and management of drainage systems,” in Precision Conservation: Geospatial Techniques for Agricultural and Natural Resources Conservation. Agronomy Monograph 59, eds J. Delgado, G. Sassenrath, and T. Mueller (Madison, WI: ASA, CSSA, and SSSA, 209–230.
Short, N., Cromp, R., Campbell, W., Tilton, J., LeMoigne, J., Fekete, G., et al. (1995). Mission to planet earth: AI views the world. IEEE Expert 10, 24–34. doi: 10.1109/64.483009
Short, N. Jr., and Dickens, L. (1995). Automatic generation of products from terabyte-size geographical information systems using planning/scheduling. Int. J. Geog. Inform. Sys. 9, 47–65. doi: 10.1080/02693799508902024
Sims, T. (2019). Exclusive: Microsoft Registers Blockchain and AI Platform for Agriculture in Brazil. CointTelegraph: The Future of Money. Available online at: https://cointelegraph.com/news/exclusive-microsoft-registers-blockchain-and-ai-platform-for-agriculture-in-brazil
Smith, D. R., Wilson, R. S., King, K. W., Zwonitzer, M., McGrath, J. M., Harmel, R. D., et al. (2018). Lake Erie, phosphorus, and microcystin: is it really the farmer's fault? J. Soil Water Conserv. 73, 48–57. doi: 10.2489/jswc.73.1.48
Smith, R. A., Schwarz, G. E., and Alexander, R. B. (1997). SPARROW Surface Water-Quality Modeling Nutrients in Watersheds of the Conterminous United States: Model Predictions for Total Nitrogen (TN) and Total Phosphorus (TP). Available online at http://water.usgs.gov/nawqa/sparrow/wrr97/results.html
Spiegal, S., Bestelmeyer, B. T., Archer, D. W., Augustine, D. J., Boughton, E. H., Boughton, R. K., et al. (2018). Evaluating strategies for sustainable intensification of US agriculture through the long-term agroecosystem research network. Environ. Res. Lett. 13:034031. doi: 10.1088/1748-9326/aaa779
Steiner, J. L., Sadler, E. J., Wilson, G. J., Hatfield, J. L., James, D. E., Vandenberg, B. C., et al. (2009). STEWARDS watershed data system: system design and implementation. Trans. ASABE. 52, 1523–1533. doi: 10.13031/2013.29141
SWCS (2003). Conservation Implications of Climate Change: Soil Erosion and Runoff From Cropland. A Report From the Soil and Water Conservation Society. Ankeny, IA: Soil and Water Conservation Society.
Tayyebi, A. (2019). High Resolution Land Cover Mapping Using Deep Learning. Available online at: https://medium.com/geoai/high-resolution-land-cover-mapping-using-deep-learning-7126fee571dd
Thompson, A., and Sudduth, K. (2018). “Terracing and contour farming,” in Precision Conservation: Geospatial Techniques for Agricultural and Natural Resources Conservation. Agronomy Monograph 59, eds J. Delgado, G. Sassenrath, and T. Mueller (Madison, WI: ASA, CSSA, and SSSA, 151–164.
Tyler, M., and Griffin, T. (2016). “Defining the barriers to telematics for precision agriculture: connectivity supply and demand,” in Southern Agricultural Economics Association 2016 Annual Meeting (San Antonio, TX). Available online at: https://ageconsearch.umn.edu/record/230090/files/Paper169.pdf
U.S. Environmental Protection Agency (2010). Inventory of U.S. Greenhouse Gas Emissions and Sinks: 1990-2008. EPA 430-R-10-006.
United Nations (2018). Statement by the Secretary-General on the IPCC Special Report Global Warming of 1.5°C. Available online at: https://www.un.org/sg/ en/content/sg/statement/2018-10-08/ statement-secretary-general-ipcc-special-report-global-warming-15-%C2%BAc (accessed October 8, 2018).
United States Environmental Protection Agency. (2015). 2015 Drinking Water Health Advisories for Two Cyanobacterial Toxins. Washington, DC: Office of Water. 820F15003. Available online at: https://www.epa.gov/sites/production/files/2017-06/documents/cyanotoxins-fact_sheet-2015.pdf
University of New Hampshire (2012). User's Guide for the DNDC Model. Institute for the Study of Earth, Oceans, and Space.
URISA (2018). Geospatial Fact Sheet: Geospatial Data Act of 2018. Available online at: https://www.urisa.org/clientuploads/directory/Documents/Advocacy/URISA_GeospatialFactShe~et_GeospatialDataAct_Nov2018.pdf
USDA-NRCS (2017). NRCS & Precision Conservation Management. Available online at: https://www.nrcs.usda.gov/wps/portal/nrcs/detail/?navtype=SUBNAVIGATION&ss=161017&cid=NRCSEPRD1372142&navid=105100000000000&pnavid=105000000000000&position=News&ttype=detail
USDA-NRCS (2018). Environmental Quality Incentives Program. Washington, DC: National Resources Conservation Service. Available online at: https://www.nrcs.usda.gov/wps/portal/nrcs/main/national/programs/financial/eqip/
Van der Wal, T. (2019). Why is Adoption of Precision ag so Slow? Available online at: https://www.futurefarming.com/Smart-farmers/Articles/2019/1/Why-is-adoption-of-precision-~ag-so-slow-385338E/
Vasisht, D.l, Kapetanovic, Z., Won, J., Jin, X., Chandra, R., Kapoor, A., Sinha, S., et al. (2017). “FarmBeats: an IoT platform for data-driven agriculture,” in Proceedings of the 14th USENIX Symposium on Networked Systems Design and Implementation (NSDI'17), March 2017 (Boston, MA).
Verdouw, C., and Kruize, J. (2015). “Digital twins in farm management: illustrations from the FIWARE accelerators SmartAgriFood and Fractals,” in 7th Asian-Australasian Conference on Precision Agriculture, 2015 (Hamilton: Precision Agriculture Association New Zealand).
Vitousek, P. M., Aber, J. D., Howarth, R. W., Likens, G. E., Matson, P. A., Schindler, D. W., et al. (1997). Human alteration of the global nitrogen cycle: sources and consequences. Ecol. Appl. 73, 737–750. doi: 10.1890/1051-0761(1997)007[0737:HAOTGN]2.0.CO;2
Walthall, C. L., Hatfield, J., Backlund, P., Lengnick, L., Marshall, E., Walsh, M., et al. (2012). Climate Change and Agriculture in the United States: Effects and Adaptation. USDA Technical Bulletin 1935. Washington, DC: USDA.
Keywords: big data, analytics, remote sensing—GIS, artificial intelligence, precision agriculture, sustainable agriculture
Citation: Delgado JA, Short NM Jr, Roberts DP and Vandenberg B (2019) Big Data Analysis for Sustainable Agriculture on a Geospatial Cloud Framework. Front. Sustain. Food Syst. 3:54. doi: 10.3389/fsufs.2019.00054
Received: 30 April 2019; Accepted: 27 June 2019;
Published: 16 July 2019.
Edited by:
Urs Feller, University of Bern, SwitzerlandReviewed by:
Luciano Gebler, Brazilian Agricultural Research Corporation (EMBRAPA), BrazilTahira Fatima, Purdue University, United States
Copyright © 2019 Delgado, Short, Roberts and Vandenberg. This is an open-access article distributed under the terms of the Creative Commons Attribution License (CC BY). The use, distribution or reproduction in other forums is permitted, provided the original author(s) and the copyright owner(s) are credited and that the original publication in this journal is cited, in accordance with accepted academic practice. No use, distribution or reproduction is permitted which does not comply with these terms.
*Correspondence: Nicholas M. Short Jr., bnNob3J0QGVzcmkuY29t