- 1Invermay Research Centre, Farm Systems and Reporduction, Digital Agriculture, AgResearch Ltd., Mosgiel, New Zealand
- 2Invermay Research Centre, Animal Genomics, Digital Agriculture, AgResearch Ltd., Mosgiel, New Zealand
- 3Ruakura Research Centre, Environmental Science, Ethical Agriculture, AgResearch Ltd., Hamilton, New Zealand
- 4Lincoln Research Centre, Bioinformatics and Statistics, Digital Agriculutre, AgResearch Ltd., Lincoln, New Zealand
- 5Ruakura Research Centre, Mathematical Modelling, Digital Agriculture, AgResearch Ltd., Hamilton, New Zealand
Digital technologies provide an opportunity to further increase the sustainability and productivity of grasslands and rangelands. Three resources are key to that change. These are the soil on which forage grows, the forages that grow on those soils and the animals that use the forage resource as food. This paper describes elements of technologies to measure and monitor these resources and provides some insights on combining that knowledge and controlling the animal's utilization through virtual fencing. There are many potential challenges to the application of digital technologies to pastoral farming. These often require the calibration of digital signals to define biophysical characteristics. The significant repository of historic data of pasture growth over many geo-climatic regions, for example, provides New Zealand with an opportunity to accelerate that development. Future advances in rangeland use, nutrient deposition, greenhouse gas emissions and the provision and utilization of high quality and quantity will be enabled by the application of digital technologies at scale, under the control of virtual fencing. Digital technologies may provide the means to maintain or enhance ruminant production from grassland in a sustainable operating space into the future.
Introduction
Digital technologies have the potential to change the way rangeland and grassland are managed. The rise of the internet-of-things and cloud storage has changed how we collect, store, and process data. Previous systems were paper-based and often transient. Users tended to internalize the knowledge gained from data collection without further storage or application. Such systems have been traditional in many cultures, where learning is passed down through generations, often using spoken histories. Written knowledge has developed significantly with formal learning approaches and records, but individual learnings at a farm or business scale have often still been assigned to experiential, with knowledge passed verbally among participants.
Digital technologies now enable both the collection and storage of data in perpetuity. This data can be transferred easily among users and interpreted remotely. Huge amounts of data, representing numbers, words, sounds and images, can be stored this way because it takes up very little physical space. This also means that the data can be transmitted to, and interpreted by, other users, including machines. The universal nature of digital data provides an enormous opportunity to collect, store, share and interpret data. New information and knowledge can be created through that interpretation.
The activities of humans across the globe has pushed natural systems to, and potentially beyond, their limits (Steffen et al., 2015). While agricultural intensification may be at the forefront of concerns (Campbell et al., 2017), ruminant grazing systems may provide an opportunity to utilize the unique resource that grassland and rangeland provides. This would enable the continued delivery of valuable products without the primary use of crops for animal production (McCoard et al., 2020).
However, current trends in production-driven grassland systems are toward intensification and highly productive and specialized breeds. For example, the Angus beef cattle breed makes up ~60% of the Bos taurus cattle numbers in the world. The trend toward using these highly productive cattle also poses a threat to sustainable land use. Spiegal et al. (2019) used GPS tracking to demonstrate that highly productive cattle (Angus x Hereford) generated twice as many hotspots (mass urine and fecal deposition sites) and less use of native food supplies than the traditional Raramuri Criollo cattle in New Mexico rangeland.
These trends toward intensification and specialization have led to pastoral degradation, reductions in biodiversity, fire protection and ecosystems services such as water provision (Battaglini et al., 2014). Shifts from traditional breeds and approaches also threaten co-developed systems such as the sylvo-pastoral dehesas system in Spain and the montados in Portugal that deliver high ecological and biodiversity values alongside animal products (Buckwell and Nadeu, 2018).
Digital technologies may be able to optimize animal productivity while minimizing environmental impacts. Technologies that provide a unique advantage for grassland and rangeland are those that can be used to characterize of the soil, the pasture/forage resources and the grazing animals. The characterization of each of these provides information on potential intervention and management options. Control of the grazing animal has traditionally been through herding and fencing to place animals in the right part of the environment to meet their nutritional needs, while providing the potential to rest some parts of the grassland to provide feed in the future. The development of virtual fencing technologies has added an extra dimension to both spatial and temporal control of animal grazing and nutrient transfer events. These technologies are not without their limitations. Algorithms need to be created, calibrated and validated to predict biophysical information from digital data. Data, both digital and biophysical contains uncertainty through both the accuracy and precision of measurement (Czarnecki and Podolak, 2013) and inherent expression of the observation (Steel and Torrie, 1980).
New Zealand has a significant history of characterizing this pasture production to understand that variation and provide resources to assist farmers in enterprise choice and systems design. These variations in climatic conditions result in large variation in the amount and seasonal distribution of pasture production (e.g., Radcliffe, 1974; Baars, 1976; Piggot et al., 1978; Roberts and Thomson, 1984). Enterprises are chosen to suit local pasture growth conditions, aimed at maximizing pasture use and minimizing imported feed (McCall and Sheath, 1993). The grazing systems employed are supplemented by some forage cropping to provide extra feed, most often to augment winter and/or summer feed supply depending on the regional imbalance (Stevens, 2009). The most regularly used forage crops, brassicas and beets, provide feed in winter at higher altitudes or higher latitudes. In these instances, usually no more than 5% of the farm might be planted in forage crops.
The range of New Zealand landscapes and their variability, both spatially and temporally, provides the opportunity for unique insight into the role and performance of digital technologies and their potential application on grazed landscapes. Documented characterization of soils, pastures and animals provides a base from which to build, and to use for calibration and reference when developing new digital technologies.
This paper explores a set of critical technologies with which the authors are currently working. It provides a perspective on the implementation of those technologies. This paper aims to provide stimulation of thinking for the reader to facilitate the integration of digital technologies to provide sustainable, productive future landscapes, using New Zealand grassland examples.
Characterizing the Landscape Resources
The ability to quantify the current resource and predict future pasture supply becomes of immense value for resource management and productive outcomes. Quantification of current and future states also provides opportunity to minimize the impacts of management on environmental outcomes such as soil disturbance, water quality and greenhouse gas emissions.
While conventional science has provided a significant base for measuring and understanding the soil and plant resources of grazing ecosystems, often these resources are expensive to implement and have been defined at relatively large scales such as farm, catchment, region or per km2. Refining both the temporal and spatial variations in those resources will drive innovation in the efficient use of, and change in the use of, those resources.
Many grasslands and rangelands are in remote locations, have significant scale per enterprise and have topography that is unsuited for conventional direct measurement techniques. Therefore, direct measurement of soil and pasture is time consuming and expensive especially if high resolution variation is present. The most promising options for broad scale grassland farming is spectral analysis for both plants (Edirisinghe et al., 2012) and soils (Yule et al., 2015).
Using remote sensing via spectral analysis can collect large amounts of data quickly and relatively inexpensively at a scale that can be varied from patch to paddock to farm. Imagery can capture the spectra of radiation that is reflected from the earth's surface (Rouse et al., 1974). These spectra consist of the range of radiation that is received by the earth's surface from the sun, e.g., ultra-violet, visual and infra-red. Spectral analysis uses the principle that the spectra of radiation that is reflected from plants and soils can be calibrated to determine the type of vegetation, the area of exposed soil, the density of plant life and the chemical composition of the plants (Rouse et al., 1974). This information can be collected in a variety of ways, with rapid collection in remote conditions being enabled through satellite imagery or aircraft surveys (Yule et al., 2015).
Resolution has increased, both from satellite, from 1.15 to 3 m2/pixel, and higher with drone footage. This means that the imagery can be used for general biomass estimation (Rouse et al., 1974), down to prediction at the grazing animal scale (Dymond et al., 2006; Edirisinghe et al., 2012). This then enables an increased range of potential decision-making layers or intervention points for management, across large tracts of grassland or rangeland.
Characterizing Grazing Animals
Where animals go and what they do in their environment has significant implications for resource use efficiency and environmental impacts, both positive and negative. The technological advances in micro-processors, genetic/genomic technologies and big data management has created the opportunity to scan large numbers of animals using advanced technologies to gather in-depth data on behavior and personalities. The combination of data from these technologies can then be used to make more informed animal selection decisions, matching animal personalities to environments to improve rangeland utilization and environmental impact. Developing grazing and environmental personalities of animals using global positioning system (GPS), inertial measurement (activity sensors) and urine sensors is explored here as a means of characterizing the grazing ruminant.
Studies of rangeland utilization of farmed ruminants using GPS units has been able to identify different individual behaviors. Bailey et al. (2004) demonstrated that individuals within a herd consistently utilized different terrain and resources than other individuals. Wesley et al. (2012) also demonstrated that individuals that consumed supplements faster in confinement utilized larger rangeland areas and spent less time close to water than individuals that consumed supplement slower. Bailey et al. (2015) was able to associate utilization of terrain type to genotype. This work indicated that several quantitative trait locus (QTL) accounted for significant proportions of variation in terrain use indexes.
The ability to add other sensors to GPS collars has also allowed more behaviors to be captured. GPS units now commonly have multiple axis accelerometers as activity sensors and, when combined with spatial data, can define behaviors such as grazing, traveling and resting (Bailey et al., 2018).
This opportunity to collect both spatial and temporal data of whole herds will allow new insight into grazing behavior, animal impacts and identification of animal genotype suitable for different environments (Bailey et al., 2015). This may increase productive potential and biodiversity retention while minimizing environmental harm and fire risk.
Animal urination is a particularly sensitive activity as it concentrates nitrogen in the environment. This has an important part to play in redistribution of nutrients and creation of hotspots of potential water contamination (Castillo et al., 2000).
Urine sensor technology has gained increasing attention to measure urine excretion from grazing livestock for developing strategies to mitigate farm nitrogen loss. This technology ranges in complexity from measuring the time of each urination event and volume using flow meters or thermistors (e.g., Betteridge et al., 2010; Ravera et al., 2015) to also measure urine nitrogen concentration (Betteridge et al., 2010; Shepherd et al., 2017a).
Urine sensor technology has been used to assess the effect of different forages on urine excretion from livestock (Bryant et al., 2017), and compare changes in farm systems on livestock urine nitrogen production (Shepherd et al., 2017b). Moreover, detailed information on the diurnal and spatial (linked with GPS technology) patterns in urination characteristics could be harnessed to develop new nitrogen mitigation strategies.
Directing Animals in the Environment
Benefits of understanding the pasture and soil resources, and the grazing behaviors and preferences of the livestock can often only be captured by directing livestock to the right part of the landscape. Without control of grazing livestock, landscapes are often under- or over-utilized.
Understocking may result in significant shifts in biodiversity and ecosystem type. For example, the ingress of woody weeds has been identified as a significant issue in many environments (e.g., Archer et al., 2001). Estimates of safe operating limits in Europe identify the need for ruminants in uncultivable grasslands to maintain biodiversity and reduce fire risk (Buckwell and Nadeu, 2018).
Overstocking is also a significant concern, often in regions where control of animals in grasslands and rangelands cannot be implemented. A lack of property rights and low social capital often leads to a lack of resource management due to the “tragedy of the commons.” Often these grazing systems are only “overstocked” because severe continuous grazing restricts pasture growth (Hodgson, 1990). The impacts of ruminants on the landscape are also associated with the uneven distribution of grazing (Bailey, 2005). Controlling animals in the environment provides a solution to overgrazing, uneven grazing and nutrient redistribution.
Controlling animals in a domesticated setting has a documented history of 8,000 to 10,000 years. The opportunity to protect animals, control feed utilization and improve environmental outcomes has made fences indispensable in modern livestock farming. However, current fencing methods are costly, time consuming and potentially not available to all, restricting their implementation and potential benefits. Virtual fencing has enabled new opportunities for animal containment through recent advances in training techniques, coupled with rapid changes in digital technology. Virtual fencing (or herding), is explored in its ability to completely rethink both capital and resource use paradigms, as well as controlling environmental impacts of the grazing animal.
There are several virtual herding systems in development, all based on each animal wearing technology housed in a neckband or collar. These include eShepherd®, NoFence®, Halter®, and Vence®. These are all based on avoidance learning using the cognitive activation theory of stress (Ursin and Eriksen, 2004). This describes a scenario where animals learn to respond to a non-aversive audio stimulus to avoid an aversive electrical pulse. Successful learning occurs when the animal perceives cues to be predictable (audio warning always precedes a pulse) and controllable (operant response to the audio cue prevents receiving the pulse) and an acceptable welfare outcome ensues. After the initial learning period (~6 approaches for 50% of cattle to learn; Campbell et al., 2018), and with coupling of the application of the audio warning consistently at every approach event, responses indicate that cattle learn the situation has high predictability and can avoid the electrical stimulation by responding to the audio cue alone (Lee et al., 2008, 2009).
A GPS receiver in a collar is used to define the area available for the animal to access. The information to set the accessible area is loaded via remote systems such as LoRa or cellular networks, once defined by the user in the associated software. Other sensors in the collar help determine the response of the fence algorithm. The e-Shepherd® for example uses the accelerometer within the collar to detect an animal running toward a virtual fence. The herding software is disabled, recognizing that a charging animal cannot be successfully contained by the training and control of the electric stimulus. The program within the collar tracks the animal and when the animal has stopped running, the fence is reset in such a way to herd the animal back to the original accessible area.
Combining Technologies to Optimize Grassland Management
Knowing where animals are going, how far they travel and what they do when they get there introduces many opportunities. How far an animal travels directly influences their energy requirements. For example, ewe lambs in a semi-intensive grazing environment traveled an average of 3.4 km/day (Johnson, unpublished data) while ewes grazing a large extensive environment traveled 5.3 km/day (Steer, 2012). However, there is large variability between individuals with 3–5-fold differences reported in the above studies, which is both repeatable and heritable (Johnson, unpublished data). Associated with this range of movements is the proportion of the potential grazing area an individual animal encounters. This provides some individuals with much greater access to the feed resource, providing potential benefits in the type and quality of feed available.
Comparative movement and extension of home range also influences the deposition of feces and urine, so altering the pattern and intensity of hot spots in the environment (Spiegal et al., 2019). The use of urine sensors to characterize the relative variation in urine deposition of animals could also be used to allocate appropriate animals to different environments, depending on the sensitivity of that environment to nitrogen overloading.
Using activity sensors to understand individual animal intakes would improve animal management through matching feed requirements with feed availability. It would also allow detection of reduced intakes. Indoor feed intake studies in sheep (and cattle) have demonstrated large between-animal variability in how animals manage their daily intake. This includes number of daily feeding events, the duration of these feeding events and the rate of intake during feeding events. If intake data could be integrated with the GPS data, it would unlock even more information about variability in grazing to be matched with pasture disappearance and soil mineral maps.
When combined to the mob level, pasture use across the landscape can be mapped. This can be combined with satellite NDVI estimates of pasture cover and soil mineral maps to understand the interactions between the soil, pasture and animal (Trotter et al., 2018). This information could be used in management decisions such as changes to grazing plans, retirement from grazing for alternative uses, differential fertilizer application, and reductions in nitrogen leaching potential.
While collars on animals provide a starting point for generating new knowledge about the animal and its use of its environment, the technology also provides an enabling opportunity through directing livestock into specific parts of the environment, or exclusion from other parts. The use of both a GPS and virtual fence, and many sensors and interpretive algorithms in a permanent collar worn by the grazing ruminant provides a range of potential benefits (Table 1).
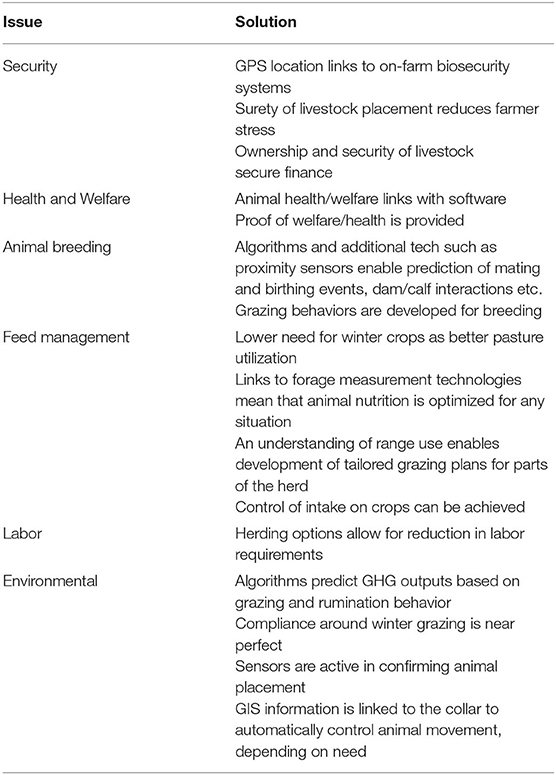
Table 1. A range of issues and solutions that digital sensors may provide when linked to the collar containing virtual herding technology, identified at a lead-user workshop in 2019, Hamilton, New Zealand.
Many farms face challenges with the installation and maintenance of fencing infrastructure (Stevens et al., 2019a). This may be due to the age of current fencing, to the erosion-prone nature of some soil types, to flooding or snow damage. The availability of virtual fencing can provide options to tailor new fencing configurations that optimize the use of the landscape. It is envisaged that early adopters farming beef in hill country will take up the virtual fencing for waterway protection in New Zealand due to government implementation of new regulations restricting waterway access for livestock. New Zealand hill country has many waterways which require livestock exclusion. The difficult terrain makes physical fencing extremely costly (Obadovic et al., 2020) and presents logistical challenges.
The much finer control of livestock grazing provides an opportunity to increase resource use efficiency, while reducing secondary losses from the system, such as nitrate through leaching and soil through sediment movement. Controlling where the animals graze and congregate can alter the distribution of nutrients in the environment. Varying this position over a series of grazing events will enable the redistribution of nutrients around the landscape, reducing the need for fertilizer inputs.
An example of innovative application of this technology is livestock security. In some parts of the world, being able to prove both ownership and location of livestock may enable greater capital investment in livestock farming, by having greater surety of current values of livestock.
Limitations and Challenges
While digital technologies show promise they all have several limitations. Calibration and validation are key to the success of digital technologies. This is because each data measure is generally only an approximation of a true value or distribution—even though there may be hundreds, thousands or millions of them. Each data point has varying levels of quality and uncertainty, for example, data collected by physical techniques vs. satellite sensing technologies. Quality and uncertainty are defined by the precision and accuracy of the data collected and expressed as the error that can be calculated from repeated sampling of any population (Steel and Torrie, 1980). Uncertainty in data, and therefore in all types of data science models, introduces the risk of poor decision outcomes because of biases, drift and lack of precision in individual sensor systems (Wolfert et al., 2017). Further, as the volume and variety of data increases, so do the uncertainties inherent within (Czarnecki and Podolak, 2013; Hariri et al., 2019)—big data is often subject to noise, incompleteness, bias and inconsistency (Hariri et al., 2019; Sharifi et al., 2020), and may often be disparate, dynamic, untrustworthy, and inter-related (Wang and Jones, 2017).
Using spectral data for soil and plant characterization has several known limitations. These include spectral saturation, cloud cover, changing satellite angle and spatial resolution. For example, the Normalized Difference Vegetation Index (NDVI) uses the difference between near infrared, which is reflected by vegetation, and red light, which is absorbed by vegetation (gisgeography.com, 2017). As NDVI approaches the upper limit of 1.0 for pastures (around 3,500 kg DM/ha), the spectra become saturated lowering the accuracy of prediction. Another known issue is the inability to perform NDVI measurements where clouds cover the area of interest. That decreases the temporal resolution of data and requires producing weekly or monthly image composites to create cloud-free imagery. Spatial resolution has significant influence on the accuracy of the measurements. While the 250 m/px imagery available daily from MODIS Terra and Aqua satellites is potentially useful tool on a national scale, it tends to mask differences between different pasture cover types and provide higher NDVI values than higher resolution satellite imagery for the same area, which eventually lead to overestimation of dry matter.
These issues mean that developing algorithms which are calibrated and validated for farms located across a range of environments remains very challenging. The work of Dymond et al. (2006) and the Pastures from Space project in Australia (Hill et al., 2004) have demonstrated potential, yet the scale of ground-truthing required remains a major obstacle. Future machine-learning and artificial intelligence building off smaller data sets holds significant promise. Lack of reliable field biomass measurements for farms located in different regions and topography is another problem that decreases the transferability of calibrations. Issues such as shading due to satellite and sun angle in hill country and the amount of dead matter in pasture may compromise the ability of a single calibration to provide repeatable prediction of herbage mass (Edirisinghe et al., 2012).
Technical challenges are apparent in creating algorithms that can be universally applied. Digital approaches are nearing a point where the precision of imagery and machine learning may help solve the calibration/validation issue. This would rely on the ability to utilize herbage mass data that was manually collected on-farm. Industry and government investment to collect and utilize farm data are increasing (e.g., FarmIQ, Farmax; Isaacs and White, 2016).
The on-going requirement for an evolving calibration of spectral analysis data poses a significant problem in situations where biomass estimates are not made. In the New Zealand context dairy farm managers often assess and report pasture biomass, albeit using a trained eye assessment (Eastwood and Dela Rue, 2017). In this circumstance machine learning or algorithm development could provide an on-going adjustment of image analysis to modify imagery interpretation, allowing the technology to be continually applied. When ground-truthing data is not available, this cannot happen. Therefore, farming systems that already measure features such as pasture quantity and quality will be much better placed to develop techniques to capture the value of these digital technologies.
One ground-based technology has been developed to address some of these issues. Farmote Systems® uses an infra-red-light source positioned within each paddock operating at night. It automatically returns data via a radio network and delivers a decision-ready report to graziers. It also uses a cross-reference to satellite imagery as available (Milsom et al., 2019). This type of approach mitigates several of the concerns of spectral analysis. For example, taking a reading at night, at near ground-level removes interference from other radiation sources. Cross-referencing provides the opportunity to modify calibrations to improve accuracy. Automated recording and reporting remove significant labor requirements to gather data. This type of technology integration provides a good example of capturing the value of digital approaches.
Technologies that reside on animals have unique issues that may limit use. Animal welfare concerns are potentially significant as harnesses and fitting may hinder movement, disrupt behavior and injure the animal. These issues are being addressed through thoughtful design and constant improvement. The general public have raised concerns about technologies such as virtual fencing. Many of the studies of virtual fencing have been directed to address these concerns (e.g., Campbell et al., 2019), though efforts to provide that information to the general public will also need to be made to aid with its use (Stampa et al., 2020).
Advances in battery engineering, computing hardware, and satellite networks have improved since the initial release in 1991 bringing animal tracking into the realm of big data research (Kays et al., 2015). However, many commercially available units still retail for considerable costs and have ongoing software licensing and data management fees. The rise of open source hardware has allowed the production of self-managed low-cost GPS units to be developed (Cain and Cross, 2018) allowing access to this technology to the masses.
Calibration requires significant resources and is often specific to one configuration of the technology. Data from animal-based sensors available through publication has been restricted to small scale studies, often with farm management objectives in mind, or the development of prediction equations for specific traits. Often calibrations are proprietary to the technology developer.
Handling and interpreting huge amounts of available data is key to extracting value in complex decision-making. Many decisions may only require simple data to make yes/no decisions. For example, knowing a critical temperature boundary has been exceeded may provide a decision-making point to declare food unsafe. Complex decisions, such as those required to manage grazing systems, use much more data, and integrate data from many sources (Gray et al., 2005).
Sources of available data will include climate, soil, plant, animal, product, and consumer (Table 2). Insights will be enhanced by understanding the associations or relationships between these variables. This data needs to be collated and integrated with technologies such as machine and deep learning, augmented reality and multi-functional decision-making. This will provide an understanding of current state of the world and generate opportunities to predict or visualize what might happen and to (re)direct for the best outcome(s).
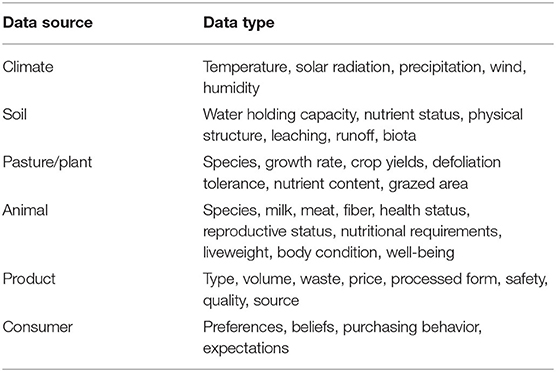
Table 2. An example of the sources and types of data that will be available to collate and integrate to inform current state, predict future states, and direct decision-making.
Approximation and Uncertainty
Biological systems are inherently variable. For example, pasture growth in a single paddock will vary greatly depending on factors such as microclimate, soil fertility and previous urine and fecal deposition. This is then overlaid by the dietary preferences of the grazing animal and their avoidance of undesirable parts of the pasture. Thus, even at the finest level, the scale at which we can collect data guarantees that the biological system introduces another layer of uncertainty, over and above that of the measurement techniques, before an outcome is realized.
The risk of poor decision outcomes is particularly true in analytics that combine non-traditional information sources such as rapidly arriving data from sensors, process models, qualitative information and user behavior (Wynne, 1992). Using multiple disparate data sources means compounding data uncertainty originating from the data collection, data curation and combination from multiple sources (Czarnecki and Podolak, 2013; Hariri et al., 2019). Communicating uncertainty in data can introduce further complexities, and uncertainties are sometimes ignored, or even explicitly denied (van der Bles et al., 2019). Uncertainty in the data collection, analysis and knowledge extension processes can lead to a lack of confidence in the resulting model outputs and decision made thereof. Communication of the uncertainties associated with findings from data modeling is vital, since, unless uncertainty is communicated effectively, decision-makers may put too much or too little faith in it (Fischhoff and Davis, 2014; van der Bles et al., 2019) leading to poor or unexpected outcomes (Meenken et al., 2020).
Matching the potential predictions and decisions of digital technologies with the expectations and experiences of the end-user will remain a challenge. This has effect in two ways. The first is the ability of the digital technologies and the data analysis to represent the biophysical situation accurately. For example, tools to measure pasture biomass need be calibrated to appropriate pastures (Eastwood and Dela Rue, 2017). If calibrations of the data, or its use in defining future states such as pasture growth, cannot match reality then the end user will dismiss the technology. Many technologies have suffered this in the past. The second effect is when the outcomes from digital data analysis provide new insights into the impacts of decision-making, or potential new ways of proceeding. In this instance the technology may be right, but not representative of the experience of the end user. In this situation, valuable progress may be dismissed.
Successful complex disparate data analysis will provide a) uncertainty evaluations that account for the combination of all types of data by applying the principles of metrological traceability and b) support decision making with excellent tools to communicate uncertainty. Quantitative and qualitative metrics of uncertainty both improve confidence in the validity of the information and provide evidence for the underlying quality of the model and data (Mastrandrea et al., 2010).
Discussion
First steps in capturing the power of digital technologies will be small, as the technologies are not yet fully formed. Gains will be made in improving the fit of resources to productive potential of both the land and the animals. For example, grazing control may improve the utilization of the grassland resource, increasing both quality and quality of forage available. This would result in an initial increase in production via pasture utilization, and a secondary increase in stocking rates and/or an increase in animal performance. Interestingly, these outcomes may augment or deplete the environment depending on the pathway chosen by the grazier. If a grazier chooses stocking rate to capture the benefits, then more nutrient leakage (from urine and fecal deposition and treading damage) may result.
Scenario testing using fine-scale GIS data of topography and aspect, combined annual and perennial thistle density data and soil nutrient and water-holding data has been used to predict the relative economic benefits of thistle control on a complex hill property (Stevens et al., 2019b). This provides an example of how digital technologies can be used at fine scale to offer new insights into farm management options. This work utilized 64 potential pasture/thistle interactions in the range of micro-geoclimatic zones present on a single farm. While this may technically provide on-farm opportunities for increasing efficiency/profitability, the current fencing would not allow optimal pasture utilization. Adding virtual fencing would aid in capturing benefits that can now be predicted using digital technologies.
As the technologies are further refined then land managers may capture benefits by fitting practices to landscape and avoiding potential conflicts of changing productivity. Ecosystems services such as biodiversity, nutrient loss minimization and water harvesting may be improved through selective use of various landscapes. This selective use may involve both time and space, to for example, vary grazing near waterways, or restrict grazing access to specific times of the year when damage is minimized, and benefits enhanced. For example, using ruminants to graze pastures in spring to prevent fuel build-up in fire-prone areas, enabled by the use of virtual fencing.
Potentially the addition of new enterprises, or changes from current to future enterprises may occur. Benefits from increased utilization and productivity of pastures may allow land of higher quality to be released for other enterprises such as arable or horticultural use. The emergence of such enterprises will require a redistribution of labor and resources. It potentially may also result in shifts of power, depending on the relative profitability of each enterprise, influencing other outcomes such as social and environmental impacts.
For example, when adding legumes into a hill farm, Dodd et al. (2020) determined that significant increases in both pasture and animal production were achievable, while reducing nitrogen fertilizer inputs. Legume introduction was targeted at specific micro-geoclimatic zones on the farm, using GIS mapping. However, realizing those gains in practice will again require much more precision in livestock control and grazing, hence requiring these technologies to be enabled by virtual fencing.
However, using the data is not all that is required. Key to the success of applying any digital information is the ability to uniquely identify the data and assign it to a space and time. Therefore, the shift to digitization must be accompanied by not only data collection, but storage and categorization in a standardized way. Many farmers collect animal liveweights for example, but current collection and storage processes are fraught with problems which include data being confined to files which have no common or systematic naming protocols, have few unique identifiers and poor time stamp control. This then means that the data is difficult to decipher and utilize beyond the farm boundary.
Digital technologies come at a cost, both financially and in time. This can be a significant barrier to technology uptake. Thus, technologies will be adopted at different rates depending on the urgency and relative cost compared to other solutions. For example, Yang et al. (2021) found that the uptake of digital technologies varied both from region to region and between farms of different demographics and herd size, depending on economies of scale, access to capital, and current infrastructure constraints. In another example, the uptake of virtual herding technology may be driven by legislative change to waterway fencing regulation (Obadovic et al., 2020) with the cost of the technology be lower than the cost of conventional fencing.
Conclusions
Key to applying digital technologies to enhance the outcomes from grasslands and rangelands is understanding the roles and interactions between principle factors that influence the balance between productivity and sustainability. Using remote digital approaches to characterize the resource base with both spatial and temporal precision will unlock a range of new options to manage biophysical resources.
Characterizing animals will provide new opportunities to match grazing behaviors to plant and soil resources, improving sustainability. While, for example, variations in grazing style (animals with different bite size for example), may not have a direct effect on productivity, the rate of prehension and subsequent rumination may have a significant effect on the extent of digestion and potential greenhouse gas emissions.
Previous research on grazing behavior tested theories through varying pasture conditions, but key to applying digital technologies is to know what variation is available between animals. It will be the application of variability in the animal to harness the variability in the soil and pasture resource that will provide the step-change in resource use efficiency in grassland-based ruminant production systems. Understanding, interpreting, and discovering new insights into the data will underpin our ability to capture value as data adequately reflects practice.
Step-change will only be realized if livestock can be directed to appropriate niches within the landscape. Virtual herding technology provides that option. Finally, new business models that make use of the shared data will change our approaches to managing grasslands and its future production. The balance between efficiency, intensity and environmental impacts will be better understood and managed.
Integration of digital technologies may provide the means to maintain ruminant production from grasslands in a sustainable operating space. We must discern the difference between the fascinating and the important in the quest to develop digital solutions. However, we must also be able to recognize when the fascinating becomes the important.
Author Contributions
DS coordinated the papers and wrote the Introduction, Characterizing the Landscape Resource, Directing Animals in the Environment, and Combining Technologies to Optimize Grassland Management. BT, PJ, and BW wrote Characterizing Grazing Animals. EM and JB wrote Approximation and Uncertainty. All authors contributed to Limitations and Challenges, Discussion, and Conclusions, provided editorial comment on the whole document, and approved the final version.
Funding
This work was completed as part of the New Zealand Bioeconomy in the Digital Age Research programme.
Conflict of Interest
The authors are employed by the company AgResearch Ltd. AgResearch Ltd is the registered business name of the New Zealand Pastoral Agricultural Research Institute, a state-owned research institute, funded by government grants. This research was done under one of those grants.
The authors declare that the research was conducted in the absence of any commercial or financial relationships that could be construed as a potential conflict of interest.
The handling editor declared a past co-authorship with one of the authors, PG.
Acknowledgments
The authors thank technology partners Agersens Ltd, Pamu Farming Ltd, and Gallagher Ltd for access to data and discussion, as well as funding from the AgResearch Strategic Science Investment fund, New Zealand Bio-economy in the Digital Age program.
References
Archer, S., Davies, K. W., Fulbright, T. E., McDaniel, K. C., Wilcox, B. P., and Predick, K. I. (2001). “Brush management as a rangeland conservation strategy: a critical evaluation,” in Conservation Benefits of Rangeland Practices: Assessment, Recommendations, and Knowledge Gaps, ed DD Briske (Washington, DC: USDA Natural Resources Conservation Service), 105–170.
Baars, J. A. (1976). Seasonal distribution of pasture production in New Zealand VIII Dargaville. N. Zeal. J. Exp. Agric. 4, 151–156. doi: 10.1080/03015521.1976.10425861
Bailey, D. W. (2005). Identification and creation of optimum habitat conditions for livestock. Rangeland Ecol. Manage. 58, 109–118. doi: 10.2111/03-147.1
Bailey, D. W., Keil, M. R., and Rittenhouse, L. R. (2004). Research observation: daily movement patterns of hill climbing and bottom dwelling cows. J. Range Manage. 57, 20–28. doi: 10.2111/1551-5028(2004)057[0020:RODMPO]2.0.CO;2
Bailey, D. W., Lunt, S., Lipka, A., Thomas, M. G., Medrano, J. F., Cánovas, A., et al. (2015). Genetic influences on cattle grazing distribution: association of genetic markers with terrain use in cattle. Rangeland Ecol. Manage. 68, 142–149. doi: 10.1016/j.rama.2015.02.001
Bailey, D. W., Trotter, M. G., Knight, C. W., and Thomas, M. G. (2018). Use of GPS tracking collars and accelerometers for rangeland livestock production research. Transl. Anim. Sci. 2, 81–88. doi: 10.1093/tas/txx006
Battaglini, L., Bovolenta, S., Gusmeroli, F., Salvador, S., and Sturaro, E. (2014). Environmental sustainability of alpine livestock farms. Ital. J. Anim. Sci. 13:3155. doi: 10.4081/ijas.2014.3155
Betteridge, K., Hoogendoorn, C., Costall, D., Carter, M., and Griffiths, W. (2010). Sensors for detecting and logging spatial distribution of urine from grazing cows and ewes. Comput. Electr. Agric. 73, 66–73. doi: 10.1016/j.compag.2010.04.005
Bryant, R. H., Miller, M. E., Greenwood, S. L., and Edwards, G. R. (2017). Milk yield and nitrogen excretion of dairy cows grazing binary and multispecies pastures. Grass Forage Sci. 72, 806–817. doi: 10.1111/gfs.12274
Buckwell, A., and Nadeu, E. (2018). What is the Safe Operating Space for EU Livestock? RISE Foundation, Brussels. Available online at: http://www.risefoundation.eu/images/files/2018/2018_RISE_LIVESTOCK_FULL.pdf (accessed September 19, 2019).
Cain, P. W., and Cross, M. D. (2018). An open-source hardware GPS data logger for wildlife radio-telemetry studies: a case study using Eastern box turtles. HardwareX 3, 82–90. doi: 10.1016/j.ohx.2018.02.002
Campbell, B. M., Beare, D. J., Bennett, E. M., Hall-Spencer, J. M., Ingram, J. S. I., Jaramillo, F., et al. (2017). Agriculture production as a major driver of the Earth system exceeding planetary boundaries. Ecol. Soc. 22:8. doi: 10.5751/ES-09595-220408
Campbell, D. L. M., Lea, J. M., Haynes, S. J., Farrer, W. J., Leigh-Lancaster, C. J., and Lee, C. (2018). Virtual fencing of cattle using an automated collar in a feed attractant trial. Appl. Anim. Behav. Sci. 200, 71–77. doi: 10.1016/j.applanim.2017.12.002
Campbell, D. L. M., Lea, J. M., Keshavarvi, H., and Lee, C. (2019). Virtual fencing is comparable to electric tape fencing for cattle behavior and welfare. Front. Vet. Sci. 6:445. doi: 10.3389/fvets.2019.00445
Castillo, A. R., Kebreab, E., Beever, D. E., and France, J. (2000). A review of efficiency of nitrogen utilisation in lactating dairy cows and its relationship with environmental pollution. J. Anim. Feed Sci. 9, 1–32. doi: 10.22358/jafs/68025/2000
Czarnecki, W. M., and Podolak, I. T. (2013). “Machine learning with known input data uncertainty measure,” in IFIP International Conference on Computer Information Systems and Industrial Management (Springer).
Dodd, M. B., Tozer, K. N., Vogeler, I., Greenfield, R., Stevens, D. R., Rhodes, T., et al. (2020). Quantifying the value proposition for white clover persistence on a New Zealand summer-dry hill-country farm. J. N. Zeal. Grasslands 82, 199–209. doi: 10.33584/jnzg.2020.82.2973
Dymond, J. R., Shepherd, J. D., Clark, H., and Litherland, A. (2006). Use of VEGETATION satellite imagery to map pasture quality for input to a methane budget of New Zealand. Int. J. Remote Sens. 27, 1261–1268. doi: 10.1080/01431160500212070
Eastwood, C. R., and Dela Rue, B. T. (2017). Identification of operational performance attributes for pasture measuring devices. J. N. Zeal. Grasslands 79, 217–222. doi: 10.33584/jnzg.2017.79.532
Edirisinghe, A., Clark, D., and Waugh, D. (2012). Spatio-temporal modelling of biomass of intensively grazed perennial dairy pastures using multispectral remote sensing. Int. J. Appl. Earth Observ. Geoinform. 16, 5–16. doi: 10.1016/j.jag.2011.11.006
Fischhoff, B., and Davis, A. L. (2014). Communicating scientific uncertainty. Proc. Natl. Acad. Sci. U.S.A. 111(Suppl. 4), 13664–71. doi: 10.1073/pnas.1317504111
gisgeography.com (2017). Available online at: https://gisgeography.com/ndvi-normalized-difference-vegetation-index/ (accessed February 15, 2020).
Gray, D. I., Reid, J. I., Kemp, P. D., Brookes, I. M., Horne, D., Kenyon, P. R., et al. (2005). Summer management of sheep on summer-wet hill country: insights into decisions made by an ‘expert' farmer. Proc. Zeal. Grassland Assoc. 67, 87–95. doi: 10.33584/jnzg.2005.67.2581
Hariri, R. H., Fredericks, E. M., and Bowers, K. M. (2019). Uncertainty in big data analytics: survey, opportunities, and challenges. J. Big Data 6:44. doi: 10.1186/s40537-019-0206-3
Hill, M. J., Donald, G. E., Hyder, M. W., and Smith, R. C. G. (2004). Estimation of pasture growth rate in the south west of Western Australia from AVHRR NDVI and climate data. Rem. Sens. Environ. 93, 528–545. doi: 10.1016/j.rse.2004.08.006
Isaacs, C., and White, T. (2016). Driving farm performance using information systems. Grassland Res. Pract. Ser. 16, 109–112. doi: 10.33584/rps.16.2016
Kays, R., Crofoot, M. C., Jetz, W., and Wikelski, M. (2015). Terrestrial animal tracking as an eye on life and planet. Ecology 1:aaa2478. doi: 10.1126/science.aaa2478
Lee, C., Fisher, A. D., Reed, M. T., and Henshall, J. M. (2008). The effect of low energy electric shock on cortisol, beta -endorphin, heart rate and behaviour of cattle. Appl. Anim. Behav. Sci. 113, 32–42. doi: 10.1016/j.applanim.2007.10.002
Lee, C., Henshall, J. M., Wark, T. J., Crossman, C. C., Reed, M. T., Brewer, H. G., et al. (2009). Associative learning by cattle to enable effective and ethical virtual fences. Appl. Anim. Behav. Sci. 119, 15–22. doi: 10.1016/j.applanim.2009.03.010
Mastrandrea, M. D., Field, C. B., Stocker, T. F., Edenhofer, O., Ebi, K. L., Frame, D. J., et al. (2010). Guidance Note for Lead Authors of the IPCC Fifth Assessment Report on Consistent Treatment of Uncertainties. Intergovernmental Panel on Climate Change (IPCC). Available online at: http://www.ipcc.ch
McCall, D. G., and Sheath, G. W. (1993). “Development of intensive grassland systems: from science to practice,” in Proceedings of the XVII International Grassland Congress, (Palmerston) 1257–1265.
McCoard, S. A., Stevens, D. R., and Whitney, T. R. (2020). “Sustainable sheep and goat production through strategic nutritional management and advanced technologies,” in Animal Agriculture: Sustainability, Challenges and Innovations, eds F. W. Bazer, G. C. Lamb, and G. Wu (London: Elsevier Academic Press), 230–248.
Meenken, E. D., Wheeler, D., Espig, M., Brown, H., Teixeira, E., and Triggs, E. (2020). “A framework for uncertainty evaluation and estimation in deterministic agricultural models,” in Nutrient Management in Farmed Landscapes. Occasional Report No. 33, eds C. L. Christensen, D. J. Horne, and R. Singh (Palmerston North: Farmed Landscape Research Centre, Massey University), 11. Available online at: http://flrc.massey.ac.nz/publications.html
Milsom, A., Bell, O., Bailey, K., Brown, S. C., Barton, R. A., Moreno Garcia, C. A., et al. (2019). Assessing the ability of a stationary pasture height sensing device to estimate pasture growth and biomass. J. N. Zeal. Grasslands 81, 61–68. doi: 10.33584/jnzg.2019.81.384
Obadovic, I., Ward, J., Box, L., Tozer, K., Brown, M., and Stevens, D. (2020). “Understanding the introduction of new technologies – a case study of virtual fencing (I) developing a multiagent framework to explore potential futures with virtual fencing,” in Proceedings of the International Farming Systems Association Symposium (Evora).
Piggot, G. H., Baars, J. A., Cumberland, G. L. B., and Honore, E. N. (1978). Seasonal distribution of pasture production in New Zealand XIII South Kaipara, Northland. N. Zeal. J. Exp. Agric. 6, 43–46. doi: 10.1080/03015521.1978.10426012
Radcliffe, J. E. (1974). Seasonal distribution of pasture production in New Zealand II. Southland Plains. N. Zeal. J. Exp. Agric. 2, 341–348. doi: 10.1080/03015521.1974.10427693
Radcliffe, J. E. (1975). Seasonal distribution of pasture production in New Zealand IV. Westport and Motueka. N. Zeal. J. Exp. Agric. 3, 239–246. doi: 10.1080/03015521.1975.10425810
Ravera, B., Bryant, R., Cameron, K., Di, H., Edwards, G., and Smith, N. (2015). Use of a urine meter to detect variation in urination behaviour of dairy cows on winter crops. Proc. N. Zeal. Soc. Anim. Prod. 75, 84–88.
Roberts, A. H. C., and Thomson, N. A. (1984). Seasonal distribution of pasture production in New Zealand XVIII South Taranaki. N. Zeal. J. Exp. Agric. 12, 83–92. doi: 10.1080/03015521.1984.10421416
Rouse, J., Haas, R., Schell, J., Deering, D., and Harlan, J. (1974). Monitoring the Vernal Advancement and Retrogradation (Green Wave Effect) of Natural Vegetation. NASA/GSFC, Type II, Final Report, Vol. 371. Greenbelt, MD.
Sharifi, M., Meenken, E., Hall, B. D., Espig, M., Finlay-Smits, S., and Wheeler, D. M. (2020). “Importance of measurement and data uncertainty in a digitally enabled agriculture system,” in Nutrient Management in Farmed Landscapes, Occasional Report No. 33, eds C. L. Christensen, D. J. Horne, and R. Singh (Palmerston North: Farmed Landscapes Research Centre, Massey University), 8. Available online at: http://flrc.massey.ac.nz/publications.html
Shepherd, M., Shorten, P., Costall, D., and Macdonald, K. A. (2017b). Evaluation of urine excretion from dairy cows under two farm systems using urine sensors. Agric. Ecosyst. Environ. 236, 285–294. doi: 10.1016/j.agee.2016.12.017
Shepherd, M. A., Welten, B. G., Costall, D., Cosgrove, G. P., Pirie, M., and Betteridge, K. (2017a). Evaluation of refractive index for measuring urinary nitrogen concentration in a sensor worn by grazing female cattle. N. Zeal. J. Agric. Res. 60, 23–31. doi: 10.1080/00288233.2016.1237979
Spiegal, S., Estell, R. E., Cibils, A. F., James, D. K., Peinetti, H. R., Browning, D. M., et al. (2019). Seasonal divergence of landscape use by heritage and conventional cattle on desert rangeland. Rangeland Ecol. Manage. 72, 590–601. doi: 10.1016/j.rama.2019.02.008
Stampa, E., Zander, K., and Hamm, U. (2020). Insights into German consumers' perceptions of virtual fencing in grassland-based beef and dairy systems: recommendations for communication. Animals 10:2267. doi: 10.3390/ani10122267
Steel, R. G. D., and Torrie, J. H. (1980). Principles and Procedures of Statistics. A Biometrical Approach, 2nd Edn. New York, NY: McGraw-Hill.
Steer, Z. (2012). Merino sheep habitat use in Canterbury high country Tall Tussock grasslands [Thesis in Fufilment of the Master of Science in Environmental Sciences]. Canterbury University, Christchurch, New Zealand.
Steffen, W., Richardson, K., Rockstrom, J., Cornell, S. E., Fetzer, I., Bennett, E. M., et al. (2015). Panetary boundaries: guiding human development on a changing planet. Science 347:1259855. doi: 10.1126/science.1259855
Stevens, D., Cottrell, B., Kingi, T., Maag, W., Whaata, T., Hemana, C., et al. (2019a). “Using benchmarking and key performance indicators to communicate between management and governance in large farming enterprises,” in Proceedings of the 22nd International Farm Management Congress (Launceston, TAS). Available online at: http://ifmaonline.org/contents/npr-using-benchmarking-and-key-performance-indicators-to-communicate-between-management-and-governance-in-large-farming-enterprises/
Stevens, D. R. (2009). Choice of forage crops for animal production in temperate perennial pasture-based grazing systems. CAB Rev. Perspect. Agric. Vet. Sci. Nutr. Nat. Resour. 4:17. doi: 10.1079/PAVSNNR20094017
Stevens, D. R., Tozer, K. N., Rhodes, T., Zydenbos, S. M., Dynes, R. A., Manning, M. J., et al. (2019b). Farm systems analysis of two thistles of differing seasonal pasture growth impacts in North Island hill country. J. N. Zeal. Grasslands 81, 149–158. doi: 10.33584/jnzg.2019.81.411
Trotter, M., Cosby, A., Manning, J., Thomson, M., Trotter, T., Graz, P., et al. (2018). Demonstrating the Value of Animal Location and Behaviour Data in the Red Meat Value Chain. Report Prepared for Meat and Livestock Australia Limited.
Ursin, H., and Eriksen, H. R. (2004). The cognitive activation theory of stress. Psychoneuroendocrinology 29, 567–592. doi: 10.1016/S0306-4530(03)00091-X
van der Bles, A. M., van der Linden, S., Freeman, A. L. J., Mitchell, J., Galvao, A. B., Zaval, L., et al. (2019). Communicating uncertainty about facts, numbers and science. R. Soc. Open Sci. 6:181870. doi: 10.1098/rsos.181870
Wang, L., and Jones, R. (2017). Big data analytics for disparate data. Am. J. Intell. Syst. 7, 39–46. doi: 10.5923/j.ajis.20170702.01
Wesley, R. L., Cibils, A. F., Mulliniks, J. T., Pollak, E. R., Petersen, M. K., and Fredrickson, E. L. (2012). An assessment of behavioural syndromes in rangeland-raised beef cattle. Appl. Anim. Behav. Sci. 139, 183–194. doi: 10.1016/j.applanim.2012.04.005
Wolfert, S., Gea, L., Verdouw, C., and Bogaardt, M. J. (2017). Big data in smart farming – a review. Agric. Syst. 153, 69–80. doi: 10.1016/j.agsy.2017.01.023
Wynne, B. (1992). Uncertainty and environmental learning: Reconceiving science and policy in the preventive paradigm. Glob. Environ. Change 2, 111–127. doi: 10.1016/0959-3780(92)90017-2
Yang, W., Edwards, J. P., Eastwood, C. R., Dela Rue, B. T., and Renwick, A. (2021). Analysis of adoption trends of in-parlor technologies over a 10-year period for labor-saving and data capture on pasture pasture-based dairy farms. J. Dairy Sci. 104, 431–442. doi: 10.3168/jds.2020-18726
Keywords: uncertainty, spectral analysis, grazing control, digital, animal phenotyping
Citation: Stevens DR, Thompson BR, Johnson P, Welten B, Meenken E and Bryant J (2021) Integrating Digital Technologies to Aid Grassland Productivity and Sustainability. Front. Sustain. Food Syst. 5:602350. doi: 10.3389/fsufs.2021.602350
Received: 03 September 2020; Accepted: 11 March 2021;
Published: 06 April 2021.
Edited by:
Pablo Gregorini, Lincoln University, New ZealandReviewed by:
Derek Wayne Bailey, New Mexico State University, United StatesKathy Soder, Agricultural Research Service, United States Department of Agriculture, United States
Copyright © 2021 Stevens, Thompson, Johnson, Welten, Meenken and Bryant. This is an open-access article distributed under the terms of the Creative Commons Attribution License (CC BY). The use, distribution or reproduction in other forums is permitted, provided the original author(s) and the copyright owner(s) are credited and that the original publication in this journal is cited, in accordance with accepted academic practice. No use, distribution or reproduction is permitted which does not comply with these terms.
*Correspondence: David R. Stevens, ZGF2aWQuc3RldmVuc0BhZ3Jlc2VhcmNoLmNvLm56