- Faculty of Veterinary and Agricultural Sciences, School of Agriculture and Food, The University of Melbourne, Melbourne, VIC, Australia
With water resources constantly becoming scarcer, and 70% of freshwater used for the agriculture sector, there is a growing need for innovative methods to increase water use efficiency (WUE) of food production systems and provide nutrient-dense food to an increasing population. Sensor technology has recently been introduced to the horticulture industry to increase resource use efficiency and minimize the environmental impacts of excessive water use. Identifying the effects of irrigation levels on crop performance is crucial for the success of sensor-based water management. This research aimed to optimize WUE in a soilless microgreen production system through identification of an optimal irrigation level using a sensor that could facilitate the development of a more efficient, low-cost automated irrigation system. A dielectric moisture sensor was implemented to monitor water levels at five irrigation setpoints: 7.5, 17.5, 25, 30, and 35 percent of the effective volume of the container (EVC) during a 14-day growth cycle. To validate the sensor performance, the same irrigation levels were applied to a parallel trial, without sensor, and water levels were monitored gravimetrically. Plant water status and stress reaction were evaluated using infrared thermal imaging, and the accumulation of osmolytes (proline) was determined. Results showed that, proline concentration, canopy temperature (Tc), canopy temperature depression (CTD), and crop water stress index (CWSI) increased at 7.5% EVC in both sensor-based and gravimetric treatments, and infrared index (Ig) and fresh yield decreased. The dielectric moisture sensor was effective in increasing WUE. The irrigation level of 17.5% EVC was found to be optimal. It resulted in a WUE of 88 g/L, an improvement of 30% over the gravimetric method at the same irrigation level. Furthermore, fresh yield increased by 11.5%. The outcome of this study could contribute to the automation of precision irrigation in hydroponically grown microgreens.
Introduction
Irrigated agriculture is the greatest water user in many countries. Due to the increasing threat of water scarcity caused by climate change, efficient irrigation management is of critical importance for sustainable food production. According to a report published by The Commonwealth Scientific and Industrial Research Organization (CSIRO, 2018), it has been predicted that, except for a few areas in the north of the country, Australia will experience decreases in rainfall and longer drought periods. Therefore, it is essential to implement research-driven sustainable agricultural practices such as optimized irrigation management to minimize water waste and promote water use efficiency.
In recent years, the adoption of modern high-tech growing strategies such as indoor soilless cultivation has contributed to improving water use efficiency (WUE) (Pignata et al., 2017). Soilless agriculture refers to methods of growing plants in a medium where nutrients are supplied by irrigation water or a nutrient solution (NS) without using soil (Olympios, 1999). At present, soilless cultivation systems contribute to a significant portion of indoor vegetable production in Europe, Canada, and the United States (O'Sullivan et al., 2019). It has been estimated that 1 kg of lettuce produced in an indoor soilless farm only needs 3% of the water that field-grown lettuce may require (O'Sullivan et al., 2019). Soilless cultivation is already well-established for some vegetables such as tomato and lettuce. Still, there is little established literature regarding optimisation of growing practices and water management in soilless cultivation systems for growing some herbs and specialty crops including the emerging class of herbs known as microgreens. The term “microgreens” refers to the young seedlings consisting of edible cotyledonary leaves attached to a tender hypocotyl, which can contain equal or even higher amounts of some nutrients than the mature plant (Xiao et al., 2012, 2016). Microgreens are prime candidates for being grown hydroponically in closed environments such as urban vertical farms (Di Gioia and Santamaria, 2015). The minimal space requirement and compatibility with indoor farms make microgreens a perfect crop of choice to be grown in indoor soilless systems such as vertical farms and plant factories (Kyriacou et al., 2016). Kale (B. oleracea var acephala) is considered a nutrient-rich leafy vegetable (Migliozzi et al., 2015), which is being widely used, either raw or cooked, in a variety of dishes. As compared to other brassica vegetables, kale ranks highest for its protein content (3.3% fresh weight basis) (Manchali et al., 2012) and containing high amounts of calcium, folate, riboflavin, vitamin C and vitamin K (Šamec et al., 2019). Due to its nutrient profile and with the recent trend toward healthy eating, kale has gained increasing popularity among both vegetarian and non-vegetarian consumers. Kale is rich in minerals at all growth stages, including as a microgreen, baby green, or fully grown vegetable (Waterland et al., 2017).
Water management impacts performance, yield, and quality of vegetables; both under- and over-irrigation may negatively affect the crop (Pignata et al., 2017). The implementation of proximal sensors could facilitate the prediction of certain critical points when plants need to receive water. Measures of water status are required for crop water management purposes where a repeatable control is needed. These measures are mainly based on water content in either substrate or the plant itself, upon which irrigation setpoints can be defined on a crop-specific basis where plants are able to extract water effectively at that level without showing drought stress symptoms. Commonly used criteria for defining an irrigation threshold include measuring volumetric water content in the substrate which can be derived through various approaches including gravimetric measurements of water mass as well as methods in which the dielectric constant of water is measured and related to the moisture content (Jones, 2007). Through the use of sensors that work in conjunction with computer-controlled systems, a degree of automation within greenhouses can be achieved. This would significantly decrease labor costs, the latter of which represents the bulk of the costs involved in greenhouse operations such as irrigation (Jadhav and Rosentrater, 2017). In doing so, growers can stay competitive while achieving better plant growth through optimized growing conditions (Ferrarezi et al., 2015). For instance, sensors can provide information to support decision-making and automate irrigation system by switching the latter on and off whenever the moisture content in the soil/substrate drops below a user-defined threshold. In a more complex way, crop water use models could be developed based on the environmental data collected by the sensors to predict when irrigation is needed and at what quantities (Lea-Cox, 2012). Considering that microgreens are often grown in small shallow containers, conventional sensors designed for soil-based systems, like tensiometers and time domain reflectometry (TDR) probes, are impractical because they require a large volume of soil, media, or substrate to operate within. Hence, there is a need to explore and identify moisture sensors specifically suitable for the soilless cultivation of microgreens. This experiment evaluated the effectiveness of a low-cost dielectric moisture sensor to reduce the impact on resource usage and to optimize WUE as well as microgreens fresh yield in a soilless system through the identification of an optimized irrigation setpoint for growing microgreens. This is the first study implementing infrared thermal imagery techniques to assess the physiological responses of microgreens to water availability.
Materials and Methods
Experimental Site and Plant Materials
Experiments were conducted in an environmentally controlled vertical farming growth room at Dookie campus, The Faculty of Veterinary and Agricultural Sciences, The University of Melbourne. Ambient temperature (Ta) and relative humidity (RH) were maintained at 22.5 °C and 65–70%. Toscano black kale (Brassica oleracea var. acephala) seeds were first sterilized by soaking for 2 min in 80% ethanol, rinsed twice with distilled water, and then oven-dried at 45°C for 40 min. Eighteen grams of sterilized seeds were evenly sown on damp PureGrown Hemp-felt mats (5 mm thick) and placed into perforated plastic trays with dimensions of 34 × 28 × 5 cm. Each perforated tray was put into a solid bottom container containing distilled water to create a simple wicking-type hydroponic system. Growing containers were kept in the dark until the emergence of the radicle (3 days) and then exposed to LED lighting (Valoya, Finland) with an 18/6 h photoperiod. The spectral output of the lighting system was quantified using a Lighting Passport spectrometer (AsenseTek, Taipei, Taiwan) (Table 1).
Sensor-Based Irrigation System Description and Treatments
As shown in Figure 2, water level was measured in the hydroponic system using a high-frequency series moisture sensor probe (VH400, Vegetronix, Sandy, UT). The sensor measures the dielectric constant in the nutrient solution surrounding the roots of kale microgreens using the transmission line technique. The effective volume of the container (EVC) was defined as a portion of the container allocated for root growth, which is the area below the surface of the growing mat and the bottom of the solid tray (~2 L in all replicates).
The sensor used in this experiment is insensitive to water salinity caused by the presence of the nutrient salts, an important quality for hydroponic systems. The sensor was connected to a data logger via a 2-meter cable. The data logger consisted of an Arduino micro-controller with a data logging shield and a real-time clock. The system converted voltage readings into the percentage of EVC using the onboard analog to digital converter and a preliminary calibration obtained for these experiments.
The sensor was calibrated by suspending it over a beaker of deionised water using a standard laboratory titration clamp. The sensor was then lowered vertically into the water in increments of 5 mm (0–80 mm). The response voltage was recorded after each increment for 10 s generating ten readings per depth level. The procedure was repeated six times, and the calibration curve for water depth (mm) vs. voltage (V) was derived based on a total of 1,020 observations (R2 = 0.996) with a curvilinear second-order fit (Figure 1). The associated percentage of EVC was then calculated, and the corresponding sensor outputs were recorded accordingly. Treatments were defined as a percentage of EVC at five levels, as summarized in Table 2. Each growing tray represented one replicate, and there were three replicates per treatment. Figure 2 shows how the moisture sensor was positioned in a tray of kale microgreens.
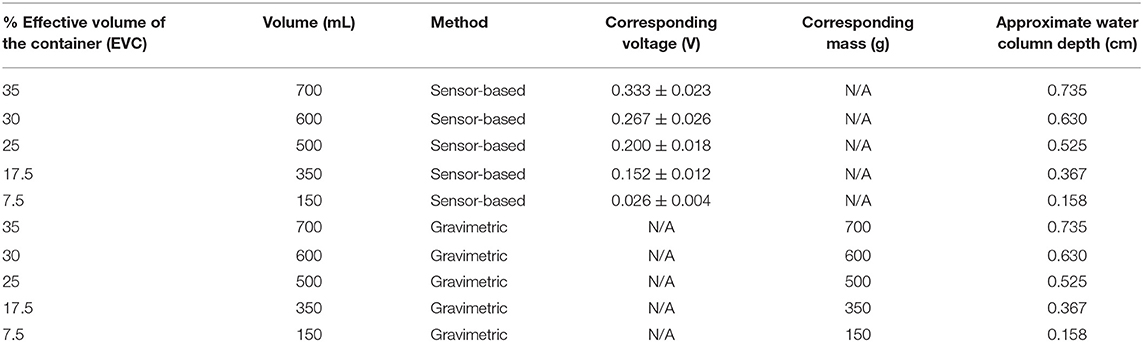
Table 2. Description of irrigation treatments in two irrigation methods and their corresponding voltage or mass.
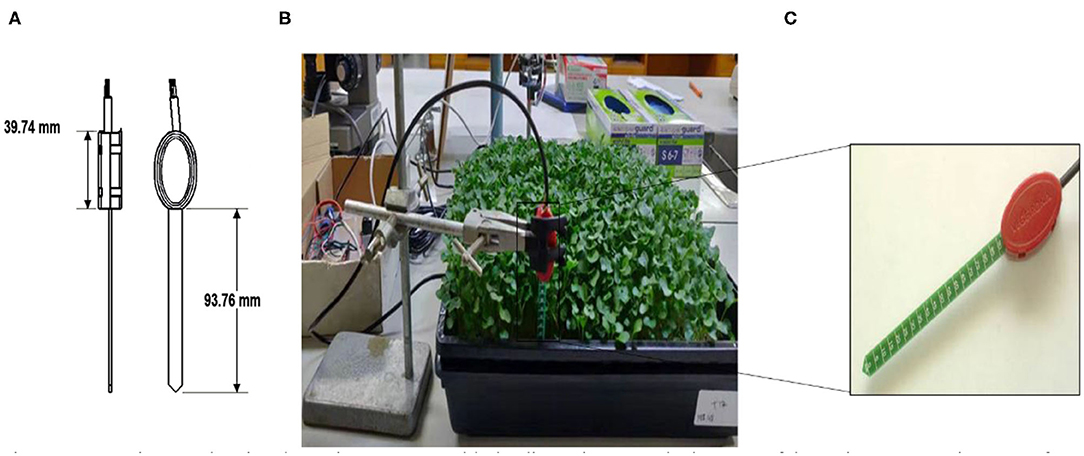
Figure 2. (A) Diagram showing the moisture sensor with the dimensions, (B) deployment of the moisture sensor in a tray of kale microgreens, and (C) individual moisture sensor. [(C) Adapted from: http://greenstemnetwork.org/playing-in-dirt-soil-moisture-sensors/].
In this experiment, depth of the growing container was 5 cm. The bottom reservoir was kept empty and the growing mats were saturated with water until 4 days after sowing. Irrigation treatments were applied on day four after sowing. Seeds were uniformly germinated in constant environmental conditions, with bottom reservoir trays kept empty. By doing so, roots were allowed to develop uniformly without any treatments affecting root growth prior to day 4, upon which treatments were added. Also, on day five, all treatments observed a decrease in water level in the reservoir trays based on gravimetric and sensor-based measurements. This would indicate that root growth was sufficient to facilitate uptake of water from the reservoir of water.
The accuracy of sensors was validated using an identical series of treatments, where the water content was determined gravimetrically, to act as a control group. In this case, the same volumes of nutrient solution were applied to the growing containers. Then the mass of each container was recorded as a setpoint for its corresponding water application. Both sensor-based and gravimetrically controlled trays were monitored every 24 h for their water content to maintain the water at the initially defined levels.
Root: Shoot (RS) Ratio, Biomass, and Water Use Efficiency (WUE) Calculations
Five individual seedlings were randomly harvested, using a sterile blade, from various spots in the middle of the trays to avoid any edge effects. The shoot and root length of each seedling was measured using a digital vernier caliper (150-mm Digital Vernier Calipers, Craftright Pty Ltd). Root: shoot ratio (RS) ratio was calculated by dividing the root length by the shoot length. All microgreens from each replicate were harvested to obtain total harvested biomass (TFW) (g). From the harvested biomass, 10 g of microgreens from each replicate were oven-dried at 70°C for 72 h to obtain dry matter (DM) (g. 100 g−1 FW). Fresh yield and water use efficiency (WUE) were calculated using the following equations:
Where TFW is the total harvested biomass from each growing container (g) and A is the area of the growing mat (m2).
Where ∑W is the total water added to each growing container (L).
Determination of Proline Content
Proline content was determined following the methodology proposed by Bates et al. (1973). Finely ground kale microgreens (0.1 g) were homogenized in 5 mL of 3% sulfosalicylic acid (C7H6O6S) using a mortar and a pestle and then filtered through Whatman No. 2 filter paper (GE Healthcare, New South Wales, Australia). Two mL of filtrate was then reacted with 2 mL of acid-ninhydrin (C9H6O4), and 2 mL of glacial acetic acid (C2H4O2) in a 20 mL glass boiling tube for 1 h at 100°C. The reaction was then terminated in an ice bath. The reaction mixture was extracted with 4 mL of toluene (C6H5-CH3) and mixed vigorously using a vortex mixer for 15–30 s. The resulting upper fraction of toluene was then transferred to glass cuvettes, and absorbance was read at 520 nm using a single beam scanning UV/visible spectrophotometer (M501, Campspec Ltd, Cambridge, UK). A proline standard curve was prepared by dissolving proline in 3% sulfosalicylic acid (C7H6O6S) to reflect proline concentration (μg. mL−1). Proline concentration in the toluene extract was determined from the standard curve and expressed on a fresh weight basis using the following equation.
Where P is the concentration of proline (μg. mL−1) in the reaction mixture, and w is the mass of the microgreen sample (g).
Estimation of Photosynthetic Pigments
Determination of chlorophyll a, b, total chlorophylls, and total carotenoids were conducted using the methods described by Arnon (1967) with a few modifications. From each replicate, a 1 g microgreen sample was homogenized with mortar and pestle in 10 mL of 80% acetone and transferred to a 10 mL centrifuge tube. Samples were then centrifuged at 3,000 rpm for 10 min at 4°C. A fraction of the supernatant was transferred to the glass cuvettes for reading the absorbance value using a single beam UV-VIS spectrophotometer at 470 (A470), 645 (A645), and 663 nm (A663), along with 80% acetone as a blank. Pigment concentrations for chlorophyll a (Chl a), b (Chl b), total chlorophyll (Chl T), and total carotenoid (Car) content were calculated using the following equations and expressed in μg of chlorophyll per g of fresh tissue (μg. g −1 FW).
Where V is the volume of supernatant (mL), W is the mass of the microgreen sample (g), A663, A645, and A470 are the absorbance at wavelengths of 663, 645, and 470 nm, respectively.
Plant Water Status Based on Infrared Thermal Imagery
Microgreens were assessed three times during the growth period, on days 8, 11, and 13 after sowing, using a thermal infrared camera (FLIR One, FLIR Systems, Portland USA), with a resolution of 90 × 60 pixels. The thermal sensitivity of the camera is −20 to 120°C. The infrared thermal sensor of the camera measures the reflective radiation of surfaces in the range of 8–14 μm and transforms it into temperature readings per pixel. Each pixel represents a temperature reading in degrees Celsius (°C). One thermal image from each replicate was taken from a constant distance of 1.5 m at a 0° Nadir angle. The infrared thermal images were processed and analyzed using a customized code written in MATLAB software and the Image Analysis Toolbox (MathWorks Inc., Natick, MA, USA) to estimate canopy temperature (Tc).
Each thermal image was cropped only to include the region of interest (ROI). The unwanted pixels, including the tray, substrate, and background pixels, were omitted by setting the minimum and maximum temperatures within the plant area. Data was automatically extracted from a pre-defined subdivision of 3 × 3 = 9 segments (Figure 3). Average plant pixel temperatures were considered to calculate the Tc (Fuentes et al., 2012) and the crop water stress index (CWSI) (Jones et al., 2002; Fuentes et al., 2012).
Where Tc represents the canopy temperature (°C), Twet is the corresponding temperature of a fully transpiring area (°C) (a wet reference) and the Tdry is the corresponding temperature of a non-transpiring area (°C). Empirical reference temperatures (Twet and Tdry) were estimated by a computational method from infrared thermal images.
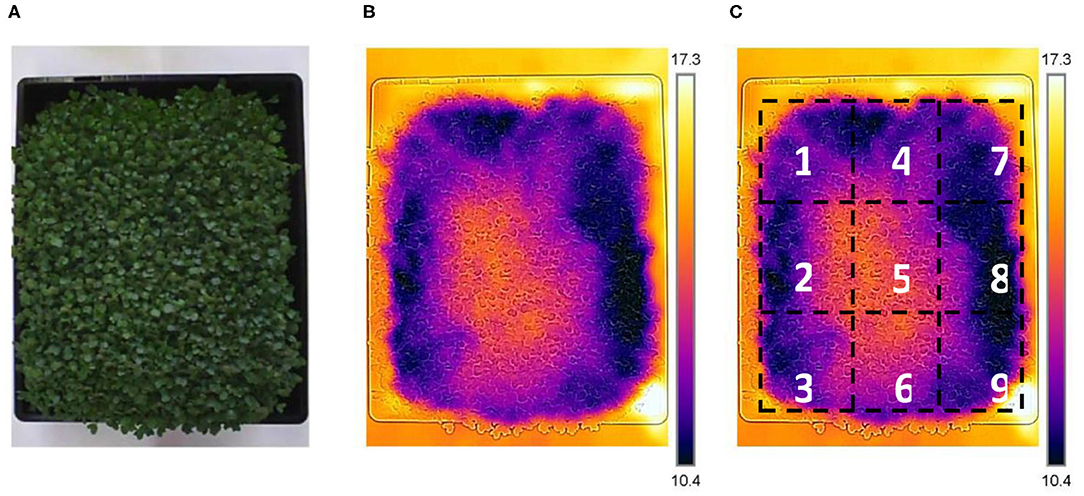
Figure 3. Sample thermal image taken by FLIR camera. Image (A) shows a normal photo, image (B); an infrared thermal photo and image (C), 3 × 3 subdivision of a thermal image to extract data from various sections of the growing container.
Canopy temperature depression (CTD) was calculated using the formula below:
Using the temperature data (Tdry, Tc, and Twet) derived from the computational analysis of the infrared images, an infrared index (Ig) was calculated from the following formula (Jones, 1999; Jones et al., 2002; Fuentes et al., 2012):
As suggested by Jones et al. (2002), Equation 10 also shows that Ig is proportional to the leaf conductance to water vapor transfer (gs). In this formula raw is the boundary layer resistance to water vapor, rHR is the parallel resistance to heat and radiative transfer, γ is the psychrometric constant, and s is the slope of the curve relating saturation vapor pressure to temperature.
Statistical Analysis
Data were subjected to analysis of variance using the General Linear Model procedures followed by the Fisher LSD test at P ≤ 0.05 in Minitab 18 software (Minitab Inc., State College, PA, USA). The data collected for various morphological and biochemical traits, as well as the data derived from thermal images, were also analyzed using multivariate analysis techniques using a custom code developed in MATLAB ver2016b (MathWorks Inc., Natick, MA, 205 USA) for principal component analysis (PCA), cluster analysis, and correlation matrix algorithms to evaluate the effects of irrigation levels and the irrigation methods.
Results
Fresh Yield, Water Use Efficiency (WUE), and Root: Shoot (RS) Ratio
Fresh yield response was not notably affected by the irrigation method, except at 17.5%EVC where sensor-based method showed significantly higher yield (p = 0.02) (Table 3). In terms of irrigation levels, no significant differences in fresh yield were detected among 35, 25, and 17.5% EVC. The average fresh yield in these irrigation levels was ~1.9 kg. m−2 in the sensor-based and 1.8 kg. m−2 in the gravimetric method; 2.3 and 2.6 times higher than the fresh yield at 7.5% EVC in sensor-based (0.8 kg. m−2) and gravimetric (0.7 kg. m−2) methods, respectively. Dry matter (DM) was similar among 35–17.5% EVC treatments in both gravimetric and sensor-controlled irrigation methods, ranging from 5.3 g to 6 g. 100 g−1 FW. However, at the lowest irrigation setpoint, in both irrigation methods (7.5% EVC), DM significantly increased to 7 g. 100 g−1 FW (Table 3).
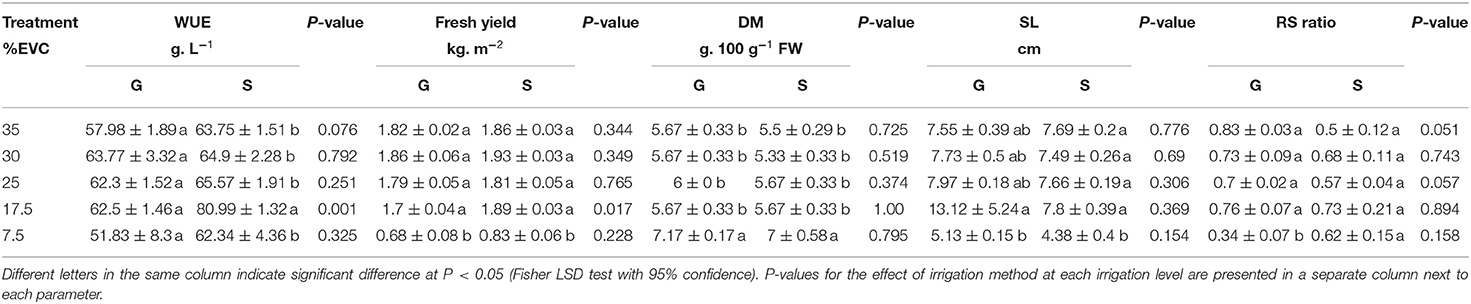
Table 3. Mean values of water use efficiency (WUE), fresh yield, dry matter (DM), shoot length (SL), and root: shoot (RS) ratio in kale microgreens grown using two irrigation methods; gravimetric (G) or sensor-based (S) in a soilless system (%EVC, effective volume of container).
Overall, a sensor-based irrigation system significantly increased WUE (by 13%) compared with the gravimetric method, showing its superiority over the gravimetric method by accurately identifying water status in a soilless system. Although statistical differences were not detected at every irrigation level, WUE was significantly improved at 17.5% EVC where the sensor-based irrigation increased this parameter by 30% in comparison with the gravimetric method (p = 0.001). Even in the well-watered treatment (35% EVC), WUE in the gravimetric method was 10% lower than the sensor-based method. For both irrigation methods, WUE decreased by increasing the irrigation setpoint except at 7.5% EVC. In sensor-based irrigation, WUE significantly increased from 62.34 g. L−1 in 7.5% EVC to 80.98 g. L−1 in 17.5% EVC, and then dropped to 65.6 g. L−1 in 25% EVC remained constant from thereon (Table 3).
In gravimetric irrigation also, WUE increased by 20% from 7.5% EVC to 17.5% EVC. It then remained constant from 17.5 to 30% EVC and slightly decreased from 30 to 35% EVC (57.98 g. L−1) (Table 3).
The root: shoot (RS) ratio was unaffected by the irrigation method and the irrigation level in the sensor-based system. In the gravimetric method, however, 7.5% EVC significantly lowered the RS ratio (0.34) (Table 3). Unlike RS ratio, shoot length reduced significantly at 7.5% EVC in both irrigation methods (p = 0.04).
Proline Content and Leaf Pigments
Proline content increased at the severe deficit irrigation level (7.5% EVC) in both irrigation methods. In the gravimetric method, proline content was about 2 to 2.5 times higher at 7.5% EVC compared to the other irrigation levels (Table 4). In the sensor-based method, 7.5% EVC resulted in 4.6–5.4 times higher proline accumulation than the rest of the irrigation levels. There were no significant differences in proline content between the two irrigation methods at each corresponding irrigation level.

Table 4. Effect of different irrigation levels and irrigation methods of either gravimetric (G) or sensor (S) based on photosynthetic pigments (Chl a, chlorophyll a; Chl b, chlorophyll b; Chl T, total chlorophyll content; Car, total carotenoids) and proline accumulation in kale microgreens (%EVC, effective volume of container).
Total chlorophyll content ranged from 94.9 to 144.3 μg. g −1 FW and total carotenoids ranged from 18.22 to 21.92 μg. g −1 FW. The effect of various irrigation levels on total chlorophylls and carotenoids of the microgreens was found to be insignificant. Chlorophyll a and b were also not affected by the irrigation level, regardless of the irrigation method. Irrespective of the treatment, microgreens in this study contained up to 2.3 mg. 100 g−1 FW of carotenoids (Table 4).
Plant Water Status Based on Infrared Thermal Imagery
The results of Tc are shown in Table 5. Canopy temperature (Tc) was lower than the ambient temperature (22°C) in all the treatments and was the highest at 7.5% EVC (15.1 and 13.8°C in sensor-based and gravimetric methods, respectively). No significant differences were detected in terms of irrigation methods except at 17.5% EVC where Tc in the gravimetric method was significantly lower than the sensor-based method (p = 0.04). Irrespective of the irrigation method, Tc at 7.5% EVC was 20–25% higher than other irrigation levels with greater water input. A similar trend was detected for CTD values, with 17.5% EVC being significantly affected by the irrigation method (p = 0.04) and the irrigation level of 7.5% EVC showing the largest CTD values (−8.2 and −6.9°C in gravimetric and sensor-based methods, respectively).

Table 5. Mean maximum temperature (T dry), minimum temperature (T wet), canopy temperature (Tc), canopy temperature depression (CTD), crop water stress index (CWSI), and infrared index (Ig) derived from infrared thermal imaging (%EVC, effective volume of container).
Means for the Crop water stress index (CWSI) are shown in Table 5, ranging from ~0.4–0.8 across both irrigation methods. CWSI was not affected by the irrigation method. Like Tc, 7.5% EVC resulted in significantly higher CWSI values in both irrigation methods (0.6 and 0.8 in gravimetric and sensor-based methods, respectively).
Table 5 shows the mean values for infrared index (Ig) in both gravimetric and sensor-based methods. The irrigation method had no significant effects on Ig values. However, the irrigation level significantly affected Ig with 7.5% EVC, showing the smallest Ig in the sensor-based method (0.4).
Multivariate Data Analysis
The results of multivariate data analysis from both irrigation methods, including gravimetric and sensor-based, are shown in Figure 4. The PCA explained a total of 83.78% (PCA 1 = 67.69 and PCA 2 =16.09) of variance for the sensor-based treatments and 86.66% (PCA 1 = 61.30 and PCA 2 = 25.36) for the gravimetric method. Figure 4A shows two distinctive groups for the sensor-based irrigation levels; 7.5% EVC (number 1) located further away from the origin of the chart in the positive direction toward proline, Tc, and CWSI, and a second group (number 2) including 17.5, 25, 30, and 35% EVC are scattered near and away from the origin in the negative direction of the mentioned traits. Figure 4B, shows three separate groups for the gravimetric treatments. The 7.5% EVC treatment (number 1) is located away from the origin in the positive direction for proline, Tc, and CTD. The 25% EVC and 17.5% EVC treatments are located close to one another, forming the second group (number 2) slightly away from the center of the graph. And finally, the 30 and 35% EVC treatments (number 3) are located close to the center of the chart and in the same direction as WUE and fresh yield.
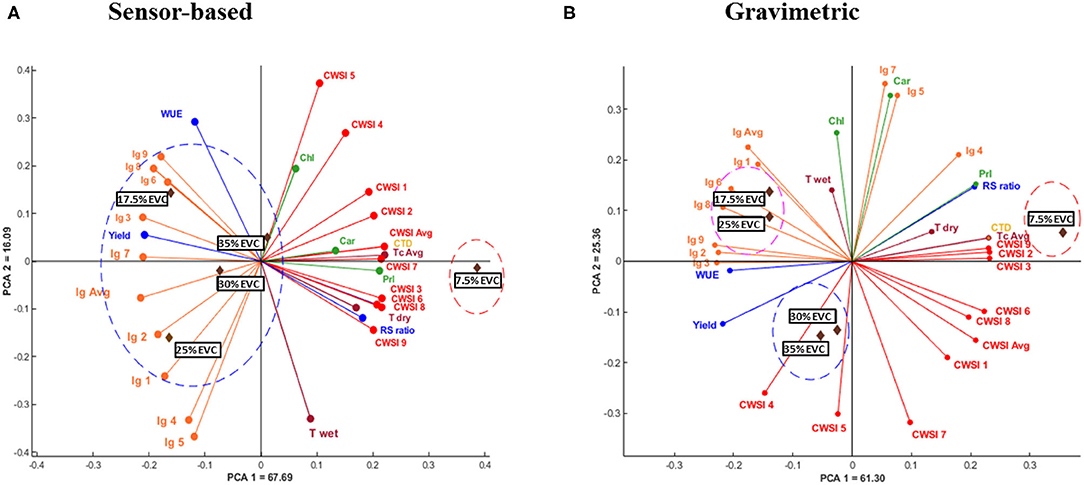
Figure 4. (A,B) Multivariate data analysis showing principal components analysis (PCA) biplot for the studied parameters including water use efficiency (WUE), root: shoot (RS) ratio, yield, average canopy temperature (Tc Avg), maximum temperature (Tdry), minimum temperature (Twet), canopy temperature depression (CTD), average crop water stress index (CWSI Avg), proline (Prl), total chlorophylls (Chl), and carotenoids (Car) content derived from (A)- sensor-based and (B)- gravimetric irrigation methods. Numbered parameters including Ig 1–Ig 9 as well as CWSI 1 -CWSI 9 refer to the infrared index (Ig) and CWSI values obtained from corresponding nine subsections in each growing container. Tc Avg, CWSI Avg, and Ig Avg represent the average of nine Tc, CWSI, and Ig values, respectively. Treatments included five irrigation levels including 7.5, 17.5, 25, 30, and 35% EVC.
Figure 5 shows the correlation matrix for the studied parameters including WUE, RS ratio, fresh yield, average canopy temperature (Tc Avg), maximum temperature (Tdry), minimum temperature (Twet), CTD, average crop water stress index (CWSI Avg), proline, total chlorophylls, and carotenoids in sensor-based and gravimetric trials. In both irrigation methods, fresh yield was negatively correlated with proline accumulation, R: S ratio, CTD, and Tc, while proline itself was positively correlated with Tc and CTD (Figures 5A,B). Fresh yield was also negatively correlated with Tdry and CWSI in the sensor-based method (Figure 5A), while it did not show significant correlations in the gravimetric method. Tdry was positively correlated with proline and carotenoid content in the sensor-based method, while it did not show any notable correlations in the gravimetric method. The average of nine CWSI values showed significant negative correlations with the average Ig values for both irrigation methods. In the sensor-based method, a negative correlation was observed between CWSI values of each subdivision showed as CWSI 1–CWSI 9) with its corresponding Ig value (Ig1–Ig9) (Figure 5A). Similar observations were found in the gravimetric trial, but it was not as consistent across all the sub-divisions (Figure 5B). CWSI 2 and CWSI 3 values were negatively correlated with fresh yield in both trials. Additionally, CWSI 8 and 7 in the sensor-based method and CWSI 9 in the gravimetric method showed significant negative correlations with fresh yield.
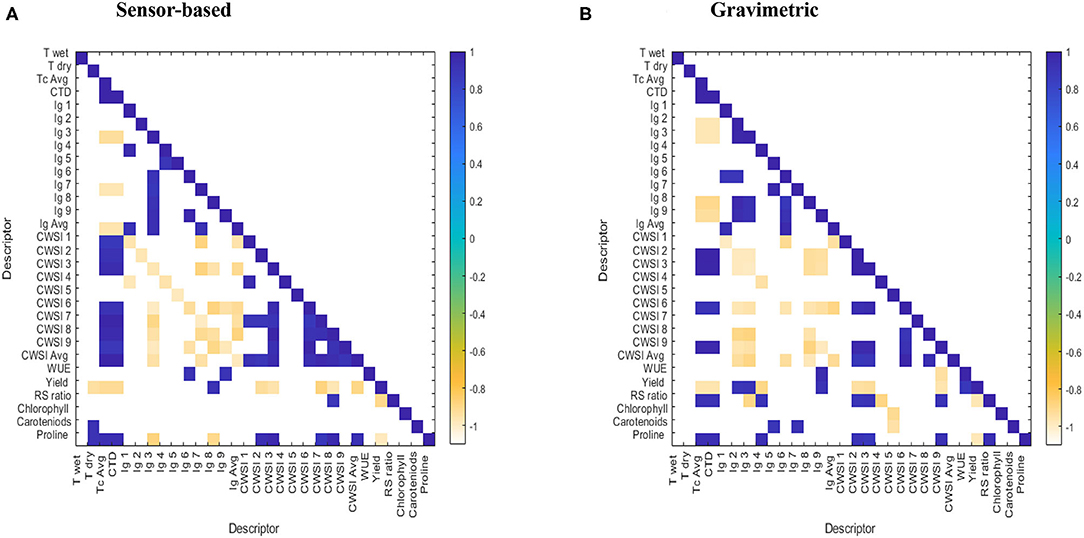
Figure 5. (A,B) Correlation matrix for the studied parameters including water use efficiency (WUE), root: shoot (RS) ratio, yield, average canopy temperature (Tc Avg), maximum temperature (Tdry), minimum temperature (Twet), canopy temperature depression (CTD), average crop water stress index (CWSI Avg), proline, total chlorophylls (Chlorophyll) and carotenoids content derived from (A)- sensor-based and (B)- gravimetric irrigation methods. Numbered parameters including Ig 1–Ig 9 as well as CWSI 1 -CWSI 9 refer to the Ig and CWSI values obtained from corresponding nine subsections in each growing container. Tc Avg, CWSI Avg, and Ig Avg represent the average of nine Tc, CWSI and Ig values, respectively. Treatments included five irrigation levels including 7.5, 17.5, 25, 30, and 35% EVC.
Discussion
The sensor-based irrigation method either improved or was the same as the gravimetric based irrigation for all physiological measurements of fresh yield, WUE, and RS ratio, demonstrating that sensor-based irrigation can be successfully implemented for precise prediction of water level without compromising fresh yield, quality, and WUE. Besides, compared with gravimetric measurements, using the moisture sensor is easier, less laborious, and better applicable to large scale soilless production systems at a low cost. Our results on WUE are in agreement with Burnett and van Iersel (2008). They reported that WUE decreased with an increasing volumetric water content of the substrate at higher irrigation set points in a sensor-based irrigation system when growing gaura plants, which is consistent with our observation of WUE at the most upper setpoint (35% EVC), which has reduced in comparison with setpoints of 17.5% EVC and greater. Water use efficiency (WUE) varies with the plant species and the environmental conditions (Blum, 2005). To limit desiccation when water is less available in the substrate, stomata tends to minimize transpiration by closing the pore. Stomata closure happens when guard cells lose more water than they intake due to ion efflux and consequently shrinkage. Closing stomata reduces gas exchange and prevents excessive water loss. This will reduce the CO2 supply, leading to RuBisCO, the main enzyme responsible for CO2 fixation, prefer fixing the excessively available O2 molecules over CO2 (Peterhansel et al., 2010). This phenomenon is called photorespiration which inhibits photosynthesis and leads to formation of reactive oxygen species (ROS) [ROS, reviewed in Murata et al. (2007)]. Therefore, in drought conditions, photosynthetic activity will be restricted due to less CO2 being supplied (Taiz and Zeiger, 1998; Vashi et al., 2020). This explains the lower WUE at 7.5% EVC and shows that less CO2 was assimilated despite minimal water use at this level. Besides lower transpiration caused by the stomata closure, it also results in higher Tc and smaller CTD values (Table 5).
In this study, low water availability at 7.5% EVC decreased the shoot length of the microgreens which is another indicator of reduced growth and photosynthesis as a result of stomata closure (Guo et al., 2020). In addition, in higher plants, growth mostly occurs by cell elongation which increases with increasing turgor potential (Taiz and Zeiger, 1998). Low water availability typically decreases turgor potential, which as a result suppresses cell elongation (Burnett and van Iersel, 2008). Higher dry biomass in the aboveground parts of microgreens, exposed to 7.5% EVC, may be explained by the primary allocation of carbohydrates to the shoots, to the detriment of roots, under stress conditions (Guo et al., 2020).
Under abiotic stresses, plants tend to increase the accumulation of certain metabolites that would help combat the stress conditions (Biju et al., 2017). Proline is an amino acid, which is excessively produced when plants are in drought conditions. Stabilizing the structure of the cell membranes and suppressing the activity of ROS causing oxidative damage, helps increase drought resistance in plants (Szabados and Savoure, 2010). Usually, the level of proline ranges between 0.5 (in control conditions) to 50 μmol. g−1 FW (stressed) (Sithtisarn et al., 2007). With all proline levels in 17.5 −35% EVC being in the range of 1.16–2.00 μmol. g−1 FW, the results suggest that microgreens were unstressed at these irrigation levels. On the contrary, 7.5% EVC resulted in much higher proline content, showing a greater impact of the minimal water supply. Sarker and Oba (2018) also reported that drought stress resulted in higher accumulation of proline in fully grown amaranth plants grown in a controlled soil-based pot trial.
Chlorophyll and carotenoids are main photosynthetic pigments responsible for light harvesting and have an important role in indicating the visual quality of leafy vegetables (Ferrante et al., 2004). Plants grown in various stressful conditions can show a reduction in the content of their photosynthetic pigments such as chlorophylls and carotenoids (Chaves et al., 2009). The insignificant effects of the irrigation levels on chlorophyll and carotenoid content in this study can be assumed to be because of the lowest irrigation level (7.5% EVC) not being severe enough to affect the pigments.
Canopy temperature (Tc) has been used in water management studies as it successfully indicates plant water status (Kumar et al., 2020). In drought stress conditions, plants tend to keep their stomata closed, leading to a lower transpiration rate, and ultimately increasing the Tc. In non-stressed conditions, though, Tc is kept at a metabolically suitable range by transpiration through the open stomata (Kashiwagi et al., 2008; Biju et al., 2018). To the best of our knowledge, this is the first study implementing infrared thermal imagery to evaluate water status in microgreens. Our results indicated that similar to fully grown plants such as lentil (Biju et al., 2018), chickpea (Kashiwagi et al., 2008), Indian mustard (Kumar et al., 2020), and olive trees (Egea et al., 2017), Tc derived from infrared images could be indicative of water status in microgreens as well.
Crop water stress index (CWSI) has been widely used for indicating plant water status and irrigation management in different crops (Egea et al., 2017). In this study, the lowest irrigation level (7.5% EVC) resulted in a notable increase in CWSI. Kumar et al. (2020) also reported that CWSI increased when plants were under drought stress in a field experiment on irrigation scheduling of Indian mustard. Crop water stress index (CWSI) ranges between zero to one, with one being severely stressed and zero being non-stressed. Hence, observing values closer to one in treatments with minimal water supply (7.5% EVC) is within expectation. Also, CWSI values obtained from some marginal subsections in various treatments showed to be negatively correlated with fresh yield which could have been caused by the edge effect.
Canopy temperature depression (CTD) shows the canopy-air temperature differences and the effectiveness of transpiration in cooling down the canopy under increasing atmospheric demand and correlates with transpiration efficiency (Karimizadeh and Mohammadi, 2011). In this study, CTD values of up to 11°C were observed (Table 5) indicating that the canopy temperature was always lower than the air temperature. Such difference could be due to the low energy input in the controlled environment growth room, the crop being grown in a hydroponic setup, minimal heat waste from the light source (LED lights) and constant air circulation in the growth room. In previous studies, CTD values of 7.5, 10, and 12°C have been reported for sorghum (Zhang et al., 2019), alfalfa (Moran et al., 1994) and soybean (Hou et al., 2019), respectively.
Canopy temperature depression (CTD) values may indicate various factors such as environmental conditions, plant phenological stage, and the available soil (media) moisture. In stressed conditions, CTD could also be indicative of the demand for photo-assimilation (Kumar et al., 2017). This study showed that the CTD values were the largest when the lowest irrigation level was applied. Amani et al. (1996) explained that for a genotype, CTD is a function of several environmental factors, including soil (media) water status, air temperature, relative humidity, and incident radiation.
Previous studies have shown that there is a linear relationship between Ig and gs in other horticultural plants, including grapevines (Jones et al., 2002; Fuentes et al., 2019), coffee plants (Craparo et al., 2017), and olive trees (Egea et al., 2017). Stomata opening rapidly responds to changing water status by regulating leaf CO2 and H2O gas exchange fluxes (Chaves et al., 2003). By opening the stomata for maximum photosynthetic gain and closing it for avoiding water loss, these fluxes consequently regulate the Tc (Egea et al., 2017). As shown in Table 5, the smallest CTD values were in 7.5% EVC while having minimal Ig, which further highlights the effect of stomatal conductance on Tc.
The PCA results in this study are consistent with the criteria developed by Sneath and Sokal (1973) who showed that the results of the PCA analysis must explain a minimum of 70% of the total variability. In both irrigation methods, the PCA biplot categorized the 7.5% EVC in a distinctive group from the other irrigation levels which further explains the effect of treatments.
Conclusion
The findings of the current study indicated that implementing the moisture sensor can increase WUE without compromising the fresh yield and quality of the microgreens. This study is the first to apply infrared thermal imaging techniques to closely monitor water status in microgreens and facilitate the identification of an optimal irrigation level. Using an irrigation setpoint of 17.5% EVC was demonstrated to be the most efficient setpoint for potential implementation of the sensor in an automated or semiautomated soilless system for growing microgreens. Although many studies have shown the benefits of sensor-based irrigation, the main constraint to applying these technologies on a commercial scale appears to be the direct expenses associated with deploying the sensors. On the contrary, the dielectric moisture sensor used in this study showed to be as effective at a lower cost. This research also showed that the sensor-based irrigation system was associated with an increase in WUE and reducing excessive water use. Therefore, using a low-cost moisture sensor can lead to reductions in environmental impacts and the pressure on water resources. The findings of this study could be applied to similar species grown as microgreens; however, the environmental impacts may be greater in crops with a longer growing cycle and/or higher water consumption. Hence, additional research on the use of moisture sensors in other microgreens, baby greens, and leafy vegetables deserves further attention due to their potential in reducing the environmental footprint of the indoor vegetable industry.
Data Availability Statement
The original contributions presented in the study are included in the article/supplementary material, further inquiries can be directed to the corresponding author/s.
Author Contributions
MT, DG, GB, SF, and AP conceived and designed the experiment. MT and BW conducted the experiment, collected and analyzed the data. MT wrote the first draft of the manuscript. DG, GB, AP, and SF assisted with the data analysis and edited the manuscript. All authors read and approved the final manuscript.
Funding
We wish to thank The University of Melbourne for the Doctoral Scholarship to MT and funding support to work on this project.
Conflict of Interest
The authors declare that the research was conducted in the absence of any commercial or financial relationships that could be construed as a potential conflict of interest.
References
Amani, I., Fischer, R., and Reynolds, M. (1996). Canopy temperature depression association with yield of irrigated spring wheat cultivars in a hot climate. J Agronomy Crop Sci. 176, 119–129. doi: 10.1111/j.1439-037X.1996.tb00454.x
Bates, L. S., Waldren, R. P., and Teare, I. (1973). Rapid determination of free proline for water-stress studies. Plant Soil 39, 205–207. doi: 10.1007/BF00018060
Biju, S., Fuentes, S., and Gupta, D. (2017). Silicon improves seed germination and alleviates drought stress in lentil crops by regulating osmolytes, hydrolytic enzymes and antioxidant defense system. Plant Physiol. Biochem. 119, 250–264. doi: 10.1016/j.plaphy.2017.09.001
Biju, S., Fuentes, S., and Gupta, D. (2018). The use of infrared thermal imaging as a non-destructive screening tool for identifying drought-tolerant lentil genotypes. Plant Physiol. Biochem. 127, 11–24. doi: 10.1016/j.plaphy.2018.03.005
Blum, A. (2005). Drought resistance, water-use efficiency, and yield potential—are they compatible, dissonant, or mutually exclusive? Aust. J. Agric. Res. 56, 1159–1168. doi: 10.1071/AR05069
Burnett, S. E., and van Iersel, M. W. (2008). Morphology and irrigation efficiency of Gaura lindheimeri grown with capacitance sensor-controlled irrigation. HortScience 43, 1555–1560. doi: 10.21273/HORTSCI.43.5.1555
Chaves, M. M., Flexas, J., and Pinheiro, C. (2009). Photosynthesis under drought and salt stress: regulation mechanisms from whole plant to cell. Ann. Bot. 103, 551–560. doi: 10.1093/aob/mcn125
Chaves, M. M., Maroco, J. P., and Pereira, J. S. (2003). Understanding plant responses to drought—from genes to the whole plant. Funct. Plant Biol. 30, 239–264. doi: 10.1071/FP02076
Craparo, A., Steppe, K., van Asten, P. J., Läderach, P., Jassogne, L. T., and Grab, S. (2017). Application of thermography for monitoring stomatal conductance of Coffea arabica under different shading systems. Sci. Total Environ. 609, 755–763. doi: 10.1016/j.scitotenv.2017.07.158
Di Gioia, F., and Santamaria, P. (2015). Microgreens: Novel Fresh and Functional Food to Explore All the Value of Biodiversity. Bari: ECO-logica.
Egea, G., Padilla-Díaz, C. M., Martinez-Guanter, J., Fernández, J. E., and Pérez-Ruiz, M. (2017). Assessing a crop water stress index derived from aerial thermal imaging and infrared thermometry in super-high density olive orchards. Agric. Water Manag. 187, 210–221. doi: 10.1016/j.agwat.2017.03.030
Ferrante, A., Incrocci, L., Maggini, R., Serra, G., and Tognoni, F. (2004). Colour changes of fresh-cut leafy vegetables during storage. J. Food Agric. Environ. 2, 40–44.
Ferrarezi, R. S., Dove, S. K., and van Iersel, M. W. (2015). An automated system for monitoring soil moisture and controlling irrigation using low-cost open-source microcontrollers. HortTechnology 25, 110–118. doi: 10.21273/HORTTECH.25.1.110
Fuentes, S., de Bei, R., Pech, J., and Tyerman, S. (2012). Computational water stress indices obtained from thermal image analysis of grapevine canopies. Irrigation Sci. 30, 523–536. doi: 10.1007/s00271-012-0375-8
Fuentes, S., Tongson, E. J., de Bei, R., Gonzalez Viejo, C., Ristic, R., Tyerman, S., et al. (2019). Non-invasive tools to detect smoke contamination in grapevine canopies, berries and wine: a remote sensing and machine learning modeling approach. Sensors 19:3335. doi: 10.3390/s19153335
Guo, T., Tian, C., Chen, C., Duan, Z., Zhu, Q., and Sun, L. Z. (2020). Growth and carbohydrate dynamic of perennial ryegrass seedlings during PEG-simulated drought and subsequent recovery. Plant Physiol. Biochem. 154, 85–93. doi: 10.1016/j.plaphy.2020.06.008
Hou, M., Tian, F., Zhang, T., and Huang, M. (2019). Evaluation of canopy temperature depression, transpiration, and canopy greenness in relation to yield of soybean at reproductive stage based on remote sensing imagery. Agric. Water Manage. 222, 182–192. doi: 10.1016/j.agwat.2019.06.005
Jadhav, H., and Rosentrater, K. (2017). Economic and environmental analysis of greenhouse crop production with special reference to low cost greenhouses: a review. ASABE Annual International Meeting, American Society of Agricultural and Biological Engineers, 1. doi: 10.13031/aim.201701178
Jones, H. G. (1999). Use of infrared thermometry for estimation of stomatal conductance as a possible aid to irrigation scheduling. Agric. Forest Meteorol. 95, 139–149. doi: 10.1016/S0168-1923(99)00030-1
Jones, H. G. (2007). Monitoring plant and soil water status: established and novel methods revisited and their relevance to studies of drought tolerance. J. Exp. Bot. 58, 119–130. doi: 10.1093/jxb/erl118
Jones, H. G., Stoll, M., Santos, T., Sousa, C. D., Chaves, M. M., and Grant, O. M. (2002). Use of infrared thermography for monitoring stomatal closure in the field: application to grapevine. J. Exp. Bot. 53, 2249–2260. doi: 10.1093/jxb/erf083
Karimizadeh, R., and Mohammadi, M. (2011). Association of canopy temperature depression with yield of durum wheat genotypes under supplementary irrigated and rainfed conditions. Aust. J. Crop Sci. 5:138.
Kashiwagi, J., Krishnamurthy, L., Upadhyaya, H., and Gaur, P. (2008). Rapid screening technique for canopy temperature status and its relevance to drought tolerance improvement in chickpea. J. SAT Agric. Res. 6, 105–104. doi: 10.1016/j.fcr.2007.07.007
Kumar, M., Govindasamy, V., Rane, J., Singh, A., Choudhary, R., Raina, S., et al. (2017). Canopy temperature depression (CTD) and canopy greenness associated with variation in seed yield of soybean genotypes grown in semi-arid environment. South African J. Bot. 113, 230–238. doi: 10.1016/j.sajb.2017.08.016
Kumar, N., Poddar, A., Shankar, V., Ojha, C. S. P., and Adeloye, A. J. (2020). Crop water stress index for scheduling irrigation of Indian mustard (Brassica juncea) based on water use efficiency considerations. J. Agronomy Crop Sci. 206, 148–159. doi: 10.1111/jac.12371
Kyriacou, M. C., Rouphael, Y., di Gioia, F., Kyratzis, A., Serio, F., Renna, M., et al. (2016). Micro-scale vegetable production and the rise of microgreens. Trends Food Sci. Technol. 57, 103–115. doi: 10.1016/j.tifs.2016.09.005
Lea-Cox, J. D. (2012). Using Wireless Sensor Networks for Precision Irrigation Scheduling. Problems, Perspectives and Challenges of Agricultural Water Management. Rijeka: InTech Press, 233–258.
Manchali, S., Murthy, K. N. C., and Patil, B. S. (2012). Crucial facts about health benefits of popular cruciferous vegetables. J. Funct. Foods 4, 94–106. doi: 10.1016/j.jff.2011.08.004
Migliozzi, M., Thavarajah, D., Thavarajah, P., and Smith, P. (2015). Lentil and kale: complementary nutrient-rich whole food sources to combat micronutrient and calorie malnutrition. Nutrients 7, 9285–9298. doi: 10.3390/nu7115471
Moran, M., Clarke, T., Inoue, Y., and Vidal, A. (1994). Estimating crop water deficit using the relation between surface-air temperature and spectral vegetation index. Remote Sens. Environ. 49, 246–263. doi: 10.1016/0034-4257(94)90020-5
Murata, N., Takahashi, S., Nishiyama, Y., and Allakhverdiev, S. I. (2007). Photoinhibition of photosystem II under environmental stress. Biochim. Biophys. Acta 1767, 414–421. doi: 10.1016/j.bbabio.2006.11.019
Olympios, C. (1999). Overview of soilless culture: advantages, constraints and perspectives for its use in Mediterranean countries. Cahiers Options Méditerranéennes 31, 307–324.
O'Sullivan, C., Bonnett, G., Mcintyre, C., Hochman, Z., and Wasson, A. (2019). Strategies to improve the productivity, product diversity and profitability of urban agriculture. Agric. Syst. 174, 133–144. doi: 10.1016/j.agsy.2019.05.007
Peterhansel, C., Horst, I., Niessen, M., Blume, C., Kebeish, R., Kürkcüoglu, S., et al. (2010). Photorespiration. Arabidopsis Book 8:e0130. doi: 10.1199/tab.0130
Pignata, G., Casale, M., and Nicola, S. (2017). Water and nutrient supply in horticultural crops grown in soilless culture: resource efficiency in dynamic and intensive systems. In: Advances in Research on Fertilization Management of Vegetable Crops. Cham: Springer doi: 10.1007/978-3-319-53626-2_7
Šamec, D., Urlić, B., and Salopek-Sondi, B. (2019). Kale (Brassica oleracea var. acephala) as a superfood: review of the scientific evidence behind the statement. Crit. Rev. Food Sci. Nutr. 59, 2411–2422. doi: 10.1080/10408398.2018.1454400
Sarker, U., and Oba, S. (2018). Drought stress effects on growth, ROS markers, compatible solutes, phenolics, flavonoids, and antioxidant activity in Amaranthus tricolor. Appl. Biochem. Biotechnol. 186, 999–1016. doi: 10.1007/s12010-018-2784-5
Sithtisarn, S., Harinasut, P., Pornbunlualap, S., and Cha-Um, S. (2007). Accumulation of glycinebetaine and betaine aldehyde dehydrogenase activity in Eucalyptus camaldulensis clone T5 under in vitro salt stress. Kasetsart University.
Sneath, P. H., and Sokal, R. R. (1973). Numerical taxonomy. The principles and practice of numerical classification, San Francisco, CA: W.H. Freeman and Co.
Szabados, L., and Savoure, A. (2010). Proline: a multifunctional amino acid. Trends Plant Sci. 15, 89–97. doi: 10.1016/j.tplants.2009.11.009
Taiz, L., and Zeiger, E. (1998). Plant Physiology (2nd edn). Sunderland: Sinaure Associates, Sunderland, Ma. 792.
Vashi, H. D., Patel, P. P., and Bardhan, K. (2020). Growth and physiological responses of vegetable crops to water deficit stress. J. Exp. Agric. Int. 42, 91–101. doi: 10.9734/jeai/2020/v42i530523
Waterland, N. L., Moon, Y., Tou, J. C., Kim, M. J., Pena-Yewtukhiw, E. M., and Park, S. (2017). Mineral content differs among microgreen, baby leaf, and adult stages in three cultivars of kale. HortScience 52, 566–571. doi: 10.21273/HORTSCI11499-16
Xiao, Z., Codling, E. E., Luo, Y., Nou, X., Lester, G. E., and Wang, Q. (2016). Microgreens of brassicaceae: mineral composition and content of 30 varieties. J. Food Compos. Anal. 49, 87–93. doi: 10.1016/j.jfca.2016.04.006
Xiao, Z. L., Lester, G. E., Luo, Y. G., and Wang, Q. (2012). Assessment of vitamin and carotenoid concentrations of emerging food products: edible microgreens. J. Agric. Food Chem. 60, 7644–7651. doi: 10.1021/jf300459b
Keywords: dielectric sensors, hydroponics, microgreens, urban agriculture, indoor farming, digital farming, irrigation management, water use efficiency
Citation: Tavan M, Wee B, Brodie G, Fuentes S, Pang A and Gupta D (2021) Optimizing Sensor-Based Irrigation Management in a Soilless Vertical Farm for Growing Microgreens. Front. Sustain. Food Syst. 4:622720. doi: 10.3389/fsufs.2020.622720
Received: 29 October 2020; Accepted: 31 December 2020;
Published: 26 January 2021.
Edited by:
Yaosheng Wang, Chinese Academy of Agricultural Sciences, ChinaReviewed by:
Felipe H. Barrios Masias, University of Nevada, Reno, United StatesZhang Yucui, Chinese Academy of Sciences (CAS), China
Copyright © 2021 Tavan, Wee, Brodie, Fuentes, Pang and Gupta. This is an open-access article distributed under the terms of the Creative Commons Attribution License (CC BY). The use, distribution or reproduction in other forums is permitted, provided the original author(s) and the copyright owner(s) are credited and that the original publication in this journal is cited, in accordance with accepted academic practice. No use, distribution or reproduction is permitted which does not comply with these terms.
*Correspondence: Dorin Gupta, ZG9yaW4uZ3VwdGFAdW5pbWVsYi5lZHUuYXU=