- 1Department of Earth Sciences, School of Science and Applied Technology, Laikipia University, Nyahururu, Kenya
- 2World Agroforestry Centre (ICRAF), Nairobi, Kenya
- 3World Agroforestry Centre (ICRAF), Dar es Salaam, Tanzania
- 4CGIAR Research Program on Climate Change, Agriculture, and Food Security (CCAFS), Kinshasa, Democratic Republic of the Congo
- 5World Agroforestry Centre (ICRAF), Kinshasa, Democratic Republic of the Congo
Climate-smart agriculture (CSA) is agriculture that increases productivity, improves resilience, and mitigates climate change. Smallholder adoption of farming technology is necessary to speed the transition to CSA. Here, we assessed the determinants of adoption of five technologies that can help achieve some of the CSA outcomes in smallholder farms in Tanzania. They included crop and livestock diversity, irrigation, application of chemical fertilizers, and agroforestry. Using data collected from 821 farming households, a multivariate probit model was employed to evaluate the determinants of adoption, allowing for examination of synergies and trade-offs between the technologies. Application of chemical fertilizers was fairly well adopted (34% of farmers), while irrigation was least adopted (26%). Some technologies, including crop diversity and irrigation as well as application of chemical fertilizer and agro-forestry, complemented each other. Trade-offs were observed between livestock diversity and irrigation. Female control of farm resources, farm location, and household resources were major determinants of adoption. We, therefore, recommend strategies that seeks to enhance building household resource as pathway for improved adoption of new technologies.
Introduction
Countries throughout Sub-Saharan Africa (SSA) need to increase food production to meet demand and predicted dietary changes (Tilman and Clark, 2014) under an increasingly inhospitable climate (van Ittersum et al., 2016). This change will have to be achieved alongside reductions in greenhouse gas (GHG) emissions. Current agricultural management practices and the associated land use changes account for one-third of total GHG emissions in Africa (IPCC, 2014). There is significant political will to increase agricultural productivity under climate change while reducing its impact on the environment. Increasing resilient productivity and reducing emissions from agriculture are central components of 42 African countries' Nationally Determined Contributions, which outline their intended climate actions under the Paris Agreement (Richards et al., 2016; Wollenberg et al., 2016). For example, the United Republic of Tanzania is creating an enabling environment for agricultural transformation, setting policies, creating learning alliances, and specifically naming Climate-Smart Agriculture as a policy priority for the country (United Republic of Tanzania, 2016).
Climate-Smart Agriculture (CSA) is an approach to agricultural development that aims to address the intertwined challenges of food security and climate change (Lipper et al., 2014). CSA targets three objectives: (i) sustainably increasing agricultural productivity to support equitable increases in farm incomes, food security, and development; (ii) adapting and building resilience of food systems to climate change; and (iii), where possible, reducing greenhouse (GHG) emissions from agriculture (FAO, 2013). Whether a technology is CSA is based on its impact on these outcomes and agricultural interventions that meet these goals are considered “climate-smart” (FAO, 2013). Interventions ranging from climate information services to field management have potential to achieve these goals (Faurès et al., 2013; Khatri-Chhetri et al., 2016; Nyasimi et al., 2017).
Synergies and co-benefits are characteristic of climate-smart agricultural interventions. Many farm-based management practices and technologies deliver two or three of the three ‘climate-smart' benefits. For example, Reppin et al. (2019) found that agroforestry system trees in western Kenya provide firewood for household consumption, timber for income generation, as well as carbon sequestration (of about 4.07 Mg C/ha). Similarly, diversifying cropping practices in Tanzania and Zimbabwe significantly improved crop productivity, income from crops, and food security indicators, as measured by food consumption scores and household dietary diversity scores (Makate et al., 2016; Kimaro et al., 2019). In addition, crop diversity increases resilience and biodiversity on farm, improves soil fertility, and controls pest and diseases (Truscott et al., 2009; Lin, 2011). In another study, adoption of conservation agriculture and agroforestry improved maize production, increased resilience/adaptation to climate change, and offered mitigation benefits in Tanzania (Kimaro et al., 2016a,b, 2019). In north-west Ethiopia, households who adopted and maintained CSA technologies between 2015 and 2017 increased farm level production by 22% over non-adopters as a result of reduced climate-related risks (Asrat and Simane, 2017). CSA technology adoption at scale could thus achieve substantially improved food availability while reducing the impacts of climate change.
Despite the potential benefits, adoption of CSA-relevant technologies is still generally low, especially in SSA. For instance, adoption of maize-legume rotation in Tanzania, minimum tillage in Malawi, and soil water conservation (ridges and soil bunds) in both Kenya and Tanzania are below 10% of farmers (Tesfaye et al., 2017). Meanwhile, other technologies have been adopted at scale. For example, improved varieties of maize are used by 62% of farmers in western Kenya (Mungai et al., 2017) and nearly 95% of farmers use improved crop varieties in Lushoto, Tanzania (Nyasimi et al., 2017). Differences in rates of diffusion suggests unique constraints to the uptake of each technology.
Diverse factors have been associated with the adoption, or dis-adoption, of technologies across a range of studies in SSA (Knowler and Bradshaw, 2007; Kassie et al., 2013, 2015; Teklewold et al., 2013; Ndiritu et al., 2016; Wainaina et al., 2016; Kurgat et al., 2018). The findings reveal that household characteristics, household asset base, institutional (i.e., input–output markets, extension services, and social groups) and farm characteristics, access to information, and belief systems influence adoption of CSA technologies. However, the pattern of influence of these factors are often context-specific, depending on location as well as the technologies evaluated. This implies that few universal factors regularly explain adoption.
As efforts to scale-up the adoption of CSA in Tanzania and across Africa continue, it is critical to understand how complex factors, including characteristics of households, farming systems, and technologies, impact adoption of agricultural innovations across diverse contexts. Here, we implemented a multi-indicator farming household survey across four regions in Tanzania, each with a different predominant agroecological zone. We examined (i) the level of adoption of five technologies often considered to be CSA across the regions, (ii) determinants to adoption of these technologies, and (iii) complementarities and substitutabilities between technologies.
Methodology
Study Site
The study was conducted in four areas in Tanzania: Iringa, Zanzibar, Dodoma, and Tabora. These regions were selected to account for the diversity of farming systems in Tanzania, which range from semi-arid maize and sorghum systems, to humid coastal agricultural systems dominated by cassava and fruit production (Table 1). More than 800 farming households were surveyed: Iringa (N = 209), Zanzibar (N = 199), Dodoma (N = 202), and Tabora (N = 211). Districts within each region were selected purposefully in consultation with local district agricultural offices to ensure the objectivity of survey teams. Within each district, households were selected randomly from all villages within sampled area.
Data Collection
Four enumerators were selected and trained in each of the four regions to ensure familiarity with local farming systems and customs. At each household, either the household head or their spouse was interviewed using the Rural Household Multi-Indicator Survey (RHoMIS), a survey instrument for rapid and comparable characterization of rural farming systems and livelihoods (Hammond et al., 2017, RHoMIS 2020; www.rhomis.org). RHoMIS collects data on household characteristics, crop and livestock production, physical and natural capital resources (e.g., land ownership, land tenure, and finances), food security, poverty, gender, and nutrition. Complementary data were also collected on the use of various agricultural technologies, including agroforestry, irrigation, use of fertilizers, and crop and livestock diversification. All data were collected in the field using Open Data Kit (ODK; Brunette et al., 2013) on Android devices and uploaded daily to a centralized ONA server.
Model Specification
We used a multivariate probit (MVP) model to capture farmers' decision-making process for adoption of single and combinations of practices and technologies. The MPV model also allows us to understand the determinants of adoption for technologies themselves and evaluate the interconnectedness of different practices by assessing their correlations, a phenomenon which univariate multinomial logit and probit models ignore (Kassie et al., 2013). A range of factors that could influence farmers' decisions to adopt CSA practices were considered, including household characteristics, asset base and institutional (Table 2).
To describe the MVP model, adoption of technologies was indicated by a series of binary variables CSAi, where each technology is assigned a unique index i taking on the values (1, 2, 3, 4, and 5) for a positive integer, in this case representing all the CSA technologies, and letting X denote a set of conditioning variables. Therefore, the CSA technology chosen by any farming household was represented by random variables (CSAi). It was assumed that each farmer may consider a combination of technologies, which were further assumed to depend on a set of the households' characteristics and resources, farm location, food security status, and other factors (X). Therefore, the MVP model was characterized by a set of binary dependent variables (CSAipn) such that
and
where, is the corresponding vector of parameters to be estimated, and is the latent variable. Equation (2) assumes that a rational farmer has a latent variable, , that captures the unobserved preferences associated with the nth choice of CSA technology. This latent variable was assumed to be a linear combination of both household characteristics, household resources, location factors, and household food security status (Xipn) that are observed to be influencing the simultaneous selection of technologies, as well as the unobserved characteristics that are captured by the stochastic error term uipn. Owing to the nature of the latent variable, the estimations in this study were based on observable binary discrete variables CSAipn, which indicate whether or not a farming household has adopted a particular technology.
Data and Description of Variables
The definition and descriptive statistics of all the variables used in the analysis are presented in Table 2. The variables are grouped as dependent (i.e., technologies and practices) and explanatory variables.
Dependent Variables
Five technologies were considered and analyzed in this paper with regard to level of adoption, complementarities, substitutability, and factors influencing adoption. These are crop diversity (planting more than one crop type in intercropping and/or rotation; livestock diversity (keeping more than on type of livestock on-farm); agroforestry (inclusion of trees in agricultural fields); irrigation (of any type, including drip irrigation and traditional systems, such as fanya juu/fanya chini); and use of fertilizers (of any type). Rather than selecting these technologies in conjunction with farmers, we selected these five technologies based on priori assumptions that each can deliver one or more CSA goals (Bell et al., 2018), they are relevant to the farming systems in question, and there is political interest in adoption of these technologies as per the Tanzanian Ministry of Agriculture CSA Guidelines. Adoption of practices was self-reported in response to yes/no questions. In addition, livestock and crop diversity was used a proxy indicator of household farm diversification. This is because our research was cross-sectional in nature, and the data were collected during one time period. A number of studies (i.e., Kassie et al., 2013; Teklewold et al., 2013; Ndiritu et al., 2016; Wainaina et al., 2016; Kurgat et al., 2018) have also used crop and livestock diversity indicator to denote diverse agricultural farming systems in SSA. However, we note that crop-livestock diversity indicator does not capture changes of crop and livestock types over a given timeframe. Therefore, the interpretation and discussion of our findings is limited to the number of livestock and crop types at the time of data collection. We have also compared our findings with previous studies which also used same indicator.
Independent Variables
(i) Household characteristics
Household demographic characteristics are associated with decision-making in the adoption of farm-level technologies (Kassie et al., 2013; Asfaw et al., 2014; Kurgat et al., 2018). We included household size, age of male and female heads of households, education level, and degree of female control of farm resources in the model. Older farmers are likely to have been exposed to extensive production technologies and environments, accrued more assets, and established wide social networks, and hence are more likely to adopt technologies. However, old age is also associated with loss of energy, risk aversion, and short-term investment planning (Kassie et al., 2013; Asfaw et al., 2014). Youths may be more likely to adopt these because of their ability to access more information on new technologies using the Internet. More educated farmers are more likely to go engage more on off-farm activities, with higher returns on labor, and will only invest in technologies if they offer better returns. On the other hand, educated farmers have a better ability to understand the benefits of CSA, and this may foster adoption. We hypothesized that the education level and age of head of household have a countervailing effect on the adoption technologies. With regards to control over farm resources, we hypothesized that those households with females controlling farm resources were more likely to adopt CSA technologies. This is because women play a critical role in enhancing food and nutritional security in Africa.
(ii) Farm resources
Land ownership, size, area under cultivation, livestock ownership, livestock holdings, as well as access to off-farm income were used to represent household resources. For example, livestock is a significant household asset in terms of food and nutritional security and income generation for farmers (Ngigi and Birner, 2013). We hypothesized that those households which access these resources were more likely to be adopters of technologies since they have the ability to pay for initial capital requirements.
With regard to livestock ownership and holding, we hypothesized a negative relationship on adoption of irrigation due to expected trade-offs of water allocation. Kurgat et al. (2018) found that those farmers who owned livestock were less likely to adopt improved irrigation systems for rural and peri-urban vegetable production in Kenya.
(iii) Location
Technology adoption studies consider agroecological factors at the farm or regional level (Kassie et al., 2015; Wainaina et al., 2016). This is because conditioning factors (such as infrastructure development, sector policies, market access, extension services, and information-related issues) more often vary between different locations as well as within farms. This explains why farmers adopt certain technologies on some farms but not on others or why they adopt certain technologies in some regions and not others. We therefore created a dummy variable for the household farm region and hypothesized that the location would influence adoption of the technologies. The dummy variable for households from Dodoma was excluded from the model due to multicollinearity.
Results
Household Demographics
Households averaged six members. Male and female heads of a household were, on average, 41 and 40 years, respectively. The majority (60%) of the households were male headed with 7 ± 4.3 years of schooling, implying that the maximum education was primary level. The vast majority (83%) of the respondents owned land, and the average landholding was 3.5 ± 5.7 acres while the land under cultivation was approximately 1.99 ± 2.3 acres on average.
Adoption Rates
Between 25 and 34% of households across the four regions had used at least one of the studied management practices and technologies on their farms during the previous growing season (Table 3). However, adoption rates varied by practice and region. Application of chemical fertilizers was 34% across all regions, with the highest adoption rates in Iringa (>42%) and use rates of just 18% in Dodoma and Zanzibar. Livestock diversity and irrigation were the least commonly used practices at 26% each across all regions, although 47% of households irrigated their farms in Zanzibar. Livestock diversity was highest in Iringa (42%) and lowest in Zanzibar (10%), where poultry were the most commonly owned livestock. Overall, 25% of the households had diverse crop production.
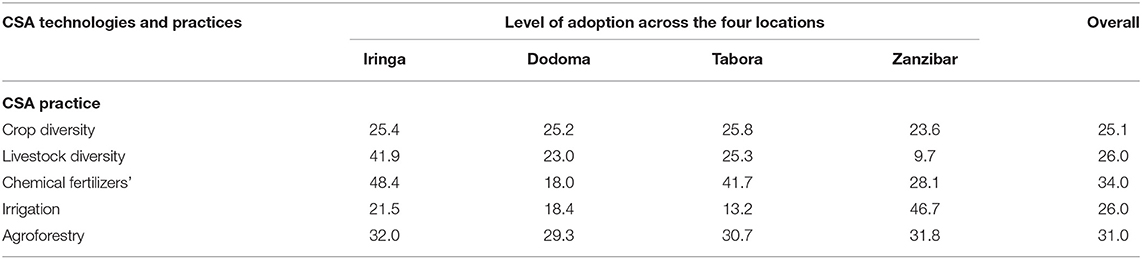
Table 3. Adoption levels of CSA technologies and practices per each location and the overall rate (%).
Improved practices were not independent events (likelihood ratio test (χ2 (10) = 30.659***). Five out of 10 coefficients of pairwise correlation were significantly correlated, indicating technologies are often, though not always, implemented in combination (Table 4).
Determinants of Adoption
The four sub-categories of independent variables had heterogeneous effects on the adoption and non-adoption (Table 5). Among the household characteristics, household size is positively associated with livestock diversity. Further, age of male headed household is associated with lower probability of uptake of irrigation while household where farm-based resources that are mainly controlled by female are more likely to diversify their crop and livestock production and apply chemical fertilizers. With regard to household resources, land ownership was positively associated with crop diversity and agroforestry, while land size cultivated, livestock ownership and holdings positively influenced diversity of livestock keeping. In addition, households that access off-farm income are likely to keep more types of livestock. Land ownership was negatively associated with livestock diversity. Households who hire farm labor were more likely to use chemical fertilizers but avoid irrigating their farms. The location dummies indicate that general location factors also play a significant role on adoption of CSA technologies.
Discussion
Similar studies in Tanzania and elsewhere in Sub-Saharan Africa, including Kenya, Malawi, and Ghana (Marenya and Barrett, 2007; Teklewold et al., 2013; Kassie et al., 2015; Kibrom et al., 2015), have found that technology use in Tanzania is often among the lowest in SSA, with rates of adoption ranging between 4 and 46%, vs. 19 and 80% in other countries, depending on the practice of interest. The rates of adoption found in this study were both higher and lower than previous studies in Tanzania. For example, Kassie et al. (2015) recorded only 4% adoption of inorganic fertilizer, while more than 30% of farmers surveyed in this study reported using inorganic fertilizer. In addition to farmer characteristics, the strong positive relationship between regions and adoption of technologies may be associated with various policy factors such as sector policies, subsidies, input–output market structures, and prices.
The numbers of farmers that use agroforestry in the four locations we sampled (31%) were less than that found by Nyasimi et al. (2017) in humid zones of the Usambara Mountains (85%). Different rates of agroforestry can most likely be linked to the biophysical characteristics to the landscapes. However, we found practically invariable rates of agroforestry adoption across our four sites and more than double (14%) that found in Mwungu et al. (2018), who surveyed farmers in similar sites to Iringa. We suspect the differences are due to external programming. Sites used by Mwungu et al. (2018) have been the focus of on-going government investment in soil management, while sites used here have a history of agroforestry programming. This suggests the stark, and perhaps catalytic, impact of the information and resources of donor funded programs. Similar magnitudes of differences in adoption rates were found for other practices, including irrigation (Kurgat et al., 2018) and crop diversity (Teklewold et al., 2013). This highlights the challenges to understanding the extent of agricultural change at a national level and reinforces the earlier work of Wainaina et al. (2016) and Kassie et al. (2013).
Disentangling the determinants of adoption is crucial to understanding causality. The determinants of adoption varied across technologies. The probability of diversifying livestock production, for example, tended to increase with the size of land holdings. This result is consistent with that of Mekuria and Mekonnen (2018) who found that each additional hectare of land in creases livestock diversity by 5.1% in the central highlands of Ethiopia. The congruence in the results in spite of radically different social and biophysical context suggests that increased land consistently facilitates livestock diversity. The likelihood of livestock diversity tended to further be influenced by the availability of off-farm income, as was found in crop-livestock systems in Ghana (Asante et al., 2017). However, it is important to note that this association is not consistent across sites, as other studies have found no effect of off-farm income (Kibrom et al., 2015). We associated the positive association between off-farm income and livestock diversity to the fact that off-farm income provides sufficient financial resources to purchase necessary inputs for livestock production (e.g., new breeds, artificial insemination services, veterinary services, and animal feeds). Another plausible explanation is that larger land areas allow farmers to cultivate a wider range of livestock feeds and crops to meet animal feed demand, thus reducing the quantity of feed purchased. Animal feed produced on-farm is relatively cheaper than purchased feeds.
The determinants of crop diversity differed from that of livestock. We found that land ownership and female control of resources were positively associated with crop diversity. Kassie et al. (2015) similarly found that land ownership drives crop diversity in maize–legume production in Tanzania. These results suggest the importance of social constructs both at household level and government institutions for crop diversity. Use of agroforestry in this study was also determined by social constructs. Households with land tenure security were more likely to practice agroforestry. This is consistent with conventional wisdom on the primary barriers to agroforestry (Pattanayak et al., 2003) and is typically related to the extended period of time necessary to reap benefits from trees.
Lack of available capital restricts adoption of other management practices as well and is a common theme throughout the agricultural technology literature (Kassie et al., 2015; Ndiritu et al., 2016; Kurgat et al., 2018). Age and the need to pay for labor were the primary negative determinants of adoption of irrigation, perhaps because the process of acquiring and setting up irrigation facilities is usually capital and labor intensive, particularly if the source of water is far from the household and if the household uses inefficient manual irrigation equipment. For instance, Danson et al. (2002) noted that, in peri-urban Ghanaian vegetable production, manual irrigation takes 38% of a farmer's time and high-water application rates (640–1,600 mm yr−1) all year round. Such energy-intensive technologies are likely to dissuade older farmers. Finally, we note that the determination of factors influencing adoption of CSA technologies using only models, such as MVP, without farmer participatory approaches as a follow-up to validate the results potentially raise the problem of reverse causality. Consequently, we note that the interpretation of our findings is limited by this problem. We also recommend further studies to include a follow-up focus discussion.
Certain technologies appear to most often be implemented alone. The negative relationship between irrigation and livestock diversity is similar to the findings of Kurgat et al. (2018) among smallholder vegetable producers in Kenya. This may suggest that farmers perceive tradeoffs or consider these practices as substitutes. Such trade-offs could take the form of competing water use needs. Farmers are more likely to allocate water to the production systems with higher water use efficiency or higher returns on investment. In addition, livestock can disturb irrigation lines, particularly in farmlands where farmers are using improved irrigation technologies, such as drip irrigation. Similarly, trade-offs between crop and livestock practices are evident with agroforestry. Land where young tree seedlings are growing needs to be protected from livestock. Location may also play a factor here: Dodoma is relatively dry and thus has low survival rate of tree seedlings (about 70–75%).
On the contrary, some of the analyzed technologies are often applied in combination. The complements involving use of chemical fertilizer and crop diversity is consistent with the work of Ndiritu et al. (2016) in maize-legume intercropping in Kenya. The complement between these practices may be due to increased fertilizer-use efficiency because different crops have different rooting systems, which increases uptake of fertilizer nutrient uptake from different soil depths. Similarly, the synergy between irrigation and crop diversity is attributed to alternative livelihood strategy and market orientation. Complements between agroforestry and crop diversity may be linked to the benefits of trees in recycling soil nutrients from below the crop root zone back to the upper soil layers. Livestock produce manure, which can substitute for expensive chemical fertilizers at minimal cost. When a set of practices complement each other, opportunities emerge to encourage farmers to create synergies on farms and adopt the package.
Conclusion
This study examined the determinants of adoption of five CSA technologies across four locations in Tanzania to gain clarity regarding what mechanisms and obstacles may hinder wide-scale change toward new agricultural practices. When considering determinants across the five practices, a few patterns emerge. First, 12 out of 16 variables analyzed significantly foster or constrain adoption. Secondly, no single variable significantly influenced adoption across all the practices. Thirdly, land ownership, female controlled resources, and farm-based resources variables significantly influenced adoption of three out the five practices, while the remaining 9 of the 12 significant ones influenced only one or two technologies. This would suggest that CSA programs in the four regions would do well to focus on improving land tenure rights and women's empowerment in household decision making. The existence of trade-offs and complements between the technologies suggests that policy and programmatic efforts that affect adoption of a given CSA technology may also influence the adoption of others. The site-specific nature of these findings reinforces the need to consider local factors and co-design solutions with the communities where programs and policies take effect. In addition, we recommend future studies that are similar to this to include participatory approach to validate the model results.
Data Availability Statement
The datasets generated for this study are available on request to the corresponding author.
Ethics Statement
This study aligns with the 1964 WMA declaration of Helsinki. Oral informed consent was built-in to the survey, survey participants had the possibility to skip questions, data were processed in anonymized way. Survey participants were not particularly vulnerable. The implementing partner, World Agroforestry Center (ICRAF), does not have an internal ethics committee. However, ICRAF has country office in Dares-salaam, Tanzania, and the mandate to conduct research in the country through Memorandum of Understanding with the Government of Tanzania. Development of the methodology and implementation of this study was guided by Senior Scientists to ensure compliance with best practice.
Author Contributions
BK, CL, and TR conceived of the experiment and designed the methodology. CL, LM, and NN collected the data. BK conducted the analysis. BK, CL, and TR wrote the manuscript. TR secured funding for the work. All authors reviewed and edited the manuscript.
Funding
This work was funded by the United Stated Department of Agriculture Foreign Agriculture Service. Supplementary support was provided by the Partnership for Scaling Climate-Smart Agriculture Project of the CGIAR Research Program on Climate Change, Agriculture, and Food Security (CCAFS) for supporting this research. CCAFS is carried out with support from the CGIAR Trust Fund and through bilateral funding agreements. For details please visit https://ccafs.cgiar.org/donors. USAID funds came from USAID to ICRAF/IPs via USDA.
Conflict of Interest
The authors declare that the research was conducted in the absence of any commercial or financial relationships that could be construed as a potential conflict of interest.
Acknowledgments
We thank all the farmers for agreeing to be interviewed.
References
Asante, B. O., Villano, R. A., Patrick, I. W., and Battese, G. E. (2017). Determinants of farm diversification in integrated crop–livestock farming systems in Ghana. Renew. Agric. Food Syst. 33, 1–19. doi: 10.1017/s174217051_60005_45
Asfaw, S., McCarthy, N., Lipper, L., Arslan, A., Cattaneo, A., and Kachulu, M. (2014). Climate Variability, Adaptation Strategies and Food Security in Malawi. ESA Working Paper No. 14-08. Rome, FAO.
Asrat, P., and Simane, B. (2017). “Adaptation benefits of climate-smart agricultural practices in the blue nile basin: empirical evidence from north-west ethiopia,” in: Climate Change Adaptation in Africa: Fostering Resilience and Capacity to Adapt, eds W. Filho, B. Simane, J. Kalungu, M. Wuta, P. Munishi, and K. Musiyiwa (AG Cham: Springer International Publishing, 45–59.
Bell, P., Namoi, N., Lamanna, C., Corner-Dollof, C., Girvetz, E., Thierfelder, C., et al. (2018). A Practical Guide to Climate-Smart Agricultural Technologies in Africa. CCAFS Working Paper no. 224. Wageningen, theNetherlands: CGIAR Research Program on Climate Change, Agriculture and Food Security (CCAFS). Available online at: www.ccafs.cgiar.org
Brunette, W. Sundt M., Dell, N. Chaudhri R., Breit, N, and Borriello, G. (2013). “Open data kit 2.0: expanding and refining information services for developing regions,” in HotMobile '13: Proceedings of the 14th Workshop on Mobile Computing Systems and Applications, eds W. Leal Filho, S. Belay, J. Kalangu, W. Menas, P. Munishi, and K. Musiyiwa, 1–6. doi: 10.1145/2444776.2444790
Danson, G., Drechsel, P., Wiafe-Antwi, T., and Gyiele, L. (2002). Income of farming systems around Kumasi, Ghana. Urban Agric. Magaz. 7, 5–6.
FAO (2013). Statistical Yearbook: World Food and Agriculture. Rome: Food and Agriculture Organization of the United Nations (FAO).
Faurès, J. M., Bartley, D., Bazza, M., Burke, J., Hoogeveen, J., Soto, D., et al. (2013). Climate Smart Agriculture Sourcebook. Rome: FAO, 557.
Hammond, J., Fraval, S., van Etten, J., Suchini, J. G., Mercado, L., Pagella, T., et al. (2017). The Rural Household Multi-Indicator Survey (RHoMIS) for rapid characterization of households to inform Climate Smart Agriculture interventions: description and applications in East Africa and Central America. Agric. Syst. 151, 225–233. doi: 10.1016/j.agsy.2016.05.003
IPCC (2014). “Climate Change 2014: Synthesis Report,” in Contribution of Working Group I, II and III to the Fifth Assessment Report of the Intergovernmental Panel on Climate Change, eds R. K. Pachauri and L. A. Meyer. (Geneva: IPPC).
Kassie, M., Jaleta, M., Shiferaw, B., Mmbando, F., and Mekuria, M. (2013). Adoption of interrelated sustainable agricultural practices in smallholder systems: evidence from rural Tanzania. Technol. Forecast. Soc. Chang. 80, 525–540. doi: 10.1016/j.techfore.2012.08.007
Kassie, M., Teklewold, H., Jaleta, M., Marenya, P., and Erenstein, O. (2015). Understanding the adoption of a portfolio of sustainable intensification practices in eastern and southern Africa. Land Use Policy 42, 400–411. doi: 10.1016/j.landusepol.2014.08.016
Khatri-Chhetri, A., Aryal, J. P., Sapkota, T. B., and Khurana, R. (2016). Economic benefits of climate-smart agricultural practices to smallholders' farmers in the indo-gangetic plains of India. Curr. Sci. 110, 1251–1256. doi: 10.18520/cs/v110/i7/1251-1256
Kibrom, T., Krishina, V., and Qaim, M. (2015). Production diversity and dietary diversity in smallholder farm households. Proc. Natl. Acad. Sci. U.S.A. 112, 10657–10662. doi: 10.1073/pnas.1510982112
Kimaro, A., Mpanda, M., Rioux, J., Aynekulu, E., Shaba, S., Thiong'o, M., Mutuo, P., et al. (2016b). Is conservation agriculture ‘climate-smart' for maize farmers in the highlands of Tanzania? Nutr. Cycl. Agroecosyst. 105, 217–228. doi: 10.1007/s10705-015-9711-8
Kimaro, A., Sererya, O., Matata, P., Uckert, G., Hafner, J., Graef, F., et al. (2016a). “Understanding the multidimensionality of climate-smartness: examples from agroforestry in Tanzania,” in The Climate-Smart Agriculture Papers, eds T. Rosenstock, A. Nowak, and E. Girvetz (Cham: Springer), 1–15.
Kimaro, A. A., Sererya, O. G., Matata, P., Uckert, G,. Hafn, J., Sieber, S., et al. (2019). “Understanding the multidimensionality of climate-smartness: examples from agroforestry in Tanzania,” in The Climate-Smart Agriculture Papers, eds T. Rosenstock, A. Nowak, E. Girvetz (Cham: Springer).
Knowler, D., and Bradshaw, B. (2007). Farmers' adoption of conservation agriculture: a review and synthesis of recent research. Food Policy 32, 25–48. doi: 10.1016/j.foodpol.2006.01.003
Kurgat, B. K., Stöber, S., Mwonga, S., Lotze-Campen, H., and Rosenstock, T. S. (2018). Livelihood and climate trade-offs in Kenyan peri-urban vegetable production. Agric. Syst. 160, 79–86. doi: 10.1016/j.agsy.2017.10.003
Lin, B. B. (2011). Resilience in agriculture through crop diversification: adaptive management for environmental change. Bioscience 61:183–193. doi: 10.1525/bio.2011.61.3.4
Lipper, L., Thornton, P., Campbell, B. M., Baedeker, T., Braimoh, A., Bwalya, M., et al. (2014). Climate-smart agriculture for food security, Nat. clim. Chang. 4, 1068–1072. doi: 10.1038/nclimate2437
Makate, C., Wang, R., Makate, M., and Mango, N. (2016). Crop diversification and livelihoods of smallholder farmers in Zimbabwe: adaptive management for environmental change. Springer Plus 5:1135. doi: 10.1186/s40064-016-2802-4
Marenya, P. P., and Barrett, C. B. (2007). Household-level determinants of adoption of improved natural resources management practices among smallholder farmers in western Kenya. Food Policy 32, 515–536. doi: 10.1016/j.foodpol.2006.10.002
Mekuria, W., and Mekonnen, K. (2018). Determinants of crop–livestock diversification in the mixed farming systems: evidence from central highlands of Ethiopia. Agric. Food Sec. 7:60. doi: 10.1186/s40066-018-0212-2
Mungai, C., Opondo, M., Outa, G., Nelson, V., Nyasimi, M., and Kimeli, P. (2017). “Uptake of climate-smart agriculture through a gendered intersectionality lens: experiences from Western Kenya,” in Climate Change Adaptation in Africa. Climate Change Management, eds W. Leal Filho, S. Belay, J. Kalangu, W. Menas, P. Munishi, and K. Musiyiwa (Cham: Springer).
Mwungu, C., Mwongera, C., Shikuku, K., Acosta, M., and Läderach, P. (2018). “Determinants of adoption of climate-smart agriculture technologies at farm plot level: an assessment from southern Tanzania,” in Handbook of Climate Change Resilience, ed W. Filho (Cham, AG: Springer International Publishing).
Ndiritu, S. W., Kassie, M., and Shiferaw, B. (2016). Are there systematic gender differences in the adoption of sustainable agricultural intensification practices? Evidence from Kenya. Food Policy, 49, 117–127. doi: 10.1016/j.foodpol.2014.06.010
Ngigi, M. W., and Birner, R. (2013). “Shocks, livestock assets and climate change adaptation in Kenya,” in Paper Prepared for Presentation at the 4th African Association of Agricultural Economics, AAEA/ AEASA Conference September 23-25 Hammamet, Tunis – Tunisia. Available online at: https://doi.org/https://ageconsearch.umn.edu/bitstream/161468/2/Marther%20W.%20Ngigi%20and%20Regina%20Birner.pdf
Nyasimi, M., Kimeli, P., Sayula, G., Radeny, M., Kinyangi, J., and Mungai, C. (2017). Adoption and dissemination pathways for climate-smart agriculture technologies and practices for climate-resilient livelihoods in lushoto, northeast tanzania. Climate 5, 2–22. doi: 10.3390/cli5030063
Pattanayak, S., Mercer, D., Sills, E., and Yang, J. (2003). Taking stock of agroforestry adoption studies. Agroforest. Syst. 57, 173–186. doi: 10.1023/A:1024809108210
Reppin, S., Kuyah, S., Neergaard, A., Oelofse, M., and Rosenstock, S. (2019). Contribution of agroforestry to climate change mitigation and livelihoods in Western Kenya. Agroforest. Syst. 94, 203–220. doi: 10.1007/s10457-019-00383-7.01234
Richards, M., Bruun, T. B., Campbell, B., Gregersen, L. E., Huyer, S., Kuntze, V., et al. (2016). How Countries Plan to Address Agricultural Adaptation and Mitigation: An Analysis of Intended Nationally Determined Contributions. CCAFS Dataset Version 1.2. Copenhagen: CGIAR Research Program on Climate Change, Agriculture and Food Security (CCAFS).
Teklewold, H., Kassie, M., Shiferaw, B., and Köhlin, G. (2013). Cropping system diversification, conservation tillage and modern seed adoption in Ethiopia: impacts on household income, agrochemical use and demand for labor. Ecol. Econ. 93, 85–93. doi: 10.1016/j.ecolecon.2013.05.002
Tesfaye, K., Kassie, M., Cairns, J., Michael, M., Stirling, C., Tsedeke Abate, M., et al. (2017). “Potential for scaling up climate smart agricultural practices: examples from sub-saharan africa,” in Climate Change Adaptation in Africa: Fostering Resilience and Capacity to Adapt, eds W. Filho, B. Simane, J. Kalungu, M. Wuta, P. Munishi, and K. Musiyiwa (Cham, AG: Springer International Publishing, 185–203
Tilman, D., and Clark, M. (2014). Global diets link environmental sustainability and human health. Nature. 515, 518–522. doi: 10.1038/nature13959
Truscott, L., Aranda, D., Nagarajan, P., Tovignan, S., and Travaglini, A. L. (2009). “A snapshot of crop diversification in organic cotton farms,” in Discussion paper. Soil Association.
United Republic of Tanzania (2016). World Food Program (WFP) Strategic Review 2016: Framework for Food and Nutrition Security in Tanzania. Available online at: https://docs.wfp.org/api/documents/WFP-0000019748/download/?_ga=2.47000800.610799115.1553932184-134515430.1553932184 (accessed March 30, 2019).
van Ittersum, M. K., van Bussel, L. G. J., Wolf, J., Grassini, P., van Wart, J., Guilpart, N., et al. (2016). Can sub-Saharan Africa feed itself? Proc. Natl. Acad. Sci. 113, 14964–14969. doi: 10.1073/pnas.1610359113
Wainaina, P., Tongruksawattana, S., and Qaim, M. (2016). Tradeoffs and complementarities in the adoption of improved seeds, fertilizer, and natural resource management technologies in Kenya. Agric. Econ. 47, 351–362. doi: 10.1111/agec.12235
Keywords: climate-smart agriculture, agricultural adoption, household surveys, multivariate probit model, Tanzania
Citation: Kurgat BK, Lamanna C, Kimaro A, Namoi N, Manda L and Rosenstock TS (2020) Adoption of Climate-Smart Agriculture Technologies in Tanzania. Front. Sustain. Food Syst. 4:55. doi: 10.3389/fsufs.2020.00055
Received: 21 October 2019; Accepted: 09 April 2020;
Published: 21 May 2020.
Edited by:
Alberto Sanz Cobeña, Centro de Estudios e Investigación para la Gestión de Riesgos Agrarios y Medioambientales (CEIGRAM), SpainReviewed by:
Mark Van Wijk, International Livestock Research Institute, KenyaPolly Joanna Ericksen, International Livestock Research Institute, Kenya
Copyright © 2020 Kurgat, Lamanna, Kimaro, Namoi, Manda and Rosenstock. This is an open-access article distributed under the terms of the Creative Commons Attribution License (CC BY). The use, distribution or reproduction in other forums is permitted, provided the original author(s) and the copyright owner(s) are credited and that the original publication in this journal is cited, in accordance with accepted academic practice. No use, distribution or reproduction is permitted which does not comply with these terms.
*Correspondence: Barnabas K. Kurgat, Ymt1cmdhdEBsYWlraXBpYS5hYy5rZQ==; Ymt1cmdhdEB5YWhvby5jb20=