- 1International Livestock Research Institute, Sustainable Livestock Systems, Nairobi, Kenya
- 2Institute for Meteorology and Climate Research, Atmospheric Environmental Research (IMK-IFU), Karlsruhe Institute of Technology (KIT), Karlsruhe, Germany
In this study we evaluate Climate Smart Agriculture (CSA) assessment tools with regard to their suitability for covering not only biophysical but also socio-economic aspects of CSA, focusing on smallholder household level in Low and Middle Income Countries (LMIC). In this opinion piece we give a concise overview of the most recent developments in measuring key indicators and metrics for the three pillars of CSA (food security, adaptation, and mitigation) and give our opinion on how we think this would allow for improvements in the current state of assessing CSA in a smallholder farming context. Our assessment shows that all tools reviewed here have a biophysical lens while looking at productivity, and largely ignore potential social (e.g., food security, gender) and economic (poverty) aspects of the sustainability of intensified production. Mitigation was also analyzed in all approaches but few tools go beyond greenhouse gas emissions to analyse environmental sustainability (for example water quality, soil health, ecosystem services) more generically. Climate change adaptation was the CSA pillar with the weakest representation within the approaches reviewed here. Based on an overview of recent advantages in work focusing on CSA our key recommendations are (i) to make better use of recent advances in indicator development for sustainability assessments, including work on quantification of water and land footprints in relation to farm management; (ii) to use household level analyses to quantify pathways from productivity toward food security and improved nutrition as well as descripting drivers of adoption of adaptation options; and (iii) to use recent advances in system specific quantification of greenhouse gas emissions through both LMIC focused modeling and empirical work.
Introduction
World hunger and food insecurity have increased in recent years, after a prolonged decline over the last decades (FAO et al., 2018). Undernourishment is especially rising in sub-Saharan Africa, affecting an estimate of 23% of the population. Partly because of the rapid population growth in sub-Saharan Africa, the total number of undernourished people has increased by more than 22% within 6 years (FAO et al., 2018). Current population trends for Africa predict a further doubling of the population by 2050 and there is therefore a crucial need to produce more food and improve food security and nutrition, especially for small producers. Most rural households produce their own food, but, rather ironically, they are often more affected by food insecurity than their urban counterparts (von Grebmer et al., 2018).
At the same time, climate change is predicted to complicate this aim, with models projecting temperature increases across sub-Saharan Africa, changes in rainfall patterns and increases in extreme events, such as heatwaves, droughts, and extreme rainfall events, although with varying confidence (Niang et al., 2014). These future changes are likely to negatively affect agricultural production and increase the risk for further food insecurity (Ongoma et al., 2017; FAO et al., 2018). Particularly those households that are already food insecure, i.e., rural households and subsistence farming systems, will also be the most vulnerable in the future (Müller et al., 2011; Vermeulen et al., 2012).
Climate Smart Agriculture (CSA) has been proposed as an integrative approach to mitigate ongoing climate change and adapt to its consequences without compromising food security. The Food and Agriculture Organization of the United Nations (FAO) defines climate-smart agriculture as consisting of three pillars:
(1) sustainably increasing agricultural productivity and incomes (food security);
(2) adapting and building resilience to climate change (adaptation); and
(3) reducing and/or removing greenhouse gas emissions (mitigation), where possible.
Ambitious and explicit targets have been set to reach millions of agricultural households with CSA (FAO, 2018). Many interventions have been identified as potentially able to contribute toward these three pillars, such as drought tolerant crops, integrated soil fertility management, water conservation techniques, better integration of livestock in smallholder mixed crop-livestock farms and land restoration in rangelands (Vanlauwe et al., 2010; Campbell et al., 2014; FAO, 2018; Rosenstock et al., 2019). However, questions remain over prioritization of measures in specific contexts, or how to measure progress toward these targets over time, the latter also given the absence of reliable baselines against which one can measure interventions. The lack of “targeting” of interventions—matching the intervention to the context—reduces efficiency and effectiveness of national and international support programs and ultimately decreases the likelihood of meeting essential sustainable development goals (SDGs) by 2030, including: 2 zero hunger, 3 good health and well-being, 12 responsible consumption and production, 13 climate action, and 15 life on land. Targeting interventions and monitoring progress via impact assessments in a way which permits reflexive learning, are, arguably, two of the greatest and least addressed challenges in scaling up CSA. There is an urgent need for tools that produce standardized, coherent, cost-effective and decision-relevant information to support efficient and effective development programming (van Wijk, 2014).
In recent years a series of tools and approaches have been developed to evaluate the “CSA-ness” of specific interventions. Recent reviews however have highlighted the limitations of current work evaluating especially the adaptation and mitigation pillars in the smallholder farming context in LMICs, e.g., Wiederkehr et al. (2018), focusing on dryland systems and Richards et al. (2018). Wiederkehr et al. (2018) highlighted the lack of standard concepts, one of their recommendations was “clear definition of used terminology (e.g., adaptation and coping concepts, migration, agro-pastoralism and pastoralism), especially regarding environmental change and stress,” while the key conclusion by Richards et al. (2018) was that “GHG calculators may poorly estimate GHG emissions in tropical developing countries by comparing calculator predictions against measurements from Africa, Asia, and Latin America.”
In this study, we assess the existing situation of Climate Smart Agriculture assessments tools, focusing on smallholder household level in Low and Middle Income Countries. In this opinion piece we give a concise overview of the most recent developments in measuring key indicators and metrics for the three pillars of CSA and give our opinion on how we think this would allow for improvements in the current state of assessing CSA in a smallholder farming context.
Description of the Framework to Assess CSA Assessments
Our framework to assess the current CSA assessment tools is based on ALL of the three CSA pillars: food security, adaptation, and mitigation (Figure 1). In this section we explain this framework and the choices we made to be able to assess the individual tools in a balanced and comparable manner. We treat the individual pillars separately in the framework, even though we are aware that this can sometimes be rather artificial. For example, the concept of “sustainability” has important consequences and nuances for both the food security and adaptation pillars, while the environmental part of sustainability could further be covered under the mitigation pillar. However, we decided to proceed with the structure of using the separate pillars with the important notion, and this will be repeated throughout the text, that some issues/developments apply to multiple pillars.
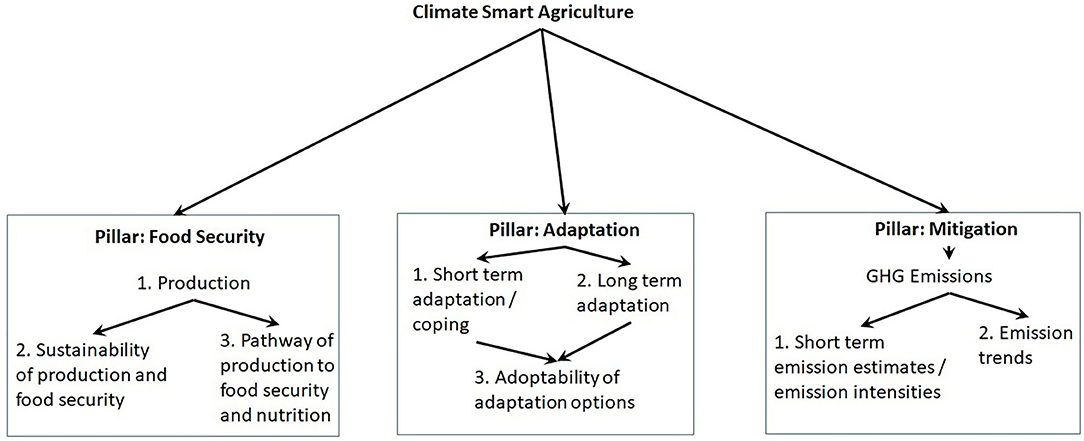
Figure 1. Scheme of the three pillars of CSA and some subcomponents. For explanation of the different aspects see text.
Pillar 1: Food Security
Most often this pillar is translated into quantifying production (even in the definition by FAO). However, focusing on production alone ignores two key aspects of the pillar: (1) it is too simplistic to equate production to food security (and one of its key indicators, nutrition). There are several examples in the literature that show that increased (crop) productivity leads to decreased food security and even malnutrition (for example introducing a more productive crop could lead to a reduction in the diversity of crops being produced) (Fraval et al., 2019). Campbell et al. (2016) also make this point that CSA ought to look beyond production to other dimensions of food security. Key aspect number (2) is the sustainability of food security. The definition of the food security pillar mentions explicitly that improvements in food security and productivity increases the need to be sustainable, without providing guidance on how this should be assessed. Sustainability is defined in many different ways (see e.g., Pretty et al., 2011, identifying more than 100 different ways to define it), but overall common elements of sustainable agriculture (which we focus on in this study) include food production and nutrition for both present and future needs, the capacity to produce desired outputs over longer periods of time, i.e., decades, resilience (ability to absorb or recover from climate shocks and stresses) and environmental friendliness with regard to regional resources, such as biodiversity, water and soil or globally, with regard to GHGs (Schiere et al., 2002; Pretty et al., 2011), and thus clearly links to the other two pillars of CSA. In the past such concepts of agricultural sustainability almost inherently lacked specificity, but there has been a recent surge in work on defining indicators and metrics to make the generic definitions actionable in a given context. Examples of this work are the sustainable intensification (SI) assessment framework, which looks at five distinct dimensions (i.e., productivity, social, human, economics and environment) and other multi-dimensional approaches like the Five Capitals (e.g., Karanja et al., 2016). As a consequence, we will expand upon the simple approach of equaling food security with the production quantity to the biophysical and socio-economic sustainability of production and explore how production translates into food and nutrition security. Sustainability is therefore encompassing many aspects that also come back in the other pillars, again highlighting the difficulty to simply cut up the CSA “cake” into three separate pillars. Key characteristics like “resilience” which are explicitly mentioned in the “adaptation” pillar will of course be kept there, and not dealt with in the “productivity” pillar. Environmental footprints and ecosystem services on the other hand from our perspective do fall under the productivity pillar and not under mitigation. However, it is clear that opinions might diverge on how exactly to differentiate the three pillars.
Pillar 2: Adaptation
A recent review by Wiederkehr et al. (2018) gives an excellent overview of the state of the art in climate change adaptation analyses. One key conclusion of their work is that the concept of adaptation is not well-defined in many studies, with the terms “coping” and “adaptation” being used inter-changeably. To assess whether the frameworks can handle both, we will therefore consider “short term adaptation,” to reflect coping strategies, for example deal with single year weather anomalies or price volatility, and thus also relevant risks. We will also explore “long term adaptation” due to the gradually changing climate, e.g., by changing cropping systems, management or use of different crop varieties. A key recommendation by Wiederkehr et al. (2018) is that the comparability of many results of local case studies needs to be improved upon to be able to draw meaningful and generalizable conclusions regarding climate change adaption and potential adoption of technologies. “Basic socio-economic characteristics of the study population (age mean/range and sex ratio of the interviewees, ethnic background, economic status of households, e.g., farm size or number of livestock, and number of household members) are known to be important factors influencing the coping and adaptation behavior of households” (citation from Wiederkehr et al., 2018) and not only relevant for the adaptation pillar. Thus, it is essential to collect such information across all studies and develop a robust “adoption indicator.” In this part of the evaluation we will assess whether the assessment frameworks differentiate technologies and farms and households in a way which informs upon their capacity to adopt certain coping and adaptation measures (termed here “adoptability”).
Pillar 3: Mitigation
Mitigation is a key pillar of CSA, and this pillar aims at reducing the environmental (climate) footprint of food production as
a) agricultural is the most important driver of environmental degradation worldwide,
b) agricultural is a major source of anthropogenic GHGs especially in LMICs,
c) agriculture can be beneficial for a number of ecosystem services (e.g., biodiversity, carbon sequestration).
Mitigation is often translated into or evaluated through GHG emissions, but in this review, we do want to stress that the boundary might be drawn more broadly to include other environmental indicators, such as nutrient use and leakage, water use and quality and biodiversity. However, following the convention we will discuss these other environmental mitigation indicators under the sustainability aspect of food security, and in this pillar only focus on mitigation of GHG emissions. Note that for example nutrient use efficiency (e.g., a key aspect of mineral fertilizer application) does affect GHG emissions, both in the short and long term. A logical starting point for the quantification of greenhouse gas (GHG) emissions from agricultural sources are emission factors (IPCC Guidelines to National Greenhouse Gas Inventories 2019). Mitigation effects of different management activities can be easily quantified for the key GHGs associated with agricultural production, i.e., CO2, CH4, and N2O and short-lived climate pollutants. In order to compare these to natural emissions and other sectors these are converted into CO2-equivalents. This is normally done for a given year, and represents a specific point in time. At the same time continuous observations will allow to detect changes in emissions and other changes over time (extremes, etc.), for example due to changes in local land use in combination with a changing climate. These observations are important bookkeeping activities in the frame of measuring, reporting and verification (MRV) at national, regional to global scales. A MRV is specifically needed if mitigation efforts will become monetarized, i.e., if pollution is penalized. MRVs are used to track emissions changes and are the basis for distributing climate funds that may become available via donations from, e.g., OECD countries to households that need to cope with climatic stress. The latter is still far away though, but the idea is that mitigation successes can from now on not only be identified but also reliably be quantified. Recent work tries to improve on the generic IPCC emission factors as most were developed on estimates derived for systems in developed countries, which are likely not transferable to low and middle income countries given significant differences in management intensity, climates, soils, or even often animal breeds (e.g., Pelster et al., 2017; Goopy et al., 2018; Ndung'u et al., 2018; Richards et al., 2018; Zhu et al., 2018, 2020). Currently, individual agricultural practices and technologies need to be similarly assessed in terms of meeting simultaneously the demands of pillars 1 and 2. A key factor not discussed here in detail is the aspect of boundary definition. What is taken into account in such farm level assessments? In most cases the farm gate is used as the boundary, thereby neglecting emissions due to transport, processing or food waste. Stating such a clear boundary for the assessment framework is an essential necessity.
The Current State of Climate Smart Agricultural Assessment Frameworks
We reviewed a wide range of CSA assessment frameworks, ranging in methodology (from data collection approaches used for empirical based assessments to theoretical model-based assessments) and integration level (from field based assessment and farm level assessments up to country wide CSA investment targeting assessment tools) to give an overview of the current state of affairs in CSA assessment tools (Table 1). We performed this short review by entering the search terms “climate smart agriculture” and “assessment” in SCOPUS, while we also browsed through key web portals of FAO, CCAFS, and Worldbank for further information regarding tools that are documented in detail, but not available in the scientific literature.
We structured the review by considering the degree to which each approach was able to assess each of the three pillars of CSA and the aspects of each pillar elaborated in Figure 1, as well as the statements given in the relevant sections above. The food security pillar (mostly interpreted as the agricultural production aspect) was quantified in all tools, covering in most cases both crop and livestock production (Table 1). The sustainability of increased production is in most cases not analyzed, and simply crop and livestock production are quantified as production per unit area (or per unit livestock) per season. Whether increased production leads to negative tradeoffs, such as enhanced environmental consequences, is not quantified in most tools, with the water footprint analyses performed within the CoolFarmTool (e.g., Hillier et al., 2012; Dangour et al., 2018) and the soil carbon simulations over time in the SHAMBA tool as clear simulation-based exceptions. The LCA4CSA tool takes a life-cycle assessment perspective of production, which takes on board aspects of sustainability. All tools reviewed here have a biophysical lens while looking at productivity, and largely ignore potential social (e.g., food security, gender) and economic (poverty) aspects of the sustainability of intensified production (Table 1).
Mitigation was the CSA pillar that was also analyzed in all approaches. In fact, one could say that as soon as the productivity and mitigation pillars are quantified, studies often claim their tool is a climate smart agriculture assessment approach. Note again our discussion above, that unless environmental sustainability is explicitly dealt with in the food security pillar, it should be an important aspect of the mitigation analyses. Most approaches use a TIER 2 approach, i.e., which implies the use of country or region-specific emission factors, in their GHG assessment. However, what is considered to be a TIER 2 approach is flexible, and differs between tools. Some consider that relatively small extensions of TIER 1 approach, i.e., use of global emission factors, is adequate to claim a TIER 2 level (e.g., incorporating more animal fodder information in Paul et al., 2017, and similar examples for crop focused analyses). A key aspect of many of the tools is the adherence to the FAO GHG emission factors with limited ability to update these numbers or to include other GHG emission model formulations. While this is logical from a modeling perspective, such consistency in analyses does mean that most assessment frameworks use emission factors that are based on estimates derived for systems in developed countries under different climates with different breeds and overall completely different production systems (e.g., Goopy et al., 2018; Ndung'u et al., 2018; Richards et al., 2018). As a consequence, this can lead to substantial over/under-estimation of GHG emissions and of GHG emission intensities (see example quantifications by Pelster et al., 2016; Goopy et al., 2018; Richards et al., 2018; Zhu et al., 2018). All assessment tools focus on short-term evaluations of GHG emissions, with only soil carbon focused tools being able to assess trends of changes in soil carbon stocks and, thus, CO2 emissions over time. However, the empirical evaluation of these changes in soil carbon stocks is limited, because changes in soil carbon are not monitored generally in agricultural systems in LMICS, and further take time to detect (several years), while changes in vegetation stocks (for example due to af-/de-forestation) are usually not accounted for.
Climate change adaptation was the CSA pillar with the weakest representation within the approaches reviewed here. Often different adaptation options were evaluated in a scenario type of analysis (in the quantitative model type of approaches) and/or in a semi-participatory way through expert panels (e.g., Andrieu et al., 2017; Brandt et al., 2017; Mwongera et al., 2017; Acosta-Alba et al., 2019; Khatri-Chhetri et al., 2019). Besides this scenario evaluation method, there is also a rapidly expanding literature on cross-sectional household survey-based work that analyses the current uptake of CSA adaptation options and the potential uptake of CSA adaptation options under continuing climate and global change (e.g., Wiederkehr et al., 2018). Clearly, few studies interlink these distinct approaches, i.e., by comparing the results of such a model-based scenario analysis with the results of a household survey to assess interest in climate change adaptation options, and the adaptive capacity of households under future scenarios.
Recent Advances in Quantification of the Three Pillars at Farm Livelihood Level That Could Underpin Improvements in the Assessment Frameworks
In this section we describe new work focusing on each of the pillars that produced and used new data or new analyses procedures that can help to further improve the existing CSA assessment frameworks. We refrain from sketching how a single assessment framework should/could look like, but rather opt for describing a range of different approaches and data sources that can underpin improvements of the wide variety of tools described in Table 1. We think maintaining such a diversity is important, with their wide range of aims and objectives, thereby serving a wide range of users.
Pillar 1: Food Security
As shown in Table 1, two aspects are clearly insufficiently addressed in the current CSA assessment tools available: (i) pathways from agricultural production to food security and nutrition and (ii) the sustainability of production increases.
Despite the surge in empirical analyses linking agricultural production (diversity) and dietary diversity (e.g., Sibhatu and Qaim, 2018; Bellon et al., 2020) there is still a distinct lack of tools that can be used to perform (CSA) assessment using all of this information. A new review by Nicholson et al. (under review) analyzed agricultural production models in a repeat of earlier work (e.g., van Wijk et al., 2014; Stephens et al., 2018) and noted the lack of integration of food security and nutritional knowledge into agricultural assessment tools. Typically, rudimentary indicators of food security (for example the proportion of household caloric needs) only focus on household-level outcomes (Nicholson et al., under review). New work tries to fill this gap, and develop conceptual and more quantitative models linking agricultural production to food security and nutritional outcomes. Exciting new approaches make use of so-called pathway models, which can be used in both qualitative and quantitative ways to link agricultural production to food security and nutritional outcomes (e.g., de Jager et al., 2017; Fraval et al., 2019). Pathway models use empirical data, normally based on household surveys, to construct relations between important variables in a predefined pathway model to evaluate how important each of the ways to obtain food is (for example one pathway is to produce food for direct consumption, but farmers also sell agricultural products to buy food, or have off farm income to do the same). By exploring how current pathways to food security look like in contrasting smallholder systems one can start building models of how changes in these systems (for example through production intensification, or through production decreases of certain crops due to climate change) could work out for food security and nutrition.
Most assessments depicted in Table 1 also do not focus on the “sustainability” of the productivity they quantify, neither in their measurements nor in their modeled values. However, it is clear that simply presenting productivity as a crop production per unit area or a livestock production per unit livestock, or at farm level an overall cash income per household, is not sufficient from a sustainability perspective. A recent review by Rasmussen et al. (2018) found that “agricultural intensification is rarely found to lead to simultaneous positive ecosystem services and well-being outcomes. This is particularly the case when ecosystem services other than food provisioning are taken into consideration.” Assessing the multi-dimensional aspects of production (increases) is therefore an essential element that CSA assessment tools should take on-board.
One recent piece of work along this indicator and metric line has been the development of the Sustainable Intensification Assessment Framework (SIAF), which takes an integral perspective across five domains when evaluating productivity increasing interventions. The domains quantified are the human, social, economic and environmental as well the productivity domains.
An example of the results that can be produced with such an integral approach is given in Table 2. In this exercise a household survey was executed in Ethiopia in 2018, interviewing 800 households, and a series of indicators were quantified across the five domains of SIAF and qualitatively evaluated together with. This analysis allows to rapidly identify those indicators that are deemed to be problematic according to local experts as well the indicators that show a lot of variation between households. Both pieces of information are key for intervention planning and adoption of interventions. If an indicator is in a bad state plus shows limited variation between households (for example poverty, months of irrigation), it is likely that interventions focused on locally improving the situation are unlikely to succeed, and probably policy or market access options working at regional scale need to be considered. However, when an indicator scores bad but shows a substantial level of variation (for example market orientation, number of sustainable land practices, crop and/or livestock productivity), this indicates that specific technologies might work for the low scoring households as well that management options and technologies that are used by the higher productive farms can be of interested for the lower scoring farms (e.g., learning from positive deviants as in Steinke et al., 2019).
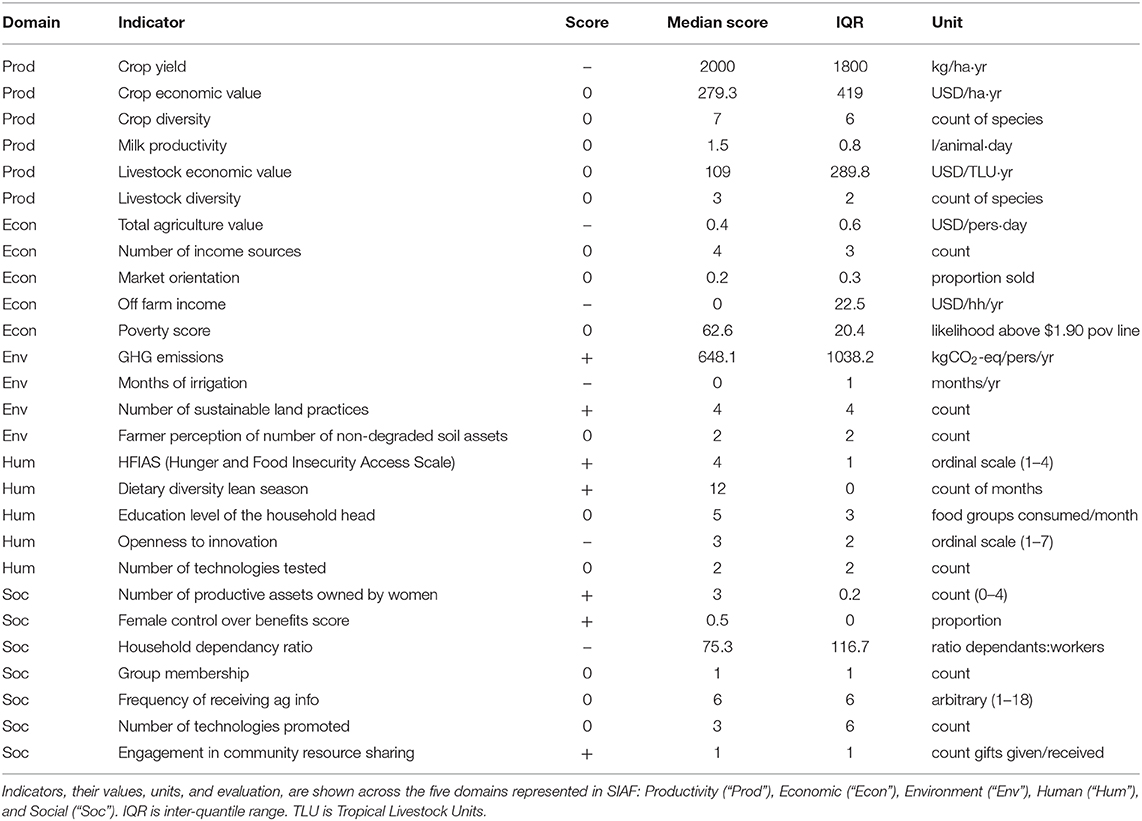
Table 2. Scoring evaluation of the Sustainable Intensification Assessment Framework (SIAF), based on data collected in Ethiopia, 2018, of 800 farm households.
Pillar 2: Adaptation
Recent work showed progress on three areas: (i) consistent, scientific evidence of the benefits of technologies and management options that can be classified as adaptation options; this we will here refer to as basic information at technology level; (ii) analyzing adaptation options at farm household level; and (iii) new work focusing on resilience and its role in farm households. Again, some of the aspects discussed here also apply to the other pillars (for example, information at technology level contains information on the effects of these technologies on production and emissions), but we discuss them here as the entry point is the adaptation angle.
A key new output recently generated is a database of CSA interventions called the CSA compendium (Rosenstock et al., 2019). Based on a systematic literature search of the effects of 102 technologies, including farm management practices (e.g., leguminous intercropped agroforestry, increased protein content of livestock diets, etc.), on 57 indicators consistent with CSA goals (e.g., yield, water use efficiency, carbon sequestration) this effort led to a single database for five countries in SSA, and it is likely continued to grow over the years to come. The studies included in the compendium showed geographic and topical clustering in a few locations, around relatively few measures of CSA and for a limited number of commodities for now (i.e., main crops like maize and beans), indicating potential for skewed results and highlighting gaps in the current evidence available for the effectiveness of CSA. However, it is the first time that such an effort was executed, resulting in a powerful database. This database can function as a starting point to which other experimental data collation efforts like the GARDIAN (led by the CGIAR; https://gardian.bigdata.cgiar.org) can contribute to. Such data is essential to underpin many of the response functions currently integrated in the CSA assessment frameworks and to create more robust and reliable ex-ante impact assessment of CSA measures and the potential pathways toward more CSA-like agriculture in LMICs.
As stressed by Wiederkehr et al. (2018), the comparability of many local case study results needs to be improved upon to be able to draw meaningful and generalizable conclusions regarding climate change adaptation. Standardized data collection tools like the Worldbank's Living Standards Measurement Study—Integrated Survey on Agriculture (LSMS-ISA in short; http://surveys.worldbank.org/lsms/programs/integrated-surveys-agriculture-ISA) and the Rural Household Multiple Indicator Survey (RHoMIS in short; www.rhomis.org; Hammond et al., 2017a) are good examples of these with wide outreach. Recent examples of work where a concrete link was made between the basic socio-economic characteristics of the study population and CC adaptation options adopted are Karanja et al. (2016) and Wichern (2019). In Karanja et al. (2016) the adaptive capacity of households was explained by using the five Capitals approach, and this approach showed a good explanatory power in terms of the number of adaptation options utilized by the farm households. In Wichern (2019) it was shown how the socio-economic status of households was directly related to the adaptation options used, as well as the immediate coping strategies employed when a bad year is foreseen (with livestock owners opting for selling part of their herd, and with the poorest households opting for eating less). In both cases integral farm household level data collection approaches were combined with adaptation adoption analyses, and the harmonized information on basic socio-economic characteristics allowed for such in-depth analysis of drivers of adaptation adoption. In both cases (the perceptions of) climate events played a minor role compared to the socio-economic wealth status of the household.
Another key qualifying factor in terms of explaining the uptake of adaptation options is the motivation of farm households to improve farm performance and their aspirations. The role of personal and societal drivers in adoption dynamics cannot be over-estimated (e.g., Rogers, 2003). Work by Dorward et al. (2009) classified farm households into categories like “hanging in,” “stepping out,” and “stepping up.” Such a grouping can explain to a large extent whether farmers are willing to innovate and adopt (new) interventions (e.g., Fraval et al., 2018). Hammond et al. (2017b) showed that around 50% of the farmers in southern China were not interested in new production intensification options, and simply wanted to continue with their farm in a “business as usual” approach. Such classifications of interested and less interested farmers is essential for the efficient targeting of different adaptation options and for helping development agencies to develop better approaches to roll out innovations. If a group of farm households is less interested in innovation, do we still want to try to reach them, and if so, how can we do that in the most efficient way? An important consideration herein is as well that these less interested farm households are often the poorest households, which are most vulnerable to climate risk and climate change. How can our targeting work reach those households that are likely to be in most urgent need for new technologies, but the least able to adopt new practices?
Despite a surge in research outputs using the term resilience in relation to smallholder farms in the recent literature (a term explicitly mentioned by the FAO definition, in tandem with adaptation), there is still a lack of concrete, generalizable analyses and approaches to tackle this term in relation to climate change and translate these findings in concrete advice for on the ground intervention planning. Theoretical analyses of the concept of resilience and possible poverty traps abound (e.g., Carter and Barrett, 2006; Tittonell and Giller, 2013; Barrett and Constas, 2014; Rigolot et al., 2017), but it is still difficult to base concrete recommendations on these analyses. Conceptually a lot of progress has been made, with much clearer definitions of the concept of resilience as the capacity to buffer shocks and/or the capacity to recover from shocks. These concepts are strongly linked to adaptability and transformability (e.g., Howden et al., 2007; Park et al., 2012) and what one could call the “inverse” of resilience, i.e., “vulnerability” (e.g., Adger, 2006). Either of these capacities/concepts is, rather unsurprisingly, strongly related to wealth status of farm households, the availability of productive resources, active presence of social safety nets and the strength of local governance. However, quantitative, empirically-based knowledge on how for example livelihood diversification strategies or specific crop varieties can help to improve the resilience of smallholders is still largely missing. One recent exemption is work by IFDC in Mozambique, where after the cyclone Idai hit the region in March 2019 they were able to show that farmers that planted improved rice varieties that are highly adaptive and resilient to climate impacts in combination with applying the right fertilizers at the right rate, at the right time, and at the right place (boosting the root development of the rice plants) suffered less from the cyclone than other farmers (Leonardo et al., 2019). There is an urgent need for more of these in-depth studies monitoring as well as evaluating interventions in shock-prone locations, so that we can better assess actual resilience/adaptation dynamics, as opposed to current approaches which often use survey questions which are purely hypothetical (e.g., “what would you do if …”).
Pillar 3: Mitigation
As stated in the section discussing Table 1, most assessment tools (logically) adhere to the FAO GHG emission factors (IPCC, 2019). New work aims on improving these emission factors by specifically considering the situation in LMIC (e.g., Ndung'u et al., 2018; Richards et al., 2018; Zhu et al., 2018). Additionally, the majority of assessments are short term focused, and two lines of research are followed to deal with both limitations of the current assessments: one is empirical work focusing on measuring GHG emissions in the field, and thereby deriving empirically well-grounded GHG emission factors for the most dominant livestock production systems, and the other one is through biogeochemical process-based modeling approaches aiming at simulating biosphere-atmosphere exchange of GHGs while accounting for differences in management, soil properties, vegetation (crops) and climate. The latter are not completely functional yet for sub Saharan African systems though, and normally only focus on arable fields.
New empirical work includes the recent development of localized GHG emissions data from livestock, manure management as well as a variety of land cover types found in smallholder agricultural settings (Pelster et al., 2016, 2017; Goopy et al., 2018; Ndung'u et al., 2018; Wanyama et al., 2018a,b; Zhu et al., 2018, 2020; Wachiye et al., 2020). This essential baseline data was collected following standardized protocols which can thus be applied to similar systems as well as to production systems that have not yet covered (Ndung'u et al., 2018). Similarly, a standardized approach will allow for tracking changes in GHG emissions (i.e., mitigation and to a certain degree also short-term adaptation/resilience to stresses) in the future. It logically contributes to reducing the uncertainty of GHG emissions estimated at local scale (i.e., from individual farms and farm components) as well as at regional scale. Simultaneously, more recent work aims at testing promising interventions to reduce GHG emissions from agricultural activities (Goopy et al., 2020). A necessity for deriving accurate TIER 2 GHG emissions estimates are detailed household level data which then allow for detailed trade-off analysis between this pillar of CSA as well as the productivity pillar.
Similarly, the newly collected in-situ data allows for validation and improvement of the second strand that allows tracking mitigation via measuring, reporting and verification (MRV) protocols, process-based estimation of GHG emission factors by applying detailed theoretical soil and livestock models. Examples of this work are Thornton and Herrero (2010); Carlson K. M. et al. (2017), and MacLeod et al. (2018). Such work allows for regional and system specific quantifications of emission factors as well as regional and continental upscaling of the results. Latest research in this area also allows to tackle the productivity pillar more accurately by not only aiming at food security but also at nutrition security (Hasegawa et al., 2018).
It is clear that an essential next step in further improving and evaluating emission factors in LMIC is to combine the empirical and theoretical approaches to harvest the best from both worlds. Whereas, the empirical work is needed to test the reliability and robustness of the theoretical emissions estimates in contrasting production systems and under different intensification pathways, the theoretical work is needed to be able to upscale this, by its nature always, localized and place-based empirical research and to look at trends over time. Combining theoretical and empirical approaches would also improve evaluation of use of, by definition, simplifying emissions factors. A second important point to make is that mitigation cannot only be GHG mitigation, but also needs to take into account agricultural water and nutrient use explicitly, as well as land use in general (see also Rasmussen et al., 2018). We in this overview cover this under environmental sustainability but want to stress here their key role in mitigation as well.
Final Remarks and Conclusions
To be able to identify realistic recommendations, we have summarized the discussion of the previous section in Figure 2. Per CSA pillar we identify the key steps forward that can be made, and how difficult they are. Or put in another way, what are the low hanging fruits to improve the current CSA assessment tools? With the rapidly increasing availability of open data on crop and livestock production and the excellent work that has been done in the AGMIP project (Agricultural Model Intercomparison and Improvement Project) there will be continuous scope to further improve the quantification of agricultural production. However, at field/farm level there is still a lack of information on land management, crop rotations, intercropping, etceteras, and such information is urgently needed to improve the production and food security estimates. As methods and data for the quantification of environmental footprints and pathways to food security are improving rapidly, also for these aspects there seems to be a lot of potential to further improve their quantification. There is a need to expand the environmental lens of most CSA tools, and the tools of the future need to include a water and land footprint analysis. This is particularly important since both resources are limited and with increasing population growth and with changes in climate the pressure on land and water resources will further increase. For the adaptation pillar a clear need is a more targeted use of repetitive household surveys and other tracking systems to allow for a more comprehensive understanding of the uptake of CSA interventions, with a special focus on monitoring risk. The tools and approaches are there, and the first, major data sources (e.g., the Worldbank LSMS-ISA data) are available. However, such longer term approach also needs to be implemented in agricultural development-oriented projects to further expand the existing data coverage. Across all three pillars more work on combining empirical and theoretical approaches is needed: e.g., integrated analyses of household survey data in combination with production and food security models, as well as field-based monitoring of GHGs in combination with biogeochemical models. This however is a long-term exercise, which needs continuous investment, collaboration and focus of empirical and theoretical researchers. One final remark deals with impacts that are highly stochastic and therefore difficult to quantify in the assessments described in Table 1: animal (livestock) health and mortality, and crop production failure. These impacts can have huge impacts on the livelihoods of smallholder farmers. Advances in quantifying and analyzing the probabilities of these disasters under different climate change scenarios and with applying different adaptation options is urgently needed. Effects of these factors on the, already, highly vulnerable small farmers could be larger than the slow change of crop yields and livestock productivity over time that the assessment tools can quantify quite well.
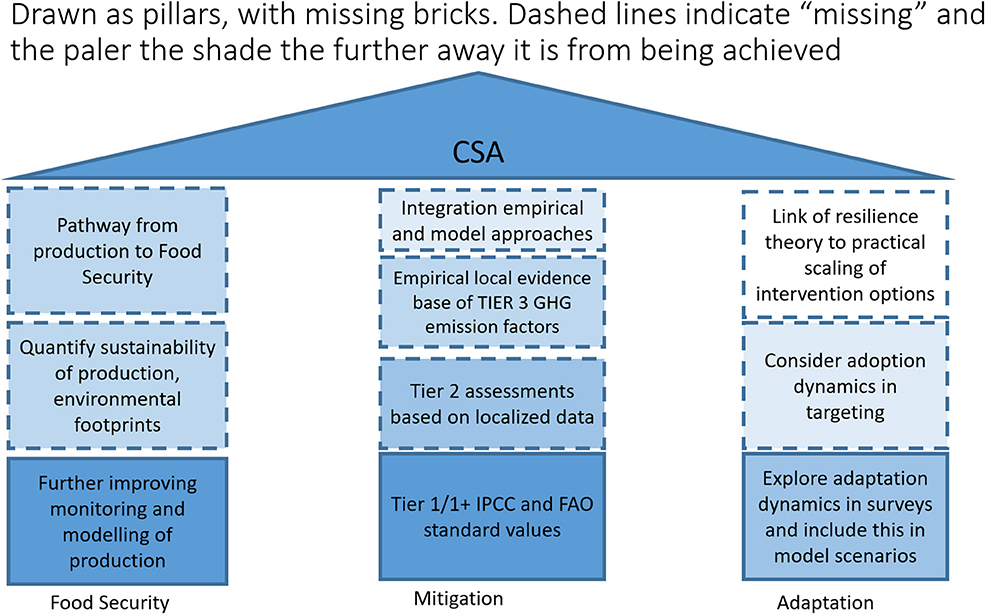
Figure 2. The three pillars of CSA, and key advances identified in this manuscript needed to improve current assessment frameworks. The level of shading represents the ease with which we think that these advances can be achieved. Darker shading indicates that advances can be easier achieved.
Author Contributions
MW wrote the manuscript, with contributions from LM, JH, and KB-B. MW, LM, JH, and KB-B contributed equally to the conceptual development of the study. All authors contributed to the article and approved the submitted version.
Conflict of Interest
The authors declare that the research was conducted in the absence of any commercial or financial relationships that could be construed as a potential conflict of interest.
Acknowledgments
The authors acknowledge the financial support of the CGIAR Fund Council, Australia (ACIAR), Irish Aid, European Union, International Fund for Agricultural Development (IFAD), the Netherlands, New Zealand, UK, USAID, and Thailand for funding to the CGIAR Research Program on Livestock. We acknowledge the support provided by the Programme for Climate-Smart Livestock (PCSL) implemented by GIZ and commissioned by the Federal Ministry for Economic Cooperation and Development (BMZ), Germany.
References
Acosta-Alba, I., Chia, E., and Andrieu, N. (2019). The LCA4CSA framework: using life cycle assessment to strengthen environmental sustainability analysis of climate smart agriculture options at farm and crop system levels. Agric. Syst. 171, 155–170. doi: 10.1016/j.agsy.2019.02.001
Adger, W. N. (2006). Vulnerability. Glob. Environ. Change 16, 268–281. doi: 10.1016/j.gloenvcha.2006.02.006
Andrieu, N., Sogoba, B., Zougmoré, R. B., Howland, F., Samake, O., Bonilla-Findji, O., et al. (2017). Prioritizing investments for climate-smart agriculture: lessons learned from Mali, 2017. Agric. Syst. 154, 13–24. doi: 10.1016/j.agsy.2017.02.008
Antle, J. M., Stoorvogel, J. J., and Valdivia, R. O. (2014). New parsimonious simulation methods and tools to assess future food and environmental security of farm populations. Philos. Trans. R. Soc. Lond. B Biol. Sci. 369:20120280. doi: 10.1098/rstb.2012.0280
Barrett, C. B., and Constas, M. A. (2014). Toward a theory of resilience for international development applications. Proc Nat. Acad. Sci. U.S.A. 111, 14625–14630. doi: 10.1073/pnas.1320880111
Bellon, M. R., Kotu, B. H., Azzarri, C., and Caracciolo, F. (2020). To diversify or not to diversify, that is the question. Pursuing agricultural development for smallholder farmers in marginal areas of Ghana. World Dev. 125:104682. doi: 10.1016/j.worlddev.2019.104682
Brandt, P., Kvakić, M., Butterbach-Bahl, K., and Rufino, M. C. (2017). How to target climate-smart agriculture? Concept and application of the consensus-driven decision support framework “targetCSA”. Agric. Syst. 151, 234–245. doi: 10.1016/j.agsy.2015.12.011
Campbell, B. M., Thornton, P. K., Zougmoré, R., van Asten, P., and Lipper, L. (2014). Sustainable intensification: what is its role in climate smart agriculture? Curr. Opin. Environ. Sustain. 8, 39–43. doi: 10.1016/j.cosust.2014.07.002
Campbell, B. M., Vermeulen, S. J., Aggarwal, P. K., Corner-Dolloff, C., Girvetz, E., Loboguerrero, A. M., et al. (2016). Reducing risks to food security from climate change. Glob. Food Security 11, 34–43. doi: 10.1016/j.gfs.2016.06.002
Carlson, B. R., Carpenter-Boggs, L. A., Higgins, S. S., Nelson, R., Stöckle, C. O., and Weddell, J. (2017). Development of a web application for estimating carbon footprints of organic farms. Comput. Electron. Agric. 142, 211–223. doi: 10.1016/j.compag.2017.09.007
Carlson, K. M., Gerber, J. S., Mueller, N. D., Herrero, M., MacDonald, G. K., Brauman, K. A., et al. (2017). Greenhouse gas emissions intensity of global croplands. Nat. Clim. Chang. 7, 63–68. doi: 10.1038/nclimate3158
Carter, M. R., and Barrett, C. B. (2006). The economics of poverty traps and persistent poverty: an asset-based approach. J. Dev. Stud. 42, 178–199. doi: 10.1080/00220380500405261
Dangour, A., Green, R., Harris, F., Joy, E., Milner, J., Hillier, J., et al. (2018). Environmental impacts of current and future diets in India. Lancet Planet. Health 2:S28. doi: 10.1016/S2542-5196(18)30113-X
de Jager, I., Abizari, A.-R., Douma, J. C., Giller, K. E., and Brouwer, I. D. (2017). Grain legume cultivation and children's dietary diversity in smallholder farming households in rural Ghana and Kenya. Food Secur. 9, 1053–1071. doi: 10.1007/s12571-017-0720-0
Dorward, A., Anderson, S., Bernal, Y. N., Vera, E. S., Rushton, J., Pattison, J., et al. (2009). Hanging in, stepping up and stepping out: livelihood aspirations and strategies of the poor. Dev. Pract. 19, 240–247. doi: 10.1080/09614520802689535
Dunnett, A., Shirsath, P. B., Aggarwal, P. K., Thornton, P., Joshi, P. K., Pal, B. D., et al. (2018). Multi-objective land use allocation modelling for prioritizing climate-smart agricultural interventions. Ecol. Model. 381, 23–35. doi: 10.1016/j.ecolmodel.2018.04.008
FAO (2018). Upscaling Climate Smart Agriculture. Lessons for Extension and Advisory Services. Occasional Papers on Innovation in Family Farming. Rome: Food and Agriculture Organization of the United Nations.
FAO IFAD, UNICEF, WFP, and WHO. (2018). The State of Food Security and Nutrition in the World 2018. Building Climate Resilience for Food Security and Nutrition. Technical report. Rome: FAO.
Fraval, S., Hammond, J., Bogard, J. R., Ng'endo, M., van Etten, J., Herrero, M., et al. (2019). Food access deficiencies in Sub-saharan Africa: prevalence and implications for agricultural interventions. Front. Sustain. Food Syst. 3:104. doi: 10.3389/fsufs.2019.00104
Fraval, S., Hammond, J., Lannerstad, M., Oosting, S. J., Sayula, G., Teufel, N., et al. (2018). Livelihoods and food security in an urban linked, high potential region of Tanzania: changes over a three year period. Agric. Syst. 160:8795. doi: 10.1016/j.agsy.2017.10.013
Goopy, J. P., Korir, D., Pelster, D., Ali, A. I. M., Wassie, S. E., Schlecht, E., et al. (2020). Severe below-maintenance feed intake increases methane yield from enteric fermentation in cattle. Br. J. Nutr. doi: 10.1017/S0007114519003350
Goopy, J. P., Onyango, A. A., Dickhoefer, U., and Butterbach-Bahl, K. (2018). A new approach for improving emission factors for enteric methane emissions of cattle in smallholder systems of East Africa–results for Nyando, Western Kenya. Agric. Syst. 161, 72–80. doi: 10.1016/j.agsy.2017.12.004
Hammond, J., Fraval, S., van Etten, J., Suchini, J. G., Mercado, L., Pagella, T., et al. (2017a). The rural household multi-indicator survey (RHoMIS) for rapid characterisation of households to inform climate smart agriculture interventions: Description and applications in East Africa and Central America. Agric. Syst. 151, 225–233. doi: 10.1016/j.agsy.2016.05.003
Hammond, J., Pagella, T., Smajgl, A., Yi, Z., van Wijk, M., Xu, J., et al. (2017b). Farm types and farmer motivations to adapt: implications for design of sustainable agricultural interventions using the example of rubber plantations in South West China. Agric. Syst. 154, 1–12. doi: 10.1016/j.agsy.2017.02.009
Hasegawa, T., Fujimori, S., Havlík, P., Valin, H., Bodirsky, B. L., Doelman, J. C., et al. (2018). Risk of increased food insecurity under stringent global climate change mitigation policy. Nat. Clim. Chang. 8:699. doi: 10.1038/s41558-018-0230-x
Hillier, J., Brentrup, F., Wattenbach, M., Walter, C., Garcia-Suarez, T., Mila-i-Canals, L., et al. (2012). Which cropland greenhouse gas mitigation options give the greatest benefits in different world regions? Climate and soil-specific predictions from integrated empirical models. Glob. Change Biol. 18, 1880–1894. doi: 10.1111/j.1365-2486.2012.02671.x
Hillier, J., Walter, C., Malin, D., Garcia-Suarez, T., Mila-i-Canals, L., and Smith, P. (2011). A farm-focused calculator for emissions from crop and livestock production. Environ. Model. Softw. 26, 1070–1078. doi: 10.1016/j.envsoft.2011.03.014
Howden, S. M., Soussana, J. F., Tubiello, F. N., Chhetri, N., Dunlop, M., and Meinke, H. (2007). Adapting agriculture to climate change. Proc. Natl. Acad. Sci. U.S.A. 104, 19691–19696. doi: 10.1073/pnas.0701890104
IPCC (2019). Climate Change and Land: an IPCC special report on climate change, desertification, land degradation, sustainable land management, food security, and greenhouse gas fluxes in terrestrial ecosystems. in Press.
Karanja, S. N., van Wijk, M. T., Rufino, M. C., and Giller, K. E. (2016). Adaptation of agriculture to climate change in semi-arid Borena, Ethiopia. Reg. Environ. Change 16, 2317–2330. doi: 10.1007/s10113-016-0940-4
Khatri-Chhetri, A., Pant, A., Aggarwal, P. K., Vasireddy, V. V., and Yadav, A. (2019). Stakeholders prioritization of climate-smart agriculture interventions: evaluation of a framework. Agric. Syst. 174, 23–31. doi: 10.1016/j.agsy.2019.03.002
Leonardo, W., Cululo, H., and Farijao, H. (2019). Climate-Smart Practices to Build Hope and Resilience After Cyclone Idai. Maputo: IFDC.
MacLeod, M. J., Vellinga, T., Opio, C., Falcucci, A., Tempio, G., Henderson, B., et al. (2018). Invited review: a position on the global livestock environmental assessment model (GLEAM). Animal 12, 383–397. doi: 10.1017/S1751731117001847
Müller, C., Cramer, W., Hare, W. L., and Lotze-Campen, H. (2011). Climate change risks for African agriculture. Proc. Natl. Acad. Sci. U.S.A. 108, 4313–4315. doi: 10.1073/pnas.1015078108
Mwongera, C., Shikuku, K. M., Twyman, J., Läderach, P., Ampaire, E., van Asten, P., et al. (2017). Climate smart agriculture rapid appraisal (CSA-RA): a tool for prioritizing context-specific climate smart agriculture technologies. Agric. Syst. 151, 192–203. doi: 10.1016/j.agsy.2016.05.009
Ndung'u, P., Bebe, B. O., Ondit, J., Butterbach-Bahl, K., Merbold, L., and Goopy, J. P. (2018). Improved region-specific emission factors for enteric methane emissions from cattle in smallholder mixed crop-livestock systems of Nandi county, Kenya. Anim. Prod. Sci. doi: 10.1071/AN17809
Niang, I., Ruppel, O. C., Abdrabo, M. A., Essel, A., Lennard, C., Padgham, J., et al. (2014). “AR5 climate change 2014: impacts, adaptation, and vulnerability,” in Part B: Regional Aspects. Contribution of Working Group II to the Fifth Assessment Report of the Intergovernmental Panel on Climate Change, eds V. R. Barros, C. B. Field, D. J. Dokken, M. D. Mastrandrea, K. J. Mach, T. E. Bilir, M. Chatterjee, K. L. Ebi, Y. O. Estrada, R. C. Genova, B. Girma, E. S. Kissel, A. N. Levy, S. MacCracken, P. R. Mastrandrea, and L. L. White (Cambridge: Cambridge University Press), 1199–1265.
Ongoma, V., Chen, H., and Gao, C. (2017). Projected changes in mean rainfall and temperature over East Africa based on CMIP5 models. Int. J. Climatol. 38, 1375–1392. doi: 10.1002/joc.5252
Park, S. E., Marshall, N. A., Jakku, E., Dowd, A. M., Howden, S. M., Mendham, E., et al. (2012). Informing adaptation responses to climate change through theories of transformation. Glob. Environ. Change 22, 115–126. doi: 10.1016/j.gloenvcha.2011.10.003
Paul, B. K., Frelat, R., Birnholz, C., Ebong, C., Gahigi, A., Groot, J. C. J., et al. (2017). Agricultural intensification scenarios, household food availability and greenhouse gas emissions in Rwanda: ex-ante impacts and trade-offs. Agric. Syst. 163, 16–26. doi: 10.1016/j.agsy.2017.02.007
Pelster, D. E., Gisore, B., Goopy, J., Korir, D., Koske, J. K., Rufino, M. C., et al. (2016). Methane and nitrous oxide emissions from cattle excreta on an East African grassland. J. Environ. Qual. 45, 1531–1539. doi: 10.2134/jeq2016.02.0050
Pelster, D. E., Rufino, M. C., Rosenstock, T., Mango, J., Saiz, G., Diaz-Pines, E., et al. (2017). Smallholder farms in eastern African tropical highlands have low soil greenhouse gas fluxes. Biogeosciences 14, 187–202.
Pretty, J., Toulmin, C., and Williams, S. (2011). Sustainable intensification in African agriculture. Int. J. Agric. Sustain. 9, 5–24. doi: 10.3763/ijas.2010.0583
Rasmussen, L. V., Coolsaet, B., Martin, A., Mertz, O., Pascual, U., Corbera, E., et al. (2018). Social-ecological outcomes of agricultural intensification. Nat. Sustain. 1, 275–282. doi: 10.1038/s41893-018-0070-8
Richards, M., Metzel, R., Chirinda, N., Ly, P., Nyamadzawo, G., Vu, O. D., et al. (2018). Limits of agricultural greenhouse gas calculators to predict soil N2O and CH4 fluxes in tropical agriculture. Sci. Rep. 6:26279. doi: 10.1038/srep26279
Rigolot, C., de Voil, P., Douxchamps, S., Prestwidge, D., Van Wijk, M., Thornton, P. K., et al. (2017). Interactions between intervention packages, climatic risk, climate change and food security in mixed crop–livestock systems in Burkina Faso. Agric. Syst. 151, 217–224. doi: 10.1016/j.agsy.2015.12.017
Rosenstock, T. S., Lamanna, C., Namoi, N., Arslan, A., and Richards, M. (2019). “What is the evidence base for climate-smart agriculture in East and Southern Africa? A systematic map,” in The Climate-Smart Agriculture Papers, eds T. Rosenstock, A. Nowak, E. Girvetz (Cham: Springer), 141–151. doi: 10.1007/978-3-319-92798-5_12
Rowshon, M. K., Dlamini, N. S., Mojid, M. A., Adib, M. N. M., Amin, M. S. M., and Lai, S. H. (2019). Modeling climate-smart decision support system (CSDSS) for analyzing water demand of a large-scale rice irrigation scheme. Agric. Water Manage. 216, 138–152. doi: 10.1016/j.agwat.2019.01.002
Sain, G., Loboguerrero, A. M., CornerDolloff, C., Lizarazo, M., Nowak, A., Martínez-Barón, D., et al. (2017). Costs and benefits of climate-smart agriculture: the case of the dry corridor in Guatemala. Agric. Syst. 151, 163–173. doi: 10.1016/j.agsy.2016.05.004
Sapkota, T. B., Jat, M. L., Aryal, J. P., Jat, R. K., and Khatri-Chhetri, A. (2015). Climate change adaptation, greenhouse gas mitigation and economic profitability of conservation agriculture: some examples from cereal systems of Indo-gangetic plains. J. Integr. Agric. 14, 1524–1533. doi: 10.1016/S2095-3119(15)61093-0
Schiere, J. B., Ibrahim, M. N. M., and van Keulen, H. (2002). The role of livestock for sustainability in mixed farming: criteria and scenario studies under varying resource allocation. Agric. Ecosyst. Environ. 90, 139–153. doi: 10.1016/S0167-8809(01)00176-1
Shikuku, K. M., Valdivia, R. O., Paul, B. K., Mwongera, C., Winowiecki, L., Läderach, P., et al. (2017). Prioritizing climate-smart livestock technologies in rural Tanzania: A minimum data approach. Agricultural Systems 151, 204–216. doi: 10.1016/j.agsy.2016.06.004
Sibhatu, K. T., and Qaim, M. (2018). Review: Meta-analysis of the association between production diversity, diets, and nutrition in smallholder farm households. Food Policy. 77, 1–18. doi: 10.1016/j.foodpol.2018.04.013
Steinke, J., Mgimiloko, G., Graef, F., Hammond, J., van Wijk, M. T., and van Etten, J. (2019). Prioritising household-specific options for agricultural development through the positive deviance approach. PLoS ONE 14:e0212926. doi: 10.1371/journal.pone.0212926
Stephens, E., Jones, A. D., and Parsons, D. (2018). Agricultural systems research and global food security in the 21st century: an overview and roadmap for future opportunities. Agric. Syst. 163, 1–6. doi: 10.1016/j.agsy.2017.01.011
Thornton, P. K., and Herrero, M. (2010). Potential for reduced methane and carbon dioxide emissions from livestock and pasture management in the tropics. Proc. Nat. Acad. Sci. U.S.A. 107, 19667–19672. doi: 10.1073/pnas.0912890107
Tittonell, P., and Giller, K. E. (2013). When yield gaps are poverty traps: the paradigm of ecological intensification in African smallholder agriculture. Field Crops Res. 143, 76–90. doi: 10.1016/j.fcr.2012.10.007
van Wijk, M. T. (2014). From global economic modelling to household level analyses of food security and sustainability: how big is the gap and can we bridge it? Food Policy 49, 378–388. doi: 10.1016/j.foodpol.2014.10.003
van Wijk, M. T., Rufino, M. C., Enahoro, D., Parsons, D., Silvestri, S., Valdivia, R. O., et al. (2014). Farm household modelling and its role in designing climate-resilient agricultural systems. Glob. Food Secur. 3, 77–84. doi: 10.1016/j.gfs.2014.05.001
Vanlauwe, B., Chianu, J., Giller, K. E., Merck, R., Mokwenye, U., Pypers, P., et al. (2010). Integrated soil fertility management: operational definition and consequences for implementation and dissemination. Outlook Agric. 39, 17–24. doi: 10.5367/000000010791169998
Vermeulen, S. J., Campbell, B. M., and Ingram, J. S. I. (2012). Climate change and food systems. Annu. Rev. Environ. Resour. 37, 195–222. doi: 10.1146/annurev-environ-020411-130608
von Grebmer, K., Bernstein, J., Patterson, F., Sonntag, A., Klaus, L. M., Fahlbusch, J., et al. (2018). Global Hunger Index. Forced Migration and Hunger. Dublin; Bonn: International Food Policy Research Institute, Welthungerhilfe, Concern Worldwide.
Wachiye, S., Merbold, L., Vesala, T., Rinne, J., Räsänen, M., Leitner, S., et al. (2020). Soil greenhouse gas emissions under different land-use types in savanna ecosystems of Kenya. Biogeosciences 17, 2149–67. doi: 10.5194/bg-2019-407
Wanyama, I., Pelster, D. E., Arias-Navarro, C., Butterbach-Bahl, K., Verchot, L. V., and Rufino, M. C. (2018a). Management intensity controls soil N2O fluxes in an Afromontane ecosystem. Sci. Total Environ. 624, 769–780. doi: 10.1016/j.scitotenv.2017.12.081
Wanyama, I., Rufino, M. C., Pelster, D. E., Wanyama, G. N., Atzberge, C., van Asten, P., et al. (2018b). Land Use, Land Use History, and Soil Type Affect Soil Greenhouse Gas Fluxes From Agricultural Landscapes of the East African Highlands. J. Geophys. Res-Biogeo. 123, 976–990. doi: 10.1002/2017JG003856
Wassmann, R., Pasco, R., Zerrudo, J., Ngo, D. M., Vo, T. B. T., and Sander, B. O. (2019). Introducing a new tool for greenhouse gas calculation tailored for cropland: rationale, operational framework and potential application. Carbon Manage. 10, 79–92. doi: 10.1080/17583004.2018.1553436
Wichern, J. (2019). Food security in a changing world. Disentangling the diversity of rural livelihood strategies across Uganda (Ph.D. thesis), Wageningen University, Wageningen, Netherlands.
Wiederkehr, C., Beckmann, M., and Hermans, K. (2018). Environmental change, adaptation strategies and the relevance of migration in Sub-Saharan drylands. Environ. Res. Lett. 13:113003. doi: 10.1088/1748-9326/aae6de
Woollen, E., Berry, N., Cross, A., Hagdorn, M., Hughes, M., Wells, G. J., et al. (2017). The Small-Holder Agriculture Mitigation Benefit Assessment Model for Estimation of Greenhouse Gas Emission Reductions And Removals That Result From Smallholder Farmers Using Climate Smart Agriculture and/or Tree Planting in the Tropics. SHAMBA v 1.1–Methodology. Edinburgh: The University of Edinburgh. Available online at: https://shambatool.files.wordpress.com/2017/03/shamba-model-description1.pdf.
Zhu, Y., Merbold, L., Leitner, S., Xia, L., Pelster, D. E., Diaz-Pines, E., et al. (2020). Influence of soil properties on N2O and CO2 emissions from excreta deposited on tropical pastures in Kenya. Soil Biol. Biochem. 140:107636. doi: 10.1016/j.soilbio.2019.107636
Keywords: climate smart agriculture (CSA), assessment, food security, adaptation, mitigation
Citation: van Wijk MT, Merbold L, Hammond J and Butterbach-Bahl K (2020) Improving Assessments of the Three Pillars of Climate Smart Agriculture: Current Achievements and Ideas for the Future. Front. Sustain. Food Syst. 4:558483. doi: 10.3389/fsufs.2020.558483
Received: 02 May 2020; Accepted: 14 August 2020;
Published: 22 September 2020.
Edited by:
Ngonidzashe Chirinda, Mohammed VI Polytechnic University, MoroccoReviewed by:
Leigh Ann Winowiecki, World Agroforestry Centre, KenyaPytrik Reidsma, Wageningen University and Research, Netherlands
Copyright © 2020 van Wijk, Merbold, Hammond and Butterbach-Bahl. This is an open-access article distributed under the terms of the Creative Commons Attribution License (CC BY). The use, distribution or reproduction in other forums is permitted, provided the original author(s) and the copyright owner(s) are credited and that the original publication in this journal is cited, in accordance with accepted academic practice. No use, distribution or reproduction is permitted which does not comply with these terms.
*Correspondence: Mark T. van Wijk, bS52YW53aWprQGNnaWFyLm9yZw==